Demystifying Sludge and Varnish(1000815)
八年级科技前沿英语阅读理解25题
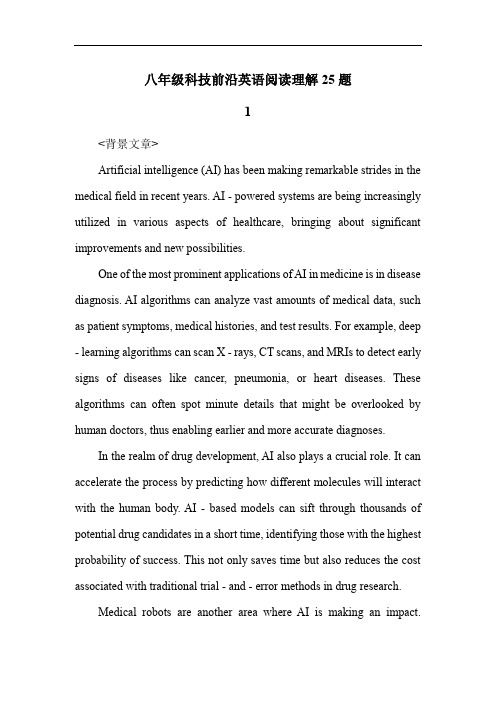
八年级科技前沿英语阅读理解25题1<背景文章>Artificial intelligence (AI) has been making remarkable strides in the medical field in recent years. AI - powered systems are being increasingly utilized in various aspects of healthcare, bringing about significant improvements and new possibilities.One of the most prominent applications of AI in medicine is in disease diagnosis. AI algorithms can analyze vast amounts of medical data, such as patient symptoms, medical histories, and test results. For example, deep - learning algorithms can scan X - rays, CT scans, and MRIs to detect early signs of diseases like cancer, pneumonia, or heart diseases. These algorithms can often spot minute details that might be overlooked by human doctors, thus enabling earlier and more accurate diagnoses.In the realm of drug development, AI also plays a crucial role. It can accelerate the process by predicting how different molecules will interact with the human body. AI - based models can sift through thousands of potential drug candidates in a short time, identifying those with the highest probability of success. This not only saves time but also reduces the cost associated with traditional trial - and - error methods in drug research.Medical robots are another area where AI is making an impact.Surgical robots, for instance, can be guided by AI systems to perform complex surgeries with greater precision. These robots can filter out the natural tremors of a surgeon's hand, allowing for more delicate and accurate incisions. Additionally, there are robots designed to assist in patient care, such as those that can help patients with limited mobility to move around or perform simple tasks.However, the application of AI in medicine also faces some challenges. Issues like data privacy, algorithmic bias, and the need for regulatory approval are important considerations. But overall, the potential of AI to transform the medical field is vast and holds great promise for the future of healthcare.1. What is one of the main applications of AI in the medical field according to the article?A. Designing hospital buildings.B. Disease diagnosis.C. Training medical students.D. Managing hospital finances.答案:B。
德罗斯特
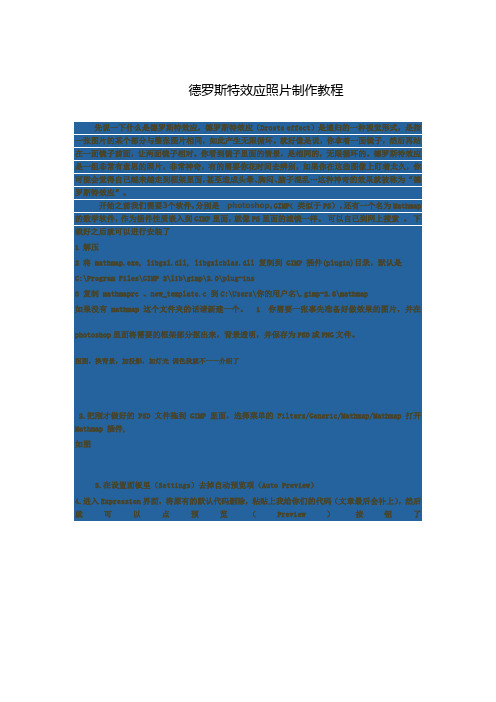
德罗斯特效应照片制作教程先说一下什么是德罗斯特效应,德罗斯特效应(Droste effect)是递归的一种视觉形式,是指一张图片的某个部分与整张图片相同,如此产生无限循环。
就好像是说,你拿着一面镜子,然后再站在一面镜子前面,让两面镜子相对。
你看到镜子里面的情景,是相同的,无限循环的。
德罗斯特效应是一组非常有意思的照片,非常神奇,有的需要你花时间去辨别,如果你在这些图像上盯着太久,你可能会觉得自己越来越走到框架里面,甚至造成头晕、胸闷、脑子混乱…这种神奇的效果就被称为“德罗斯特效应”。
开始之前我们需要3个软件,分别是photoshop,GIMP(类似于PS),还有一个名为Mathmap 的数学软件,作为插件性质嵌入到GIMP里面,就像PS里面的滤镜一样。
可以自己到网上搜索。
下载好之后就可以进行安装了1 解压2 将 mathmap.exe, libgsl.dll, libgslcblas.dll 复制到 GIMP 插件(plugin)目录,默认是C:\Program Files\GIMP 2\lib\gimp\2.0\plug-ins3 复制 mathmaprc 、new_template.c 到C:\Users\你的用户名\.gimp-2.8\mathmap如果没有mathmap这个文件夹的话请新建一个。
1 你需要一张事先准备好做效果的图片,并在photoshop里面将需要的框架部分抠出来,背景透明,并保存为PSD或PNG文件。
抠图,换背景,加投影,加灯光调色我就不一一介绍了2.把刚才做好的PSD文件拖到GIMP里面,选择菜单的 Filters/Generic/Mathmap/Mathmap 打开Mathmap 插件,如图3.在设置面板里(Settings)去掉自动预览项(Auto Preview)4.进入Expression界面,将原有的默认代码删除,粘贴上我给你们的代码(文章最后会补上),然后就可以点预览(Preview)按钮了5.切换到 User Variables 面板,勾选 Tile Based on Transparency 和 Transparency Points In 项,然后点左边的预览(Preview)按钮感谢代码for Mathmap的原作者Breic 和改进者Pisco Bandito附代码########################################## Droste Effect code for Mathmap #### Original Code by Breic (Ben) #### Adapted by Pisco Bandito (Josh) #### Version 4.0 #### This version for Windows and Linux ###########################################You may need to alter the values of the following 9 variables to suit your image. r1=.4; # r1 is the inner radius. r1 is greater than zero and less than r2r2=1; # r2 is the outer radius. r2 is greater than r1 and less than 1p1=1; # periodicity - the number of times the image will repeat per cyclep2=1; # number of strands - the number of "arms" the spiral will have# procedural scaling and rotationzoom=.1; #Between .1 and 10rotate=-30; #Between -360 and 360# Procedural ShiftingxShift=.2; #Between -1 and 1yShift=0; #Between -1 and 1### To avoid framing problems on the largest annulus when tiling based on transparency, look# outside (levelsToLookOut) levels to see if something farther out should cover up this pixel# Try setting to 0 to see framing errors; 1 should be sufficient unless you have three or more# image layers contributing to some pixel (in which case set it to 2 or more). Larger values# slow the code down, and may lead to floating point errors.##levelsToLookOut=3;########################################################################################################################## You should not have to change anything below this line ##########################################################################################################################imageX=W; # image size, in pixelsimageY=H;minDimension=min(imageX, imageY);## User Variables, set these on the User Settings Tab ##retwist=user_bool("Do Not Retwist (Leave Unchecked for Droste Effect)");retwist=!retwist;### Tiling can be based on transparency (if the input image is a tiff), or simply based on the# radius. Using transparency, there can be protrusions between different annular layers.# Tiling based on transparency, you can decide whether you want to look inward or # outward from a transparent pixel. For example, with a frame you'll want to look inward,# while for a flower you'll want to look outward.##tileBasedOnTransparency=user_bool("Tile Based on Transparency?"); transparentPointsIn=user_bool("Transparency Points In?");# Miscellaneous variablestrue=1;false=0;epsilon=.01;##Correct the Rotation Variablerotate=pi/180*rotate;### Droste-effect code starts here# Set Droste effect parameters##alpha=atan(p2/p1*log(r2/r1)/(2*pi));f=cos(alpha);beta=f*exp(I*alpha);# the angle of rotation between adjacent annular levelsif (p2 > 0)then angle = 2*pi*p1;elseangle =-2*pi*p1;end;### Code to set up the viewport properly##if (retwist) thenxbounds=[-r2,r2];ybounds=[-r2,r2];elseybounds=[0,2.1*pi];xbounds=[-log(r2/r1), log(r2/r1)];end;xymiddle=ri:[0.5*(xbounds[0]+xbounds[1]),0.5*(ybounds[0]+ybounds[1])];xyrange=xy:[xbounds[1]-xbounds[0], ybounds[1]-ybounds[0]];aspectRatio=W/H;xyrange[0]=xyrange[1]*aspectRatio;xbounds=[xymiddle[0]-0.5*xyrange[0],xymiddle[0]+0.5*xyrange[0]];z=ri:[(xbounds[0]+(xbounds[1]-xbounds[0])*(x+W/2)/W)+xShift,(ybounds[0]+(ybound s[1]-ybounds[0])*(y+H/2)/H)+yShift];if (retwist) then # only allow for procedural zooming/scaling in the standard coordinateszinitial=z;z=xymiddle+(z-xymiddle)/zoom*exp(-I*rotate);elsezinitial=r1*exp(z); # save these coordinates for drawing a frame laterzinitial=zinitial*zoom*exp(I*rotate);end;### The Droste effect math all takes place over the next six lines.# All the rest of the code is for niceties.##if (retwist) thenz2=log(z/r1);elsez2 = z;end;logz=z2; # save these coordinates for drawing a grid laterz=p1*z2/beta;rotatedscaledlogz=z; # save these coordinates for drawing a grid laterz=r1*exp(z);## End Droste effect math## Tilingif (tileBasedOnTransparency && levelsToLookOut > 0) thenif ( transparentPointsIn) then ratio=r1/r2*exp(-I*angle); end;if (!transparentPointsIn) then ratio=r2/r1*exp( I*angle); end;z=z*exp(levelsToLookOut*log(ratio));end;### When tiling based on transparency, color is accumulated into the colorSoFar variable,# while alphaRemaining tells how much remains for lower layers to contribute (initially 1,# finally 0).##colorSoFar=rgba:[0,0,0,0];alphaRemaining=1;ix=minDimension/2*z[0];iy=minDimension/2*z[1];color=origValXY(ix,iy);colorSoFar = colorSoFar + (color*(alpha(color)*alphaRemaining)); alphaRemaining=alphaRemaining*(1-alpha(color));# do we need to look inward from the current point, or outward?sign=0;if (tileBasedOnTransparency) thenif ( transparentPointsIn && alphaRemaining > epsilon) then sign=-1; end;if (!transparentPointsIn && alphaRemaining > epsilon) then sign= 1; end;elseradius=sqrt(z[0]*z[0]+z[1]*z[1]);if (radius < r1) then sign=-1; end;if (radius > r2) then sign= 1; end;end;if (sign < 0) then ratio=r2/r1*exp( I*angle); end;if (sign > 0) then ratio=r1/r2*exp(-I*angle); end;### Iteratively move inward or outward, until# the point has radius r in [r1, r2), if tileBasedOnTransparency=false# or until alphaRemaining=0, if tileBasedOnTransparency=true# In the latter case, we accumulate color at each step##iteration=0; maxiteration=10;while (sign != 0 && iteration < maxiteration) doz2=z*ratio;z=z2;rotatedscaledlogz=rotatedscaledlogz+ri:[0,-sign*angle];ix=minDimension/2*(z[0]);iy=minDimension/2*(z[1]);color=origValXY(ix,iy);colorSoFar = colorSoFar + (color*(alpha(color)*alphaRemaining));alphaRemaining=alphaRemaining*(1-alpha(color));radius=sqrt(z[0]*z[0]+z[1]*z[1]);sign=0;if (tileBasedOnTransparency) thenif ( transparentPointsIn && alphaRemaining > epsilon) then sign=-1; end;if (!transparentPointsIn && alphaRemaining > epsilon) then sign= 1; end;elseradius=sqrt(z[0]*z[0]+z[1]*z[1]);if (radius < r1) then sign=-1; end;if (radius > r2) then sign= 1; end;end;iteration=iteration+1;end;color=colorSoFar;color=rgba:[color[0], color[1], color[2], 1]; # set the alpha value to 1 (it could be <1 if the loop terminated at iteration maxiteration)#This last line is important, it returns the pixel value for the current pixel color。
海外文献原文-推荐参考文献列表

海外文献推荐-第一期参考文献:[1] I-Cheng Yeh, Che-Hui Lien, Tao-Ming Ting, 2015, Building multi-factor stock selection models using balanced split regression trees with sorting normalisation and hybrid variables, Foresight and Innovation Policy, V ol. 10, No. 1, 48-74[2] Eugene F.Fama, KennethR.French, 2015, A Five-factor Asset Pricing Model, Journal of Financial Economics 116, 1-22[3] Achim BACKHAUS, Aliya ZHAKANOV A ISIKSAL, 2016, The Impact of Momentum Factors on Multi Asset Portfolio, Romanian Journal of Economic Forecasting XIX (4), 146-169[4] Francisco Barillas, Jay Shanken, 2016, Which Alpha? Review of Financial Studies海外文献推荐-第二期参考文献:[1] PRA VEEN KUMAR, DONGMEI LI, 2016, Capital Investment, Innovative Capacity, and Stock Returns, The Journal of Finance, VOL. LXXI, NO. 5, 2059-2094[2] Houda Ben Mabrouk, Abdelfettah Bouri, 2013, New insight on the CAPM: a copula-based approach Tunisian and international evidence, Accounting and Finance, Vol. 4, No. 1, 35-62 [3] FERHAT AKBAS, 2016, The Calm before the Storm, The Journal of Finance, VOL. LXXI, NO. 1,225-266海外文献推荐-第三期参考文献:[1] Yufeng Han, Guofu Zhou, Yingzi Zhu, 2016, A trend factor: Any economic gains from using information over investment horizons? Journal of Financial Economics 122, 352-375[2] Andrea Frazzini, LasseHeje Pedersen, 2014, Betting against beta, Journal of Financial Economics 111, 1-25[3] Doron Avramov, Si Cheng, and Allaudeen Hameed, 2016, Time-Varying Liquidity and Momentum Profits, JOURNAL OF FINANCIAL AND QUANTITATIVE ANAL YSI, Vol. 51, No. 6, 1897-1923[4] Nicholas Barberis, Abhiroop Mukherjee, Baolian Wang, 2014, Prospect Theory and Stock Returns: An Empirical Test, Review of Financial Studies海外文献推荐-第四期参考文献:[1] Brad M. Barber, Xing Huang, Terrance Odean, 2014, Which risk factors matter to investors? Evidence from mutual fund flows, Review of Financial Studies[2] MICHAEL J. COOPER, HUSEYIN GULEN, & MICHAEL J. SCHILL. (2008). Asset growth and the cross‐section of stock returns. Social Science Electronic Publishing, 63(4), 1609–1651.[3]Bollerslev, T., Li, S. Z., & Todorov, V. (2016). Roughing up beta: continuous versus discontinuous betas and the cross section of expected stock returns. Journal of Financial Economics, 120(3), 464-490.[4]Baker, M., Wurgler, J., & Yuan, Y. (2012). Global, local, and contagious investor sentiment ⋆. Journal of Financial Economics, 104(2), 272-287.海外文献推荐-第五期参考文献:[1] Nicole Choi, Mark Fedenia, Tatyana Sokolyk, 2017, Portfolio Concentration and Performance of Institutional Investors Worldwide, Journal of Financial Economics[2]Cronqvist, H., Siegel, S., & Yu, F. (2015). Value versus growth investing: why do differentinvestors have different styles? ☆. Journal of Financial Economics, 117(2), 333-349.[3]Rapach, D. E., Ringgenberg, M. C., & Zhou, G. (2016). Short interest and aggregate stock returns . Journal of Financial Economics, 121(1), 46-65.[4]Novy-Marx, R. (2013). The other side of value: the gross profitability premium ☆. Journal of Financial Economics, 108(1), 1-28.海外文献推荐-第六期参考文献:[1] Suk Joon Byun, Sonya S. Limy, and Sang Hyun Yun, 2012, Continuing Overreaction and Stock Return Predictability, Journal of Financial and Quantitative Analysis[2]Eugene F. Fama, & Kenneth R. French. (2016). International tests of a five-factor asset pricing model. Journal of Financial Economics, 123.[3]Keloharju, M., Linnainmaa, J. T., & Nyberg, P. (2016). Return seasonalities. Journal of Finance, 71(4), n/a-n/a.[4]Seasholes, M. S., & Wu, G. (2007). Predictable behavior, profits, and attention. Journal of Empirical Finance, 14(5), 590-610.[5]PA VEL SAVOR, & MUNGO WILSON. (2016). Earnings announcements and systematic risk. The Journal of Finance, 71(1).海外文献推荐-第七期参考文献:[1] Cary Frydman and Colin Camerer, 2016, Neural Evidence of Regret and its Implications for Investor Behavior, Review of Financial Studies 29, 3108-3139[2] Haghani, V., & Dewey, R. (2016). A case study for using value and momentum at the asset class level. Journal of Portfolio Management, 42(3), 101-113.[3] Tarun, C., Amit, G., & Narasimhan, J. (2011). Buyers versus sellers: who initiates trades, and when?. Journal of Financial & Quantitative Analysis, 51(5), 1467-1490.[4] Hartzmark, M. S. (2015). The worst, the best, ignoring all the rest: the rank effect and trading behavior. Review of Financial Studies, 28(4), 1024.[5] Daniel, K., & Moskowitz, T. J. (2016). Momentum crashes. Journal of Financial Economics, 122(2), 221-247.海外文献推荐-第八期参考文献:[1]Hua, R., Kantsyrev, D., & Qian, E. (2012). Factor-timing model.Journal of Portfolio Management,39(1), 75-87.[2]Leshem, R., Goldberg, L. R., & Cummings, A. (2015). Optimizing value.Journal of Portfolio Management,42(2).[3]Chemmanur, Thomas J., Gang Hu and Jiekun Huang, 2015, Institutional Investors and the Information Production Theory of Stock Splits,Journal of Financial and Quantitative Analysis50(3), 413–445.海外文献推荐-第九期参考文献:[1]Penaranda, F. (2016). Understanding portfolio efficiency with conditioning information. Economics Working Papers, 51(3), 985-1011.[2]Cederburg, S., & O'Doherty, M. S. (2016). Does it pay to bet against beta? on the conditional performance of the beta anomaly. Journal of Finance, 71(2), 737-774.[3]Lindsey, R. R., & Weisman, A. B. (2016). Forced liquidations, fire sales, and the cost of illiquidity. Journal of Portfolio Management, 20(1), 45-57.海外文献推荐-第十期参考文献:[1] Easley, D., Hvidkjaer, S., & O'Hara, M. (2010). Factoring information into returns. Journal of Financial & Quantitative Analysis, 45(2), 293-309.[2]Babenko, I., Boguth, O., & Tserlukevich, Y. (2016). Idiosyncratic cash flows and systematic risk. Journal of Finance, 71(1).[3]Chow, V., & Lai, C. W. (2015). Conditional sharpe ratios. Finance Research Letters, 12, 117-133.海外文献推荐-第十一期参考文献:[1] Mladina, P. (2017). Illuminating hedge fund returns to improve portfolio construction. Social Science Electronic Publishing, 41(3), 127-139.[2] Choi, N., Fedenia, M., Skiba, H., & Sokolyk, T. (2016). Portfolio concentration and performance of institutional investors worldwide. Journal of Financial Economics.[3] Martijn Boons, 2016, State variables, macroeconomic activity, and the cross section of individual stocks, Journal of Financial Economics 119, 489-511海外文献推荐-第十二期参考文献:[1] Blanchett, D., & Ratner, H. (2015). Building efficient income portfolios. Journal of Portfolio Management, 41(3), 117-125.[2] Özde Öztekin. (2015). Capital structure decisions around the world: which factors are reliably important?. Journal of Financial & Quantitative Analysis, 50(3).[3] 2015, Does the number of stocks in a portfolio influence performance? Investment Sights海外文献推荐-第十三期参考文献:[1]Glushkov, D., & Statman, M. (2016). Classifying and measuring the performance of socially responsible mutual funds.Social Science Electronic Publishing,42(2), 140-151.[2]KLAUS ADAM, ALBERT MARCET, & JUAN PABLO NICOLINI. (2016). Stock market volatility and learning.The Journal of Finance,71(1), 419–438.[3]Miller, K. L., Li, H., Zhou, T. G., & Giamouridis, D. (2012). A risk-oriented model for factor timing decisions.Journal of Portfolio Management,41(3), 46-58.海外文献推荐-第十四期参考文献:[1]Feldman, T., Jung, A., & Klein, J. (2015). Buy and hold versus timing strategies: the winner is ….Journal of Portfolio Management,42(1), 110-118.[2]Eric H Sorensen, Nicholas F Alonso. The Resale Value of Risk-Parity Equity Portfolios[J]. Journal of Portfolio Management, 2015, 41(2):23-32.海外文献推荐-第十五期参考文献:[1]Barroso, P., & Santa-Clara, P. (2015). Momentum has its moments ☆.Journal of Financial Economics,116(1), 111-120.[2]Bender, J., & Nielsen, F. (2015). Earnings quality revisited.Social Science Electronic Publishing,39(4), 69-79.海外文献推荐-第十六期参考文献:[1]Greenberg, D., Abhilash, B., & Ang, A. (2016). Factors to assets: mapping factor exposures to asset allocations. Journal of Portfolio Management, 42(5), 18-27.[2]Goyal, A., Ilmanen, A., & Kabiller, D. (2015). Bad habits and good practices. Journal of Portfolio Management, 41(4), 97-107.海外文献推荐-第十七期参考文献:[1]Vermorken, M. A., Medda, F. R., & Schröder, T. (2012). The diversification delta: a higher-moment measure for portfolio diversification. Journal of Portfolio Management, 39(1), 67-74.[2]Asl, F. M., & Etula, E. (2012). Advancing strategic asset allocation in a multi-factor world.Journal of Portfolio Management,39(1), 59-66.海外文献推荐-第十八期参考文献:[1]Chakrabarty, B., Moulton, P. C., & Trzcinka, C. (2016). The performance of short-term institutional trades. Social Science Electronic Publishing, 1-26.[2]Stubbs, R. A., & Jeet, V. (2015). Adjusted Factor-Based Performance Attribution. USXX.海外文献推荐-第十九期参考文献:[1]Copeland, M., & Copeland, T. (2016). Vix versus size. Journal of Portfolio Management, 42(3), 76-83.[2]Kritzman, M., & Turkington, D. (2016). Stability-adjusted portfolios. Journal of Portfolio Management, 42(5), 113-122.海外文献推荐-第二十期参考文献:[1]Benos, E., Brugler, J., Hjalmarsson, E., & Zikes, F. (2016). Interactions among high-frequency traders. Journal of Financial & Quantitative Analysis, 52, 1-28.[2]Richardson, S., Sloan, R., & You, H. (2011). What makes stock prices move? fundamentals vs. investor recognition. Financial Analysts Journal, 68(2), 30-50.海外文献推荐-第二十一期参考文献:[1]Bogousslavsky, V. (2016). Infrequent rebalancing, return autocorrelation, and seasonality. Journal of Finance, 71(6), 2967-3006.[2]Marcos, L. D. P. (2015). The future of empirical finance. Journal of Portfolio Management, 41(4), 140-144.海外文献推荐-第二十二期参考文献:[1] Fabian, H., & Marcel, P. (2016). Estimating beta. Journal of Financial & Quantitative Analysis, 51(4), 1437-1466.[2] Christopher Cheung, George Hoguet, & Sunny Ng. (2014). Value, size, momentum, dividend yield, and volatility in china’s a-share market. Journal of Portfolio Management, 41(5), 57-70.海外文献推荐-第二十三期参考文献:[1]Mclean, R. D., & Zhao, M. (2014). The business cycle, investor sentiment, and costly external finance.Journal of Finance, 69(3), 1377–1409.[2]Kaniel, R., & Parham, R. (2017). The impact of media attention on consumer and mutual fund investment decisions. Journal of Financial Economics, 123, págs. 337-356海外文献推荐-第二十四期参考文献:[1]Chang, X., Chen, Y., & Zolotoy, L. (2017). Stock liquidity and stock price crash risk. Journal of Financial & Quantitative Analysis.[2]Bisetti, E., Favero, C. A., Nocera, G., & Tebaldi, C. (2013). A multivariate model of strategic asset allocation with longevity risk. Ssrn Electronic Journal.海外文献推荐-第二十五期参考文献:[1] Lou, X., & Shu, T. (2013). Price impact or trading volume: why is the amihud (2002) measure priced?. Social Science Electronic Publishing.[2]Lins, K. V., Servaes, H., & Tamayo, A. (2017). Social capital, trust, and firm performance: the value of corporate social responsibility during the financial crisis. Journal of Finance, 72.海外文献推荐-第二十六期参考文献:[1] Golez, B., & Koudijs, P. (2014). Four centuries of return predictability. Social Science Electronic Publishing.[2]Ledoit, O., and Wolf, M. (2017). Nonlinear shrinkage of the covariance matrix for portfolio selection: Markowitz meets Goldilocks. The Review of Financial Studies, 30(12), 4349-4388.海外文献推荐-第二十七期参考文献:[1]Ray Dalio, Bob Prince, Greg Jensen (2015), our thoughts about risk parity and all weather, Bridgewater Associates, LP[2]Thierry, R. and Guillaume, W. (2013). Risk Parity Portfolios with Risk Factors. MPRA Paper No. 44017.海外文献推荐-第二十八期参考文献:[1] Golubov, A., & Konstantinidi, T. (2015). Where is the risk in value? evidence from a market-to-book decomposition. Social Science Electronic Publishing.[2] Moreira, A., and Muir, T. (2017). Volatility‐Managed Portfolios. Journal of Finance, 72(4).海外文献推荐-第二十九期参考文献:[1]Wahalab S. Style investing, comovement and return predictability ☆[J]. Journal of Financial Economics, 2013, 107(1).[2]Pástor Ľ, Stambaugh R F, Taylor L A. Do funds make more when they trade more?[J]. The Journal of Finance, 2017, 72(4): 1483-1528.海外文献推荐-第三十期参考文献:[1] K Hou, C Xue, L Zhang, Digesting Anomalies: An Investment Approach, NBER Working Papers, 2015, 28(3)[2]Berk, J. B., & Binsbergen, J. H. V. (2013). Measuring skill in the mutual fund industry. Journal of Financial Economics, 118(1), 1-20.海外文献推荐-第三十一期参考文献:[1]Klein, Rudolf F. and V. K. Chow. "Orthogonalized factors and systematic risk decomposition." Quarterly Review of Economics & Finance 53.2(2013):175-187.[2]Sorensen E H, Hua R, Qian E E. Contextual Fundamentals, Models, and Active Management[J]. Journal of Portfolio Management 32.1(2005):23-36.海外文献推荐-第三十二期参考文献:[1] Hong, H. Torous, W. & Valkanov, R. (2007). Do industries lead stock markets? Journal of Financial Economics,83 (2), 367-396.[2]Dhillon, J. Ilmanen, A. & Liew, J. (2016). Balancing on the life cycle: target-date funds need better diversification. Journal of Portfolio Management, 42(4), 12-27.海外文献推荐-第三十三期参考文献:[1]Kenneth Froot and Melvyn Teo, Style Investing and Institutional Investors, JOURNAL OF FINANCIAL AND QUANTITATIVE ANALYSIS V ol. 43, No. 4, Dec. 2008, pp. 883–906.[2]Israel R, Palhares D, Richardson S A. Common factors in corporate bond returns[J]. Social Science Electronic Publishing, 2015.海外文献推荐-第三十四期参考文献:[1] DM Smith, N Wang, Y Wang, EJ Zychowicz, Sentiment and the Effectiveness of Technical Analysis: Evidence from the Hedge Fund Industry,Journal of Financial & Quantitative Analysis, 2016 , 51 (6) :1991-2013[2]Ronen Israel, Sarah Jiang, and Adrienne Ross (2018). Craftsmanship Alpha: An Application to Style Investing. Journal of Portfolio Management.海外文献推荐-第三十五期参考文献:[1] Huang J. The customer knows best: The investment value of consumer opinions [J]. Journal of Financial Economics, 2018.[2]Alberg J, Lipton Z C. Improving Factor-Based Quantitative Investing by Forecasting Company Fundamentals, Time Series Workshop at the 31st Conference on Neural Information Processing Systems (NIPS 2017). 2017.海外文献推荐-第三十六期参考文献:[1] Davis, J. H., Aliagadiaz, R. A., Ahluwalia, H., & Tolani, R. (2017). Improving U.S. stock return forecasts: a 'fair-value' cape approach.Social Science Electronic Publishing.海外文献推荐-第三十七期参考文献:[1] Fama, E. F., & French, K. R.(2018). Choosing factors. Journal of Financial Economics, 128: 234–252.[2] Bruder, Benjamin, Culerier, Leo, & Roncalli, Thierry. (2013). How to design target-date funds?. Ssrn Electronic Journal.海外文献推荐-第三十八期参考文献:[1] David Aboody, Omri Even-Tov, Reuven Lehavy, Brett Trueman. (2018). Overnight Returns and Firm-Specific Investor Sentiment. Journal of Financial and Quantitative Analysis.[2] Arnott R, Beck N, Kalesnik V, et al. How Can 'Smart Beta' Go Horribly Wrong?[J]. Social Science Electronic Publishing, 2017.海外文献推荐-第三十九期参考文献:[1] CS Asness, A Frazzini, LH PedersenDM, 2013,Quality Minus Junk,Social Science Electronic Publishing[2] Stein, M, & Rachev, S. T. (2011). Style-neutral funds of funds: diversification or deadweight? Journal of Asset Management, 11(6), 417-434.海外文献推荐-第四十期参考文献:[1] Li Y, Sun Q, Tian S. The impact of IPO approval on the price of existing stocks: Evidence from China[J]. Journal of Corporate Finance, 2018.[2] Jennifer Bender,Xiaole Sun,Ric Thomas,V olodymyr Zdorovtsov, The Journal of Portfolio Management , 2018 , 44 (4) :79-92海外文献推荐-第四十一期参考文献:[1] Yi Fang & Haiping Wang (2015) Fund manager characteristics and performance, Investment Analysts Journal, 44:1, 102-116.[2] Roni Israelov, Harsha Tummala. An Alternative Option to Portfolio Rebalancing. The Journal of Derivatives Spring 2018, 25 (3) 7-32海外文献推荐-第四十二期参考文献:[1] Robert Capone, Adam Akant, (2016), Trend Following Strategies in Target-Date Funds, AQR Capital Management.[2] Loh, R. K., & Stulz, R. M. (2018). Is sell‐side research more valuable in bad times?. Journal of Finance, 73(3): 959-1013.海外文献推荐-第四十三期参考文献:[1] Asness, C. S., Frazzini, A., Israel, R., & Moskowitz, T. J. (2015). Fact, fiction, and value investing. Final version published in Journal of Portfolio Management, V ol. 42, No.1[2] Gu, S., Kelly, B. T., & Xiu, D. (2018). Empirical asset pricing via machine learning. Social Science Electronic Publishing.海外文献推荐-第四十四期参考文献:[1] David P. Morton, Elmira Popova, Ivilina Popova, Journal of Banking & Finance 30 (2006) 503–518海外文献推荐-第四十五期参考文献:[1] Lleo, S., & Ziemba, W. T. (2017). A tale of two indexes: predicting equity market downturns in china. Social Science Electronic Publishing海外文献推荐-第四十六期参考文献:[1] Alquist, R., Israel, R., & Moskowitz, T. J. (2018). Fact, fiction, and the size effect. Social Science Electronic Publishing.[2] Kacperczyk M, NIEUWERBURGH S V A N, Veldkamp L. Time-varying fund manager skill[J]. The Journal of Finance, 2014, 69(4): 1455-1484.海外文献推荐-第四十七期参考文献:[1] Tom Idzorek, 2008, Lifetime Asset Allocations: Methodologies for Target Maturity Funds, Ibbotson Associates Research Paper,29-47[2] Da, Z., Huang, D., & Yun, H. (2017). Industrial electricity usage and stock returns. Journal of Financial & Quantitative Analysis, 52(1), 37-69.海外文献推荐-第四十八期参考文献:[1] Clifford Asness and Andrea Frazzini, 2013, The Devil in HML’s Details, The Journal of Portfolio Management, volume 39 number 4.[2] Carvalho, R. L. D., Xiao, L., & Moulin, P. (2011). Demystifying equity risk-based strategies: a simple alpha plus beta description.Journal of Portfolio Management,38(3), 56-70.海外文献推荐-第四十九期参考文献:[1]Jordan Brooks, Diogo Palhares, Scott Richardson, Style investing in fixed income, Journal of Portfolio Management.[2] R Ball,J Gerakos,JT Linnainmaa,V Nikolaev,2015,Deflating profitability,Journal of Financial Economics, 117 (2) :225-248海外文献推荐-第五十期参考文献:[1] Padmakar Kulkarni, Abhishek Gupta, Stuart Doole, 2018, How can Factors be Combined, MSCI.[2] Hsieh, C. C., Hui, K. W., & Zhang, Y. (2016). Analyst report readability and stock returns. Journal of Business Finance & Accounting, 43(1-2), págs. 98-130.海外文献推荐-第五十一期参考文献:[1] Cici G, Rosenfeld C. A study of analyst-run mutual funds: The abilities and roles of buy-side analysts [J]. Journal of Empirical Finance, 2016, 36:8-29.[2] U-Wen Kok, CFA, Jason Ribando, CFA, and Richard Sloan Facts about Formulaic Value Investing Financial Analysts Journal. V olume 73, Issue 2海外文献推荐-第五十二期参考文献:[1] Morningstar Manager Research.(2018)Target-Date Fund Landscape. 7 May 2018[2] Yong Chen, Gregory W. Eaton, Bradley S. Paye, Micro(structure) before Macro? The Predictive Power of Aggregate llliquidity for Stock Returns and Economic Activity, Journal of Financial Economics (2018), doi: 10.1016/j.jfineco.2018.05.011海外文献推荐-第五十三期参考文献:[1]Arnott R D, Chaves D B, Chow T. King of the Mountain:, Shiller P/E and Macroeconomic Conditions[J]. Social Science Electronic Publishing, 2015, 44(1):55-68.[2]Risk Parity Portfolio vs. Other Asset Allocation Heuristic Portfolios [J]. The Journal of Investing. 2010 December海外文献推荐-第五十四期参考文献:[1]Cliff's Perspective, Our Model Goes to Six and Saves Value From Redundancy Along the Way,AQR Capital Management, December 17, 2014[2]D Avramov,S Cheng,A Schreiber,K Shemer,2017,Scaling up Market Anomalies,Social Science Electronic Publishing,26 (3) :89-105海外文献推荐-第五十五期参考文献:[1]Aurélien Philippot,Analysts’ reinitiations of coverage and market underreaction,Journal of Banking and Finance , 94 (2018) 208–220海外文献推荐-第五十六期参考文献:[1]Michael W. Brandt, Earnings Announcements are Full of Surprises,Social Science Electronic Publishing, January 22, 2008[2]Sujin Pyo, Jaewook Lee,Exploiting the low-risk anomaly using machine learning to enhance the Black–Litterman framework: Evidence from South Korea,Pacific-Basin Finance Journal,51 (2018) 1–12[3]Robert F Engle and Andrew J Patton,What good is a volatility model?,Robert F Engle and Andrew J Patton海外文献推荐-第五十七期参考文献:[1]Nic Schaub, The Role of Data Providers as Information Intermediaries,Social Science Electronic Publishing, 2015 :1-34海外文献推荐-第五十八期参考文献:[1]Binu George and Hardik Shah, ESG: Improving Your Risk-Adjusted Returns in Emerging Markets,GMO White Paper, Mar 2018海外文献推荐-第五十九期参考文献:[1]Campbell R. Harvey and Yan Liu. Backtesting. Journal of portfolio management, 2015海外文献推荐-第六十期参考文献:[1]Mclean R D, Pontiff J. Does Academic Research Destroy Stock Return Predictability?[J]. Journal of Finance, 2016, 71(1)海外文献推荐-第六十一期参考文献:[1]Israelov R, Tummala H. Which Index Options Should You Sell?[J]. Social Science Electronic Publishing, 2017海外文献推荐-第六十二期参考文献:[1]Eric H. Sorensen, Keith L. Miller, and Chee K. Ooi,2000,The Decision Tree Approach to Stock Selection,The Journal of Portfolio Management,42-52海外文献推荐-第六十三期参考文献:[1]Donangelo A, Gourio F, Kehrig M, et al. The cross-section of labor leverage and equity returns[J]. Journal of Financial Economics, 2018海外文献推荐-第六十四期参考文献:[1]Qang Bu. Do Persistent Fund Alphas Indicate Manager Skill? [J]. Journal of Wealth Management,2017,20(2)82-93海外文献推荐-第六十五期参考文献:[1]Miguel A. Lejeune A VaR Black–Litterman model for the construction of absolute return fund-offunds [J] Quantitative Finance · January 2009海外文献推荐-第六十六期参考文献:[1]Fan J H, Zhang T. Demystifying Commodity Futures in China [J]. Social Science Electronic Publishing, 2018海外文献推荐-第六十七期参考文献:[1]Jon Hale, Sustainable Funds U.S. Landscape Report. Morningstar Research, 2018.海外文献推荐-第六十八期参考文献:[1]Sun Z, Wang A, Zheng L. Only Winners in Tough Times Repeat: Hedge Fund Performance Persistence over Different Market Conditions[J]. Journal of Financial and Quantitative Analysis, 2018.海外文献推荐-第六十九期参考文献:[1] A´LVARO CARTEA,SEBASTIAN JAIMUNGAL. RISK METRICS AND FINE TUNING OF HIGH-FREQUENCY TRADING STRATEGIES [J]. Mathematical Finance, V ol. 00, No. 0 (xxx 2013), 1-36.海外文献推荐-第七十期参考文献:[1] Dopfel, Frederick E. , and L. Ashley . "Optimal Blending of Smart Beta and Multifactor Portfolios." The Journal of Portfolio Management 44.4(2018):93-105.海外文献推荐-第七十一期参考文献:[1] Avraham Kamara, Robert Korajczyk, Xiaoxia Lou and Ronnie Sadka,2018,Short-Horizon Beta or Long-Horizon Alpha?, The Journal of Portfolio Management,45(1),96-105海外文献推荐-第七十二期参考文献:[1] Masulis, Ronald W., and Emma Jincheng Zhang. "How valuable are independent directors? Evidence from external distractions." Journal of Financial Economics (2018).海外文献推荐-第七十三期参考文献:[1] Hunter D, Kandel E, Kandel S, et al. Mutual fund performance evaluation with active peer benchmarks[J]. Journal of Financial economics, 2014, 112(1): 1-29.海外文献推荐-第七十四期参考文献:[1]Michael Stein and Svetlozar T. Rachev. Style Neutral Funds of Funds: Diversification or Deadweight? [J]. Journal of Asset Management, February 2011, V olume 11, Issue 6, pp 417–434海外文献推荐-第七十五期参考文献:[1] Elisabeth Kashner, 2019.01.31, Bogle led this investing Fee War, ;[2] Cinthia Murphy,2017,03.31, how to launch a successful ETF, ;[3] Drew V oros, 2019.01.23, how a small ETF Issuer Competes, ;[4] 2019.01.04, Invesco focusing on scale,海外文献推荐-第七十六期参考文献:[1] Shpak I , Human B , Nardon A . Idiosyncratic momentum in commodity futures[J]. Social Science Electronic Publishing, 2017.海外文献推荐-第七十六期参考文献:[1] Ehsani S , Linnainmaa J T . Factor Momentum and the Momentum Factor[J]. Social Science Electronic Publishing, 2017.海外文献推荐-第七十七期参考文献:[1] Iuliia Shpak*, Ben Human and Andrea Nardon. 2017.09.11, Idiosyncratic momentum in commodity futures. ResearchGate海外文献推荐-第七十八期参考文献:[1] Joel Hasbrouck. High-Frequency Quoting: Short-Term V olatility in Bids and Offers. JOURNAL OF FINANCIAL AND QUANTITATIVE ANALYSIS海外文献推荐-第七十九期参考文献:[1] Tarun Gupta and Bryan Kelly. Factor Momentum Everywhere. Institutional Investor Journals海外文献推荐-第八十期参考文献:[1] MICHAEL A. BABYAK , P H D. What You See May Not Be What You Get: A Brief, Nontechnical Introduction to Overfitting in Regression-Type Models. S T A T I S T I C A L C O R N E R海外文献推荐-第八十一期参考文献:[1] Eric Jondeau , Qunzi Zhang , Xiaoneng Zhu. Average Skewness Matters.海外文献推荐-第八十二期参考文献:[1] JOHN A. HASLEM. Morningstar Mutual Fund Measures and Selection Model. THE JOURNAL OF WEALTH MANAGEMENT海外文献推荐-第八十三期参考文献:[1] EUGENE F. FAMA and KENNETH R. FRENCH. Luck versus Skill in the Cross-Section of Mutual Fund Returns. THE JOURNAL OF FINANCE海外文献推荐-第八十四期参考文献:[1] How Transparent Are ETFs?[2] Lara Crigger. Nontransparent Active: Next ETF Revolution?.海外文献推荐-第八十五期参考文献:[1] Olivier Rousse and Benoît Sévi. Informed Trading in Oil-Futures Market. Fondazione Eni Enrico Mattei (FEEM)海外文献推荐-第八十六期参考文献:[1] Ari Levine and Lasse Heje Pedersen. Which Trend is Your Friend?。
Effects_of_gamma_irradiation_on_the_structure_and_mechanical_properties_of_wild_silkworms_and_Bombyx

Effects of Gamma Irradiation on The Structure and MechanicalProperties of Wild Silkworms and Bombyx Mori Silk Fibroin Films Siyong Xiong1,2, Yamei Xu1,Yuhong Jiao1,Lu Wang1, Mingzhong Li1,a1National Engineering Laboratory for Modern Silk, College of Textile and Clothing Engineering, Soochow University, No. 199 Ren’ai Road, Industrial Park, Suzhou 215123, China2Suzhou Institute of Scientific & Technical Information, No. 979 Renming Road, Suzhou 215002,Chinaa mzli@Keywords: Silk Fibroin; Film; Structure; Mechanical Properties;Gamma IrradiationAbstract: The structure and mechanical properties of A. yamamai, A. perny and B. mori silk fibroin films irradiated by gamma ray with various doses of 0, 25, 50, 100 and 200 kGy, respectively were determined by XRD, FT-IR, DSC and Instron 3365 equipment. Results showed that the aggregation structure and molecular conformation of A. yamamai, A. perny and B. mori silk fibroin films irradiated by gamma ray with those doses mentioned above were not significantly changed. However, with the increase of radiation intensity, the thermal stability of silk fibroin films declined slightly, and the breaking strength and extensibility reduced significantly, due to the breakdown of parts of secondary bonds and covalent bonds. These results suggested that, when these silk fibroin materials were sterilized by gamma irradiation, smaller radiation doses should be used, otherwise irreversible damages on these materials would be caused.IntroductionSilk fibroin produced from cultivated silkworms or wild silkworms has been famous for the outstanding mechanical properties, and silk fibroin materials had been studied for new biomedical applications due to its excellent biocompatibility, slow degradability and permeability to oxygen.Regenerated silk fibroin film is one of the important form of silk fibroin materials and it has been shown to support stem cell adhesion, proliferation and differentiation in vitro and promote tissue repair in vivo[1-4]. However, biomedical materials had to be sterilized for clinical application and there were various methods for sterilization[5-8]. Gamma radiation is one of the common sterilization methods, it is necessary to explore the effects of gamma radiation on the structure and properties of silk fibroin films, which could provide theoretical basis for medical applications in the future. Researchers had studied the changes in the fine structure of Bombyx mori (B. mori) silk fibroin fibers irradiated by gamma ray[9]. However, there were few papers studied about the changes in structure and properties of Antheraea yamamai (A. yamamai), Antheraea perny (A. perny) and B. mori silk fibroin films irradiated by gamma ray.In this paper, we mainly focused on the effects of gamma radiation on the structure and mechanical properties of A. yamamai, A. perny silk fibroin films. The changes in the structure and mechanical properties of B. mori silk fibroin films irradiated by gamma ray were taken as controls.Materials and methodsPreparation of regenerated A. yamamai, A. perny and B. mori silk fibroin solutionThe A. yamamai cocoons were treated three times with 0.35 wt % Na2CO3 solution at 98~100°C for 30 min to remove sericin. Degummed A. yamamai silk fibers were dissolved in the melting Calcium Nitrate solutions for 1 h at 90°C and the liquor ratio was 1:20. Then the mixed solution was dialyzed in the flowing distilled water for 96h. The A. yamamai silk fibroin solution withconcentration of about 3.0 wt % was obtained. The preparation of A. perny silk fibroin solution wasaqueous Natrium Carbonicum solutions (0.25 wt %), the liquor ratio (1:10), and the dissolving time (5 hours).According to the literature[10], the B. mori silks were treated three times with 0.05 wt % Na2CO3 solution at 98~100°C for 30 min to remove sericin, respectively. The pure silk fibroin fibers were dissolved in the triadic solvent CaCl2·CH3CH2OH·H2O (mole ratio=1:2:8) for 1 h at 78±2°C through stirring and the liquor ratio was 1:10. The prepared solution was purified by dialyzed for 96h. The regenerated B. mori silk fibroin solution with concentration of about 3.0 wt % was obtained. Preparation of silk fibroin films and gamma irradiationThe silk fibroin films were prepared by casting the same volume of silk fibroin solution (40mL) on a polyethylene dish and air-dried at 20°C. Then A. yamamai, A. perny and B. mori silk fibroin films were obtained. Each type of silk fibroin films was divided into five portion irradiated by gamma ray with 0, 25, 50, 100, 200kGy at room temperature, respectively. The irradiations were carried out by a 6.4×104 Curie 60Co irradiation facility located at Soochow University, China.MeasurementsXRD was performed by a Rigaku D/Max-3C diffractometer with Cu-Kα radiation (λ= 0.15418 nm). The X-ray source was operated at 40 kV and 40 mA. Diffraction intensity was measured in reflection mode at scanning rate of 2°/min for 2θ=5~45°.FT-IR spectra were obtained by a Nicolet Avatar-IR360 in the spectral region of 400~1800 cm-1.The DSC measurements were carried out under Nitrogen by a Perkin-Elmer Diamond DSC/TG instrument at a heating rate of 15 °C/min.The mechanical properties of silk fibroin films (15 mm × 2 mm) were determined on Instron 3365 equipment under relative humidity of 65% at 20 °C. The crosshead speed was 10mm/min. Results and discussionXRD(1)(2)Figure 1 XRD curves of silk fibroin films irradiated by gamma ray with various doses: (1) A. yamamai silk fibroin films;(2) B. mori silk fibroin films; a—silk fibroin films without gamma irradiation; b, c, d, e—silk fibroin films irradiated with25, 50, 100 and 200 kGy, respectively.In Figure 1(1), there are some apparent diffraction peaks around 11.8º and 22.0º in XRD curve (a) of A. yamamai silk fibroin films without gamma irradiation. It indicates that silk fibroin film mainly consists of α-helix structure, according to the studies on wild silkworms silk fibroin molecular conformation[11-18], and a certain amount of amorphous structure is possibly existed. In addition, with the increase of irradiation doses, these diffraction peaks around 11.8º and 22.0º in curve b to e almost have no changes, indicating that the crystal structure of A. yamamai silk fibroin films has no significant changes after gamma irradiation. XRD curves of A. perny silk fibroin films irradiated by gamma ray with various doses are similar to that of A. yamamai silk fibroin films, due to their approximate primary structure.In Figure 1(2), based on those previous studies of B. mori silk fibroin[10, 19], there are some apparent diffraction peaks around 9.1º (w) in XRD curve (a) of B. mori silk fibroin films withouthas no significant changes, indicating that the crystal structure of B. mori silk fibroin films has no visible changes.FTIR spectra(1)(2)Figure 2 FTIR spectra of silk fibroin films irradiated by gamma ray with various doses: (1) A. yamamai silk fibroin films;(2) B. mori silk fibroin films; a—silk fibroin films without gamma irradiation; b, c, d, e—silk fibroin films irradiated with25, 50, 100 and 200 kGy, respectively.In Figure 2(1), these bands at 1652cm-1 (amide I), 1540 cm-1 (amide II), 892 cm-1 (amide IV), 622 cm-1 (amide V) in FT-IR spectra (a-e) are attributed to α-helix structure[11-15], suggesting that these A. yamamai silk fibroin films contain much α-helix structure. The bands at 1238 cm-1 (amide III), 966 cm-1(amide IV) indicated that it still contained a few β-sheet structure. With the increase of irradiation doses, the band at 660 cm-1 (amide V) assigned to random coil disappeared gradually and the bands shifted from 622 cm-1 (amide V) and 660 cm-1 (amide V) to 696 cm-1 (amide V). It indicates that the molecular conformation of silk fibroin films was very likely to transform from random coil or α-helix to β-sheet. And there is no obvious change in the molecular conformation of A. yamamai silk fibroin films with gamma irradiation compared with that of the control samples, which was closely consistent with the results of XRD profile. The FT-IR spectra of A. perny silk fibroin films irradiated by gamma ray with various doses are also similar to that of A. yamamai silk fibroin films.In Figure 2(2), the FT-IR spectra (a-e) of B. mori silk fibroin films irradiated by gamma ray with various doses are similar. The intense bands at 1660 cm-1 (amide I), 1652 cm-1 (amide I), 1540 cm-1 (amide II) and 1536 cm-1(amide III) are assigned to random coil[16-18], indicating that it mainly contained random coil in these silk fibroin films. The bands at 1652 cm-1 (amide I), 1520 cm-1 (amide II) and 700 cm-1 (amide V) show that both silk I and silk II existed. With the increase of irradiation doses, there is no obvious change in the molecular conformation of B. mori silk fibroin films.DSC(1)(2)Figure 3 DSC curves of silk fibroin films irradiated by gamma ray with various doses: (1) A. yamamai silk fibroin films;(2) B. mori silk fibroin films; a—silk fibroin films without gamma irradiation; b, c, d, e—silk fibroin films irradiated with25, 50, 100 and 200 kGy, respectively.In Figure 3(1), the A. yamamai silk fibroin films without gamma irradiation exhibited an endothermal to exothermal transition at around 230 °C, attributed to the pyrolysis of silk fibroin films[20]endothermic peak at about 354.80 °C is due to the thermal decomposition of silk fibroin molecules with unoriented β-sheet configuration[18]. With the increase of irradiation doses, the thermal decomposition temperature of silk fibroin films reduced gradually but slightly, which was also similar to the results of DSC curves of A. perny silk fibroin films irradiated by gamma ray.In Figure 3(2), the B. mori silk fibroin films exhibited visible endothermal to exothermal transition peak from 207°C to 231 °C in curves a to e, especially the exothermal peak at around 218 °C, which is attributed to the transition of silk fibroin from amorphous to silk II[10], suggesting that these silk fibroin films still contain lots of amorphous structure. The endothermic peak at around 282 °C was attributed to the thermal decomposition. With the increase of irradiation doses, the thermal decomposition temperature of B. mori silk fibroin films reduced slightly. That is because when silk fibroin films were irradiated by the high-energy electron produced by gamma ray, the silk fibroin molecules absorbed radiation energy and the original thermodynamic equilibrium in these films was broken. Then it was ionized and polarized to produce various kinds of active particles (such as ions, secondary electrons, free radicals, etc.), leading to the breakage of hydrogen bonds and covalent bonds between silk fibroin molecules, which was very likely to damage the molecular chains. Meanwhile, the thermal movement of the silk fibroin molecules in the amorphous and crystalline regions increased with the increase of irradiation doses, the molecular chains rearrange to disorder silk fibroin chains and weaken intermolecular force, resulting in the scission of peptide bonds in the amorphous regions, the decrease of molecular orientation, and then the thermal stability declined[9]. Mechanical properties(1)(2)(3)Figure 4 The relation curves between irradiation dose and breaking strength or elongation at break of silk fibroin films in dry state: (1) A. yamamai silk fibroin films; (2) A. perny silk fibroin films; (3) B. mori silk fibroin films.In Figure 4(1) to (3), with the increase of irradiation doses, the breaking strength and elongation at break of silk fibroin films in dry state show a significant downtrend, especially the breaking strength. The breaking strength and elongation at break of B. mori silk fibroin films present almost a linear downtrend. These results were consistent with the analyses stated above. When the silk fibroin films were irradiated by gamma ray with various doses, free radical formation, main chains breakage, and disordering of silk fibroin chains were caused due to the broken of hydrogen bonds and peptide bonds in amorphous region, consequently, the average molecular orientation declined. Therefore, the breaking strength and elongation at break of silk fibroin films decreased[9]. However, there were no significant changes in the molecular conformation and crystal structure of silk fibroin films irradiated by gamma ray within the dosage range in this paper, but the breaking strength and elongation at break of silk fibroin films decreased significantly.ConclusionsThe effects of gamma radiation on the changes of structure and mechanical properties of A. yamamai, A. perny silk fibroin films were studied in this paper. Meanwhile, the changes in the structure and mechanical properties of B. mori silk fibroin films irradiated by gamma ray were taken as controls. Compared with silk fibroin films without gamma irradiation, the crystal structure and molecular conformation of those silk fibroin films irradiated by gamma ray with 25, 50, 100 and 200 kGy had no significant changes. With the increase of irradiation doses, the thermal stability of silk fibroin filmssignificantly. Therefore, considering mechanical properties, when sterilizing silk fibroin biomaterials by gamma irradiation, it is suggested that small doses had better be used.AcknowledgementsThis work was financially supported by National Natural Science Foundation of China (No. 30970714), College Natural Science Research Project of Jiangsu Province (No. 07KJA43010) and Nature Science Foundation of Jiangsu Province (No. BK2010252).References[1] Y. Wang, H.J. Kim, G. Vunjak-Novakovic, D.L. Kaplan. Biomaterials, 2006, 27(36): 6064-6082.[2] U.J. Kim, J. Park, H.J. Kim, M. Wada, D.L. Kaplan. Biomaterials, 2005, 26(15): 2275-2285.[3] D.W. Hutmacher. Biomaterials, 2000, 21: 2529-2543.[4] B.D. Lawrence, J.K. Marchant, M.A. Pindrus, et al. Biomaterials, 2009, 30:1299-1308.[5] Y. Shamis, S. Patel, A. Taube, et al. Tissue Engineering Part C: Methods, 2009, 15(3):445-454.[6] L. Morejon-Alonso, R.G. Carrodeguas, et al. Materials Research, 2007, 10(1):15-20.[7] M.S. Jahan, D.E. Thomas, M.D. Ridley. Materials Science Forum, 2003, 426-432(4):3139-3144.[8] K. Filipczak, M. Wozniak, P. Ulanski, et al. Macromolecular Bioscience, 2006, 6(4):261-273.[9] T. Masuhiro, et al. Journal of Applied Polymer Science, 1994, 51(5):823-829.[10] M.Z. Li, S.Z. Lu, Z.Y. Wu, et al. Journal of Applied Polymer Science, 2001, 79:2185-2191.[11] K. Hirabayashi, Y. Kondo, Y.Go. Sen-i Gakkaishi, 1967, 23(5): 199-207.[12] M.Z. Li, W. Tao, et al. Polymers for advanced technologies, 2003, 14: 694-698.[13] Y. Kondo, K. Hirabayashi, E. Iizuka, Y. Go. Sen-i Gakkaishi1, 1967, 23(7):311-315.[14] K. Hirabayashi, M. Tsukada. Journal of Sericulture Science, Japan, 1976, 45(6): 473-478.[15] M. Tsukada. Journal of Polymer Science Part B: Polymer Physics, 1986, 24: 457-460.[16] H.Y. Kweon, Y. H. Park. Journal of Applied Polymer Science, 2001, 82:750-758.[17] H.Y. Kweon, I.C. Um, Y.H. Park. Polymer, 2000, 41:7361-7367.[18] M. Tsukada, et al. Journal of Polymer Science Part B: Polymer Physics, 1994, 32:1407-1412.[19] M.Z. Li, et al. Journal of Dong Hua University (Nature Science Edition), 2001, 27: 12-19.[20] H. Kweon, S.O. Woo, Y.H. Park. Journal of Applied Polymer Science, 2001, 81(9): 2271-2276.New and Advanced Materialsdoi:10.4028//AMR.197-198Effects of Gamma Irradiation on the Structure and Mechanical Properties of Wild Silkworms and Bombyx Mori Silk Fibroin Filmsdoi:10.4028//AMR.197-198.27。
Environmentally hazardous substances

GMMOs and GMOs which do not meet the definition of toxic substances (see 2.6.2) or infectious substances (see 2.6.3) shall be assigned to UN 3245. GMMOs or GMOs are not subject to these Regulations when authorized for use by the competent authorities of the countries of origin, transit and destination. Genetically modified live animals shall be transported under terms and conditions of the competent authorities of the countries of origin and destination.
These designations are used for substances and mixtures which are dangerous to the aquatic environment that do not meet the classification criteria of any other class or another substance within Class 9. These designations may also be used for wastes not otherwise subject to these Regulations but which are covered under the Basel Convention on the Control of Transboundary Movements of Hazardous Wastes and their Disposal and for substances designated to be environmentally hazardous substances by the competent authority of the country of origin, transit or destination which do not meet the criteria for an environmentally hazardous substance according to these Regulations or for any other hazard Class. The criteria for substances which are hazardous to the aquatic environment are given in section 2.9.3. Genetically modified micro-organisms (GMMOs) and genetically modified organisms (GMOs) 3245 3245 GENETICALLY MODIFIED MICRO-ORGANISMS or GENETICALLY MODIFIED ORGANISMS
铝合金表面原位自组装超疏水膜层的制备及耐蚀性能_李松梅
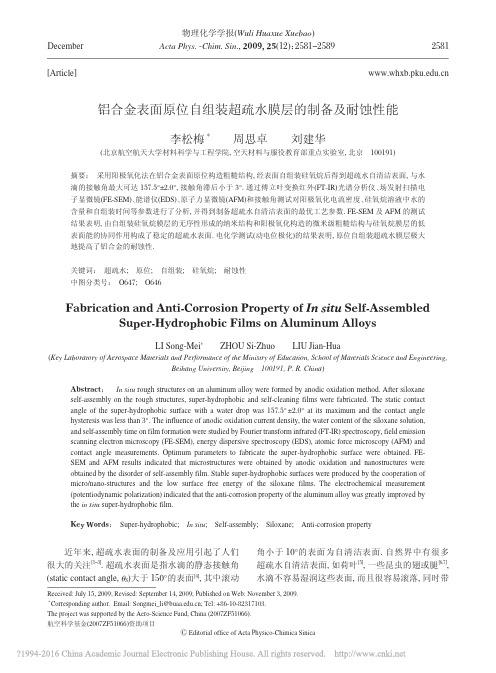
铝合金表面原位自组装超疏水膜层的制备及耐蚀性能李松梅*周思卓刘建华(北京航空航天大学材料科学与工程学院,空天材料与服役教育部重点实验室,北京100191)摘要:采用阳极氧化法在铝合金表面原位构造粗糙结构,经表面自组装硅氧烷后得到超疏水自清洁表面,与水滴的接触角最大可达157.5°±2.0°,接触角滞后小于3°.通过傅立叶变换红外(FT -IR)光谱分析仪、场发射扫描电子显微镜(FE -SEM)、能谱仪(EDS)、原子力显微镜(AFM)和接触角测试对阳极氧化电流密度、硅氧烷溶液中水的含量和自组装时间等参数进行了分析,并得到制备超疏水自清洁表面的最优工艺参数.FE -SEM 及AFM 的测试结果表明,由自组装硅氧烷膜层的无序性形成的纳米结构和阳极氧化构造的微米级粗糙结构与硅氧烷膜层的低表面能的协同作用构成了稳定的超疏水表面.电化学测试(动电位极化)的结果表明,原位自组装超疏水膜层极大地提高了铝合金的耐蚀性.关键词:超疏水;原位;自组装;硅氧烷;耐蚀性中图分类号:O647;O646Fabrication and Anti -Corrosion Property of In situ Self -AssembledSuper -Hydrophobic Films on Aluminum AlloysLI Song -Mei *ZHOU Si -ZhuoLIU Jian -Hua(Key Laboratory of Aerospace Materials and Performance of the Ministry of Education,School of Materials Science and Engineering,Beihang University,Beijing 100191,P.R.China )Abstract :In situ rough structures on an aluminum alloy were formed by anodic oxidation method.After siloxane self -assembly on the rough structures,super -hydrophobic and self -cleaning films were fabricated.The static contact angle of the super -hydrophobic surface with a water drop was 157.5°±2.0°at its maximum and the contact angle hysteresis was less than 3°.The influence of anodic oxidation current density,the water content of the siloxane solution,and self -assembly time on film formation were studied by Fourier transform infrared (FT -IR)spectroscopy,field emission scanning electron microscopy (FE -SEM),energy dispersive spectroscopy (EDS),atomic force microscopy (AFM)and contact angle measurements.Optimum parameters to fabricate the super -hydrophobic surface were obtained.FE -SEM and AFM results indicated that microstructures were obtained by anodic oxidation and nanostructures were obtained by the disorder of self -assembly film.Stable super -hydrophobic surfaces were produced by the cooperation of micro/nano -structures and the low surface free energy of the siloxane films.The electrochemical measurement (potentiodynamic polarization)indicated that the anti -corrosion property of the aluminum alloy was greatly improved by the in situ super -hydrophobic film.Key Words :Super -hydrophobic;In situ ;Self -assembly;Siloxane;Anti -corrosion property[Article]物理化学学报(Wuli Huaxue Xuebao )Acta Phys.-Chim.Sin .,2009,25(12):2581-2589近年来,超疏水表面的制备及应用引起了人们很大的关注[1-3].超疏水表面是指水滴的静态接触角(static contact angle,θS )大于150°的表面[4],其中滚动角小于10°的表面为自清洁表面.自然界中有很多超疏水自清洁表面,如荷叶[5],一些昆虫的翅或腿[6,7],水滴不容易湿润这些表面,而且很容易滚落,同时带December Received:July 15,2009;Revised:September 14,2009;Published on Web:November 3,2009.*Corresponding author.Email:Songmei_li@;Tel:+86-10-82317103.The project was supported by the Aero -Science Fund,China (2007ZF51066).航空科学基金(2007ZF51066)资助项目鬁Editorial office of Acta Physico -Chimica Sinica2581Acta Phys.-Chim.Sin.,2009Vol.25走表面的污物,从而达到自清洁的效果.这种现象被称为荷叶效应.Barthlott等[8]进行对荷叶表面研究,发现其表面微小的柱状突起构成的粗糙结构和表面的疏水性蜡质的协同作用是造成其表面具有特殊湿润性能的原因.随后,Jiang等[9]提出荷叶表面存在的微米-纳米的分等级的粗糙结构使得与水滴接触的表面孔隙中存在空气,增大了表观接触角,减小了接触角滞后(contact angle hysteresis,CAH=θadv-θrec,其中θadv为前进接触角,θrec为后退接触角),最终造成超疏水自清洁现象.人工超疏水表面的制备一直以来都是以模仿荷叶效应作为手段,即在疏水性材料表面构造粗糙结构[10-12]或对粗糙表面进行疏水性处理[13-17],衍生出很多独特的制备方法.这些方法制备的超疏水表面虽然疏水性能优异,但在很多领域之所以还没有成功的应用,主要原因在于其综合性能均有或多或少的缺陷,所以改进制备方法,提高超疏水表面的综合性能是其走向实际应用的第一步.在诸多制备超疏水表面的方法中,模板法的工艺成熟且成本相对较低,有很大的发展空间.模板法主要采用纯铝的多孔阳极氧化膜作为模板,在对聚合物进行热挤压成型后构造超疏水表面[18-20].但是,此方法得到的聚合物膜由于其与基体的结合力差、施工困难等缺陷,往往难于应用到金属表面.如果在零件上原位制备超疏水膜层则解决了以上难点.在金属基体上制备的超疏水自清洁表面不仅能够使其获得装饰效果,而且自清洁性能避免了腐蚀介质与金属的长时间接触,从而极大地提高了金属的耐腐蚀性能[21,22].本文即采用具有诸多优异性能的在现代日用、工业上应用最多的LY12铝合金作为制备超疏水表面的基体材料,对其表面进行阳极氧化后自组装低表面能的聚硅氧烷膜层,通过表面粗糙结构以及低表面能材料的协同作用在铝合金表面原位制备了超疏水自清洁表面.此外,硅氧烷水解后可以在阳极氧化铝合金表面形成强的化学键,保证了聚硅氧烷膜层与基体之间具有很好的结合力[23,24];而且,通过缩聚形成的硅氧网络的高键能使硅氧烷基聚合物具有良好的热稳定性[25].铝合金阳极氧化工艺不仅能在铝合金表面构造粗糙结构,而且还能够增加基体的耐蚀性,同时原位生长的方式使得超疏水结构与基体之间具有良好结合力.1实验部分1.1试剂材料LY12CZ航空铝合金板材切割为尺寸60mm×40mm×2mm,其成分列于表1.十二氟庚基丙基三甲氧基硅烷(G502,雪佳氟硅化学有限公司);甲醇,硫酸及氯化钠(分析纯,北京化工厂);十八水合硫酸铝(分析纯,天津市福晨化学试剂厂).1.2试样前处理LY12CZ铝合金首先经NaOH(40g·L-1)溶液除去表面包铝层;水磨砂纸打磨至1000#,去离子水超声波清洗10min;基体随后采用NaOH(40g·L-1)在60℃下碱洗2min,室温下采用三酸出光液(CrO3/ HNO3/HF)出光1min,去离子水清洗等处理后备用.1.3铝合金阳极氧化预处理后的LY12CZ铝合金采用硫酸恒电流阳极氧化工艺,硫酸浓度为180g·L-1,溶液中Al3+离子的浓度为10g·L-1,控制温度为30℃,控制电流密度在1.00至8.00A·dm-2之间,阳极氧化2h后得到不同粗糙结构的表面.阳极氧化后的试样经去离子水冲洗数次,以去除表面残留的溶液,立即进行下一步处理.1.4自组装低表面能材料修饰表面配制总体积100mL不同摩尔比例的甲醇及去离子水溶液,滴加G502并控制浓度为0.01mol·L-1, 30℃下磁力搅拌,老化2h,溶液呈半透明的乳白色.将阳极氧化后或预处理后的试样浸入该溶液中,恒温水浴30℃反应若干小时后取出.去离子水洗数次以去除表面未反应的小分子和溶剂,80℃下固化1 h后获得的试样进行下一步测试.1.5膜层性能的测试及表征试样表面粗糙结构的表征采用场发射扫描电镜FE-SEM(Hitachi S-4800SEM).采用能谱仪EDS (Hitachi S-530,Oxford Link2SISI)和傅立叶变换红外光谱分析仪FT-IR(Avatar-360,Nicolet)分别分析试样表面元素组成和低表面能聚合物与表面的键合情表1LY12CZ铝合金名义成分(w,质量分数)Table1Nominal chemical components of aluminum alloy LY12CZ(w,mass fraction) Element Cu Mg Fe Mn Si Zn Ti Cr others Alw(%) 3.8-4.9 1.2-1.80.500.30-0.900.500.250.150.100.15balance 2582No.12李松梅等:铝合金表面原位自组装超疏水膜层的制备及耐蚀性能况.在FE-SEM及EDS测试前试样表面喷金30s以确保具有良好的导电性.采用原子力显微镜AFM(MultiMode Nanoscope IIIa,Veeco Instruments Ins.)分析试样微区的表面形貌;测试采用轻敲模式(Tapping Mode),测试区域为5μm×5μm,扫描速率为10μm·s-1.表面与水滴的静态接触角及动态接触角测试采用Dataphysics OCA20接触角测试仪,测试均在室温下进行;滴液管缓慢地将2μL去离子水滴放在试样表面,得到静态接触角θS;动态接触角测试首先在试样表面滴加1μL水滴后,以1μL·s-1的滴加速度滴加至5μL,接触角增加至基本恒定且液滴即将滚动时测试得到的接触角为前进接触角θadv;相应的,将水滴吸入滴液管至液滴恰好离开试样表面或接触角恒定时测得的接触角为后退接触角θrec.接触角测试均选取试样表面5个以上不同点的测量结果的平均值作为接触角值.动电位极化曲线测试采用三电极体系,只经前处理的铝合金、阳极氧化后的铝合金和自组装超疏水膜层后的铝合金分别作为研究电极,铂丝电极为辅助电极,饱和甘汞电极(SCE)为参比电极,采用3.5% (w)的NaCl水溶液作为介质.测试采用电化学工作站(PARSTAT2273,Ametek,American),测试软件为PowerSuite测试系统,试样测试面积为7cm2,扫描速率为3mV·s-1.2结果与讨论2.1阳极氧化电流密度对表面湿润性的影响通过控制铝合金阳极氧化电流密度在1.00-8.00A·dm-2区间内制备了一系列不同粗糙结构的表面,经自组装十二氟庚基丙基三甲氧基硅烷(G502)后,得到了电流密度与2μL去离子水滴在表面的静态接触角及接触角滞后的关系曲线,如图1所示.在讨论电流密度的影响时,自组装G502溶液浓度固定为0.01mol·L-1,每100mL溶液中水的含量为3.7mol,自组装时间固定为4h.未经阳极氧化的铝合金表面自组装G502后得到几乎理想的光滑表面,与水滴的静态接触角θS=110.2°±1.0°,接触角滞后CAH>19°.当阳极氧化电流密度小于2.00A·dm-2时,静态接触角随电流密度的增加迅速增大,接触角滞后迅速降低.当电流密度在2.00至3.00 A·dm-2范围内时,静态接触角均大于150°,表面呈超疏水状态,且接触角滞后很小.电流密度在3.00至8.00A·dm-2范围内时,静态接触角均在150°附近,而接触角滞后略有上升.通过图1中接触角变化趋势的示意曲线分析得到,试样表面由疏水性向超疏水性的转变在2.00A·dm-2附近很窄的电流密度区间内发生.为了得到最优的电流密度参数,在1.00至3.00A·dm-2区间内依次在多个电流密度下制备了疏水表面,并测试了与水滴的静态接触角,电流密度及测试的接触角值的结果见表2.由表2可以得到当电流密度为2.25A·dm-2时,静态接触角达到最大,θS=157.5°±2.0°,并且经动态接触角测试,接触角滞后小于3°.图2为在电流密度2.25A·dm-2下制备的超疏水表面与水滴的接触照片及接触角测试照片.从图2(a,b)中可以清楚看出,水滴在其表面的静态接触角很大,且试样稍有倾斜或外加微小的扰动,水滴会表2不同电流密度制备的疏水表面与水滴的静态接触角及标准误差Table2Static contact angles and standard deviations(SD)of hydrophobic surfaces fabricatedwith different current densities图1水滴在试样表面的静态接触角(θS)及接触角滞后(CAH)与阳极氧化电流密度(I)的关系曲线Fig.1Dependence of static contact angle(θS)and contact angle hysteresis(CAH)of the water droplets on the surfaces on the current density(I)of anodicoxidationI/(A·dm-2)θS/(°)SD(°)1.00138.3 4.31.25131.4 4.91.50146.0 1.11.75144.9 1.42.00153.9 6.12.25157.5 2.02.50151.90.72.75154.1 2.13.00153.7 3.02583Acta Phys.-Chim.Sin.,2009Vol.25图3阳极氧化铝合金表面的FE -SEM 照片Fig.3FE -SEM images of the surface of anodized aluminum alloy(a,b)FE -SEM images with different amplified parameters of anodized film (current density:2.25A ·dm -2)without G502(dodecafluoroheptyl -propyl -trimethoxylsilane)self -assembly;(c-h)FE -SEM images with different amplified parameters of anodized films at different anodic current densities of1.00A ·dm -2(c,d),2.25A ·dm -2(e,f),and 8.00A ·dm -2(g,h)after G502self -assembly迅速滚落.图2d 显示出滴管上的水滴不会由于超疏水表面的粘滞作用而脱落,说明超疏水表面的接触角滞后非常小,具有优异的自清洁性能.铝合金在不同阳极氧化电流密度下的表面形貌见图3.由一定电流密度下制备的阳极氧化表面自组装G502前(图3(a,b))与自组装G502后(图3(e,f))对比可知,自组装膜层对阳极氧化表面微米尺度的形貌没有影响.不同电流密度下制备的阳极氧化膜层的表面形貌(如图3(c,e,g)所示)表明,阳极氧化电流密度的变化直接决定了表面的微观形貌,所以图2水滴在试样表面的状态照片及轮廓图Fig.2Photographs and profiles of the water droplets on the surfaces(a)digital photo of the water drops on super -hydrophobic surface;(b)profile of water droplet on super -hydrophobic surface;(c)profile of water droplet on the surface of anodized aluminum alloy without G502self -assembly;(d)behavior of water droplet on the super -hydrophobic surface(the directions of the arrowheads were the movements of the surfaces)2584No.12李松梅等:铝合金表面原位自组装超疏水膜层的制备及耐蚀性能图4不同阳极氧化电流密度下经自组装G502后铝合金表面形貌的AFM照片Fig.4AFM images of the surfaces at different anodic current densities after G502self-assemblyI/(A·dm-2):(a)1.00,(b)2.25,(c)8.00;scanning area:5μm×5μm在讨论阳极氧化对表面形貌的影响时,自组装膜层的影响可以忽略.由图3(c,d,e,f)可知,在1.00、2.25A·dm-2电流密度下,铝合金表面形成了致密粗糙的氧化层,且2.25A·dm-2电流密度下生成的氧化层比1.00A·dm-2下沟壑更深且宽,粗糙度更大.由图3(e,f,g,h)可看出,电流密度为8.00A·dm-2时制备的表面出现了10μm数量级的较宽沟壑,且在高倍下的表面形貌对比(图3(f,h))也显示出电流密度为8.00A·dm-2时比2.25A·dm-2时制备的表面沟壑密度更大,具有更大的粗糙度.采用AFM对不同阳极氧化电流密度下制备的氧化膜表面形貌及粗糙度进行分析,结果如图4所示,从图中可以看出随着电流密度增大,表面起伏增大,与FE-SEM照片的结果一致.随着阳极氧化电流密度的增大,表面形貌发生改变,表面粗糙度增大.表面形貌的变化直接影响了表面湿润性.接触角测试及表面形貌的结果表明,随着电流密度的增大,表面粗糙结构发生了变化.当阳极氧化电流密度小于2.00A·dm-2时,接触角随着电流密度的增加———即粗糙度的增加———而增大,水滴在其表面呈Wenzel态[26,27],cosθr=r cosθ0(1)其中θ0为液滴与光滑表面的杨氏接触角,θr为液滴与真实粗糙表面的表观接触角,r为试样真实表面积与表观面积的比值,且r>1.水滴在表面显示出大的接触角滞后,是由于表面的微观沟壑深度较浅,水滴由于自身重力进入到沟壑内,完全湿润了固体表面并形成了连续的固液接触线,在表面的钳制作用下,三相接触线在较小的能量下不能移动[28].当阳极氧化电流密度在2.00A·dm-2附近时,表面沟壑较深.由于水滴与试样表面接触时存在毛细作用,表面微观沟壑中存留有空气,所以其接触面为三相接触界面,即水滴在试样表面呈Cassie-Baxter态[29-31], cosθf=f SL cosθ0-1+f SL(2)其中,θf为液滴与真实粗糙表面的表观接触角,fSL为液滴固相接触面积占总投影面积的比例,fSL≤1.根据Cassie-Baxter方程,随着fSL的减小,表观接触角迅速增大,同时接触角滞后迅速减小,表面呈超疏水状态.当电流密度更大时,基体局部出现了较快的溶解,出现了更大更宽的孔隙,液滴因自身重力容易渗入孔隙中,从而减小了静态接触角,增大了接触角滞后.2.2自组装低表面能材料对表面湿润性的影响未经自组装G502的阳极氧化铝合金表面为亲水表面,与水滴的接触角几乎为0°(图2c),而阳极氧化铝合金表面经自组装G502膜层后可达到超疏水性能.因此,在粗糙表面自组装低表面能的硅氧烷是获得超疏水性能的关键之一.本节中首先对阳极氧化铝合金表面的自组装G502膜层进行了表征,随后讨论了自组装溶液的含水量和自组装时间对湿润性能的影响.为了保证自组装前表面粗糙结构的一致性,阳极氧化电流密度均控制为2.25A·dm-2,阳极氧化的其他参数不变.2.2.1自组装膜层的结构及组成的表征阳极氧化铝合金表面自组装膜层采用FT-IR谱及EDS表征.由FT-IR谱(图5)可知,阳极氧化铝合金经自组装十二氟庚基丙基三甲氧基硅烷(G502)得到的超疏水表面在1249cm-1的吸收峰为—CF2和—CF3基团中C—F键的伸缩振动峰,在2854cm-1的吸收峰为C—H键的伸缩振动吸收峰[32],说明自2585Acta Phys.-Chim.Sin.,2009Vol.25组装G502在氧化铝膜表面含有大量—CF3和—CF2以及—CH2等疏水基团,这些向外伸展的疏水基团是构成低自由能表面的关键.G502发生水解和缩聚反应,生成聚硅氧烷,即形成Si—O—Si键,但由于其吸收峰的位置(约为1100cm-1)与氧化铝的红外光谱位置相近,且测试样品中自组装膜含量小,Si—O—Si键的伸缩振动峰并不明显[24,33].超疏水表面的EDS分析(图6)说明表面存在C、O、F、Al和Si等元素,进一步证实了在阳极氧化铝合金表面经G502处理后存在聚硅氧烷分子层.铝合金表面的聚硅氧烷膜层不仅降低了表面自由能,而且由于硅氧烷在氧化铝合金表面的生长方式不同从而形成了具有纳米等级的粗糙结构.铝合金在2.25A·dm-2电流密度下阳极氧化后自组装G502前后的表面形貌如图7所示.无论是否存在自组装膜层,其表面微米级尺度上均存在形貌相近的沟壑,构成了微米等级的粗糙结构.图7(a,b)中局部放大照片的对比显示,自组装G502后的表面在微米等级的“平台”上存在纳米等级的粗糙结构;未经自组装G502的表面“平台”相对光滑,不存在纳米等级的粗糙结构.AFM对微区的表面形貌分析(图4)表明,表面自组装膜层构成了纳米级结构.阳极氧化铝合金微米级粗糙表面的自组装膜层形成的纳米级结构主要是由于G502在氧化物表面存在多种反应机制.含有大量—CF3、—CF2、—CH2等疏水基团的G502经水解后,生成具有三个—OH 活性基团的硅醇(—Si—OH),与阳极氧化铝合金表面富集的—OH基团(—Al—OH)反应脱去H2O分子,通过共价键与基体键合(—Al—O—Si—),含氟的长碳链则向外伸展.G502在溶液中水解后,在富含活性基团的氧化铝合金表面可能发生多种类型的反应,如存在残留—Si—OH的小分子聚合物与基体表面的键合,—Si—OH间的横向缩合或纵向缩合形成接枝聚硅氧烷[34],见图8,这些互相竞争的反应导致了分子膜层的无序性,从而构成了独特的纳米级结构[33,35].这些纳米级结构与阳极氧化构成的微米级粗糙结构共同构成了微米-纳米分等级的粗糙结构,从而使超疏水性能更稳定[23,36],即当液滴由Cassie-Baxter态转变为Wenzel态时需要越过更高的能垒.图5铝合金表面阳极氧化膜经G502自组装前后的FT-IR谱Fig.5FT-IR spectra of anodic oxide films ofaluminum alloy before and after G502self-assembly图7阳极氧化铝合金表面自组装G502前(a)后(b)的FE-SEM照片Fig.7FE-SEM images of the surface of anodizedaluminum alloy before(a)and after(b)G502self-assembly图6阳极氧化铝合金表面自组装G502后的能谱Fig.6EDS spectrum of the surface of anodizedaluminum alloy after G502self-assembly2586No.12李松梅等:铝合金表面原位自组装超疏水膜层的制备及耐蚀性能G502在富含—OH的活性表面自组装反应的机制对溶液中水的含量、反应时间、温度、表面活性基团数量等因素很敏感,所以控制反应条件对超疏水表面的制备非常重要[37].本文选取了硅氧烷溶液的含水量及自组装时间作为控制条件,详细讨论了自组装硅氧烷对表面疏水性能的影响.2.2.2自组装溶液的含水量对表面疏水性能的影响经阳极氧化后的试样浸入含水量不同的G502溶液自组装4h得到的表面与水滴的静态接触角关系曲线见图9.当G502的甲醇溶液中不含水时,只有很少量的硅氧烷在甲醇溶液中水解并键合到试样表面,使得低表面能的硅氧烷在试样表面的覆盖率很低,θS=16.9°±5.1°,表面呈亲水性.当每100mL溶液中水的含量小于约3.7mol时,静态接触角随着溶液中水的含量增加而增大;这是由于随着水含量的增加,硅氧烷在溶液中的水解愈加充分,增大了硅氧烷在试样表面的覆盖率,从而增大了疏水性.当每100mL溶液中水的含量约为3.7mol时,试样表面呈超疏水性,静态接触角最大达157.5°±2.0°.当每100mL溶液中水的含量约大于5.3mol时,静态接触角迅速减小,使表面呈弱亲水性,这可能是由于两方面原因:(1)硅氧烷在水中的溶解度很小,在溶液中无法通过扩散与试样表面键合,导致硅氧烷在试样表面覆盖率很低,影响了表面疏水性;(2)由于大量水的存在,硅氧烷水解迅速且容易发生自缩合,从而消耗了大量的—OH活性基团,导致其缺乏活性基团而无法键合到试样表面,降低了疏水性能.从图9中分析得到,每100mL硅氧烷溶液中水的含量在3-5mol的区间内,硅氧烷既可以在老化过程中充分水解,又不影响硅氧烷在自组装过程中的扩散和与表面的键合,得到的膜层疏水性能最佳.2.2.3自组装时间对表面疏水性能的影响经阳极氧化后的试样浸入到G502溶液中,每100mL自组装溶液的含水量为3.7mol,自组装时间分别设置为10min及0.5、1、1.5、2、4、6、8、120h.水滴在表面的静态接触角与自组装时间关系曲线,如图10所示.自组装10min的试样,接触角几乎为0°.当自组装时间较短时(<1.5h),接触角随着自组装时间的增加而缓慢增大.认为水解后的硅氧烷只在试样表面很小的面积上发生键合,而没有完全覆盖试样表面.根据硅氧烷在—OH活性表面的生长动力学,其首先在表面形成较小的岛状聚硅氧烷,覆盖图9水滴在试样表面的静态接触角与G502溶液(100mL0.01mol·L-1)中的含水量关系曲线Fig.9Relation curve of static contact angles of water droplets on the surfaces and the quantities of water in 100mL0.01mol·L-1G502solution图10水滴在试样表面的静态接触角与自组装时间的关系曲线Fig.10Relationship curve of static contact angles of water droplets on the surfaces and immersion time图8G502与试样表面键合示意图Fig.8Scheme graph of the surface bonding betweenG502and the sample2587Acta Phys.-Chim.Sin.,2009Vol.25率很小,随着时间的延长,岛状聚硅氧烷的生长增大了覆盖率,表现为疏水性的提高[38,39].在自组装1.5-2h的区间内,接触角急剧增大,表面由亲水性转变为疏水性,这是由于在这一区间内出现了完全覆盖的临界值,越过这一临界值,接触角即迅速增大.当自组装时间为4h,θS=157.5°±2.0°,表面呈超疏水性.当自组装时间大于4h所制备的表面与水的静态接触角缓慢下降.另外,当自组装时间延长至120h时,θS=103.6°±5.5°,与水滴在平滑聚硅氧烷表面的接触角(110.2°±1.0°)相近,可能是由于硅氧烷在表面长时间均匀生长,填补了表面微米级的起伏,使表面趋于平滑,失去了构成三相界面的孔隙,导致表面呈弱疏水性.由以上分析可以看出,经阳极氧化后的试样浸入到硅氧烷溶液中自组装4h得到的表面疏水性能最佳.2.3耐蚀性能测试利用动电位极化的方法对比了经工艺优化后制备的超疏水膜层、阳极氧化后的铝合金试样和经前处理后的铝合金试样的耐电化学腐蚀性能.其中,制备阳极氧化铝合金试样所采用的阳极氧化电流密度为2.25A·dm-2,制备超疏水膜层试样所采用的工艺参数为阳极氧化电流密度2.25A·dm-2,每100mL自组装溶液含水量为3-5mol,自组装时间为4h.图11为超疏水膜层、阳极氧化铝合金以及经前处理后的铝合金的动电位极化曲线.由图11以及试样的自腐蚀电位(Ecorr)和通过Tafel直线外推法计算得到的腐蚀电流密度(icorr)(见表3)可以得到,超疏水膜层Ecorr 为-0.53V,而只经前处理后的铝合金Ecorr为-0.75V,超疏水膜层Ecorr正移大于0.2V;同时,表面为超疏水膜层的铝合金与只经前处理后的铝合金相比,icorr下降了约2-3个数量级.Ecorr和icorr的结果表明,超疏水膜层的极化曲线的阳极分支和阴极分支都向电位正的方向移动,且腐蚀电流也保持在一个很低的数值(5.6×10-9A·cm-2),有效地提高了铝合金基体的耐蚀性.只经阳极氧化处理而表面未自组装硅氧烷的铝合金Ecorr为-0.64V,与只经前处理后的铝合金(-0.75V)和超疏水膜层(-0.53V)相比,说明铝合金表面原位生长的阳极氧化膜不仅为构造超疏水性能提供了粗糙结构,而且在一定程度上提高了铝合金的耐蚀性.这是由于在NaCl水溶液中阳极氧化膜层虽然起到了一定阻挡层的作用,但Cl-易渗入多孔的氧化铝膜中,限制了氧化膜层的耐蚀作用[40],而在原位生长的阳极氧化膜上自组装的超疏水膜层有效地防止了溶液中Cl-的渗入,极大地提高了铝合金的耐蚀性.另外,由图10中三种试样极化曲线的阴极极化区的形状及电流密度可以看出,只经前处理和经阳极氧化的铝合金试样几乎一致,而超疏水膜层阴极极化区电流密度非常小,说明在阴极极化区超疏水膜层很好地保护了铝合金基体.3结论(1)讨论了阳极氧化电流密度和自组装溶液中水的含量及自组装时间对表面疏水性的影响,并得到了制备超疏水表面的最佳工艺参数,即阳极氧化电流密度为2.25A·dm-2,每100mL自组装溶液含水量为3-5mol,自组装时间为4h.(2)通过阳极氧化在铝合金表面原位构造微米尺度的粗糙结构,协同表面自组装低表面能的聚硅氧烷膜层制备了静态接触角θS=157.5°±2.0°,且接触角滞后小于3°的超疏水自清洁表面.(3)FE-SEM及AFM测试结果表明,由自组装表3经前处理后的铝合金、阳极氧化后的铝合金和铝合金表面超疏水膜层在3.5%(w)NaCl水溶液中的自腐蚀电位及腐蚀电流密度Table3Corrosion potential(E corr)and corrosioncurrent density(i corr)of pre-treated aluminum alloy,anodized aluminum alloy and super-hydrophobic filmon aluminum alloy in3.5%(w)NaCl aqueous solutions图11经前处理后的铝合金(a)、阳极氧化后的铝合金(b)和铝合金表面超疏水膜层(c)在3.5%(w)NaCl水溶液中的动电位极化曲线Fig.11Potentiodynamic polarization curves of pre-treated aluminum alloy(a),anodized aluminum alloy (b)and super-hydrophobic film on aluminum alloy(c)in3.5%(w)NaCl aqueous solutionsSample E corr/mV(vs SCE)i corr/(A·cm-2) pre-treated aluminum alloy-0.75 2.1×10-6 anodized aluminum alloy-0.64 1.0×10-6 super-hydrophobic film-0.53 5.6×10-92588。
浅海遥感水深反演研究及其软件设计与实现
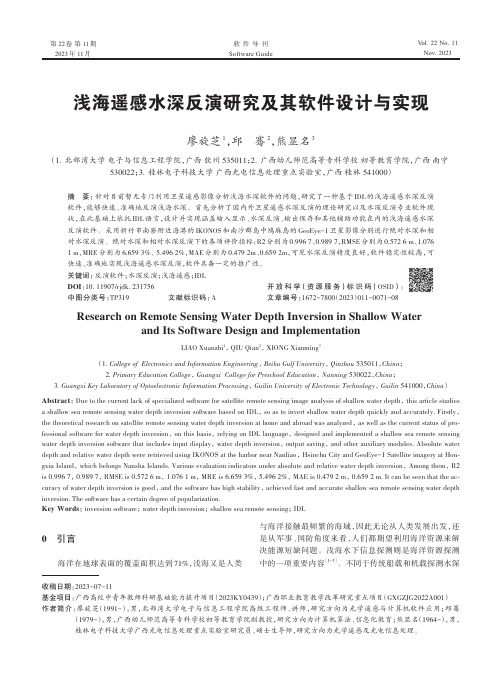
第 22卷第 11期2023年 11月Vol.22 No.11Nov.2023软件导刊Software Guide浅海遥感水深反演研究及其软件设计与实现廖旋芝1,邱骞2,熊显名3(1.北部湾大学电子与信息工程学院,广西钦州 535011;2.广西幼儿师范高等专科学校初等教育学院,广西南宁530022;3.桂林电子科技大学广西光电信息处理重点实验室,广西桂林 541000)摘要:针对目前暂无专门利用卫星遥感影像分析浅海水深软件的问题,研究了一种基于IDL的浅海遥感水深反演软件,能够快速、准确地反演浅海水深。
首先分析了国内外卫星遥感水深反演的理论研究以及水深反演专业软件现状,在此基础上依托IDL语言,设计并实现涵盖输入显示、水深反演、输出保存和其他辅助功能在内的浅海遥感水深反演软件。
采用新竹市南寮附近海港的IKONOS和南沙群岛中鸿庥岛的GeoEye-1卫星影像分别进行绝对水深和相对水深反演。
绝对水深和相对水深反演下的各项评价指标:R2分别为0.996 7、0.989 7,RMSE分别为0.572 6 m、1.0761 m,MRE分别为6.659 3%、 5.496 2%,MAE分别为0.479 2m、0.659 2m,可见水深反演精度良好,软件稳定性较高,可快速、准确地实现浅海遥感水深反演,软件具备一定的推广性。
关键词:反演软件;水深反演;浅海遥感;IDLDOI:10.11907/rjdk.231756开放科学(资源服务)标识码(OSID):中图分类号:TP319 文献标识码:A文章编号:1672-7800(2023)011-0071-08Research on Remote Sensing Water Depth Inversion in Shallow Waterand Its Software Design and ImplementationLIAO Xuanzhi1, QIU Qian2, XIONG Xianming3(1.College of Electronics and Information Engineering, Beibu Gulf University, Qinzhou 535011,China;2.Primary Education College, Guangxi College for Preschool Education, Nanning 530022,China;3.Guangxi Key Laboratory of Optoelectronic Information Processing, Guilin University of Electronic Technology, Guilin 541000,China)Abstract:Due to the current lack of specialized software for satellite remote sensing image analysis of shallow water depth, this article studies a shallow sea remote sensing water depth inversion software based on IDL, so as to invert shallow water depth quickly and accurately. Firstly,the theoretical research on satellite remote sensing water depth inversion at home and abroad was analyzed, as well as the current status of pro‑fessional software for water depth inversion, on this basis, relying on IDL language, designed and implemented a shallow sea remote sensing water depth inversion software that includes input display, water depth inversion, output saving, and other auxiliary modules. Absolute water depth and relative water depth were retrieved using IKONOS at the harbor near Nanliao, Hsinchu City and GeoEye-1 Satellite imagery at Hon‑gxiu Island, which belongs Nansha Islands. Various evaluation indicators under absolute and relative water depth inversion, Among them, R2 is 0.996 7, 0.989 7, RMSE is 0.572 6 m, 1.076 1 m, MRE is 6.659 3%, 5.496 2%, MAE is 0.479 2 m, 0.659 2 m. It can be seen that the ac‑curacy of water depth inversion is good, and the software has high stability, achieved fast and accurate shallow sea remote sensing water depth inversion. The software has a certain degree of popularization.Key Words:inversion software; water depth inversion; shallow sea remote sensing; IDL0 引言海洋在地球表面的覆盖面积达到71%,浅海又是人类与海洋接触最频繁的海域,因此无论从人类发展出发,还是从军事、国防角度来看,人们都期望利用海洋资源来解决能源短缺问题。
The Asymmetry of Galaxies Physical Morphology for Nearby and High Redshift Galaxies
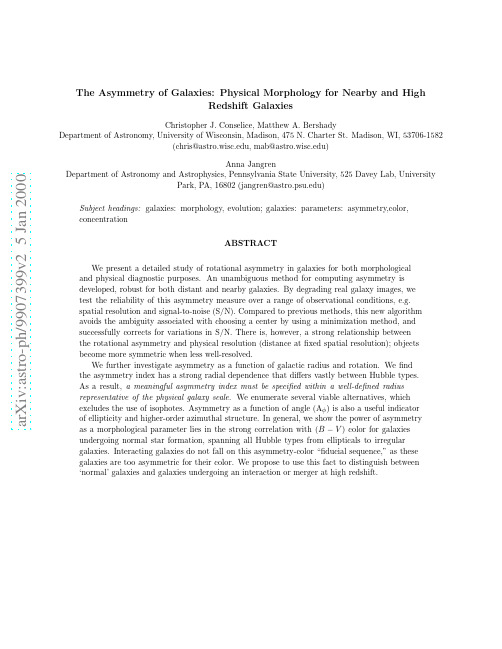
a r X i v :a s t r o -p h /9907399v 2 5 J a n 2000The Asymmetry of Galaxies:Physical Morphology for Nearby and HighRedshift GalaxiesChristopher J.Conselice,Matthew A.BershadyDepartment of Astronomy,University of Wisconsin,Madison,475N.Charter St.Madison,WI,53706-1582(chris@,mab@)Anna JangrenDepartment of Astronomy and Astrophysics,Pennsylvania State University,525Davey Lab,UniversityPark,PA,16802(jangren@)Subject headings:galaxies:morphology,evolution;galaxies:parameters:asymmetry,color,concentrationABSTRACTWe present a detailed study of rotational asymmetry in galaxies for both morphologicaland physical diagnostic purposes.An unambiguous method for computing asymmetry isdeveloped,robust for both distant and nearby galaxies.By degrading real galaxy images,we test the reliability of this asymmetry measure over a range of observational conditions,e.g.spatial resolution and signal-to-noise (S/N).Compared to previous methods,this new algorithm avoids the ambiguity associated with choosing a center by using a minimization method,and successfully corrects for variations in S/N.There is,however,a strong relationship betweenthe rotational asymmetry and physical resolution (distance at fixed spatial resolution);objects become more symmetric when less well-resolved.We further investigate asymmetry as a function of galactic radius and rotation.We findthe asymmetry index has a strong radial dependence that differs vastly between Hubble types.As a result,a meaningful asymmetry index must be specified within a well-defined radiusrepresentative of the physical galaxy scale.We enumerate several viable alternatives,whichexcludes the use of isophotes.Asymmetry as a function of angle (A φ)is also a useful indicator of ellipticity and higher-order azimuthal structure.In general,we show the power of asymmetry as a morphological parameter lies in the strong correlation with (B −V )color for galaxiesundergoing normal star formation,spanning all Hubble types from ellipticals to irregulargalaxies.Interacting galaxies do not fall on this asymmetry-color “fiducial sequence,”as these galaxies are too asymmetric for their color.We propose to use this fact to distinguish between ‘normal’galaxies and galaxies undergoing an interaction or merger at high redshift.1.Introduction1.1.Galaxy MorphologyEver since galaxies were recognized as distinct physical systems,one of the main goals in extragalactic astronomy has been to characterize their forms,or morphology,and to determine how this classification relates to physical properties.This basic taxonomical process is indeed the basis for any observational science.Thefirst attempts at classification were on a subjective level,and began with the work of Curtis (1918),Hubble(1926,1936),and Sandage(1961).As more images of galaxies became available,the morphological system developed by Hubble was generally adopted by all astronomers,and later refined by van den Bergh(1960a,1960b)and de Vaucoulers(1959).Other morphological systems were also developed by Morgan(1958)based on the correlation of the physical characteristics of galaxy spectra with the concentration of the light profiles.Yet since the time of Hubble’s1926work,the system of morphology for galaxies has changed little.When Hubble developed his original morphological system,his sample consisted of mostly nearby,luminous galaxies,with only3%“irregular”galaxies.These galaxies were not well incorporated in his sequence,but for his purposes,the morphological system developed was adequate for classifying97%of his sample.As deeper galaxy catalogues emerged,however,more and more galaxies fell into the catch-all,“irregular”morphological class.Today,Hubble Space Telescope(HST)imaging reveals that a large fraction of distant galaxies have morphologies that do notfit into the the elliptical-spiral Hubble sequence.The Hubble sequence also fails to be useful when classifying galaxies in clusters,with most galaxies classified as S0or E–classifications which fail to account of the wide range of cluster galaxy properties(e.g.Koopmann &Kinney1998).Spectral parameters are often more useful in these cases(e.g.Dressler&Gunn1992). Today we classify irregular galaxies not simply in a morphological system,but with regard to the physical mechanisms in operation or the salient physical conditions(e.g.starburst galaxies,interacting galaxies, gas-rich and gas-poor).A morphological classification which reflects these physical differences would be a powerful tool for studying the mechanisms driving galaxy evolution.Such studies naturally must include high redshift galaxies.Therefore,a morphological system that encompasses all galaxies,and works sensibly over a wide range in redshift is absolutely essential,but at present does not exist.Recently,new methods of classifying galaxies have been proposed.One line of effort has been to train artificial neural networks to reproduce the Hubble scheme in an objective way(Burda&Feitzinger1992, Storrie-Lombardi et al.1992,Serra-Ricart et al.1993,Odewahn1995,Naim et al.1995,Odewahn et al. 1996).Spiekermann’s(1992)approach using fuzzy logic was along this line.The number of galaxy images in modern surveys,such as the“Sloan Digital Sky Survey”will be enormous,and hence such automatable methods of morphological classification are desirable.However,a Hubble classification carries with it the limitations mentioned above;a system that can classify galaxies in a straightforward and quantitative manner that is based on a sound physical and morphological basis would be preferable.Another approach to galaxy classification has been to develop sets of quantitative measures of the bulk image structures of galaxies.These methods have the potential to either replace,modify,or improve the current Hubble scheme.The new classifications generally rely on a set of photometric and/or spectral properties that are internally correlated,and correspond also with the apparent morphology of galaxies. Morgan’s(1958,1959)use of central light concentration was thefirst example of such a classification. Indeed,the use of concentration indices for inferring the morphology of galaxies has continued to improve, and along with surface-brightness and asymmetry has become one of the major tools for classifying both nearby and distant galaxies(e.g.Okamura,Kodaira,&Watanabe1984;Doi et al.1993;Abraham et al.1994;Jangren et al.1999).A different method–applicable for spirals–has been suggested by Elmegreen &Elmegreen(1982):measures of spiral arm morphology,particularly their patchiness,can be used for classification.Related attempts to classify galaxies have included the use of principle component analysis of photometric structures(Whitmore1984;Watanabe et al.1985;Han1995).These systems revealed correlations of physical and morphological features of galaxies,but have not been generally adopted for practical use,and the basic Hubble(1926)system still lives on.A key element missing from recent work listed above is the connection made by Morgan between image structure and stellar content(i.e.between light concentration,or central surface-brightness,and spectral type).Ironically,in parallel to the above efforts to quantify image structure,there has been considerable effort to develop quantitative methods of spectral classification based on broad-band colors(Bershady1995) and spectra(Connolly et al.1995,Zaritsky et al.1995,Folkes et al.1996,Bromley et al.1998,Ronenet al.1999).What is needed,then,is to go full circle to where Morgan left off,by tying together the spectral types with the quantitative classification based image structure.Here,we propose that using a measure of asymmetry and color for galaxies is a powerful method towards accomplishing this goal.In an accompanying paper(Jangren et al.1999)we explore the additional parameters of size,surface-brightness and image concentration.1.2.Asymmetry as a Physical ClassifierSymmetry has always been one the most basic features and assumptions of most galaxy morphology systems,but also one of the most overlooked for more detailed study.The earliest galaxy morphology papers by Curtis(1918)and Hubble(1926)described galaxies in terms of their symmetry,in most cases a 180◦symmetry.Hubble(1926)describes elliptical and spirals galaxies in their most basic terms as systems “characterized by rotational symmetry about dominating nuclei”.In fact,it is striking how symmetric galaxy systems are,and as we will show,almost always have a minimum asymmetry at a180◦rotation angle.Models of galaxies often assume that the mass distribution of a galaxy is symmetric.Galaxies are, tofirst order,dynamically relaxed systems.Understanding how and in what manner the distribution of galaxy light is asymmetric can help reveal dynamical processes in galaxies.For example,galaxies disturbed by interactions or mergers will tend to have large asymmetries.For quite some time there has been considerable effort to characterize the asymmetry in HI gas in spiral galaxies(e.g.Baldwin et al.1980; Richter&Sancisi1994);attempts to do this in optical light are relatively recent(e.g.Rix&Zaritsky 1995;Kornreich,Haynes,&Lovelace1998;Rudnick&Rix1998).While HI-studies benefit directly from kinematic data,optical studies offer complementary information since optical photons predominantly come from stars,which are collisionless,and are believed to trace well the underlying matter distribution in the disk.The quantitative use of asymmetry as a morphological parameter was usedfirst by Schade et al.(1995) as a characterization of distant galaxies observed with the Hubble Space Telescope(HST).Further use of symmetry for galaxies in deep HST images has been carried out by Abraham et al.(1996a,1996b)and van den Bergh et al.(1996).These papers,however,use asymmetry only as a crude,type-characterization of distant galaxies in the framework of the Hubble Sequence.Attempts to characterize asymmetry for nearby galaxies,and its usefulness as a morphological parameter within existing frameworks wasfirst carried out by Conselice(1997;hereafter C97).In C97it was shown that asymmetry increased with Hubble Type,but with a large spread.Potentially more important was the strong correlation found between color and asymmetry,and a lack of a strong correlation between luminosity and asymmetry for the narrow absolute magnitude of the C97sample.In this paper we investigate further the relationship between asymmetry and other physical parameters, such as color and luminosity,and the usefulness of asymmetry as a morphological parameter.From these results,our expectations are that asymmetry can be incorporated as a pillar of a new classification system which better describes and correlates with physical features and parameters than the Hubble sequence.The paper proceeds as follows.The calibration data set of local galaxies used for this study is described in the next section.In§3,we then compare different methods for computing asymmetry,and propose a new procedure for measuring asymmetry as a robust quantity.This method includes an iterative scheme forfinding the center of rotation(§3.3),a noise correction(§3.4),and a well-defined radius of extraction. We demonstrate how asymmetry changes as a function of both the rotation angle used to compute it(§4.1), and as a function of galactic radius(§4.2).We also discuss the correlation between asymmetry and other physical parameters,i.e.the(B−V)color of a galaxy(§4.3),the Hubble type,and the concentration of light(§4.4).Resolution effects are considered in§5.1.In§4.3we also discuss the causes of asymmetry, concluding that asymmetries are produced by either star formation in spiral arms,or dynamical effects related to interactions with other galaxies.We show these two causes can be distinguished by examining their position in a color-asymmetry diagram.These results are directly applicable to distant galaxies with resolved structure,such as those in the Hubble Deep Field(HDF).2.Calibration Data SetThe data used in this present study are the full set of113galaxies in the Frei et al.(1996)catalog (hereafter referred to as the Frei sample).In contrast,C97was limited to face-on spirals,or ellipticals from the same sample;inclined systems,irregulars and active galaxies were left out.2.1.PhotometryThe current sample under study consists of82galaxies imaged in the B J and R bands(Gullixon et al. 1995),and31in the Gunn g,r,and i bands.The scale for the B J and R images is1.34”per pixel with a field of view of7’x11’;for the g,r,and i images the scale was1.19”pixel−1with afield of view of16’x16’. The g,r,and i images have relatively lower S/N than the B J and R images because of substantially shorter exposures times.In addition to the usual processing(bias subtraction andflat-fielding),Frei et al.removed foreground stars.Occasionally,a star that is projected on a bright part of the nucleus was not removed, and causes a false high value for the asymmetry number for those galaxies.For consistency between the above photometric subsets,we use the B−V colors listed in the Third Reference Catalogue of Bright Galaxies(de Vaucouleurs et al.1991,RC3)for purely photometric purposes. (Structural parameters are computed from the images described above.)The adopted B−V colors are observed,i.e.uncorrected for Galactic and internal extinction,and heliocentric velocity(the k-correction). These corrections are small and hence have no qualitative impact on the conclusions of this paper.We adopt the distances and B absolute magnitudes given by Tully(1988).We note,however,that these M B include the above corrections.(A more consistant treatment is presented in Jangren et al.1999.)Theadopted values of M B,B−V,and distance are listed in Tables1and2.2.2.Sample CharacteristicsThe Frei sample includes Hubble types from early ellipticals and S0s,to late-type spirals,irregulars and galaxies with peculiar features.Several of the galaxies in the sample contain features such as rings and bars. Most of the galaxies in the sample are nearby,with a large portion of the ellipticals coming from the Virgo cluster.All of the galaxies were chosen away from the galactic equator and are generally near the Northern Galactic Cap.However,the Frei sample consists only of bright,high surface brightness galaxies.Hence,we are not considering the large population of LSB or dwarf galaxies that make up the bulk of all galaxies in the universe.It is important to realize that the Frei sample is not likely to be an accurate representation of the entire local galaxy population,but only samples a range of Hubble types for the brightest,nearby galaxies.For illustrative purposes,in the remainder of this paper we have selected21galaxiesthat are representative examples of total sample of113used in this study.These21galaxies span of T-types,inclinations and colors.The remaining sample can be viewed at/∼frei/galaxy.3.The Asymmetry AlgorithmThe asymmetry Aφ,whereφis the angle of rotation,is a quantitative parameter based on comparing a source image with its rotated counterpart.In principle Aφis straight-forward and quick to measure in its simplest form.We compare several different methods,including those presented by Abraham et al.(1996a; hereafter A96)and C97in the context of aφ=180◦rotation,to determine if they are robust morphological measures over a range of astronomical conditions.On this basis,we develop a more sophisticated approach, ultimately based on the algorithm of A96.3.1.Image PreparationBefore a rotational asymmetry measurement can be made,basic digital image processing must be completed(i.e.bias subtraction,fieldflattening,fringe correction,cosmic-ray removal,and bad pixel interpolation).In addition,any objects in the galaxy’s image which are not part of the galaxy,such as foreground stars,or foreground and background galaxies must be removed by blanking the contaminated portion of the images,or subtracting the contaminating source.Contaminating sources tend to be at random locations with respect to the primary source,and hence residuals from this decontamination process will add to the asymmetry depending on their amplitude relative to the source brightness.In the case of unresolved contaminating sources,e.g.stars,the removal is straightforward.Foreground and background galaxies are more difficult to remove or exclude both because of their more complex and extended image structure,and because their identification as separate from the primary source can require further information and judgment.This complication becomes particularly important in ultra-deep images such as the HDF,but we do not pursue this further here as it is not an issue for the current study.In addition to any foreground stellar images,the mean background level for each image must becarefully subtracted.This will become clear in the next section where we define the specific computational algorithm.A significant non-zero background level can radically alter the measured asymmetry value.After a successful background subtraction and object decontamination,however,it is then possible to perform the asymmetry measurement without further image calibration or processing.3.2.Methods of ComputationThe rotational asymmetry measurement procedure consists of(1)defining an image center and “extraction”region;(2)rotating the image about this center by angleφ,and(3)performing a comparison of the resultant rotated image with the original image,within the extracted region.This comparison,however, can have several mathematical forms.In published studies(e.g.C97,A96),the comparison is made by subtracting the rotated image from the original,although in principle the product or ratio might be useful. We have found that only subtraction appears to work well,and discuss only this possible algorithm further. The details of the centering,and choice of rotation angle(§3.3and§4.1respectively)are critical,but do not depend on the mathematical form of the asymmetry algorithm.Once the rotated image is subtracted from the original image,we then have a residual image of all asymmetric features of the galaxy.The asymmetry measurement is completed by measuring the amplitude of the pixel values in the residual image,and normalizing this amplitude by a corresponding measure in the original image.Here again,several mathematical possibilities exist.The method used in C97consists of summing the square of the pixels in the residual image,and normalizing this value by dividing by the sum of the square of the pixels in the original image.Since the pixel values in the residual image will be,on average,half negative and half positive pixel values,half the above ratio gives gives the asymmetry number for that galaxy:Σ(I o−Iφ)2A2rms=.2Σ|I o|Again,the sum is over all pixels within a prescribed,matching region of the original and rotated images. We will call this procedure the“ABS”asymmetry method.For both methods the lowest possible value for the asymmetry parameter is0,while the highest is1.A value of0corresponds to a galaxy that is completely symmetric,that is the difference(I o−Iφ)=0at all points in the difference image formed from subtracting the rotated image from the original image.A values of1corresponds to a galaxy that is completely asymmetric such that on average|(I o−Iφ)|=I o.Values for most galaxies lie between these two extremes(see Tables1,2,3,and4forφ=90◦and180◦).Comparing the above two asymmetry computations forφ=180◦rotations,wefind that the values for A abs are similar to the the values found for A rms,as measured in the red.The A abs values have slightlylarger asymmetries at high A as can be seen in Figure1from the positive value of A abs-A rms at large A rms. As a function of wavelength,the RMS asymmetries show a clear trend towards higher differences in A(blue) -A(red)at larger(red)asymmetries,as seen in Figure2;this can be seen to a lesser extent for the ABS method in Figure3.(Here,‘blue’corresponds either to the B or g bands,and‘red’corresponds to either R or r bands.)The average difference in A(blue)-A(red)for the RMS method is0.015overall,and0.05at A(red)>0.1.The ABS method yields asymmetries that are fairly constant between bands with an average A(blue)-A(red)of0.005over all asymmetry values.However,wefind the ABS method gives a tighter correlation between asymmetry and color than the RMS method,contrary to our expectations.The RMS method was expected to be a better indicator of star formation since it weights higher the brighter,asymmetric features.The brightness of a star forming region in a galaxy is a function of the density,squared,and hence the RMS method should better trace higher contribution from denser regions of star formation.While either method can be used to get physical information,the better correlation of ABS asymmetries with color lead us to use the ABS method for the remainder of the paper.3.3.Centering CorrectionsOne of the most crucial aspects of the rotational asymmetry computation is the choice of a center of rotation.Centers that differ by only a small amount(relative to a galaxy’s characteristic size)typically produce substantially different asymmetry values.For example,a change in the center of rotation by just one pixel for the Frei et al.sample(roughly1%of a half-light radius)can change the value of the asymmetry by as much as50%.However,this becomes less of a problem when the scale of the galaxy becomes smaller, such that as the sampling decreases so does the need for precise centering.To overcome this centering problem,we define the center of rotation to be the position which yields a minimum value for theφ=180◦asymmetry.Tofind such a center in practice,an initial guess is made for a galaxy’s rotational center.Thisfirst step can be automated by choosing the mean,or mode of the light distribution as a reasonable initial guess;our tests indicate that the initial guess does not alter the final asymmetry.In most cases,there is a clear central pixel which is approximately in the center of the galaxy,for example,the brightest pixel in the galaxy.For irregular and edge-on galaxies however,there is not a clear-cut brightest point,or even a well defined center,and it is for these galaxies that this method of minimum center is most effective.Operationally,after the asymmetry is computed at that initial position,the asymmetry is computed again for centers at the surrounding eight points in a3×3grid.The distance from the central point to the eight surrounding points can be set at any value.In this work,we use a distance of0.1pixels,corresponding to roughly0.1%of the half-light radius.We use the task’rotate’in IRAF to perfom the asymmetry measurements via bi-linear interpolation.If the asymmetry parameter at the center is lower than the asymmetry value at any of the eight surrounding points,then the asymmetry parameter is taken to be the value at the center.The algorithm effectively creates a synthetic grid of asymmetry values arranged by x,y center positions.If the center pixel does not give the asymmetry minimum,as is usually the case,then the procedure repeats,with the new center the pixel value where the minimum was located.This process continues until a minimum is found for the asymmetry.Once this location is found,we define this to be the ‘asymmetry centroid’and use that computed asymmetry for the asymmetry parameter of the galaxy.One possible problem with this method offinding the minimum asymmetry is the existence of localasymmetry minima.We have tested this by computing the asymmetry parameter over a wide range of centers for a set of21representative galaxies from the Frei sample.The second columns of Figures4,5, 6,and7are pictorial representations of the asymmetries values at all pixel locations about the inner3x3 arcsec of these21galaxies.It can be seen in these images that no significant local minima exist throughout the image.While the detailed shape of these‘asymmetry planes’differ from galaxy to galaxy(and indeed, contain considerable information about the light distribution)a well-defined minimum exists in each case. In the presence of sufficient noise,this condition will break down.We characterize this behavior via simulations in the following section.3.4.Noise CorrectionsThe rotational asymmetry,as defined here,is by its very nature a pixel-by-pixel difference algorithm, and hence can be substantially affected by noise.Clearly this effect must be accounted for if asymmetry is to be a robust classifier.An example of the effects of noise on the asymmetry value of a galaxy is illustrated in Figure8.The effects of both correlated and uncorrelated noise are relevant.Here,however,we develop a correction for uncorrelated noise and defer handling of correlated noise to a later study on the Hubble Deep Field.The effects of uncorrelated noise in practice are easy to correct by simultaneously performing the asymmetry measurement on both the source and a neighboring,blank area of the image(see A96).The method for computing the asymmetry of the‘blank’area is the same as before,with one exception:there is no normalization by the sum of the original pixels,since in the case of the sky-subtracted background,the sum is zero on average.This procedure is then repeated in a‘centering’grid until a minimum of the noise is found,precisely the same way the minimum asymmetry of the object is found.This‘blank’asymmetry value is then subtracted from the value measured for the object,thereby correcting statistically for theeffects of uncorrelated noise present in the object image.Thefinal formula used to compute the asymmetrycan be written as:A abs=min[Σ|(I o−Iφ)|Σ|I o|],where I represents the image pixels,and B represents the blank region(background)pixels.Note that the possible range of asymmetry values now spans from0to2.However,due to the application of the minimization condition,the values are rarely ever greater than1,and this primarily occurs whenφ=90◦.When defining a blank region it is necessary to either use an extraction region the same size as the one used on the galaxy,or more practically,scale the sum of the blank region by the relative size of the object to‘blank region’areas.The extraction region used to define the background should be big enough to be representative,but should be small and distant enough from the galaxy so as to not include any diffuse light –and hence gradients–from the sources.Figure8shows the differences between the computed asymmetry of a galaxy with added noise and the original asymmetry as a function of S/N.It can be seen from this plot that the asymmetry differences becomes very large at lower S/N.To test how successful our algorithm of removing noise is at effectively reproducing the correct value for the asymmetry parameter,we artificially degraded the Frei et al.galaxy images by adding simulated, random noise.The noise-corrected asymmetry values measured in these images allowed us to assess the systematic behavior with S/N.We compute the S/N as ratio of the signal from the galaxy within the half-light radius to the noise contribution from the sky,source,and detector read-noise within the same aperture.Results of these S/N tests are shown in Figure9.We use35galaxies from our sample which spanall Hubble types and inclinations to perform these simulations.Wefind that for all these various galaxies, the value of asymmetry parameter found at lower S/N is,on average,still near the values found in the original images.Below S/N∼100,the noise begins to heavily dominate the asymmetry.In this regime,the rotation center yielding the minimum asymmetry(described in Section3.3)is determined largely by the noisefield. This is compensated in the noise correction since wefind the minimum again for the blankfield where the background correction is calculated.If we did not re-center on the blank region whenfinding the background-level asymmetry,this correction would get relatively larger than the galaxy’s asymmetry at successively lower S/N values.As a consequence,the corrected asymmetry would systematically become negative at low S/N values.In our current scheme,we avoid this pit-fall.Even for S/N<100,our simulations show that measured asymmetries have error bars that still overlap with the actual value, although the errors are very large.Errors for images with S/N>500are typically around0.02(rms),while at S/N between100and300have errors around0.05(rms).At S/N<100,the error on the asymmetry become very high,i.e.exceeding0.60(rms)for S/N<50.From Figure9however,we conclude that for all galaxies with S/N values>100their asymmetries can be computed reliably,which we define to be when the rms errors are less than0.05.A feature of our asymmetry algorithm,which comes naturally from the noise corrections,is an ability to estimate an error on the computed,corrected asymmetries.We have tested this via Monte Carlo methods andfind that these estimates are accurate.14.ResultsThe following presentation is based on the results in Tables1and2,which list the asymmetry values and their estimated errors for the113galaxies in the Lowell and Palomar sample forφ=180◦and90◦, computed within the radius r(η=0.2),and calculated as described in the previous section with centering and noise corrections.The extraction aperture based on theη-function is described in section§4.2.4.1.Asymmetry as a function ofφRotational asymmetry,as we have defined it,has heretofore been explored only in the context of 180◦rotation.However,rotation by other angles can potentially yield more physical and morphological information.In particular,the azimuthal variations of galaxy light profiles can be probed,akin to the seminal work of Schweizer(1976a,1976b).While it is not our intent to pursue such a detailed study here, we do show that other angles of rotation can provide diagnostics which allow us to improve upon the utility of the180◦asymmetry correlation with color.4.1.1.180◦Rotation:flocculent and dynamical asymmetriesThe rotation of a galaxy by180◦should yield the minimum rotational asymmetry since most galaxies appear to have a strong azimuthal axi-symmetry.As such,A180is expected to be sensitive both to either。
高一科学探索英语阅读理解25题
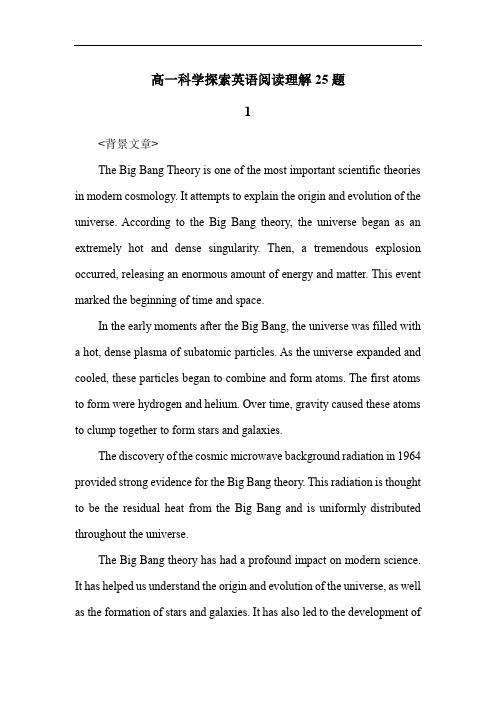
高一科学探索英语阅读理解25题1<背景文章>The Big Bang Theory is one of the most important scientific theories in modern cosmology. It attempts to explain the origin and evolution of the universe. According to the Big Bang theory, the universe began as an extremely hot and dense singularity. Then, a tremendous explosion occurred, releasing an enormous amount of energy and matter. This event marked the beginning of time and space.In the early moments after the Big Bang, the universe was filled with a hot, dense plasma of subatomic particles. As the universe expanded and cooled, these particles began to combine and form atoms. The first atoms to form were hydrogen and helium. Over time, gravity caused these atoms to clump together to form stars and galaxies.The discovery of the cosmic microwave background radiation in 1964 provided strong evidence for the Big Bang theory. This radiation is thought to be the residual heat from the Big Bang and is uniformly distributed throughout the universe.The Big Bang theory has had a profound impact on modern science. It has helped us understand the origin and evolution of the universe, as well as the formation of stars and galaxies. It has also led to the development ofnew technologies, such as telescopes and satellites, that have allowed us to study the universe in greater detail.1. According to the Big Bang theory, the universe began as ___.A. a cold and empty spaceB. an extremely hot and dense singularityC. a collection of stars and galaxiesD. a large cloud of gas and dust答案:B。
西格玛基础知识4课件.ppt
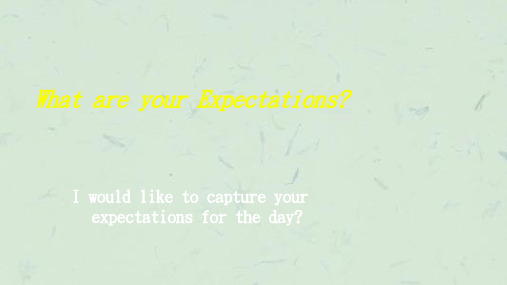
Our fundamental objective (Everyones overriding responsibility) Total customer satifaction
Our fundamental objective
Total customer satisfaction Key beilfes How we will always act Key goals What we must accomplish Key initiatives How we will do it
+ -
Virtually no defects (3.4 ppm)
Six sigma as a quality term
Six Sigma = 3.4 ppm defective
Six sigma is a way to change an organizations culture
Provides a means of delivering Total customer satisfaction Focuses everyone in the organization on the same objective
There is no such thing as “We’re different”
WE’RE DIFFERENT
Functions in a typical organization
Accounting Advertising Distribution Energy management Environmental affairs External affairs Facilities Finance Health & safety Human resources legal
Kongsberg TOPAS PS18参数子底探测器产品说明书

TOPAS PS18 is designed for sub-bottom profiling with very high spatial resolution in water depths from less than 20 metres to full ocean depth. The +80% relative bandwidth, low frequency signal is generated in the water column by non-linear interaction between two high frequency signals (centred symmetrically around 18 kHz). Similarly, a sum frequency signal is also generated. However, only the low frequencysignal is used for sub-bottom profiling.PARAMETRIC SUB-BOTTOM PROFILERSystem specificationsThe Kongsberg TOPAS PS 18 parametric sub-bottom profiler comprises the following units:• Transducer-8 x 16 channels• Transceiver Unit-Linear, switched mode power amplifier-Built-in T/R-switch-Low noise receiver with 24 bit ADC-High dynamic range; >110 dB• Operator Console-MMI-Real-time processing-PC-based platform (Windows)• Optional-Multi-channel receiver (MCR) and beamformerThe parametric sources have the advantage of generating a low frequency signal beam with no distinct sidelobe structure. The beam tapers off smoothly, reducing the possibility of spurious signals due to sidelobes in the received signal. The transducer is hull mounted. The advantages are no deploy-ment or recovery of tow-fishes during the survey, which results in more efficient survey time and higher positioning accuracy for the profile. Manoeuvrability of the vessel is also improved.In a “low noise” vessel, excellent profiling results are achieved even at survey speeds of +14 knots.The system can operate with various signal waveforms for optimum performance: Typically Ricker pulses are used for very high resolution work, Chirp pulses are used for deep water, high penetration work and CW pulses are used for narrow band, frequency sensitive work.The transmitted beam is electronically stabilised in both roll, pitch and heave (requires input from a vertical reference unit) ensuring that the insonified area on the sea floor is accurately positioned. The transmitter can be used in a sequentially beam steering mode covering a larger sector. This is of importance in object detection/ location applications. Penetration performance depends on sediment characteristics, water depth, transmitted signature, noise level etc. Penetration of more than 200 metres has been achieved in water depthsof more than 3,800 metres with a sediment layer resolution of typically 15 cm or better.July 2019TYPICAL SPECIFICATION Primary frequency 15 – 21 kHz Secondary frequency 0.5 – 6.0 kHz Output power >32 kW Beamwidth -Primary ~3.5 degBeamwidth -Secondary ~4.5 x 4.5 deg Source level (4 kHz) ~209 dB/ 1μPa@1m Dynamic range >110 dB Range resolution <0.15 m Penetration capability >200 mDepth range <20 – >11,000 m Beam steering sectors – across/ along 80/ 20 degREAL-TIME PROCESSING • Digital band-pass filter• Spiking deconvolution/ Matched filter • Time Varying Filter (TVF)• Bad trace removal • Dereverberation• Time Variable Gain (TVG)• Automatic Volume Control (AVC)• Stacking (Trace mixing)• Swell filter• Manual/automatic gain • Attribute processing • Statistics• Power Spectral Density (PSD) display ADDITIONAL OFF-LINE PROCESSING• Synthetic Aperture Sonar processing (SAS)• Interpretation/ Digitization of interfaces • TOPAS tracks displayed/ selected in SIS DIAMENSIONS AND WEIGHT• Tranceiver: 600 x 756 x 1188 mm, 170 Kg• Transducer array: 1142 x 1140 x 260 mm, 525 KgSYSTEM INTERFACES• Navigation input – NMEA 0183 (rs232/ UDP)• Depth/slope input – NMEA 0183 (rs232/ UDP)• Ethernet• Printer/ recorder – analogue/ digital • VRU (rs422/ rs232)• Synchronizing unit (K-Sync) – TTLSpecifications subject to change without any further notice.TECHNICAL SPECIFICATIONSSYSTEM BENEFITS• Narrow acoustic beam • High bandwidth• Chirp and short pulse modes • No sidelobes• Electronic beam steering• Single ping, multi ping and burst ping modes • Real-time processing• Heave, roll and pitch stabilised beam • Hull mounted transducer •High spatial resolutionJ u l y 2019E-mail:*******************************.comOutline dimensions for Transceiver UnitOutline of transducer casing with mounted elements。
斯仑贝谢所有测井曲线英文名称解释精编版

斯仑贝谢所有测井曲线英文名称解释OCEAN DRILLING PROGRAMACRONYMS USED FOR WIRELINE SCHLUMBERGER TOOLSACT Aluminum Clay ToolAMS Auxiliary Measurement Sonde APS Accelerator Porosity SondeARI Azimuthal Resistivity ImagerASI Array Sonic ImagerBGKT Vertical Seismic Profile Tool BHC Borehole Compensated Sonic Tool BHTV Borehole TeleviewerCBL Casing Bond LogCNT Compensated Neutron ToolDIT Dual Induction ToolDLL Dual Laterolog DSI Dipole Sonic ImagerFMS Formation MicroScannerGHMT Geologic High Resolution MagneticToolGPIT General Purpose Inclinometer ToolGR Natural Gamma RayGST Induced Gamma Ray SpectrometryToolHLDS Hostile Environment LithodensitySondeHLDT Hostile Environment LithodensityToolHNGS Hostile Environment Gamma RaySondeLDT Lithodensity ToolLSS Long Spacing Sonic ToolMCD Mechanical Caliper DeviceNGT Natural Gamma Ray SpectrometryToolNMRT Nuclear Resonance Magnetic ToolQSST Inline Checkshot ToolSDT Digital Sonic ToolSGT Scintillation Gamma Ray ToolSUMT Susceptibility Magnetic ToolUBI Ultrasonic Borehole ImagerVSI Vertical Seismic ImagerWST Well Seismic ToolWST-3 3-Components Well Seismic ToolOCEAN DRILLING PROGRAMACRONYMS USED FOR LWDSCHLUMBERGER TOOLSADN Azimuthal Density-NeutronCDN Compensated Density-NeutronCDR Compensated Dual ResistivityISONIC Ideal Sonic-While-DrillingNMR Nuclear Magnetic ResonanceRAB Resistivity-at-the-BitOCEAN DRILLING PROGRAMACRONYMS USED FOR NON-SCHLUMBERGER SPECIALTY TOOLSMCS Multichannel Sonic ToolMGT Multisensor Gamma ToolSST Shear Sonic ToolTAP Temperature-Acceleration-Pressure ToolTLT Temperature Logging Tool OCEAN DRILLING PROGRAMACRONYMS AND UNITS USED FOR WIRELINE SCHLUMBERGER LOGSAFEC APS Far Detector Counts (cps) ANEC APS Near Detector Counts (cps) AX Acceleration X Axis (ft/s2)AY Acceleration Y Axis (ft/s2)AZ Acceleration Z Axis (ft/s2) AZIM Constant Azimuth for DeviationCorrection (deg)APLC APS Near/Array Limestone PorosityCorrected (%)C1 FMS Caliper 1 (in)C2 FMS Caliper 2 (in)CALI Caliper (in)CFEC Corrected Far Epithermal Counts (cps)CFTC Corrected Far Thermal Counts (cps)CGR Computed (Th+K) Gamma Ray (APIunits)CHR2 Peak Coherence, Receiver Array,Upper DipoleCHRP Compressional Peak Coherence,Receiver Array, P&SCHRS Shear Peak Coherence, ReceiverArray, P&SCHTP Compressional Peak Coherence,Transmitter Array, P&SCHTS Shear Peak Coherence, TransmitterArray, P&SCNEC Corrected Near Epithermal Counts(cps)CNTC Corrected Near Thermal Counts (cps)CS Cable Speed (m/hr)CVEL Compressional Velocity (km/s)DATN Discriminated Attenuation (db/m)DBI Discriminated Bond IndexDEVI Hole Deviation (degrees)DF Drilling Force (lbf)DIFF Difference Between MEAN andMEDIAN in Delta-Time Proc. (microsec/ft)DRH HLDS Bulk Density Correction (g/cm3)DRHO Bulk Density Correction (g/cm3)DT Short Spacing Delta-Time (10\'-8\'spacing; microsec/ft)DT1 Delta-Time Shear, Lower Dipole(microsec/ft)DT2 Delta-Time Shear, Upper Dipole (microsec/ft)DT4P Delta- Time Compressional, P&S (microsec/ft)DT4S Delta- Time Shear, P&S (microsec/ft))DT1R Delta- Time Shear, Receiver Array, Lower Dipole (microsec/ft)DT2R Delta- Time Shear, Receiver Array, Upper Dipole (microsec/ft)DT1T Delta-Time Shear, Transmitter Array, Lower Dipole (microsec/ft)DT2T Delta-Time Shear, Transmitter Array, Upper Dipole (microsec/ft)DTCO Delta- Time Compressional (microsec/ft)DTL Long Spacing Delta-Time (12\'-10\' spacing; microsec/ft)DTLF Long Spacing Delta-Time (12\'-10\' spacing; microsec/ft)DTLN Short Spacing Delta-Time (10\'-8\' spacing; microsec/ft DTRP Delta-Time Compressional, ReceiverArray, P&S (microsec/ft)DTRS Delta-Time Shear, Receiver Array,P&S (microsec/ft)DTSM Delta-Time Shear (microsec/ft)DTST Delta-Time Stoneley (microsec/ft)DTTP Delta-Time Compressional,Transmitter Array, P&S (microsec/ft)DTTS Delta-Time Shear, Transmitter Array,P&S (microsec/ft)ECGR Environmentally Corrected GammaRay (API units)EHGR Environmentally Corrected HighResolution Gamma Ray (API units)ENPH Epithermal Neutron Porosity (%)ENRA Epithermal Neutron RatioETIM Elapsed Time (sec)FINC Magnetic Field Inclination (degrees)FNOR Magnetic Field Total Moment(oersted)FX Magnetic Field on X Axis (oersted)FY Magnetic Field on Y Axis (oersted)FZ Magnetic Field on Z Axis (oersted)GR Natural Gamma Ray (API units)HALC High Res. Near/Array LimestonePorosity Corrected (%)HAZI Hole Azimuth (degrees)HBDC High Res. Bulk Density Correction(g/cm3)HBHK HNGS Borehole Potassium (%)HCFT High Resolution Corrected FarThermal Counts (cps)HCGR HNGS Computed Gamma Ray (APIunits)HCNT High Resolution Corrected NearThermal Counts (cps)HDEB High Res. Enhanced Bulk Density(g/cm3)HDRH High Resolution Density Correction(g/cm3)HFEC High Res. Far Detector Counts (cps)HFK HNGS Formation Potassium (%)HFLC High Res. Near/Far Limestone Porosity Corrected (%)HEGR Environmentally Corrected High Resolution Natural Gamma Ray (API units)HGR High Resolution Natural Gamma Ray (API units)HLCA High Res. Caliper (inHLEF High Res. Long-spaced Photoelectric Effect (barns/e-)HNEC High Res. Near Detector Counts (cps)HNPO High Resolution Enhanced Thermal Nutron Porosity (%)HNRH High Resolution Bulk Density (g/cm3)HPEF High Resolution Photoelectric Effect (barns/e-)HRHO High Resolution Bulk Density (g/cm3)HROM High Res. Corrected Bulk Density (g/cm3) HSGR HNGS Standard (total) Gamma Ray(API units)HSIG High Res. Formation Capture CrossSection (capture units)HSTO High Res. Computed Standoff (in)HTHO HNGS Thorium (ppm)HTNP High Resolution Thermal NeutronPorosity (%)HURA HNGS Uranium (ppm)IDPH Phasor Deep Induction (ohmm)IIR Iron Indicator Ratio [CFE/(CCA+CSI)]ILD Deep Resistivity (ohmm)ILM Medium Resistivity (ohmm)IMPH Phasor Medium Induction (ohmm)ITT Integrated Transit Time (s)LCAL HLDS Caliper (in)LIR Lithology Indicator Ratio[CSI/(CCA+CSI)]LLD Laterolog Deep (ohmm)LLS Laterolog Shallow (ohmm)LTT1 Transit Time (10\'; microsec)LTT2 Transit Time (8\'; microsec)LTT3 Transit Time (12\'; microsec)LTT4 Transit Time (10\'; microsec)MAGB Earth\'s Magnetic Field (nTes)MAGC Earth Conductivity (ppm)MAGS Magnetic Susceptibility (ppm)MEDIAN Median Delta-T Recomputed(microsec/ft)MEAN Mean Delta-T Recomputed(microsec/ft)NATN Near Pseudo-Attenuation (db/m)NMST Magnetometer Temperature (degC)NMSV Magnetometer Signal Level (V)NPHI Neutron Porosity (%)NRHB LDS Bulk Density (g/cm3)P1AZ Pad 1 Azimuth (degrees)PEF Photoelectric Effect (barns/e-)PEFL LDS Long-spaced Photoelectric Effect (barns/e-)PIR Porosity Indicator Ratio[CHY/(CCA+CSI)]POTA Potassium (%)RB Pad 1 Relative Bearing (degrees)RHL LDS Long-spaced Bulk Density (g/cm3)RHOB Bulk Density (g/cm3)RHOM HLDS Corrected Bulk Density(g/cm3)RMGS Low Resolution Susceptibility (ppm)SFLU Spherically Focused Log (ohmm)SGR Total Gamma Ray (API units)SIGF APS Formation Capture Cross Section (capture units)SP Spontaneous Potential (mV)STOF APS Computed Standoff (in)SURT Receiver Coil Temperature (degC) SVEL Shear Velocity (km/s)SXRT NMRS differential Temperature(degC)TENS Tension (lb)THOR Thorium (ppm)TNRA Thermal Neutron RatioTT1 Transit Time (10\' spacing; microsec)TT2 Transit Time (8\' spacing; microsec)TT3 Transit Time (12\' spacing; microsec)TT4 Transit Time (10\' spacing; microsec)URAN Uranium (ppm)V4P Compressional Velocity, from DT4P(P&S; km/s)V4S Shear Velocity, from DT4S (P&S; km/s)VELP Compressional Velocity (processedfrom waveforms; km/s)VELS Shear Velocity (processed fromwaveforms; km/s)VP1 Compressional Velocity, from DT,DTLN, or MEAN (km/s)VP2 Compressional Velocity, from DTL,DTLF, or MEDIAN (km/s)VCO Compressional Velocity, from DTCO(km/s)VS Shear Velocity, from DTSM (km/s)VST Stonely Velocity, from DTST km/s)VS1 Shear Velocity, from DT1 (LowerDipole; km/s)VS2 Shear Velocity, from DT2 (UpperDipole; km/s)VRP Compressional Velocity, from DTRP(Receiver Array, P&S; km/s)VRS Shear Velocity, from DTRS (ReceiverArray, P&S; km/s)VS1R Shear Velocity, from DT1R (ReceiverArray, Lower Dipole; km/s)VS2R Shear Velocity, from DT2R (ReceiverArray, Upper Dipole; km/s)VS1T Shear Velocity, from DT1T (Transmitter Array, Lower Dipole; km/s)VS2T Shear Velocity, from DT2T (Transmitter Array, Upper Dipole; km/s)VTP Compressional Velocity, from DTTP (Transmitter Array, P&S; km/s)VTS Shear Velocity, from DTTS (Transmitter Array, P&S; km/s)#POINTS Number of Transmitter-Receiver Pairs Used in Sonic ProcessingW1NG NGT Window 1 counts (cps)W2NG NGT Window 2 counts (cps)W3NG NGT Window 3 counts (cps)W4NG NGT Window 4 counts (cps)W5NG NGT Window 5 counts (cps)OCEAN DRILLING PROGRAMACRONYMS AND UNITS USED FOR LWD SCHLUMBERGER LOGSAT1F Attenuation Resistivity (1 ft resolution; ohmm) AT2F Attenuation Resistivity (2 ftresolution; ohmm)AT3F Attenuation Resistivity (3 ftresolution; ohmm)AT4F Attenuation Resistivity (4 ftresolution; ohmm)AT5F Attenuation Resistivity (5 ftresolution; ohmm)ATR Attenuation Resistivity (deep; ohmm)BFV Bound Fluid Volume (%)B1TM RAB Shallow Resistivity Time afterBit (s)B2TM RAB Medium Resistivity Time afterBit (s)B3TM RAB Deep Resistivity Time after Bit(s)BDAV Deep Resistivity Average (ohmm)BMAV Medium Resistivity Average (ohmm)BSAV Shallow Resistivity Average (ohmm)CGR Computed (Th+K) Gamma Ray (APIunits)DCAL Differential Caliper (in)DROR Correction for CDN rotationaldensity (g/cm3).DRRT Correction for ADN rotationaldensity (g/cm3).DTAB AND or CDN Density Time after Bit(hr)FFV Free Fluid Volume (%)GR Gamma Ray (API Units)GR7 Sum Gamma Ray WindowsGRW7+GRW8+GRW9-Equivalent toWireline NGT window 5 (cps)GRW3 Gamma Ray Window 3 counts(cps)-Equivalent to Wireline NGT window1GRW4 Gamma Ray Window 4 counts(cps)-Equivalent to Wireline NGT window2GRW5 Gamma Ray Window 5 counts (cps)-Equivalent to Wireline NGT window 3GRW6 Gamma Ray Window 6 counts (cps)-Equivalent to Wireline NGT window 4GRW7 Gamma Ray Window 7 counts (cps)GRW8 Gamma Ray Window 8 counts (cps)GRW9 Gamma Ray Window 9 counts (cps)GTIM CDR Gamma Ray Time after Bit (s)GRTK RAB Gamma Ray Time after Bit (s)HEF1 Far He Bank 1 counts (cps)HEF2 Far He Bank 2 counts (cps)HEF3 Far He Bank 3 counts (cps)HEF4 Far He Bank 4 counts (cps)HEN1 Near He Bank 1 counts (cps)HEN2 Near He Bank 2 counts (cps)HEN3 Near He Bank 3 counts (cps)HEN4 Near He Bank 4 counts (cps) MRP Magnetic Resonance PorosityNTAB ADN or CDN Neutron Time after Bit(hr)PEF Photoelectric Effect (barns/e-)POTA Potassium (%) ROPE Rate ofPenetration (ft/hr)PS1F Phase Shift Resistivity (1 ft resolution;ohmm)PS2F Phase Shift Resistivity (2 ft resolution;ohmm)PS3F Phase Shift Resistivity (3 ft resolution;ohmm)PS4F Phase Shift Resistivity (4 ft resolution;ohmm)PS5F Phase Shift Resistivity (5 ft resolution;ohmm)PSR Phase Shift Resistivity (shallow; ohmm)RBIT Bit Resistivity (ohmm)RBTM RAB Resistivity Time After Bit (s)RING Ring Resistivity (ohmm)ROMT Max. Density Total (g/cm3) fromrotational processingROP Rate of Penetration (m/hr)ROP1 Rate of Penetration, average overlast 1 ft (m/hr).ROP5 Rate of Penetration, average overlast 5 ft (m/hr)ROPE Rate of Penetration, averaged overlast 5 ft (ft/hr)RPM RAB Tool Rotation Speed (rpm)RTIM CDR or RAB Resistivity Time after Bit(hr)SGR Total Gamma Ray (API units)T2 T2 Distribution (%)T2LM T2 Logarithmic Mean (ms)THOR Thorium (ppm)TNPH Thermal Neutron Porosity (%)TNRA Thermal RatioURAN Uranium (ppm)OCEAN DRILLING PROGRAMADDITIONAL ACRONYMS AND UNITS(PROCESSED LOGS FROM GEOCHEMICAL TOOL STRING)AL2O3 Computed Al2O3 (dry weight %)AL2O3MIN Computed Al2O3 Standard Deviation (dry weight %)AL2O3MAX Computed Al2O3 Standard Deviation (dry weight %)CAO Computed CaO (dry weight %)CAOMIN Computed CaO Standard Deviation (dry weight %)CAOMAX Computed CaO Standard Deviation (dry weight %)CACO3 Computed CaCO3 (dry weight %)CACO3MIN Computed CaCO3 Standard Deviation (dry weight %)CACO3MAX Computed CaCO3 Standard Deviation (dry weight %)CCA Calcium Yield (decimal fraction) CCHL Chlorine Yield (decimal fraction)CFE Iron Yield (decimal fraction)CGD Gadolinium Yield (decimal fraction)CHY Hydrogen Yield (decimal fraction)CK Potassium Yield (decimal fraction)CSI Silicon Yield (decimal fraction)CSIG Capture Cross Section (capture units)CSUL Sulfur Yield (decimal fraction)CTB Background Yield (decimal fraction)CTI Titanium Yield (decimal fraction)FACT Quality Control CurveFEO Computed FeO (dry weight %)FEOMIN Computed FeO StandardDeviation (dry weight %)FEOMAX Computed FeO StandardDeviation (dry weight %)FEO* Computed FeO* (dry weight %)FEO*MIN Computed FeO* StandardDeviation (dry weight %)FEO*MAX Computed FeO* StandardDeviation (dry weight %)FE2O3 Computed Fe2O3 (dry weight %)FE2O3MIN Computed Fe2O3 StandardDeviation (dry weight %)FE2O3MAX Computed Fe2O3 StandardDeviation (dry weight %)GD Computed Gadolinium (dry weight %)GDMIN Computed Gadolinium StandardDeviation (dry weight %)GDMAX Computed Gadolinium StandardDeviation (dry weight %)K2O Computed K2O (dry weight %)K2OMIN Computed K2O StandardDeviation (dry weight %)K2OMAX Computed K2O StandardDeviation (dry weight %)MGO Computed MgO (dry weight %)MGOMIN Computed MgO Standard Deviation (dry weight %)MGOMAX Computed MgO Standard Deviation (dry weight %)S Computed Sulfur (dry weight %)SMIN Computed Sulfur Standard Deviation (dry weight %)SMAX Computed Sulfur Standard Deviation (dry weight %)SIO2 Computed SiO2 (dry weight %)SIO2MIN Computed SiO2 Standard Deviation (dry weight %)SIO2MAX Computed SiO2 Standard Deviation (dry weight %)THORMIN Computed Thorium Standard Deviation (ppm)THORMAX Computed Thorium Standard Deviation (ppm)TIO2 Computed TiO2 (dry weight %) TIO2MIN Computed TiO2 Standard Deviation (dry weight %)TIO2MAX Computed TiO2 Standard Deviation (dry weight %)URANMIN Computed Uranium Standard Deviation (ppm)URANMAX Computed Uranium Standard Deviation (ppm)VARCA Variable CaCO3/CaO calcium carbonate/oxide factor。
MHS-15合CURY 氧化物系统产品说明书

Minimum investment and low operating costs The MHS-15 Mercury/Hydride System is a manual accessory for high-sensitivity determination of mercury and hydride-forming elements, such as As, Se, Sb, Te,Bi and Sn, by flame atomic absorp-tion (AA) spectroscopy. Mercury/hydride atomic absorption is the preferred technique for trace deter-mination of mercury and the metal-lic hydride-forming elements,because it offers the best possible detection limits, down to the ng range, while minimizing capital investment and operating costs.The technique is simple, easy-to-handle, and reliable and has been proven in tens of thousands of laboratories worldwide.Simple operationThe MHS-15 system includes a reaction assembly and a quartz-cell assembly. The analyzer is free-standing and is placed adjacent to the AA spectrometer sample compartment. It includes a reaction flask, a reservoir for the reducing agent and all pneumatic compo-nents for carrier transportation of the metallic vapors to the quartz cell.The cell mounts on a standard10-cm PerkinElmer ®burner head,permitting the cell to be heated in the spectrometer flame. The quartz cell is positioned in the light beam of the spectrometer and alignment is provided by the instrument’s burner adjustment controls. ItsMHS-15 Mercury/Hydride SystemP R O D U C T N O T EMinimum investment Low operating costs Simple operationWorks with all PerkinElmeratomic absorption spectrometersKey BenefitsAT O M I C A B S O R P T I O Nquartz cell can be easily tilted out of the flame using a swivel mount.Due to the reaction chemistry in the mercury/hydride process, the ana-lyte is completely separated from its matrix during analysis, eliminating interferences.Operating procedure During analysis, the sample contain-ing the analyte in ionic form is placed in the reaction flask. The flask is then installed into the analyzer assembly.By manually actuating a pneumatic valve, an argon stream transports the reducing agent (NaBH 4) from a reser-voir into the sample. The special conical form of the immersion tube and reaction flask provides excellent mixing of the sample and NaBH 4,guaranteeing spontaneous reaction.No stirring device is required for this procedure.Hydrogen, liberated during the reac-tion, reduces the metal ions to gaseous hydrides. In the case of mercury determination, NaBH 4reduces mer-cury into its metallic state. Excess hydrogen and the argon carrier gas transport the hydride (or metallic mercury) vapor into the quartz cell where the absorption of the metal is measured. During mercury determi-nation, the cell remains at ambient temperatures. Mercury determina-tion can also be performed using stannous chloride (SnCl 2) as the reducing agent. For the determina-tion of hydride-forming elements,the quartz cell is heated by an air/acetylene flame to thermally decompose the metal hydrides.For a complete listing of our global offices, visit /lasoffices©2004 PerkinElmer, Inc. All rights reserved. The PerkinElmer logo and design are registered trademarks of PerkinElmer, Inc. AAnalyst is a trademark and PerkinElmer is a registered trademark of PerkinElmer, Inc. or its subsidiaries, in the United States and other countries. All other trademarks not owned by PerkinElmer, Inc. or its subsidiaries that are depicted herein are theproperty of their respective owners. PerkinElmer reserves the right to change this document at any time without notice and disclaims liability for editorial, pictorial or typographical errors.006385C_01ELEC110400Printed in USAPerkinElmer Life and Analytical Sciences 710 Bridgeport AvenueShelton, CT 06484-4794 USA Phone: (800) 762-4000 or (+1) 203-925-4602Technical dataAbsorption cell Quartz cell, 165 mm long, 12 mm diameter Cell heating Up to approx. 1000 °C with air/acetylene flameReaction flaskPolypropylene flask with special, conically formed inner side.Connection to the analyzer assembly with special joint with O-ring gasket.Sample volume Max. 50-mL volume Reducing agent NaBH 4or SnCl 2Inert gas supply Argon, inlet pressure 2.5-3.5 bar (36-51 psig) approx. consumption 1.1 L/min Power supply No electrical supply is required.Dimensions 70 mm wide x 160 mm deep x 375 mm high WeightApproximately 4.3 kgDetection limits using the MHS-15 with the AAnalyst™ 700(EDL lamps were used for all elements)。
复发性阿弗他溃疡病因与治疗药物研究进展

(13)阿祥仁,张鑫生.利舒康胶囊对高原红细胞增多症患者体内氧自由基代谢指标的影响中草药,2006,37(11)!705-1706. (14)更藏加.基于文献整理和数据挖掘的藏医治疗HAPC用药规律及多血康胶囊临床研究[D].成都:成都中医药大学2017. (15)倪惠珍,马川.益心康泰胶囊对高原红细胞增多症患者体内同型半胱氨酸、一氧化氮和一氧化氮合酶水平的影响()•高原医学杂志,2008,18(1)!5-16.(16)史桂平.血府逐瘀汤治疗高原红细胞增多症52例疗效观察()•高原医学杂志,2006,16(4):31.(17)赵文玲,杨如意,曹昌霞,等.通脉活血汤联合温针法对高原红细胞增多症患者血液流变学及抗氧化能力的影响()•海南医学院学报,2017,23(15):2037-2039.(18)张玮.丹红注射液联合奥扎格雷钠治疗高原红细胞增多症的疗效观察(/CD).中西医结合心血管病电子杂志,2015,3(17):107-108.(19)MUSTAPIC Z,BASIC-JUKIC N,LOVCIC V,et al.Pottransplant erythrocytosis[J).Acta Med Croatica,2011,65(4):3313361(0)刘玲,杨新生,岳京丽.茶色素对血液流变学作用的临床观察()•河南诊断与治疗杂志,1998,12(3):151-153.[21)SHARMA S,GRALL AJ,ORDONEZ JG,et al.Acetazolamide .综述.and N-acetylcysteineinthetreatmentofchronic mountainsickness(Monge's disease)[J).Respir Physiol Neurobiol,2017,246:1-8.(2)陈浩,次仁罗布,刘金风,等.益心康泰胶囊对高原红细胞增多症患者血液流变学的影响()•西藏医药杂志,2010,31(4)!-3. (3)李燕,夏伟国.葛根素治疗高红症性脑病45例疗效观察().西南国防医药,2006,16(5)!27-528(24)HEINRIHEC#WUL#LAWRENCEESetal1GeneticvariantsattheEGLN1locusassociated withhigh-altitudeadaptationin Tibetans are absent or found at low frequency in highland Andeans (J).Ann Hum Genet201983(3)!171-176.(25)HE G,WANG Z,SU Y,et al.Genetic structure and forensic characteristics of Tibeto-Burman-speaking U-Tsang and Kham Tibetan Highl"ndersreve"ledby27Y-chromosom"lSTRs(J).SciRep20199(1)!7739.(26)COTTO KC#WAGNER AH#FENG YY et"l.DGIdb3.0!"re-design"ndexp"nsionofthedrug-geneinter"ctiond"t"b"se(J).NucleicAcidsRes201846(D1)!D1068-1073.(收稿日期:2020-07-29修回日期:2020-12-30)复发性阿弗他溃疡病因与治疗药物研究进展杨波综述,张良明,刘基,陈彦清,袁晓燕#审校(攀枝花市中心医院,四川攀枝花617067)[摘要]复发性阿弗他溃疡(RAU)是一种影响口腔黏膜的溃疡性疾病,其发病因素与诸多因素有关。
DISPOSAL OF ASH, SLUDGE, AND THE LIKE INTO WATER
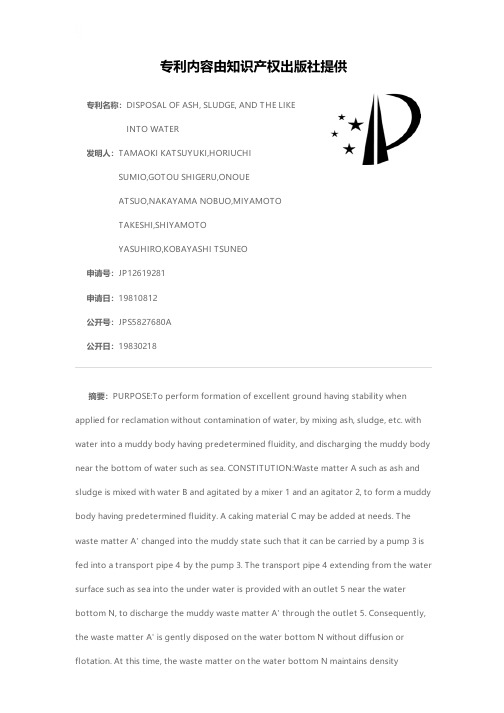
专利名称:DISPOSAL OF ASH, SLUDGE, AND THE LIKE INTO WATER发明人:TAMAOKI KATSUYUKI,HORIUCHISUMIO,GOTOU SHIGERU,ONOUEATSUO,NAKAYAMA NOBUO,MIYAMOTOTAKESHI,SHIYAMOTOYASUHIRO,KOBAYASHI TSUNEO申请号:JP12619281申请日:19810812公开号:JPS5827680A公开日:19830218专利内容由知识产权出版社提供摘要:PURPOSE:To perform formation of excellent ground having stability when applied for reclamation without contamination of water, by mixing ash, sludge, etc. with water into a muddy body having predetermined fluidity, and discharging the muddy body near the bottom of water such as sea. CONSTITUTION:Waste matter A such as ash and sludge is mixed with water B and agitated by a mixer 1 and an agitator 2, to form a muddy body having predetermined fluidity. A caking material C may be added at needs. The waste matter A' changed into the muddy state such that it can be carried by a pump 3 is fed into a transport pipe 4 by the pump 3. The transport pipe 4 extending from the water surface such as sea into the under water is provided with an outlet 5 near the water bottom N, to discharge the muddy waste matter A' through the outlet 5. Consequently, the waste matter A' is gently disposed on the water bottom N without diffusion or flotation. At this time, the waste matter on the water bottom N maintains densitydetermined during the agitation, so that the waste matter A' can be made to have proper strength.申请人:SHIMIZU KENSETSU KK更多信息请下载全文后查看。
高规模数据中心计算安全解密:超规模计算受网络和安全限制影响说明书
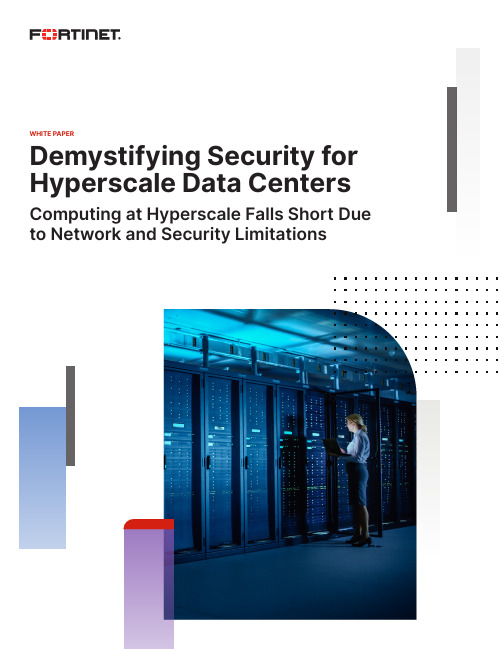
WHITE PAPERDemystifying Security for Hyperscale Data Centers Computing at Hyperscale Falls Short Due to Network and Security LimitationsExecutive SummaryMany industries have embraced hyperscale computing to address a variety of different use cases. For example, large enterprises building hybrid IT architectures to launch applications quickly require super-fast communication between assets that are spread across physical and virtual domains. Advanced research institutions, such as those performing genomic or aerospace research, require the ability to transfer massive datasets over their networks. Ecommerce companies, including high-velocity eRetail, use hyperscale architectures to manage connection bursts on major shopping holidays like Cyber Monday, and to deal with surges in activity resulting from the COVID-19 pandemic.One problem with hyperscale data centers, however, is that they lack adequate security. Why? Because turning on security often creates a network bottleneck if security solutions struggle to keep pace, or if the IT infrastructure isn’t properly segmented. With underqualified network firewalls acting as bottlenecks, many network engineering and operations leaders bypass the security altogether. However, this leaves the organization open to various types of attacks that can affect how the business performs even basic functions.Securing hyperscale data centers requires an updated strategy and the technology to match.IntroductionDigital innovation (DI) initiatives and business demands have changed how enterprise data centers are used and the performance metrics they must meet. Adapting to the demand for new network capabilities has driven the evolution of hyperscale data centers.A hyperscale data center is one that scales efficiently and performs dynamically to meet escalating business demands. Hyperscale architectures are designed to meet unprecedented requirements for enormous capacity and astronomical performance. These demands can vary from industry to industry. Examples of activities that require hyperscale architectures include:n Large enterprises including cloud service providers. Organizations using virtualization to create massively scalable nvirtual networks require large-scale network segmentations based on Virtual Extensible Local-Area Networks (VXLANs) and fast communications between services that are co-hosted on physical and virtual platforms.n Dynamic ecommerce including high-velocity eRetail. Event-based connection bursts, such as shopping holidays, nonline tax filing, and filing for unemployment benefits, require the ability to handle massive numbers of userconnections per second.1n Advanced research of pharmaceuticals, oil and gas, and aerospace. The use of big data and machine-learning (ML) nalgorithms for advanced research requires the ability to transmit 40 Gbps and 100 Gbps “elephant flows.”2n Stock exchanges. Electronic trading infrastructure requires that market data be received with the lowest possible nlatency.3n Hyperscalers (massive, global technology companies). High-speed data-center interconnects between cloud data ncenters to replicate data across disaster recovery (DR) sites require high-speed interfaces and high-throughput IPsec tunneling capabilities to achieve data privacy and confidentiality.4In many cases, organizations operating in these industries have invested in the network infrastructure that they require. However, sourcing security solutions capable of meeting these needs presents a challenge since existing next-generation firewalls (NGFWs) do not meet the massive scale and performance needs of hyperscale architectures. These existing NGFWs struggle if organizations want to perform admission control on tens of millions of user connections per second,or implement anti-distributed denial-of-service (DDoS)) attacks along with essential Layer 4 firewalling. The resulting performance degradation causes many organizations to simply disable security functionality, fearing it will slow down their business and prevent them from optimizing the throughput and latency of their network infrastructure. But this is a dangerous trade-off: The organization’s choice of delivering on escalating demands without adequate security controls is like rolling dice and hoping it won’t be attacked.Challenges of Hyperscale ArchitecturesEvery deployment environment presents challenges for hyperscale security.Pains of running massively scalable, virtualized servicesBusinesses need to be able to launch services in the most agile fashion possible to increase productivity and revenue.To maximize return on investment (ROI) benefits, services need to interoperate between physical and virtual assets.By leveraging massively scalable technologies like VXLAN, customers can segment all virtualized services to realizea magnitude of scale that is not possible with VLAN. Virtualized services can scale up and down and move without significant operational overhead. These services are often required to communicate with other services located in the existing physical infrastructure. Most current solutions, however, suffer from low performance and high latency, and lack the essential Layer 4 security to track session state and admission controls that can decide who is allowed versus not allowed. They also don’t offer the advanced Layer 7 security that would further detect threats, and enforce policy to achieve compliance and manage risks.Event-based connection bursts overwhelm inelastic securityIn other industries, the volume of each individual connection is not as significant as the total number of connections that an organization must be capable of processing in a very short time frame. On major shopping holidays like Black Friday and Cyber Monday, ecommerce sites experience extremely high volumes of consumer traffic over 24 hours—as much as 1.5 times more than the next-largest shopping days of the year.5Similar event-based connection bursts occur during tax season, or the opening of ticket sales for major events or on holidays such as the Lunar New Year, and in online gaming environments, especially massively multiplayer games, in which hundreds of players may participate concurrently in 30-minute bursts.In response, hyperscale architectures enable online tax filers, retailers, and game-hosting services to accept and efficiently process millions of incoming connections per second. The business case for investing in hyperscale is simple: Dropped connections or slow responses can result in lost sales and damage to brand reputation. As an example, on average, one to three seconds of delay in page load time results in a 32% increase in customers abandoning the site.6Large network flows vulnerable to attackArtificial intelligence (AI) and ML applications require enormous datasets, often reaching multiple terabytes,7 for algorithm training and testing. Organizations in pharmaceutical, biotech, genomics, and oil/gas industries all require these large datasets for research. In order to process and analyze the data, these research institutions need to be able to transmit massive datasets efficiently over the network. However, transmitting these datasets efficiently requires network bandwidth that can reach up to 100 Gbps, or what’s known as an “elephant flow.”Theoretically, research institutions should be able to leverage hyperscale network architectures built on routers and switches to deliver this bandwidth. However, these devices do not track session state and offer essential Layer 4 security. As DDoS attacks become more common, these devices are also vulnerable to attacks.Additionally, the data they transmit over these connections is often sensitive and covered by data protection laws like the EU’s General Data Protection Regulation (GDPR) or the Health Insurance Portability and Accountability Act (HIPAA). These laws mandate various access controls, which means network traffic must be routed through security technology such as firewalls and encrypt the flow of messages. However, most NGFWs cannot handle connection bandwidths of more than 10 Gbps. And this not only slows down research by a significant margin but also inhibits organizations to maximize ROI on their existing wide-area network (WAN) links that are procured to transmit data at 40 Gbps and 100 Gbps, all because a single flow through the existing NGFWs can only support 10 Gbps without crashing.Firewall latency can lead to losses of millionsFor stock market trading, competitive gaming, and similar industries, low network latency is extremely important. Even small delays in round-trip time (RTT) of network traffic can have a significant impact on profitability or performance.As a result, organizations in the financial industry commonly invest in network infrastructure that provides extremelylow latency for their data centers. Electronic trading infrastructure tolerates no more than 5 μs of latency.8 In latency-sensitive contexts, many organizations configure their NGFWs in monitor mode, sacrificing security for network throughput.9High-speed data-center interconnects require high-throughput IPsec connectivityFor cloud service providers and organizations operating content distribution networks (CDNs), the ability to replicate data across multiple regional sites is essential. The primary reason organizations use regional sites to host full copies of stored data is that they increase resiliency, decrease the latency of responses to customer requests, and lessen the load on the primary data center.To make this possible, organizations require data-center interconnects (DCIs)—high-bandwidth links between regional sites tasked with supporting the synchronization of the network.10 Since cloud service providers and CDNs often transmit sensitive or proprietary data, these links are often implemented as IPsec tunnels. Yet, at the same time, Layer 4 network security requires NGFWs to be capable of processing IPsec traffic with the same throughput as the network links. But, because most existing NGFWs can’t achieve IPsec throughput in excess of 10 Gbps, the NGFWs securing these links can slow down the overall transfer of large amounts of data across data centers.ConclusionDI efforts, intended to improve efficiency and customer experience, require network infrastructure to evolve. Hyperscale data centers are designed to support massive network flows, connection bursts, and several other use cases.While many organizations have deployed hyperscale network architecture, achieving hyperscale security is more of a challenge. Disabling NGFWs or placing them in monitoring mode, to eliminate network bottlenecks, leaves an organization open to attack and potentially out of compliance with data protection regulations. Not segmenting applications and IT infrastructure opens the opportunity for an intruder to enter and reach the core of the network after penetrating the edge. And these dangerous results compound even more if the attacks originate from internal and trusted users. Hyperscale data centers require a completely radical approach to security solutions, one that can scale with escalating business demands. Unless a hyperscale security solution can handle massive user connections at scale, process tens of millions of connections per second, support 100 Gbps elephant flows, efficiently segment massive virtual environments, protect the enterprise edge with high-performance, essential Layer 4 security, and prevent DDoS attacks, all bets are off and tilt the advantage toward attackers aiming to launch various cyberattacks to disrupt the business, cause bad press, and eventually shut down operations.1 Marisa Sanfilippo, “The Best Days for Holiday Sales: A Guide for Businesses,” Business News Daily, December 2, 2019.2 Rajiv Kohli and Nigel P. Melville, “Digital innovation: A review and synthesis,” Information Systems Journal, January 29, 2018.3 “Deterministic Communications for Secure High-speed Performance: Fortinet Protects Connections to Electronic Trading Platforms with the Industry’s Lowest Latency and Jitter Rates,” Fortinet, September 23, 2019.4 “What is DCI?” Ciena, May 16, 2019.5 Marisa Sanfilippo, “The Best Days for Holiday Sales: A Guide for Businesses,” Business News Daily, December 2, 2019.6 “Find Out How You Stack Up to New Industry Benchmarks for Mobile Page Speed,” Google, March 2017.7 Mohammad Shaikh and Harsha Gurukar, “Machine Learning and HPC in Pharma Research and Development,” Super Computing 2019, November 2019.8 “Deterministic Communications for Secure High-speed Performance: Fortinet Protects Connections to Electronic Trading Platforms with the Industry’s Lowest Latency and Jitter Rates,” Fortinet, September 23, 2019.9 Jason Pappalexis, “The NGFW Today: A Staple of Network Security in Spite of Challenges,” NSS Labs, March 11, 2019.10 “What is DCI?” Ciena, May 16, 2019. Copyright © 2021 Fortinet, Inc. All rights reserved. Fortinet, FortiGate, FortiCare and FortiGuard, and certain other marks are registered trademarks of Fortinet, Inc., and other Fortinet names herein may also be registered and/or common law trademarks of Fortinet. All other product or company names may be trademarks of their respective owners. Performance and other metrics contained herein were attained in internal lab tests under ideal conditions, and actual performance and other results may vary. Network variables, different network environments and other conditions may affect performance results. Nothing herein represents any binding commitment by Fortinet, and Fortinet disclaims all warranties, whether express or implied, except to the extent Fortinet enters a binding written contract, signed by Fortinet’s General Counsel, with a purchaser that expressly warrants that the identified product will perform according to certain expressly-identified performance metrics and, in such event, only the specific performance metrics expressly identified in such binding written contract shall be binding on Fortinet. For absolute clarity, any such warranty will be limited to performance in the same ideal conditions as in Fortinet’s internal lab tests. Fortinet disclaims in full any covenants, representations, and guarantees pursuant hereto, whether express or implied. Fortinet reserves the right to change, modify, transfer, or otherwise revise this publication without notice, and the most current version of the publication shall be applicable. Fortinet disclaims in full any covenants, representations, and guarantees pursuant hereto, whether express or implied. Fortinet reserves the right to change, modify, transfer, or otherwise revise this publication without notice, and the most current version of the publication shall be applicable.February 25, 2021 3:25 PM。
- 1、下载文档前请自行甄别文档内容的完整性,平台不提供额外的编辑、内容补充、找答案等附加服务。
- 2、"仅部分预览"的文档,不可在线预览部分如存在完整性等问题,可反馈申请退款(可完整预览的文档不适用该条件!)。
- 3、如文档侵犯您的权益,请联系客服反馈,我们会尽快为您处理(人工客服工作时间:9:00-18:30)。
Demystifying Sludge and VarnishJim Fitch, Noria CorporationTags: varnish, contamination controlMachinery Lubrication (1/2002)You could tar your roof with it. It sticks to everything. It spreads like cancer. You thought you were getting rid of it with the oil change and flush, but it is back again - lurking in your oil and gripping the insides of your machine.Does this sound familiar? Maybe you have it now . . . sludge and varnish. Do you know what causes it and how to stop it from spreading? Do you know how to prevent it from coming back later? More importantly, do you understand its destructive potential?More Cases of AfflictionIn industrial equipment, Noria has seen a surprising surge in sludge and varnish (S&V) cases in recent years. There are many possible explanations, but perhaps the biggest contributor evolves from the increasing demand for machinery reliability. Today, there is more awareness in the user community of the impact that S&V has on lubrication and machinery health. People are making closer inspections, asking more questions and are less tolerant of its presence.Additionally, we are seeing increasing use of low-solvency basestocks (hydrocracked and PAOs for instance) that could be amplifying problems. These basestocks, on one hand, are more thermally and oxidatively robust. On the other hand, they may be more prone to lay down and coagulate oil degradation products (oxides, salts, carbon fines, etc.), leading to sludge and varnish.We are also noticing more users pushing lubricants to thelimits by extending oil drains and in some cases, improperlytop-treating in-service oils with additive concentrates. Manyused oils are now being reconditioned and returned toservice or blended with new oil. In certain instances, thesesomewhat progressive practices may lead to more frequentand severe S&V problems.In addition, many organizations are doing a better job atcontrolling leakage through better maintenance. Oddly, thiscan contribute to more frequent cases of sludge and varnishproblems. When leakage is reduced, so too is thereplenishment of fresh additives and base-stocks that comein with makeup oil. This has the practical effect of shortening the oil’s life.In contrast, companies generally are maintaining equipment cooler, cleaner and dryer than in the past, and are better at deploying oil analysis. These improved practices normally have a positive and stabilizing effect on lubricant health and reduce the risk of S&V problems.Causes of Sludge and VarnishThe approach of detecting and analyzing S&V problems in machinery is not the same as used oil analysis. In many instances this is because the evidence is not always in the oil. The sludge and varnish should be analyzed directly, using a completely different set of tests and evaluation parameters. Still, used oil analysis plays an important diagnostic role to help reveal candidate causes as well as to rule-out others.The conditions that commonly lead to S&V problems vary, which complicates the process of identifying the root cause analytically. There are at least 25 unique lubricant degradation mechanisms leading to sludge or varnish formation. A few of these include:•Aeration of the fluid•Sparking from static electricity•Bulk thermal degradation•Antifreeze contamination•Soot coagulation•Bulk oil oxidation•Hydrolysis•Prolonged cold storage•Grease contaminated oil•Caustic detergent contamination•Nitration•Coking on hot surfaces•Radiological contamination•Poor engine combustion efficiency and blowby•Highly aromatic fuels•Sulfation (fuel, H2S, etc.)•Lead corrosion reactions•Reactive compressor gases•Additive incompatibilities•Base oil incompatibilitiesUpon looking at the above list, it is obvious that the prescribed corrective action relies on the accurate discovery of the specific and often-elusive root cause. Without this, correcting the problem is reduced to the costly and lengthy process of trial-and-error. There is a unique remedy for each cause.It is always good advice to keep an accurate history of the conditions and observations that lead up to the occurrence of S&V. The troubleshooting process depends on building a case file containing each little piece of information and time line.Machine InspectionsLubricants degrade in different ways, and the products of this degradation are essentially referred to as sludge and varnish. These products are generally unstable in the oil and, as such, are looking for a place to land, that is, to deposit themselves. In certain instances, the deposits form on machine surfaces at the exact location where the oil has degraded, for example, hot surface coking. In other cases, the oil degrades in one location but deposits condensed on a surface elsewhere.Over time, some deposits can thermally cure (become baked-on) to a tough enamel-like coating. Other types of deposits, generally in cooler zones, remain soft or gummy. Sludge is not always black or even dark. It may appear clear and grease-like, similar to petroleum jelly. The following are examples of where and how S&V might occur:•Black crusty deposits on mechanical seals•Gold adherent films on spool valves in EHC hydraulic systems•Charcoal-like deposits on babbitt sleeve bearings•Gooey-brown mayonnaise on diesel engine oil filters•Black scabby deposits on thrust-bearing pads•Lumpy, tar-like globs in dryer bearing drain lines (paper machine)•Grayish gummy deposits on NG engine discharge ports•Carbonaceous residue of servo strainers•Hard black enamel on piston crown and ring lands•Cottage cheese-like gunk clinging to engine valve covers•Drab-color slime on compressor oil filtersDamage CausedThe deposits that form on machine surfaces interfere with the reliable performance of the fluid and the machine’s mechanical movements. They can also contribute to wear and corrosion or simply just cling to surfaces. For example, deposits on the spool of a servo control valve can tighten the interference fit between the spool and bore. Compounding this are the adherence properties of varnish, which can stick particles from the oil to silt lands, leading to common silt-lock valve failure.Other types of sludge and varnish-type failures include plugged orifices, damaged mechanical seals, plugged discharged ports on compressors, journal-bearing failure, premature plugging of oil filters and diesel engine combustion-zone wear.Most organizations that suffer from S&V problems have these three needs:1. They want to know the root cause of the problem.2. They need simple solutions to quickly return the lubricant and machine back to service.3. They want to know how to prevent the root cause from reoccurring.Because of the impact S&V has on lubrication and machine reliability, for the past several years, Noria has been on a crusade to learn everything we could about it. We have conducted extensive laboratory investigations. We have amassed stacks of analytical material. We have conferred with experts and academics. We have combed through hundreds of pages of technical literature. And we have gone to the field to see it in the machines where it lives.Do you have a problem you’ve encountered relating to sludge and varnish? Have you learned something new about how it forms or had a success in solving a problem? If so, please share your experiences.。