外文文献资料(Canny 算子)
canny sobel算子
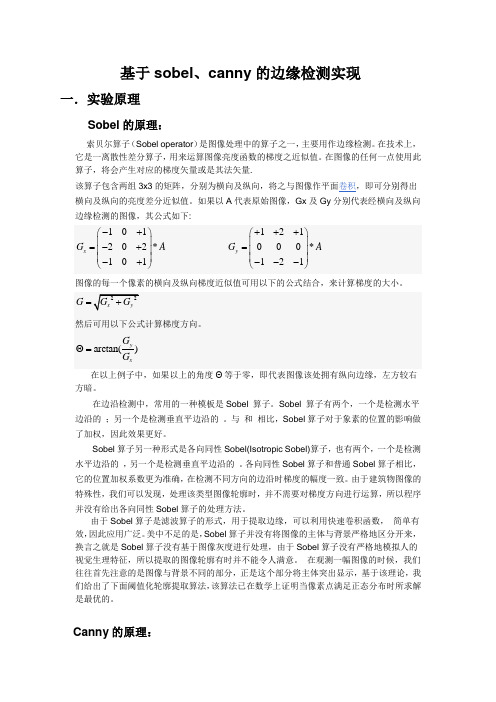
基于sobel 、canny 的边缘检测实现一.实验原理Sobel 的原理:索贝尔算子(Sobel operator )是图像处理中的算子之一,主要用作边缘检测。
在技术上,它是一离散性差分算子,用来运算图像亮度函数的梯度之近似值。
在图像的任何一点使用此算子,将会产生对应的梯度矢量或是其法矢量.该算子包含两组3x3的矩阵,分别为横向及纵向,将之与图像作平面卷积,即可分别得出横向及纵向的亮度差分近似值。
如果以A 代表原始图像,Gx 及Gy 分别代表经横向及纵向边缘检测的图像,其公式如下:101202*101x G A -+⎛⎫ ⎪=-+ ⎪ ⎪-+⎝⎭ 121000*121y G A +++⎛⎫ ⎪= ⎪ ⎪---⎝⎭图像的每一个像素的横向及纵向梯度近似值可用以下的公式结合,来计算梯度的大小。
在以上例子中,如果以上的角度Θ等于零,即代表图像该处拥有纵向边缘,左方较右方暗。
在边沿检测中,常用的一种模板是Sobel 算子。
Sobel 算子有两个,一个是检测水平边沿的 ;另一个是检测垂直平边沿的 。
与 和 相比,Sobel 算子对于象素的位置的影响做了加权,因此效果更好。
Sobel 算子另一种形式是各向同性Sobel(Isotropic Sobel)算子,也有两个,一个是检测水平边沿的 ,另一个是检测垂直平边沿的 。
各向同性Sobel 算子和普通Sobel 算子相比,它的位置加权系数更为准确,在检测不同方向的边沿时梯度的幅度一致。
由于建筑物图像的特殊性,我们可以发现,处理该类型图像轮廓时,并不需要对梯度方向进行运算,所以程序并没有给出各向同性Sobel 算子的处理方法。
由于Sobel 算子是滤波算子的形式,用于提取边缘,可以利用快速卷积函数, 简单有效,因此应用广泛。
美中不足的是,Sobel 算子并没有将图像的主体与背景严格地区分开来,换言之就是Sobel 算子没有基于图像灰度进行处理,由于Sobel 算子没有严格地模拟人的视觉生理特征,所以提取的图像轮廓有时并不能令人满意。
Canny算子
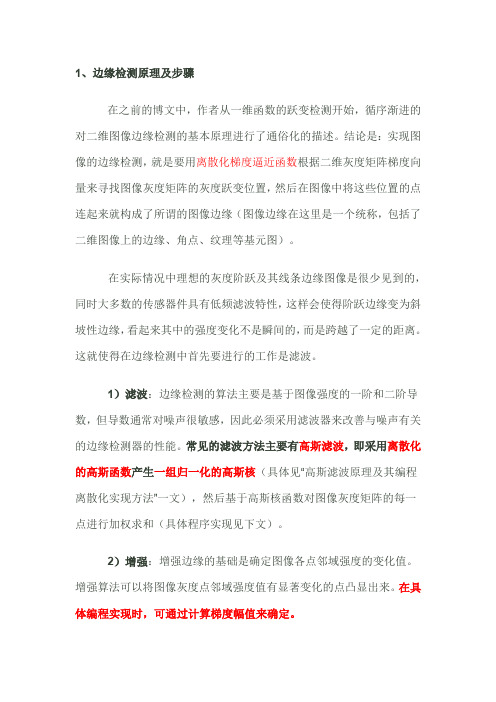
1、边缘检测原理及步骤在之前的博文中,作者从一维函数的跃变检测开始,循序渐进的对二维图像边缘检测的基本原理进行了通俗化的描述。
结论是:实现图像的边缘检测,就是要用离散化梯度逼近函数根据二维灰度矩阵梯度向量来寻找图像灰度矩阵的灰度跃变位置,然后在图像中将这些位置的点连起来就构成了所谓的图像边缘(图像边缘在这里是一个统称,包括了二维图像上的边缘、角点、纹理等基元图)。
在实际情况中理想的灰度阶跃及其线条边缘图像是很少见到的,同时大多数的传感器件具有低频滤波特性,这样会使得阶跃边缘变为斜坡性边缘,看起来其中的强度变化不是瞬间的,而是跨越了一定的距离。
这就使得在边缘检测中首先要进行的工作是滤波。
1)滤波:边缘检测的算法主要是基于图像强度的一阶和二阶导数,但导数通常对噪声很敏感,因此必须采用滤波器来改善与噪声有关的边缘检测器的性能。
常见的滤波方法主要有高斯滤波,即采用离散化的高斯函数产生一组归一化的高斯核(具体见“高斯滤波原理及其编程离散化实现方法”一文),然后基于高斯核函数对图像灰度矩阵的每一点进行加权求和(具体程序实现见下文)。
2)增强:增强边缘的基础是确定图像各点邻域强度的变化值。
增强算法可以将图像灰度点邻域强度值有显著变化的点凸显出来。
在具体编程实现时,可通过计算梯度幅值来确定。
3)检测:经过增强的图像,往往邻域中有很多点的梯度值比较大,而在特定的应用中,这些点并不是我们要找的边缘点,所以应该采用某种方法来对这些点进行取舍。
实际工程中,常用的方法是通过阈值化方法来检测。
2、Canny边缘检测算法原理JohnCanny于1986年提出Canny算子,它与Marr(LoG)边缘检测方法类似,也属于是先平滑后求导数的方法。
本节对根据上述的边缘检测过程对Canny检测算法的原理进行介绍。
2.1 对原始图像进行灰度化Canny算法通常处理的图像为灰度图,因此如果摄像机获取的是彩色图像,那首先就得进行灰度化。
对一幅彩色图进行灰度化,就是根据图像各个通道的采样值进行加权平均。
基于改进的Canny算子图像信息提取算法研究
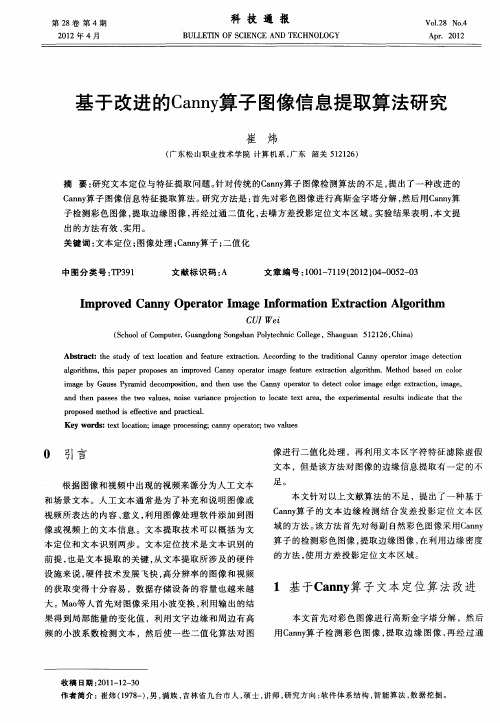
( 东 松 山职 业 技 术 学 院 计 算 机 系 , 东 韶关 5 2 2 ) 广 广 1 16
摘 要: 研究文 本定位 与特征提取 问题 。 针对传统 的C n y an算子 图像检测算法 的不足 , 提出了一种改进的 C ny a n算子 图像信息特征提取算法。 研究方法是 : 首先对彩色图像进行高斯金字塔分解 , 然后用C n y an 算
用 C n y 子 检 测 彩 色 图像 , 取 边 缘 图 像 , 经 过 通 an 算 提 再
1 基 于C n y a n 算子 文本 定 位 算 法 改进
频 的小波系数检测文本 ,然后使一些二值化算法对 图
收 稿 日期 : 0 1 1 — 0 2 1- 2 3
前 提 , 是 文 本 提 取 的关 键 , 文 本 提 取所 涉 及 的 硬 件 也 从
算子 的检测彩色 图像 , 提取边缘图像 , 利用边 缘密度 在
的方 法 , 用 方 差 投 影 定 位 文 本 区域 。 使
设 施来说 , 硬件技术发展飞快 , 高分辨率的图像和视频 的获取变得 十分容易 ,数据存储设备的容量也越来越 大。 o Ma等人首先对 图像采用小波变换 , 利用输 出的结 果得到局部能量的变化值 ,利用文字边缘和周边有高 本文首先对彩色 图像进行高斯金字塔分解 ,然后
I p o e n y Op r t rI g n o ma i n Ex r ci n Alo i m m r v d Ca n e a o ma e I f r t ta t g rt o o h
canny算子边缘检测原理

canny算子边缘检测原理
Canny算子是一种常用的边缘检测算法,其原理如下:
1. 高斯滤波:首先对图像进行高斯滤波,以减少噪声的影响。
高斯滤波是利用高斯函数对图像进行平滑操作,可以抑制高频噪声。
2. 计算梯度幅值和方向:对平滑后的图像进行梯度计算,通过计算像素点的梯度幅值和方向,可以找到图像中的边缘。
常用的梯度算子包括Sobel算子和Prewitt算子。
3. 非极大值抑制:在梯度图像中,对于每个像素点,通过比较其梯度方向上的两个相邻像素点的梯度幅值,将梯度幅值取最大值的点保留下来,其他点置为0。
这样可以剔除非边缘的像素。
4. 双阈值处理:将梯度幅值图像中的像素分为强边缘、弱边缘和非边缘三类。
设置两个阈值:高阈值和低阈值。
如果某个像素的梯度幅值大于高阈值,则将其标记为强边缘。
如果某个像素的梯度幅值小于低阈值,则将其剔除。
对于梯度幅值介于低阈值和高阈值之间的像素,如果其与某个强边缘像素相连,则将其标记为强边缘,否则将其标记为弱边缘。
5. 边缘连接:通过将强边缘和与其相连的弱边缘进行连接,找到完整的边缘。
这里通常使用8连通或4连通算法来判断两个像素是否相连。
通过以上步骤,Canny算子可以得到图像中的边缘信息,并且相对其他算法能够更好地抑制噪声和保持边缘的连续性。
边缘分割(Canny算子)
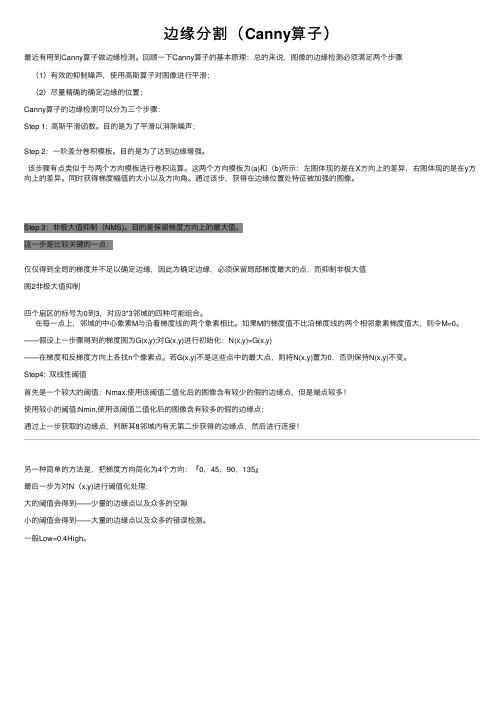
边缘分割(Canny算⼦)最近有⽤到Canny算⼦做边缘检测。
回顾⼀下Canny算⼦的基本原理:总的来说,图像的边缘检测必须满⾜两个步骤(1)有效的抑制噪声,使⽤⾼斯算⼦对图像进⾏平滑;(2)尽量精确的确定边缘的位置;Canny算⼦的边缘检测可以分为三个步骤:Step 1: ⾼斯平滑函数。
⽬的是为了平滑以消除噪声;Step 2:⼀阶差分卷积模板。
⽬的是为了达到边缘增强。
该步骤有点类似于与两个⽅向模板进⾏卷积运算。
这两个⽅向模板为(a)和(b)所⽰:左图体现的是在X⽅向上的差异,右图体现的是在y⽅向上的差异。
同时获得梯度幅值的⼤⼩以及⽅向⾓。
通过该步,获得在边缘位置处特征被加强的图像。
Step 3:⾮极⼤值抑制(NMS)。
⽬的是保留梯度⽅向上的最⼤值。
这⼀步是⽐较关键的⼀点:仅仅得到全局的梯度并不⾜以确定边缘,因此为确定边缘,必须保留局部梯度最⼤的点,⽽抑制⾮极⼤值图2⾮极⼤值抑制四个扇区的标号为0到3,对应3*3邻域的四种可能组合。
在每⼀点上,邻域的中⼼象素M与沿着梯度线的两个象素相⽐。
如果M的梯度值不⽐沿梯度线的两个相邻象素梯度值⼤,则令M=0。
——假设上⼀步骤得到的梯度图为G(x,y);对G(x,y)进⾏初始化:N(x,y)=G(x,y)——在梯度和反梯度⽅向上各找n个像素点。
若G(x,y)不是这些点中的最⼤点,则将N(x,y)置为0,否则保持N(x,y)不变。
Step4: 双线性阈值⾸先是⼀个较⼤的阈值:Nmax.使⽤该阈值⼆值化后的图像含有较少的假的边缘点,但是端点较多!使⽤较⼩的阈值:Nmin,使⽤该阈值⼆值化后的图像含有较多的假的边缘点;通过上⼀步获取的边缘点,判断其8邻域内有⽆第⼆步获得的边缘点,然后进⾏连接!另⼀种简单的⽅法是,把梯度⽅向简化为4个⽅向:『0,45,90,135』最后⼀步为对N(x,y)进⾏阈值化处理:⼤的阈值会得到——少量的边缘点以及众多的空隙⼩的阈值会得到——⼤量的边缘点以及众多的错误检测。
canny算子
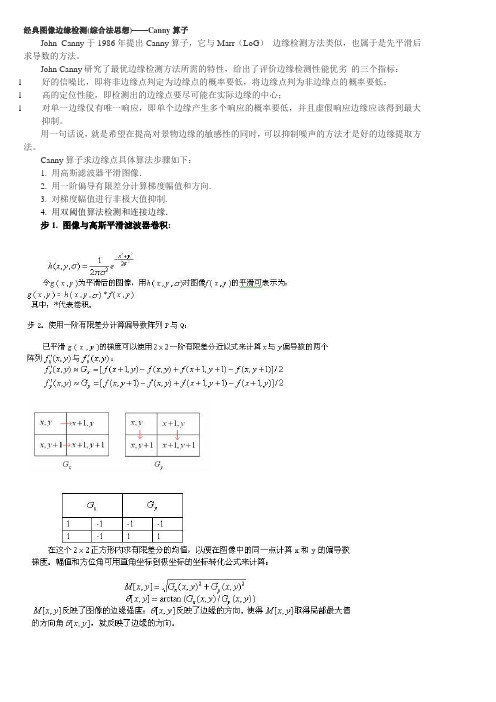
经典图像边缘检测(综合法思想)——Canny算子John Canny于1986年提出Canny算子,它与Marr(LoG)边缘检测方法类似,也属于是先平滑后求导数的方法。
John Canny研究了最优边缘检测方法所需的特性,给出了评价边缘检测性能优劣的三个指标:l好的信噪比,即将非边缘点判定为边缘点的概率要低,将边缘点判为非边缘点的概率要低;l高的定位性能,即检测出的边缘点要尽可能在实际边缘的中心;l对单一边缘仅有唯一响应,即单个边缘产生多个响应的概率要低,并且虚假响应边缘应该得到最大抑制。
用一句话说,就是希望在提高对景物边缘的敏感性的同时,可以抑制噪声的方法才是好的边缘提取方法。
Canny算子求边缘点具体算法步骤如下:1. 用高斯滤波器平滑图像.2. 用一阶偏导有限差分计算梯度幅值和方向.3. 对梯度幅值进行非极大值抑制.4. 用双阈值算法检测和连接边缘.步1. 图像与高斯平滑滤波器卷积:步3. 对梯度幅值进行非极大值抑制(non_maxima suppression,NMS):仅仅得到全局的梯度并不足以确定边缘,因此为确定边缘,必须保留局部梯度最大的点,而抑制非极大值。
解决方法:利用梯度的方向:步4. 用双阈值算法检测和连接边缘:对非极大值抑制图像作用两个阈值th1和th2,两者关系th1=0.4th2。
我们把梯度值小于th1的像素的灰度值设为0,得到图像1。
然后把梯度值小于th2的像素的灰度值设为0,得到图像2。
由于图像2的阈值较高,去除大部分噪音,但同时也损失了有用的边缘信息。
而图像1的阈值较低,保留了较多的信息,我们可以以图像2为基础,以图像1为补充来连结图像的边缘。
链接边缘的具体步骤如下:对图像2进行扫描,当遇到一个非零灰度的像素p(x,y)时,跟踪以p(x,y)为开始点的轮廓线,直到轮廓线的终点q(x,y)。
考察图像1中与图像2中q(x,y)点位置对应的点s(x,y)的8邻近区域。
Canny算子在织物防水性能自动识别中的应用
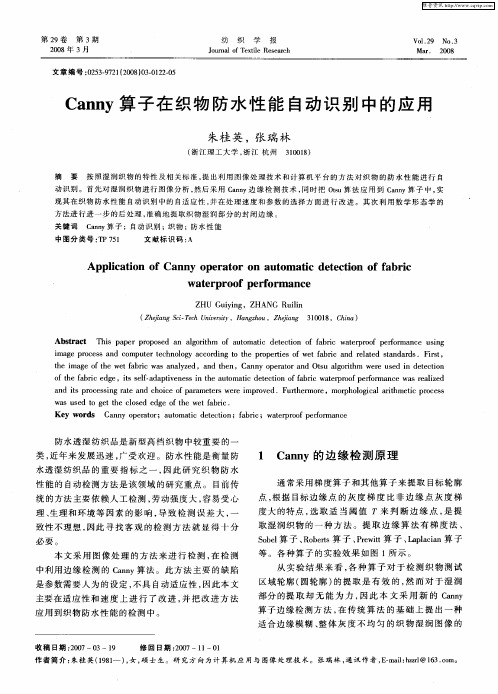
点 , 据 目标边 缘 点 的灰 度 梯 度 比非 边 缘 点灰 度 梯 根 度大 的特 点 , 取 适 当阈 值 来 判 断 边缘 点 , 提 选 是
统 的方 法 主要 依 赖人工 检测 , 劳动 强度 大 , 易受心 容
理、 生理 和环 境等 因素 的影 响 , 导致 检 测 误差 大 , 一
ZHU i i g, Z Gu y n HANG ii Ru l n
( hj n c— eh U i rt Z ea gS lTc nv sy,Ha gh u,Z 4a g 3 0 1  ̄ ei n zo h in 10 8,C ia) hn
Ab t a t T i a e rpoe n lo t m f a tmai ee to f fb c waep o f p roma c u i g s r c h s p p rp o s d a ag r h o u o tc d tcin o a r tr r o e r n e sn i i f i g r c s n o u e e h oo y a c r i g t h r p riso tfb c a d rlt d sa d r s.Fis , ma ep o e sa d c mp trtc n lg c od n o t e p o ete fwe a r n eae tn ad i rt t e i g ft ewe a rc wa n lz d,a d t e h ma e o h tfb sa ay e i n h n,Ca n p rtra d Otu ag rt n y o eao n s lo hm r s d i ee t n i wee u e n d tci o o h a rc e g ft ef i d e,i efa a tv n s n t e a tmai ee to ffb c wae ro roma c sr aie b t s l-d p ie e si h uo t d tcin o r tr o f f r n e wa e l d s c ai p pe z a d isp o e sn ae a d c oc fp r mee swee i r v d.F rh r r n t r c si gr t n h ie o aa t r r mp o e u emoe,mo h lg c la t mei rc s t p r oo ia r h t p o e s i c wa s d t e h ls d e g ft e w tfb c. su e o g tt e coe d e o h e a r i K e r s Ca n p rtr u o tc d tci n;fb c;wae ro e o a c y wo d n y o e ao ;a tmai ee t o ar i tr o fp r r n e p f m
基于canny算子的边缘检测算法应用研究
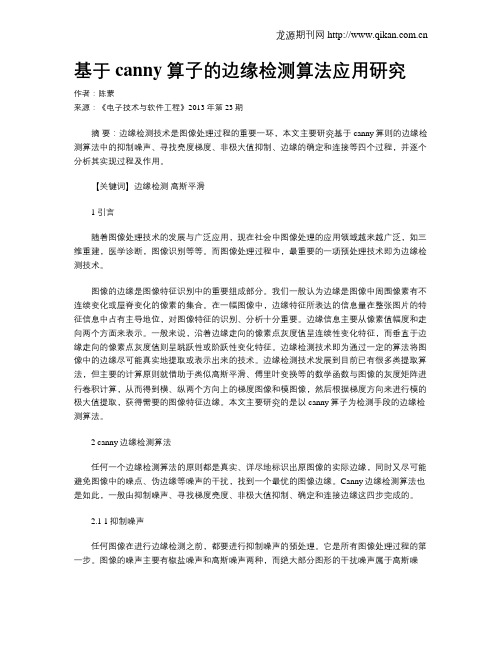
基于canny算子的边缘检测算法应用研究作者:陈蒙来源:《电子技术与软件工程》2013年第23期摘要:边缘检测技术是图像处理过程的重要一环,本文主要研究基于canny算则的边缘检测算法中的抑制噪声、寻找亮度梯度、非极大值抑制、边缘的确定和连接等四个过程,并逐个分析其实现过程及作用。
【关键词】边缘检测高斯平滑1 引言随着图像处理技术的发展与广泛应用,现在社会中图像处理的应用领域越来越广泛,如三维重建,医学诊断,图像识别等等。
而图像处理过程中,最重要的一项预处理技术即为边缘检测技术。
图像的边缘是图像特征识别中的重要组成部分。
我们一般认为边缘是图像中周围像素有不连续变化或屋脊变化的像素的集合。
在一幅图像中,边缘特征所表达的信息量在整张图片的特征信息中占有主导地位,对图像特征的识别、分析十分重要。
边缘信息主要从像素值幅度和走向两个方面来表示。
一般来说,沿着边缘走向的像素点灰度值呈连续性变化特征,而垂直于边缘走向的像素点灰度值则呈跳跃性或阶跃性变化特征。
边缘检测技术即为通过一定的算法将图像中的边缘尽可能真实地提取或表示出来的技术。
边缘检测技术发展到目前已有很多类提取算法,但主要的计算原则就借助于类似高斯平滑、傅里叶变换等的数学函数与图像的灰度矩阵进行卷积计算,从而得到横、纵两个方向上的梯度图像和模图像,然后根据梯度方向来进行模的极大值提取,获得需要的图像特征边缘。
本文主要研究的是以canny算子为检测手段的边缘检测算法。
2 canny边缘检测算法任何一个边缘检测算法的原则都是真实、详尽地标识出原图像的实际边缘,同时又尽可能避免图像中的噪点、伪边缘等噪声的干扰,找到一个最优的图像边缘。
Canny边缘检测算法也是如此,一般由抑制噪声、寻找梯度亮度、非极大值抑制、确定和连接边缘这四步完成的。
2.1 1抑制噪声任何图像在进行边缘检测之前,都要进行抑制噪声的预处理。
它是所有图像处理过程的第一步。
图像的噪声主要有椒盐噪声和高斯噪声两种,而绝大部分图形的干扰噪声属于高斯噪声,因此canny算法的第一步采用的是运用二维高斯平滑模板与原图像数据进行卷积计算,而得到抑制噪声后的待处理图像。
Canny算子c++

Canny算⼦c++、功能简介及其运⾏(⼀)、功能:该程序实现了canny算⼦求图⽚的边缘,其中主要包括七⼤部分:1、对传⼊的彩⾊图⽚⼆值化2、对⼆值化后的图⽚进⾏⾼斯滤波3、使⽤sobel算⼦对滤波之后的图形分别求x,y⽅向上的梯度4、计算出梯度幅值5、对梯度图像做⾮极⼤抑制6、对⾮极⼤抑制后的图像做双阈值处理7、对双阈值处理后的图像进⾏连接操作(处理阈值中间的点)(⼆)、运⾏:注意要在release⽂件夹下运⾏exe运⾏程序后终端会显⽰所⽤到的sobel算⼦矩阵和⾼斯滤波矩阵,同时会弹出多张图⽚,他包括:1、原图⽚:Lena.jpg2、⼆值化后的图⽚:gray.jpg3、⾼斯滤波后的图⽚:guss,jpg4、x,y⽅向梯度的图⽚:gradit_x.jpg gradint_y.jpg5、梯度图⽚:gradint.jpg6、⾮极⼤抑制后的图⽚:afterNms.jpg7、双阈值后的图⽚:afterDT.jpg8、连接操作后的图⽚(即最终的canny算法图⽚)canny.jpg9、使⽤opencv⾃带函数得到的canny算⼦图⽚(⽤于对⽐)弹出的同时,会将这些图⽚保存在当前路径的⽂件下,命名为上⾯的命名。
⼆、开发环境操作系统:windowsOpencv版本:2.4编译器:vs2015语⾔:c++请助教运⾏release下的exe。
三、程序思路⼤体思路其实与上⾯七⼤部分相同,这⾥做⼀个概述,并说明⼤体实现,具体通过这个简单流程图来说明(流程图viso⽂件附在压缩包内))⼀、具体实现(1)图像灰度化:图像灰度画函数:Mat getGray(Mat &image)作⽤:返回⼀张彩⾊图⽚的灰度图⽚实现⽅式:才⽤标准的转化公式:Gray = R*0.299 + G*0.587 + B*0.114循环的取出每⼀个像素,对每⼀个彩⾊像素做该运算就可得到灰度图⽚。
(2)获取⾼斯核图像灰度画函数:double **getGussKernel(int size, double sigma)作⽤:返回⼀个⼆维double类型指针,⾥⾯是对应size和sigma的⾼斯卷积核实现⽅式:通过查阅资料和博客我们知道⾼斯卷积核公式:由这个公式,我们可以容易写出⼀下核⼼代码:最后记得要做归⼀化,之后返回指针g即可。
改进canny算子的亚像素定位算法
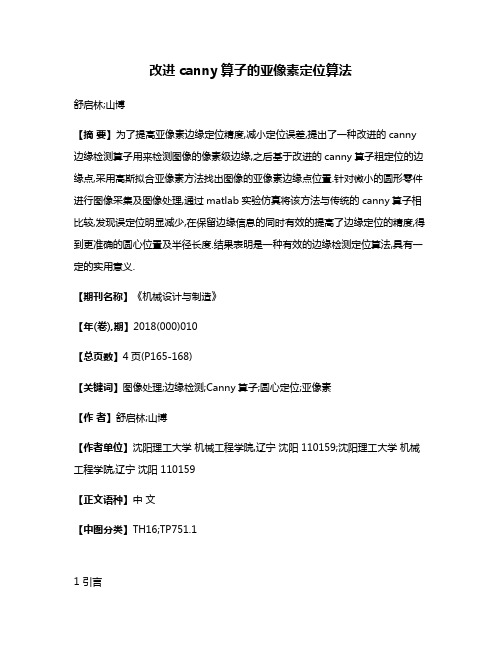
改进canny算子的亚像素定位算法舒启林;山博【摘要】为了提高亚像素边缘定位精度,减小定位误差,提出了一种改进的canny 边缘检测算子用来检测图像的像素级边缘,之后基于改进的canny算子粗定位的边缘点,采用高斯拟合亚像素方法找出图像的亚像素边缘点位置.针对微小的圆形零件进行图像采集及图像处理,通过matlab实验仿真将该方法与传统的canny算子相比较,发现误定位明显减少,在保留边缘信息的同时有效的提高了边缘定位的精度,得到更准确的圆心位置及半径长度.结果表明是一种有效的边缘检测定位算法,具有一定的实用意义.【期刊名称】《机械设计与制造》【年(卷),期】2018(000)010【总页数】4页(P165-168)【关键词】图像处理;边缘检测;Canny算子;圆心定位;亚像素【作者】舒启林;山博【作者单位】沈阳理工大学机械工程学院,辽宁沈阳 110159;沈阳理工大学机械工程学院,辽宁沈阳 110159【正文语种】中文【中图分类】TH16;TP751.11 引言在现代生产中,微小机械零件的应用越来越广泛,对于微小机械零件尺寸的测量要求也愈来越多。
经调查发现,当今国内市场上一些微小型的零件主要测量方式为传统的人工测量,传统的测量方法包括游标卡尺、千分尺、量规、百分表、千分表等测量方法,有些微小零件的测量甚至要借助显微镜进行,虽然操作起来比较直接,但测量过程中会存在一些问题,比如不易持握,测量过程中受人为因素和环境因素的影响较大,难以保证精度,更不要说速度,无法实现自动测量和非接触式测量。
图像测量技术具有非接触、动态测量范围大的特点,对于微小的被测机械零件可以通过调节摄像系统的放大倍数,方便的实现毫米量级、微米量级甚至纳米量级的参数测量。
而且只要能够保证采集图片的摄像系统有足够高的分辨率,就能通过算法实现较高的测量精度。
技术主要是通过对待加工零件的图像进行处理从而获得其尺寸及位置信息,其中,图像边缘信息检测是该技术的关键之处。
基于小波变换的Canny算子边缘检测算法

1 基于小波变换 的图像增强
1 1 二维小 波变 换分 析 .
设相 应 的尺度 函数 为 为 ( 小波 函数 为 ( 二维 尺度 函数 ( Y ), ), ,)是 可分离 的 . 即
( ) = ( ( ) , ) ) ) , , , () 1 () 2 () 3 () 4
在 的问题 , 作者 在原 有 C ny边缘 检测 算法 的基础 上 加 以改进 , 出了一种 适用 于低 对 比度 图像 的边 缘 an 提
检测方法. 首先利用 M lt a a小波变换对原始进行图像增强预处理 , l 提高图像灰度级的动态 范围; 然后采 用传统 的 C ny算子 进行 边缘 检测 , an 获得 最终 的输 出 .
D ( n =厂 ,) . ,) m,) ( , Y . , (
变换 来说 , 以通过 二维 M lt 可 a a快速算 法 来实 现 , 图像 处 理 中用 到 的二 l 在 维 小波 变换通 常都 是这 种类 型 的小波 变换 . 幅 N 的 图像 经过 一层 二 维 Maa快 速 算法 实 现 小波 一 xN lt l 分 解后 , 将得 到 4个大 小均 为 Ⅳ /2xN/2的子带 图像 , 即一 个逼 近 信 号 ( 应 着 C, 平 和垂 直方 向 对 水 均 为低 频分量 ) 3个 细节 信号 ( 和 对应 着上 面 的 D , D , 平 和垂直 方 向至少 有一 个高 频分 量 ) D , 水 .
维普资讯
3 6
上海师范大学学报(自然科学版 )
20 06正
那么, 二维 小波 基 可 以通 过 以下伸 缩 平移实 现 ( Y =2 J 2 J — m,~Y—n √, n ∈ Z, ,) - ̄ ( - h 2 ) m, i= 12,. , 3
基于Canny算子的焊缝图像边缘提取技术
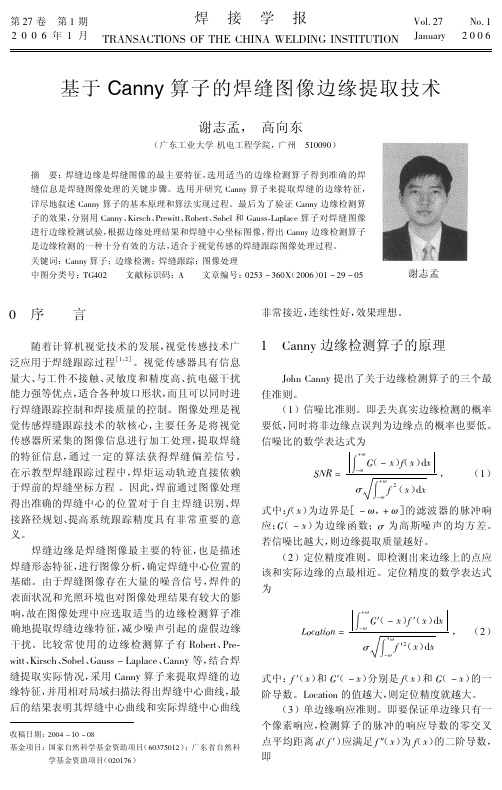
图1 Fig. 1
扇区示意图 Sector figure
( 4 )对梯度图像进行双阈值操作和边缘连接。 选用两个阈值 T1 和 T2 , T2 = 2 X T1 。对于经过非极 大值压抑处理的图像 ( L, J) , 如果像素点的梯度值 M ( L, J) 则直接把此像素点标记为边缘像素点; > T2 , 如像素点的梯度值 M ( L, J) 则把此像素点标记 < T1 , 为非边缘像素点; 如像素点的梯度值 T1 < M ( L, J )< T2 , 则标记此像素点为 “ 准像素点” 。在双阈值标记 完之后, 搜索图像中的 “ 准像素点” , 并选择其 8 个 邻域点的位置寻找是否有梯度值 M ( L, J) > T2 的点 存在。如存在, 则标记此像素点为边缘, 否则标记此 像素点为非边缘像素点。
dx 叫 f ( x) !
-!
Location =
叫
+!
-!
G' ( - x) f( ' x) dx dx 叫 f '( x) !
2 -! +!
, (2)
"
式中:f ( ' x) 和 G' ( - x) 分别是 ( f x) 和G ( - x) 的一 阶导数。Location 的值越大, 则定位精度就越大。 ( 3 )单边缘响应准则。即要保证单边缘只有一 个像素响应, 检测算子的脉冲的响应导数的零交叉 点平均距离 d ( f ') 应满足 f ( ~ x) 为( f x) 的二阶导数, 即
Canny 焊缝边缘检测算法的实现
根据 Canny 边缘检测原理, 可以得到相应的边
Robert、 SobeI 和 Gauss - LapIace 算子对图 2 的焊缝 原始图像进行边缘检测试验。在进行边缘检测前, 首先对原始图像进行中值滤波。边缘检测结果如 图 3 所示, 其中分图题表示算子名称。
外文文献资料(Canny-算子)
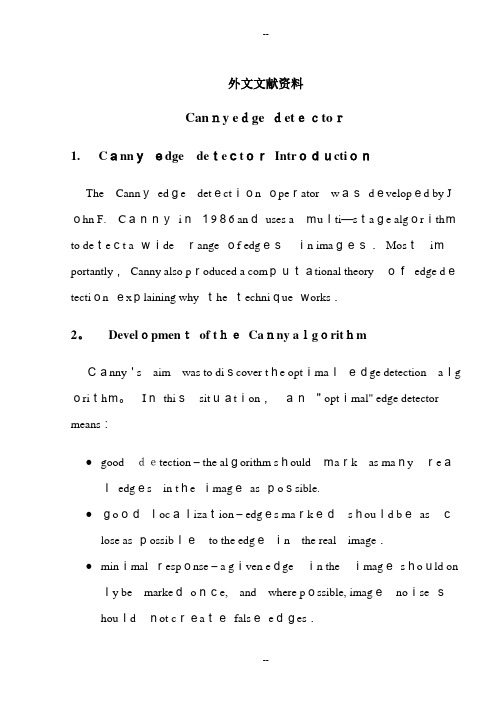
外文文献资料Canny edge detector1.Cannyedge detectorIntroductionThe Cannyedge detection operator wasdeveloped by J ohn F.Cannyin1986 anduses amulti—stage algorithmto detect a widerange of edgesin images.Mostimportantly,Canny also produced a computational theoryofedge detection explaining why the technique works.2。
Developmentof theCanny algorithmCanny's aim was to discover the optimaledge detection alg orithm。
Inthissituation,an"optimal" edge detector means:●gooddetection – the algorithm shouldmark as manyrealedges in the imageas possible.●goodlocalization – edges markedshould beasclose as possibleto the edgein the real image.●minimal response – a given edgein theimageshould only be markedonce,and where possible, imagenoise shouldnot createfalseedges.To satisfy these requirements Canny usedthe calculus ofvariations– a techniquewhich finds the function which optimizes a given functional. The optimal function in Canny's d etector isdescribedby the sum of four exponential terms,but canbe approximatedby the firstderivative of a Gaussian。
数学形态学与Canny算子在木材腐朽图像特征提取中的应用
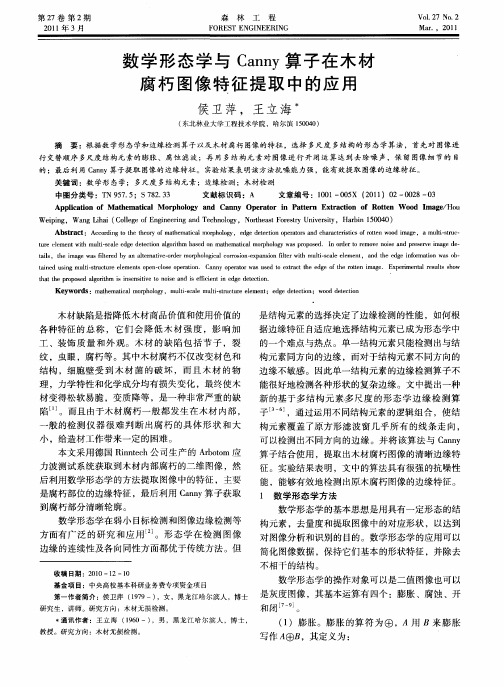
木 材缺 陷是 指降低 木材 商 品价值 和使 用价值 的 各种 特 征 的 总 称 ,它 们 会 降 低 木 材 强 度 ,影 响 加 工 、装 饰 质 量 和外 观 。木 材 的缺 陷 包 括 节 子 ,裂
是 结构 元素 的选择 决定 了边缘 检 测 的性 能 ,如何 根 据 边缘 特征 自适应 地选 择结 构元 素 已成 为形 态学 中 的一个 难点 与热 点 。单 一结 构元 素 只能检 测 出与结 构 元 素 同方 向的边缘 ,而对 于结 构元 素不 同方 向的
摘
要 :根 据数 学形态学和边缘检 测算子 以及木材腐朽 图像的特征 ,选择 多尺度 多结构的形 态学算法 ,首先对 图像进
行 交替顺序 多尺度结构元素的膨胀、腐蚀 滤 波 ;再 用多结构元 素对 图像进 行 开闭运 算达到 去除噪 声 ,保 留 图像 细节 的 目 的 ;最后利用 C n y算子提取 图像 的边缘特征 。实验 结果表 明该 方法抗噪 能力强,能有 效提取 图像 的边缘特征 。 an 关键词 :数学形 态学;多尺度 多结构元素 ;边缘检测 ;木材检 测 中图分类号 :T 5 . ;S7 2 3 N9 7 5 8 .3 文献标识码 :A 文章编号 :10 0 5 (0 1 2— 0 8— 3 0 1— 0 X 2 1 )O 0 2 0
Ke wo d :m te aia m rhlg ,m l cl m l-rc r e met ded t t n odd t t n y r s ahm t l op oo c y ut sa uts t e l n ;eg ee i ;w o ee i i e it u e u co co
A bsr c t a t: Ac odn otete r fmah maia rh lg , e g ee t no eaosa dc aa trsiso otnwo di g , amut src c r igt h h oyo te tclmop oo y d ed tci p rtr n h rceit frt o ma e li tu — o c e —
Canny算子与数学形态学算子相结合实现胸水细胞的边缘提取
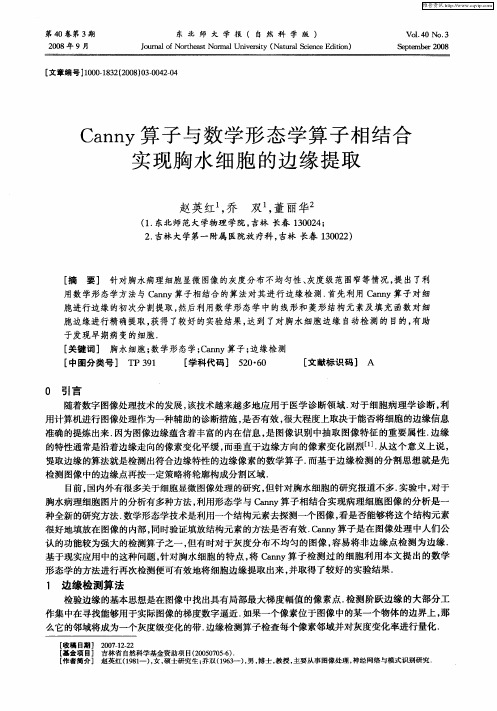
维普资讯
第3 期
赵英红 , : an 等 C n y算子与数学形 态学算 子相 结合 实现胸水 细胞 的边缘提取
4 3
C n y边 缘检 测算 法 步骤 如下 : an ( ) 高斯 滤波 器平 滑 图像 ; 1用 () 2 用一 阶偏 导 的有 限差 分来 计算 梯 度 的幅值 和方 向 ; () 3 对梯 度 幅值 进行 非极 大值 抑 制 ;
来具 体 解决 这个 问题 .
图 1 胸 水 细胞 灰 度 图像
图 2 C n y算子检测后 的图片 an
2 形 态 学 边缘 检 测
2 1 形 态学 基本 原理 及在 图像处 理 中的应 用 .
数 学形态 学 是一 门建 立在 严格 数 学理论 基 础上 的学科 , 基 本 思 想 和方 法 对 图像 处 理理 论 和技 术 其 产 生 了重大影 响 . 的基本 思 想是 用具 有一 定形 态 的结 构元 素度 量 和提 取 图像 中 的对应 形状 , 它 以达 到对 图像 的分 析 和识别 的 目的 . 学形态 学 的数 学基 础 是集 合 论 , 可 用 于 简化 图像 数 据 , 持 它们 基 本 的 数 它 保
很好 地 填放 在 图像 的 内部 , 同时验证 填放结 构元 素 的方 法是 否有 效 . an 子是 在 图像 处 理 中人 们 公 C ny算 认 的功 能较 为强 大 的检测算 子之 一 , 有 时对 于灰 度分 布 不 均匀 的 图像 , 但 容易 将 非 边 缘 点 检测 为 边 缘 .
Vl . 0 No 3 0 4 . 1 S pe e 0 8 e tmb r2 0
[ 文章编号 ]0 01 3 (0 8 0 —0 20 1 0 8 2 2 0 )304 —4
canny算子原理
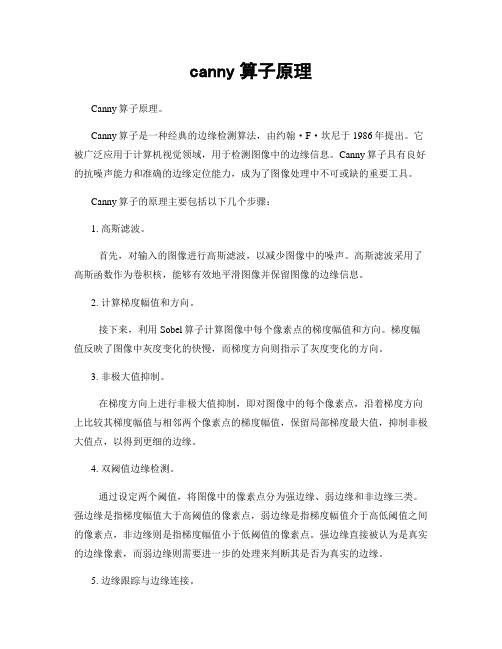
canny算子原理Canny算子原理。
Canny算子是一种经典的边缘检测算法,由约翰·F·坎尼于1986年提出。
它被广泛应用于计算机视觉领域,用于检测图像中的边缘信息。
Canny算子具有良好的抗噪声能力和准确的边缘定位能力,成为了图像处理中不可或缺的重要工具。
Canny算子的原理主要包括以下几个步骤:1. 高斯滤波。
首先,对输入的图像进行高斯滤波,以减少图像中的噪声。
高斯滤波采用了高斯函数作为卷积核,能够有效地平滑图像并保留图像的边缘信息。
2. 计算梯度幅值和方向。
接下来,利用Sobel算子计算图像中每个像素点的梯度幅值和方向。
梯度幅值反映了图像中灰度变化的快慢,而梯度方向则指示了灰度变化的方向。
3. 非极大值抑制。
在梯度方向上进行非极大值抑制,即对图像中的每个像素点,沿着梯度方向上比较其梯度幅值与相邻两个像素点的梯度幅值,保留局部梯度最大值,抑制非极大值点,以得到更细的边缘。
4. 双阈值边缘检测。
通过设定两个阈值,将图像中的像素点分为强边缘、弱边缘和非边缘三类。
强边缘是指梯度幅值大于高阈值的像素点,弱边缘是指梯度幅值介于高低阈值之间的像素点,非边缘则是指梯度幅值小于低阈值的像素点。
强边缘直接被认为是真实的边缘像素,而弱边缘则需要进一步的处理来判断其是否为真实的边缘。
5. 边缘跟踪与边缘连接。
最后,利用强边缘像素点进行边缘跟踪,将与强边缘像素点相邻的弱边缘像素点连接到边缘上,从而得到完整的边缘信息。
Canny算子通过以上几个步骤,能够有效地检测图像中的边缘信息,并且对噪声具有较好的抑制能力。
它在图像处理和计算机视觉领域有着广泛的应用,如人脸识别、目标检测、图像分割等方面都能看到它的身影。
总的来说,Canny算子是一种经典且高效的边缘检测算法,它的原理简单清晰,能够准确地提取图像中的边缘信息,因此在实际应用中具有重要的意义。
希望本文对Canny算子的原理有所帮助,让读者对其有一个更深入的理解。
canny算子原理

canny算子原理Canny算子是一种常用于边缘检测的算法,由John F. Canny于1986年提出。
Canny算子是一种基于梯度的算法,它可以在图像中检测出明显的边缘,并将其转化为二值图像,便于后续的处理。
Canny算子的原理主要包括以下几个步骤:1.高斯滤波在进行边缘检测之前,首先需要对原始图像进行高斯滤波,以去除图像中的噪声。
高斯滤波是一种线性平滑滤波器,它可以将图像中的噪声平滑化,同时保留图像中的细节信息。
2.计算梯度幅值和方向在进行边缘检测之前,需要对图像进行梯度计算,以确定图像中的边缘。
梯度是一个向量,它表示函数在某一点的变化率。
在图像中,梯度的方向指向最大变化的方向,梯度的大小表示变化的程度。
在Canny算子中,使用Sobel算子来计算梯度幅值和方向。
Sobel 算子是一种离散差分算子,它可以在图像中计算出每个像素点的梯度幅值和方向。
3.非极大值抑制由于图像中的梯度方向可能非常复杂,如何确定哪些像素点是边缘点是一个非常困难的问题。
为了解决这个问题,Canny算子采用了非极大值抑制的方法。
非极大值抑制的基本思想是,在梯度方向上,只有局部的最大值才可能是真正的边缘点。
因此,对于每个像素点,只有当其梯度方向上的幅值是局部最大值时,才会被保留下来。
4.双阈值处理在进行非极大值抑制之后,图像中的边缘已经被明显地检测出来了。
但是,由于图像中可能存在一些噪声,因此可能会出现一些假边缘。
为了解决这个问题,Canny算子采用了双阈值处理的方法。
双阈值处理的基本思想是,将图像中的像素点分为三类:强边缘、弱边缘和非边缘。
强边缘是指梯度幅值大于高阈值的像素点,非边缘是指梯度幅值小于低阈值的像素点,弱边缘是指梯度幅值在高低阈值之间的像素点。
在双阈值处理中,强边缘被保留下来,非边缘被丢弃,而弱边缘则需要进一步处理。
通常情况下,弱边缘会被与强边缘连接起来,形成完整的边缘。
5.边缘跟踪在进行双阈值处理之后,图像中的边缘已经被明显地检测出来了。
canny算法
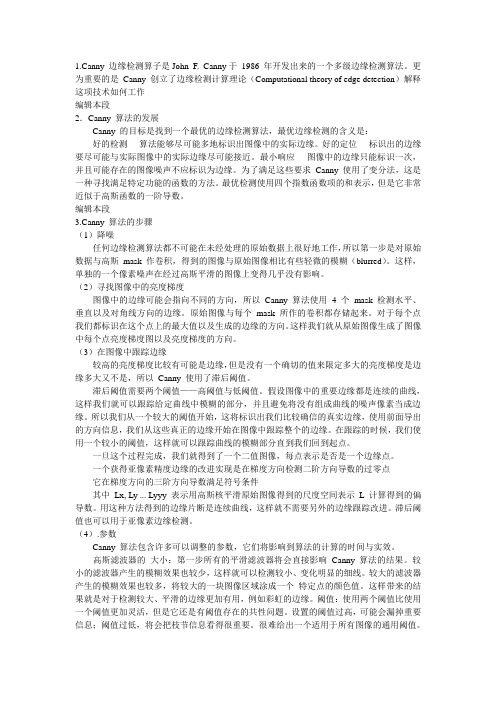
1.Canny 边缘检测算子是John F. Canny于1986 年开发出来的一个多级边缘检测算法。
更为重要的是Canny 创立了边缘检测计算理论(Computational theory of edge detection)解释这项技术如何工作编辑本段2.Canny 算法的发展Canny 的目标是找到一个最优的边缘检测算法,最优边缘检测的含义是:好的检测- 算法能够尽可能多地标识出图像中的实际边缘。
好的定位- 标识出的边缘要尽可能与实际图像中的实际边缘尽可能接近。
最小响应- 图像中的边缘只能标识一次,并且可能存在的图像噪声不应标识为边缘。
为了满足这些要求Canny 使用了变分法,这是一种寻找满足特定功能的函数的方法。
最优检测使用四个指数函数项的和表示,但是它非常近似于高斯函数的一阶导数。
编辑本段3.Canny 算法的步骤(1)降噪任何边缘检测算法都不可能在未经处理的原始数据上很好地工作,所以第一步是对原始数据与高斯mask 作卷积,得到的图像与原始图像相比有些轻微的模糊(blurred)。
这样,单独的一个像素噪声在经过高斯平滑的图像上变得几乎没有影响。
(2)寻找图像中的亮度梯度图像中的边缘可能会指向不同的方向,所以Canny 算法使用 4 个mask 检测水平、垂直以及对角线方向的边缘。
原始图像与每个mask 所作的卷积都存储起来。
对于每个点我们都标识在这个点上的最大值以及生成的边缘的方向。
这样我们就从原始图像生成了图像中每个点亮度梯度图以及亮度梯度的方向。
(3)在图像中跟踪边缘较高的亮度梯度比较有可能是边缘,但是没有一个确切的值来限定多大的亮度梯度是边缘多大又不是,所以Canny 使用了滞后阈值。
滞后阈值需要两个阈值——高阈值与低阈值。
假设图像中的重要边缘都是连续的曲线,这样我们就可以跟踪给定曲线中模糊的部分,并且避免将没有组成曲线的噪声像素当成边缘。
所以我们从一个较大的阈值开始,这将标识出我们比较确信的真实边缘,使用前面导出的方向信息,我们从这些真正的边缘开始在图像中跟踪整个的边缘。
- 1、下载文档前请自行甄别文档内容的完整性,平台不提供额外的编辑、内容补充、找答案等附加服务。
- 2、"仅部分预览"的文档,不可在线预览部分如存在完整性等问题,可反馈申请退款(可完整预览的文档不适用该条件!)。
- 3、如文档侵犯您的权益,请联系客服反馈,我们会尽快为您处理(人工客服工作时间:9:00-18:30)。
外文文献资料Canny edge detector1.Canny edge detector IntroductionThe Canny edge detection operator was developed by John F. Canny in 1986 and uses a multi-stage algorithm to detect a wide range of edges in images. Most importantly, Canny also produced a computational theory of edge detection explaining why the technique works.2. Development of the Canny algorithmCanny's aim was to discover the optimal edge detection algorithm. In this situation, an "optimal" edge detector means:●good detection – the algorithm should mark as many real edges in the imageas possible.●good localization – edges marked should be as close as possible to the edgein the real image.●minimal response – a given edge in the image should only be marked once,and where possible, image noise should not create false edges.To satisfy these requirements Canny used the calculus of variations – a technique which finds the function which optimizes a given functional. Theoptimal function in Canny's detector is described by the sum of four exponential terms, but can be approximated by the first derivative of a Gaussian.3. Stages of the Canny algorithm3.1 Noise reductionThe Canny edge detector uses a filter based on the first derivative of a Gaussian, because it is susceptible to noise present on raw unprocessed image data, so to begin with, the raw image is convolved with a Gaussian filter. The result is a slightly blurred version of the original which is not affected by a single noisy pixel to any significant degree.3.2 Finding the intensity gradient of the imageAn edge in an image may point in a variety of directions, so the Canny algorithm uses four filters to detect horizontal, vertical and diagonal edges in the blurred image. The edge detection operator (Roberts, Prewitt, Sobel for example) returns a value for the first derivative in the horizontal direction (Gy) and the vertical direction (Gx). From this the edge gradient and direction can be determined.3.3 Tracing edges through the image and hysteresis thresholdingIntensity gradients which are large are more likely to correspond to edges than if they are small. It is in most cases impossible to specify a threshold at which agiven intensity gradient switches from corresponding to an edge into not doing so. Therefore Canny uses thresholding with hysteresis.Thresholding with hysteresis requires two thresholds – high and low. Making the assumption that important edges should be along continuous curves in theimage allows us to follow a faint section of a given line and to discard a few noisy pixels that do not constitute a line but have produced large gradients. Therefore we begin by applying a high threshold. This marks out the edges we can be fairly sure are genuine. Starting from these, using the directional information derived earlier, edges can be traced through the image. While tracing an edge, we apply the lower threshold, allowing us to trace faint sections of edges as long as we find a starting point.Once this process is complete we have a binary image where each pixel ismarked as either an edge pixel or a non-edge pixel. From complementary output from the edge tracing step, the binary edge map obtained in this way can also be treated as a set of edge curves, which after further processing can be represented as polygons in the image domain.Differential geometric formulation of the Canny edge detector :0222=++yy y xy y x xx x L L L L L L L (3-1)A more refined approach to obtain edges with sub-pixel accuracy is by using the approach of differential edge detection, where the requirement ofnon-maximum suppression is formulated in terms of second- and third-orderderivatives computed from a scale-space representation (Lindeberg 1998) – see the article on edge detection for a detailed description.Variational-geometric formulation of the Haralick-Canny edge detector :0333223<+++yyy y xyy y x xxy y x xxx x L L L L L L L L L L (3-2)A variational explanation for the main ingredient of the Canny edge detector, that is, finding the zero crossings of the 2nd derivative along the gradient direction, was shown to be the result of minimizing a Kronrod-Minkowski functional while maximizing the integral over the alignment of the edge with the gradient field(Kimmel and Bruckstein 2003) see article on regularized Laplacian zero crossings and other optimal edge integrators for a detailed description.4 ParametersThe Canny algorithm contains a number of adjustable parameters, which can affect the computation time and effectiveness of the algorithm.The size of the Gaussian filter: the smoothing filter used in the first stagedirectly affects the results of the Canny algorithm. Smaller filters cause less blurring, and allow detection of small, sharp lines. A larger filter causesmore blurring, smearing out the value of a given pixel over a larger area of the image. Larger blurring radii are more useful for detecting larger,smoother edges – for instance, the edge of a rainbow.Thresholds: the use of two thresholds with hysteresis allows more flexibility than in a single-threshold approach, but general problems of thresholdingapproaches still apply. A threshold set too high can miss importantinformation. On the other hand, a threshold set too low will falsely identifyirrelevant information (such as noise) as important. It is difficult to give ageneric threshold that works well on all images. No tried and testedapproach to this problem yet exists.5 ConclusionThe Canny algorithm is adaptable to various environments. Its parameters allow it to be tailored to recognition of edges of differing characteristics depending on the particular requirements of a given implementation. In Canny's original paper, the derivation of the optimal filter led to a Finite Impulse Response filter, which can be slow to compute in the spatial domain if the amount of smoothing required is important (the filter will have a large spatial support in that case). For this reason, it is often suggested to use Rachid Deriche's Infinite Impulse Response form of Canny's filter (the Canny-Deriche detector), which is recursive, and which can be computed in a short, fixed amount of time for any desired amount of smoothing. The second form is suitable for real time implementations in FPGAs or DSPs, or very fast embedded PCs. In this context, however, the regular recursive implementation of the Canny operator does not give a good approximation of rotational symmetry and therefore gives a bias towards horizontal and vertical edges.中文翻译稿Canny算子1 Canny算子简介Canny 边缘检测算子是John F. Canny于1986 年开发出来的一个多级边缘检测算法。