商务与经济统计作业(仅供参考)
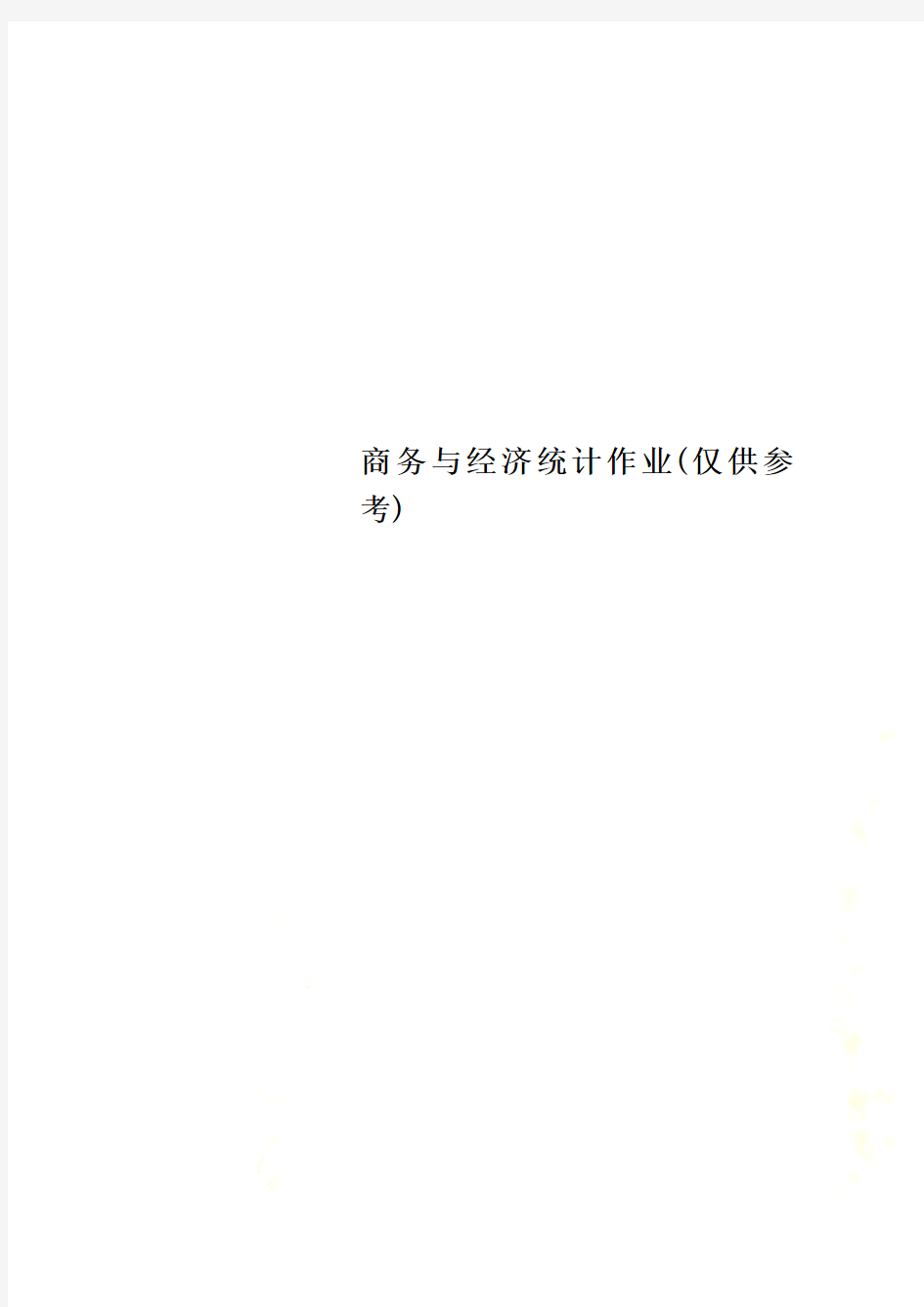
- 1、下载文档前请自行甄别文档内容的完整性,平台不提供额外的编辑、内容补充、找答案等附加服务。
- 2、"仅部分预览"的文档,不可在线预览部分如存在完整性等问题,可反馈申请退款(可完整预览的文档不适用该条件!)。
- 3、如文档侵犯您的权益,请联系客服反馈,我们会尽快为您处理(人工客服工作时间:9:00-18:30)。
商务与经济统计作业(仅供参考)
第一章数据与统计资料
P 18
25. 表1-8是一个由25只影子股票组成的数据集,(表略)
a 数据集中有几个变量?答:数据集中有5个变量。
b哪些变量是数量变量?哪些变量是品质变量?
答:市场价值、市盈率和毛利率属于数量变量;交易所和股票代码是品质变量。
c对交易所变量,计算AMEX、NYSE 和OTC 频数或百分数频数。绘制类似于图1-5的交易所变量的条形图。
交易所频数交易所百分数(%)
AMEX 5 AMEX 20
NYSE 3 NYSE 12
OTC 17 OTC 68
总计25
e 平均市盈率是多少?答:利用EXCEL的求平均值功能得出平均市盈率是20.2
第二章表格法和图形法
P 23
5按字母顺序,美国最常见的6个姓氏为:布朗、戴维斯、约翰逊、琼斯、史密斯和威廉姆斯。假
设根据一个由50个人组成的样本,得到如下的姓氏数据(图略)
a相对频数分布和百分数频数分布。
name frequency Percentage( %)
Brown 7 14 Davis 6 12 Johnson 10 20 Jones 7 14 Smith 12 24 William
s 8 16 total 50
b构建条形图
c 构建饼形图
d根据这些数据,最常见的3个姓氏是哪些?
答:最常见的3个姓氏分别是史密斯、约翰逊和威廉姆斯。
P50
51 表2-17 给出了50家《财富》500强公司的所有者权益、市场价值和利润数据。(图略)a.构建所有者权益和利润变量的交叉分组表。对利润数据以0-200,200-400,…,1000-1200分组,对所有者权益数据以0-1200,1200-2400,…,4800-6000分组。
计数项:Company Profit
Stockholders'
Equity 0-200 200-400 400-600 600-800 800-1000 1000-1200 总计
0-1200 10 1 11 1200-2400 4 10 2 16 2400-3600 4 3 3 1 1 12
3600-4800 1 2 2 5 4800-6000 2 3 1 6 总计18 17 6 2 5 2 50
b. 计算(a)中交叉分组表的行百分数。
计数项:Company Profit
Stockholders'Equ
ity 0-200 200-400 400-600 600-800 800-1000 1000-1200
0-1200 90.90% 9.10%
1200-2400 25%62.50% 12.50%
2400-3600 33.30% 25% 25% 8.30% 8.30%
3600-4800 20% 40% 40% 4800-6000 33.30% 50% 16.70%
P 51
53. 参考表2-17中的数据集
a. 绘出显示利润和所有者权益变量之间关系的
散点图。
b. 评价这两个变量之间的关系。
答:二者呈正相关的关系,即所有者权益增加,利润也增加。但因为所有点并不在一条直线上,所以这种关系不是完全的。
案例2-1 Pelican 商店
1. 主要变量的百分数频数分布
顾客类型频数
(%) 项目
频数
(%) 支付方法频数(%)
Promotion
al 70 1 29 Discover 4
Regular 30 2 27 Proprietar
y Card 70
3 10 MasterCar
d 14
4 10 Visa 10
5 9 American
Express 2
6 7
7 1
8 1
9 3
10 1
13 1
17 1
性别频数(%) 婚姻频数(%)
Male 7 Married 84
Female 93 Single 16
净销售额
组宽最大值频数
0-50 50 39
51-100 100 35 101-150 150 16 151-200 200 6 201-250 250 1 251-300 300 3
年龄
组宽最大值频数
0-20 20 2
21-40 40 42
41-60 60 47
61-80 80 9
2. 条形图或饼形图,以显示因促销活动而使顾客购买的百分数。
3. 顾客类型(常规性或奖励性)与销售额的交叉分组表,并评价其相似性与差异性。
答:根据该交叉分组表,说明Pelican商店所推出的促销活动取得了显著成效。使用折扣赠券购买的奖励性顾客占全体顾客总数的70%,分布于各个销售额区域,尤其在销售额100内的范围里做出了突出贡献,尽管未使用折扣赠券的常规性顾客也主要集中在该销售额区域,但比重明显低于奖励性顾客,且在200以上的销售额区域则无常规性顾客,奖励性顾客的消费金额也扩大到300。
4. 考察净销售额与顾客年龄关系的散点图
根据上图,净销售额与顾客年龄之间没有明显的相关关系。
总之,Pelican商店所推出的促销活动取得了成效,净销售额明显增加,客户群有所扩大。
第二次作业
第三章描述统计学II :数值方法
P 87
63 人们每天去上班时,可以乘坐公交车或开私家车。下面是这两种方式所花费时间的样本数据,时间以分钟计。
a. 计算每种方式所花费时间的样本平均数。
乘坐公交车所花费时间的样本平均数:32
乘坐私家车所花费时间的样本平均数:32
b.计算每种方法的样本标准差
乘坐公交车的样本标准差:4.643