Neyman-Fisher, Theorem
neymanpearson准则 统计决策
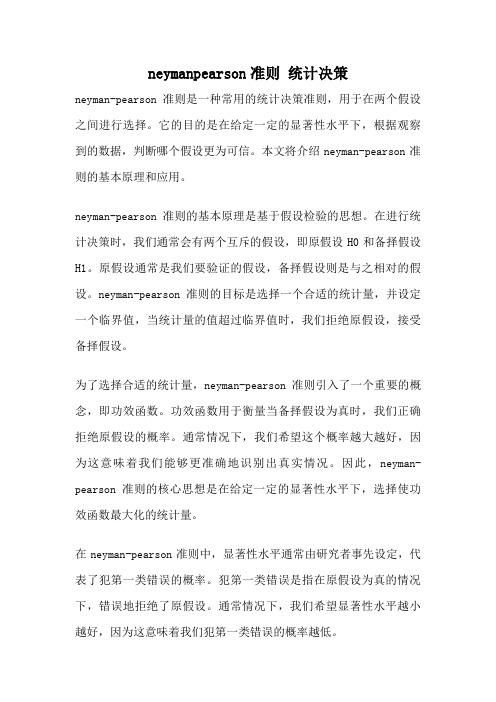
neymanpearson准则统计决策neyman-pearson准则是一种常用的统计决策准则,用于在两个假设之间进行选择。
它的目的是在给定一定的显著性水平下,根据观察到的数据,判断哪个假设更为可信。
本文将介绍neyman-pearson准则的基本原理和应用。
neyman-pearson准则的基本原理是基于假设检验的思想。
在进行统计决策时,我们通常会有两个互斥的假设,即原假设H0和备择假设H1。
原假设通常是我们要验证的假设,备择假设则是与之相对的假设。
neyman-pearson准则的目标是选择一个合适的统计量,并设定一个临界值,当统计量的值超过临界值时,我们拒绝原假设,接受备择假设。
为了选择合适的统计量,neyman-pearson准则引入了一个重要的概念,即功效函数。
功效函数用于衡量当备择假设为真时,我们正确拒绝原假设的概率。
通常情况下,我们希望这个概率越大越好,因为这意味着我们能够更准确地识别出真实情况。
因此,neyman-pearson准则的核心思想是在给定一定的显著性水平下,选择使功效函数最大化的统计量。
在neyman-pearson准则中,显著性水平通常由研究者事先设定,代表了犯第一类错误的概率。
犯第一类错误是指在原假设为真的情况下,错误地拒绝了原假设。
通常情况下,我们希望显著性水平越小越好,因为这意味着我们犯第一类错误的概率越低。
选择合适的临界值是neyman-pearson准则的另一个重要步骤。
临界值的选择要根据实际问题和样本数据来确定。
一般情况下,我们会根据显著性水平和备择假设的特点来选择临界值。
如果我们希望更加保守,即降低犯第一类错误的概率,我们可以选择较小的临界值;如果我们希望更加激进,即提高功效函数的值,我们可以选择较大的临界值。
neyman-pearson准则在实际应用中有着广泛的应用。
例如,在医学研究中,我们可以使用neyman-pearson准则来判断某种药物是否对疾病有治疗效果。
充分统计量的证明方法及几个重要定理

充分统计量的证明方法及几个重要定理刘冬喜(湖南娄底职业技术学院计算机系湖南娄底417000)摘要:本文讨论了充分统计量的充分性,给出了统计量的充分性的两种主要证明方法,介绍了几个重要的定理,它们可以用来间接地证明统计量的充分性.关键词:统计量;充分统计量;因子分解定理;统计结构;Fisher信息Proof method of Sufficient statistic and several important theoremsLiu Dong-xi(Loudi Vocational and Technical College,Loudi Hunan 417000)Abstract:In this paper,we discuss the sufficient statistic sufficiency and the two main proof methods to statistic sufficiency. Several important theorems are introduced and they may be used to prove the sufficiency of statistic indirectlyKey words:Statistic, sufficient statistic, factoring theorem, statistical structure, Fisher information一、统计量与充分统计量统计量是样本的函数,定义在可测空间(X, ,B)上的统计量T=T(x),实际上是对样本X=(X1,…,Xn)进行某种加工和提炼的结果,把样本中所含的总体的相关信息集中起来,针对不同问题构造出样本的适当函数,这种加工从本质上体现了统计量压缩数据的功能.从直观上看,样本的不同的观察值,统计量T可能有相同的值,如:样本均值和样本方差不会随样本观察的排列顺序的改变而改变,这体现了统计量的“压缩数据”的功能.从理论上看,若T是在(T,C)上取值的可测映照,那么对σ代数C中任一元素c在B中都有一个原像T﹣1(C)={x:T(x)∈C}∈B .把所有原像的全体记为T-1(C)={T-1(C):C∈C} ⊂B。
博弈论与组合优化中的对偶问题
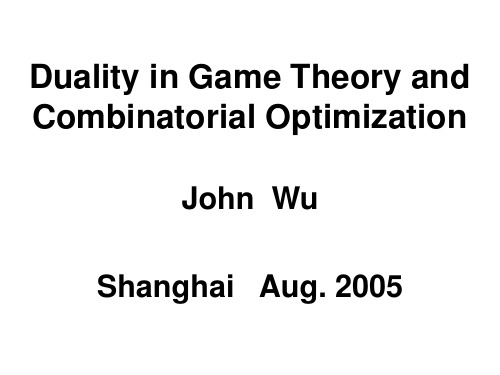
1.1. 博弈论发展过程中的四个 研究纲领
• • • • von Neumann纲领 1928 Nash纲领1950 Aumann纲领1974 重复博弈中的有限自动机博弈理论、 复杂性理论和学习理论 1993
1.1 博弈论发展过程中的四个 研究纲领
von Neumann纲领 线性规划对偶性
Nash纲领
1.5 二人一般博弈与非线性规划对偶 之间的对应关系
• 从Nash纲领到Aumann纲领的转向得到了很多博 弈论学者的支持: • 首先是Selten、Harsanyi等人在Aumann纲领正式 提出前对均衡精化的研究 • 其次是Hart和Schmeidler、Nau和McCardle、 Myerson、Fudenberg和Levine、Foster和Vohra、 Hart和Mas-Colell等人在Aumann纲领正式提出后 对均衡粗化具体内容的研究
1.6 策略复杂性与复杂性与简单性对 偶之间的对应关系
• 但策略复杂性研究目前没有充分反映博弈的对偶 性质的新概念 • 原因在于忽视了博弈论与组合优化的联系 • Shubik(1997)关于博弈的复杂性与简单性的思考 或许提示了博弈的一种新的对偶性
1.7 研究次序
• 问题在于:必须先将Aumann纲领中隐含的精化 和粗化的对偶性研究清楚,才能进一步研究策略 复杂性和学习理论的对偶性 • 故应先研究精化和粗化的对偶性
1.5 二人一般博弈与非线性规划对偶 之间的对应关系
• Kakad e和Foster : • 他们的结论与Hart和Mas-Colell(2003b)的结论 并不冲突,因为后者的学习设定不允许从博弈行 动的历史中提取丰富统计数据。 • 看来Nash均衡与均衡粗化之间还有很多关系有待 人们去发现,Nash均衡与均衡粗化的统计学基础 还有待加强。 • 这就导致了策略复杂性和学习理论的研究
信号检测与估计2006-第3讲LM,一般MVU&BLUE

协方差矩阵为 Cθˆ = ( H T C -1 H ) −1
对于一般的线性模型, MVU估计量是有效的,它达到了CRLB
General Minimum Variance Unbiased Estimation
一般MVU估计
重要概念:充分统计量 重要定理:
Neyman-Fisher因子分解定理 Rao-Blackwell-Lehmann-Scheffe定理
对于线性模型, MVU估计量是有效的,它达到了CRLB
Linear Models
(4.8) (4.9)
线性模型
ˆ ˆ (4.8)式代入到(4.9)式,易证 θ 是无偏的,由于 θ 是 ˆ 高斯随机矢量 x 的线性变换,因此 θ 的性能就可完全确定 (不仅仅是均值和方差),因而
θˆ ∼ N(θ ,σ 2 (H T H )−1)
(4.9) (4.26)
线性模型的扩展
根据(4.9)式,θ的MVU 估计量为 θˆ = ( H ′T H ′) −1 H ′T x′=( H T DT DH ) −1 H T DT Dx 所以
θˆ = ( H T C −1 H ) −1 H T C −1x
(4.25)
类似地,可求得 Cθˆ = ( H ′T H ′) −1 或最终得到: Cθˆ = ( H T C −1 H ) −1 (4.26)
T T −1 T −1
所以矩阵D起到白化变换的作用。因此,如果将一般线性模型 x=Hθ +w 变换到x′=Dx=DHθ + Dw=H ′θ + w′,由于w′=Dw ∼ N (0, I ), 所以噪声将 被白化,并且得到通常意义下的线性模型。
Linear Models
Cθˆ = ( H T C −1 H ) −1
统计学的产生与发展

绪论第一节统计学的产生与发展统计学的产生与统计实践活动是密不可分的,统计作为一种社会实践活动,已有四五千年的历史。
早在原始社会,人们按部落居住在一起,打猎、捕鱼后就要算算有多少人、多少食物,以便分配食物;我国夏禹时代就有了人口数据的记载;为了赋税、徭役和兵役的需要,历代都有田亩和户口的记录。
而统计学的理论和方法,则是在长期统计实践活动的基础上发展起来的,距今已有三百多年的历史。
从统计学的发展过程来看,可以大致分为三个阶段。
一、古典统计学时期17世纪中叶至18世纪中叶是古典统计学时期,在这一时期,统计学理论初步形成了一定的学术派别,主要有国势学派和政治算术学派。
1.国势学派国势学派又称记述学派,产生于17世纪的德国。
由于该学派主要以文字记述国家的显著事项,故称记述学派。
其主要代表人物是海尔曼·康令(Hermann Conring,1606-1681)和阿亨华尔(Gottfried Achenwall,1719-1772)。
康令于1660年把国势学从法学、史学和地理学等学科中独立出来,在大学中讲授“实际政治家所必需的知识”;阿亨华尔在哥廷根大学开设“国家学”课程,其主要著作是《近代欧洲各国国势学纲要》,书中讲述“一国或多数国家的显著事项”,主要用对比分析的方法研究了国家组织、领土、人口、资源财富和国情国力,比较了各国实力的强弱,为德国的君主政体服务。
因在外文中“国势”与“统计”词义相通,后来正式命名为“统计学”。
国势学派只是对国情的记述,偏重事物性质的解释,未能进一步揭示社会经济现象的规律,也不研究事物的计量分析方法,不注重数量对比和数量计算,只是用比较级和最高级的词汇对事物的状态进行描述。
所以,人们也把它叫做记述学派(旧学派或德国学派),并认为国势学派有统计学之名而无统计学之实。
2.政治算术学派政治算术学派产生于19世纪中叶的英国,创始人威廉·配第(William Petty,1623-·2·统计学概论1687),其代表作是他于1676年完成的《政治算术》一书,这本书是经济学和统计学史上的重要著作,这里的“政治”是指政治经济学,“算术”是指统计方法。
T.W. ANDERSON (1971). The Statistical Analysis of Time Series. Series in Probability and Ma

425 BibliographyH.A KAIKE(1974).Markovian representation of stochastic processes and its application to the analysis of autoregressive moving average processes.Annals Institute Statistical Mathematics,vol.26,pp.363-387. B.D.O.A NDERSON and J.B.M OORE(1979).Optimal rmation and System Sciences Series, Prentice Hall,Englewood Cliffs,NJ.T.W.A NDERSON(1971).The Statistical Analysis of Time Series.Series in Probability and Mathematical Statistics,Wiley,New York.R.A NDRE-O BRECHT(1988).A new statistical approach for the automatic segmentation of continuous speech signals.IEEE Trans.Acoustics,Speech,Signal Processing,vol.ASSP-36,no1,pp.29-40.R.A NDRE-O BRECHT(1990).Reconnaissance automatique de parole`a partir de segments acoustiques et de mod`e les de Markov cach´e s.Proc.Journ´e es Etude de la Parole,Montr´e al,May1990(in French).R.A NDRE-O BRECHT and H.Y.S U(1988).Three acoustic labellings for phoneme based continuous speech recognition.Proc.Speech’88,Edinburgh,UK,pp.943-950.U.A PPEL and A.VON B RANDT(1983).Adaptive sequential segmentation of piecewise stationary time rmation Sciences,vol.29,no1,pp.27-56.L.A.A ROIAN and H.L EVENE(1950).The effectiveness of quality control procedures.Jal American Statis-tical Association,vol.45,pp.520-529.K.J.A STR¨OM and B.W ITTENMARK(1984).Computer Controlled Systems:Theory and rma-tion and System Sciences Series,Prentice Hall,Englewood Cliffs,NJ.M.B AGSHAW and R.A.J OHNSON(1975a).The effect of serial correlation on the performance of CUSUM tests-Part II.Technometrics,vol.17,no1,pp.73-80.M.B AGSHAW and R.A.J OHNSON(1975b).The influence of reference values and estimated variance on the ARL of CUSUM tests.Jal Royal Statistical Society,vol.37(B),no3,pp.413-420.M.B AGSHAW and R.A.J OHNSON(1977).Sequential procedures for detecting parameter changes in a time-series model.Jal American Statistical Association,vol.72,no359,pp.593-597.R.K.B ANSAL and P.P APANTONI-K AZAKOS(1986).An algorithm for detecting a change in a stochastic process.IEEE rmation Theory,vol.IT-32,no2,pp.227-235.G.A.B ARNARD(1959).Control charts and stochastic processes.Jal Royal Statistical Society,vol.B.21, pp.239-271.A.E.B ASHARINOV andB.S.F LEISHMAN(1962).Methods of the statistical sequential analysis and their radiotechnical applications.Sovetskoe Radio,Moscow(in Russian).M.B ASSEVILLE(1978).D´e viations par rapport au maximum:formules d’arrˆe t et martingales associ´e es. Compte-rendus du S´e minaire de Probabilit´e s,Universit´e de Rennes I.M.B ASSEVILLE(1981).Edge detection using sequential methods for change in level-Part II:Sequential detection of change in mean.IEEE Trans.Acoustics,Speech,Signal Processing,vol.ASSP-29,no1,pp.32-50.426B IBLIOGRAPHY M.B ASSEVILLE(1982).A survey of statistical failure detection techniques.In Contribution`a la D´e tectionS´e quentielle de Ruptures de Mod`e les Statistiques,Th`e se d’Etat,Universit´e de Rennes I,France(in English). M.B ASSEVILLE(1986).The two-models approach for the on-line detection of changes in AR processes. In Detection of Abrupt Changes in Signals and Dynamical Systems(M.Basseville,A.Benveniste,eds.). Lecture Notes in Control and Information Sciences,LNCIS77,Springer,New York,pp.169-215.M.B ASSEVILLE(1988).Detecting changes in signals and systems-A survey.Automatica,vol.24,pp.309-326.M.B ASSEVILLE(1989).Distance measures for signal processing and pattern recognition.Signal Process-ing,vol.18,pp.349-369.M.B ASSEVILLE and A.B ENVENISTE(1983a).Design and comparative study of some sequential jump detection algorithms for digital signals.IEEE Trans.Acoustics,Speech,Signal Processing,vol.ASSP-31, no3,pp.521-535.M.B ASSEVILLE and A.B ENVENISTE(1983b).Sequential detection of abrupt changes in spectral charac-teristics of digital signals.IEEE rmation Theory,vol.IT-29,no5,pp.709-724.M.B ASSEVILLE and A.B ENVENISTE,eds.(1986).Detection of Abrupt Changes in Signals and Dynamical Systems.Lecture Notes in Control and Information Sciences,LNCIS77,Springer,New York.M.B ASSEVILLE and I.N IKIFOROV(1991).A unified framework for statistical change detection.Proc.30th IEEE Conference on Decision and Control,Brighton,UK.M.B ASSEVILLE,B.E SPIAU and J.G ASNIER(1981).Edge detection using sequential methods for change in level-Part I:A sequential edge detection algorithm.IEEE Trans.Acoustics,Speech,Signal Processing, vol.ASSP-29,no1,pp.24-31.M.B ASSEVILLE, A.B ENVENISTE and G.M OUSTAKIDES(1986).Detection and diagnosis of abrupt changes in modal characteristics of nonstationary digital signals.IEEE rmation Theory,vol.IT-32,no3,pp.412-417.M.B ASSEVILLE,A.B ENVENISTE,G.M OUSTAKIDES and A.R OUG´E E(1987a).Detection and diagnosis of changes in the eigenstructure of nonstationary multivariable systems.Automatica,vol.23,no3,pp.479-489. M.B ASSEVILLE,A.B ENVENISTE,G.M OUSTAKIDES and A.R OUG´E E(1987b).Optimal sensor location for detecting changes in dynamical behavior.IEEE Trans.Automatic Control,vol.AC-32,no12,pp.1067-1075.M.B ASSEVILLE,A.B ENVENISTE,B.G ACH-D EVAUCHELLE,M.G OURSAT,D.B ONNECASE,P.D OREY, M.P REVOSTO and M.O LAGNON(1993).Damage monitoring in vibration mechanics:issues in diagnos-tics and predictive maintenance.Mechanical Systems and Signal Processing,vol.7,no5,pp.401-423.R.V.B EARD(1971).Failure Accommodation in Linear Systems through Self-reorganization.Ph.D.Thesis, Dept.Aeronautics and Astronautics,MIT,Cambridge,MA.A.B ENVENISTE and J.J.F UCHS(1985).Single sample modal identification of a nonstationary stochastic process.IEEE Trans.Automatic Control,vol.AC-30,no1,pp.66-74.A.B ENVENISTE,M.B ASSEVILLE and G.M OUSTAKIDES(1987).The asymptotic local approach to change detection and model validation.IEEE Trans.Automatic Control,vol.AC-32,no7,pp.583-592.A.B ENVENISTE,M.M ETIVIER and P.P RIOURET(1990).Adaptive Algorithms and Stochastic Approxima-tions.Series on Applications of Mathematics,(A.V.Balakrishnan,I.Karatzas,M.Yor,eds.).Springer,New York.A.B ENVENISTE,M.B ASSEVILLE,L.E L G HAOUI,R.N IKOUKHAH and A.S.W ILLSKY(1992).An optimum robust approach to statistical failure detection and identification.IFAC World Conference,Sydney, July1993.B IBLIOGRAPHY427 R.H.B ERK(1973).Some asymptotic aspects of sequential analysis.Annals Statistics,vol.1,no6,pp.1126-1138.R.H.B ERK(1975).Locally most powerful sequential test.Annals Statistics,vol.3,no2,pp.373-381.P.B ILLINGSLEY(1968).Convergence of Probability Measures.Wiley,New York.A.F.B ISSELL(1969).Cusum techniques for quality control.Applied Statistics,vol.18,pp.1-30.M.E.B IVAIKOV(1991).Control of the sample size for recursive estimation of parameters subject to abrupt changes.Automation and Remote Control,no9,pp.96-103.R.E.B LAHUT(1987).Principles and Practice of Information Theory.Addison-Wesley,Reading,MA.I.F.B LAKE and W.C.L INDSEY(1973).Level-crossing problems for random processes.IEEE r-mation Theory,vol.IT-19,no3,pp.295-315.G.B ODENSTEIN and H.M.P RAETORIUS(1977).Feature extraction from the encephalogram by adaptive segmentation.Proc.IEEE,vol.65,pp.642-652.T.B OHLIN(1977).Analysis of EEG signals with changing spectra using a short word Kalman estimator. Mathematical Biosciences,vol.35,pp.221-259.W.B¨OHM and P.H ACKL(1990).Improved bounds for the average run length of control charts based on finite weighted sums.Annals Statistics,vol.18,no4,pp.1895-1899.T.B OJDECKI and J.H OSZA(1984).On a generalized disorder problem.Stochastic Processes and their Applications,vol.18,pp.349-359.L.I.B ORODKIN and V.V.M OTTL’(1976).Algorithm forfinding the jump times of random process equation parameters.Automation and Remote Control,vol.37,no6,Part1,pp.23-32.A.A.B OROVKOV(1984).Theory of Mathematical Statistics-Estimation and Hypotheses Testing,Naouka, Moscow(in Russian).Translated in French under the title Statistique Math´e matique-Estimation et Tests d’Hypoth`e ses,Mir,Paris,1987.G.E.P.B OX and G.M.J ENKINS(1970).Time Series Analysis,Forecasting and Control.Series in Time Series Analysis,Holden-Day,San Francisco.A.VON B RANDT(1983).Detecting and estimating parameters jumps using ladder algorithms and likelihood ratio test.Proc.ICASSP,Boston,MA,pp.1017-1020.A.VON B RANDT(1984).Modellierung von Signalen mit Sprunghaft Ver¨a nderlichem Leistungsspektrum durch Adaptive Segmentierung.Doctor-Engineer Dissertation,M¨u nchen,RFA(in German).S.B RAUN,ed.(1986).Mechanical Signature Analysis-Theory and Applications.Academic Press,London. L.B REIMAN(1968).Probability.Series in Statistics,Addison-Wesley,Reading,MA.G.S.B RITOV and L.A.M IRONOVSKI(1972).Diagnostics of linear systems of automatic regulation.Tekh. Kibernetics,vol.1,pp.76-83.B.E.B RODSKIY and B.S.D ARKHOVSKIY(1992).Nonparametric Methods in Change-point Problems. Kluwer Academic,Boston.L.D.B ROEMELING(1982).Jal Econometrics,vol.19,Special issue on structural change in Econometrics. L.D.B ROEMELING and H.T SURUMI(1987).Econometrics and Structural Change.Dekker,New York. D.B ROOK and D.A.E VANS(1972).An approach to the probability distribution of Cusum run length. Biometrika,vol.59,pp.539-550.J.B RUNET,D.J AUME,M.L ABARR`E RE,A.R AULT and M.V ERG´E(1990).D´e tection et Diagnostic de Pannes.Trait´e des Nouvelles Technologies,S´e rie Diagnostic et Maintenance,Herm`e s,Paris(in French).428B IBLIOGRAPHY S.P.B RUZZONE and M.K AVEH(1984).Information tradeoffs in using the sample autocorrelation function in ARMA parameter estimation.IEEE Trans.Acoustics,Speech,Signal Processing,vol.ASSP-32,no4, pp.701-715.A.K.C AGLAYAN(1980).Necessary and sufficient conditions for detectability of jumps in linear systems. IEEE Trans.Automatic Control,vol.AC-25,no4,pp.833-834.A.K.C AGLAYAN and R.E.L ANCRAFT(1983).Reinitialization issues in fault tolerant systems.Proc.Amer-ican Control Conf.,pp.952-955.A.K.C AGLAYAN,S.M.A LLEN and K.W EHMULLER(1988).Evaluation of a second generation reconfigu-ration strategy for aircraftflight control systems subjected to actuator failure/surface damage.Proc.National Aerospace and Electronic Conference,Dayton,OH.P.E.C AINES(1988).Linear Stochastic Systems.Series in Probability and Mathematical Statistics,Wiley, New York.M.J.C HEN and J.P.N ORTON(1987).Estimation techniques for tracking rapid parameter changes.Intern. Jal Control,vol.45,no4,pp.1387-1398.W.K.C HIU(1974).The economic design of cusum charts for controlling normal mean.Applied Statistics, vol.23,no3,pp.420-433.E.Y.C HOW(1980).A Failure Detection System Design Methodology.Ph.D.Thesis,M.I.T.,L.I.D.S.,Cam-bridge,MA.E.Y.C HOW and A.S.W ILLSKY(1984).Analytical redundancy and the design of robust failure detection systems.IEEE Trans.Automatic Control,vol.AC-29,no3,pp.689-691.Y.S.C HOW,H.R OBBINS and D.S IEGMUND(1971).Great Expectations:The Theory of Optimal Stop-ping.Houghton-Mifflin,Boston.R.N.C LARK,D.C.F OSTH and V.M.W ALTON(1975).Detection of instrument malfunctions in control systems.IEEE Trans.Aerospace Electronic Systems,vol.AES-11,pp.465-473.A.C OHEN(1987).Biomedical Signal Processing-vol.1:Time and Frequency Domain Analysis;vol.2: Compression and Automatic Recognition.CRC Press,Boca Raton,FL.J.C ORGE and F.P UECH(1986).Analyse du rythme cardiaque foetal par des m´e thodes de d´e tection de ruptures.Proc.7th INRIA Int.Conf.Analysis and optimization of Systems.Antibes,FR(in French).D.R.C OX and D.V.H INKLEY(1986).Theoretical Statistics.Chapman and Hall,New York.D.R.C OX and H.D.M ILLER(1965).The Theory of Stochastic Processes.Wiley,New York.S.V.C ROWDER(1987).A simple method for studying run-length distributions of exponentially weighted moving average charts.Technometrics,vol.29,no4,pp.401-407.H.C S¨ORG¨O and L.H ORV´ATH(1988).Nonparametric methods for change point problems.In Handbook of Statistics(P.R.Krishnaiah,C.R.Rao,eds.),vol.7,Elsevier,New York,pp.403-425.R.B.D AVIES(1973).Asymptotic inference in stationary gaussian time series.Advances Applied Probability, vol.5,no3,pp.469-497.J.C.D ECKERT,M.N.D ESAI,J.J.D EYST and A.S.W ILLSKY(1977).F-8DFBW sensor failure identification using analytical redundancy.IEEE Trans.Automatic Control,vol.AC-22,no5,pp.795-803.M.H.D E G ROOT(1970).Optimal Statistical Decisions.Series in Probability and Statistics,McGraw-Hill, New York.J.D ESHAYES and D.P ICARD(1979).Tests de ruptures dans un mod`e pte-Rendus de l’Acad´e mie des Sciences,vol.288,Ser.A,pp.563-566(in French).B IBLIOGRAPHY429 J.D ESHAYES and D.P ICARD(1983).Ruptures de Mod`e les en Statistique.Th`e ses d’Etat,Universit´e deParis-Sud,Orsay,France(in French).J.D ESHAYES and D.P ICARD(1986).Off-line statistical analysis of change-point models using non para-metric and likelihood methods.In Detection of Abrupt Changes in Signals and Dynamical Systems(M. Basseville,A.Benveniste,eds.).Lecture Notes in Control and Information Sciences,LNCIS77,Springer, New York,pp.103-168.B.D EVAUCHELLE-G ACH(1991).Diagnostic M´e canique des Fatigues sur les Structures Soumises`a des Vibrations en Ambiance de Travail.Th`e se de l’Universit´e Paris IX Dauphine(in French).B.D EVAUCHELLE-G ACH,M.B ASSEVILLE and A.B ENVENISTE(1991).Diagnosing mechanical changes in vibrating systems.Proc.SAFEPROCESS’91,Baden-Baden,FRG,pp.85-89.R.D I F RANCESCO(1990).Real-time speech segmentation using pitch and convexity jump models:applica-tion to variable rate speech coding.IEEE Trans.Acoustics,Speech,Signal Processing,vol.ASSP-38,no5, pp.741-748.X.D ING and P.M.F RANK(1990).Fault detection via factorization approach.Systems and Control Letters, vol.14,pp.431-436.J.L.D OOB(1953).Stochastic Processes.Wiley,New York.V.D RAGALIN(1988).Asymptotic solutions in detecting a change in distribution under an unknown param-eter.Statistical Problems of Control,Issue83,Vilnius,pp.45-52.B.D UBUISSON(1990).Diagnostic et Reconnaissance des Formes.Trait´e des Nouvelles Technologies,S´e rie Diagnostic et Maintenance,Herm`e s,Paris(in French).A.J.D UNCAN(1986).Quality Control and Industrial Statistics,5th edition.Richard D.Irwin,Inc.,Home-wood,IL.J.D URBIN(1971).Boundary-crossing probabilities for the Brownian motion and Poisson processes and techniques for computing the power of the Kolmogorov-Smirnov test.Jal Applied Probability,vol.8,pp.431-453.J.D URBIN(1985).Thefirst passage density of the crossing of a continuous Gaussian process to a general boundary.Jal Applied Probability,vol.22,no1,pp.99-122.A.E MAMI-N AEINI,M.M.A KHTER and S.M.R OCK(1988).Effect of model uncertainty on failure detec-tion:the threshold selector.IEEE Trans.Automatic Control,vol.AC-33,no12,pp.1106-1115.J.D.E SARY,F.P ROSCHAN and D.W.W ALKUP(1967).Association of random variables with applications. Annals Mathematical Statistics,vol.38,pp.1466-1474.W.D.E WAN and K.W.K EMP(1960).Sampling inspection of continuous processes with no autocorrelation between successive results.Biometrika,vol.47,pp.263-280.G.F AVIER and A.S MOLDERS(1984).Adaptive smoother-predictors for tracking maneuvering targets.Proc. 23rd Conf.Decision and Control,Las Vegas,NV,pp.831-836.W.F ELLER(1966).An Introduction to Probability Theory and Its Applications,vol.2.Series in Probability and Mathematical Statistics,Wiley,New York.R.A.F ISHER(1925).Theory of statistical estimation.Proc.Cambridge Philosophical Society,vol.22, pp.700-725.M.F ISHMAN(1988).Optimization of the algorithm for the detection of a disorder,based on the statistic of exponential smoothing.In Statistical Problems of Control,Issue83,Vilnius,pp.146-151.R.F LETCHER(1980).Practical Methods of Optimization,2volumes.Wiley,New York.P.M.F RANK(1990).Fault diagnosis in dynamic systems using analytical and knowledge based redundancy -A survey and new results.Automatica,vol.26,pp.459-474.430B IBLIOGRAPHY P.M.F RANK(1991).Enhancement of robustness in observer-based fault detection.Proc.SAFEPRO-CESS’91,Baden-Baden,FRG,pp.275-287.P.M.F RANK and J.W¨UNNENBERG(1989).Robust fault diagnosis using unknown input observer schemes. In Fault Diagnosis in Dynamic Systems-Theory and Application(R.Patton,P.Frank,R.Clark,eds.). International Series in Systems and Control Engineering,Prentice Hall International,London,UK,pp.47-98.K.F UKUNAGA(1990).Introduction to Statistical Pattern Recognition,2d ed.Academic Press,New York. S.I.G ASS(1958).Linear Programming:Methods and Applications.McGraw Hill,New York.W.G E and C.Z.F ANG(1989).Extended robust observation approach for failure isolation.Int.Jal Control, vol.49,no5,pp.1537-1553.W.G ERSCH(1986).Two applications of parametric time series modeling methods.In Mechanical Signature Analysis-Theory and Applications(S.Braun,ed.),chap.10.Academic Press,London.J.J.G ERTLER(1988).Survey of model-based failure detection and isolation in complex plants.IEEE Control Systems Magazine,vol.8,no6,pp.3-11.J.J.G ERTLER(1991).Analytical redundancy methods in fault detection and isolation.Proc.SAFEPRO-CESS’91,Baden-Baden,FRG,pp.9-22.B.K.G HOSH(1970).Sequential Tests of Statistical Hypotheses.Addison-Wesley,Cambridge,MA.I.N.G IBRA(1975).Recent developments in control charts techniques.Jal Quality Technology,vol.7, pp.183-192.J.P.G ILMORE and R.A.M C K ERN(1972).A redundant strapdown inertial reference unit(SIRU).Jal Space-craft,vol.9,pp.39-47.M.A.G IRSHICK and H.R UBIN(1952).A Bayes approach to a quality control model.Annals Mathematical Statistics,vol.23,pp.114-125.A.L.G OEL and S.M.W U(1971).Determination of the ARL and a contour nomogram for CUSUM charts to control normal mean.Technometrics,vol.13,no2,pp.221-230.P.L.G OLDSMITH and H.W HITFIELD(1961).Average run lengths in cumulative chart quality control schemes.Technometrics,vol.3,pp.11-20.G.C.G OODWIN and K.S.S IN(1984).Adaptive Filtering,Prediction and rmation and System Sciences Series,Prentice Hall,Englewood Cliffs,NJ.R.M.G RAY and L.D.D AVISSON(1986).Random Processes:a Mathematical Approach for Engineers. Information and System Sciences Series,Prentice Hall,Englewood Cliffs,NJ.C.G UEGUEN and L.L.S CHARF(1980).Exact maximum likelihood identification for ARMA models:a signal processing perspective.Proc.1st EUSIPCO,Lausanne.D.E.G USTAFSON, A.S.W ILLSKY,J.Y.W ANG,M.C.L ANCASTER and J.H.T RIEBWASSER(1978). ECG/VCG rhythm diagnosis using statistical signal analysis.Part I:Identification of persistent rhythms. Part II:Identification of transient rhythms.IEEE Trans.Biomedical Engineering,vol.BME-25,pp.344-353 and353-361.F.G USTAFSSON(1991).Optimal segmentation of linear regression parameters.Proc.IFAC/IFORS Symp. Identification and System Parameter Estimation,Budapest,pp.225-229.T.H¨AGGLUND(1983).New Estimation Techniques for Adaptive Control.Ph.D.Thesis,Lund Institute of Technology,Lund,Sweden.T.H¨AGGLUND(1984).Adaptive control of systems subject to large parameter changes.Proc.IFAC9th World Congress,Budapest.B IBLIOGRAPHY431 P.H ALL and C.C.H EYDE(1980).Martingale Limit Theory and its Application.Probability and Mathemat-ical Statistics,a Series of Monographs and Textbooks,Academic Press,New York.W.J.H ALL,R.A.W IJSMAN and J.K.G HOSH(1965).The relationship between sufficiency and invariance with applications in sequential analysis.Ann.Math.Statist.,vol.36,pp.576-614.E.J.H ANNAN and M.D EISTLER(1988).The Statistical Theory of Linear Systems.Series in Probability and Mathematical Statistics,Wiley,New York.J.D.H EALY(1987).A note on multivariate CuSum procedures.Technometrics,vol.29,pp.402-412.D.M.H IMMELBLAU(1970).Process Analysis by Statistical Methods.Wiley,New York.D.M.H IMMELBLAU(1978).Fault Detection and Diagnosis in Chemical and Petrochemical Processes. Chemical Engineering Monographs,vol.8,Elsevier,Amsterdam.W.G.S.H INES(1976a).A simple monitor of a system with sudden parameter changes.IEEE r-mation Theory,vol.IT-22,no2,pp.210-216.W.G.S.H INES(1976b).Improving a simple monitor of a system with sudden parameter changes.IEEE rmation Theory,vol.IT-22,no4,pp.496-499.D.V.H INKLEY(1969).Inference about the intersection in two-phase regression.Biometrika,vol.56,no3, pp.495-504.D.V.H INKLEY(1970).Inference about the change point in a sequence of random variables.Biometrika, vol.57,no1,pp.1-17.D.V.H INKLEY(1971).Inference about the change point from cumulative sum-tests.Biometrika,vol.58, no3,pp.509-523.D.V.H INKLEY(1971).Inference in two-phase regression.Jal American Statistical Association,vol.66, no336,pp.736-743.J.R.H UDDLE(1983).Inertial navigation system error-model considerations in Kalmanfiltering applica-tions.In Control and Dynamic Systems(C.T.Leondes,ed.),Academic Press,New York,pp.293-339.J.S.H UNTER(1986).The exponentially weighted moving average.Jal Quality Technology,vol.18,pp.203-210.I.A.I BRAGIMOV and R.Z.K HASMINSKII(1981).Statistical Estimation-Asymptotic Theory.Applications of Mathematics Series,vol.16.Springer,New York.R.I SERMANN(1984).Process fault detection based on modeling and estimation methods-A survey.Auto-matica,vol.20,pp.387-404.N.I SHII,A.I WATA and N.S UZUMURA(1979).Segmentation of nonstationary time series.Int.Jal Systems Sciences,vol.10,pp.883-894.J.E.J ACKSON and R.A.B RADLEY(1961).Sequential and tests.Annals Mathematical Statistics, vol.32,pp.1063-1077.B.J AMES,K.L.J AMES and D.S IEGMUND(1988).Conditional boundary crossing probabilities with appli-cations to change-point problems.Annals Probability,vol.16,pp.825-839.M.K.J EERAGE(1990).Reliability analysis of fault-tolerant IMU architectures with redundant inertial sen-sors.IEEE Trans.Aerospace and Electronic Systems,vol.AES-5,no.7,pp.23-27.N.L.J OHNSON(1961).A simple theoretical approach to cumulative sum control charts.Jal American Sta-tistical Association,vol.56,pp.835-840.N.L.J OHNSON and F.C.L EONE(1962).Cumulative sum control charts:mathematical principles applied to their construction and use.Parts I,II,III.Industrial Quality Control,vol.18,pp.15-21;vol.19,pp.29-36; vol.20,pp.22-28.432B IBLIOGRAPHY R.A.J OHNSON and M.B AGSHAW(1974).The effect of serial correlation on the performance of CUSUM tests-Part I.Technometrics,vol.16,no.1,pp.103-112.H.L.J ONES(1973).Failure Detection in Linear Systems.Ph.D.Thesis,Dept.Aeronautics and Astronautics, MIT,Cambridge,MA.R.H.J ONES,D.H.C ROWELL and L.E.K APUNIAI(1970).Change detection model for serially correlated multivariate data.Biometrics,vol.26,no2,pp.269-280.M.J URGUTIS(1984).Comparison of the statistical properties of the estimates of the change times in an autoregressive process.In Statistical Problems of Control,Issue65,Vilnius,pp.234-243(in Russian).T.K AILATH(1980).Linear rmation and System Sciences Series,Prentice Hall,Englewood Cliffs,NJ.L.V.K ANTOROVICH and V.I.K RILOV(1958).Approximate Methods of Higher Analysis.Interscience,New York.S.K ARLIN and H.M.T AYLOR(1975).A First Course in Stochastic Processes,2d ed.Academic Press,New York.S.K ARLIN and H.M.T AYLOR(1981).A Second Course in Stochastic Processes.Academic Press,New York.D.K AZAKOS and P.P APANTONI-K AZAKOS(1980).Spectral distance measures between gaussian pro-cesses.IEEE Trans.Automatic Control,vol.AC-25,no5,pp.950-959.K.W.K EMP(1958).Formula for calculating the operating characteristic and average sample number of some sequential tests.Jal Royal Statistical Society,vol.B-20,no2,pp.379-386.K.W.K EMP(1961).The average run length of the cumulative sum chart when a V-mask is used.Jal Royal Statistical Society,vol.B-23,pp.149-153.K.W.K EMP(1967a).Formal expressions which can be used for the determination of operating character-istics and average sample number of a simple sequential test.Jal Royal Statistical Society,vol.B-29,no2, pp.248-262.K.W.K EMP(1967b).A simple procedure for determining upper and lower limits for the average sample run length of a cumulative sum scheme.Jal Royal Statistical Society,vol.B-29,no2,pp.263-265.D.P.K ENNEDY(1976).Some martingales related to cumulative sum tests and single server queues.Stochas-tic Processes and Appl.,vol.4,pp.261-269.T.H.K ERR(1980).Statistical analysis of two-ellipsoid overlap test for real time failure detection.IEEE Trans.Automatic Control,vol.AC-25,no4,pp.762-772.T.H.K ERR(1982).False alarm and correct detection probabilities over a time interval for restricted classes of failure detection algorithms.IEEE rmation Theory,vol.IT-24,pp.619-631.T.H.K ERR(1987).Decentralizedfiltering and redundancy management for multisensor navigation.IEEE Trans.Aerospace and Electronic systems,vol.AES-23,pp.83-119.Minor corrections on p.412and p.599 (May and July issues,respectively).R.A.K HAN(1978).Wald’s approximations to the average run length in cusum procedures.Jal Statistical Planning and Inference,vol.2,no1,pp.63-77.R.A.K HAN(1979).Somefirst passage problems related to cusum procedures.Stochastic Processes and Applications,vol.9,no2,pp.207-215.R.A.K HAN(1981).A note on Page’s two-sided cumulative sum procedures.Biometrika,vol.68,no3, pp.717-719.B IBLIOGRAPHY433 V.K IREICHIKOV,V.M ANGUSHEV and I.N IKIFOROV(1990).Investigation and application of CUSUM algorithms to monitoring of sensors.In Statistical Problems of Control,Issue89,Vilnius,pp.124-130(in Russian).G.K ITAGAWA and W.G ERSCH(1985).A smoothness prior time-varying AR coefficient modeling of non-stationary covariance time series.IEEE Trans.Automatic Control,vol.AC-30,no1,pp.48-56.N.K LIGIENE(1980).Probabilities of deviations of the change point estimate in statistical models.In Sta-tistical Problems of Control,Issue83,Vilnius,pp.80-86(in Russian).N.K LIGIENE and L.T ELKSNYS(1983).Methods of detecting instants of change of random process prop-erties.Automation and Remote Control,vol.44,no10,Part II,pp.1241-1283.J.K ORN,S.W.G ULLY and A.S.W ILLSKY(1982).Application of the generalized likelihood ratio algorithm to maneuver detection and estimation.Proc.American Control Conf.,Arlington,V A,pp.792-798.P.R.K RISHNAIAH and B.Q.M IAO(1988).Review about estimation of change points.In Handbook of Statistics(P.R.Krishnaiah,C.R.Rao,eds.),vol.7,Elsevier,New York,pp.375-402.P.K UDVA,N.V ISWANADHAM and A.R AMAKRISHNAN(1980).Observers for linear systems with unknown inputs.IEEE Trans.Automatic Control,vol.AC-25,no1,pp.113-115.S.K ULLBACK(1959).Information Theory and Statistics.Wiley,New York(also Dover,New York,1968). K.K UMAMARU,S.S AGARA and T.S¨ODERSTR¨OM(1989).Some statistical methods for fault diagnosis for dynamical systems.In Fault Diagnosis in Dynamic Systems-Theory and Application(R.Patton,P.Frank,R. Clark,eds.).International Series in Systems and Control Engineering,Prentice Hall International,London, UK,pp.439-476.A.K USHNIR,I.N IKIFOROV and I.S AVIN(1983).Statistical adaptive algorithms for automatic detection of seismic signals-Part I:One-dimensional case.In Earthquake Prediction and the Study of the Earth Structure,Naouka,Moscow(Computational Seismology,vol.15),pp.154-159(in Russian).L.L ADELLI(1990).Diffusion approximation for a pseudo-likelihood test process with application to de-tection of change in stochastic system.Stochastics and Stochastics Reports,vol.32,pp.1-25.T.L.L A¨I(1974).Control charts based on weighted sums.Annals Statistics,vol.2,no1,pp.134-147.T.L.L A¨I(1981).Asymptotic optimality of invariant sequential probability ratio tests.Annals Statistics, vol.9,no2,pp.318-333.D.G.L AINIOTIS(1971).Joint detection,estimation,and system identifirmation and Control, vol.19,pp.75-92.M.R.L EADBETTER,G.L INDGREN and H.R OOTZEN(1983).Extremes and Related Properties of Random Sequences and Processes.Series in Statistics,Springer,New York.L.L E C AM(1960).Locally asymptotically normal families of distributions.Univ.California Publications in Statistics,vol.3,pp.37-98.L.L E C AM(1986).Asymptotic Methods in Statistical Decision Theory.Series in Statistics,Springer,New York.E.L.L EHMANN(1986).Testing Statistical Hypotheses,2d ed.Wiley,New York.J.P.L EHOCZKY(1977).Formulas for stopped diffusion processes with stopping times based on the maxi-mum.Annals Probability,vol.5,no4,pp.601-607.H.R.L ERCHE(1980).Boundary Crossing of Brownian Motion.Lecture Notes in Statistics,vol.40,Springer, New York.L.L JUNG(1987).System Identification-Theory for the rmation and System Sciences Series, Prentice Hall,Englewood Cliffs,NJ.。
阿尔茨海默病假说
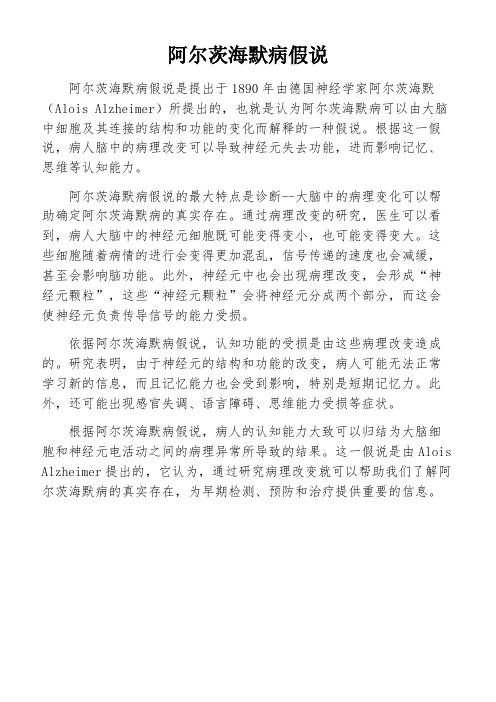
阿尔茨海默病假说
阿尔茨海默病假说是提出于1890年由德国神经学家阿尔茨海默(Alois Alzheimer)所提出的,也就是认为阿尔茨海默病可以由大脑中细胞及其连接的结构和功能的变化而解释的一种假说。
根据这一假说,病人脑中的病理改变可以导致神经元失去功能,进而影响记忆、
思维等认知能力。
阿尔茨海默病假说的最大特点是诊断--大脑中的病理变化可以帮
助确定阿尔茨海默病的真实存在。
通过病理改变的研究,医生可以看到,病人大脑中的神经元细胞既可能变得变小,也可能变得变大。
这
些细胞随着病情的进行会变得更加混乱,信号传递的速度也会减缓,
甚至会影响脑功能。
此外,神经元中也会出现病理改变,会形成“神
经元颗粒”,这些“神经元颗粒”会将神经元分成两个部分,而这会
使神经元负责传导信号的能力受损。
依据阿尔茨海默病假说,认知功能的受损是由这些病理改变造成的。
研究表明,由于神经元的结构和功能的改变,病人可能无法正常
学习新的信息,而且记忆能力也会受到影响,特别是短期记忆力。
此外,还可能出现感官失调、语言障碍、思维能力受损等症状。
根据阿尔茨海默病假说,病人的认知能力大致可以归结为大脑细
胞和神经元电活动之间的病理异常所导致的结果。
这一假说是由Alois Alzheimer提出的,它认为,通过研究病理改变就可以帮助我们了解阿尔茨海默病的真实存在,为早期检测、预防和治疗提供重要的信息。
阿兹海默氏病的诊断、预后和监测中的寡聚体Aβ[发明专利]
![阿兹海默氏病的诊断、预后和监测中的寡聚体Aβ[发明专利]](https://img.taocdn.com/s3/m/eaea0110aef8941ea66e05d0.png)
专利名称:阿兹海默氏病的诊断、预后和监测中的寡聚体Aβ专利类型:发明专利
发明人:丹尼尔·基德,约翰尼斯·鲁尔夫·施特雷费尔
申请号:CN201380021783.5
申请日:20130313
公开号:CN104662423A
公开日:
20150527
专利内容由知识产权出版社提供
摘要:本发明提供了用于阿兹海默氏病的诊断、预后和监测的方法。
所述方法包括测量从受试者获得的样品中合并的单体和寡聚体Aβ的量和单体Aβ的量,并确定比率。
所述比率可用于诊断、预后和/或监测阿兹海默氏病。
申请人:杨森阿尔茨海默氏症免疫治疗公司
地址:爱尔兰都柏林
国籍:IE
代理机构:中原信达知识产权代理有限责任公司
更多信息请下载全文后查看。
Estimation Theory
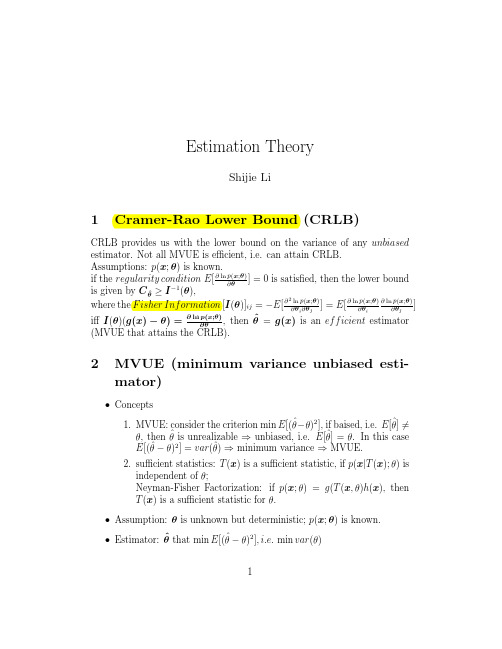
Estimation TheoryShijie Li1Cramer-Rao Lower Bound(CRLB)CRLB provides us with the lower bound on the variance of any unbiased estimator.Not all MVUE is efficient,i.e.can attain CRLB. Assumptions:p(x;θ)is known.if the regularity condition E[∂ln p(x;θ)∂θ]=0is satisfied,then the lower boundis given by Cˆθ≥I−1(θ),where the F isher Information[I(θ)]ij=−E[∂2ln p(x;θ)∂θi∂θj ]=E[∂ln p(x;θ)∂θi∂ln p(x;θ)∂θj]iffI(θ)(g(x)−θ)=∂ln p(x;θ)∂θ,thenˆθ=g(x)is an efficient estimator(MVUE that attains the CRLB).2MVUE(minimum variance unbiased esti-mator)•Concepts1.MVUE:consider the criterion min E[(ˆθ−θ)2],if baised,i.e.E[ˆθ]=θ,thenˆθis unrealizable⇒unbiased,i.e.E[ˆθ]=θ.In this caseE[(ˆθ−θ)2]=var(ˆθ)⇒minimum variance⇒MVUE.2.sufficient statistics:T(x)is a sufficient statistic,if p(x|T(x);θ)isindependent ofθ;Neyman-Fisher Factorization:if p(x;θ)=g(T(x,θ)h(x),thenT(x)is a sufficient statistic forθ.•Assumption:θis unknown but deterministic;p(x;θ)is known.•Estimator:ˆθthat min E[(ˆθ−θ)2],i.e.min var(θ)1•General way tofind MVUE(RBLS)1.find the sufficient statistic T(x)(Neyman-Fisher factorization the-orem);2.determin whether T(x)is complete(all exponential family of PDFsare complete);3.find a function of T(x)that is unbaised,i.e.ˆθ=g(T(x)),thenˆθis the MVUE.Alternately,evaluateˆθ=E[ˇθ|T(x)](ˇθis unbaised),can also get the MVUE,but too tedious.3BLUE(best linear unbiased estimator)•Assumptions:θis unknown but deterministic;data model:x=Hθ+w, where w with zero mean and covariance C(no specific PDF is needed).•Estimator:ˆθ=Ax and min E[(ˆθ−θ)2],i.e.min var(θ)Soˆθ=(H T C−1H)−1H T C−1x•Comments:Generally,BLUE is a suboptimal estimator,not necessarilya MVUE.However,for this model,if w is Gaussian,then BLUE willbe a MVUE.4MLE(maximum likelihood estimator)•Assumptions:θis unknown but deterministic;p(x;θ)is known.•Estimator:ˆθthat max p(x;θ)•Comments:if an efficient estimator exists,then MLE turns out to bean efficient MVUE;if not,MLE is asymptotically efficient,andˆθisasymptotically distributed according to N(θ,I−1(θ)).•special caselinear model:x=Hθ+w,where H:full rank;w∼N(0,C)Thenˆθ=(H T C−1H)−1H T C−1x.In this case,ˆθis an efficientestimator,hence the MVUE.25LSE (least squares estimator)•Assumption:data model is x =s (θ)+w ,where s =Hθand H is of full rank.•Estimator:ˆθthat min (x −Hθ)T (x −Hθ)⇒ˆθ=(H T H )−1H T x (use geometrical interpretation to understand)•Comments:if C =δ2I ,then LSE is identical to the MLE,however,we do not need to know the specific PDF p (x ;θ).6Bayesian Estimation Approaches•Assumptions:θis an unknown,random vector;prior probability of θ(P (θ)),likelihood (P (x |θ))and the cost function are known.According to different cost functions,different estimators are listed below:•Estimators:1.MMSE (minimum mean squar error)cost function:C ( )= 2min E [(θi −ˆθ)2],w.r.t.the joint PDF P (x ,θi )⇒ˆθ=E [θ|x ].Comments:MMSE is a conditional mean estimator .The diffi-culty of MMSE estimator lie in the closed form of E [θ|x ].Hence,in non-Gaussian case,it’s difficult to implement (multidimensional integration).2.MMAE (minimum mean absolute error)cost function:C ( )=| |ˆθthat min E [|θ−ˆθ|]⇒ˆθis the point such that P (θ<t |x )≤P (θ>t |x ),t <ˆθP (θ<t |x )≥P (θ>t |x ),t >ˆθComments:MMAE estimator is a conditional median estimator .3.MAP (maxmimum a posterior)cost function:C ( )= 0,| |<δ1,| |>δmax P (θ|x )equals to max P (x |θ)P (θ)Comments:MAP is a conditional mode estimator ,so for posterior3PDFs whos mean and mode are the same,MMSE and MAP will be the same(eg.Gaussian PDF).4.LMMSE(linear minimum mean square error)Assumptions:thefirst two moments ofθ,x are known,i.e.E[θ],E[x],C xx,Cθθ,C xθ,Cθx.cost function:the same with MMSE,but with the constraint that the estimator is linear,i.e.ˆθ=AxComments:it is a suboptimal estimator,however,if the MMSE estimator(ˆθ=E[θ|x])happens to be linear,the LMMSE will be optimal and identical to MMSE.4。
marginal fisher analysis -回复
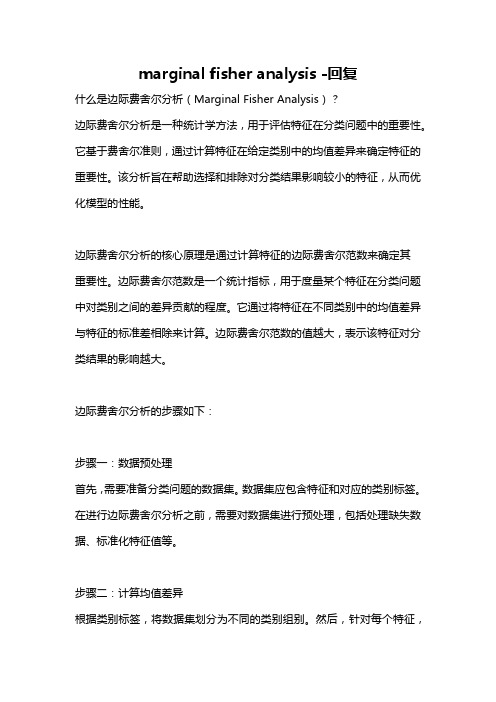
marginal fisher analysis -回复什么是边际费舍尔分析(Marginal Fisher Analysis)?边际费舍尔分析是一种统计学方法,用于评估特征在分类问题中的重要性。
它基于费舍尔准则,通过计算特征在给定类别中的均值差异来确定特征的重要性。
该分析旨在帮助选择和排除对分类结果影响较小的特征,从而优化模型的性能。
边际费舍尔分析的核心原理是通过计算特征的边际费舍尔范数来确定其重要性。
边际费舍尔范数是一个统计指标,用于度量某个特征在分类问题中对类别之间的差异贡献的程度。
它通过将特征在不同类别中的均值差异与特征的标准差相除来计算。
边际费舍尔范数的值越大,表示该特征对分类结果的影响越大。
边际费舍尔分析的步骤如下:步骤一:数据预处理首先,需要准备分类问题的数据集。
数据集应包含特征和对应的类别标签。
在进行边际费舍尔分析之前,需要对数据集进行预处理,包括处理缺失数据、标准化特征值等。
步骤二:计算均值差异根据类别标签,将数据集划分为不同的类别组别。
然后,针对每个特征,在每个类别组别中计算其均值。
这些均值将用于计算特征的均值差异,即该特征在不同类别之间的差异程度。
步骤三:计算标准差同样,对于每个特征,在每个类别组别中计算其标准差。
标准差度量了特征值的变化范围,用于衡量特征对分类结果的稳定性和一致性。
步骤四:计算边际费舍尔范数根据均值差异和标准差,计算每个特征的边际费舍尔范数。
边际费舍尔范数的计算公式为均值差异除以标准差。
步骤五:特征选择和排除根据特征的边际费舍尔范数,可以确定哪些特征对分类结果的影响最大。
边际费舍尔范数越大的特征越重要,在模型训练过程中应优先考虑。
相反,边际费舍尔范数较小的特征可以被排除,以减少特征维度,提高模型的性能。
步骤六:模型训练和评估在确定了重要特征后,可以使用这些特征来训练分类模型。
根据实际情况选择适当的分类模型,如逻辑回归、支持向量机等。
使用训练好的模型对新数据进行分类,并根据评估指标(如准确率、召回率等)评估模型的性能。
教材中“关于酶本质的探索”的几点探讨
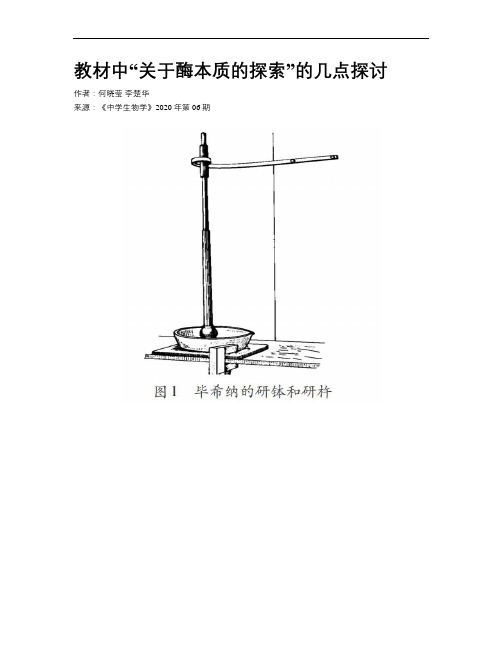
教材中“关于酶本质的探索”的几点探讨作者:何晓莹李楚华来源:《中学生物学》2020年第06期摘要人教版高中生物必修1的教材在“关于酶本质的探索”这部分科学史中的相关描述较为简略,易使师生产生疑问和误解。
通过查阅相关文献,尝试对巴斯德、毕希纳实验的背景和具体过程等问题进行探讨和分析,为该部分科学史的教学设计提供一些参考和依据。
关键词巴斯德毕希纳无细胞酵解实验中图分类号Q-49文献标志码 E在人教版高中生物《必修1.分子与细胞》的第五章第一节“降低化学反应活化能的酶”中插入了“关于酶本质的探索”的科学史内容,但是关于巴斯德、李比希、毕希纳的相关描述比较简略,容易引发师生的疑问和误解。
例如,巴斯德实验的反应物是葡萄糖,这是真的吗?当巴斯德和李比希的观点相悖时,伟大的科学家巴斯德竟想不出做活酵母和死亡酵母提取物的对比实验吗?如果巴斯德尝试了,为什么不成功?毕希纳所做的实验看起来比较简单,在30年间,竟没有一个人尝试这种方法吗?毕希纳的实验过程真的是这样的吗?通过查阅国内外的相关文献,发现,有关毕希纳的无细胞酵解实验在国内的文章中极少,国外则比较多。
在此基础上,尝试对上述的问题做出较科学的回答,呈现较清晰的有关酶的发现的科学史,以便为该部分的教学设计提供一定的参考。
1 背景酶在古代就已经比较广泛地应用于生产和生活实践,比如我国人民夏禹时代就会酿酒,周朝就会制饴、制酱,春秋战国时期就会用曲来治疗消化不良的疾病等。
但在很长一段时间内,人们并不清楚发酵的具体过程是怎样的。
直至18世纪末,人们才认识到,在发酵过程中糖类分解成了酒精和二氧化碳。
1810年,盖·吕萨克(CayLussac)表明发酵后酒精和二氧化碳的重量之和几乎等于糖的重量。
当时人们认为,发酵中产生的酵母不过是其中的一种副产品。
在1837年,拉图尔(Cagniard Latour)、库茨因格(Friedrich Kutzing)和施旺(Theodor Schwann)先后用显微镜(放大倍数约为500倍)观察酵母,他们得出了一致的结论:酵母是活的有机体。
“老年超人”越老越聪明大脑保持年轻态

“老年超人”越老越聪明大脑保持年轻态腾讯科技[微博]2015年02月07日07:08最新研究显示,“老年超人”具有独特的大脑,比同龄人的智力等级更高,记忆力更强。
腾讯科学讯据国外媒体报道,目前,研究人员发现存在着“老年超人”,他们虽然已有80多岁,却拥有50岁人群的大脑智力。
他们发现老年超人与正常老年人的大脑存在着差异性,他们具有年轻人的记忆能力,深入理解老年超人的独特大脑,有助于老年痴呆症的治疗。
这项最新研究报告发表在1月28日出版的《神经科学》杂志上,首次量化识别了老年超人与正常老年人之间的大脑差异性。
2007年,美国西北大学认知神经学家首次识别发现老年超人,这些特殊人群与同龄人群相比,具有3种独特的大脑特征——皮质层较厚;神经缠结较少和大量von Economo神经细胞,据悉,神经缠结较多是阿兹海默症的主要标志,von Economo神经细胞与较高社交智商密切相关。
认知神经学和阿兹海默症研究中心的资深教授钱吉兹-盖乌拉(Changiz Geula)是该项研究负责人,他说:“当老年超人与普通老年人进行对比,他们存在独特的差异性或者大脑结构特殊性。
其形成原因可能是特殊基因表现,或者是提供保护性的综合因素。
”研究报告第一作者、西北大学范伯格医学院临床神经心理学博士研究生塔马尔-格芬(Tamar Gefen)指出,识别老年超人独特记忆能力的形成因素,将有助于我们提高老年人群的认知能力,并对治疗某些痴呆症带来新的希望。
科学家认为von Economo神经细胞对于快速传输与社会互动行为有关信息具有至关重要的作用,同时,这种神经细胞还有助于形成较好的记忆能力。
vonEconomo神经细胞存在于一些动物体内,例如:鲸鱼、大象、海豚和较高智商猿类物种。
(悠悠/编译)。
统计学ppt(全)

1 -2
经济、管理类 基础课程
统计学
第一节 统计与统计学
一. 统计与统计学的含义 二. 统计学的性质和作用
1 -3
经济、管理类 基础课程
统计学
1 -4
一、什么是统计?
1. 统计工作
收集数据的活动
2. 统计数据
▪ 对现象计量的结果
3. 统计学
分析数据的方法与技术
经济、管理类 基础课程
统计学
什么是统计学?
总量指标、相对指标和平均指标
3. 按计量单位
实物指标、价格指标和劳动量指标
1 - 35
经济、管理类 基础课程
统计学
统计指标体系
由若干个相互联
系相互制约的统计指 标组成的一个统计指 标系统
•基本统计指标体系
•专题统计指标体系
1 - 36
经济、管理类 基础课程
2. 17世纪中叶的政治算术学派可看作是统计学的开端
3. 19世纪,沿着约翰·格朗特所开创的人口统计以及 沿着威廉·配第所开创的经济统计有了进一步的发 展
4. 威廉·配第为以后经济统计的发展开拓了道路;约 翰·格朗特为人口统计的发展开拓了道路
5. 政治算术学派则为后来的社会经济统计的发展奠定 了基础
Thomas Robert Malthus (马尔萨斯) (1766-1834)
1 - 19
Johann Gregor Mendel (孟德尔) (1822-1884) Pierre Simon Laplace (拉普拉斯) (1749-1827)
经济、管理类 基础课程
统计学
历史上著名的统计学家
Jacob Bernoulli (伯努利) (1654-1705) Edmond Halley (哈雷) (1656-1742) De Moivre (棣美佛) (1667-1754) Thomas Bayes (贝叶斯) (1702-1761) Leonhard Euler (欧拉) (1707-1783) Pierre Simon Laplace (拉普拉斯) (1749-1827) Adrien Marie Legendre (勒让德) (1752-1833) Thomas Robert Malthus (马尔萨斯) (1766-1834) Friedrich Gauss (高斯) (1777-1855) Johann Gregor Mendel (孟德尔) (1822-1884) Karl Pearson (皮尔森) (1857-1936) Ronald Aylmer Fisher (费歇) (1890-1962) Jerzy Neyman (内曼)(1894-1981) Egon Sharpe Pearson (皮尔森) (1895-1980)
充分 完备

一.充分性充分性和完备性是数理统计中两个很重要的基本概念,它也有助于寻找一致最小方差无偏估计。
例1设总体ξ服从正态分布N(μ,1),要求估计均值。
在例2.5中已经指出样本均值是μ的一个有效估计。
由于一个容量为n的样本包含了n个值。
而现在估计μ时,对这n个资料进行了“压缩”和“精简”,仅用一个单值函数,这里自然的会提出一个问题,这种压缩是否合理?也就是说,是否已经包含了样本关于μ的全部信息?用样本的个别值或其它形式能否更多地知道μ呢?下面就回答这个问题。
由于是n维空间中的一个点,而当已知时,样本是n-1维空间中的点,因而上面提出的问题就归结为假若已知时,进一步知道样本在这n-1维空间中的位置,关于μ能否获得更多的信息?为说明简便,对n=2时进行考虑。
此时样本空间可以用平面来表示,表示该平面中的一条直线。
在这平面上,服从二元正态分布,它的分布密度函数是在直线上,的分布可以用条件分布密度函数描写这里所以由上式可见条件密度函数与μ无关,这说明在已知时,如果再进一步知道样本点在这条直线上的位置,关于μ已不能再给出任何新的信息。
换句话说,已充分的提出了样本中所包含的关于μ的全部信息。
所以关于μ的任何推断,不需要记录全部数据,只需记录就足够了,基于关于μ的任何分析与基于全部=样本数据关于μ的分析是同等有效的。
此时称是μ的充分统计量。
下面给出充分统计量的一般定义。
定义 3.3.1 设是从具有分布族为的总体中抽取的一个样本,是一统计量(可以是向量)。
如果给定,的条件分布(离散型变量为条件概率,连续型变量为条件密度)与参数θ无关,则称统计量是分布族的充分统计量。
或称是θ的充分统计量。
按照定义3.3.1,显然是一个充分性统计量,但它没有起到“压缩”数据的作用,因此是没有价值的。
例2设总体ξ服从贝努里分布b(1,p),是一样本。
,其中ν表示中取值为1的频数。
下面计算关于的条件概率是(若设)它与p无关,所以是p的充分统计量。
Neyman-Pearson基本引理

犯第二类错误的概率
( ) P ( X W ) 1 P ( X W ), 1
() 1- () 不同
( ) P ( X W ) 1 P ( X W )
(n ) k n P (T c) e , k! k 0
c 1
给定(0,1),有且只有下列两种情况: i) 存在00,使G(0)=
0
G ( )
ii) 存在00,使G(0)< G(0-0)
0 与 1 是 的互不相交的非空子集.
给定H0和H1就等于给定检验问题,记为检验问题(H0,H1).
2. 定义:在检验问题(H0,H1)中,检验法则(简称检验法或检验) 就是设法把样本空间X划分为互不相交的两个可测集:
X W W
并规定: 当观测值xW时,就拒绝原假设H0,认为备择假设H1成立. 当观测值xW时(即 x W ),就不拒绝H0,认为原假设H1成立, 称W为检验的拒绝域. 选定了检验法确定了拒绝域 chap7
在0 时,g()≤ 的检验称为水平为的检验,记为(,0, 1)检验.
常取0.1,0.05,0.01等值.
chap7
根据检验的水平确定临界值c
(n ) k n e 是的严格增函数. 例 1 中 g ( ) k! k c 给定,在≤1(H0)时控制犯第一类错的概率 g ( )
1.
由以上两式可知 n 固定时不可能使得犯两类错的概率都减少.
(n ) k n P(T k ) e , k 0,1, chap7 k!
6
4.势函数的定义: 称样本观察值落在拒绝域的概率为检验的势函数(功效函数), 记为
g ( ) P ( X W ),
应用数理统计常用专业英语
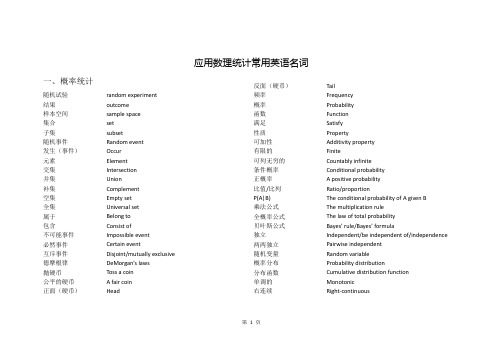
应用数理统计常用英语名词一、概率统计随机试验结果样本空间集合子集随机事件发生(事件)元素交集并集补集空集全集属于包含不可能事件必然事件互斥事件德摩根律抛硬币公平的硬币正面(硬币)random experimentoutcomesample spacesetsubsetRandom eventOccurElementIntersectionUnionComplementEmpty setUniversal setBelong toConsist ofImpossible eventCertain eventDisjoint/mutually exclusiveDeMorgan’s lawsToss a coinA fair coinHead反面(硬币)频率概率函数满足性质可加性有限的可列无穷的条件概率正概率比值/比列P(A|B)乘法公式全概率公式贝叶斯公式独立两两独立随机变量概率分布分布函数单调的右连续TailFrequencyProbabilityFunctionSatisfyPropertyAdditivity propertyFiniteCountably infiniteConditional probabilityA positive probabilityRatio/proportionThe conditional probability of A given BThe multiplication ruleThe law of total probabilityBayes’ rule/Bayes’ formulaIndependent/be independent of/independencePairwise independentRandom variableProbability distributionCumulative distribution functionMonotonicRight-continuous离散型随机变量分布律可能的取值伯努利分布参数二项分布泊松分布连续性型随机变量概率密度函数均匀分布区间示性函数指数分布无记忆性正态分布标准正态分布分位数数字特征期望/均值常数方差标准差矩二阶矩原点矩中心矩Discrete random variableProbability mass functionPossible valueBernoulli distributionParameterBinomial distributionPoisson distributionContinuous random variableProbability density functionUniform distributionIntervalIndicator functionExponential distributionMemoryless propertyNormal distribution/Gauss distributionStandard normal distributionQuartileNumerical characteristicsExpectation/meanConstantVarianceStandard deviationMomentThe second momentOrigin momentCentral moment特征函数联合分布联合分布函数边缘分布边缘分布函数联合分布率边缘分布率联合概率密度函数边缘概率密度函数协方差相关不相关的相关系数大数定律弱大数定律强大数定律弱收敛依分布收敛依概率收敛几乎处处几乎必然切比雪夫不等式中心极限定理标准化近似Characteristic functionJoint distributionJoint distribution functionMarginal distributionMarginal distribution functionJoint probability mass functionMarginal probability mass functionJoint probability density functionMarginal probability density functionCovarianceCorrelationUncorrelatedCorrelation coefficientThe law of large numbersWeak law of large numbersStrong law of large numbersWeak convergenceConvergence in distributionConvergence in probabilityAlmost everywhereAlmost surelyChebyshev’s inequalityThe central limit theoremStandardizeApproximation二、统计推断总体随机样本独立同分布样本容量观测统计量压缩样本均值样本方差样本标准差抽样分布卡方分布t分布F分布自由度自由度n的t分布分布族Gamma分布族Fisher Z分布族Beta分布族韦布尔分布族多项分布族指数型分布族参数空间PopulationRandom samplesIndependent and identically distributedSample sizeObservation(sample)statisticReductionSample meanSample varianceSample standard deviationSampling distributionChi-square distributionT-DistributionF-DistributionDegree of freedomT-distribution with n degree of freedomFamilyGamma familyFisher Z familyBeta familyWeibull familyMultinomial familyExponential familyParameter space维数可识别的感兴趣的参数自然参数典则形式参数分布族k维正态分布族矩阵向量对称正定矩阵对称正态分布经验分布函数次序统计量样本中位数稳健样本分位数充分性充分统计量因子分解定理完备性完备统计量探索性数据分析直方图窗宽DimensionIdentifiableParameter of interestNatural parameterCanonical formParametric familyK-dimension normal familyMatrixVectorSymmetric positive definite matrixLog-normal distributionEmpirical distribution functionOrder statisticsSample medianRobustEmpirical quartileSufficiencySufficient statisticThe factorization theoremComplete statisticComplete statisticExploratory data analysisHistogramBin width/bandwidth散点图茎叶图箱线图五数概括最小值下四分位数中位数上四分位数最大值异常值IQR折线图饼图统计推断估计估计量估计值点估计无偏性无偏估计偏差渐进无偏性有效性更有效的均方误差渐近分布Scatter plotStem-and-leaf plotBox plotFive-number summaryMinimumLower quartileMedianUpper quartileMaximumOutlierInter-quartile rangeLine chart/line graphPie chart/pie graph/pie diagramStatistical inferenceEstimationEstimatorestimatePoint estimatorUnbiasednessUnbiased estimatorBiasAsymptotic unbiasednessEfficiencyMore efficientMean square error(MSE)Asymptotic distribution渐近正态性渐进方差矩估计极大似然估计似然函数对数似然函数似然方程变换一致最小方差无偏估计Fisher 信息矩阵信息不等式区间估计置信集置信区间置信水平置信系数置信下限置信上限分位点大样本大样本置信区间单侧置信区间枢轴量一致最精确置信区间假设Asymptotic distributionAsymptotic varianceMoment estimatorMaximum likelihood estimator(MLE)Likelihood functionLoglikelihood functionLikelihood equationTransformationUniformly minimum variance unbiased estimator(UMVUE)Fisher information matrixInformation inequalityInterval estimator/estimateConfidence setConfidence intervalConfidence levelConfidence coefficientLower confidence boundUpper confidence boundCritical valueLarge sampleLarge sample confidence intervalOne-sided confidence intervalPivotal quantityUniformly most accurate(UMA)confidence intervalHypothesis假设检验原假设备择假设检验统计量拒绝接受拒绝域接受域临界值显著性水平检验法则检验函数第一类错误第二类错误功效函数随机化的p值单样本的t检验两样本的t检验NP引理最大功效检验一致最大功效检验似然比似然比检验拟合优度检验列联表Hypothesis test/testingNull/original hypothesisAlternative hypothesisTest statisticRejectAcceptRejection regionAcceptance regionCritical valueSignificance levelTest rulesTesting functionType I errorType II errorPower functionRandomizedP-ValueOne-sample t-testTwo-sample t-testNeyman-Pearson lemmaMost powerful test(MPT)Uniformly most powerful test(UMPT)Likelihood ratioLikelihood ratio test(LRT)Goodness of fit testContingency tableKS检验符号符号检验Wilcoxon符号秩检验Wilcoxon-Mann-Whitney秩和检验游程检验置换置换检验秩秩统计量秩检验简单线性回归模型趋势数据集双变量的单变量的回归直线截距斜率解释变量x响应变量y随机波动最小二乘估计残差方差齐性Kolmogorov-Smirnov testSignSign testWilcoxon signed rank testWilcoxon-Mann-WhitneyRank sun testRun testPermutationPermutation testRankRank statisticRank testSimple linear regression modelTrend/patterndatasetBivariateUnivariateRegression lineInterceptSlopeExplanatory variableResponse variableRandom fluctuationLeast squares estimationResidualHomoscedasticity异方差性多元线性回归Heteroscedasticity Multiple linear regression。
阿尔茨海默病的非治疗药物及新靶点研究进展

o n t h e o r i e s o f 1 3 - a m y l o i d ( A 3 1 ) d e p o s i t i o n , t a u h y p e r p h o s p h o r y l a t i o n a n d n e u r o n  ̄d e g e n e r a t i o n a n d d e a t h a n d o t h e r s .
[ 关 键 词】 阿 尔茨海默 病 ; 非 治 疗药 物 ; 新靶 点 ; 预 防
【 中图分 类 号】R 7 4 9 . 1 6
【 文 献标 识码】 A
[ 文章 编 号】1 6 7 3 — 7 2 1 0( 2 0 1 4) 0 2( c ) 一 0 1 6 2 一 O 5
Re s e a r c h p r o g r e s s f o r n o n —t r e a t me n t d r u g a n d n e w t a r g e t s i n Al z h e i me r
d i s e a s e
HE Yi n g WANG Ch a o WANG De c a l
Ha r b i n Me d i c a l U n i v e r s i t y , He i l o n g j i a n g P r o v i n c e , Ha r b i n 1 5 0 0 8 6 , C h i n a
[ Ke y wo r d s 】 A l z h e i me r d i s e a s e ; No n -t r e a t m e n t d ug r ; N e w t a r g e t ; P r e v e n t
贝叶斯估计
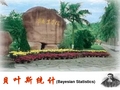
贝塔分布(beta distribution)
若 0, 0 为两个实数,则由下列密度函数
1 1 1 x (1 x ) f ( x) B( , ) 0 0 x 1 x 0, x 1
其中 B( , )
设自然状态有k种, 1,2,…, k, P(i)表示自然状态i发生的先验概率分布, P(x︱i)表示在状态i条件,事件为x的概 率。 P(i ︱x )为i发生的后验概率。 全概率公式:P(x)为x在各种状态下可能出现 的概率综合值。
全概率公式: P ( x) P ( x | i ) P ( i )
p ( x; ) , 它表示在参数空间 { } 中不同的 对应不
同的分布。可在贝叶斯统计中记为 p( x | ) ,它表示 在随机变量 给定某个值时,总体指标 X 的条件分 布。 2、 根据参数 的先验信息确定先验分布 ( ) (prior distribution)。这是贝叶斯学派在最近几十年里重点 研究的问题。已获得一大批富有成效的方法。
( | x)
h( x, ) p( x | ) ( ) m( x) p( x | ) ( )d
这就是贝叶斯公式的密度函数形式。 这个在样本 x 给定 下, 的条件分布被称为 的后验分布。它是集中了总 体、 样本和先验等三种信息中有关 的一切信息, 而又 排除一切与 无关的信息之后所得到的结果。
( )( ) , 确定的随机变量 X 的分布称为贝塔分 ( )
布,记为 beta( , ) 贝塔分布 beta( , ) 的均值 E ( X ) ,
方差 Var ( X ) ( )2 ( 1)
生物化学的创始人费歇尔

生物化学的创始人费歇尔年级文件hxs0107.doc标题生物化学的创始人费歇尔关键词生物化学/蛋白质/化学史内容19世纪下半叶和20世纪之初,在有机化学领域中,德国的费歇尔是最知名的学者之一。
他发觉了苯肼,对糖类、嘌呤类有机化合物的研究取得了突出的成就,因而荣获1902年的诺贝尔化学奖。
他是第二个荣获此项荣誉的化学家,可见科学界对他的推崇。
关于大多数诺贝尔奖获得者来说。
获奖的成果能够说是他一生中在科学上最要紧的奉献。
然而对费歇尔来说,他在科学征途上更令人仰慕的成就,却是在他获得诺贝尔奖之后完成的。
他对科学进展的奉献,归纳起来,要紧有以下四个方面,一、对糖类的研究;二、对嘌呤类化合物的研究:三、对蛋白质;要紧是氨基酸、多肽的研究;四、在化工生产和化学教育上的奉献。
由此可见,他的研究领域集中在对有机化学中那些与人类生活、生命有紧密关系的有机物质的探究。
能够说他是生物化学的创始人。
献身科学的一生经历艾米尔·费歇尔于1852年10月9日主于德国科隆市邻近的奥伊尔斯金亨镇。
两个哥哥早亡,余下的是五个姐姐,因此他既是幼子又是独子,在家里受到大伙儿的喜爱,他父亲劳伦斯。
费歇尔是个富有商人,除经营葡萄酒、啤酒外,依旧一些啤酒厂、毛纺厂、钢管厂、玻璃厂及矿山企业的董事。
在艾米尔青年时代,他父亲正倾注全力进展他的毛纺厂,亲自动手建立了一个小染坊,把买来的染料反复调和进行试验。
由于缺乏化学知识,实验总不象作买卖那么顺心,为此他常唠叨:“假如家里有一个化学家,这些困难便好解决了。
”后来相继建立的钢铁厂、水泥厂也迫切需要化学知识,致使他父亲对化学这门科学更加崇拜。
父亲的这一思想给费歇尔留下了深刻印象,他暗暗下定决心,今后一定要作一个化学家。
1869年艾米尔以第一名的成绩从中学毕业,他没有不记得父亲过去的叮嘱:“要把自己的一生献给科学,你就应该选择化学。
”毅然决定投考大学化学系。
当他将这一决定付诸于行动时,他父亲却犹疑了,那么大的家产和企业由谁来继承?只有艾米尔。
- 1、下载文档前请自行甄别文档内容的完整性,平台不提供额外的编辑、内容补充、找答案等附加服务。
- 2、"仅部分预览"的文档,不可在线预览部分如存在完整性等问题,可反馈申请退款(可完整预览的文档不适用该条件!)。
- 3、如文档侵犯您的权益,请联系客服反馈,我们会尽快为您处理(人工客服工作时间:9:00-18:30)。
Neyman-Fisher, Theorem
Better known as “Neyman-Fisher Factorization Criterion”, it provides a relatively simple procedure either to obtain sufficient statistics or check if a specific statistic could be sufficient.
Fisher was the first who established the Factorization Criterion like a sufficient condition for sufficient statistics in 1922. Years later, Neyman demonstrated it necessity under certain restrictive conditions in 1935. Finally, Halmos and Savage extended it in 1949 as follows:
Let ℘={Pθ,θ∈Ω} be a family of probability measures on a measurable
space (ΘX,A) absolutely continuous with respect to a σ-finite measure µ.
Let suppose that its probability densities in the Radon-Nicodym sense
pθ=dPθ/dµ exist a.s.[µ] (almost sure for µ).
A necessary and sufficient condition for the sufficiency with respect to
℘ of a statistic T transforming the probability space (ΘX,A,Pθ) into
(ΘT,B,P T) is the existence ∀θ∈Ω of a T-1(B)-measurable function gθT(x)
and an A-measurable function h(x)≠0 a.s.[Pθ], both defined ∀x∈ΘX, non-
negatives and µ-integrable, such that pθ(x)= gθT(x)·h(x), a.s.[µ].
Densities pθcan be either probability density functions from absolutely continuous random variables or probability functions from discrete random variables among other possibilities, depending on the nature and definition of µ.
In common economic practice, this Factorization Criterion adopts simpler appearances. Thereby, in Estimation Theory under random sampling, the criterion is usually enounced as follows:
Let X be a r a ndom variable belonging to a regular family of distributions
F(x;θ) which depends on a parameter θ (mixture of absolutely
continuous and discrete random variables on values not depending on the
parameter) representing some characteristic of certain population.
Moreover, let x=(X1, X2, …, X n) represent a random sample size n of X,
extracted from such a population.
A necessary and sufficient condition for the sufficiency of a statistic
T=t(x) with respect to the family of distributions F(x;θ) is that the sample
likelihood function L n(x;θ) could be factorized like L n(x;θ)=g(T;θ)·h(x).
Here, “g” and “h” are non-negative real functions, “g” depending on
sample observations through the statistic exclusively and “h” not
depending on the parameter.
When the random variable is absolutely continuous (discrete), function g(t;θ) is closely related to the probability density function (probability function) of the statistic T. Thus, the criterion could be equivalently enounced assuming the function g(t;θ) to be exactly such probability density function (probability function). In this case, the usually complex work of deducing the distribution x|T of the original observations conditioned to the statistic T becomes easier, being specified by h(x).
According to this Factorization Criterion, any invertible function of a sufficient statistic T*=k(T) is a sufficient statistic too.
Likewise, exponential family defined by probability density functions like f(x;θ)=k(x)·p(θ)·exp[c(θ)’T(x)] always admits a sufficient statistic T.
References:
Fisher,R.A. (1922) “On the mathematical foundation of theoretical statistics” Philos. Trans. Roy. Soc. serie A., 222:309-368.
Neyman,J. (1935) “Sur un teorema concernente le cosidette statistiche sufficienti” Giorn.Ist. Ital. Att., 6:320-334.
Halmos,P.R. and Savage,L.J. (1949) “Application of the Radon-Nikodym theorem to the theory of sufficient statistics” Ann. Math. Statist., 20:225-241.
Zacks,S. (1971) The Theory of Statistical Inference. John Wiley & Sons, Inc. 38-48.。