压缩感知的去噪应用
压缩感知信号处理技术在图像处理中的应用
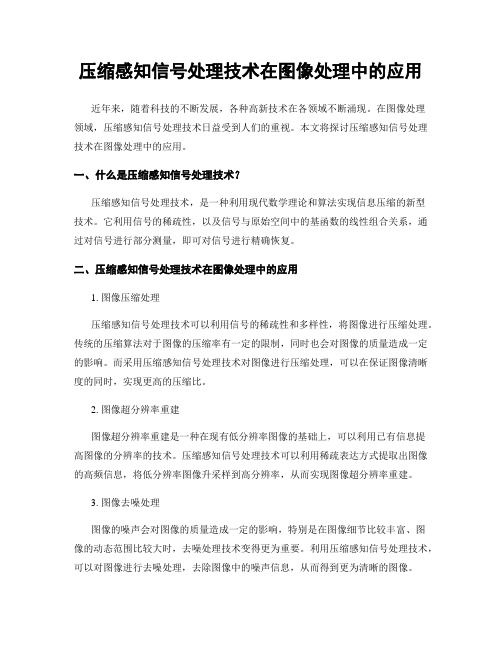
压缩感知信号处理技术在图像处理中的应用近年来,随着科技的不断发展,各种高新技术在各领域不断涌现。
在图像处理领域,压缩感知信号处理技术日益受到人们的重视。
本文将探讨压缩感知信号处理技术在图像处理中的应用。
一、什么是压缩感知信号处理技术?压缩感知信号处理技术,是一种利用现代数学理论和算法实现信息压缩的新型技术。
它利用信号的稀疏性,以及信号与原始空间中的基函数的线性组合关系,通过对信号进行部分测量,即可对信号进行精确恢复。
二、压缩感知信号处理技术在图像处理中的应用1. 图像压缩处理压缩感知信号处理技术可以利用信号的稀疏性和多样性,将图像进行压缩处理。
传统的压缩算法对于图像的压缩率有一定的限制,同时也会对图像的质量造成一定的影响。
而采用压缩感知信号处理技术对图像进行压缩处理,可以在保证图像清晰度的同时,实现更高的压缩比。
2. 图像超分辨率重建图像超分辨率重建是一种在现有低分辨率图像的基础上,可以利用已有信息提高图像的分辨率的技术。
压缩感知信号处理技术可以利用稀疏表达方式提取出图像的高频信息,将低分辨率图像升采样到高分辨率,从而实现图像超分辨率重建。
3. 图像去噪处理图像的噪声会对图像的质量造成一定的影响,特别是在图像细节比较丰富、图像的动态范围比较大时,去噪处理技术变得更为重要。
利用压缩感知信号处理技术,可以对图像进行去噪处理,去除图像中的噪声信息,从而得到更为清晰的图像。
4. 图像分割处理图像分割是指将图像中物体与背景分离的过程。
传统的图像分割技术常常基于像素点的相似性或颜色信息进行。
而利用压缩感知信号处理技术,可以利用稀疏性对图像进行分割,从而得到更为准确的图像分割结果。
三、总结压缩感知信号处理技术在图像处理领域中有非常广泛的应用。
通过利用信号稀疏性和多样性,可以对图像进行压缩、超分辨率重建、去噪和分割等多方面的处理。
虽然压缩感知信号处理技术已经被广泛应用,但是它仍然是一个不断发展和完善的领域。
相信在不久的未来,压缩感知信号处理技术将在图像处理领域中扮演更为重要的角色。
压缩感知理论及其在图像处理中的应用

压缩感知理论及其在图像处理中的应用近年来,随着数字图像在我们日常生活中的普及和广泛应用,如何快速高效地实现对大量图像数据的处理成为了一个难题。
传统的数字图像处理技术需要高带宽高速率的数据传输,计算机高速缓存、内存等硬件设备的昂贵需求,而压缩感知理论(Compressive Sensing, CS)的出现,则为解决这一难题提供了新的思路。
一、压缩感知理论的提出压缩感知理论是由2006年图像处理领域的国际权威科学家Emmanuel J. Candès 率先提出的。
该理论认为,只有在信号的采样和重构过程中,才能更好地利用信号的特性和结构,减少无用信息和冗余信息,从而实现对信号的高效处理。
也就是说,我们可以对信息进行压缩处理,以更快更高效地存储和处理数据。
与传统的压缩技术相比,压缩感知理论具有以下优点:1. 压缩效率更高:传统的压缩技术往往只能压缩部分信号能量,而压缩感知理论则可以在采样过程中,直接压缩信号本身。
2. 重构精度更高:压缩感知理论采用某些稀疏变换方法,具有更高的重构精度。
同时,针对一些非常难处理的图像信号,在压缩感知理论的框架下,其重构精度可以得到进一步提升。
二、压缩感知理论在图像处理中的应用由于压缩感知理论具有较多的优点,使得其在大量图像处理领域中有广泛的应用。
1. 图像压缩图像压缩是对大量数字数据的压缩性能测试、可视化和度量等方面的技术。
对于大量数据,我们可以采用压缩感知理论来进行压缩,这样可以极大程度地减少数据存储的空间,加速数据读写和传输的速度。
压缩过的图像,可以减少对存储设备的空间占用,提高传输的速度等,是一种非常实用的技术。
2. 图像分类在机器学习中,需要大量分类样本进行模型训练。
需要对训练的样本进行压缩,得到表征样本的特征向量,然后通过学习的分类器对其进行分类。
在这个过程中,压缩感知理论可以很好地处理各种图像分类问题。
3. 图像处理图像处理是数字图像处理中一个非常重要的领域。
基于压缩感知的磁共振成像降噪处理方法
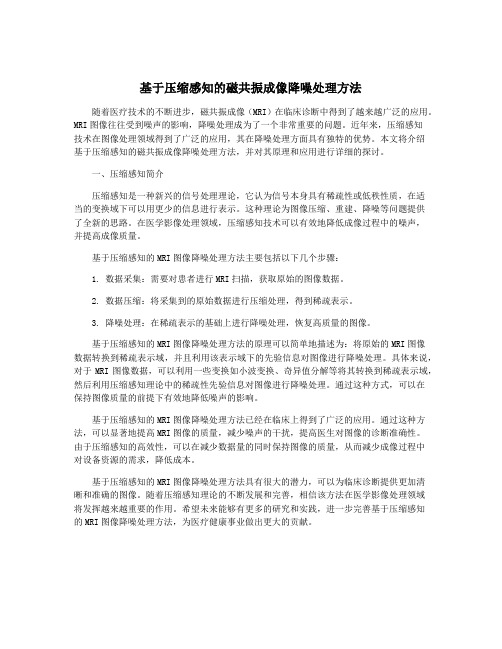
基于压缩感知的磁共振成像降噪处理方法随着医疗技术的不断进步,磁共振成像(MRI)在临床诊断中得到了越来越广泛的应用。
MRI图像往往受到噪声的影响,降噪处理成为了一个非常重要的问题。
近年来,压缩感知技术在图像处理领域得到了广泛的应用,其在降噪处理方面具有独特的优势。
本文将介绍基于压缩感知的磁共振成像降噪处理方法,并对其原理和应用进行详细的探讨。
一、压缩感知简介压缩感知是一种新兴的信号处理理论,它认为信号本身具有稀疏性或低秩性质,在适当的变换域下可以用更少的信息进行表示。
这种理论为图像压缩、重建、降噪等问题提供了全新的思路。
在医学影像处理领域,压缩感知技术可以有效地降低成像过程中的噪声,并提高成像质量。
基于压缩感知的MRI图像降噪处理方法主要包括以下几个步骤:1. 数据采集:需要对患者进行MRI扫描,获取原始的图像数据。
2. 数据压缩:将采集到的原始数据进行压缩处理,得到稀疏表示。
3. 降噪处理:在稀疏表示的基础上进行降噪处理,恢复高质量的图像。
基于压缩感知的MRI图像降噪处理方法的原理可以简单地描述为:将原始的MRI图像数据转换到稀疏表示域,并且利用该表示域下的先验信息对图像进行降噪处理。
具体来说,对于MRI图像数据,可以利用一些变换如小波变换、奇异值分解等将其转换到稀疏表示域,然后利用压缩感知理论中的稀疏性先验信息对图像进行降噪处理。
通过这种方式,可以在保持图像质量的前提下有效地降低噪声的影响。
基于压缩感知的MRI图像降噪处理方法已经在临床上得到了广泛的应用。
通过这种方法,可以显著地提高MRI图像的质量,减少噪声的干扰,提高医生对图像的诊断准确性。
由于压缩感知的高效性,可以在减少数据量的同时保持图像的质量,从而减少成像过程中对设备资源的需求,降低成本。
基于压缩感知的MRI图像降噪处理方法具有很大的潜力,可以为临床诊断提供更加清晰和准确的图像。
随着压缩感知理论的不断发展和完善,相信该方法在医学影像处理领域将发挥越来越重要的作用。
基于压缩感知的磁共振成像降噪处理方法
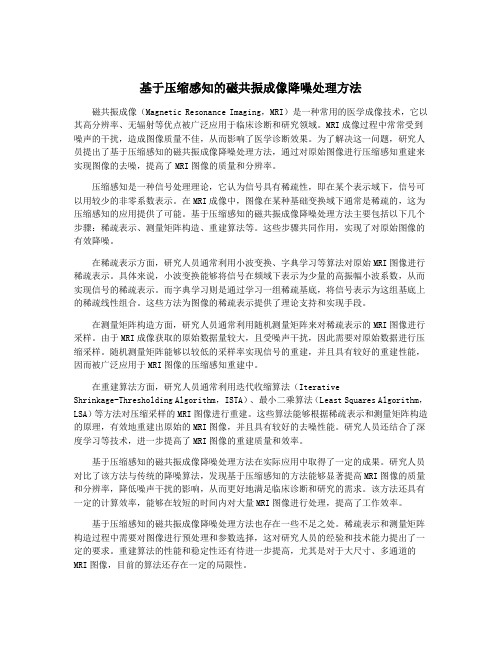
基于压缩感知的磁共振成像降噪处理方法磁共振成像(Magnetic Resonance Imaging,MRI)是一种常用的医学成像技术,它以其高分辨率、无辐射等优点被广泛应用于临床诊断和研究领域。
MRI成像过程中常常受到噪声的干扰,造成图像质量不佳,从而影响了医学诊断效果。
为了解决这一问题,研究人员提出了基于压缩感知的磁共振成像降噪处理方法,通过对原始图像进行压缩感知重建来实现图像的去噪,提高了MRI图像的质量和分辨率。
压缩感知是一种信号处理理论,它认为信号具有稀疏性,即在某个表示域下,信号可以用较少的非零系数表示。
在MRI成像中,图像在某种基础变换域下通常是稀疏的,这为压缩感知的应用提供了可能。
基于压缩感知的磁共振成像降噪处理方法主要包括以下几个步骤:稀疏表示、测量矩阵构造、重建算法等。
这些步骤共同作用,实现了对原始图像的有效降噪。
在稀疏表示方面,研究人员通常利用小波变换、字典学习等算法对原始MRI图像进行稀疏表示。
具体来说,小波变换能够将信号在频域下表示为少量的高振幅小波系数,从而实现信号的稀疏表示。
而字典学习则是通过学习一组稀疏基底,将信号表示为这组基底上的稀疏线性组合。
这些方法为图像的稀疏表示提供了理论支持和实现手段。
在测量矩阵构造方面,研究人员通常利用随机测量矩阵来对稀疏表示的MRI图像进行采样。
由于MRI成像获取的原始数据量较大,且受噪声干扰,因此需要对原始数据进行压缩采样。
随机测量矩阵能够以较低的采样率实现信号的重建,并且具有较好的重建性能,因而被广泛应用于MRI图像的压缩感知重建中。
在重建算法方面,研究人员通常利用迭代收缩算法(IterativeShrinkage-Thresholding Algorithm,ISTA)、最小二乘算法(Least Squares Algorithm,LSA)等方法对压缩采样的MRI图像进行重建。
这些算法能够根据稀疏表示和测量矩阵构造的原理,有效地重建出原始的MRI图像,并且具有较好的去噪性能。
压缩感知在电能质量扰动信号去噪中的应用
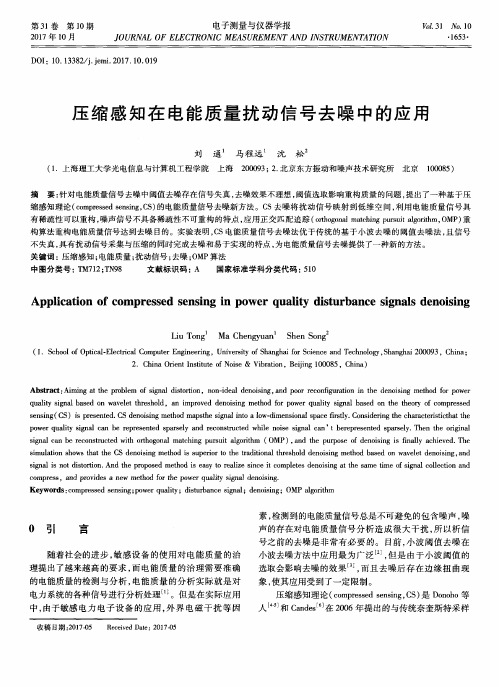
中图分类号 : T M7 1 2 ; T N 9 8 文献标识码 : A 国家标准学科分类代码 : 5 1 0
App l i c a t i o n o f c o m pr e s s e d s e ns i n g i n po we r q ua l i t y d i s t u r ba nc e s i g na l s d e n o i s i ng
s e n s i n g ( C S )i s p r e s e n t e d . C S d e n o i s i n g m e t h o d m a p s t h e s i g n l a i n t o a l o w - d i m e n s i o n a l s p a c e i f r s t l y . C o n s i d e i r n g t h e c h a r a c t e r i s t i c t h a t t h e
q u a l i t y s i g n l a b a s e d o n w a v e l e t t h r e s h o l d,a n i mp r o v e d d e n o i s i n g me t h o d or f p o w e r q u li a t y s i g n a l b a s e d o n t h e t h e o r y o f c o mp r e s s e d
p o we r q u a l i t y s i g n l a c a n b e r e p r e s e n t e d s p a r s e l y a n d r e c o n s t r u c t e d wh i l e n o i s e s i g n a l c a n’ t b e r e p r e s e n t e d s p a r s e l y . T h e n t h e o ig r i n l a
基于压缩感知技术的图像去噪研究
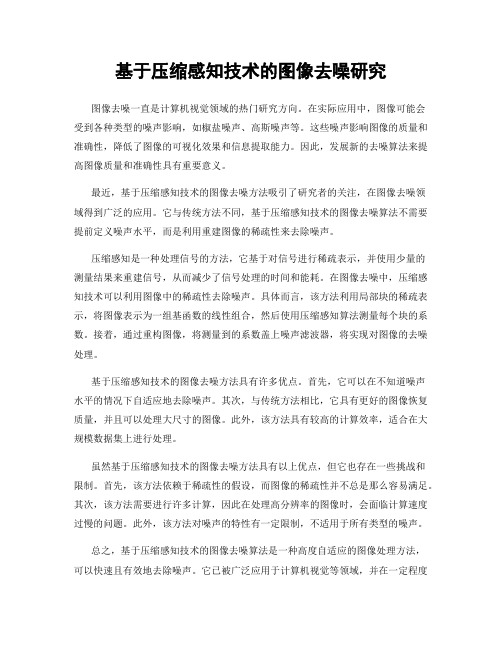
基于压缩感知技术的图像去噪研究图像去噪一直是计算机视觉领域的热门研究方向。
在实际应用中,图像可能会受到各种类型的噪声影响,如椒盐噪声、高斯噪声等。
这些噪声影响图像的质量和准确性,降低了图像的可视化效果和信息提取能力。
因此,发展新的去噪算法来提高图像质量和准确性具有重要意义。
最近,基于压缩感知技术的图像去噪方法吸引了研究者的关注,在图像去噪领域得到广泛的应用。
它与传统方法不同,基于压缩感知技术的图像去噪算法不需要提前定义噪声水平,而是利用重建图像的稀疏性来去除噪声。
压缩感知是一种处理信号的方法,它基于对信号进行稀疏表示,并使用少量的测量结果来重建信号,从而减少了信号处理的时间和能耗。
在图像去噪中,压缩感知技术可以利用图像中的稀疏性去除噪声。
具体而言,该方法利用局部块的稀疏表示,将图像表示为一组基函数的线性组合,然后使用压缩感知算法测量每个块的系数。
接着,通过重构图像,将测量到的系数盖上噪声滤波器,将实现对图像的去噪处理。
基于压缩感知技术的图像去噪方法具有许多优点。
首先,它可以在不知道噪声水平的情况下自适应地去除噪声。
其次,与传统方法相比,它具有更好的图像恢复质量,并且可以处理大尺寸的图像。
此外,该方法具有较高的计算效率,适合在大规模数据集上进行处理。
虽然基于压缩感知技术的图像去噪方法具有以上优点,但它也存在一些挑战和限制。
首先,该方法依赖于稀疏性的假设,而图像的稀疏性并不总是那么容易满足。
其次,该方法需要进行许多计算,因此在处理高分辨率的图像时,会面临计算速度过慢的问题。
此外,该方法对噪声的特性有一定限制,不适用于所有类型的噪声。
总之,基于压缩感知技术的图像去噪算法是一种高度自适应的图像处理方法,可以快速且有效地去除噪声。
它已被广泛应用于计算机视觉等领域,并在一定程度上改善了图像处理的准确性和质量。
然而,其仍需要克服一些限制和挑战,以提高其性能和适用范围。
在未来的研究中,我们可以通过改进稀疏性的假设和优化算法设计,来进一步拓展基于压缩感知技术的图像去噪的应用场景和性能。
压缩感知框架下MCA与模态分解联合去噪方法
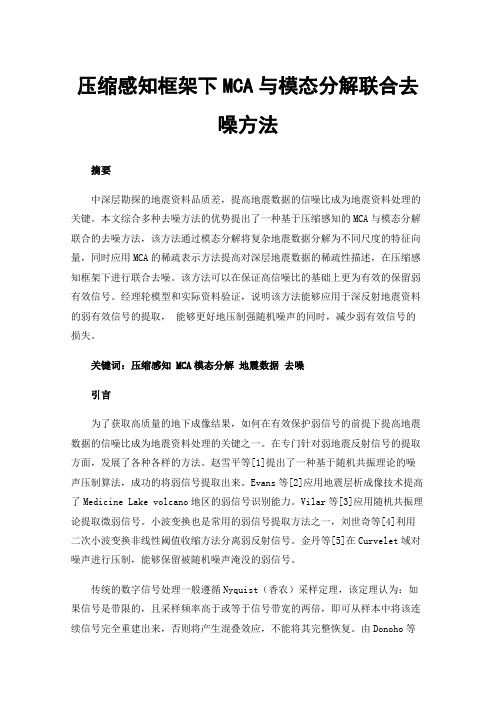
压缩感知框架下MCA与模态分解联合去噪方法摘要中深层勘探的地震资料品质差,提高地震数据的信噪比成为地震资料处理的关键。
本文综合多种去噪方法的优势提出了一种基于压缩感知的MCA与模态分解联合的去噪方法,该方法通过模态分解将复杂地震数据分解为不同尺度的特征向量,同时应用MCA的稀疏表示方法提高对深层地震数据的稀疏性描述,在压缩感知框架下进行联合去噪。
该方法可以在保证高信噪比的基础上更为有效的保留弱有效信号。
经理轮模型和实际资料验证,说明该方法能够应用于深反射地震资料的弱有效信号的提取,能够更好地压制强随机噪声的同时,减少弱有效信号的损失。
关键词:压缩感知 MCA模态分解地震数据去噪引言为了获取高质量的地下成像结果,如何在有效保护弱信号的前提下提高地震数据的信噪比成为地震资料处理的关键之一。
在专门针对弱地震反射信号的提取方面,发展了各种各样的方法。
赵雪平等[1]提出了一种基于随机共振理论的噪声压制算法,成功的将弱信号提取出来。
Evans等[2]应用地震层析成像技术提高了Medicine Lake volcano地区的弱信号识别能力。
Vilar等[3]应用随机共振理论提取微弱信号。
小波变换也是常用的弱信号提取方法之一,刘世奇等[4]利用二次小波变换非线性阈值收缩方法分离弱反射信号。
金丹等[5]在Curvelet域对噪声进行压制,能够保留被随机噪声淹没的弱信号。
传统的数字信号处理一般遵循Nyquist(香农)采样定理,该定理认为:如果信号是带限的,且采样频率高于或等于信号带宽的两倍,即可从样本中将该连续信号完全重建出来,否则将产生混叠效应,不能将其完整恢复。
由Donoho等[6]提出的压缩感知理论冲破了香农采样理论的限定,认为:如果待恢复数据具有可压缩性,即在某个变换域可以用极少数的值来描述,就可以构建测量矩阵,通过该矩阵观测被处理的数据,再通过各种稀疏反演算法实现数据的准确恢复。
经验模态分解(Empirical Mode Decomposition,EMD)方法是由Huang提出 [7],该方法会按照分解信号的局部特征时间尺度,把复杂的原始信号分解成具有不同特征尺度的本征模态函数(Intrinsic Mode Function,IMF)分量。
基于压缩感知的图像去噪的综述

而 忙
得
y @ x =  ̄v t = - O t
( 2 . 2 )
其 中 西为 测 量 矩 阵 , 大 小 为 Mx N。 @ = 、 I , 为 Mx N 的矩 阵 ,叫作 投影 矩 阵,Y被 叫做 t 在投影矩阵下的测量值 ,大小为 Mx 1 , 则压缩 感知的测量过程 如图 2所示 。 目前 ,如何 设计观 测矩 阵是 压缩 感知 理
是 保护边缘和细节信 息,输出一幅更适合于人 眼观 察或计算机能够进 一步研究的去噪图像 。 去 噪后的图像可信度更 高,图像更清晰,更适 合 人眼检测和计算机识 别,分析。 目前 图像去 噪技 术在 遥感图像 、自动识 别、医学 图像处理 、 计 算机视觉等更多领域发挥着重要作 用。 1 . 2图像 去噪的研究现状
ma g e&Mu l t i me d i a T e c h n o l o g y・ 图像与多媒体技术
基 于压 缩感知的图像去噪的综述
文/ 张丽娟 纪峰
压 缩感 知是 近 年来 新兴 的 一 门数 据 采样 技 术, 其主要 思 想 就 是 利用较 少 的 采样数 据 对信 号 进 行 重构 恢 复。压 缩感 知颠 覆 了传 统 的信 号 采样 方 法,它 采用 信 号 的稀 疏表 示 法 来保证 原 始信 号 的 主要 结 构,再 通 过 重构 算 法对 原 始信 号 进行 精 确 重构 。本文 将 对 图像 去 噪运 用 压缩 感知 理 论 ,利 用 压缩 感知 的优 点,对 含噪 图像 进 行稀 疏表 示,再 采用 不 同 的重 构 算法 对原 始 图像 进 行 恢复 ,从 而完 成 噪 声 的去 除 。
先 定 义 向量 x= { x 。 , X 2 , …x )的 P 一 范数
压缩感知下梯度投影在图像去噪中的应用
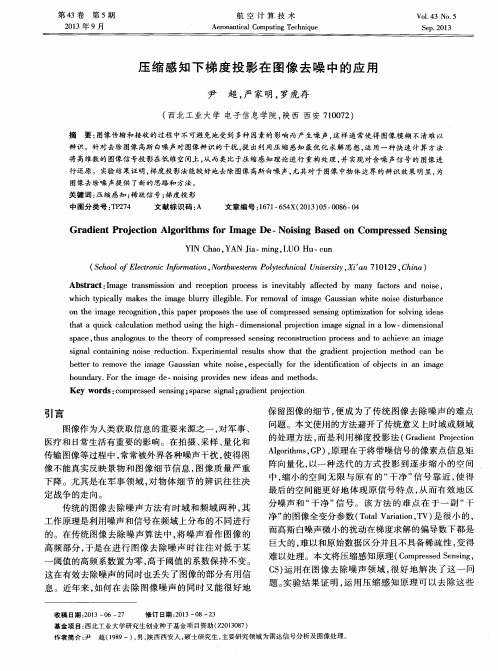
s p a c e, t h u s a n a l o g o u s t o t h e t h e o r y o f c o mp r e s s e d s e n s i n g r e c o n s t r u c t i o n p r o c e s s a n d t o a c h i e v e a n i ma g e
t h a t a q u i c k c a l c u l a t i o n me t h o d u s i n g t h e h i g h - - d i me n s i o n a l p r o j e c t i o n i m a g e s i g n a l i n a l o w- - d i m e n s i o n a l
o n t h e i ma g e r e c o g n i t i o n, t h i s pa p e r pr o p o s e s t h e us e o f c o mp r e s s e d s e n s i n g o p t i mi z a t i o n f o r s o l v i ng i de a s
Ab s t r a c t : I ma g e t r a n s mi s s i o n a n d r e c e p t i o n p r o c e s s i s i n e v i t a b l y a f f e c t e d b y ma n y f a c t o r s a n d n o i s e , w h i c h t y p i c a l l y ma k e s t h e i ma g e b l u r r y i l l e g i b l e . F o r r e mo v a l o f i ma g e Ga u s s i a n w h i t e n o i s e d i s t u r b a n c e
基于压缩感知的磁共振成像降噪处理方法
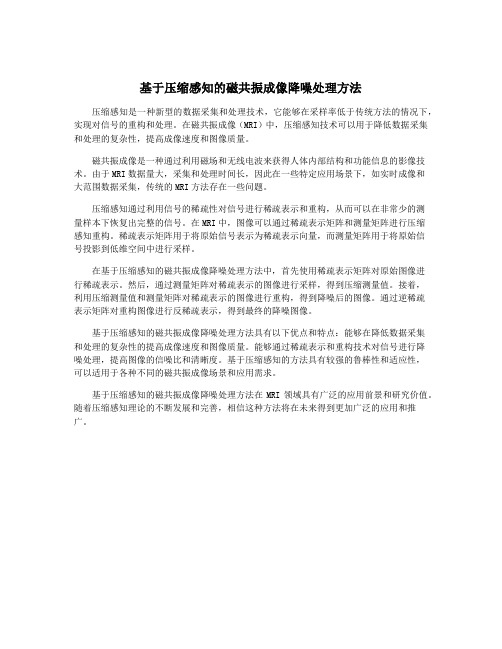
基于压缩感知的磁共振成像降噪处理方法压缩感知是一种新型的数据采集和处理技术,它能够在采样率低于传统方法的情况下,实现对信号的重构和处理。
在磁共振成像(MRI)中,压缩感知技术可以用于降低数据采集和处理的复杂性,提高成像速度和图像质量。
磁共振成像是一种通过利用磁场和无线电波来获得人体内部结构和功能信息的影像技术。
由于MRI数据量大,采集和处理时间长,因此在一些特定应用场景下,如实时成像和大范围数据采集,传统的MRI方法存在一些问题。
压缩感知通过利用信号的稀疏性对信号进行稀疏表示和重构,从而可以在非常少的测量样本下恢复出完整的信号。
在MRI中,图像可以通过稀疏表示矩阵和测量矩阵进行压缩感知重构。
稀疏表示矩阵用于将原始信号表示为稀疏表示向量,而测量矩阵用于将原始信号投影到低维空间中进行采样。
在基于压缩感知的磁共振成像降噪处理方法中,首先使用稀疏表示矩阵对原始图像进行稀疏表示。
然后,通过测量矩阵对稀疏表示的图像进行采样,得到压缩测量值。
接着,利用压缩测量值和测量矩阵对稀疏表示的图像进行重构,得到降噪后的图像。
通过逆稀疏表示矩阵对重构图像进行反稀疏表示,得到最终的降噪图像。
基于压缩感知的磁共振成像降噪处理方法具有以下优点和特点:能够在降低数据采集和处理的复杂性的提高成像速度和图像质量。
能够通过稀疏表示和重构技术对信号进行降噪处理,提高图像的信噪比和清晰度。
基于压缩感知的方法具有较强的鲁棒性和适应性,可以适用于各种不同的磁共振成像场景和应用需求。
基于压缩感知的磁共振成像降噪处理方法在MRI领域具有广泛的应用前景和研究价值。
随着压缩感知理论的不断发展和完善,相信这种方法将在未来得到更加广泛的应用和推广。
压缩感知_基于TV的去噪去模糊 以及GPSR方法的介绍.
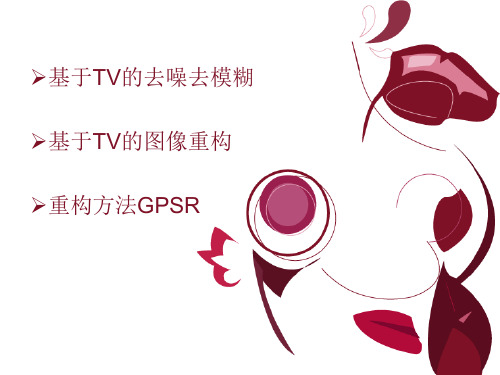
F
L( f ) x y
F
F
T L max M 0 M0 x
F
TV与OMP重构对比
采样率 方法 时间(s) 峰值信噪比
60% 50% 30%
OMP
TV
OMP
TV
OMP
TV
7.8489 27.6108 5.8305 25.2130 2.9458 19.9112
28.4592 43.7244 26.7119 40.6278 12.2554 34.4614
15
10
0.04 0.02
5
0 0
50 100 150 200 Number of non-zero components
250
0 0
50 100 150 200 Number of non-zero components
250
注:信号长度为4096,测量次数为1024; 运行10次,计算平均值。
2
L 1 x arg min{ x ( x f ( xk 1 ) 则可通过解 k k 1 2 L xC
TV ( x)} 获得重构图像。
F
?
问题一:求 f 问题二:求 L( f )
T f ( x) M 0 ( M 0 x y)
f ( x) f ( y)
x 1
(k ) 的数作为 ,并置 z ( k 1) ( z ( k ) ( k )F ( z ( k ) ));
Step 4 判断 :若满足收敛条件,则停止,并以 z ( k 1)为最终近似解; 否则令k=k+1返回Step2.
GPSR-BB
基于共轭梯度的除偏法
使用梯度投影法得到的解向量为 最优解向量 。 ,则可以得到最终的
基于压缩感知的磁共振成像降噪处理方法
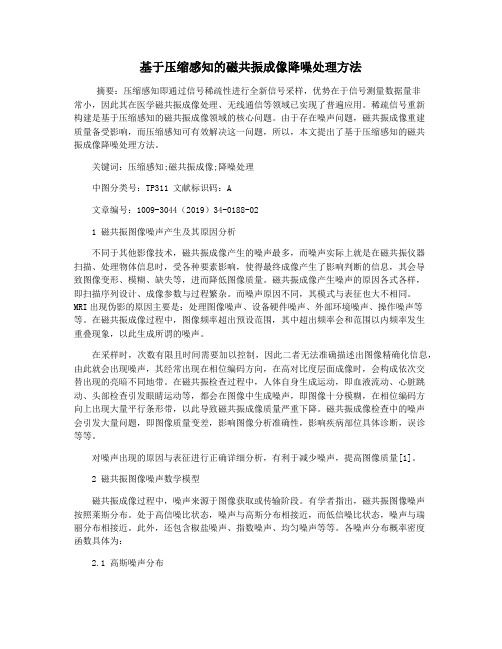
基于压缩感知的磁共振成像降噪处理方法摘要:压缩感知即通过信号稀疏性进行全新信号采样,优势在于信号测量数据量非常小,因此其在医学磁共振成像处理、无线通信等领域已实现了普遍应用。
稀疏信号重新构建是基于压缩感知的磁共振成像领域的核心问题。
由于存在噪声问题,磁共振成像重建质量备受影响,而压缩感知可有效解决这一问题,所以,本文提出了基于压缩感知的磁共振成像降噪处理方法。
关键词:压缩感知;磁共振成像;降噪处理中图分类号:TP311 文献标识码:A文章编号:1009-3044(2019)34-0188-021 磁共振图像噪声产生及其原因分析不同于其他影像技术,磁共振成像产生的噪声最多,而噪声实际上就是在磁共振仪器扫描、处理物体信息时,受各种要素影响,使得最终成像产生了影响判断的信息,其会导致图像变形、模糊、缺失等,进而降低图像质量。
磁共振成像产生噪声的原因各式各样,即扫描序列设计、成像参数与过程繁杂。
而噪声原因不同,其模式与表征也大不相同。
MRI出现伪影的原因主要是:处理图像噪声、设备硬件噪声、外部环境噪声、操作噪声等等。
在磁共振成像过程中,图像频率超出预设范围,其中超出频率会和范围以内频率发生重叠现象,以此生成所谓的噪声。
在采样时,次数有限且时间需要加以控制,因此二者无法准确描述出图像精确化信息,由此就会出现噪声,其经常出现在相位编码方向,在高对比度层面成像时,会构成依次交替出现的亮暗不同地带。
在磁共振检查过程中,人体自身生成运动,即血液流动、心脏跳动、头部检查引发眼睛运动等,都会在图像中生成噪声,即图像十分模糊,在相位编码方向上出现大量平行条形带,以此导致磁共振成像质量严重下降。
磁共振成像检查中的噪声会引发大量问题,即图像质量变差,影响图像分析准确性,影响疾病部位具体诊断,误诊等等。
对噪声出现的原因与表征进行正确详细分析,有利于减少噪声,提高图像质量[1]。
2 磁共振图像噪声数学模型磁共振成像过程中,噪声来源于图像获取或传输阶段。
压缩感知在图像去噪中的应用研究
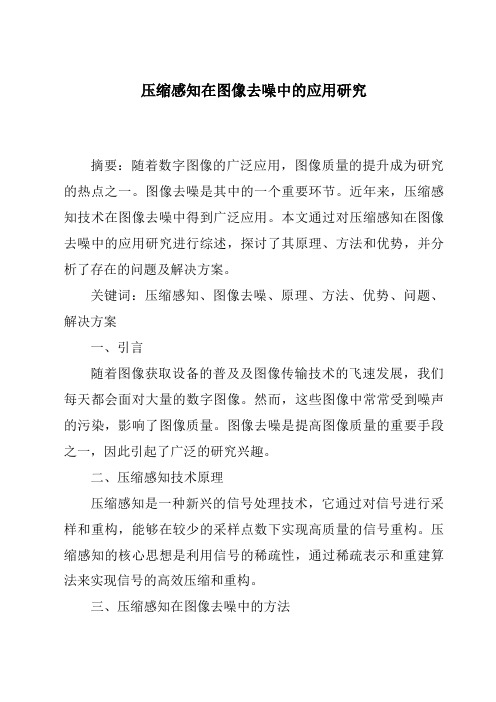
压缩感知在图像去噪中的应用研究摘要:随着数字图像的广泛应用,图像质量的提升成为研究的热点之一。
图像去噪是其中的一个重要环节。
近年来,压缩感知技术在图像去噪中得到广泛应用。
本文通过对压缩感知在图像去噪中的应用研究进行综述,探讨了其原理、方法和优势,并分析了存在的问题及解决方案。
关键词:压缩感知、图像去噪、原理、方法、优势、问题、解决方案一、引言随着图像获取设备的普及及图像传输技术的飞速发展,我们每天都会面对大量的数字图像。
然而,这些图像中常常受到噪声的污染,影响了图像质量。
图像去噪是提高图像质量的重要手段之一,因此引起了广泛的研究兴趣。
二、压缩感知技术原理压缩感知是一种新兴的信号处理技术,它通过对信号进行采样和重构,能够在较少的采样点数下实现高质量的信号重构。
压缩感知的核心思想是利用信号的稀疏性,通过稀疏表示和重建算法来实现信号的高效压缩和重构。
三、压缩感知在图像去噪中的方法压缩感知在图像去噪中的方法主要包括以下几个步骤:首先,对含有噪声的图像进行稀疏表示,通常使用离散余弦变换或小波变换等方法;然后,利用压缩感知算法对稀疏表示的系数进行采样,得到采样数据;最后,通过重建算法对采样数据进行重构,得到去噪后的图像。
四、压缩感知在图像去噪中的优势相比传统的图像去噪方法,压缩感知在图像去噪中具有以下优势:首先,由于采样点数较少,可以大大减少数据传输和存储的开销;其次,通过稀疏表示和重建算法,能够更好地保留图像的细节信息;此外,压缩感知还能够有效抑制噪声的传播,提高图像去噪的效果。
五、存在的问题及解决方案尽管压缩感知在图像去噪中具有诸多优势,但仍然存在一些问题,如重构误差较大、计算复杂度较高等。
针对这些问题,研究人员提出了一系列解决方案,如改进重构算法、优化采样策略等。
六、结论本文综述了压缩感知在图像去噪中的应用研究,探讨了其原理、方法和优势,并分析了存在的问题及解决方案。
压缩感知技术在图像去噪中具有广阔的应用前景,相信通过进一步的研究和改进,将能够更好地提高图像质量,满。
压缩感知及其图像处理应用研究
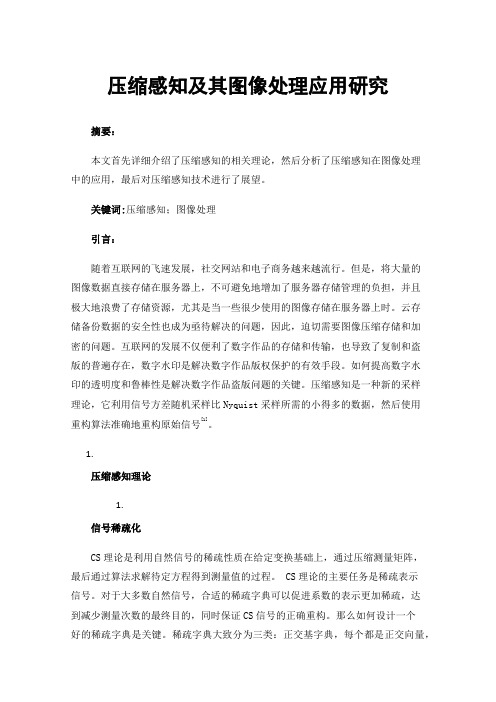
压缩感知及其图像处理应用研究摘要:本文首先详细介绍了压缩感知的相关理论,然后分析了压缩感知在图像处理中的应用,最后对压缩感知技术进行了展望。
关键词:压缩感知;图像处理引言:随着互联网的飞速发展,社交网站和电子商务越来越流行。
但是,将大量的图像数据直接存储在服务器上,不可避免地增加了服务器存储管理的负担,并且极大地浪费了存储资源,尤其是当一些很少使用的图像存储在服务器上时。
云存储备份数据的安全性也成为亟待解决的问题,因此,迫切需要图像压缩存储和加密的问题。
互联网的发展不仅便利了数字作品的存储和传输,也导致了复制和盗版的普遍存在,数字水印是解决数字作品版权保护的有效手段。
如何提高数字水印的透明度和鲁棒性是解决数字作品盗版问题的关键。
压缩感知是一种新的采样理论,它利用信号方差随机采样比Nyquist采样所需的小得多的数据,然后使用重构算法准确地重构原始信号[1]。
1.压缩感知理论1.信号稀疏化CS理论是利用自然信号的稀疏性质在给定变换基础上,通过压缩测量矩阵,最后通过算法求解待定方程得到测量值的过程。
CS理论的主要任务是稀疏表示信号。
对于大多数自然信号,合适的稀疏字典可以促进系数的表示更加稀疏,达到减少测量次数的最终目的,同时保证CS信号的正确重构。
那么如何设计一个好的稀疏字典是关键。
稀疏字典大致分为三类:正交基字典,每个都是正交向量,例如小波变换基;紧凑框架字典,使用Ridgelet、Curvelet、Bandlet 和Contourlet 来表示信号;过完备字典,其中有更多使用冗余原子库的分散信号。
当字典中任意N个原子是线性独立的,可以表示任意N维信号时,当然字典中的原子个数大于N,则称其为过完备字典。
我们可以通过人工构建或通过训练学习来获得过完备字典。
1.1.信号压缩测量在测量CS信号的过程中,可以看出测量矩阵在压缩采样过程中起着关键作用,是CS理论的重要组成部分。
当前的测量安排分为两类:一种是任意测量阵列,另一种是确定性测量阵列。
基于压缩感知的磁共振成像降噪处理方法
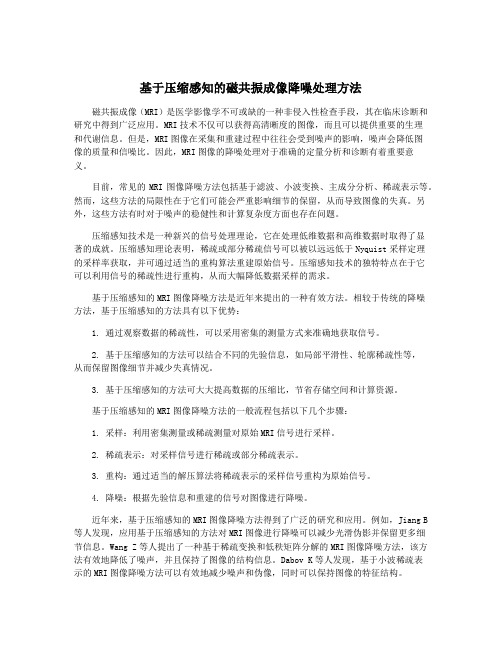
基于压缩感知的磁共振成像降噪处理方法磁共振成像(MRI)是医学影像学不可或缺的一种非侵入性检查手段,其在临床诊断和研究中得到广泛应用。
MRI技术不仅可以获得高清晰度的图像,而且可以提供重要的生理和代谢信息。
但是,MRI图像在采集和重建过程中往往会受到噪声的影响,噪声会降低图像的质量和信噪比。
因此,MRI图像的降噪处理对于准确的定量分析和诊断有着重要意义。
目前,常见的MRI图像降噪方法包括基于滤波、小波变换、主成分分析、稀疏表示等。
然而,这些方法的局限性在于它们可能会严重影响细节的保留,从而导致图像的失真。
另外,这些方法有时对于噪声的稳健性和计算复杂度方面也存在问题。
压缩感知技术是一种新兴的信号处理理论,它在处理低维数据和高维数据时取得了显著的成就。
压缩感知理论表明,稀疏或部分稀疏信号可以被以远远低于Nyquist采样定理的采样率获取,并可通过适当的重构算法重建原始信号。
压缩感知技术的独特特点在于它可以利用信号的稀疏性进行重构,从而大幅降低数据采样的需求。
基于压缩感知的MRI图像降噪方法是近年来提出的一种有效方法。
相较于传统的降噪方法,基于压缩感知的方法具有以下优势:1. 通过观察数据的稀疏性,可以采用密集的测量方式来准确地获取信号。
2. 基于压缩感知的方法可以结合不同的先验信息,如局部平滑性、轮廓稀疏性等,从而保留图像细节并减少失真情况。
3. 基于压缩感知的方法可大大提高数据的压缩比,节省存储空间和计算资源。
基于压缩感知的MRI图像降噪方法的一般流程包括以下几个步骤:1. 采样:利用密集测量或稀疏测量对原始MRI信号进行采样。
2. 稀疏表示:对采样信号进行稀疏或部分稀疏表示。
3. 重构:通过适当的解压算法将稀疏表示的采样信号重构为原始信号。
4. 降噪:根据先验信息和重建的信号对图像进行降噪。
近年来,基于压缩感知的MRI图像降噪方法得到了广泛的研究和应用。
例如,Jiang B 等人发现,应用基于压缩感知的方法对MRI图像进行降噪可以减少光滑伪影并保留更多细节信息。
基于压缩感知的磁共振成像降噪处理方法
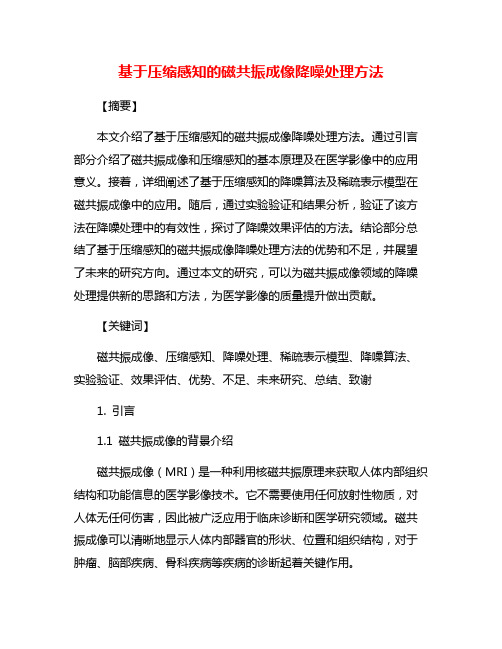
基于压缩感知的磁共振成像降噪处理方法【摘要】本文介绍了基于压缩感知的磁共振成像降噪处理方法。
通过引言部分介绍了磁共振成像和压缩感知的基本原理及在医学影像中的应用意义。
接着,详细阐述了基于压缩感知的降噪算法及稀疏表示模型在磁共振成像中的应用。
随后,通过实验验证和结果分析,验证了该方法在降噪处理中的有效性,探讨了降噪效果评估的方法。
结论部分总结了基于压缩感知的磁共振成像降噪处理方法的优势和不足,并展望了未来的研究方向。
通过本文的研究,可以为磁共振成像领域的降噪处理提供新的思路和方法,为医学影像的质量提升做出贡献。
【关键词】磁共振成像、压缩感知、降噪处理、稀疏表示模型、降噪算法、实验验证、效果评估、优势、不足、未来研究、总结、致谢1. 引言1.1 磁共振成像的背景介绍磁共振成像(MRI)是一种利用核磁共振原理来获取人体内部组织结构和功能信息的医学影像技术。
它不需要使用任何放射性物质,对人体无任何伤害,因此被广泛应用于临床诊断和医学研究领域。
磁共振成像可以清晰地显示人体内部器官的形状、位置和组织结构,对于肿瘤、脑部疾病、骨科疾病等疾病的诊断起着关键作用。
随着医学影像技术的不断发展,磁共振成像的分辨率和信噪比不断提高,但在实际应用中仍然存在着噪声干扰的问题。
这些噪声来源于磁场不均匀、设备本身的电子噪声等多方面因素,严重影响了影像质量和诊断准确性。
为了解决磁共振成像中的噪声问题,研究人员开始探索基于压缩感知的降噪处理方法。
压缩感知是一种新兴的信号处理技术,通过对信号进行稀疏表示和重构,可以在保持信号质量的前提下大幅降低数据获取和处理的复杂度。
将压缩感知应用于磁共振成像的降噪处理,可以有效提高影像的清晰度和质量,为临床诊断提供更准确的信息。
1.2 压缩感知的原理和应用压缩感知是一种通过观察信号的稀疏性来降低采样率以实现高效采样和重建的信号处理方法。
在数字信号处理领域,压缩感知技术已经被广泛应用于图像处理、语音处理、通信等领域。
压缩感知算法及其应用研究
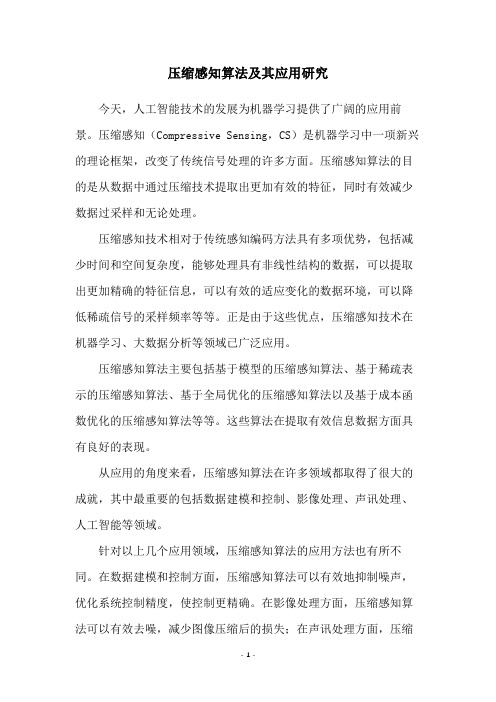
压缩感知算法及其应用研究今天,人工智能技术的发展为机器学习提供了广阔的应用前景。
压缩感知(Compressive Sensing,CS)是机器学习中一项新兴的理论框架,改变了传统信号处理的许多方面。
压缩感知算法的目的是从数据中通过压缩技术提取出更加有效的特征,同时有效减少数据过采样和无论处理。
压缩感知技术相对于传统感知编码方法具有多项优势,包括减少时间和空间复杂度,能够处理具有非线性结构的数据,可以提取出更加精确的特征信息,可以有效的适应变化的数据环境,可以降低稀疏信号的采样频率等等。
正是由于这些优点,压缩感知技术在机器学习、大数据分析等领域已广泛应用。
压缩感知算法主要包括基于模型的压缩感知算法、基于稀疏表示的压缩感知算法、基于全局优化的压缩感知算法以及基于成本函数优化的压缩感知算法等等。
这些算法在提取有效信息数据方面具有良好的表现。
从应用的角度来看,压缩感知算法在许多领域都取得了很大的成就,其中最重要的包括数据建模和控制、影像处理、声讯处理、人工智能等领域。
针对以上几个应用领域,压缩感知算法的应用方法也有所不同。
在数据建模和控制方面,压缩感知算法可以有效地抑制噪声,优化系统控制精度,使控制更精确。
在影像处理方面,压缩感知算法可以有效去噪,减少图像压缩后的损失;在声讯处理方面,压缩感知算法可以提高信号识别精度。
在人工智能方面,压缩感知算法可以提高学习效率、提高学习精度。
综上所述,压缩感知算法是机器学习中一项及其重要的理论框架,正在以及将在许多不同的领域发挥着重要的作用。
由于压缩感知算法涉及到多种学科和技术,因此它的研究非常有价值,有望为未来学术和实际应用研究提供新的思路和视角。
因此,深入研究压缩感知算法的理论和应用具有重要的现实意义。
当前,压缩感知的研究仍处于起步阶段。
首先,在理论上,压缩感知方法的效率和可行性仍要进一步加强。
其次,在应用上,要进一步扩大压缩感知的应用范围,以及在压缩感知方法上学习和发掘新的知识。
压缩感知在图像降噪中的应用开题报告

中北大学毕业设计开题报告学生姓名:学号:学院:信息与通信工程学院专业:设计题目:压缩感知在图像降噪中的应用指导教师:2014年3月10日毕 业 设 计 开 题 报 告 1.结合毕业设计情况,根据所查阅的文献资料,撰写2000字左右的文献综述:文 献 综 述1.1研究目的和意义数字图像处理技术是随着计算机技术发展而开拓出来的一个新的应用领域,汇聚了光学、电子学、数学、摄影技术、计算机技术等学科的众多方面。
它把图像转换成一个数据矩阵,在计算机上对其进行处理.计算机图像处理和计算机图形学的结合已经成为计算机辅助设计的主要基础。
随着计算机规模和速度的大幅度提高,数字图像处理技术的发展前途和应用领域将更加广阔。
人们可以通过多种不同方法获取图像,对这些图像进行数字化处理,可以使图像的视觉效果得到增强或者得到特殊的效果,以满足人们不同的需要。
从遥感、遥测、医学等许多重要的民用和军事成像领域,很多因素会导致图像质量的退化,比如图像的混叠、降晰和扭曲等噪声更是无处不在,图像在采集、传输和转换中常常受到成像设备和外部环境的干扰,在原图像中夹杂了噪声的干扰,使得图像降质,影响了图像的视觉效果,而且对图像进行进一步的处理也带来了不利。
从数字图像处理的技术角度讲,图像去噪处理属于图像回复的技术范畴;从整个图像分析的流程讲,图像去噪处理属于图像处理的预处理范畴。
因而图像去噪去噪方法的研究具有重要意义,主要表现在:(1)对含噪图像进行噪声处理,可以有效的保证正确识别图像的信息.当获得的图像中含有色噪声较严重时,图像变得模糊,使图像失去了存储信息的实际意义。
(2)图像降噪后不但可以提高视觉对信息识别的准确性,而且对进一步做图像处理 提供了基础,如果直接对含噪图像进行特征提取或融合等处理,则很难获得令人满意的处理结果。
(3)目前,虽然人们提出了许多的图像去噪方法,但是这些方法并不是完美的,进一步完善已有的图像去噪方法,或者研究新的图像去噪方法意义依然重大。
压缩感知理论在语音信号去噪中的应用
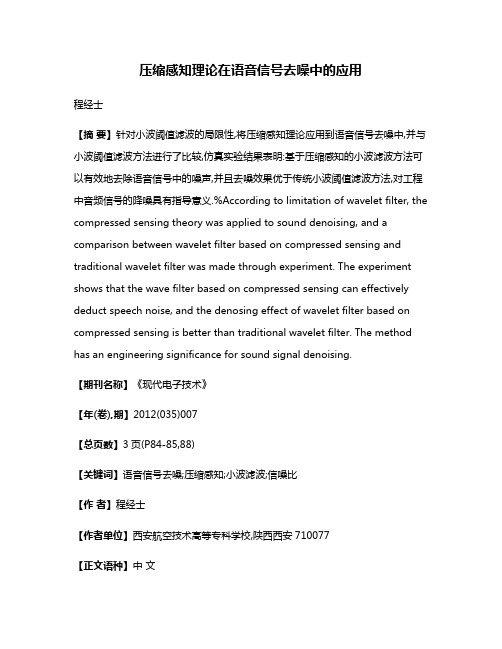
压缩感知理论在语音信号去噪中的应用程经士【摘要】针对小波阈值滤波的局限性,将压缩感知理论应用到语音信号去噪中,并与小波阈值滤波方法进行了比较,仿真实验结果表明:基于压缩感知的小波滤波方法可以有效地去除语音信号中的噪声,并且去噪效果优于传统小波阈值滤波方法,对工程中音频信号的降噪具有指导意义.%According to limitation of wavelet filter, the compressed sensing theory was applied to sound denoising, and a comparison between wavelet filter based on compressed sensing and traditional wavelet filter was made through experiment. The experiment shows that the wave filter based on compressed sensing can effectively deduct speech noise, and the denosing effect of wavelet filter based on compressed sensing is better than traditional wavelet filter. The method has an engineering significance for sound signal denoising.【期刊名称】《现代电子技术》【年(卷),期】2012(035)007【总页数】3页(P84-85,88)【关键词】语音信号去噪;压缩感知;小波滤波;信噪比【作者】程经士【作者单位】西安航空技术高等专科学校,陕西西安710077【正文语种】中文【中图分类】TN911.7-340 引言噪声不仅影响语音可懂度和清晰度,而且造成人耳听觉疲劳,因此语音去噪技术是语音信号处理的一个重要分支,它在解决噪声污染、改进语音质量、提高语音可懂度等方面发挥着重要的作用。
压缩感知去噪代码 -回复
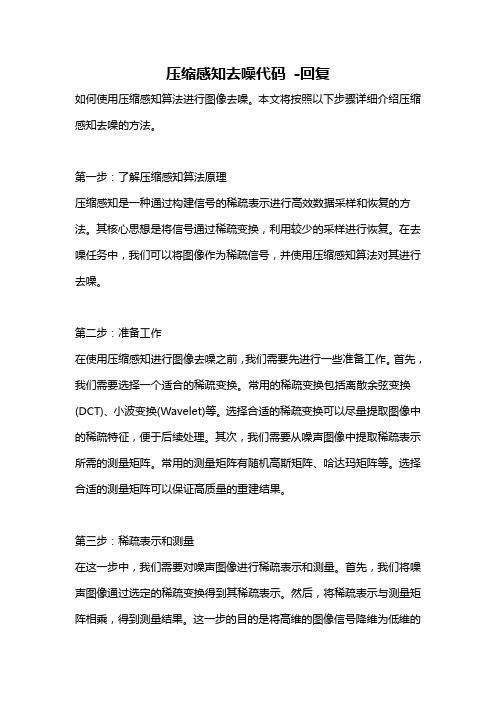
压缩感知去噪代码-回复如何使用压缩感知算法进行图像去噪。
本文将按照以下步骤详细介绍压缩感知去噪的方法。
第一步:了解压缩感知算法原理压缩感知是一种通过构建信号的稀疏表示进行高效数据采样和恢复的方法。
其核心思想是将信号通过稀疏变换,利用较少的采样进行恢复。
在去噪任务中,我们可以将图像作为稀疏信号,并使用压缩感知算法对其进行去噪。
第二步:准备工作在使用压缩感知进行图像去噪之前,我们需要先进行一些准备工作。
首先,我们需要选择一个适合的稀疏变换。
常用的稀疏变换包括离散余弦变换(DCT)、小波变换(Wavelet)等。
选择合适的稀疏变换可以尽量提取图像中的稀疏特征,便于后续处理。
其次,我们需要从噪声图像中提取稀疏表示所需的测量矩阵。
常用的测量矩阵有随机高斯矩阵、哈达玛矩阵等。
选择合适的测量矩阵可以保证高质量的重建结果。
第三步:稀疏表示和测量在这一步中,我们需要对噪声图像进行稀疏表示和测量。
首先,我们将噪声图像通过选定的稀疏变换得到其稀疏表示。
然后,将稀疏表示与测量矩阵相乘,得到测量结果。
这一步的目的是将高维的图像信号降维为低维的测量结果。
第四步:优化问题在这一步中,我们需要将去噪问题转化为一个优化问题。
我们试图通过最小化原始信号与稀疏表示之间的误差,以及测量结果与原始信号之间的误差来恢复原始信号。
通常使用的优化算法有L1范数最小化、L2范数最小化等。
我们可以通过求解最小化误差的优化问题来得到图像的估计。
第五步:还原原始图像在这一步中,我们将通过求解优化问题得到的估计结果,进行反变换得到最终的去噪图像。
具体的反变换操作与选定的稀疏变换有关。
第六步:性能评估在进行图像去噪时,我们需要对去噪效果进行评估。
常用的评估指标包括均方误差(MSE)、峰值信噪比(PSNR)等。
通过比较去噪图像与原始图像之间的差异,我们可以得到压缩感知去噪算法的性能。
总结:压缩感知去噪算法是一种利用稀疏特性进行图像去噪的方法。
通过合适的稀疏变换和测量矩阵,可以有效地提取图像中的稀疏特征,并进行去噪处理。
- 1、下载文档前请自行甄别文档内容的完整性,平台不提供额外的编辑、内容补充、找答案等附加服务。
- 2、"仅部分预览"的文档,不可在线预览部分如存在完整性等问题,可反馈申请退款(可完整预览的文档不适用该条件!)。
- 3、如文档侵犯您的权益,请联系客服反馈,我们会尽快为您处理(人工客服工作时间:9:00-18:30)。
Noise reduction through Compressed SensingJ.F.Gemmeke,B.CranenDept.of Linguistics,Radboud University,Nijmegen,The Netherlands{J.Gemmeke, B.Cranen}@let.ru.nlAbstractWe present an exemplar-based method for noise reduction using missing data imputation:A noise-corrupted word is sparsely represented in an over-complete basis of exemplar(clean) speech signals using only the uncorrupted time-frequency ele-ments of the word.Prior to recognition the parts of the spectro-gram dominated by noise are replaced by clean speech estimates obtained by projecting the sparse representation in the basis. Since at low SNRs individual frames may contain few,if any, uncorrupted coefficients,the method tries to exploit all reliable information that is available in a word-length time window.We study the effectiveness of this approach on the Interspeech2008 Consonant Challenge(VCV)data as well as on AURORA-2 ing oracle masks,we obtain obtain accuracies of36-44%on the VCV data.On AURORA-2we obtain an accuracy of91%at SNR-5dB,compared to61%using a conventional frame-based approach,clearly illustrating the great potential of the method.Index Terms:Automatic Speech Recognition,Missing Data Techniques,Compressed Sensing1.IntroductionAutomatic speech recognition(ASR)performance degrades substantially when speech is corrupted by background noises. Missing Data Techniques(MDT)[1,2]provide a powerful way to mitigate the impact of both stationary and non-stationary noise for moderate Signal-to-Noise(SNR)ratios.The gen-eral idea behind MDT is that it is possible to estimate−prior to decoding−which spectro-temporal elements of the acoustic representations are reliable(i.e.,dominated by speech energy) and which are unreliable(i.e.,dominated by background noise). These reliability estimates are used to treat reliable and unreli-able features differently and are referred to as a spectrographic mask.This information can for instance be used to replace the unreliable features by clean speech estimates,a process called imputation([3]).Although recognition accuracy can be improved substan-tially with MDT,at SNRs(≤0dB)the gain in recognition per-formance appears generally too small to be of practical use.A possible explanation is that at SNRs≤0dB a substantial num-ber of frames may contain few,if any,reliable features.As the number of reliable coefficients in a frame decreases,it becomes more difficult to safely impute the missing coefficients because in most MDT approaches imputation is performed on a frame by frame(i.e.strictly local)basis.This cannot but degrade recognition performance.Due to continuity constraints imposed by the speech pro-duction system,speech energy is distributed over the time-frequency plane in patches which cannot be of arbitrary small ing a(much)wider time window than a single frame might provide a way to exploit this continuity over time for im-puting missing data thus avoiding local information scarcity.Inthis paper we propose a novel,exemplar based,data imputationfront-end that tries to take advantage of the dependencies be-tween neighboring frames by using a larger spectro-temporalcontext.The technique is dubbed sparse imputation and isbased on work in the emergentfield of Compressed Sensing[4,5].Following an approach similar to[6],we treat entire wordsas units and represent them byfixed length vectors.We rep-resent unknown words as a linear combination of as few aspossible exemplar words in a training database.Work in Com-pressed Sensing has shown that if such a linear combination is sparse,the weight vector can be determined using only a small part of the elements of the feature vector representing the un-known item.We exploit this property by using only the featuresthat were considered reliable in the noisy input according tothe spectrographic mask.Next,the linear combination of cleanexemplar words is used for reconstructing the unreliable coeffi-cients of the noisy words.Finally,the imputed feature vectorsare processed by a conventional HMM-based ASR.In this paper we present a feasibility study to show that theCompressed Sensing approach is also potentially beneficial for speech recognition.We explore the effectiveness of our methodby applying it to the Interspeech2008Consonant Challenge(VCV)data[7])using two different mask types:the’oracle’mask1and a mask which estimates reliability based on a har-monic decomposition,dubbed harmonicity mask([8]).Addi-tionally,we compare the recognition performance that we ob-tained by means of our new,whole word based sparse imputa-tion method with the results from a classical,frame based im-putation approach using the AURORA-2digit recognition task,which also allows us to investigate relative improvement as afunction of SNR.We explain why the improvement is larger fororacle than for harmonicity masks.2.Method2.1.Speech materials and classification taskTwo word recognition tasks were performed.First,we per-formed intervocalic English consonant recognition using the VCV data(consisting of1clean and6noisy subsets)which are described in[7].Second,we carried out a single-digit recogni-tion/classification task using test set A from the AURORA-2cor-pus which comprises1clean and24noisy subsets,with fournoise types(subway,car,babble,exhibition hall)at six SNRlevels,SNR=20,15,10,5,0,−5dBDue to the constraint that the current implementation ofour exemplar-based imputation technique operates on whole-1Oracle masks are masks in which reliability decisions are based on exact knowledge about the extent to which each time-frequency cell is dominated by either noise or speechword units,we constructed training and test sets in which the words were surrounded by only a minimal amount of”silence”. The noisy single-digit data were created by extracting individ-ual digits from the utterances in the AURORA-2corpus using the segmentation obtained from a forced alignment of the clean speech utterances with their reference transcription.From the VCV utterances we removed leading and trailing silences using the offset data provided.2.2.Speech decodersFor recognition of the VCV words,we use the baseline HTK decoder described in[7].Imputation was carried out on mel frequency log power spectra(FBANK E),after which the recon-structed spectra were converted to standard mel cepstral coef-ficients(MFCC Z D A E)prior to recognition.With separate HMMs for initial andfinal vowels we used303-state mono-phone models(24consonants plus2×3=6vowels)consisting of24Gaussian mixtures.For recognition of the AURORA-2digits and comparison with a frame-based imputation method,we used a MATLAB im-plementation of the missing data recognition system described in[3].Acoustic feature vectors consisted of mel frequency log power spectra,which are then converted to PROSPECT features [3].We trained11whole-word models with16states per word using clean speech.In the baseline recognizer,unreliable fea-tures are replaced by estimated values using maximum likeli-hood per Gaussian-based imputation[3].In the sparse imputa-tion system,the spectrographic data arefirst cleaned with the method described below after which they are recognized using the baseline decoder with a spectrographic mask that considers every time-frequency cell as reliable.2.3.Fixed length vector representation of wordsSince the method described in the following sections works on observation vectors offixed size,we converted the acous-tic feature representations to a time normalized version(afixed number of acoustic feature frames).The re-sampling was done by applying spline interpolation to the spectrographic represen-tation and then re-sampling the23mel frequency log-energy coefficients individually such that afixed number of acoustic vectors per word resulted.In our experiment we used60time frames per word for the VCV-data and35time frames per word for AURORA-2digit data(i.e.,the mean number of time frames per word in the training sets).For the sparse imputation tech-nique the time-frames were then concatenated to form a single,fixed length observation vector.The baseline recognizer used the same,time-normalized spectra.A pilot study revealed that the recognition accuracies did not decrease after applying the resampling procedure.2.4.Sparse representationFollowing[6]we consider a test word y to be a linear com-bination of exemplar words w n,where the index n denotes a specific exemplar word(1≤n≤N)and N the total number of exemplar words in the training corpus.We write:y=NX n=1αn w nwith weightsαn∈R.Denoting the k th vector element of w n by w k n,and recall-ing that each word in the example set is represented by a vector with dimensionality K,we write our set of N exemplar words as a matrix A with dimensionality K×N:A=B B BB B@w11w12...w1N−1w1Nw21w22...w2N−1w2N......w K1w K2...w K N−1w K N1C C CC C AWe can now express any word y asy=A x(1) with x=[α1α2...αN−1αN]T an N-dimensional vector that will be sparsely represented in A(i.e.,most coefficientsαare zero).For the VCV-data the set of exemplar words comprised the entire training set:N=6,664.The number of exemplar words that could be taken from the clean train set of AURORA-2is 27,748words.In order to make classification times practical, we did not use all of them and reduced the number of columns N in A by randomly selecting a subset of the training set.We used N=4,000(yielding an average of about360tokens for each of the11digit words),which in a pilot study was found to give nearly the same recognition accuracy as using the full set.2.5.l1minimizationIn order to determine the sparse vector x representing a word y, we need to solve the system of linear equations of Eq.1.Typ-ically,the number of exemplar words will be much larger than the dimensionality of the feature representation of the vowels (K≪N).Thus,the system of linear equations in Eq.1is under-determined and has,generally speaking,no unique solu-tion.Research in thefield of Compressed Sensing[4,5],how-ever,has shown that if x is sparse,x can be determined by solving:min||x||1subject to y=A x(2) with||.||1the l1norm(i.e.minimization of the sum of absolute values of elements)which serves as an approximation of the l0 norm(i.e.,the number of nonzero elements).The approxima-tion is necessary since minimizing the l0norm is an NP-hard combinatorial problem[9],while l1minimization can be done efficiently in polynomial time.Since in practice it may be im-possible to express a word exactly as a superposition of exem-plar words,we use a noise robust version of Eq.2(cf.[10]): min||x||1subject to||y−A x||2≤ǫ(3) with a small constantǫsuch that the error e satisfies||e||2<ǫ.2.6.Spectrographic maskA spectrographic mask M is a matrix with the same dimen-sions as the spectrographic representation of a word.We used two different masks to describe the reliability of time-frequency cells in the spectrographic representation of a word:1)an ora-cle mask and2)an estimated mask:The harmonicity mask[8]. For the computation of the harmonicity mask the noisy speech signal isfirst decomposed into a harmonic and a random part. Next,a time-frequency cell is defined as unreliable if the energy of the random part exceeds that of the harmonic part.For use in the sparse imputation framework,we reshape the mask M to form a vector m by concatenating subsequent time frames as described in2.3.Table1:VCV consonant recognition accuracy.test setmethod1234567 baseline86.77.6 5.0 5.5 3.98.9 5.5 oracle-44.843.036.039.641.740.9 harmonicity-7.39.97.89.67.0 6.0 2.7.Sparse imputationGiven an observation vector y(representing an entire word), we denote y r consisting of the reliable coefficients of y.These are the elements for which the corresponding elements of mask vector m are equal to one.Similarly,we denote the unreliable coefficients of y(for which the corresponding elements of mask vector m are equal to zero)by y u.Without loss of generality we reorder y and A as in[11]so that we can write:»y r y u–=…A r A u«x(4) with A r and A u pertaining to the rows of A indicated by the reliable and unreliable coefficients in y.Since we consider the values of the y r to be valid representatives of clean speech,we solve Eq.3using only y r instead of y.After obtaining the sparse representation x we use this vector to impute clean esti-mates y i for the unreliable coefficients y u using the support of x in the basis A u:ˆy=»y r y i–=»y r A u x–(5)yielding a new observation vectorˆy.In order to perform recog-nition we restore the original ordering and reshapeˆy of Eq.5 to a time framed spectrographic representation.Obviously,no restoration of the unreliable coefficients in y is possible if there are no reliable coefficients to base the estima-tion on.If we denote the number of reliable coefficients in y by K r=dim(y r),in practice,effective restoration of the unreli-able coefficients will be difficult below some threshold K r<δ. For several reasons it is impossible to determineδtheoretically. First,it is impossible to predict the sparsity of x obtained in Eq.4.Second,δwill depend on the structure of the spec-trographic mask and therefore on the underlying speech signal and environmental noise:features cannot be restored if the re-maining reliable features do not carry sufficient information to predict the value of the unreliable ones.Accepting that faulty restorations of y are unavoidable when the number of reliable features drops below the unknown threshold K r,we decided to always perform sparse imputation except when K r=0.3.ResultsTable1shows that the accuracy on clean speech(86.7%)of our baseline recognizer is slightly lower than the accuracies re-ported in[7](88.5%).This is probably caused by the use of log energy only rather than the combination of log energy and zeroth cepstral coefficient.As can be inferred from Table1, using a harmonicity mask to control the reconstruction process does not yield consistent improvements.In fact,in some cases the accuracies even deteriorate.The recognition accuracies ob-tained with the sparse imputation method using an oracle mask range from36-45%,which amounts to a very substantial im-provement over the baseline.Figure1:AURORA-2single digit recognition accuracy.The figure shows results for both normal Missing Data Imputation (NI)as well as sparse imputation(SI)for the oracle mask and the harmonicity mask respectively..Figure1shows the recognition accuracies(i.e.,averages over the four noise types in test set A)on the single-digit task (AURORA-2).Thefigure shows recognition accuracies using the baseline missing data recognizer,as well as the sparse im-putation method.With the estimated(harmonicity)mask sparse imputation does not improve and even slightly degrades perfor-mance.Also,for both imputation methods recognition accu-racies at SNRs≤0dB,the SNRs in the VCV data,decrease rapidly.With the oracle masks,however,the whole word sparse imputation technique substantially outperforms the frame based imputation technique at low SNRs:Recognition accuracy at SNR-5dB is91%compared to61%.4.Discussion4.1.Reconstruction using estimated masksThe small but consistent differences in recognition accuracy be-tween the imputation methods observed on AURORA-2are most likely due to the fact that only in the frame-based imputation method bounded imputation is employed:The estimated coeffi-cients are bounded by the observation energy assuming additive noise,while the sparse imputation makes no such assumption. Apparently,bounded imputation is better suited to compensate for the little amount of reliable information marked as such by the harmonicity mask.More important,however,is the fact that at low SNRs the use of a wider context does not help to improve recognition performance compared to the frame based approach.It was shown in[12]that the harmonicity mask la-bels significantly fewer time-frequency cells reliable when com-pared to the oracle mask:Our results therefore indicate that at low SNRs this specific mask estimation method simply does not generate enough reliable coefficients(according to the criterion K r>δcf.section2.7)to allow successful reconstruction of the unreliable ones.Additionally,more speech-like noises like present in the VCV test sets inevitably causes mislabeling since the mask estimation method uses harmonic decomposition to la-bel cells reliable.If the corrupting noise is a competing speaker, such as in test set2,the harmonicity mask is likely to label noisy spectrographic regions reliable and possibly even label targetspeech regions unreliable,causing the two lower-than-baseline accuracies.Obviously more advanced mask estimation techniques are required(cf.[13]for a comprehensive survey)if the full poten-tial of missing data techniques at low SNRs is to be exploited.4.2.Reconstruction using oracle masksAs might be expected,recognition accuracies using the ora-cle mask show significant improvement over baseline results. For the VCV-data accuracies obtained with the the sparse im-putation method range from36-45%indicating that a substan-tial amount,but by far not all corrupted features can be recon-structed with our method.This is not unexpected since even native listeners display a decrease in recognition accuracy of 14-27%[7].Still,the fact that the drop in accuracy of human listeners is lower than in our method indicates that our reliable features contain not enough information:The sparse imputation technique,and imputation based MDT in general,can only re-construct unreliable coefficients if the reliable coefficients alone are sufficient to discriminate between the alternatives in the vo-cabulary.For AURORA-2this condition holds much better since even at an SNR of-5dB an accuracy of91%is attainable.The difference in recognition performance between the VCV and AURORA-2task using oracle masks can be understood from the nature of the recognition tasks:consonants typically have lower energy than vowels.At SNRs≤0dB,in general only the vowels will have spectrographic regions dominating the noise.With the digits in AURORA-2the vowels will often contain sufficient information to predict the surrounding conso-nants,if only because not every consonant occurs in all possible vowel contexts.In this respect,the VCV set is much less pre-dictable:Each consonant can occur all vowel contexts.As a consequence,the vowels themselves will be of little use to dis-criminate between words,and only the VC and CV transition regions may help to discriminate between consonants.Unfortunately we did not have a MDT frame-based decoder for the VCV-data at our disposal.From comparing the recogni-tion accuracy of91%at SNR-5dB on AURORA-2to the61% recognition accuracy of the baseline MDT-decoder,an increase of30%absolute,it is clear that a frame based approach is only partially successful in exploiting redundant information.The results on AURORA-2suggest that using a wider time context when doing imputation is beneficial to avoid local information scarcity and that sparse imputation can be a powerfull method to utilize this wider time context.4.3.Future workThe success of the sparse imputation method using oracle masks makes further research desirable.In order to be used as a gen-eral front-end for ASR systems the method needs to be extended to work in a continuous time setting.One way to do this is to use a sliding time-window using several neighboring time frames as generally used in frame-based Support Vector Machine and Neural Net classification tasks.Another approach is used in [14],in which a larger basis is defined using time-shifted copies of the original basis.The practical applicability and computa-tional feasibility of either method is left as future work.5.ConclusionsWe introduced a missing data imputation front-end which works byfinding a sparse representation of the noisy speech signal, using only the information of the speech signal labeled reli-able by a spectrographic mask.The sparse representation isfound by expressing entire words as a linear combination ofexemplar words.The sparse representation is then used to es-timate the missing(unreliable)coefficients of the speech sig-nal after which classic speech recognition can take place.Re-sults on both the Interspeech Consonant Challenge data and the AURORA-2digits underline that recognition accuracy depends on the success with which the spectrographic mask can be esti-mated and to what extent the reliable features carry informationabout the unreliable ones.Experiments on AURORA-2usingan oracle mask,however,also clearly show the potential of thepresented method:A recognition accuracy of91%at SNR=-5dB is obtained,an increase of30%absolute over a state-of-the art missing data speech recognizer using frame by frameimputation.This shows that even at very low SNRs enough in-formation about the speech signal may be preserved to success-fully perform imputation solely on the basis of reliable time-frequency cells provided enough time-context is used.6.AcknowledgmentsThe research of Jort Gemmeke was carried out in the MIDASproject,granted under the Dutch-Flemish STEVIN program.7.References[1] B.Raj,R.Singh,and R.Stern,“Inference of missing spectro-graphic features for robust automatic speech recognition,”in Pro-ceedings International Conference on Spoken Language Process-ing,1998,pp.1491–1494.[2]M.Cooke,P.Green,L.Josifovksi,and A.Vizinho,“Robust au-tomatic speech recognition with missing and unreliable acoustic data,”Speech Communication,vol.34,pp.267–285,2001. [3]H.Van hamme,“Prospect features and their application to miss-ing data techniques for robust speech recognition,”in Interspeech-2004,2004,pp.101–104.[4] D.L.Donoho,“Compressed sensing,”IEEE Transactions on In-formation Theory,vol.52,no.4,pp.1289–1306,2006.[5] E.J.Candes,“Compressive sampling,”in Proceedings of the In-ternational Congress of Mathematicians,2006.[6] A.Y.Yang,J.Wright,Y.Ma,and S.S.Sastry,“Feature selectionin face recognition:A sparse representation perspective,”submit-ted to IEEE Transactions Pattern Analysis and Machine Intelli-gence,August2007.[7]M.Cooke and O.Scharenborg,“The interspeech2008consonantchallenge,”submitted to Interspeech2008,2008.[8]H.Van hamme,“Robust speech recognition using cepstral do-main missing data techniques and noisy masks,”in Proceedings of IEEE ICASSP,vol.1,2004,pp.213–216.[9] B.K.Natarajan,“Sparse approximate solutions to linear systems,”SIAM put.,vol.24,no.2,pp.227–234,1995.[10] D.L.Donoho,“For most large underdetermined systems of equa-tions,the minimal l1-norm near-solution approximates the spars-est near-solution,”Communications on Pure and Applied Mathe-matics,vol.59,no.7,pp.907–934,2006.[11]Y.Zhang,“When is missing data recoverable?”Technical Report,2006.[12]J.Gemmeke,B.Cranen,and L.ten Bosch,“On the relation be-tween statistical properties of spectrographic masks and recog-nition accuracy,”in Proceeding(599)Signal Processing,Pattern Recognition,and Applications-2008,2008,pp.200–206. [13] C.Cerisara,S.Demange,and J.-P.Haton,“On noise masking forautomatic missing data speech recognition:A survey and discus-sion,”Comput.Speech Lang.,vol.21,no.3,pp.443–457,2007.[14]M.Mœrup and M.N.Schmidt,“Shift invariant sparse coding ofimage and music data,”Submitted to Journal of Machine Learning Research,2008.。