测量系统评价2
六西格玛测量系统分析

* 注意:5.15标准差代表99%的测量系 统变异,5.15标准差为工业标准
2.测量变异指标:%R&R值→测量变异量/流程总变异量
%R&R=
σ测量 σ总变量
●判断标准:
×100%
●最佳情形:≤10% ●可接受情形:≤30%
测量系统变异(Ⅰ)
• 仪器偏差 - 不同的仪器即使侧量同一物件,平均值也会造成 可以察觉的不同
仪器一偏差量
Master Value
仪器二偏差量
仪器一
平均数
仪器二
平均数
Precision(变异性)
• 测量系统中的总变异 • 测量重复值的自然变异 • 名词: Random Error, Spread, Test/ Retest error • 重复性与再现性
• 描述为 no drift , sudden shifts , cyclic trends , etc. • 使用趋势图( Trend Chart )来评估
时间1 时间2
校验数值 ( 参考标准)
测量稳定性
稳定性是指:对同一部品间隔一段时间测量所得平均的差异.
Time 2 Time 1 测量系统的磨损,气温,温度等对测量结果的影响
种类的数目 1数据类
2-4数据类
控制
可以用于控制,只有当:
1)当流程变化与规格 比较时,其值小
2)在预期流程变化范 围内,损失功效是 扁平的
3)变化的主源引起平
均变化
1)基于流程分布,可以 使用半变量控制技术
2)可以产生非敏感变量 控制图
分析
1)对于评估流程参数和 指数是不能接受的
2)只表示流程是否正在 产生一致或不一致部分
1
2
3
测量系统分析(MSA)2

一.测量系统分析(MSA)21.定义:稳固性——测量系统在某连续时刻内测量同一基准或零件的单一特性时获得的测量值总变差。
2.使用均值和极差操纵图,该操纵图可提供方法以分离阻碍所有测量结果的缘故产生的变差〔一般变差〕和专门条件产生的变差〔专门缘故变差〕。
凡信号显现在操纵值外点均表现〝失控〞或〝不稳固〞。
3.研究:绘出标准〔样件〕重复读数X或R,图中失控信号即为需核准测量系统的标志。
4.操作要领:必须认真策划操纵图技术〔如取样时刻、环境等〕,以防样本容量、频率等导致失误信号。
5.稳固性改进①从过程中排除专门缘故——由超出的点反应。
②减少操纵限宽度——排除一般缘故造成的变差。
图2测量系统特性图二.偏倚1.定义:偏倚——测量结果的观看平均值与基准的差值。
2.操作方式:①对一件样件进行周密测量。
②由同一评判人用被评判单个量具测量同一零件至少十次。
③运算读数平均值。
④偏倚=基准值-平均值3.产生较大偏倚的缘故①基准误差②磨损的零件③制造的仪器尺寸不对④测量错误的特性⑤外表未正确校准⑥评判人使用仪器不正确。
三.重复性1.定义:重复性——由一个评判人采纳一种测量器具,多次测量同一零件的同一特性时获得的差值。
2.测量过程的重复性意味着测量系统自身的变异是一致的。
重复性可用极差图显示测量过程的一致性。
3.重复性或量具变差的估量:σe=5.15×R/d2d2——常数〔查表得〕与零件数量、试验次数有关。
5.15——代表正态分布的90%的测量结果。
四.再现性1.定义:再现性——由不同评判人采纳相同测量器具测量同一零件的同一特性时测量平均值的变差。
2.测量过程的再现性说明评判人的差异性是一致的。
假设评判人变异存在,那么每位评判人所有平均值将会不同,可采纳均值图来显示。
3.估量评判人标准偏差σo=5.15×R o/d2d2——常数〔查表得〕与零件数量、试验次数有关。
5.15——代表正态分布的90%的测量结果。
测量系统分析(MSA)-实例

03 实例测量系统分析
偏倚分析
确定测量系统的准确性
通过比较测量系统所得结果与已知标准值或参考值之间的差异, 评估测量系统的偏倚程度。
计算偏倚值
将测量系统的结果与标准值或参考值进行对比,计算出偏倚值。
判断偏倚是否可接受
根据所允许的偏倚范围,判断测量系统的偏倚是否在可接受的范围 内。
线性分析
1 2
测量系统分析(MSA)-实例
目录
• 测量系统分析概述 • 实例选择与数据收集 • 实例测量系统分析 • 实例测量系统评价 • 实例总结与改进建议
01 测量系统分析概述
定义与目的
定义
测量系统分析(MSA)是对测量系 统的误差来源、大小及分布进行评 估的过程。
目的
识别测量系统的变异性来源,确 保测量系统能够满足产品质量和 过程控制的要求。
测量系统分析的重要性
提高产品质量的可预测性和可靠性
01
通过对测量系统进行全面分析,可以了解测量误差的大小和分
布,从而更准确地预测产品质量。
优化生产过程控制
02
准确的测量数据是生产过程控制的基础,对测量系统进行有效
的分析有助于提高过程控制的稳定性和有效性。
降低成本
03
通过减少测量误差,可以减少重复测量、检验和返工等不必要
的操作,从而降低生产成本。
测量系统分析的步骤
确定分析范围和对象
明确需要分析的测量设备、工 具或方法,以及相关的操作人
员和环境条件。
数据收集
收集一定数量、具有代表性的 测量数据,包括重复测量、再 现性数据等。
数据分析
对收集到的数据进行统计分析 ,识别测量系统的变异性来源 。
结果评估与改进
测量系统评价报告
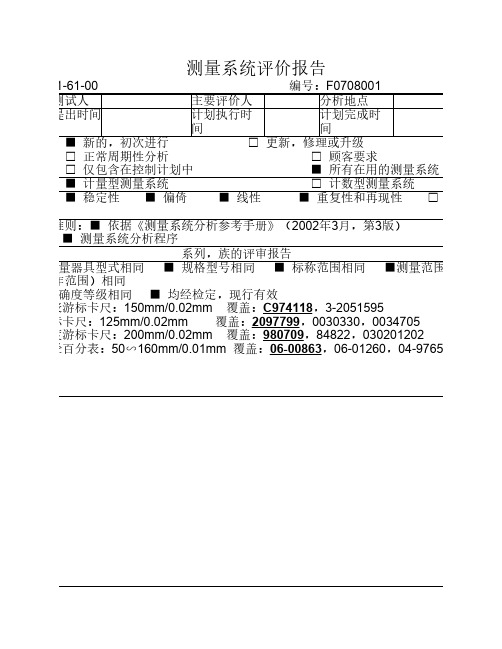
状态:■ □ □ 方法:■ 项目:■ 其他: 接受准则:■ 依据《测量系统分析参考手册》(2002年3月,第3版) ■ 测量系统分析程序 系列,族的评审报告 ■ 计量器具型式相同 ■ 规格型号相同 ■ 标称范围相同 ■测量范围 (工作范围)相同 ■ 准确度等级相同 ■ 均经检定,现行有效 1.带表游标卡尺:150mm/0.02mm 覆盖:C974118,3-2051595 2.游标卡尺:125mm/0.02mm 覆盖:2097799,0030330,0034705 3.深度游标卡尺:200mm/0.02mm 覆盖:980709,84822,030201202 4.内径百分表:50∽160mm/0.01mm 覆盖:06-00863,06-01260,04-9765
分析:
结论: 编制/日期 审核/日期 批准/日-00 主要测试人 计划提出时间 编号:F0708001 主要评价人 分析地点 计划执行时 计划完成时 间 间 新的,初次进行 □ 更新,修理或升级 正常周期性分析 □ 顾客要求 仅包含在控制计划中 ■ 所有在用的测量系统 计量型测量系统 □ 计数型测量系统 稳定性 ■ 偏倚 ■ 线性 ■ 重复性和再现性 □
测量系统分析(2)

测量系统分析Measurement Systems Analysis一、测量系统所应具有之统计特性1.测量系统必须处于统计控制中,这意味着测量系统中的变差只能是由于普通原因而不是由于特殊原因造成的。
这可称为统计稳定性。
2.测量系统的变差必须比制造过程的变差小。
3.变差应小于公差带。
4.测量精度应高于过程变差和公差带两者中精度较高者,一般来说,测量精度是过程变差和公差带两者中精度较高者的十分之一。
5.测量系统统计特性可能随被测项目的改变而变化。
若真的如此,则测量系统的最大的变差应小于过程变差和公差带两者中的较小者。
二、标准6.国家标准7.第一级标准(连接国家标准和私人公司、科研机构等)8.第二级标准(从第一级标准传递到第二级标准)9.工作标准(从第二级标准传递到工作标准)三、测量系统的评定10.测量系统的评定通常分为两个阶段,称为第一阶段和第二阶段11.第一阶段:明白该测量过程并确定该测量系统是否满足我们的需要。
第一阶段试验主要有二个目的:1.确定该测量系统是否具有所需要的统计特性,此项必须在使用前进行。
2.发现哪种环境因素对测量系统有显着的影响,例如温度、湿度等,以决定其使用之空间及环境。
3.第二阶段的评定4.目的是在验证一个测量系统一旦被认为是可行的,应持续具有恰当的统计特性。
5.常见的就是“量具R&R”是其中的一种型式。
四、各项定义6.量具: 任何用来获得测量结果的装置,包括用来测量合格/不合格的装置。
7.测量系统:用来获得表示产品或过程特性的数值的系统,称之为测量系统。
测量系统是与测量结果有关的仪器、设备、软件、程序、操作人员、环境的集合。
8.量具重复性:指同一个评价人,采用同一种测量仪器,多次测量同一零件的同一特性时获得的测量值(数据)的变差。
9.量具再现性:指由不同的评价人,采用相同的测量仪器,测量同一零件的同一特性时测量平均值的变差。
10.稳定性:指测量系统在某持续时间内测量同一基准或零件的单一特性时获得的测量值总变差。
MSA测量系统分析2
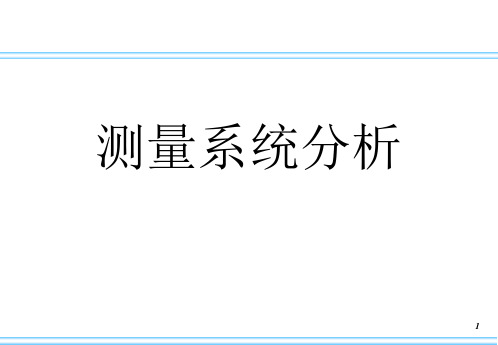
何谓标准
• 国家标准
–在美国是由NIST保持或追踪。
• 一级标准
–直接从国家标准直接复制或传递而来的标准。
• 二级标准
–从一级标准传递而来的标准
• 工作标准
–从二级标准传递而来的标准
51
测量系统的评定
• 第一阶段:明白该测量过程并确定该测量系统是否 满足我们的需要。主要有二个目的
–确定该测量系统是否具有所需要的测量特性,此项必须 在使用前进行。
35
测量的变异说明
36
环境因素
• 温度 • 湿度 • 振动 • 照明 • 腐蚀 • 磨耗 • 污染(油脂)
37
人性因素
• 训练 • 技能 • 疲劳 • 无聊 • 眼力 • 舒适 • 零件的复杂性 • 检验的速度 • 指导书的误解
38
测量系统的特性
• Discrimination分辨力(ability to tell things apart)
• 测量系统的变异必须比制造过程的变异小。 • 变异应小于公差带。
49
• 测量精密应高于过程变异和公差带两者中精度 较高者,一般来说,测量精度是过程变异和公 差带两者中精度较高者的十分之一。
• 测量系统统计特可能随被被测项目的改变而变 化。若真的如此,则测量系统的最大的变差应 小于过程变差和公差带两者中的较小者。
10
线性
• 测量系统预期操作范围内偏倚误差值的差别。换句 话说,线性表示操作范围内多个和独立的偏倚误差 值的相关性。
11
被测体
• 在规定条件下被测量的特殊数量或对象,对于测量 应用一个定义的系列规范。
12
测量系统
• 用于量化一个测量单位或确定被测特性性质的仪器 或量具、标准、操作、方法、夹具、软件、人员、 环境、和条件的集合; 用来获得测量的整个过程 。
测量系统分析-MSA-2
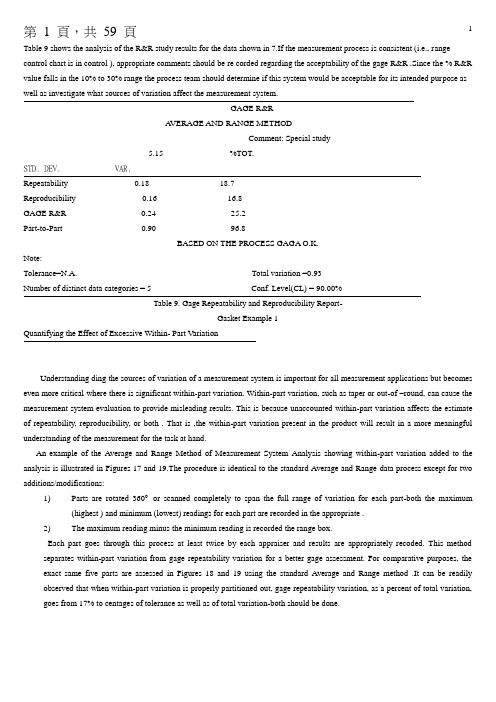
Table 9 shows the analysis of the R&R study results for the data shown in 7.If the measurement process is consistent (i.e., range control chart is in control ), appropriate comments should be re corded regarding the acceptability of the gage R&R .Since the % R&R value falls in the 10% to 30% range the process team should determine if this system would be acceptable for its intended purpose as well as investigate what sources of variation affect the measurement system.GAGE R&RA VERAGE AND RANGE METHODComment: Special study5.15 %TOT.STD. DEV. VAR.Repeatability 0.18 18.7Reproducibility 0.16 16.8GAGE R&R 0.24 25.2Part-to-Part 0.90 96.8BASED ON THE PROCESS GAGA O.K.Note:Tolerance=N.A. Total variation =0.93Number of distinct data categories = 5 Conf. Level(CL) = 90.00%Table 9. Gage Repeatability and Reproducibility Report-Gasket Example 1Quantifying the Effect of Excessive Within- Part VariationUnderstanding ding the sources of variation of a measurement system is important for all measurement applications but becomes even more critical where there is significant within-part variation. Within-part variation, such as taper or out-of –round, can cause the measurement system evaluation to provide misleading results. This is because unaccounted within-part variation affects the estimate of repeatability, reproducibility, or both . That is ,the within-part variation present in the product will result in a more meaningful understanding of the measurement for the task at hand.An example of the Average and Range Method of Measurement System Analysis showing within-part variation added to the analysis is illustrated in Figures 17 and 19.The procedure is identical to the standard Average and Range data process except for two additions/modifications:1)Parts are rotated 3600 or scanned completely to span the full range of variation for each part-both the maximum(highest ) and minimum (lowest) readings for each part are recorded in the appropriate .2)The maximum reading minus the minimum reading is recorded the range box.Each part goes through this process at least twice by each appraiser and results are appropriately recoded. This method separates within-part variation from gage repeatability variation for a better gage assessment. For comparative purposes, the exact same five parts are assessed in Figures 18 and 19 using the standard Average and Range method .It can be readily observed that when within-part variation is properly partitioned out, gage repeatability variation, as a percent of total variation, goes from 17% to centages of tolerance as well as of total variation-both should be done.Figure 16. Gage Repeatability and Reproducibility Data Sheet- Including Within-part VariationFigure 17 Gage Repeatability and Reproducibility Report – Including Within – Part VariationFigure 18. Standard Average and Range Method Method Gage Repeatability and Reproducibility Data SheetFigure 19. Standard Average and Range Method Method Gage Repeatability and Reproducibility ReportGage Repeat. Reprod . Study Repeat Reprod. Gage Part/Part Total R&R % Tot. Alone as Alone as Type Var. Var. R&R WIV Var. Proc. Var. Proc. Var. % TV %TV WithWIV 0.0534 0.177 0.182 0.218 1.144 1.178 15.9% 4.2% 15.3%W/OWIV 0.200 0.178 0.269 0 1.144 1.175 22.9% 17.0 % 15.3%Table 10. Gage Study Comparison – Same Five, With andW/O within-Part Variation(WIV)Analysis of Variance (ANOVE) MethodAnalysis of variance (ANOV A) is a standard statistical technique and can be used to analyze the mea surement error and other sources of variability of data in a measurement systems study. In the analysis of variance, the variation can be decomposed into four categories: Parts, appraisers, interaction between between parts and appraisers , and appraisers, and replication error due to the gag e.The advantages of ANOV A techniques as compared with Average and Range methods are: they are capable of handling any experimental set-up ;can estimate the variances more accurately; and extractmore information (such as interaction between Parts and appraisers effect)from the experimental data.the disadvantages are that the numerical computations require a computer and users require a certain degree of knowledge to interpret the results. The ANOV A method as described in the following sections can be found in the Appendix.andRandomizationalceIndependenS tatisticaThe method of collecting the data is important an ANOV A method. lf the data are not collected in a random manner, this can lead to a source of bias values. A simple way to assure a balanced design for (n) parts, (k) appraisers, and (r) trials is through randomization . One common approach to randomization isto wrte Ai on a slip of paper to denote the measurement for the first appraiser on the first part. Do this upto A(n) for the measurement by the first appraiser on the nth part. Follow the same procedure for the next appraiser up to and including the kth appraiser. The similar notation will be used where BI, CI denotes the measurement for second and third appraiser on the first part. Once all nk combinations are written, then the slips of paper can be put in a hat or bowl. One at a time, a slip of paper is selected. These combinations (A1,B2….) are the measuring order in which the gage study will be performed. Once all nk combinations are selected, they are put back into the hat and the procedure is followed again. This is done for a total of times to determine the order of experiments for each repeat. A better method would be to use a random number table.In general, all efforts should be taken to assure statistical independence within the study. Using the PDSA cycle, the study should first be laid out completely and then conducted as specified.Analyzing the DataThe data can be collected in a random manner using a form similar to Table 7. For our example, there Are ten parts and three appraisers, and the experiment has been performed in random order twice for each Part and appraiser combination.Plotting the DataAny of the graphical methods given in Figure 13 can be used in the graphical analysis of the data these methods can be used to confirm and provide further insight to the data (i.e., trends, cycles, etc. ).one graphical method that is suggested is called an interaction plot. this plot confirms the results of the f test on whether or not the interaction is significant. in this particular interaction plot, the average measure ment per appraiser per part vs. part number (1,2,…, etc.) is graphed in Figure 20.The points for each appraiser average measurement per part are connected to form k(number of appraisers) lines. The way to interpret the graph is if the k lines are parallel there is no interaction is term. When the lines are nonparallel (i.e., will eventually cross or intersect each other),the interaction is significant The larger the angle of intersection, the greater the interaction. Appropriate measures should be taken to eliminate the causesfor the interaction. In the example in Figure 20, the lines are nearly parallel, indicating no significant interaction.Another graph sometimes of interest is the residuals plot . This graph is more a check for the validity of the assumptions. This assumption is that the gage (error) is a random variable from a normal distribu tion .The residuals, which are the differences between the observed readings and predicted values vs. the predicted values, are plotted. Predicted value is the average of the repeated readings for each appraiser for each part. If the residuals are not randomly scattered above and below zero (horizontal reference line),it could be because the assumptions are incorrect and further investigation of the data is suggested.Figure 20. Part by Appraiser PlotThe histogram plot, Figure 21, is a graph that displays the frequency distribution of the gage error of Appraisers who participated in the study. It also shows their combined frequency distribution.If the reference values are available:Error = Observed Value – Reference ValueOtherwise:Error = Observed Value – Part AverageThe histogram plot provides a quick visual overview of how the gage error is distributed. Issues such As whether bias or lack of consistency exist in the measurements taken by the appraisers, can be identified Even before the data are analyzed.The numerical analysis can be done according to the formulas in Table 13 (Page 73). This is what is called the Analysis of Variance (ANOV A) table. The ANOVA table is composed of six columns . The source column is the cause of variation. The DF column is the degree of freedom associated with the source. The SS or sum of squares column is the deviation around the mean of the source . The MS or mean square col- umn is the sum of squares divided by degrees of freedom. The F ratio column is calculated only for the interaction; it is determined by the mean square of interaction divided by mean square gage (error). The EMS or expected mean square column determines the linear combination of variance components for Each MS. The ANOV A table decomposes the total source of variation into four components: parts, apprais- Ers, interaction of appraisers and parts, and replication error due to the gage.The estimate of variance components for each source is given in Table 11.E s t i m a t e V a r l a n c eGage MS t e=2InteractionrMS MSre op)(2-=Appraiser nrMS MS wOP)(02-=PartkrMS MS Qop p )(2-=Table 11. Estimate of Variance Components1 In these guidelines, all components of variation are assumed to be random effects.Since each mean square is a sample quantity subject to sampling variation and the computations involve differences of mean squares, then negative variance components estimates are possible. This isa bit of a problem since the “master ” variance components are equal or close to zero or have a small sample size. For analysis purposes, the negative variance component is set to zero.Standard deviation is easier to interpret than variance because it has the same unit of measurement As the original observation.5.15 sigma spread for a measure of repeatability called equipment variation (EV) and measure of reproducibility called appraiser variation (A V). If the interaction of part and appraiser is significant we have a non-additive model and therefore an estimate of its variance components given. The R & R in Table 16 is the total of measurement system variation.MS eEV 15.5=nrAV MS MS OPO-=15.5rI Aoorauser Part n Interactio MS MSe OP-=⨯15.5 )())()222((&I AV EV R R ++= krPV ion PartVariat MS MS op p )(15.5)(-=Table 12. 5.15 Sigma SpreadIn the additive model, the interaction is not significant and the variance components for each sourceIs determined as follows: First, the sum of square of gage error (SS efrom Table 13) is added to the sum ofSquare of part x appraiser interaction SS opfrom Table 13) and then is added to the sum of squares pooled)(SSPoolwith nkr –n –k+1 degrees of freedom. Then the)(SSPoolwill be divided by the ( nkr-n-k+1) toCalculate)(MSPoolThe 5.15 sigma spread limit then will be:MSPoolEV 15.5=nrAV MS MS Pool O )(15.5-=)()22(&AV EV R R +=krPV MS MS Poolp -=(15.5NOTE: In order to determine if the interaction is significant,the F statistics of part X appraiser interaction (see Table 13).compare this F statistic to an upper percentage point of an F distribution with numerator and denominator degrees offreedom taken from the ANOV A (Table 13).In order to decrease the risk of falsely concluding that there is no interaction effect, choose a high Significance level. Once we have decided what the R&R is then we can calculate the %R&R in relation to Process Performance.nkrkri x x SSni p.....212-=∑= ∑∑∑===-=rm k j n i n k rijmTSS x X 12211... nkrnrj x x SS nj o....212.-=∑= ][SS SS SS SSO P P O eTSS ++-=1,...r M 1,......k J 1,......n I ..1...21 (12).212===+--=∑∑∑∑====kj kj kri ni rji ni OPnkr X nrj x xxSSANOV ASource DF SS MS EMS FAppraiser k-1 SS oMS SS o O k =-1/ n r w ry 222++τParts n-1 SS pms SS p P n =-1/ δτkr ry 222++Appraiser x Part (n-1)(k-1) SS opms SS op OP k n =--)1)(1/(22ry +τMSMS eOPGage (Error) nk (r-1)SS eMS SS e e r nk =-)1(/ τ2Total nkr-1 TSSAppraiser ~ N(o,)2wParts ~ N(0,o 2)Appraiser ⨯Part ~ N(o,2γ) Gage (Error) ~ N(o,2τ)Table 13. Analysis of Variance (ANOV A)Table 14 shows the ANOV A calculations for our example data from Table 7. Table 15 shows the comparison of the ANOV A method with the Average and Range method .Table 16 shows the gage R&R report For the ANOV A method.Source DF SS MS F EMS Appraiser 2 0.04800 0.02400 222202ωγτ++ Parts 19 20.5871 0.22875 222602ωγτ++ Appraiser x Part 18 0.10367 0.00575 4.45* 222γτ+ Gage (Error) 30 0.03875 0.00129 2τ Total 59 2.2491 *Significant at a = 0.25 levelEstimate Std. 90% Conf. Limit % Study % Of Variance Dev.(σ) for Std. Dev. 5.15 (σ) Variation Contribution2τ=0.00129 0.0359 (0.030, 0.046) EV=0.19 17.6 3.1 (Repeatability)2ω=0.00091 0.0302 (0.007,0.152) A V=0.16 14.8 2.2 (Operator )2γ=0.00223 0.0472 (0.029,0.074)INT=0.24 23.2 5.4 (Interaction)R&R=0.00443 0.0666 (0.060,0.163) R&R=0.34 32.7 10.7 (222ωγτ++)0371641.02=σ 0.1928 (0.121,0.334) PV=0.99 94.5 89.3 (Part)Study (or total) Variation, TV=05.1 (()99.0)34.0&2222=+=+PV RR% (study) Variation =100[⎥⎦⎤⎢⎣⎡)var ( 15.5)( 15.5iation study a components a % Contribution =100)var ( 15.5)( 15.52⨯⎪⎪⎭⎫ ⎝⎛iation study a components aTable 14. ANOV A CalculationMethod Lower 5.15 Upper %Total (Study)90%CL Std. Dev. 90%CL Variation GRR Sheet*EV 0.13 0.18 0.26 18.7EV 0.09 0.16 0.69 16.8R&R 0.18 0.24 0.33 25.2 PART 0.65 0.90 1.55 96.8 ANOV AEV 0.15 0.19 0.24 17.6A V 0.04 0.16 0.78 14.8 INTERACTION 0.15 0.24 0.38 23.2R&R 0.31 0.34 0.84 32.7 PART 0.62 0.99 1.72 94.5*In average and range method the interaction component cannot be estimated.Table 15. Comparison of ANOV A and Average and Range MethodsGA File: GASKET GAGE R&RANOV A METHODSTUDY #: 1 STUDY DATE: MM-DD-YYGAGE - #: X-2034 DESC: Thickness Gage TYPE: 0- 10.0 MMCHAR- #:1 DESC: NAME: One3 Apr 10 Parts 2 Trials Comment :Special studyLOWER 5.15 UPER %TPTAL PERCENTCL STD.DEV CL V ARIATION CONTRIBUTIO Repeatability 0.15 0.19 0.24 17.6 3.1 Reproducibility 0.04 0.16 0.78 14.8 2.2Part x Appraiser 0.15 0.24 0.38 23.2 5.4GAGE R&R 0.31 0.34 0.84 32.7 10.7Part-to-Part 0.62 0.99 1.72 94.5 89.3BASED ON DATA CATEGORIES, GAGE O.K.Note:Tolerance = N.A. Study variation = 1.05 Number of distinct data categories = 4 Conf Level (CL) =90.00%Table 16. Gage R&R ANOV A MethodGUIDELINES FOR DETERMING LINEARITYLinearity can be determined using the following guidelines:1) Select five parts whose measurements, due to process variation, cover the operating range of the gage. 2) Have each part measured by layout inspection to determine its reference and to confirm that theoperating range of the subject gage is encompassed.3) Have each part measured 12 times to the subject gage by one of the operators who normally use thegage.- Select the Part at random to minimize bias in the measurements.4) Calculate the part average and the bias average for each part. average from the part reference value. 5) Plot the bias average and the reference values as shown in Figure 12. 6) Calculate the linear regression line that best fits these points and the goodness of fit (R 2) of the lineusing the following equations.y=a+bx,where x = reference value y = bias averagea = slope∑∑⨯-=)(nx a n y b Goodness of fit = R 2=⎥⎥⎦⎤⎢⎢⎣⎡⎪⎪⎭⎫ ⎝⎛-⨯⎥⎥⎦⎤⎢⎢⎣⎡⎪⎪⎭⎫ ⎝⎛-⎥⎥⎦⎤⎢⎢⎣⎡-∑∑∑∑∑∑∑n y y n x x n y X xy 22222Linearity =Slope x Process variation% Linearity = 100[linearity / process variation] ∑∑∑∑-∑-=n n x xy a x x y22)()(Section 5GAGE PERFORMANCE CURVE 1The purpose of developing a Gage Performance Curve “(GPC) is to determine the probability of either Accepting or rejecting a part of some reference value. Ideally, the GPC for a measurement without error is Shown in Figure 22. However , this is the exception for measurement systems, rather than what normally Occurs.Once the amount of error has been determined, it is possible to calculate the probability are normally distributes with some variance , s 2. As previously described, error consists primarily of lack of repeatability, Reproducibility and bias. This is normally distributed with a mean x T , the reference value, plus the bias, And has some variance , s 2.In other words:Gage Error =N(),2s B X T +The probability of accepting a part of some reference value is given by the relationship⎰+=ulllT adxB N S X p ),(2Using the standard normal table⎪⎪⎭⎫ ⎝⎛+-Φ-⎪⎪⎭⎫ ⎝⎛+-Φ=S B LL S B UL X X p T T a )()( where⎰∞-+=⎪⎪⎭⎫ ⎝⎛+-ΦUL T T dx B N S B UL s X X ),()(2⎰∞+=⎪⎪⎭⎫ ⎝⎛+-ΦLLT T dx B N S B LL s X X ),()(2EXAMPLE: Determine the probability of accepting a part when the reference torque value is 0.5mm,0. 7mm,0.9mm. Using data from a previously conducted study we know: bias = B = 0.05mm GRR = 0.24upper specification = UL = 1.0mm s = 0.24/5.15 =0.05mm lower specification = UL = 0.6mm1 Adapted with permission from “Analysis of Attribute Gage Systems ” by J. McCaslin & G Gruska ,ASQC,1976.⎪⎪⎭⎫ ⎝⎛+-Φ-⎪⎪⎭⎫ ⎝⎛+-Φ=S B LL S B UL X X P T T a )(( ⎪⎭⎫ ⎝⎛+-Φ-⎪⎭⎫ ⎝⎛+-Φ=05.0)05.05.0(6.005.0)05.05.0(0.1( ())0.1(0.9Φ-Φ=84.00.1-=16.0=When the part has a reference value of 0.5 mm it will be rejected approximately 84% of the time.Gage Performance Curve Example mm XT7.0=⎪⎭⎫ ⎝⎛+-Φ-⎪⎭⎫ ⎝⎛+-Φ=05.0)05.07.0(6.005.0)05.07.0(.1Pa)0.3()0.5(-Φ-Φ==0.999If the reference value of the part is 0.7mm then it will be rejected approximately less than 0.1% of the time.mm XT9.0=⎪⎭⎫ ⎝⎛+-Φ-⎪⎭⎫ ⎝⎛+-Φ=05.0)05.09.0(6.005.05.09.0(0.1P a )0.7()0.1(-Φ-Φ==0.84Another interpretation of the example above is that a part whose reference value is 0.9mm would be rejected approximately 16% of the time using that particular measurement system.If the probability of acceptance is calculated for all values of XTand plotted, then the Gage Performance Curve, as shown inFigure 23. would be obtained.In addition , this same curve can be more easily plotted on normal probability paper, as shown in Figure 24. Aa can be observed, the GPC gives the probability of accepting a part over all part sizes. In addition, the GPC can be used to calculate the repeatability and reproducibility error , and the bias error. The R&R can be determined by finding that the XTvalue that corresponds to,995.0=Paand theXTvalue that correspondsto005.0=Pafor either of the two limits .The R&R is the difference between theTwoXTvalues, as shown graphically in Figure 24.An estimate of the bias is determined by finding the XT,for either the upper or lower limit, that corresponds to,5.0=P aandB=UL or LL X T -=-X T BDepending on at which limit XTis chosen.(Note: This assumes that the measurement system is linear over the operatingrange.)Section 6ATTRIBUTE GAGE STUDYATTRIBUTE GAGE STUDY (SHORT METHOD)An attribute gage is one that compares each part to a specific set of limits and accepts the part if theLimits are satisfied, otherwise it rejects the part. Most such gages are set up to accept and reject a set of Master parts . Unlike a variable gage, an attribute gage cannot indicate how good or how bad a part is ,but Only that the part is accepted or rejected.The short study is conducted by selecting 20 parts. Two appraisers then measure all parts twice in a manner that will prevent appraiser bias (see Section 3, page 39.) In selecting the 20 parts, it is desirablethat some of the parts are slightly below and above both specification limits.The gage is acceptable if all measurement decisions (four per part ) agree. If the measurement decisions Do not agree, the gage must be improved and re-evaluated. If the gage cannot be improved, it is unacceptableA typical form for the short method attribute gage study, is used in Table 17:RUBBER HOSE I.DAs with any measurement system the stability of the process should be verified and, if necessary, monitored. For attribute measurement systems, attribute control charting of a constant sample over time is a common way of verifying stability.For an attribute gage, the concept of the Gage Performance Curve is used for developing a gage study , Which is used to assess the amount of repeatability and bias of the gage. This analysis can be used on both single and double limit gages. For a double limit gage, only one limit need be examined with the assumptions of linearity and uniformity of error. For convenience, the lower limit will be used for discussion.In general, the attribute gage study consists of obtaining the reference values for several selected parts. These parts are evaluated a number of times of times, (m), with the total number of accepts (a), for each part being recorded. Form the results, repeatability and bias can be assessed.The first stage of the attribute study is the part selection. It is essential that the reference value is known for each part used in the study. Eight parts should be selected at as nearly equidistant intervals as practical. The maximum and minimum values should represent the process range. Although this selection does not affect confidence in the results, it the results, it does affect the total number of parts needed to complete to complete the gage study. The eight parts must be run through the gage, m=20 times, and the number of accepts, (a)recorded.For the total study, the smallest part must have the value a=0; the largest part, 20;and the six other parts,1.19≤≤a If these criteria are not satisfied, more parts with known reference values, (x),must be run through the gage until the above conditions are met. If, for the smallest yalue a ,20≠ then larger and larger parts are taken until a =20. if six of the parts do not have 1,19≤≤a additional parts can be taken at selected points throughout the range. These points are taken at the midpoints of the part measurements already measured in the study. The first interval at the smallest measurement where a =20. For best results, samples should be taken at = 0 ands and worked toward the middle of the part range. If necessary, the procedure cam be repeated until the criteria are met.Once the data collection criteria have been satisfied, the probabilities of acceptance must be calculated for each part using the following equations:The adjustments cover the instances where 1,191≤≤a For the instances where a =0 set ex a p'=Cept for the largest reference value with a = 0 , in which.025.0'=apFor the instances where a = 20 then1'=apexcept for the smallest reference value with a =20 in which.975.0'=ap1. Adapted with permission from “Analysis of Attribute Gage Systems ” by J. McCaslin &the GPC can be presented graphically (see Figure 26), use of normal probability paper (see Figure 25) yields more accurate estimates of the repeatability and bias.The calculated probabilities are plotted on probability paper and a line of best fit is drawn through these points. The bias is equal to the lower limit minus the reference value measurement that corresponds toor ap,5.0'=Limit Lower at Bias atp X)5.0('-==The repeatability is determined by finding the differences of the reference value measurements corresponding to1.08 of factor adjustment an by dividing and 005.0 and 995.0'P '===a apRepeatability=08.1)005.0 ()995.0a(p p''=-=aTTat at XXTo determine if the bias is significantly different from zero, the following statistic is used: y p e a t a b i l i tB i a s t Re 3.31⨯=If this calculated value is greater than 2.093 (0.025,19), then the bias is significantly from zero.An example will clarify the attribute study data collection of repeatability and bias.Example An attribute gage is being used to measure a dimension that has a tolerance of .010.0± The gage is an end of line 100% automatic inspection gage that is affected by repeatability and bias. To perform the attribute study, eight parts with reference values at intervals of 0.002 from –0.016 to –0.002 are run through the gage 20 times each. The number of accepts for accepts for each part are:T Xa0.016 0 -0.014 30.012 8 0.010 20 0.008 20 0.006 20 0.004 20 0.002 20Since there are two reference values with 1,19≤≤a at least four more parts must be found. These reference values and the number of accepts are: -0.015 1-0.013 1 -0.011 16Now we have five reference values values with .191≤≤a The procedure requires that one more part be Found with .191≤≤a Therefore, the following part is evaluated:-0.0105 18Now that the data collection criteria have been satisfied, satisfied, the probabilities of acceptance can be calculated using the binomial adjustments shown on page 80.a TX 'P a -0.016 0 0.025 -0.015 1 0.075 -0.014 3 0.175 -0.013 5 0.275 -0.012 8 0.425 -0.011 16 0.775 -0.0105 18 0.875 -0.010 20 0.975-0.008 20 1.00These probabilities are plotted on normal probability as shown in Figure 25. By plotting a best fit line through these points the bias and repeatability can be determined. The bias is equal to the lower limit minus the reference value measurement that corresponds to.5.0'=apFrom Figure 25, we have : Bias =-0.0123-(-0.010)=-0.0023The repeatability is determined by finding the differences of the reference value measurements corresponding toand ap995.0'=005.0'=apand dividing by 1.08. From Figure 25, we have:0073.008.10079.008.1)0163.0(0084.0==---=RTo determine if the bias is significantly different from zero, calculate.86.90073.0)0023.0(3.313.31===RB tSince,093.219,025.0=tthe bias is significantly different from zero. Like the variable Gage PerformanceCurve shown in Figure 23, the attribute GPC can also be plotted on plain graph paper (see Figure26). This can be accomplished in either of two ways. The first approach would be to run the sample study, only at the other limit of the specification. In the example, the long method for attribute study would also have to be conducted at the high limit of the specification, and the calculated values plotted accordingly. However, because of the previously mentioned assumptions, it would not be necessary to run the study again. Since the shape of the curve at the high limit should be a “mirror image ” of the curve at the low limit, the only consideration necessary is the location of the curve with respect toXTvalues. This location is determined by the bias. Thecorrect position of the curve would be defined at the point where the ,5.0=P a and at the。
【MSA】测量系统评估的基本要求
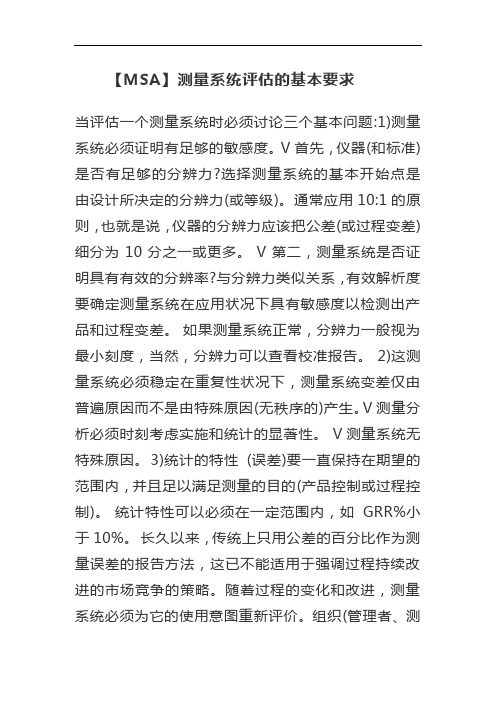
【MSA】测量系统评估的基本要求
当评估一个测量系统时必须讨论三个基本问题:1)测量系统必须证明有足够的敏感度。
V首先,仪器(和标准)是否有足够的分辨力?选择测量系统的基本开始点是由设计所决定的分辨力(或等级)。
通常应用10:1的原则,也就是说,仪器的分辨力应该把公差(或过程变差)细分为10分之一或更多。
V第二,测量系统是否证明具有有效的分辨率?与分辨力类似关系,有效解析度要确定测量系统在应用状况下具有敏感度以检测出产品和过程变差。
如果测量系统正常,分辨力一般视为最小刻度,当然,分辨力可以查看校准报告。
2)这测量系统必须稳定在重复性状况下,测量系统变差仅由普遍原因而不是由特殊原因(无秩序的)产生。
V测量分析必须时刻考虑实施和统计的显著性。
V测量系统无特殊原因。
3)统计的特性(误差)要一直保持在期望的范围内,并且足以满足测量的目的(产品控制或过程控制)。
统计特性可以必须在一定范围内,如GRR%小于10%。
长久以来,传统上只用公差的百分比作为测量误差的报告方法,这已不能适用于强调过程持续改进的市场竞争的策略。
随着过程的变化和改进,测量系统必须为它的使用意图重新评价。
组织(管理者、测
量规划者、生产操作者和质量分析人员)理解测量的目的并且进行适当的评价是不可或缺的。
测量系统分析
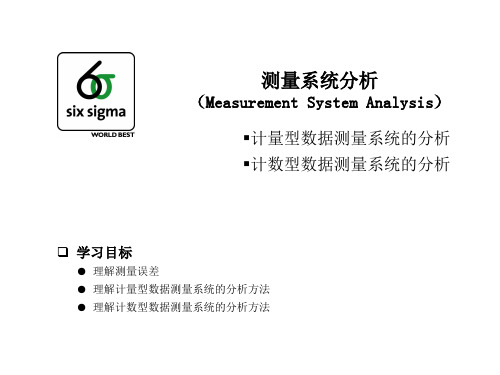
阶段 1
Gage R&R计划
阶段 3 准确度检讨
阶段2
随机取样测量
阶段 4 精确度(Gage R&R)检讨
阶段3 Minitab分析:Gage R&R Study
阶段 5 稳定性检讨
阶段4 Minitab分析:Gage Run Chart
阶段 5
后续措施实施
Proprietary to Samsung Electronics Company
测量系统分析- 13
Rev 7.0
计量型数据Gage R&R
▪ 注意事项
-随机顺序测量 -盲测:测试者需在事先不知测量哪种样品条件下进行,防止霍索恩效应
(Hawthorne) -测量设备读取数值应为最接近的数值,可能的话,最小刻度的一半为好
例如,最小刻度为0.1的话,读取推测值为0.05 -各测量者为了求得测量值,需使用相同的步骤(包含所有阶段)
“Bad”
样品范围 > 流程分散 测量系统的评价指标要 比实际要好
Good !!
样品范围 = 流程分散
“Bad”
样品范围 < 流程分散 测量系统的评价指标 比实际要差
Proprietary to Samsung Electronics Company
测量系统分析- 16
Rev 7.0
阶段 2
样品测量 - 尽可能地对测量者或产品进行随机测量
测量系统分析- 11
Rev 7.0
识别能力
- 所谓的识别能力指测量系统可探知所测量特性微细变化的能力,也称为分辨率 - 测量系统的识别能力不足的话,就不能将它作为确定流程变动的分析依据 - 测量系统的最小测量单位,应该能够精确到测量出流程分散的范围及规格幅度
测量系统分析通用课件

数据处理软件
用于对测量数据进行处理、分 析和存储,通常与测量仪器集 成在一起。
人员
负责维护、校准和使用测量系 统的人员,需具备相关技能和
知识。
02 测量系统的评价
CHAPTER
测量系统的误差来源
随机误差
01
由于随机因素引起的测量值无规律变化的误差,如温度、湿度
波动等。
系统误差
02
由于测量系统本身的不完善、测量原理的近似、测量器具的精
预防措施
针对常见故障制定预防措施,避免故障再次发生 。
04 测量系统分析的实际应用
CHAPTER
在生产质量控制中的应用
测量系统分析在生产质量控制中发挥着关键作用,通过对 生产过程中的各种参数进行准确测量和评估,确保产品质 量的稳定性和可靠性。
在生产过程中,测量系统分析通过对原材料、半成品和成 品的质量检测数据进行统计分析,识别出潜在的质量问题 ,并采取相应的措施进行改进。
环境监测领域
测量系统在环境监测领域的应用前景广阔,能够 实现环境参数的实时监测和数据分析,为环境保 护提供科学依据。
医疗领域
测量系统在医疗领域的应用前景广阔,能够实现 生理参数的实时监测和数据分析,为医疗诊断和 治疗提供支持。
提高测量系统性能的途径与方法
采用先进的传感器技术
采用高精度、高稳定性的传感器是提高测量系统性能的关键,能 够实现更准确、更可靠的测量。
此外,测量系统分析还可以用于评估生产设备的性能和精 度,确保生产过程的稳定性和一致性,提高生产效率和产 品质量。
在产品研发与设计中的应用
在产品研发与设计阶段,测量系统分析同样具有重要意义。通过 对产品性能参数的准确测量和评估,有助于优化产品设计,提高 产品的性能和可靠性。
测量系统分析2

(3)再现性(Reproducibility)
不同的测量人员、使用不同设备、在 不同实验室、在不同时间,采用相同的方 法对同一另件的同一特性测量的结果,其 相互接近的程度。
—— ISO 5725-1
再现性
不同的测量人员 不同/相同一量具 相同另件的 同一特性
3、测量系统(Measurement System)
用以对被测特性赋值的作业、方法、
步
骤、量具、设备、软件、人员
的集合。
为获得测量结果的完整过程。 ——引自QS 9000参考手册
测量系统的要素:
测量方法 测量环境 仪器设备
测量系统 被测量对 测量人员 计量基准 象的特征
测量系统的组成:
传感器:感受被测物理量/特征量的变化(长度、 温度、重量、磁场、均匀性、舒适度等)
转换器:物理量/特征量的转换/放大(磁-电、 光-电、热-电等)
读 出:模拟显示、数字显示、磁记录、观测记 录等(显示器、记录器、观测人员等)
阻尼器:减少测量系统的高频振荡,有助于测 量结果的平稳输出(滤波、磨擦、阻 抗等)
记录员记录读数。 上述循环重复3遍,测量顺序打乱。
(a)确定重复性 Repeatability
选取样件(n)和评价人(r) 确定测量次数(m) 重复测量并记录(xijk)i=1,…,r
j=1,…,n k=1,…,m
重复性计算:
子组极差: Rij max{xij1,...,xijm} min{xij1,...,xijm}
测量注意事项:
盲测——随机抽取 估读——最小刻度的1/2 记录——防止混淆
(1)小样法实例
另件号 1 2 3 4 5
测量系统分析(MSA)

测量系统分析(MSA)测量系统可分为“计数型”及“计量型”测量系统两类。
测量后能够给出连续性的测量数值的为计量型测量系统;而只能定性地给出测量结果的为计数型测量系统。
“计量型”测量系统分析通常包括(Bias)、稳定性(Stability)、(Linearity)、以及重复性和再现性(Repeatability&Reproducibility,简称R&R)。
在测量系统分析的实际运作中可同时进行,亦可选项进行,根据具体使用情况确定。
测量:是指以确定实体或系统的量值大小为目标的一整套作业。
我们通常用分辨力、偏倚、稳定性、线性、重复性和再现性等评价测量系统的优劣,并用它们控制测量系统的偏倚和波动,以使测量获得的数据准确可靠。
有效测量的十原则:1.确定测量的目的及用途。
一个尤其重要的例子就是测量在质量改进中的应用。
在进行最终测量的同时,还必须包括用于诊断的过程间测量。
2.强调与顾客相关的测量,这里的顾客包括内部顾客与外部顾客。
3.聚集于有用的测量,而非易实现的测量。
当量化很困难时,利用替代的测量至少可以提供关于输出的部分理解。
4.在从计划到执行测量的全程中,提供各个层面上的参与。
那些不使用的测量最终会被忽略。
5.使测量尽量与其相关的活动同时执行,因为时效性对于诊断与决策是有益的。
6.不仅要提供当期指标,同时还要包括先行指标和滞后指标。
对现在及以前的测量固然必要,但先行指标有助于对未来的预测。
7.提前制订数据采集、存储、分析及展示的计划。
8.对数据记录、分析及展示的方法进行简化。
简单的检查表、数据编码、自动测量等都非常有用,图表展示的方法尤为有用。
9.测量的准确性、完整性与可用进行阶段评估。
其中,可用性包括相关性、可理解性、详细程度、可读性以及可解释性。
10.要认识到只通过测量是无法改进产品及过程。
基本概念:3.稳定性:测量系统保持其位置变差和宽度变差随时间恒定的能力。
4.偏倚:观测平均值(在重复条件下的测量)与一参考值之间的差值。
测量系统分析(MSA)
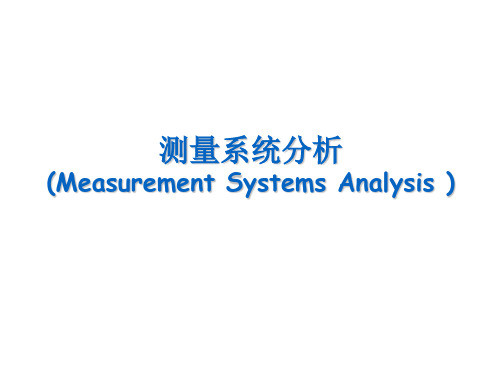
稳定性好
真值 时间 1
时间 1
真值
稳定性差
时间 2
时间2
时间 3
时间3
Y的测量系统评价 对散布的评价
- 精密度 : 根据测量系统反复性和再现性的总变动
- 反复性 : 重新测量也有相同的结果吗 ?
- 再现性 : 用其他测量系统也有相同的结果吗 ?
Y的测量系统评价
精密度
- 测量系统中的总散布 术语: 随机误差( Random Error ), 分散( Spread ), 测试/再测试误差( Test/Retest error ) 重复性和再现性
据的信赖性,通过研究测量系统所发生的 Nhomakorabea动对工程散布的影响,从 而判断该测量系统的适合性
MSA 概要
测量系统评价的重要性
1.测量数据 1)作为分析判断的基本依据,有必要评价其信赖性; 2)依据测量系统进行观测和评价
2.测量系统的分析 是6SIGMA活动的最基本的工作和最重要的部分之一
3.测量系统分析被强调的原因 1)所有的产品通常都是由许多部件构成的; 2)产品的小型化趋势使产品的误差界限缩小; 3)部件更换或组装时通常要求有互换性; 4)为了能大量生产,通常有增大自动组装的必要性
计量型数据的 Gage R&R P/T 比
P / T = 5.15*s MS
Tolerance
一般用 %表现
说明有多少百分比的公差 由测量误差所占据
包括重复性和再现性
作为目标,我们追求 P/T < 30%
注意 : 5.15标准偏差占测量系统散布的 99%. 5.15是产业标准.
计量型数据的 Gage R&R
70
80
Process
测量系统分析第四版
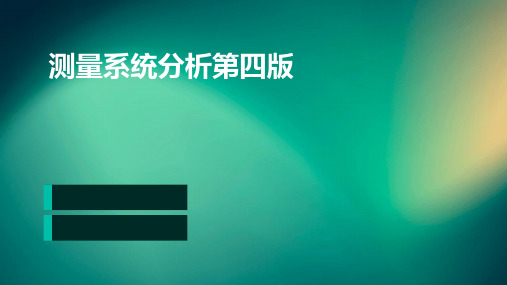
在实际应用中,测量系统分析 将更加注重与具体行业的结合 ,为解决行业内的复杂测量问 题提供定制化的解决方案。
此外,随着全球化的不断深入 ,测量系统分析的国际交流和 合作也将进一步加强,促进测 量系统分析领域的共同进步和 发展。
THANKS FOR WATCHING
感谢您的观看
线性度
表示测量系统在测量范围内是否呈线性关系。线性度越高, 表示测量系统在测量范围内响应与输入呈线性关系,误差 越小。
非线性误差
非线性误差是由于测量系统的非线性响应引起的误差,可 以通过拟合直线的方法来评估非线性误差的大小。
校准函数
校准函数是描述测量系统响应与输入之间关系的函数,通 常是一个多项式函数。校准函数可以用来评估线性度和非 线性误差。
未来趋势
随着数字化和智能化技术的不断发展,未来的测量系统将更加自动化、智能化和集成化。 同时,随着数据分析技术的不断发展,测量系统分析将更加依赖于数据挖掘和机器学习等 技术来提高评估的准确性和效率。
02 测量系统的基本组成
测量对象
01
测量对象是测量系统所要测量的实体或事物,可以是物理量、 化学量、生物量等。
测量系统分析的重要性
提高产品质量
降低生产成本
测量系统是产品质量控制中的重要环节, 一个高质量的测量系统能够提供准确的测 量结果,从而保证产品的质量和稳定性。
通过优化测量系统,可以减少生产过程中 的重复测量和返工,从而降低生产成本。
提高生产效率
增强企业竞争力
一个稳定、可靠的测量系统可以减少生产 过程中的等待和调试时间,从而提高生产 效率。
在质量控制中,测量系统分析 还可以用于评估生产过程中的 控制参数,以确保生产过程的 稳定性和产品质量。
通用测量系统指南(MSA)分析、评定测量系统的方法

分析/评定测量系统的方法测量系统变差的类型:●偏倚●重复性●再现性●稳定性●线性偏倚:●定义:值。
又称为“准确度”。
注:基准值可通过更高级别的测量设备进行多次测量取平均值。
●确定方法:1)在工具室或全尺寸检验设备上对一个基准件进行精密测量;2)让一位评价人用正被评价的量具测量同一零件至少10次;3)计算读数的平均值。
●偏倚原因:1)基准的误差;2)磨损的零件;3)制造的仪器尺寸不对;4)仪器测量非代表性的特性;5)仪器没有正确校准;6)评价人员使用仪器不正确。
●定义:次测量同一零件的同一特性时获得的测量值变差。
测量过程的重复性意味着测量系统自身的变异是一致的。
●确定方法:1)采用极差图;2)如果极差图受控,则仪器变差及测量过程在研究期间是一致的;3)重复性标准偏差或仪器变差距(σe)的估计为R/d2*;4)仪器变差或重复性将为5.15R/d2*或4.65 R;注(假定为两次重复测量,评价人数乘以零件数量大于15)5)此时代表正态分布测量结果的99%。
●极差图失控:1)调查识别为失控不一致性原因加以纠正;2)例外:当测量系统分辨率不足时。
●定义:测量同一零件的同一特性时测量平均值的变差。
●确定方法:1)确定每一评价人所有平均值;2)从评价人最大平均值减去最小的得到极差(R0)来估计;3)再现性的标准偏差(σ0)估计为R0/d2*;4)再现性为5.15R0/d2*或3.65 R0;5)代表正态分布测量结果的99%。
定义:Array是测量系统在某持续时间内测量同一基准或零件的单一特性时获得的测量值总变差。
零件间变差:●定义:――零件间固有的差异;――不包含测量的变差。
●确定方法:使用均值控制图:1)子组平均值反映出零件间的差异;2)零件平均值的控制限值以重复性误差为基础,而不是零件间的变差;3)没有一个子组平均值在这些限值之外,则零件间变差隐蔽在重复性中,测量变差支配着过程变差,如果这些零件用来代表过程变差,则此测量系统用于分析过程是不可接受的;4)如果越多的平均值落在限值之外,该测量越有用。
实验室测量系统的准确度评价方法
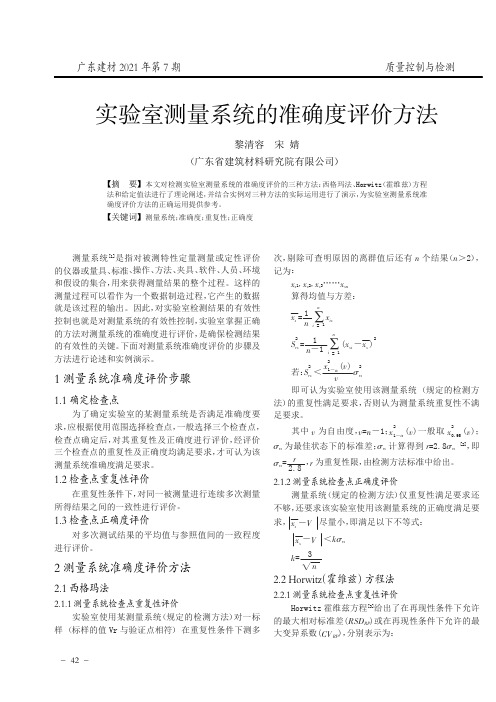
由公式 RSDRP=2(1-0.51gw)%,w=Vr=0.163%,计算得到:
RSDRP=2.63%
相对标准差的判定值
R
SDrP=(
2 3
)R SDRP=1.75%
测量结果的相对标准差 1.60%小于判定值 1.75%,
故实验室该测量系统在测碳含量为 0.163%点的重复性
合格。
同理,可以计算得出:
4 结束语
为 0.212%点及 0.45%这两个检查点的重复性合格。
结合实例对实验室测量系统的准确度评价方法进
对所选定各检查点均满足要求的重复性,因此,实 行了演示,结果表明三种方法获得的评价结果一致,三
验室该测量系统的重复性合格。
种方法对实验室测量系统的准确度评价有较好的适用
3.2 采用正确度 t 检验判断测量系统的正确度
用该测量系统在重复性条件下测试三个标样,每个 标样测试六次,结果如表 1。
表 1 测试结果
标样 碳含量 V r
六次测试结果
平均值xs
1
0.163
0.162、0.158、0.164、 0.160、0.162、0.165
0.162
2
0.212
0.220、0.215、0.213、 0.211、0.212、0.214
0.214
3
0.45
0.446、0.454、0.442、 0.450、0.455、0.448
0.449
(%) 标准差 srs
0.0026 0.0032 0.0049
3.1 采用西格玛法进行评价
标准 GB/T 4336 中给出用测量系统测钢材中碳含
量的重复性限函数为:
lgr=0.6648lgm-1.7576 m=V r 计算得到:
测量系统分析方法
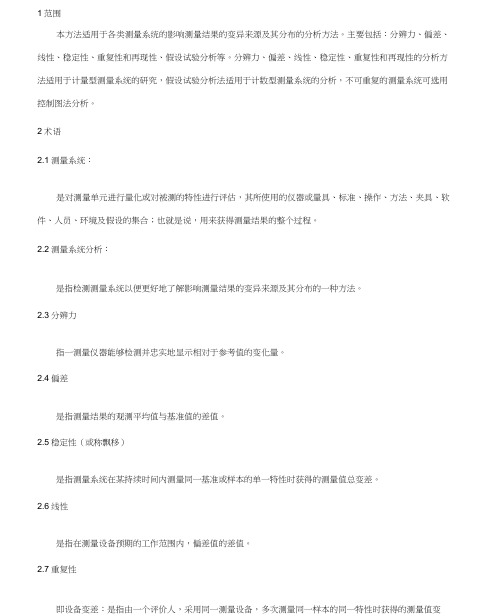
本方法适用于各类测量系统的影响测量结果的变异来源及其分布的分析方法。
主要包括:分辨力、偏差、线性、稳定性、重复性和再现性、假设试验分析等。
分辨力、偏差、线性、稳定性、重复性和再现性的分析方法适用于计量型测量系统的研究,假设试验分析法适用于计数型测量系统的分析,不可重复的测量系统可选用控制图法分析。
2术语2.1测量系统:是对测量单元进行量化或对被测的特性进行评估,其所使用的仪器或量具、标准、操作、方法、夹具、软件、人员、环境及假设的集合;也就是说,用来获得测量结果的整个过程。
2.2测量系统分析:是指检测测量系统以便更好地了解影响测量结果的变异来源及其分布的一种方法。
2.3分辨力指一测量仪器能够检测并忠实地显示相对于参考值的变化量。
2.4偏差是指测量结果的观测平均值与基准值的差值。
2.5稳定性(或称飘移)是指测量系统在某持续时间内测量同一基准或样本的单一特性时获得的测量值总变差。
2.6线性是指在测量设备预期的工作范围内,偏差值的差值。
2.7重复性即设备变差:是指由一个评价人,采用同一测量设备,多次测量同一样本的同一特性时获得的测量值变2.8 再现性即评价人变差:是指由不同的评价人,采用同一测量设备,测量同一样本的同一特性时获得的测量平均值变差。
2.9 计数型测量系统测量数值为一有限的分类数量的测量系统。
2.10计量型测量系统能获得一连串数值结果的测量系统。
3准备工作3.1应该事先决定好测量员数量,测量样本的数量及重复测量的次数。
3.2测量员应该从那些平时经常操作测量设备的人中选出。
3.3测试的样本必须从流程测量中选出,并代表该流程的控制范围,每个样本应被看作代表产品偏差的整个范围来进行分析的,每个样本将会进行多次测量,为了便于认别每个样本,必须对它们进行编号。
3.4按照指定的测量程序,确保测量方式正确。
3.5所有的分析方法都应确保每次读数的统计独立性,为了减少可能得出的错误的结果,应该采取下列步骤:a)测量必须是随机进行,以确保在分析研究中任何测岀的偏差或改变随机分布。
- 1、下载文档前请自行甄别文档内容的完整性,平台不提供额外的编辑、内容补充、找答案等附加服务。
- 2、"仅部分预览"的文档,不可在线预览部分如存在完整性等问题,可反馈申请退款(可完整预览的文档不适用该条件!)。
- 3、如文档侵犯您的权益,请联系客服反馈,我们会尽快为您处理(人工客服工作时间:9:00-18:30)。
• 由重复值的 Pooled standard deviation 估计所得 • Repeatability总是比系统的总变异要小
再现性变异
• 在不同状况下进行测量所得之变异
- 不同操作者 - 不同设定 - 不同测试零件 - 不同环境条件状态 - 长期
Operator-Part Interaction Plot
Operator
1 2 3
Average
1.1 1.0 0.9 0.8 0.7 0.6 0.5 0.4
Sample
◆ ■ ▲
1 2 3
4 5 6 7
8 9 10
• 每一条线代表每一位操作员对每个零件所测量的平均值 • 若不同操作员的线有交点,则存在显著的交互作用 • 我们希望所有操作员的线是互相平行的 • 若Operator-Pat interactions 确实存在,我们必须了解并加以解决
测量系统的误差: 测量系统的误差: 偏差、重复性、再现性、稳定性、和线性. ★ 偏差、重复性、再现性、稳定性、和线性.
鉴别力
•分辨率是:检测特性的微小的变化的能力 . 分辨率是: 分辨率是
Discrimination
•当仪器不能辨别工件偏差或确定单个零件特性数值偏差时,分辨率 当仪器不能辨别工件偏差或确定单个零件特性数值偏差时, 当仪器不能辨别工件偏差或确定单个零件特性数值偏差时 不可以接受. 不可以接受. •不能够检测工艺偏差和特殊原因造成的偏差. 不能够检测工艺偏差和特殊原因造成的偏差. 不能够检测工艺偏差和特殊原因造成的偏差
杨劲松
2003、 2003、3、12
目 录
测量系统的评价( 测量系统的评价(MSA) ) ★ ★ ★ ★ 测量系统的概念 离散型数据评价 连续型数据评价 破坏性实验评价
项目追踪
项目叙述 Process Map 流程图 C & E Matrix 因 果矩阵 初步的 FMEA MSA 测量系统分析
测量 MEASURE
- 如果这样,并且 R-Chart 是在控制中,那么我们就可以确 定有多少百分比的变异是由测量系统所造成的
R-Chart 指标
• 探讨不充分的览别力,如果
- Range Chart 在控制指标中少于4个显著的level - 该全距中存在4个以上的显著level,但有1/4的值为0
• 若Range Chart显示out-of-control,则此测量系统的重复 性(repeatability)是有问题的 • 若某一操作员的全距为out-of-control而其他操作人员不 是如此,则该测量方法令人质疑 • 若某一操作员之全距皆为out-of-control,则该系统对操 作员的技术非常敏感
● 测量系统%R&R值 和 测量系统%R& P/T比率,直观反映该 比率, 测量系统的变异量在总 变异量中所占比率。 变异量中所占比率。 ● 检测选用的测量系统 所必需的样品是否合 包括取样数、 适。( 包括取样数、 样品规格变异量等 )
●说明样品变异性如何. 说明样品变异性如何.
150 100 50 0
1.1 1 0.9 0.8 0.7 0.6 0.5 0.4 0.3
●考察操作者和样品之间 的交互影响作用. 的交互影响作用. (希望:每条线平行,而 希望:每条线平行, 且不交叉才好; 且不交叉才好; 最好各条线全 部重合。) 部重合。) ● 考察操作者的差异. 考察操作者的差异.
1.1 1.0 0.9 0.8 0.7 0.6 0.5 0.4 1 2 3 4 5 6 7 8 9 10
Xbar/R 表 辨别力=.001
UCL=0.1444 Mean=0.1397
0.145
0.140
0.135 0 5 10 15 20 25
LCL=0.1350
0.02 UCL=0.01717 0.01
R=0.00812
LCL=0
0.00
Distinct Data Categories
LSL USL
Sample Range
R Chart by Operato
0.15
3 .0SL=0.1252
Sample Range
0.10 0.05 0.00
— R=0.03833 -3.0SL=0.00E+00
• Range chart 有助于确认不充分的览别力 • 我们希望在控制指标中至少有4个资料点
—
X-Chart 指标
.28 .279 .2794 .28 .282 .2822
直 尺 卡 尺 千 分 尺
.28 .282 .2819
.28 .279 .2791
鉴别力
系统所能量测出的小数位数。测量渐进单位应为产品 规格或流程变异计量单位的10分之1 鉴别力不足
1 2 3 4 5
鉴别力良好
1 2 3 4 5
测量的鉴别力
检验员 A 检验员 B 检验员 C 检验员B 检验员B 检验员C 检验员C 检验员 A
• 从不同测量状况所得测量值平均数的标准差估计所得
再现性变异
由不同人员操作相同或不同的仪器,测量同一特性所 得的测量平均值的差异
Master Value
良好 Repeatability
不良 Repeatability
流程变异的总和
产品变异 (真正的变异)
测量变异
总变异 ( 观察所得变异)
变异的基本模式
2
产品变异
σ
2
=σ 总体变异 =σ 测量变异
+ σ
2
测量系统变异
σ
2
2
重复性
+ σ
2
再现性
Variables (连续型 ) MSA 分析 连续型
两种常见资料形态
ATTRIBUTE - 不连续的,计数资料 如: 1 , 2 , 3 , 4 等… 好/坏 机器 1 , 2 , 3 … VARIABLES - 连续性的,计量资料 如: 重量 =10.2 磅 厚度 =11.211 口 寸
Source Total Gage R&R Repeatability Reproducibility Operator Oper*Part Part-To-Part Total Variation %Contribution 10.97 3.10 7.56 2.19 5.37 89.33 100.00 %StdDev 32.66 17.62 27.50 14.81 23.17 94.52 100.00 %Tolerance 68.61 37.02 57.77 31.11 48.68 198.56 210.08
1.50 1.00 0.50 0.00 1 2 3 4 5 6 7 8
(考察:所有样品的纵向 考察: 波动范围) 波动范围) ●说明同一样品不同测试 下的差异度。 下的差异度。 (考察:同一样品的数据 考察: 点的重合性)
测量系统 重复 再现
样品
Minitab – 输出
% R&R %P/T
Gage R&R
2.测量系统必须稳定: ★ 测量系统的变化应尽量排除特殊原因的干扰.
3.测量系统的误差在预期范围内要一致,并且对于所 测量的目标(产品或流程)而言是充分的.
测量系统的构成要素
• 测量工具:
- 硬件 - 软件
• 使用这些工具的所有步骤 :
- 选择测量员 - 设定并执行各步骤 - 离线计算及资料登录 - 校准频率及技术
100% Noise LSL USL
Attribute Data LSL USL
Low Grade Variable Data
MWCC6sigma
控制和分析活动中流程分布的不同类型数目的影响
种类的数目 控制
可以用于控制,只有当: 1)当流程变化与规格 比较时,其值小 2)在预期流程变化范 围内,损失功效是 扁平的 3)变化的主源引起平 均变化 1)基于流程分布,可以 使用半变量控制技术 2)可以产生非敏感变量 控制图
Components of Variation 变异的组成
150 100
Percent
% Total Var % Study Var % Toler
Xbar Chart by Operato
1.1 1 0.9 0.8 0.7 0.6 0.5 0.4 0.3
3 .0SL=0.8796 = X=0.8075 -3.0SL=0.7354
• 我们希望看到在X Chart 中的资料点超出控制指 之外的变化 • 这表示零件间的变异 • 如果没有则此样本可能未 包含生产过程的正常范围
• 若操作员间的平均值不同,则此测量系统的再现性 (reproducibility)是有问题的 • 我们希望有更多的平均值落于 control limits 之外, 对全部操作员而言是一致的
- 这表示变异多来自不同的零件,这是我们所要的
• 我们希望图中大多数的点落于 control limits 之外
测量系统变异(Ⅳ) 测量系统变异(
● 说明所取样品的测量 值波动区间。 值波动区间。 ●说明取样范围大小是否 合适, 合适,如果大部分点落 在控制线之外, 在控制线之外,则能近 似体现测量系统量程。 似体现测量系统量程。 Geger& Geger&R(ANOVA)for Response ANOVA)
●相邻两个样品的测量值 之差的波动表。 之差的波动表。 ●要求:符合品质管理图 要求: 表的判断标准. 表的判断标准.
0.15 0.10 0.05 0.00
1.1 1 0.9 0.8 0.7 0.6 0.5 0.4 1 2 3
(希望:每个操作者的数 希望: 据点集中才好; 据点集中才好; 不同操作者的 平均点连线是 直线才好。) 直线才好。)