托福阅读素材:人脸识别
ETS为托福防作弊出台新政人脸识别朗读保密协议

ETS为托福防作弊出台新政人脸识别朗读保密协议去年6月,中国大陆地区的托福考场已开始启用人脸识别系统。
除了拍照之外,系统还会对考生面部进行识别,判断考生和身份证照片里的人,是不是同一个人。
和小编一起来看看ETS为托福防作弊出台新政人脸识别朗读保密协议。
近几年不断出现因考试作弊而爆出的诚信问题,另一方面是不断增长的留学被劝退现象,暴露了一部分留学生在海外求学这件事上缺乏足够的认知。
在美国,最常见被认为是学术不诚信的行为包括:考试作弊、作业抄袭、代写代考、申请材料造假、引用不规范、篡改成绩等。
这些行为轻则被学校处罚,重则被开除、导致身份失效,甚至会面临牢狱之灾。
值得注意的是,中国留学生的学术诚信问题已经引起了部分外国教授的警觉。
阅读、听力以多卷形式考查自2017年3月4日以来,托福阅读、听力部分出现了前所未有的新趋势。
3月之后,托福阅读、听力均以多卷形式出现。
一场考试中,阅读篇目、听力篇目的总数都在20篇左右。
试卷由计算机随机分配,每位考生遇到的题目都不完全一致。
但多套试卷的难度基本持平,考试公平性完全可以得到保证。
这项改革主要是为了打击那些提前贩卖考题的不法分子、严惩在考试时利用高科技手段作弊的考生,并增加机经的预测难度。
因为每个人抽到的阅读、听力试题都不一样,所以即使买到了所谓的“正确答案”,考试时也遇不到一模一样的题;而想要在考试过程中作弊,更是天方夜谭,因为大家做的根本不是同一套卷子。
经典加试几乎退出历史舞台2017年以来,托福阅读、听力加试频频出现,而加试内容几乎清一色全是非经典加试,以往那些在网上轻轻松松就能搜索到的经典加试考题,似乎已经退出了历史的舞台。
同样,这也是ETS为了保证考试公平而放出的大招。
在之前,加试成绩虽然不计入总分,但是如果大家提前背过经典加试答案,那在考试时刷刷刷,在2分钟内就能把加试做完,多出的18分钟的时间就可以重点做其他3篇阅读了。
而对于没背过加试答案的考生来说,这其实是不公平的。
facial recognition阅读理解

facial recognition阅读理解一、简介Facial Recognition是一种基于人工智能的技术,能够识别和分析图像中的面孔,从而对目标对象进行身份验证和定位。
在本文中,我们将讨论这项技术的基本概念、应用场景、优缺点以及相关法律法规。
二、基本原理Facial Recognition的核心原理是基于图像处理和机器学习算法。
它首先对输入的图像进行预处理,如光线调整、面部对齐等,以改善识别精度。
然后,使用机器学习算法训练一个模型,该模型能够从图像中提取面部的特征,并与数据库中的已知面孔进行比对。
如果匹配成功,则识别成功。
三、应用场景1. 安全监控:在公共场所,如机场、车站、大型活动现场等,使用Facial Recognition技术可以进行人员身份验证和流量控制,提高安全保障。
2. 人脸识别门禁:在住宅小区、办公大楼等场所,Facial Recognition门禁系统可以方便快捷地验证访客身份,提高门禁管理效率。
3. 社交媒体应用:许多社交媒体应用使用Facial Recognition技术来识别用户上传的照片中的人物,并提供相关标签和推荐。
4. 执法机构:在刑事调查和人口统计中,Facial Recognition也发挥着重要作用。
四、优缺点优点:1. 准确度高:随着技术的进步,Facial Recognition的准确度越来越高。
2. 快速高效:相对于传统的人工比对方法,Facial Recognition可以大大提高识别的速度和效率。
3. 非接触式:Facial Recognition系统通常是非接触式的,对用户来说更加方便和舒适。
缺点:1. 隐私问题:Facial Recognition涉及个人隐私,需要遵守相关的法律法规。
2. 技术局限性:Facial Recognition技术在一些特殊情况下(如化妆、发型改变、面部遮挡等)可能无法正常工作。
3. 安全风险:如果系统被黑客攻击或滥用来识别无辜公民,可能会引发安全风险。
关于人脸识别的英语阅读理解
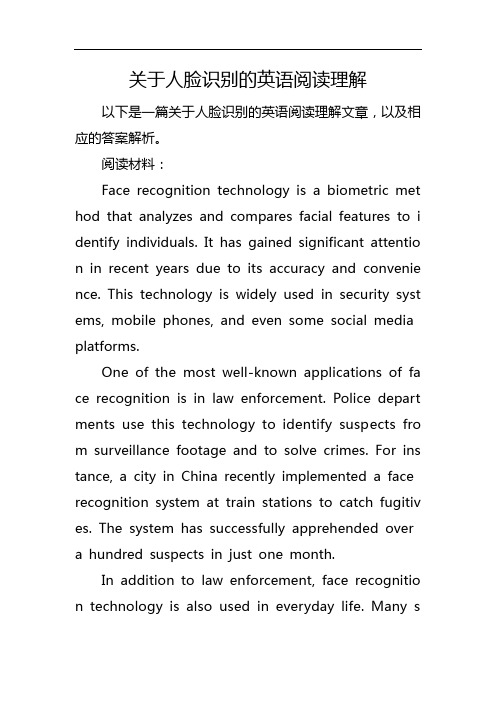
关于人脸识别的英语阅读理解以下是一篇关于人脸识别的英语阅读理解文章,以及相应的答案解析。
阅读材料:Face recognition technology is a biometric met hod that analyzes and compares facial features to i dentify individuals. It has gained significant attentio n in recent years due to its accuracy and convenie nce. This technology is widely used in security syst ems, mobile phones, and even some social media platforms.One of the most well-known applications of fa ce recognition is in law enforcement. Police depart ments use this technology to identify suspects fro m surveillance footage and to solve crimes. For ins tance, a city in China recently implemented a face recognition system at train stations to catch fugitiv es. The system has successfully apprehended over a hundred suspects in just one month.In addition to law enforcement, face recognitio n technology is also used in everyday life. Many smartphones now come with facial recognition soft ware, allowing users to unlock their phones simply by looking at them. This feature adds an extra lay er of security to the device.However, face recognition technology is not wi thout its challenges. Privacy concerns have been ra ised, as people worry about their personal informa tion being stored and used without their consent. There are also concerns about the accuracy of the technology, as it can sometimes mistake one perso n for another.Despite these challenges, face recognition tech nology continues to improve and expand its applic ations. It is now being used in airports, hotels, and even some retail stores. As the technology becom es more advanced, it is likely to play an even grea ter role in our lives.问题与答案解析:1. What is face recognition technology?Answer: Face recognition technology is a biom etric method that analyzes and compares facial fea tures to identify individuals.2. How is face recognition technology used in law enforcement?Answer: Police departments use face recognitio n technology to identify suspects from surveillance footage and to solve crimes.3. What are some everyday applications of face recognition technology?Answer: Everyday applications of face recogniti on technology include using it to unlock smartpho nes and improve security.4. What are some concerns about face recogni tion technology?Answer: Privacy concerns and accuracy issues a re two main concerns about face recognition techn ology.5. Despite the challenges, what is the future of face recognition technology likely to be?Answer: The future of face recognition technol ogy is expected to see continued improvement an d expansion of its applications.通过阅读这篇文章,读者可以了解到人脸识别技术的定义、应用领域、面临的挑战以及未来的发展趋势。
托福阅读练习:人脸识别Facial Recognition
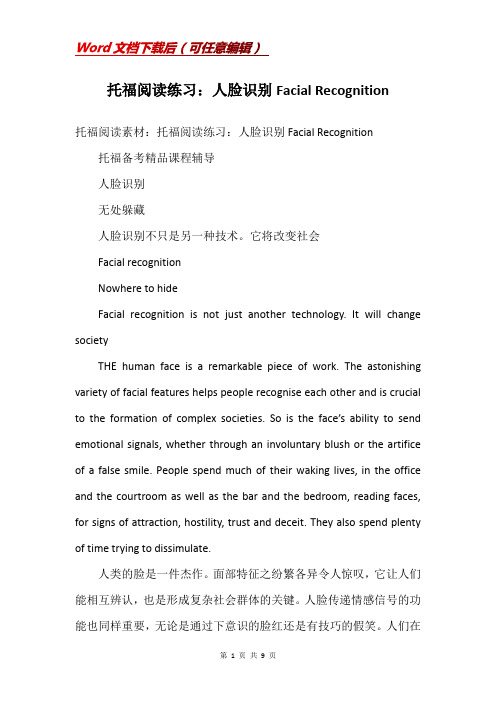
托福阅读练习:人脸识别Facial Recognition托福阅读素材:托福阅读练习:人脸识别Facial Recognition 托福备考精品课程辅导人脸识别无处躲藏人脸识别不只是另一种技术。
它将改变社会Facial recognitionNowhere to hideFacial recognition is not just another technology. It will change societyTHE human face is a remarkable piece of work. The astonishing variety of facial features helps people recognise each other and is crucial to the formation of complex societies. So is the face’s ability to send emotional signals, whether through an involuntary blush or the artifice of a false smile. People spend much of their waking lives, in the office and the courtroom as well as the bar and the bedroom, reading faces, for signs of attraction, hostility, trust and deceit. They also spend plenty of time trying to dissimulate.人类的脸是一件杰作。
面部特征之纷繁各异令人惊叹,它让人们能相互辨认,也是形成复杂社会群体的关键。
人脸传递情感信号的功能也同样重要,无论是通过下意识的脸红还是有技巧的假笑。
“对待人脸识别技术的态度”非连续性文本阅读训练及答案
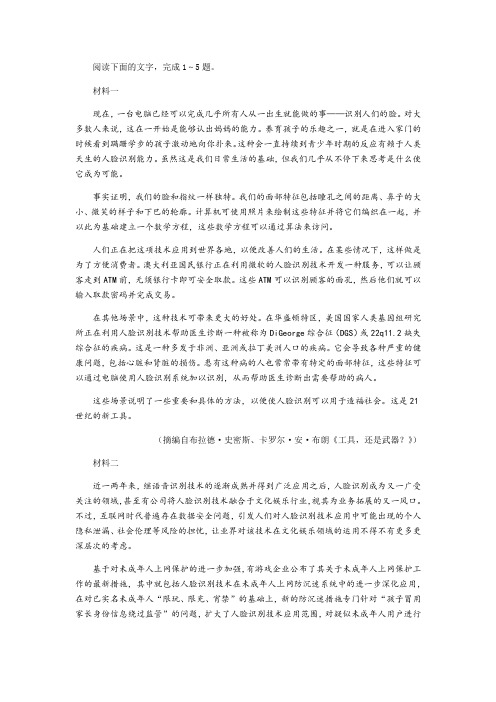
阅读下面的文字,完成1~5题。
材料一现在,一台电脑已经可以完成几乎所有人从一出生就能做的事——识别人们的脸。
对大多数人来说,这在一开始是能够认出妈妈的能力。
养育孩子的乐趣之一,就是在进入家门的时候看到蹒跚学步的孩子激动地向你扑来。
这种会一直持续到青少年时期的反应有赖于人类天生的人脸识别能力。
虽然这是我们日常生活的基础,但我们几乎从不停下来思考是什么使它成为可能。
事实证明,我们的脸和指纹一样独特。
我们的面部特征包括瞳孔之间的距离、鼻子的大小、微笑的样子和下巴的轮廓。
计算机可使用照片来绘制这些特征并将它们编织在一起,并以此为基础建立一个数学方程,这些数学方程可以通过算法来访问。
人们正在把这项技术应用到世界各地,以便改善人们的生活。
在某些情况下,这样做是为了方便消费者。
澳大利亚国民银行正在利用微软的人脸识别技术开发一种服务,可以让顾客走到ATM前,无须银行卡即可安全取款。
这些ATM可以识别顾客的面孔,然后他们就可以输入取款密码并完成交易。
在其他场景中,这种技术可带来更大的好处。
在华盛顿特区,美国国家人类基因组研究所正在利用人脸识别技术帮助医生诊断一种被称为DiGeorge综合征(DGS)或22q11.2缺失综合征的疾病。
这是一种多发于非洲、亚洲或拉丁美洲人口的疾病。
它会导致各种严重的健康问题,包括心脏和肾脏的损伤。
患有这种病的人也常常带有特定的面部特征,这些特征可以通过电脑使用人脸识别系统加以识别,从而帮助医生诊断出需要帮助的病人。
这些场景说明了一些重要和具体的方法,以便使人脸识别可以用于造福社会。
这是21世纪的新工具。
(摘编自布拉德·史密斯、卡罗尔·安·布朗《工具,还是武器?》)材料二近一两年来,继语音识别技术的逐渐成熟并得到广泛应用之后,人脸识别成为又一广受关注的领域,甚至有公司将人脸识别技术融合于文化娱乐行业,视其为业务拓展的又一风口。
不过,互联网时代普遍存在数据安全问题,引发人们对人脸识别技术应用中可能出现的个人隐私泄漏、社会伦理等风险的担忧,让业界对该技术在文化娱乐领域的运用不得不有更多更深层次的考虑。
《识别人脸的技术》阅读答案
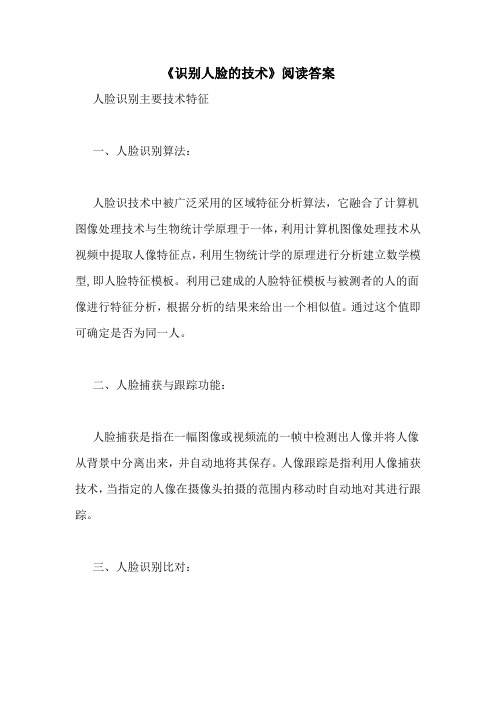
《识别人脸的技术》阅读答案人脸识别主要技术特征一、人脸识别算法:人脸识技术中被广泛采用的区域特征分析算法,它融合了计算机图像处理技术与生物统计学原理于一体,利用计算机图像处理技术从视频中提取人像特征点,利用生物统计学的原理进行分析建立数学模型,即人脸特征模板。
利用已建成的人脸特征模板与被测者的人的面像进行特征分析,根据分析的结果来给出一个相似值。
通过这个值即可确定是否为同一人。
二、人脸捕获与跟踪功能:人脸捕获是指在一幅图像或视频流的一帧中检测出人像并将人像从背景中分离出来,并自动地将其保存。
人像跟踪是指利用人像捕获技术,当指定的人像在摄像头拍摄的范围内移动时自动地对其进行跟踪。
三、人脸识别比对:人脸识别分核实式和搜索式二种比对模式。
核实式是对指将捕获得到的人像或是指定的人像与数据库中已登记的某一对像作比对核实确定其是否为同一人。
搜索式的比对是指,从数据库中已登记的所有人像中搜索查找是否有指定的人像存在。
四、人脸的建模与检索:可以将登记入库的人像数据进行建模提取人脸的特征,并将其生成人脸模板(人脸特征文件)保存到数据库中。
在进行人脸搜索时(搜索式),将指定的人像进行建模,再将其与数据库中的所有人的模板相比对识别,最终将根据所比对的相似值列出最相似的人员列表。
五、真人鉴别功能:系统可以识别得出摄像头前的人是一个真正的人还是一幅照片。
以此杜绝使用者用照片作假。
此项技术需要使用者作脸部表情的配合动作。
六、图像质量检测:图像质量的好坏直接影响到识别的效果,图像质量的检测功能能对即将进行比对的照片进行图像质量评估,并给出相应的建议值来辅助识别。
时代光华《E-Learning技术》课后答案课程学习????课程评估课后测试课后测试如果您对课程内容还没有完全掌握,可以点击这里再次观看。
观看课程测试成绩:100.0分。
恭喜您顺利通过!单选题1. E-Learning应用平台的核心是:√ABCD知识管理系统学习社区考试系统学习管理系统正确答案: D2. 下列学习管理系统中,属于非正式学习的是:√ABCD课程直播系统知识库系统在线学习系统考试系统正确答案: B3. 下列选项中,不属于学习技术体系基础设施的是:√ AB软件硬件C E-Learning应用平台D网络正确答案: C4. 在线考试系统题库的公共标准是:√A QTI标准B SCORM标准C ISO标准D GB/T标准正确: A多选题5. 学习管理系统中常见的角色包括:√ A学习者 BHR经理 C培训管理员 D课程管理员正确答案: A B C D6. 下列选项中,属于在线学习社区的辅助应用的是:A微博/博客 B辅助知识库建设 C辅助培训项目 D辅助业务应用正确答案: A B C D7. 关于虚拟教室系统,下列表述正确的是:√ A虚拟教室系统又称同步教学系统 B能够通过网络提供远程师生同步授课√C以视频传输为主D 能够满足语音、视频、PPT讲义、文字等的同步交流正确答案: A B D判断题8.人力资源管理方向是学习管理的最佳状态。
《人脸识别,且行且改善》阅读练习及答案

人脸识别,且行且改善前不久,北京师范大学全部宿舍楼安装了人脸识别门禁系统,无论何人进门,都得刷脸才能放行;杭州一家餐厅也推出了刷脸支付,整个过程不超过10秒……人脸识别正在走进人们的生活。
人脸识别广受欢迎并得到推广应用,是因其优势巨大。
人脸识别具有生物自然属性和简易性。
当然,具有生物自然属性的识别还有语音识别和体形识别,但不如直接看脸识别简便。
人脸识别的简易性还体现在非接触性和非强制性,可以获取被识别的人脸图像信息而不被个体察觉。
此外,人脸识别效率更高,在实际应用场景中可以同时进行多个人脸的分拣、判断和识别。
人脸识别可以应用于安全监控。
随着“平安城市建设”的推进,越来越多的高清摄像头部署在各种公共场所,如机场、地铁、火车站、汽车站,这些摄像头中就可以安装人脸识别软件,可以自动侦测视频画面中的人脸,并与数据库中的人脸数据进行一一比对,从而发现和追捕犯罪潜逃者。
除此之外,人脸识别还可以应用到从门禁、设备登录到个体识别等广泛领域。
对于个体身份辨认,人脸识别可以用于验证身份证、驾照、护照、签证、选票等;可以用于控制设备存取、车辆访问、智能ATM、电脑接入、程序接入、网络接入等;也能用于智能卡用户验证、人脸数据库人脸检索、人脸标记、人脸分类、多媒体管理人脸搜索、人脸视频分割和拼接,以及人机交互式游戏、主动计算等。
尽管如此,人脸识别也有盲区和弱点。
小米8手机就是采用了人脸识别解锁技术。
但是,有人经过试验后发现,骗过小米8手机的人脸识别易如反掌:只要有主人的红外照片,而且照片不反光,就可以解锁手机。
而且,把手机主人的普通彩色照片用黑白打印机打印出来,用铅笔把相片上的眼睛涂黑,脸上的阴影涂重,就可以解锁手机。
人脸识别系统的漏洞还不止这些。
如同世界上没有两片完全相同的绿叶一样,世界上也不可能有两张完全相似的人脸,即便同卵孪生子的脸也不可能完全相同,人脸的外形很不稳定,人可以通过脸部的变化产生很多表情,在不同观察角度,人脸的视觉图像也相差很大。
人脸识别英文文献
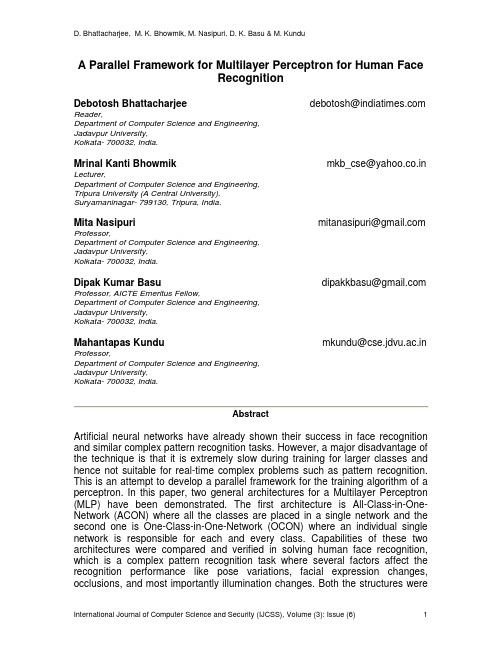
A Parallel Framework for Multilayer Perceptron for Human FaceRecognitionDebotosh Bhattacharjee debotosh@ Reader,Department of Computer Science and Engineering,Jadavpur University,Kolkata- 700032, India.Mrinal Kanti Bhowmik mkb_cse@yahoo.co.in Lecturer,Department of Computer Science and Engineering,Tripura University (A Central University),Suryamaninagar- 799130, Tripura, India.Mita Nasipuri mitanasipuri@ Professor,Department of Computer Science and Engineering,Jadavpur University,Kolkata- 700032, India.Dipak Kumar Basu dipakkbasu@ Professor, AICTE Emeritus Fellow,Department of Computer Science and Engineering,Jadavpur University,Kolkata- 700032, India.Mahantapas Kundu mkundu@cse.jdvu.ac.in Professor,Department of Computer Science and Engineering,Jadavpur University,Kolkata- 700032, India.AbstractArtificial neural networks have already shown their success in face recognition and similar complex pattern recognition tasks. However, a major disadvantage of the technique is that it is extremely slow during training for larger classes and hence not suitable for real-time complex problems such as pattern recognition. This is an attempt to develop a parallel framework for the training algorithm of a perceptron. In this paper, two general architectures for a Multilayer Perceptron (MLP) have been demonstrated. The first architecture is All-Class-in-One-Network (ACON) where all the classes are placed in a single network and the second one is One-Class-in-One-Network (OCON) where an individual single network is responsible for each and every class. Capabilities of these two architectures were compared and verified in solving human face recognition, which is a complex pattern recognition task where several factors affect the recognition performance like pose variations, facial expression changes, occlusions, and most importantly illumination changes. Both the structures wereimplemented and tested for face recognition purpose and experimental results show that the OCON structure performs better than the generally used ACON ones in term of training convergence speed of the network. Unlike the conventional sequential approach of training the neural networks, the OCON technique may be implemented by training all the classes of the face images simultaneously.Keywords:Artificial Neural Network, Network architecture, All-Class-in-One-Network (ACON), One-Class-in-One-Network (OCON), PCA, Multilayer Perceptron, Face recognition.1. INTRODUCTIONNeural networks, with their remarkable ability to derive meaning from complicated or imprecise data, can be used to extract patterns and detect trends that are too complex to be noticed by either humans or other computer techniques. A trained neural network can be thought of as an "expert" in the category of information it has been given to analyze [1]. This proposed work describes the way by which an Artificial Neural Network (ANN) can be designed and implemented over a parallel or distributed environment to reduce its training time. Generally, an ANN goes through three different steps: training of the network, testing of it and final use of it. The final structure of an ANN is generally found out experimentally. This requires huge amount of computation. Moreover, the training time of an ANN is very large, when the classes are linearly non-separable and overlapping in nature. Therefore, to save computation time and in order to achieve good response time the obvious choice is either a high-end machine or a system which is collection of machines with low computational power.In this work, we consider multilayer perceptron (MLP) for human face recognition, which has many real time applications starting from automatic daily attendance checking, allowing the authorized people to enter into highly secured area, in detecting and preventing criminals and so on. For all these cases, response time is very critical. Face recognition has the benefit of being passive, nonintrusive system for verifying personal identity. The techniques used in the best face recognition systems may depend on the application of the system.Human face recognition is a very complex pattern recognition problem, altogether. There is no stability in the input pattern due to different expressions, adornments in the input images. Sometimes, distinguishing features appear similar and produce a very complex situation to take decision. Also, there are several other that make the face recognition task complicated. Some of them are given below.a) Background of the face image can be a complex pattern or almost same as the color of theface.b) Different illumination level, at different parts of the image.c) Direction of illumination may vary.d) Tilting of face.e) Rotation of face with different angle.f) Presence/absence of beard and/or moustacheg) Presence/Absence of spectacle/glasses.h) Change in expressions such as disgust, sadness, happiness, fear, anger, surprise etc.i) Deliberate change in color of the skin and/or hair to disguise the designed system.From above discussion it can now be claimed that the face recognition problem along with face detection, is very complex in nature. To solve it, we require some complex neural network, which takes large amount of time to finalize its structure and also to settle its parameters.In this work, a different architecture has been used to train a multilayer perceptron in faster way. Instead of placing all the classes in a single network, individual networks are used for each of theclasses. Due to lesser number of samples and conflicts in the belongingness of patterns to their respective classes, a later model appears to be faster in comparison to former.2. ARTIFICIAL NEURAL NETWORKArtificial neural networks (ANN) have been developed as generalizations of mathematical models of biological nervous systems. A first wave of interest in neural networks (also known as connectionist models or parallel distributed processing) emerged after the introduction of simplified neurons by McCulloch and Pitts (1943).The basic processing elements of neural networks are called artificial neurons, or simply neurons or nodes. In a simplified mathematical model of the neuron, the effects of the synapses are represented by connection weights that modulate the effect of the associated input signals, and the nonlinear characteristic exhibited by neurons is represented by a transfer function. The neuron impulse is then computed as the weighted sum of the input signals, transformed by the transfer function. The learning capability of an artificial neuron is achieved by adjusting the weights in accordance to the chosen learning algorithm. A neural network has to be configured such that the application of a set of inputs produces the desired set of outputs. Various methods to set the strengths of the connections exist. One way is to set the weights explicitly, using a priori knowledge. Another way is to train the neural network by feeding it teaching patterns and letting it change its weights according to some learning rule. The learning situations in neural networks may be classified into three distinct sorts. These are supervised learning, unsupervised learning, and reinforcement learning. In supervised learning,an input vector is presented at the inputs together with a set of desired responses, one for each node, at the output layer. A forward pass is done, and the errors or discrepancies between the desired and actual response for each node in the output layer are found. These are then used to determine weight changes in the net according to the prevailing learning rule. The term supervised originates from the fact that the desired signals on individual output nodes are provided by an external teacher [3]. Feed-forward networks had already been used successfully for human face recognition. Feed-forward means that there is no feedback to the input. Similar to the way that human beings learn from mistakes, neural networks also could learn from their mistakes by giving feedback to the input patterns. This kind of feedback would be used to reconstruct the input patterns and make them free from error; thus increasing the performance of the neural networks. Of course, it is very complex to construct such types of neural networks. These kinds of networks are called as auto associative neural networks. As the name implies, they use back-propagation algorithms. One of the main problems associated with back-propagation algorithms is local minima. In addition, neural networks have issues associated with learning speed, architecture selection, feature representation, modularity and scaling. Though there are problems and difficulties, the potential advantages of neural networks are vast. Pattern recognition can be done both in normal computers and neural networks. Computers use conventional arithmetic algorithms to detect whether the given pattern matches an existing one. It is a straightforward method. It will say either yes or no. It does not tolerate noisy patterns. On the other hand, neural networks can tolerate noise and, if trained properly, will respond correctly for unknown patterns. Neural networks may not perform miracles, but if constructed with the proper architecture and trained correctly with good data, they will give amazing results, not only in pattern recognition but also in other scientific and commercial applications [4].2A. Network ArchitectureThe computing world has a lot to gain from neural networks. Their ability to learn by example makes them very flexible and powerful. Once a network is trained properly there is no need to devise an algorithm in order to perform a specific task; i.e. no need to understand the internal mechanisms of that task. The architecture of any neural networks generally used is All-Class-in-One-Network (ACON), where all the classes are lumped into one super-network. Hence, the implementation of such ACON structure in parallel environment is not possible. Also, the ACON structure has some disadvantages like the super-network has the burden to simultaneously satisfy all the error constraints by which the number of nodes in the hidden layers tends to be large. The structure of the network is All-Classes-in-One-Network (ACON), shown in Figure 1(a) where one single network is designed to classify all the classes but in One-Class-in-One-Network(OCON), shown in Figure 1(b) a single network is dedicated to recognize one particular class. For each class, a network is created with all the training samples of that class as positive examples, called the class-one, and the negative examples for that class i.e. exemplars from other classes, constitute the class-two. Thus, this classification problem is a two-class partitioning problem. So far, as implementation is concerned, the structure of the network remains the same for all classes and only the weights vary. As the network remains same, weights are kept in separate files and the identification of input image is made on the basis of feature vector and stored weights applied to the network one by one, for all the classes.(a)(b)Figure 1: a) All-Classes-in-One-Network (ACON) b) One-Class-in-One-Network (OCON). Empirical results confirm that the convergence rate of ACON degrades drastically with respect to the network size because the training of hidden units is influenced by (potentially conflicting) signals from different teachers. If the topology is changed to One Class in One Network (OCON) structure, where one sub-network is designated and responsible for one class only then each sub-network specializes in distinguishing its own class from the others. So, the number of hidden units is usually small.2B. Training of an ANNIn the training phase the main goal is to utilize the resources as much as possible and speed-up the computation process. Hence, the computation involved in training is distributed over the system to reduce response time. The training procedure can be given as:(1) Retrieve the topology of the neural network given by the user,(2) Initialize required parameters and weight vector necessary to train the network,(3) Train the network as per network topology and available parameters for all exemplars of different classes,(4) Run the network with test vectors to test the classification ability,(5) If the result found from step 4 is not satisfactory, loop back to step 2 to change the parameters like learning parameter, momentum, number of iteration or even the weight vector,(6) If the testing results do not improve by step 5, then go back to step 1,(7) The best possible (optimal) topology and associated parameters found in step 5 and step 6 are stored.Although we have parallel systems already in use but some problems cannot exploit advantages of these systems because of their inherent sequential execution characteristics. Therefore, it is necessary to find an equivalent algorithm, which is executable in parallel.In case of OCON, different individual small networks with least amount of load, which are responsible for different classes (e.g. k classes), can easily be trained in k different processors and the training time must reduce drastically. To fit into this parallel framework previous training procedure can be modified as follows:(1) Retrieve the topology of the neural network given by the user,(2) Initialize required parameters and weight vector necessary to train the network,(3) Distribute all the classes (say k) to available processors (possibly k) by some optimal process allocation algorithm,(4) Ensure the retrieval the exemplar vectors of respective classes by the corresponding processors,(5) Train the networks as per network topology and available parameters for all exemplars of different classes,(6) Run the networks with test vectors to test the classification ability,(7) If the result found from step 6 is not satisfactory, loop back to step 2 to change the parameters like learning parameter, momentum, number of iteration or even the weight vector,(8) If the testing results do not improve by step 5, then go back to step 1,(9) The best possible (optimal) topology and associated parameters found in step 7 and step 8 are stored,(10) Store weights per class with identification in more than one computer [2].During the training of two different topologies (OCON and ACON), we used total 200 images of 10 different classes and the images are with different poses and also with different illuminations. Sample images used during training are shown Figure 2. We implemented both the topologies using MATLAB. At the time of training of our systems for both the topologies, we set maximum number of possible epochs (or iterations) to 700000. The training stops if the number of iterations exceeds this limit or performance goal is met. Here, performance goal was considered as 10-6. We have taken total 10 different training runs for 10 different classes for OCON and one single training run for ACON for 10 different classes. In case of the OCON networks, performance goal was met for all the 10 different training cases, and also in lesser amount of time than ACON. After the completion of training phase of our two different topologies we tested our both the network using the images of testing class which are not used in training.2C. Testing PhaseDuring testing, the class found in the database with minimum distance does not necessarily stop the testing procedure. Testing is complete after all the registered classes are tested. During testing some points were taken into account, those are:(1) The weights of different classes already available are again distributed in the available computer to test a particular image given as input,(2) The allocation of the tasks to different processors is done based on the testing time and inter-processor communication overhead. The communication overhead should be much less than the testing time for the success of the distribution of testing, and(3) The weight vector of a class matter, not the computer, which has computed it.The testing of a class can be done in any computer as the topology and the weight vector of that class is known. Thus, the system can be fault tolerant [2]. At the time of testing, we used total 200 images. Among 200 images 100 images are taken from the same classes those are used during the training and 100 images from other classes are not used during the training time. In the both topology (ACON and OCON), we have chosen 20 images for testing, in which 10 images from same class those are used during the training as positive exemplars and other 10 images are chosen from other classes of the database as negative exemplars.2D. Performance measurementPerformance of this system can be measured using following parameters:(1) resource sharing: Different terminals remain idle most of the time can be used as a part of this system. Once the weights are finalized anyone in the net, even though not satisfying the optimal testing time criterion, can use it. This can be done through Internet attempting to end the “tyranny of geography”,(2) high reliability: Here we will be concerned with the reliability of the proposed system, not the inherent fault tolerant property of the neural network. Reliability comes from the distribution of computed weights over the system. If any of the computer(or processor) connected to the network goes down then the system works Some applications like security monitoring system, crime prevention system require that the system should work, whatever may be the performance, (3) cost effectiveness: If we use several small personal computers instead of high-end computing machines, we achieve better price/performance ratio,(4) incremental growth: If the number of classes increases, then the complete computation including the additional complexity can be completed without disturbing the existing system. Based on the analysis of performance of our two different topologies, if we see the recognition rates of OCON and ACON in Table 1 and Table 2 OCON is showing better recognition rate than ACON. Comparison in terms of training time can easily be observed in figures 3 (Figure 3 (a) to (k)). In case of OCON, performance goals met for 10 different classes are 9.99999e-007, 1e-006, 9.99999e-007, 9.99998e-007, 1e-006, 9.99998e-007,1e-006, 9.99997e-007, 9.99999e-007 respectively, whereas for ACON it is 0.0100274. Therefore, it is pretty clear that OCON requires less computational time to finalize a network to use.3. PRINCIPAL COMPONENT ANALYSISThe Principal Component Analysis (PCA) [5] [6] [7] uses the entire image to generate a set of features in the both network topology OCON and ACON and does not require the location of individual feature points within the image. We have implemented the PCA transform as a reduced feature extractor in our face recognition system. Here, each of the visual face images is projected into the eigenspace created by the eigenvectors of the covariance matrix of all the training images for both the ACON and OCON networks. Here, we have taken the number of eigenvectors in the eigenspace as 40 because eigenvalues for other eigenvectors are negligible in comparison to the largest eigenvalues.4. EXPERIMENTS RESULTS USING OCON AND ACONThis work has been simulated using MATLAB 7 in a machine of the configuration 2.13GHz Intel Xeon Quad Core Processor and 16 GB of Physical Memory. We have analyzed the performance of our method using YALE B database which is a collection of visual face images with various poses and illumination.4A. YALE Face Database BThis work has been simulated using MATLAB 7 in a machine of the configuration 2.13GHz Intel Xeon Quad Core Processor and 16 GB of Physical Memory. We have analyzed the performance of our method using YALE B database which is a collection of visual face images with various poses and illumination.This database contains 5760 single light source images of 10 subjects each seen under 576 viewing conditions (9 poses x 64 illumination conditions). For every subject in a particular pose, an image with ambient (background) illumination was also captured. Hence, the total number of images is 5850. The total size of the compressed database is about 1GB. The 65 (64 illuminations + 1 ambient) images of a subject in a particular pose have been "tarred" and "gzipped" into a single file. There were 47 (out of 5760) images whose corresponding strobe did not go off. These images basically look like the ambient image of the subject in a particular pose. The images in the database were captured using a purpose-built illumination rig. This rig is fitted with 64 computer controlled strobes. The 64 images of a subject in a particular pose were acquired at camera frame rate (30 frames/second) in about 2 seconds, so there is only small change in head pose and facial expression for those 64 (+1 ambient) images. The image with ambient illumination was captured without a strobe going off. For each subject, images were captured under nine different poses whose relative positions are shown below. Note the pose 0 is the frontal pose. Poses 1, 2, 3, 4, and 5 were about 12 degrees from the camera optical axis (i.e., from Pose 0), while poses 6, 7, and 8 were about 24 degrees. In the Figure 2 sample images of per subject per pose with frontal illumination. Note that the position of a face in an image varies from pose to pose but is fairly constant within the images of a face seen in one of the 9 poses, since the 64 (+1 ambient) images were captured in about 2 seconds. The acquired images are 8-bit (gray scale) captured with a Sony XC-75 camera (with a linear response function) and stored in PGM raw format. The size of each image is 640(w) x 480 (h) [9].In our experiment, we have chosen total 400 images for our experiment purpose. Among them 200 images are taken for training and other 200 images are taken for testing purpose from 10 different classes. In the experiment we use total two different networks: OCON and ACON. All the recognition results of OCON networks are shown in Table 1, and all the recognition results of ACON network are shown in Table 2. During training, total 10 training runs have been executed for 10 different classes. We have completed total 10 different testing for OCON network using 20 images for each experiment. Out of those 20 images, 10 images are taken form the same classes those were used during training, which acts as positive exemplars and rest 10 images are taken from other classes that acts as negative exemplars for that class. In case of OCON, system achieved 100% recognition rate for all the classes. In case of the ACON network, only one network is used for 10 different classes. During the training we achieved 100% as the highest recognition rate, but like OCON network not for all the classes. For ACON network, on an average, 88% recognition rate was achieved.Figure 2: Sample images of YALE B database with different Pose and different illumination.Class Total number oftesting images Number of imagesfrom the trainingclassNumber ofimages fromother classesRecognitionrateClass-1 20 10 10 100% Class-2 20 10 10 100% Class-3 20 10 10 100% Class-4 20 10 10 100% Class-5 20 10 10 100% Class-6 20 10 10 100% Class-7 20 10 10 100% Class-8 20 10 10 100% Class-9 20 10 10 100% Class-10 20 10 10 100%Table 1: Experiments Results for OCON.Class Total number oftesting images Number of imagesfrom the trainingclassNumber ofimages fromother classesRecognitionrateClass - 1 20 10 10 100%Class - 2 20 10 10 100%Class - 3 20 10 10 90%Class - 4 20 10 10 80%Class - 5 20 10 10 80%Class - 6 20 10 10 80%Class - 7 20 10 10 90%Class - 8 20 10 10 100%Class - 9 20 10 10 90%Class-10 20 10 10 70%Table 2: Experiments results for ACON.In the Figure 3, we have shown all the performance measure and reached goal during 10 different training runs in case of OCON network and also one training phase of ACON network.We set highest epochs 700000, but during the training, in case of all the OCON networks, performance goal was met before reaching maximum number of epochs. All the learning rates with required epochs of OCON and ACON networks are shown at column two of Table 3.In case of the OCON network, if we combine all the recognition rates we have the average recognition rate is 100%. But in case of ACON network, 88% is the average recognition rate i.e.we can say that OCON showing better performance, accuracy and speed than ACON. Figure 4 presents a comparative study on ACON and OCON results.Total no. of iterations Learning Rate(lr)Class Figures Network Used 290556 lr > 10-4 Class – 1 Figure 3(a) 248182 lr =10-4 Class – 2 Figure 3(b) 260384 lr =10-5 Class – 3 Figure 3(c) 293279 lr < 10-4 Class - 4 Figure 3(d) 275065 lr =10-4 Class - 5 Figure 3(e) 251642 lr =10-3 Class – 6 Figure 3(f) 273819 lr =10-4 Class – 7 Figure 3(g) 263251 lr < 10-3Class – 8 Figure 3(h) 295986 lr < 10-3 Class – 9 Figure 3(i) 257019 lr > 10-6 Class - 10 Figure 3(j) OCON Highest epochreached(7, 00, 000)Performance goal not met For all Classes (class -1,…,10) Figure 3(k) ACONTable 3: Learning Rate vs. Required Epochs for OCON and ACON.Figure 3 (a) Class – 1 of OCON Network.Figure 3 (b) Class – 2 of OCON Network.Figure 3 (c) Class – 3 of OCON Network.D. Bhattacharjee, M. K. Bhowmik, M. Nasipuri, D. K. Basu & M. KunduFigure 3 (d) Class – 4 of OCON Network.Figure 3 (e) Class – 5 of Ocon Network.International Journal of Computer Science and Security (IJCSS), Volume (3): Issue (6)11D. Bhattacharjee, M. K. Bhowmik, M. Nasipuri, D. K. Basu & M. KunduFigure 3 (f) Class – 6 of OCON Network.Figure 3 (g) Class – 7 of OCON Network.International Journal of Computer Science and Security (IJCSS), Volume (3): Issue (6)12D. Bhattacharjee, M. K. Bhowmik, M. Nasipuri, D. K. Basu & M. KunduFigure 3 (h) Class – 8 of OCON Network.Figure 3 (i) Class – 9 of OCON Network.International Journal of Computer Science and Security (IJCSS), Volume (3): Issue (6)13D. Bhattacharjee, M. K. Bhowmik, M. Nasipuri, D. K. Basu & M. KunduFigure 3 (j) Class – 10 of OCON Network.3 (k) of ACON Network for all the classes.International Journal of Computer Science and Security (IJCSS), Volume (3): Issue (6)14D. Bhattacharjee, M. K. Bhowmik, M. Nasipuri, D. K. Basu & M. KunduFigure 4: Graphical Representation of all Recognition Rate using OCON and ACON Network. The OCON is an obvious choice in terms of speed-up and resource utilization. The OCON structure of neural network makes it most suitable for incremental training, i.e., network upgrading upon adding/removing memberships. One may argue that compared to ACON structure, the OCON structure is slow in retrieving time when the number of classes is very large. This is not true because, as the number of classes increases, the number of hidden neurons in the ACON structure also tends to be very large. Therefore ACON is slow. Since the computation time of both OCON and ACON increases as number of classes grows, a linear increasing of computation time is expected in case of OCON, which might be exponential in case of ACON.5. CONCLUSIONIn this paper, two general architectures for a Multilayer Perceptron (MLP) have been demonstrated. The first architecture is All-Class-in-One-Network (ACON) where all the classes are placed in a single network and the second one is One-Class-in-One-Network (OCON) where an individual single network is responsible for each and every class. Capabilities of these two architectures were compared and verified in solving human face recognition, which is a complex pattern recognition task where several factors affect the recognition performance like pose variations, facial expression changes, occlusions, and most importantly illumination changes. Both the structures were implemented and tested for face recognition purpose and experimental results show that the OCON structure performs better than the generally used ACON ones in term of training convergence speed of the network. Moreover, the inherent non-parallel nature of ACON has compelled us to use OCON for the complex pattern recognition task like human face recognition.ACKNOWLEDGEMENTSecond author is thankful to the project entitled “Development of Techniques for Human Face Based Online Authentication System Phase-I” sponsored by Department of Information Technology under the Ministry of Communications and Information Technology, New Delhi110003, Government of India Vide No. 12(14)/08-ESD, Dated 27/01/2009 at the Department of Computer Science & Engineering, Tripura University-799130, Tripura (West), India for providingInternational Journal of Computer Science and Security (IJCSS), Volume (3): Issue (6)15。
实用类文本阅读人脸识别技术阅读练习及答案
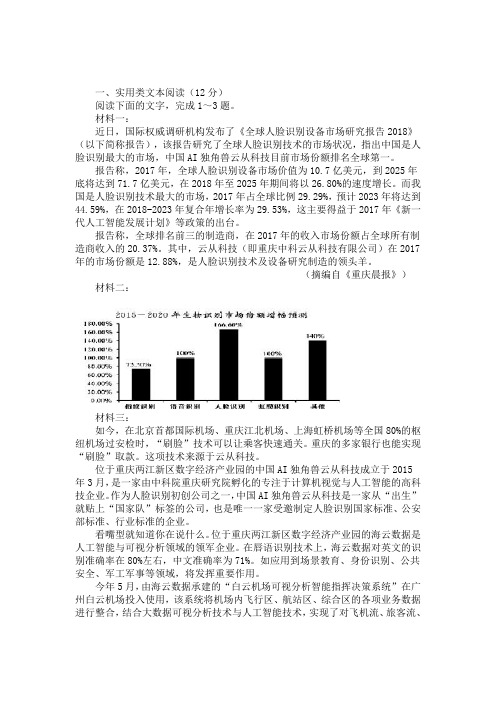
一、实用类文本阅读(12分)阅读下面的文字,完成1~3题。
材料一:近日,国际权威调研机构发布了《全球人脸识别设备市场研究报告2018》(以下简称报告),该报告研究了全球人脸识别技术的市场状况,指出中国是人脸识别最大的市场,中国AI独角兽云从科技目前市场份额排名全球第一。
报告称,2017年,全球人脸识别设备市场价值为10.7亿美元,到2025年底将达到71.7亿美元,在2018年至2025年期间将以26.80%的速度增长。
而我国是人脸识别技术最大的市场,2017年占全球比例29.29%,预计2023年将达到44.59%,在2018-2023年复合年增长率为29.53%,这主要得益于2017年《新一代人工智能发展计划》等政策的出台。
报告称,全球排名前三的制造商,在2017年的收入市场份额占全球所有制造商收入的20.37%。
其中,云从科技(即重庆中科云从科技有限公司)在2017年的市场份额是12.88%,是人脸识别技术及设备研究制造的领头羊。
(摘编自《重庆晨报》)材料二:材料三:如今,在北京首都国际机场、重庆江北机场、上海虹桥机场等全国80%的枢纽机场过安检时,“刷脸”技术可以让乘客快速通关。
重庆的多家银行也能实现“刷脸”取款。
这项技术来源于云从科技。
位于重庆两江新区数字经济产业园的中国AI独角兽云从科技成立于2015年3月,是一家由中科院重庆研究院孵化的专注于计算机视觉与人工智能的高科技企业。
作为人脸识别初创公司之一,中国AI独角兽云从科技是一家从“出生”就贴上“国家队”标签的公司,也是唯一一家受邀制定人脸识别国家标准、公安部标准、行业标准的企业。
看嘴型就知道你在说什么。
位于重庆两江新区数字经济产业园的海云数据是人工智能与可视分析领域的领军企业。
在唇语识别技术上,海云数据对英文的识别准确率在80%左右,中文准确率为71%。
如应用到场景教育、身份识别、公共安全、军工军事等领域,将发挥重要作用。
今年5月,由海云数据承建的“白云机场可视分析智能指挥决策系统”在广州白云机场投入使用,该系统将机场内飞行区、航站区、综合区的各项业务数据进行整合,结合大数据可视分析技术与人工智能技术,实现了对飞机流、旅客流、行李流、货物流、交通流的全区域覆盖、全流程管控,每分钟可处理6万条信息。
《“刷脸时代”来临,您准备好了吗?》说明文阅读附答案
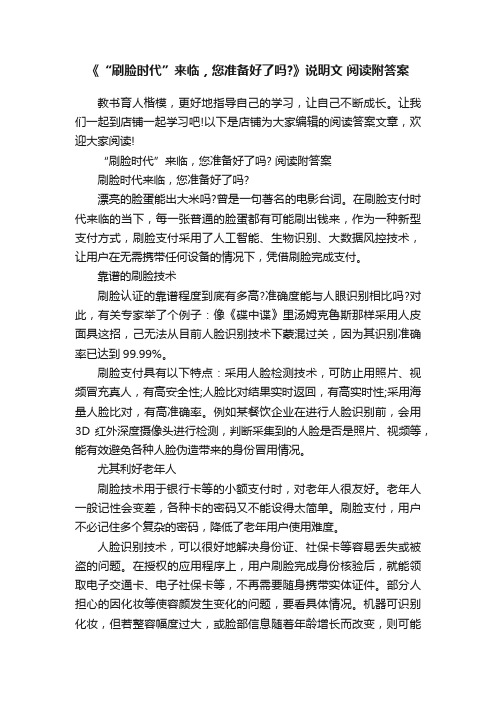
《“刷脸时代”来临,您准备好了吗?》说明文阅读附答案教书育人楷模,更好地指导自己的学习,让自己不断成长。
让我们一起到店铺一起学习吧!以下是店铺为大家编辑的阅读答案文章,欢迎大家阅读!“刷脸时代”来临,您准备好了吗? 阅读附答案刷脸时代来临,您准备好了吗?漂亮的脸蛋能出大米吗?曾是一句著名的电影台词。
在刷脸支付时代来临的当下,每一张普通的脸蛋都有可能刷出钱来,作为一种新型支付方式,刷脸支付采用了人工智能、生物识别、大数据风控技术,让用户在无需携带任何设备的情况下,凭借刷脸完成支付。
靠谱的刷脸技术刷脸认证的靠谱程度到底有多高?准确度能与人眼识别相比吗?对此,有关专家举了个例子:像《碟中谍》里汤姆克鲁斯那样采用人皮面具这招,己无法从目前人脸识别技术下蒙混过关,因为其识别准确率已达到99.99%。
刷脸支付具有以下特点:采用人脸检测技术,可防止用照片、视频冒充真人,有高安全性;人脸比对结果实时返回,有高实时性;采用海量人脸比对,有高准确率。
例如某餐饮企业在进行人脸识别前,会用3D红外深度摄像头进行检测,判断采集到的人脸是否是照片、视频等,能有效避免各种人脸伪造带来的身份冒用情况。
尤其利好老年人刷脸技术用于银行卡等的小额支付时,对老年人很友好。
老年人一般记性会变差,各种卡的密码又不能设得太简单。
刷脸支付,用户不必记住多个复杂的密码,降低了老年用户使用难度。
人脸识别技术,可以很好地解决身份证、社保卡等容易丢失或被盗的问题。
在授权的应用程序上,用户刷脸完成身份核验后,就能领取电子交通卡、电子社保卡等,不再需要随身携带实体证件。
部分人担心的因化妆等使容颜发生变化的问题,要看具体情况。
机器可识别化妆,但若整容幅度过大,或脸部信息随着年龄增长而改变,则可能无法识别。
不过,使用者只需去系统更新脸部照片就可解决。
进入弱隐私时代刷脸支付就好比是一把芝麻开门的钥匙,开启系统进入应用过程中,大量用户的人脸信息被采集并储存。
与之连通的商业机构等均有可能正当地获取用户的个人信息,包括姓名、职业、手机号还有你的脸,甚至你不同的表情等。
gmat阅读关于机器识别表情不准的文章
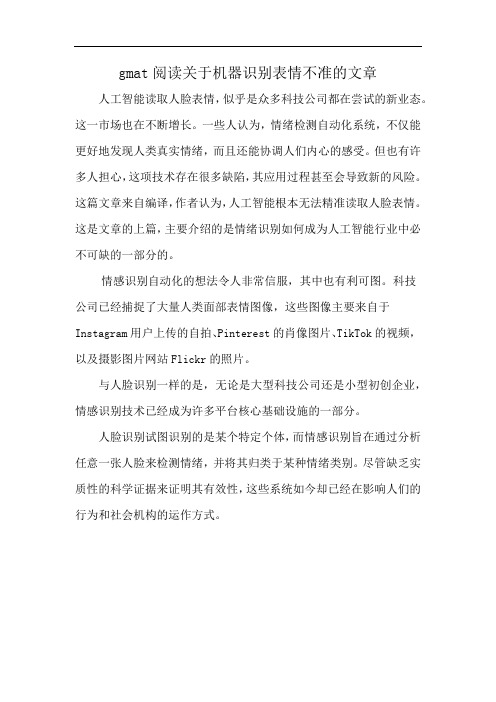
gmat阅读关于机器识别表情不准的文章人工智能读取人脸表情,似乎是众多科技公司都在尝试的新业态。
这一市场也在不断增长。
一些人认为,情绪检测自动化系统,不仅能更好地发现人类真实情绪,而且还能协调人们内心的感受。
但也有许多人担心,这项技术存在很多缺陷,其应用过程甚至会导致新的风险。
这篇文章来自编译,作者认为,人工智能根本无法精准读取人脸表情。
这是文章的上篇,主要介绍的是情绪识别如何成为人工智能行业中必不可缺的一部分的。
情感识别自动化的想法令人非常信服,其中也有利可图。
科技
公司已经捕捉了大量人类面部表情图像,这些图像主要来自于Instagram用户上传的自拍、Pinterest的肖像图片、TikTok的视频,以及摄影图片网站Flickr的照片。
与人脸识别一样的是,无论是大型科技公司还是小型初创企业,情感识别技术已经成为许多平台核心基础设施的一部分。
人脸识别试图识别的是某个特定个体,而情感识别旨在通过分析任意一张人脸来检测情绪,并将其归类于某种情绪类别。
尽管缺乏实质性的科学证据来证明其有效性,这些系统如今却已经在影响人们的行为和社会机构的运作方式。
人脸识别“热”与“冷”思考-2024年高考语文月度热点素材新鲜速递

人脸识别“热”与“冷”思考——最新作文素材、角度、评论、时文 :最新素材:为规范人脸识别技术应用,国家网信办起草了《人脸识别技术应用安全管理规定(试行)(征求意见稿)》,于2023年8月8日向社会公开征求意见。
征求意见稿提出,使用人脸识别技术处理人脸信息应当取得个人的单独同意或者依法取得书面同意。
人脸识别技术当前广泛应用于安防、支付、交通、门禁等领域,极大便利了人们生活,但随着应用场景的不断拓展,被滥用的趋势也愈发显现。
比如有的服务商强制将“刷脸”作为提供服务的前提条件;有的经营者私自对人脸信息进行统计分析,以实现精准营销或防止“飞单”。
更值得警惕的是,因人脸信息等身份信息泄露导致“被贷款”“被诈骗”等情况时有发生。
如不能及时有效对人脸识别技术应用进行规范和限制,那么个人的隐私、财产等权益都可能受到侵犯。
只有让人脸识别技术在法治轨道上规范运行,才能将技术可能带来的风险降至最低。
目前,我国网络安全法、数据安全法、个人信息保护法等法律法规,对人脸识别技术的应用都有相关规定。
国家标准《信息安全技术人脸识别数据安全要求》,对人脸识别应用场景进行了一定约束。
但由于人脸信息具有唯一性,一旦泄露,则无从弥补,因此,严格并细化人脸识别技术的应用规范显得十分必要和迫切。
此次国家网信办制定专门治理规则,明确只有在具有特定的目的和充分的必要性,并采取严格保护措施的情形下,才能使用并且要征得个人同意。
同时,从人脸识别设备安装、图像采集、数据处理等,对人脸识别技术应用进行了全流程规范,不仅有助于提升人脸识别技术的规范应用与合规水平,而且能最大限度降低安全风险水平,切实维护用户权益、社会秩序和公共安全。
角度、立意、评论:1、创新,更要安全,福祉大众技术是把双刃剑。
对人脸识别技术来说,安全性永远是首要前提,必须在尊重用户权益和维护公共利益基础上规范使用。
这需要通过立法引导技术向善,也需要强化执法司法力度,健全行业自律机制,为人脸识别技术应用加固安全锁,让“刷脸”看起来美,用起来放心,真正造福大众。
《人脸识别技术》阅读答案
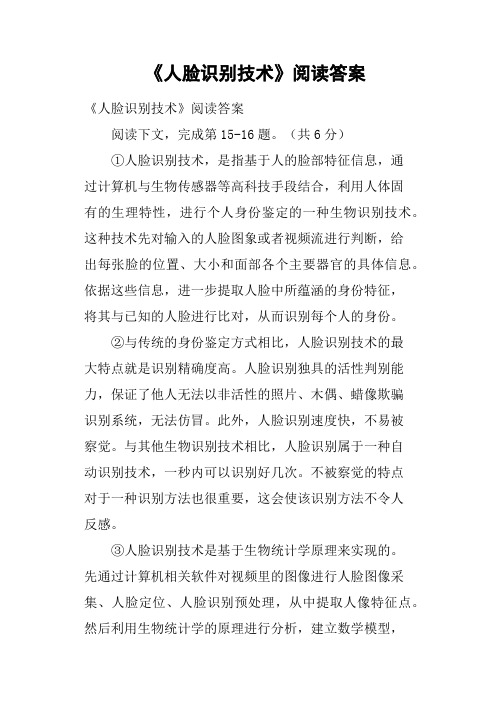
《人脸识别技术》阅读答案《人脸识别技术》阅读答案阅读下文,完成第15-16题。
(共6分)①人脸识别技术,是指基于人的脸部特征信息,通过计算机与生物传感器等高科技手段结合,利用人体固有的生理特性,进行个人身份鉴定的一种生物识别技术。
这种技术先对输入的人脸图象或者视频流进行判断,给出每张脸的位置、大小和面部各个主要器官的具体信息。
依据这些信息,进一步提取人脸中所蕴涵的身份特征,将其与已知的人脸进行比对,从而识别每个人的身份。
②与传统的身份鉴定方式相比,人脸识别技术的最大特点就是识别精确度高。
人脸识别独具的活性判别能力,保证了他人无法以非活性的照片、木偶、蜡像欺骗识别系统,无法仿冒。
此外,人脸识别速度快,不易被察觉。
与其他生物识别技术相比,人脸识别属于一种自动识别技术,一秒内可以识别好几次。
不被察觉的特点对于一种识别方法也很重要,这会使该识别方法不令人反感。
③人脸识别技术是基于生物统计学原理来实现的。
先通过计算机相关软件对视频里的图像进行人脸图像采集、人脸定位、人脸识别预处理,从中提取人像特征点。
然后利用生物统计学的原理进行分析,建立数学模型,即人脸特征模板。
将已建成的人脸特征模板与被测者的面像进行特征比对,根据分析的结果给出一个相似值。
通过这个值即可确定是否为同一人。
现在这一技术已得到广泛应用。
④例如,由于儿童被拐卖事件时有发生,为了保护孩子的安全,有些幼儿园安装了面部识别系统。
这些系统主要采用人脸识别加IC/ID卡(非接触式智能卡)双重认证。
每一位儿童在入学注册登记时必须提供IC/ID卡号、儿童面像、接送者面像等相关资料。
每次入园、出园时都应刷卡并进行家长人脸认证。
如果认证成功,拍照放行;如果认证失败,拍照后报警通知管理员。
不论识别成功与否,系统都会记录下被识别者的详细资料。
有的系统还有短信扩展功能,家长可在手机上看到认证时所拍的照片以及整个接送过程。
这样,有效防止了儿童被拐事件的发生。
⑤目前,人脸识别技术是生物科技领域在可行性、稳定性和准确性等专业技术指标中数值最高的技术,也是各行各业安全保卫工作中运用最广、效果最好的一种技术。
托福写作高频话题思路拓展和实用词汇句式素材整理分享:人工智能
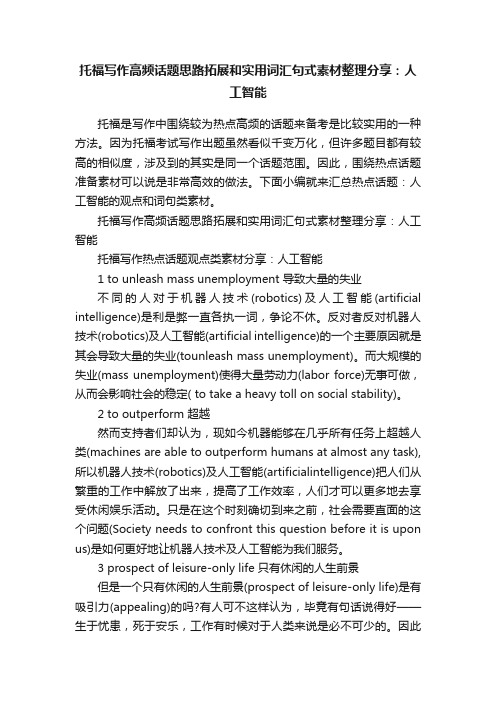
托福写作高频话题思路拓展和实用词汇句式素材整理分享:人工智能托福是写作中围绕较为热点高频的话题来备考是比较实用的一种方法。
因为托福考试写作出题虽然看似千变万化,但许多题目都有较高的相似度,涉及到的其实是同一个话题范围。
因此,围绕热点话题准备素材可以说是非常高效的做法。
下面小编就来汇总热点话题:人工智能的观点和词句类素材。
托福写作高频话题思路拓展和实用词汇句式素材整理分享:人工智能托福写作热点话题观点类素材分享:人工智能1 to unleash mass unemployment 导致大量的失业不同的人对于机器人技术(robotics)及人工智能(artificial intelligence)是利是弊一直各执一词,争论不休。
反对者反对机器人技术(robotics)及人工智能(artificial intelligence)的一个主要原因就是其会导致大量的失业(tounleash mass unemployment)。
而大规模的失业(mass unemployment)使得大量劳动力(labor force)无事可做,从而会影响社会的稳定( to take a heavy toll on social stability)。
2 to outperform 超越然而支持者们却认为,现如今机器能够在几乎所有任务上超越人类(machines are able to outperform humans at almost any task),所以机器人技术(robotics)及人工智能(artificialintelligence)把人们从繁重的工作中解放了出来,提高了工作效率,人们才可以更多地去享受休闲娱乐活动。
只是在这个时刻确切到来之前,社会需要直面的这个问题(Society needs to confront this question before it is upon us)是如何更好地让机器人技术及人工智能为我们服务。
- 1、下载文档前请自行甄别文档内容的完整性,平台不提供额外的编辑、内容补充、找答案等附加服务。
- 2、"仅部分预览"的文档,不可在线预览部分如存在完整性等问题,可反馈申请退款(可完整预览的文档不适用该条件!)。
- 3、如文档侵犯您的权益,请联系客服反馈,我们会尽快为您处理(人工客服工作时间:9:00-18:30)。
托福阅读素材:人脸识别本文从网络收集而来,上传到平台为了帮到更多的人,如果您需要使用本文档,请点击下载按钮下载本文档(有偿下载),另外祝您生活愉快,工作顺利,万事如意!对于托福阅读来说,科技类的文章在托福阅读的题库里面占有一定的比重,那么下面就和出国留学网的小编来看看托福阅读素材:人脸识别。
人脸识别无处躲藏人脸识别不只是另一种技术。
它将改变社会Facial recognitionNowhere to hideFacial recognition is not just another technology. It will change societyTHE human face is a remarkable piece of work. The astonishing variety of facial features helps people recognise each other and is crucial to the formation of complex societies. So is the face’s ability to send emotional signals,whether through an involuntary blush or the artifice of a false smile. People spend much of their waking lives,in the office and the courtroom as well as the bar and the bedroom,reading faces,forsigns of attraction,hostility,trust and deceit. They also spend plenty of time trying to dissimulate.人类的脸是一件杰作。
面部特征之纷繁各异令人惊叹,它让人们能相互辨认,也是形成复杂社会群体的关键。
人脸传递情感信号的功能也同样重要,无论是通过下意识的脸红还是有技巧的假笑。
人们在清醒时花费大量时光研读一张张面孔——在办公室,在法庭,在酒吧,在卧室,寻找着兴趣、敌意、信任和欺骗的迹象。
他们也花大把的时间试图掩饰自己的神色。
Technology is rapidly catching up with the human ability to read faces. In America facial recognition is used by churches to track worshippers’attendance;in Britain,by retailers to spot past shoplifters. This year Welsh police used it to arrest a suspect outside a football game. In China it verifies the identities of ride-hailing drivers,permits tourists to enter attractions and lets people pay for things with a smile. Apple’s new iPhone is expected to use it to unlock the homescreen.科技正迅速赶上人类研读脸孔的能力。
在美国,教堂使用人脸识别来追踪教徒做礼拜的出席情况;在英国,零售商用它来辨认有扒窃前科的顾客。
今年,威尔士警方利用人脸识别在足球场外逮捕了一名嫌疑犯。
在中国,人脸识别被用于验证网约车司机的身份、让游客刷脸进景点、让顾客微微一笑就能刷脸买单。
苹果的新款iPhone预计将用这一技术来解锁屏幕。
Set against human skills,such applications might seem incremental. Some breakthroughs,such as flight or the internet,obviously transform human abilities;facial recognition seems merely to encode them. Although faces are peculiar to individuals,they are also public,so technology does not,at first sight,intrude on something that is private. And yet the ability to record,store and analyse images of faces cheaply,quickly and on a vast scale promises one day to bring about fundamental changes to notions of privacy,fairness and trust.与人类的技能相比,这样的应用看似只是锦上添花。
飞行或互联网这样的重大突破明显改变了人类的能力,而人脸识别似乎只是对面孔进行编码。
尽管人的面孔为个人独有,但也是公开的,因此乍看起来,技术并没有侵犯隐私之嫌。
但是,低成本、快速、大量地记录、存储和分析人脸图像的能力终有一天会使隐私、公平和信任等观念发生根本性的改变。
The final frontierStart with privacy. One big difference between faces and other biometric data,such as fingerprints,is that they work at a distance. Anyone with a phone can take a picture for facial-recognition programs to use. FindFace,an app in Russia,compares snaps of strangers with pictures on VKontakte,a social network,and can identify people with a 70% accuracy rate. Facebook’s bank of facial images cannot be scraped by others,but the Silicon Valley giant could obtain pictures of visitors to a car showroom,say,and later use facial recognition to serve them ads for cars. Even if private firms are unable to join the dots between images and identity,the state often can. China’s government keeps a record of its citizens’faces;photographs of half of America’s adult population are stored in databases that can be used by the FBI. Law-enforcement agencies now have a powerful weapon in their ability to track criminals,but at enormous potential cost to citizens’privacy.终极战线先说隐私。
人脸相比指纹等其他生物特征数据的一个巨大区别就是它们能够远距离起作用。
人们只要有手机就可以拍下照片,供人脸识别程序使用。
俄罗斯的一款应用FindFace抓拍陌生人的照片与社交网络VKontakte上的照片比对,识别人的准确率达70%。
Facebook的面部图片库不能被其他人提取,但是,举个例子,这家硅谷巨头可以获得汽车展厅内到访者的照片,然后使用人脸识别技术在自己的网站上找到这些人,向他们发送汽车广告。
即使私人公司无法将照片和身份联系起来,国家往往可以做到。
中国政府有公民的面部记录;美国半数成年人口的照片储存在数据库中,可供FBI使用。
如今,执法机关在追踪罪犯方面拥有了一个强大的武器,但它可能会令公民隐私遭受巨大的损害。
The face is not just a name-tag. It displays a lot of other information—and machines can read that,too. Again,that promises benefits. Some firms are analysing faces to provide automated diagnoses of rare genetic conditions,such as Hajdu-Cheney syndrome,far earlier than would otherwise be possible. Systems that measure emotion may give autistic people a grasp of social signals they find elusive. But the technology also threatens. Researchers at Stanford University have demonstrated that,when shown pictures of one gayman,and one straight man,the algorithm could attribute their sexuality correctly 81% of the time. Humans managed only 61%. In countries where homosexuality is a crime,software which promises to infer sexuality from a face is an alarming prospect.人脸不仅仅能表明身份,它还显示了许多其他信息,同样能由机器读取。