改进差分进化算法及其在模糊聚类分析中的应用
基于改进的聚类算法的图像分割技术研究
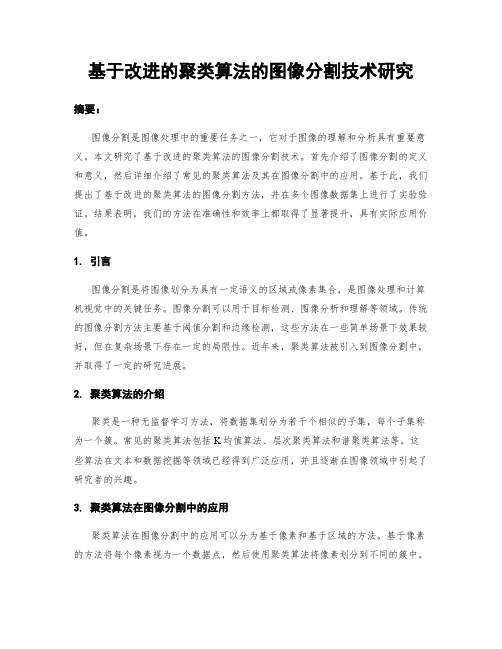
基于改进的聚类算法的图像分割技术研究摘要:图像分割是图像处理中的重要任务之一,它对于图像的理解和分析具有重要意义。
本文研究了基于改进的聚类算法的图像分割技术。
首先介绍了图像分割的定义和意义,然后详细介绍了常见的聚类算法及其在图像分割中的应用。
基于此,我们提出了基于改进的聚类算法的图像分割方法,并在多个图像数据集上进行了实验验证。
结果表明,我们的方法在准确性和效率上都取得了显著提升,具有实际应用价值。
1. 引言图像分割是将图像划分为具有一定语义的区域或像素集合,是图像处理和计算机视觉中的关键任务。
图像分割可以用于目标检测、图像分析和理解等领域。
传统的图像分割方法主要基于阈值分割和边缘检测,这些方法在一些简单场景下效果较好,但在复杂场景下存在一定的局限性。
近年来,聚类算法被引入到图像分割中,并取得了一定的研究进展。
2. 聚类算法的介绍聚类是一种无监督学习方法,将数据集划分为若干个相似的子集,每个子集称为一个簇。
常见的聚类算法包括K均值算法、层次聚类算法和谱聚类算法等。
这些算法在文本和数据挖掘等领域已经得到广泛应用,并且逐渐在图像领域中引起了研究者的兴趣。
3. 聚类算法在图像分割中的应用聚类算法在图像分割中的应用可以分为基于像素和基于区域的方法。
基于像素的方法将每个像素视为一个数据点,然后使用聚类算法将像素划分到不同的簇中。
基于区域的方法首先将图像划分为相似的区域,然后使用聚类算法进一步合并或分割这些区域。
这些方法都在不同程度上提高了图像分割的准确性和效率。
4. 基于改进的聚类算法的图像分割方法为了提高图像分割的准确性和效率,我们提出了一种基于改进的聚类算法的图像分割方法。
首先,我们使用K均值算法初始化聚类中心,并与传统的K均值算法相比,我们通过引入自适应权重和距离约束来提高其准确性。
然后,我们采用一种改进的层次聚类算法,通过考虑区域的相似性和距离约束来减少误差传播。
最后,我们使用谱聚类算法来进一步优化分割结果,以提高图像的连续性和整体性。
改进差分进化算法及其在模糊聚类分析中的应用
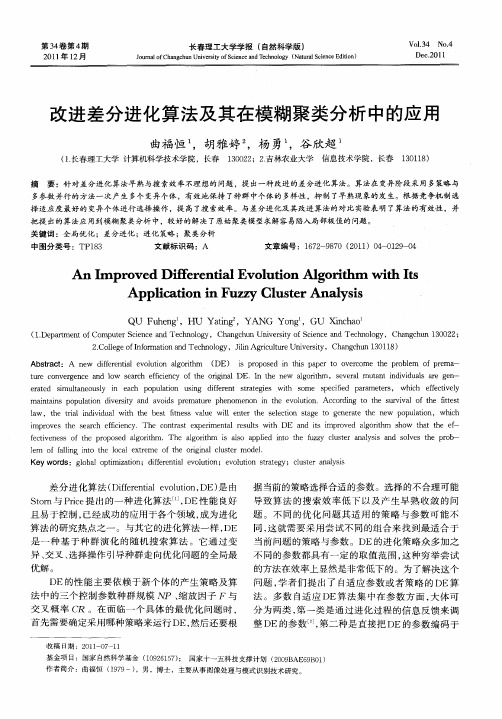
第 3 卷 第4 4 期
2 1 年 1 月 01 2
长春理工大学学报 ( 自然 科 学 版 )
Jun l f h n c u iesyo ce c n eh oo y ( trl ce c dt n o ra C ag h nUnvri fS i ea dT cn lg Naua in eE io ) o t n S i
把提 出的算法应 用到模 糊聚类分析 中,较 好的解决 了原始聚类模型求解容 易陷入局 部极 值的问题 。
关 键 词 :全 局 优 化 ; 差 分进 化 ;进 化 策略 ;聚 类 分 析
中图分类
文章编号 :1 7-9 7 (0 1 4 0 2 - 4 6 2 8 0 2 1 )0 - 19 0
fc i e e s f t e p o o e ag r h .Th ag r h e t n s o h r p s d l o i v t m e l o i m s a s p l d i t t e f z y cu t r a l ss a d s l e h r b t i lo a p i n o h u z ls e nay i n o v s t e p o — e
摘 要 :针对差分进化 算法早 熟与搜 索效 率不理 想的 问题 ,提 出一种改进的差分进化算 法。算法在 变异阶段 采用 多策略 与 多参数 并行的方法一 次产 生 多个变异个体 ,有 效地保持 了种群 中个体 的多样性 ,抑制 了早熟现 象的发 生。根 据竞争机制 选 择适应度 最好 的 变异个体进行 选择 操作 ,提 高 了搜 索效率 。与差分进化及 其改进 算法的对 比实验表 明 了算法的有效性 ,并
差分进化算法及应用研究
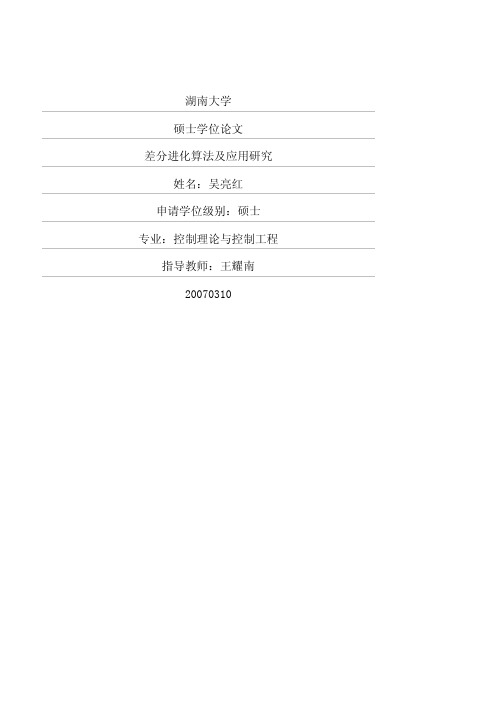
湖南大学硕士学位论文差分进化算法及应用研究姓名:吴亮红申请学位级别:硕士专业:控制理论与控制工程指导教师:王耀南20070310硕士学位论文摘要论文首先介绍了智能优化算法的产生对现代优化技术的重要影响,阐述了智能优化算法的研究和发展对现代优化技术和工程实践应用的必要性,归纳总结了智能优化算法的主要特点,简要介绍了智能优化算法的主要研究内容及应用领域。
对差分进化算法的原理进行了详细的介绍,给出了差分进化算法的伪代码。
针对混合整数非线性规划问题的特点,在差分进化算法的变异操作中加入取整运算,提出了一种适合于求解各种混合整数非线性规划问题的改进差分进化算法。
同时,采用时变交叉概率因子的方法以提高算法的全局搜索能力和收敛速率。
用四个典型测试函数进行了实验研究,实验结果表明,改进的差分进化算法用于求解混合整数非线性规划问题时收敛速度快,精度高,鲁棒性强。
采用非固定多段映射罚函数法处理问题的约束条件,提出了一种用改进差分进化算法求解非线性约束优化问题的新方法。
结合差分进化算法两种不同变异方式的特点,引入模拟退火策略,使算法在搜索的初始阶段有较强的全局搜索能力,而在后阶段有较强的局部搜索能力,以提高算法的全局收敛性和收敛速率。
用几个典型Benchmarks函数进行了测试,实验结果表明,该方法全局搜索能力强,鲁棒性好,精度高,收敛速度快,是一种求解非线性约束优化问题的有效方法。
为保持所求得的多目标优化问题Pareto最优解的多样性,提出了一种精英保留和根据目标函数值进行排序的多目标优化差分进化算法。
对排序策略中目标函数的选择方式进行了分析和比较,并提出了一种确定进化过程中求得的精英解是否进入Pareto最优解集的阈值确定方法。
用多个经典测试函数进行了实验分析,并与NSGA-Ⅱ算法进行了比较。
实验结果表明,本文方法收敛到问题的Pareto前沿效果良好,获得解的散布范围广,能有效保持所求得的Pareto最优解的多样性。
差分进化算法精品PPT课件

引言
开始
根据实际问题进行编码 设置参数
生成初始种群
计算个体适应值
是否满足进 化终止条件
是
算法结束, 输出最优个体
一般演化算法的过程
问题
遗传操作, 生成新种群
否
1、遗传操作象 ✓ 种群中所有个体 ✓ 种群中部分个体 2、遗传操作顺序 ✓ 重叠 ✓ 非重叠 3、新种群重组方式
DE的改进方法
为了提高DE的寻优能力、加快收敛速度、 克服启发式算法常见的早熟收敛现象,许多学 者对DE算法进行改进:
▪ 控制参数的改进。 ▪ 差分策略的改进。 ▪ 选择策略的改进。 ▪ 种群重构 ▪ 混合算法。
DE的改进方法---多种扩展模式
DE算法的多种变形形式常用符号DE /x/y/ z 以 示区分,其中:
开开开开开
基本原理
求解非线性函数f (x 1, x 2, ⋯, x n)的最小值问题, x i满足:
xi t xi,1 t , xi,2 t , , xi,n t
i 1, 2, , M ; t 1, 2, tmax.
令xi 是t 第t代的第i个染色体, 则
xiLj xij xiUj j 1, 2, n
行变异操作;
▪ :一般在[ 0, 2 ]之间选择, 通常取0. 5;
▪ CR:一般在[ 0, 1 ]之间选择, 比较好的选择应在0. 3 左右,
CR 大些收敛速度会加快, 但易发生早熟现象。
差异演化算法的优缺点
和其它进化算法相比, 差异演化具有以下优点:
▪ 差异演化在求解非凸、多峰、非线性函数优化问题表 现极强的稳健性。
差分进化算法及其应用研究
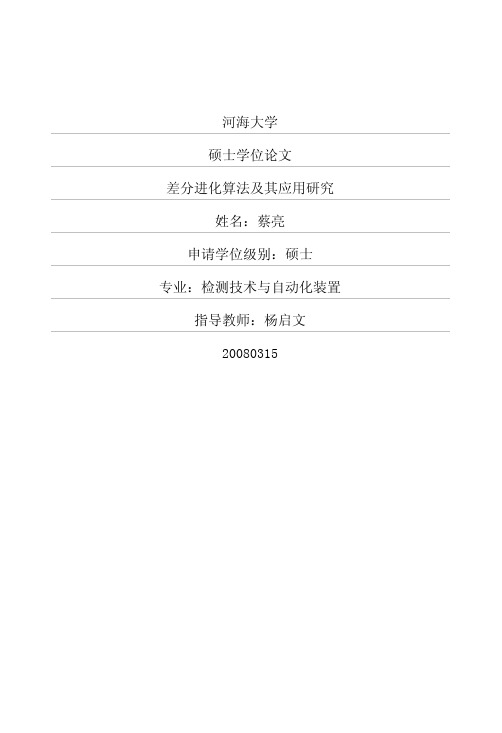
第四,针对建模在应用中带来的不便,以及不确定性的因素造成的被控对象 时变和非线性,基于ITAE控制律,提出了差分进化PID控制器在线进化结构, 实现了PID参数在线整定。该方法不要求事先获取被控对象的精确数学模型,因 而具有一定的工程应用价值。
关键词:进化计算、差分进化、差分策略、早熟收敛、PID整定
河海大学硕士学位论文
Abstract
Differential Evolution(DE)iS a heuristic optimization algorithm based on population divcrsity.Due tO its simple structure,less control parameters and good robustness.DE has received more and more attention by researchers and is widely used in many practices.There are many differential strategies.The typical strategies among them which attracted more attention and got more application btit still owned certain faults are DE/rand/1 and DE/best/1.Premature convergence and loeal searching ability ale still the main issue.So for enhancing the Ix'rformance ofDE,it is
模糊聚类的原理和应用
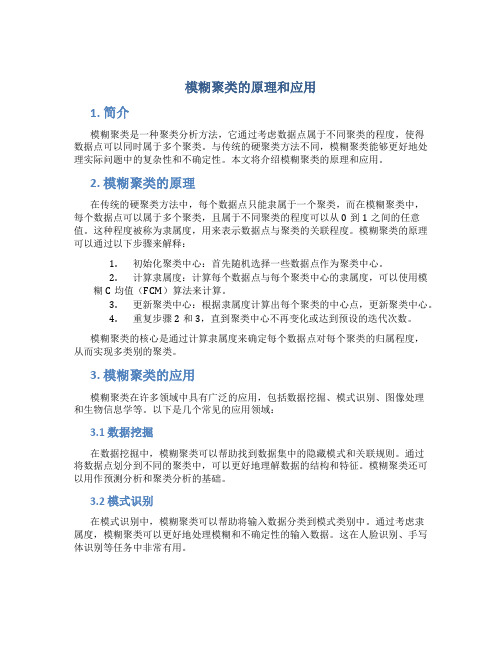
模糊聚类的原理和应用1. 简介模糊聚类是一种聚类分析方法,它通过考虑数据点属于不同聚类的程度,使得数据点可以同时属于多个聚类。
与传统的硬聚类方法不同,模糊聚类能够更好地处理实际问题中的复杂性和不确定性。
本文将介绍模糊聚类的原理和应用。
2. 模糊聚类的原理在传统的硬聚类方法中,每个数据点只能隶属于一个聚类,而在模糊聚类中,每个数据点可以属于多个聚类,且属于不同聚类的程度可以从0到1之间的任意值。
这种程度被称为隶属度,用来表示数据点与聚类的关联程度。
模糊聚类的原理可以通过以下步骤来解释:1.初始化聚类中心:首先随机选择一些数据点作为聚类中心。
2.计算隶属度:计算每个数据点与每个聚类中心的隶属度,可以使用模糊C均值(FCM)算法来计算。
3.更新聚类中心:根据隶属度计算出每个聚类的中心点,更新聚类中心。
4.重复步骤2和3,直到聚类中心不再变化或达到预设的迭代次数。
模糊聚类的核心是通过计算隶属度来确定每个数据点对每个聚类的归属程度,从而实现多类别的聚类。
3. 模糊聚类的应用模糊聚类在许多领域中具有广泛的应用,包括数据挖掘、模式识别、图像处理和生物信息学等。
以下是几个常见的应用领域:3.1 数据挖掘在数据挖掘中,模糊聚类可以帮助找到数据集中的隐藏模式和关联规则。
通过将数据点划分到不同的聚类中,可以更好地理解数据的结构和特征。
模糊聚类还可以用作预测分析和聚类分析的基础。
3.2 模式识别在模式识别中,模糊聚类可以帮助将输入数据分类到模式类别中。
通过考虑隶属度,模糊聚类可以更好地处理模糊和不确定性的输入数据。
这在人脸识别、手写体识别等任务中非常有用。
3.3 图像处理在图像处理中,模糊聚类被广泛应用于图像分割和图像压缩等任务。
通过将图像像素划分到不同的聚类中,可以实现图像的分割和压缩。
模糊聚类还可以用于图像特征提取和图像检索等应用。
3.4 生物信息学在生物信息学中,模糊聚类被用于处理基因表达数据和蛋白质序列数据等。
差分进化算法的实际应用

差分进化算法的实际应用差分进化算法(Differential Evolution,DE)是一种优化算法,最初由Storn和Price 在1995年提出。
该算法通过模拟自然选择的过程,不断优化目标函数,达到最优解。
近年来,差分进化算法在各个领域得到了广泛的应用。
差分进化算法在工程优化领域是被广泛应用的。
例如,在室内设计领域,使用差分进化算法来优化各种室内设计的元素,如家具布置、灯具设计等,优化的结果可以增强空间美感和舒适性,延长家具和设备的使用寿命,降低了设计成本。
在管道系统和化学工业中,差分进化算法也能够用来解决复杂工艺的问题。
例如,在纸浆和纸制品制造中,使用差分进化算法进行预测的生产条件,以便减少生产成本,优化生产过程;另外,差分进化算法亦被应用于工业口味的优化、生产中的卫生质量控制、物流和生产计划的优化等。
2. 差分进化算法在信号与图像处理中的应用差分进化算法在信号和图像处理中得到了广泛的应用。
例如,在图像压缩技术中,差分进化算法被用来找出最佳的图像的变换参数,包括图像的分辨率和压缩比例。
在音频信号中,差分进化算法常常与混响器和均衡器相伴。
通过差分进化算法来优化这些音频效果器的性能参数,提神音频品质,使音频的全局调整更为精准和高效。
差分进化算法在机器嗅觉领域得到了广泛的应用。
例如,食品行业使用差分进化算法来判定食品的成分。
此外,差分进化算法还在汽车领域被应用于车内高温时的臭味测量,以及疲劳驾驶时的呼吸测量。
差分进化算法在金融领域中的应用,主要是预测股票和外汇的市场行情,进行投资决策。
例如,在股票市场中,差分进化算法被用来挖掘股票与其他市场之间的关联性。
此外,差分进化算法还可以通过自动化的方式,完成对阿尔法交易策略等的优化。
结论总体来说,差分进化算法是一种强大而灵活的优化算法,涵盖了诸多领域的应用,在实际应用中所表现的效果也非常出色。
差分进化算法的发展也处于一个不断成熟和完善的过程,随着时间的推移,相信该算法在更多领域中应用的空间也会越来越广阔。
改进遗传算法在污水处理工艺优选决策指标权值确定中的应用
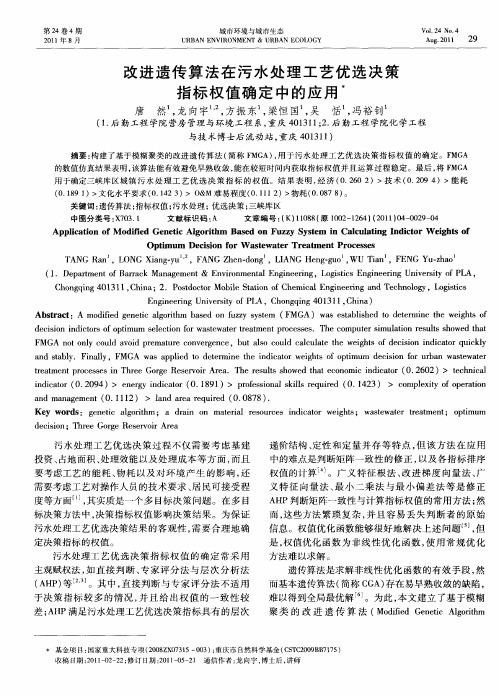
E g er gU iesyo L C o g ig4 , hn ) ni e n nvr t f A, h n qn 0 C ia n i i P 11 31
A s at df dgn t loi m b sd o uz yt ( MG b t c:A moie e e ca rh ae n fzyss m F A)w set l h dt d t mietew i t o r i i g t e a s bi e o ee n h e hs f a s r g
Op i um cso f r W a t wat r Tr a me tPr c s e tm De ii n o se e e t n o e ss TANG n Ra ,LONG a g—u 一,F Xin y ANG e d n ,L ANG n - u , U a ,FENG Zh n— o g I He g g o W Tin Yu—h o za
差 ;H A P满足 污水 处理 工艺 优选决 策 指标 具 有 的层 次
聚 类 的 改 进 遗 传 算 法 ( dfd G nt l rh Moie eec Agi m i i ot
基金项 目: 国家重大科 技专项 (0 8 X 7 1 0 3 ; 20 Z 0 3 5— 0 ) 重庆市 自然科学基金 ( S C 0 9 B 1 5 C T 2 0 B 77 ) 收稿 日期 :0 1 0 — 2 修订 日期 :0 1 0 — 1 通信作者 : 向宇 , 士后 , 2 1- 2 2 ; 2 1— 5 2 龙 博 讲师
方法 难 以求解 。
遗传 算法 是求 解非 线 性 优化 函数 的 有效 手 段 , 然
而基 本 遗传算 法 ( 简称 C A) 在 易早熟 收敛 的缺 陷 , G 存 难 以得 到全局 最优 解 。为 此 , 文 建 立 了基 于模 糊 本
基于改进差分进化的K-均值聚类算法
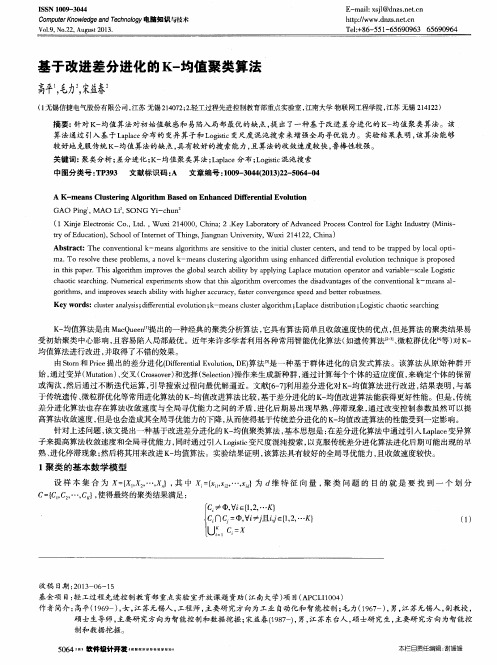
( 1 Xi n j e E l e c t r o n i c C o . , L t d . , Wu x i 2 1 4 0 0 0 , Ch i n a ; 2. Ke y L a b o r a t o r y o f A d v a n c e d P r o c e s s C o n t r o l f 0 r L i g h t I n d u s t y ( r Mi n i s -
I S SN 1 0 0 9 -3 0 4 4
E — m a i l : x s j l @ d n z s . n e t . c a
h t t p : / / ww w. d n z s . n e t . e n T e l : + 8 6 — 5 5 1 — 6 5 6 9 0 9 6 3 6 5 6 9 0 9 6 4
Ab s t r a c t : Th e c o n v e n t i o n a l k -me a n s a l g o i r t h ms a r e s e n s i t i v e t o t h e i n i t i l a c l u s t e r c e n t e r s , a n d t e n d t O b e t r a p p e d b y l o c a l o p t i -
算 法通过 引入基 于L a p l a c e 分布的 变异算子和 L o g i s t i c 变尺度混沌搜 索来增强全局寻优能力。 实验结果表明 , 该算法能够 较好地克服传统 K一 均值算法的缺 点, 具有较好 的搜 索能力 , 且算法的收敛速度较快 , 鲁棒性较 强。
关键词: 聚类分析 ; 差分进 化; K 一 均值聚类算法; L a p l a c e 分布 ; L e . i g s t i c 混沌搜 索 中图分类号 : T P 3 9 3 文献标识码 : A 文章编号 : 1 0 0 9 — 3 0 4 4 ( 2 0 1 3 ) 2 2 — 5 0 6 4 — 0 4
进化算法在图像处理中的应用
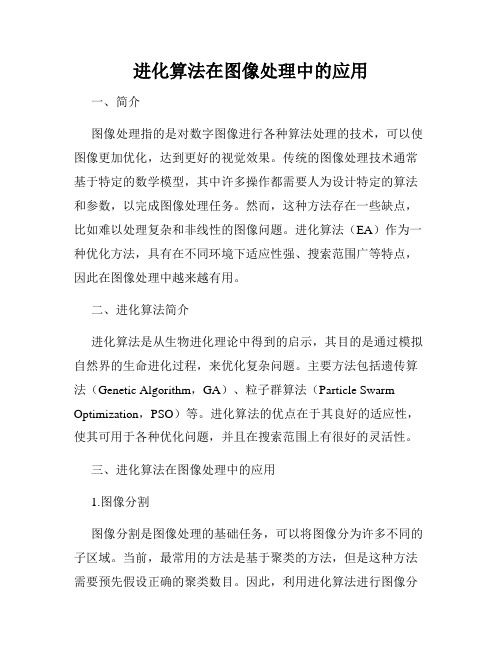
进化算法在图像处理中的应用一、简介图像处理指的是对数字图像进行各种算法处理的技术,可以使图像更加优化,达到更好的视觉效果。
传统的图像处理技术通常基于特定的数学模型,其中许多操作都需要人为设计特定的算法和参数,以完成图像处理任务。
然而,这种方法存在一些缺点,比如难以处理复杂和非线性的图像问题。
进化算法(EA)作为一种优化方法,具有在不同环境下适应性强、搜索范围广等特点,因此在图像处理中越来越有用。
二、进化算法简介进化算法是从生物进化理论中得到的启示,其目的是通过模拟自然界的生命进化过程,来优化复杂问题。
主要方法包括遗传算法(Genetic Algorithm,GA)、粒子群算法(Particle Swarm Optimization,PSO)等。
进化算法的优点在于其良好的适应性,使其可用于各种优化问题,并且在搜索范围上有很好的灵活性。
三、进化算法在图像处理中的应用1.图像分割图像分割是图像处理的基础任务,可以将图像分为许多不同的子区域。
当前,最常用的方法是基于聚类的方法,但是这种方法需要预先假设正确的聚类数目。
因此,利用进化算法进行图像分割是一种很好的方法。
利用进化算法可以自动确定合适的聚类数目、聚类中心和分割阈值,以获得分割结果。
2.图像去噪图像去噪是图像处理中的另一项重要任务,它旨在通过减少随机噪声和伪像来提高图像的质量。
利用进化算法中的PSO算法可以优化噪声模型,使其更准确地反映噪声的统计特性,从而提高去噪效果。
3.图像修复在图像处理中,图像修复是一项非常具有挑战性的任务,包括去除图像的瑕疵、填充缺失的区域、恢复模糊的边界等。
利用进化算法可以自适应地优化目标函数,以提高修复效果。
4.图像增强图像增强是一项使图像更加清晰亮丽的处理任务,进化算法可以应用于图像增强的目标函数设计和参数优化。
例如,利用遗传算法可以改进图像直方图均衡化算法,使其对各种图像更加适用。
四、结论进化算法是一种灵活的优化算法,其在图像处理领域中得到了广泛应用。
差分进化算法原理
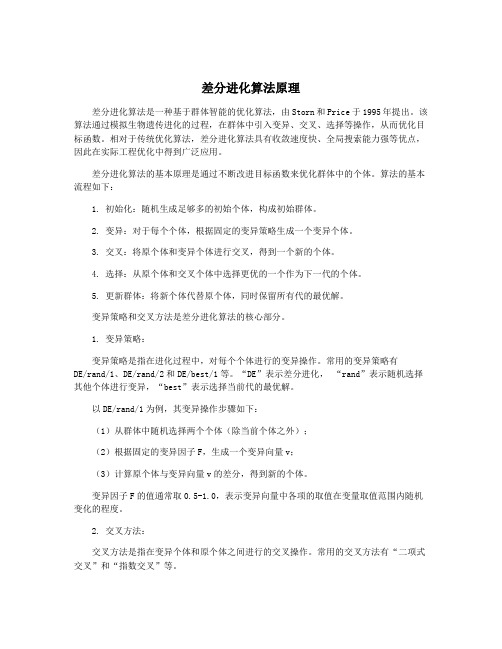
差分进化算法原理差分进化算法是一种基于群体智能的优化算法,由Storn和Price于1995年提出。
该算法通过模拟生物遗传进化的过程,在群体中引入变异、交叉、选择等操作,从而优化目标函数。
相对于传统优化算法,差分进化算法具有收敛速度快、全局搜索能力强等优点,因此在实际工程优化中得到广泛应用。
差分进化算法的基本原理是通过不断改进目标函数来优化群体中的个体。
算法的基本流程如下:1. 初始化:随机生成足够多的初始个体,构成初始群体。
2. 变异:对于每个个体,根据固定的变异策略生成一个变异个体。
3. 交叉:将原个体和变异个体进行交叉,得到一个新的个体。
4. 选择:从原个体和交叉个体中选择更优的一个作为下一代的个体。
5. 更新群体:将新个体代替原个体,同时保留所有代的最优解。
变异策略和交叉方法是差分进化算法的核心部分。
1. 变异策略:变异策略是指在进化过程中,对每个个体进行的变异操作。
常用的变异策略有DE/rand/1、DE/rand/2和DE/best/1等。
“DE”表示差分进化,“rand”表示随机选择其他个体进行变异,“best”表示选择当前代的最优解。
以DE/rand/1为例,其变异操作步骤如下:(1)从群体中随机选择两个个体(除当前个体之外);(2)根据固定的变异因子F,生成一个变异向量v;(3)计算原个体与变异向量v的差分,得到新的个体。
变异因子F的值通常取0.5-1.0,表示变异向量中各项的取值在变量取值范围内随机变化的程度。
2. 交叉方法:交叉方法是指在变异个体和原个体之间进行的交叉操作。
常用的交叉方法有“二项式交叉”和“指数交叉”等。
以二项式交叉为例,其交叉操作步骤如下:(1)对于变异向量v中的每一维,以一定的概率Cr选择变异向量中的该维,否则选择原个体中的该维;(2)得到新的个体。
Cr表示交叉率,通常取值在0.1-0.9之间。
差分进化算法的收敛性和全局搜索能力与变异策略和交叉方法的选择密切相关。
聚类分析的算法优化与改进
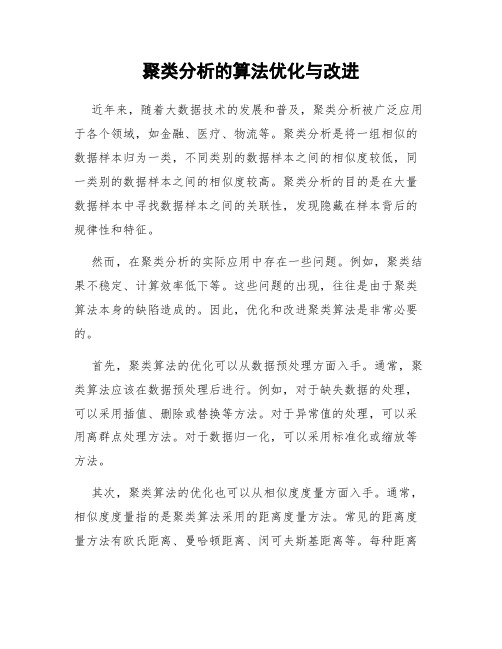
聚类分析的算法优化与改进近年来,随着大数据技术的发展和普及,聚类分析被广泛应用于各个领域,如金融、医疗、物流等。
聚类分析是将一组相似的数据样本归为一类,不同类别的数据样本之间的相似度较低,同一类别的数据样本之间的相似度较高。
聚类分析的目的是在大量数据样本中寻找数据样本之间的关联性,发现隐藏在样本背后的规律性和特征。
然而,在聚类分析的实际应用中存在一些问题。
例如,聚类结果不稳定、计算效率低下等。
这些问题的出现,往往是由于聚类算法本身的缺陷造成的。
因此,优化和改进聚类算法是非常必要的。
首先,聚类算法的优化可以从数据预处理方面入手。
通常,聚类算法应该在数据预处理后进行。
例如,对于缺失数据的处理,可以采用插值、删除或替换等方法。
对于异常值的处理,可以采用离群点处理方法。
对于数据归一化,可以采用标准化或缩放等方法。
其次,聚类算法的优化也可以从相似度度量方面入手。
通常,相似度度量指的是聚类算法采用的距离度量方法。
常见的距离度量方法有欧氏距离、曼哈顿距离、闵可夫斯基距离等。
每种距离度量方法有其特点和适用的场景。
选择合适的距离度量方法对聚类算法的效果至关重要。
最后,聚类算法的改进可以从聚类结果的评估方面入手。
通常,评估聚类结果需要采用有效性指标,例如轮廓系数、DB指数、Dunn指数等。
这些指标可以用来评估聚类结果的准确度和稳定性。
通过评估聚类结果,可以不断改进聚类算法,提高算法的准确性和稳定性。
综上所述,聚类算法的优化和改进可以从多个方面入手,例如数据预处理、相似度度量和聚类结果评估。
在实际应用过程中,我们应该根据具体的场景和需求选择合适的优化和改进方法,以达到最好的聚类效果。
基于改进差分进化算法的网络空间入侵检测研究

由通信网络入侵信息模型,提取入侵信息的异常谱特征 .采用访问接口控制的方法,重构网 络 入 侵 信 息 模 型,
建立多尺度卷积神经通信网络入侵 信 息 的 模 糊 关 联 规 则 分 布 集,提 取 多 尺 度 卷 积 神 经 通 信 网 络 入 侵 信 息 的
谱特征量;采用改进差分进化法进行 多 尺 度 卷 积 神 经 通 信 网 络 入 侵 信 息 的 多 维 模 糊 度 特 征 检 测 和 信 息 聚 类
行多尺度卷积神经通信网络入侵信息的聚类检测,
得到入侵特征序列满足:
(
σ1 > …σL >σL+1 = …σ4P =0
15)
多尺度卷积神经通信网络入侵信号的效用度
限 为 1.
58,网 络 入 侵 的 信 噪 比 为 SNR =0~10
dB,差分进化 的 迭 代 步 数 为 1000,时 间 分 布 参 数
Da
t
a5
92114
22154
92351
神经通信网络入侵信息的二元编码,由此得到多尺
度卷积神经通信网络入侵信息的谱特征提取为:
X1(
k)=
,
FFT x1(
k),
x1(
k +1),
.
.
.
.
.
.
x1(
k +N -1)
T
(
1
7)
X2(
k)=
T
,
FFT x2(
k),
x2(
k +1),
.
.
.
.
.
.
x2(
k +N -1)
神经通信网络入侵信息模型重构
进行多尺度卷积神经通信网络空间入侵检测,
物流配送论文题目(推荐题目107个)

物流配送论文题目(推荐题目107个) 物流配送是物流的一个缩影或在某小范围中物流全部活动的体现。
一般的物流是运输及保管,而配送则是分拣配货及运输,其更关注于按待运输货物的目的地来将其区分,以便于物流操作。
物流配送的主要工作有:备货、储存、加工、分拣及配货、配装、配送运输、送达服务。
以下是整理好的关于物流配送论文题目107个,供大家参考。
物流配送论文题目一: 1、京东自建物流配送模式研究 2、民用无人机在物流配送行业中的分析与设计 3、基于Spark的并行遗传算法在物流配送问题中的应用 4、“大数据”思维下的烟草物流配送中心设备管理系统开发与应用 5、互联网+视角下农村电商物流配送运作模式分析 6、O2O模式下电商物流配送策略探析 7、基于共享经济背景的农村物流配送体系构建——以新型城镇化背景下的长株潭农村地区为例 8、基于无人机物流配送的战时快速卫勤保障体系探讨 9、RFID技术应用于农超对接物流配送系统的经济效应 10、基于蚁群算法的物流配送路径的研究 11、城市物流配送车辆调度模型及优化 12、基于电商环境的农产品物流配送体系构建 13、物流配送的绩效评价体系的构建——以苏宁易购为例 14、我国零售业连锁经营的物流配送模式优化研究 15、大型物流配送中心的主动式仓储调度策略及其性能分析 16、农村电子商务物流配送改革策略分析 17、浅谈京东物流配送模式的优化 18、国内连锁超市物流配送优化方案研究 19、大数据背景下电子商务物流配送模式研究 20、B2C电子商务企业物流配送模式比较研究 21、基于组合拍卖的B2C电商物流配送研究 22、国内连锁经营企业物流配送模式对库存水平影响的实证研究 23、物联网技术下的农产品冷链物流配送优化研究 24、基于改进自适应遗传算法的物流配送路径优化研究 25、基于城市道路拥堵的物流配送车辆停车收费定价研究 物流配送论文题目二: 26、鑫威超市基于顾客满意度的物流配送管理研究 27、浅析生鲜农产品电商物流配送模式的优化 28、借鉴欧美城市物流配送的经验做法解决好我国城市物流“最后一公里”问题 29、改进人工人群搜索算法在基于LBS物流配送中的应用 30、基于层次化网络优化的烟草物流配送网络的开发与设计 31、基于模拟退火算法最优物流配送问题的应用 32、改进差分进化算法在物流配送中的多目标优化研究 33、一种面向智慧城市的自动物流配送系统初探 34、农产品电商综合物流配送模式研究——以广西海吉星电商综合配送模式为例 35、茶产业发展中物流配送模式研究 36、TSP模型在蔬菜基地物流配送中的应用 37、O2O模式下物流配送研究综述 38、基于Flexsim的山区生鲜农产品冷链物流配送网络仿真分析 39、O2O模式下零售企业物流配送网络节点的优化布局 40、电子商务环境下物流配送网络协同性研究 41、共享经济视角下我国乡村地区最后一公里物流配送模式优化研究 42、7-11物流配送模式分析 43、基于RFID&GPS/GPRS技术下的电商生鲜物流配送 44、柳州融水电子商务物流配送路径优化 45、基于直觉模糊集的中小型企业第三方物流配送服务商选择研究 46、城市冷链物流配送车辆路径问题研究 47、基于容器标准化的智能物流配送 48、基于城乡双向互动的物流配送网络创新研究 49、农村连锁超市物流配送问题及解决途径探究 50、O2O模式连锁企业农产品物流配送路径优化 物流配送论文题目三: 51、物流配送线路优化方案设计 52、充换电站模式下电动汽车参与物流配送的电能补给方式规划 53、我国生鲜农产品冷链物流配送路径优化研究 54、农村物流配送网络建设的影响因素分析 55、腐败率线性可变的冷链物流配送中心选址优化 56、基于车联网的物流配送系统设计与实现 57、京东物流配送模式优化分析 58、电商终端物流配送模式比较与创新 59、电子商务企业物流配送路径优化研究 60、基于区域经济学视角的我国城市商业物流配送体系构建研究 61、城乡融合背景下农产品新零售物流配送模式探析 62、基于系统动力学的冷链物流配送效率因素分析及提升策略 63、装配线平衡与物流配送路径协同优化 64、低成本卷烟物流配送网络的研究 65、基于改进FMEA的冷链物流配送过程风险分析 66、基于客户厌恶度的冷链物流配送网络优化 67、基于遗传算法的生鲜农产品物流配送路径聚类优化 68、用混合遗传算法求解物流配送路径优化问题 69、电子商务背景下茶产业的物流配送模式研究 70、物流配送车辆最优网络路径选取仿真 71、农产品物流配送中心选址优化研究 72、青岛大鲍岛干海货城物流配送中心规划与设计 73、城市物流配送中心选址方法及应用研究 74、我国C2C电子商务物流配送模式的问题及对策 75、生鲜农产品电商物流配送模式研究 物流配送论文题目四: 76、基于电动汽车的物流配送优化问题研究综述 77、基于Flexsim的烟草物流配送中心规划仿真 78、基于蚁群算法和禁忌搜索算法的物流配送优化研究 79、带时间窗的同城物流配送区域划分与路径优化 80、生鲜农产品冷链物流配送网络优化 81、基于电子商务的农村物流配送体系优化研究 82、电子商务环境下企业物流配送模式选择 83、基于ThinkPHP的物流配送系统的设计与实现 84、基于O2O的物流配送综合成本评价研究 85、某电子商务物流配送中心布局规划设计 86、农产品电子商务物流配送体系构建研究 87、电商企业农村物流配送模式对比分析 88、我国B2C电子商务的物流配送模式研究——以京东商城为例 89、共享条件下的地下物流配送路线优化 90、基于时空模式的仓库物流配送路径优化 91、我国生鲜电商物流配送模式比较分析 92、浅谈农村电商物流配送解决方案 93、基于BIRCH聚类的物流配送设施选址算法 94、大数据下可视化物流配送优化选址仿真 95、生鲜农产品冷链物流配送路径优化探究 96、创新驱动发展战略下珠三角高校校园物流配送研究 97、同城物流配送车辆调度问题研究——以西安飞雕电器为例 98、基于混合遗传算法的物流配送路径优化研究 99、基于循环经济的电商环境下城乡物流配送模式探讨 100、基于改进的鸟群算法求解农产品冷链物流配送路径优化问题 物流配送论文题目五: 101、区域农产品供给物流配送组织模式研究 102、基于层次分析法的物流配送车辆选型研究 103、生鲜农产品冷链物流配送模式优化策略研究 104、“互联网+”江门区域物流配送中心构建研究 105、RFID技术在物流配送中的应用 106、浅谈农村电商物流配送解决方案 107、小型医药企业物流配送网络设计。
基于改进多目标萤火虫算法的模糊聚类_朱书伟
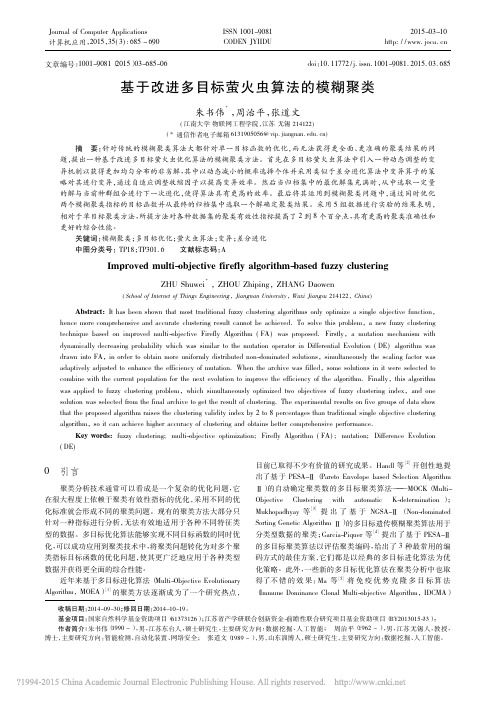
with
automatic
Kdetermination ) ;
Mukhopadhyay 等
dominated Ⅱ ( Non提 出 了 基 于 NGSA-
Sorting Genetic Algorithm Ⅱ) 的多目标遗传模糊聚类算法用于 Piquer 等[4] 提出了基于 PESA分类型数据的聚类; GarciaⅡ 的多目标聚类算法以评估聚类编码, 给出了 3 种最常用的编 码方式的最佳方案, 它们都是以经典的多目标进化算法为优 化策略。此外, 一些新的多目标优化算法在聚类分析中也取
[5 ] 得了不 错 的 效 果: Ma 等 将 免 疫 优 势 克 隆 多 目 标 算 法
的聚类方法逐渐成为了一个研究热点,
( Immune Dominance Clonal Multiobjective Algorithm,IDCMA)
收稿日期: 2014-09-30 ; 修回日期: 2014-10-19 。 33 ) 。 基金项目: 国家自然科学基金资助项目( 61373126 ) ; 江苏省产学研联合创新资金前瞻性联合研究项目基金资助项目( BY2013015男, 江苏东台人, 硕士研究生, 主要研究方向: 数据挖掘、 人工智能; 周治平( 1962 - ) , 男, 江苏无锡人, 教授, 作者简介: 朱书伟( 1990 - ) , 博士, 主要研究方向: 智能检测、 自动化装置、 网络安全; 张道文( 1989 - ) , 男, 山东淄博人, 硕士研究生, 主要研究方向: 数据挖掘、 人工智能。
[11 ]
。近
Objective Firefly Yang[12] 对 多 目 标 萤 火 虫 算 法 ( Multi来, Algorithm,MOFA) 也进行研究分析, 相对于几种常用的多目 标优化算法而言能更好地解决连续性优化问题 。 袁际军
Weka平台上解决聚类的改进差分进化算法
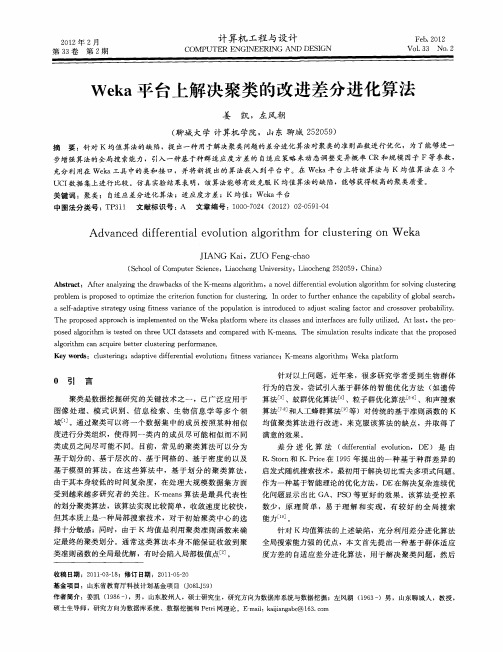
UC 数据集上进行 比较 。仿真 实验 结果表 明,该 算法能够有效克服 K均值算法的缺 陷,能够获得较 高的聚类质量 。 I
关键 词 :聚类 ; 自适应差分进化算法 ;适应度 方差;K均值 ;Wea平 台 k
中图法分类号 : 3 1 文献标识号 : TP 1 A 文 章 编 号 : 0 07 2 (0 2 20 9 —4 1 0—0 4 2 1 )0 —5 10
a g r h c n a q ie b te l s e i g p ro m a c . l o i m a c ur e t r cu t rn e f r n e t Ke r s cu t r g;a a tv i e e t le o u in;f n s a i n e y wo d : l s e i n d p ie d f r n i v l t f a o i e sv ra c ;K- a sa g rt m ;W e a p a f r t me n lo ih k lto m
图像 处 理 、模 式 识 别 、信 息检 索 、生 物信 息学 等 多个 领 域n 。通过聚类可 以将一个 数 据集 中的成 员按 照某种 相似 ] 度进行分类组织 ,使 得 同一类 内的成 员尽 可能 相似 而不 同 类成员之间尽 可能不 同。 目前 ,常见 的聚类 算法 可 以分 为 基于划分 的、基 于层 次的 、基 于 网格 的 、基 于密度 的以及 基 于模型 的算 法 。在 这些 算 法 中 ,基 于划 分 的 聚类 算 法 ,
2 1 年 2月 02
计算机 工程 与设计
COM PUTER ENGI NEERI NG AND S GN DE I
F b 2 1 e.02
Vo . 3 NO 2 13 .
差分进化算法的改进及在约束优化中的应用
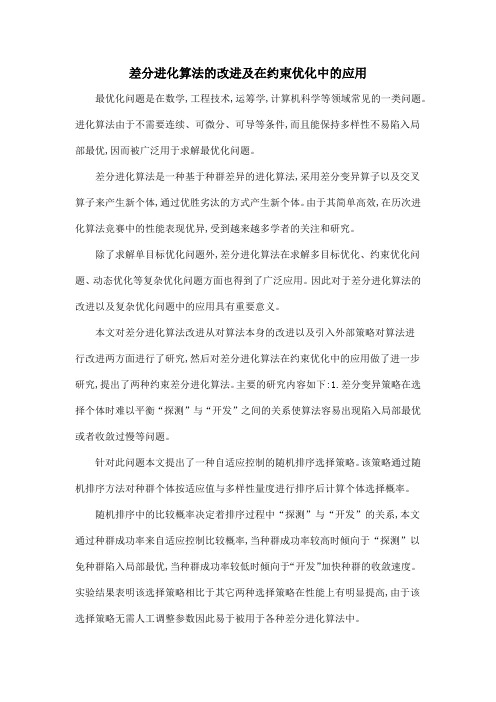
差分进化算法的改进及在约束优化中的应用最优化问题是在数学,工程技术,运筹学,计算机科学等领域常见的一类问题。
进化算法由于不需要连续、可微分、可导等条件,而且能保持多样性不易陷入局部最优,因而被广泛用于求解最优化问题。
差分进化算法是一种基于种群差异的进化算法,采用差分变异算子以及交叉算子来产生新个体,通过优胜劣汰的方式产生新个体。
由于其简单高效,在历次进化算法竞赛中的性能表现优异,受到越来越多学者的关注和研究。
除了求解单目标优化问题外,差分进化算法在求解多目标优化、约束优化问题、动态优化等复杂优化问题方面也得到了广泛应用。
因此对于差分进化算法的改进以及复杂优化问题中的应用具有重要意义。
本文对差分进化算法改进从对算法本身的改进以及引入外部策略对算法进行改进两方面进行了研究,然后对差分进化算法在约束优化中的应用做了进一步研究,提出了两种约束差分进化算法。
主要的研究内容如下:1.差分变异策略在选择个体时难以平衡“探测”与“开发”之间的关系使算法容易出现陷入局部最优或者收敛过慢等问题。
针对此问题本文提出了一种自适应控制的随机排序选择策略。
该策略通过随机排序方法对种群个体按适应值与多样性量度进行排序后计算个体选择概率。
随机排序中的比较概率决定着排序过程中“探测”与“开发”的关系,本文通过种群成功率来自适应控制比较概率,当种群成功率较高时倾向于“探测”以免种群陷入局部最优,当种群成功率较低时倾向于“开发”加快种群的收敛速度。
实验结果表明该选择策略相比于其它两种选择策略在性能上有明显提高,由于该选择策略无需人工调整参数因此易于被用于各种差分进化算法中。
2.反向学习策略是一种改进差分进化算法的外部策略,该策略通过生成反向种群使种群有更大几率接近全局最优解。
由于该策略在个体的所有维度上均取反向值,在求解多维问题时可能使得种群在某些维度上远离最优解。
针对此问题本文通过生成部分反向解来加强反向学习的维度开发能力,然而生成所有的部分反向解会极大增加函数评价次数使收敛变慢,因此本文利用正交设计均匀分布的特性仅生成少量代表性的部分反向个体,在加强反向学习维度开发能力与减少函数评价次数之间取得了平衡,从而增强了算法性能。
一种改进的差分进化自动聚类算法

( eat et f o p t cec n eh o g ,G agogU i r t o i ne D pr n o m ue SineadT cnl y undn nv sy f n c , m C r o e i F a G aghuG a gog50 2 ,C ia unzo und n 15 1 hn )
ABS TRACT : tmai l se n loih a e n e ou in c mp t t n h v lw c n e g n e r ts I h s Auo t c u t r g ag r ms b s d o v l t o u ai a e s o v r e c ae . n t i c i t o o o
ppr a e ,we p o o e a mo iid l o t r p s dfe ag r hm t a tr c n ege e r t s d o ifr n il v l i n The de ru e i wih f se o v r nc ae ba e n d fee ta e outo . pat r
关键词 : 自动 聚 类 ; 分进 化 ; 局优 化 差 全
中 图 分 类 号 :P 8 r 1 r 文 献 标 识 码 : A
A o i e M d f d Aut m a i u t r ng Al o ih i o tc Cl s e i g r t m f r Dif r n i lEv l to 0 f e e ta o u i n
第2卷 第1期 7 1
文 章 编 号 :06— 3 8 2 1 1 —06 o 10 9 4 ( 00) 1 0 9一 4
计
差分变异变异的改进
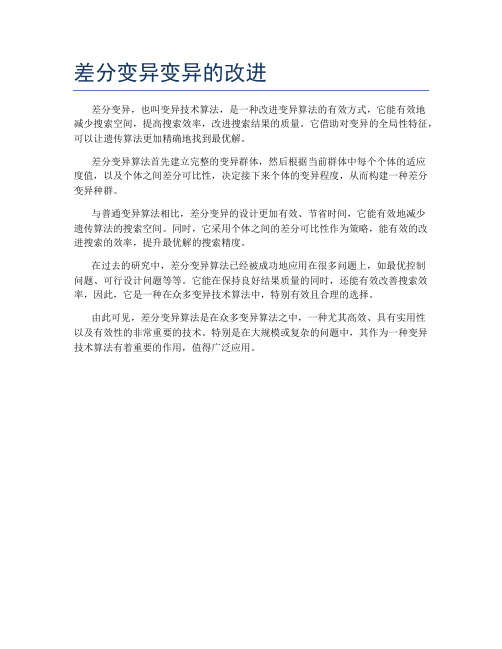
差分变异变异的改进
差分变异,也叫变异技术算法,是一种改进变异算法的有效方式,它能有效地
减少搜索空间,提高搜索效率,改进搜索结果的质量。
它借助对变异的全局性特征,可以让遗传算法更加精确地找到最优解。
差分变异算法首先建立完整的变异群体,然后根据当前群体中每个个体的适应
度值,以及个体之间差分可比性,决定接下来个体的变异程度,从而构建一种差分变异种群。
与普通变异算法相比,差分变异的设计更加有效、节省时间,它能有效地减少
遗传算法的搜索空间。
同时,它采用个体之间的差分可比性作为策略,能有效的改进搜索的效率,提升最优解的搜索精度。
在过去的研究中,差分变异算法已经被成功地应用在很多问题上,如最优控制
问题、可行设计问题等等。
它能在保持良好结果质量的同时,还能有效改善搜索效率,因此,它是一种在众多变异技术算法中,特别有效且合理的选择。
由此可见,差分变异算法是在众多变异算法之中,一种尤其高效、具有实用性
以及有效性的非常重要的技术。
特别是在大规模或复杂的问题中,其作为一种变异技术算法有着重要的作用,值得广泛应用。
《吸引子传播聚类算法的理论改进及其应用》

《吸引子传播聚类算法的理论改进及其应用》一、引言随着大数据时代的到来,数据挖掘和机器学习技术得到了广泛的应用。
其中,聚类算法作为数据挖掘的重要手段之一,受到了越来越多的关注。
吸引子传播聚类算法(Affinity Propagation Algorithm)是一种新型的聚类方法,以其高效和良好的聚类效果得到了众多研究者的青睐。
本文将重点介绍吸引子传播聚类算法的理论改进及其应用。
二、吸引子传播聚类算法概述吸引子传播聚类算法是一种基于数据点之间相似性的无监督聚类方法。
该算法通过计算数据点之间的吸引度值和归属度值,实现数据的自动聚类。
该算法的优点在于不需要预先设定聚类的数量,且在处理大规模数据集时具有较高的效率。
然而,该算法在处理某些复杂数据集时仍存在一些不足,如对噪声和异常值的敏感性、参数设置的复杂性等。
三、理论改进针对吸引子传播聚类算法的不足,本文提出以下理论改进措施:1. 引入新的相似度度量方法:传统的吸引子传播聚类算法通常采用欧氏距离等简单的方法计算数据点之间的相似度。
本文提出采用更复杂的相似度度量方法,如核方法和深度学习特征等方法,提高数据点的相似性度量准确性。
2. 参数优化策略:吸引子传播聚类算法中的一些参数设置对聚类效果具有重要影响。
本文提出一种基于自适应阈值的参数优化策略,通过动态调整参数,使算法在处理不同类型的数据集时能够取得更好的效果。
3. 结合其他算法的优点:本文将吸引子传播聚类算法与其他聚类算法相结合,如K-means、谱聚类等,形成混合聚类算法。
通过结合不同算法的优点,提高聚类的准确性和鲁棒性。
四、应用改进后的吸引子传播聚类算法在多个领域得到了应用:1. 图像分割:在图像分割领域,吸引子传播聚类算法可以用于图像的自动分割和分类。
通过对图像的像素或特征点进行聚类,实现图像的语义分割和区域分割等任务。
2. 文本聚类:在文本处理领域,本文利用改进后的吸引子传播聚类算法进行文本的自动分类和聚类。
- 1、下载文档前请自行甄别文档内容的完整性,平台不提供额外的编辑、内容补充、找答案等附加服务。
- 2、"仅部分预览"的文档,不可在线预览部分如存在完整性等问题,可反馈申请退款(可完整预览的文档不适用该条件!)。
- 3、如文档侵犯您的权益,请联系客服反馈,我们会尽快为您处理(人工客服工作时间:9:00-18:30)。
收稿日期:2011-07-11基金项目:国家自然科学基金(10926157);国家十一五科技支撑计划(2009BAE69B01)作者简介:曲福恒(1979-),男,博士,主要从事图像处理与模式识别技术研究。
长春理工大学学报(自然科学版)Journal of Changchun University of Science and Technology (Natural Science Edition )第34卷第4期2011年12月Vol.34No.4Dec.2011改进差分进化算法及其在模糊聚类分析中的应用曲福恒1,胡雅婷2,杨勇1,谷欣超1(1.长春理工大学计算机科学技术学院,长春130022;2.吉林农业大学信息技术学院,长春130118)摘要:针对差分进化算法早熟与搜索效率不理想的问题,提出一种改进的差分进化算法。
算法在变异阶段采用多策略与多参数并行的方法一次产生多个变异个体,有效地保持了种群中个体的多样性,抑制了早熟现象的发生。
根据竞争机制选择适应度最好的变异个体进行选择操作,提高了搜索效率。
与差分进化及其改进算法的对比实验表明了算法的有效性,并把提出的算法应用到模糊聚类分析中,较好的解决了原始聚类模型求解容易陷入局部极值的问题。
关键词:全局优化;差分进化;进化策略;聚类分析中图分类号:TP183文献标识码:A文章编号:1672-9870(2011)04-0129-04An Improved Differential Evolution Algorithm with ItsApplication in Fuzzy Cluster AnalysisQU Fuheng 1,HU Yating 2,YANG Yong 1,GU Xinchao 1(1.Department of Computer Science and Technology ,Changchun University of Science and Technology ,Changchun 130022;2.College of Information and Technology ,Jilin Agriculture University ,Changchun 130118)Abstract :A new differential evolution algorithm (DE )is proposed in this paper to overcome the problem of prema-ture convergence and low search efficiency of the original DE.In the new algorithm ,several mutant individuals are gen-erated simultaneously in each population using different strategies with some specified parameters ,which effectively maintains population diversity and avoids premature phenomenon in the evolution.According to the survival of the fittest law ,the trial individual with the best fitness value will enter the selection stage to generate the new population ,which improves the search efficiency.The contrast experimental results with DE and its improved algorithm show that the ef-fectiveness of the proposed algorithm.The algorithm is also applied into the fuzzy cluster analysis and solves the prob-lem of falling into the local extreme of the original cluster model.Key words :global optimization ;differential evolution ;evolution strategy ;cluster analysis差分进化算法(Differential evolution ,DE )是由Storn 与Price 提出的一种进化算法[1],DE 性能良好且易于控制,已经成功的应用于各个领域,成为进化算法的研究热点之一。
与其它的进化算法一样,DE 是一种基于种群演化的随机搜索算法。
它通过变异、交叉、选择操作引导种群走向优化问题的全局最优解。
DE 的性能主要依赖于新个体的产生策略及算法中的三个控制参数种群规模NP 、缩放因子F 与交叉概率CR 。
在面临一个具体的最优化问题时,首先需要确定采用哪种策略来运行DE ,然后还要根据当前的策略选择合适的参数。
选择的不合理可能导致算法的搜索效率低下以及产生早熟收敛的问题。
不同的优化问题其适用的策略与参数可能不同,这就需要采用尝试不同的组合来找到最适合于当前问题的策略与参数。
DE 的进化策略众多加之不同的参数都具有一定的取值范围,这种穷举尝试的方法在效率上显然是非常低下的。
为了解决这个问题,学者们提出了自适应参数或者策略的DE 算法。
多数自适应DE 算法集中在参数方面,大体可分为两类,第一类是通过进化过程的信息反馈来调整DE 的参数[2],第二种是直接把DE 的参数编码于长春理工大学学报(自然科学版)2011年进化个体中直接参与进化[3]。
最近,学者对DE的策略进行了自适应方面的研究,提出了自适应策略的DE算法[4],取得了一定的进展并提高了DE的性能,值得指出的是算法不需要设定任何参数具有较好的可控性[5]。
综上可知,DE算法性能依赖于策略与参数的选择,选择不当会导致算法的早熟和搜索效率低下。
针对这个问题,本文提出了一种多策略多参数并行的DE算法。
在种群的演化过程中,对每个目标个体采用多个策略与多个参数,一次产生多个试验个体。
利用竞争规则确定进入选择阶段的优势个体,有效的保持了种群内个体的多样性,提高了搜索效率,在一定程度上避免了早熟收敛现象的发生。
1差分进化算法DE算法是一种简单高效的基于种群演化的随机全局优化技术,由Storn和Price于1995年提出[6]。
与其它进化算法一样,DE从某一随机初始化的群体开始,通过多种操作(变异和交叉)产生新的个体,在按照一定的规则进行选择操作决定进入下一代的个体,最终引导种群走向全局最优解。
下面以典型的DE算法-DE/rand/1/bin来叙述各个操作的细节。
变异操作假设在当前第g代种群中个体向量为x i g=(x i g,1,x i g,2,…,x i g,D),i=1,2,…,NP,g=0,1,…,g max。
对于每个第g代个体x i g,它的变异向量v i g定义为:v i g=x r1g+F(x r2g-x r3g)这里r l(l=1,2,3)是从集合{1,2,…,NP}中随机选择的互不相同整数。
F∈[0,2]为缩放因子,它体现了新向量中差分向量x r2g-x r3g所占的比重。
交叉操作对目标个体x i g的每个分量x ig,j(j=1,2,…,D)进行交叉操作生成实验个体u i g:u i g,j=ìíîv i g,j,如果(rand(0,1)≤CR或j=j rand);x ig,j,其它.CR∈[0,1)是交叉概率,j rand∈{1,2,…,D}是一个均匀分布随机数发生器产生的整数。
选择操作选择操作决定在第g代中目标个体x ig与试验个体u i g哪一个进入第g+1代。
对于最小化问题,选择操作具体如下:x ig+1=ìíîu i g,如果f(u i g)<f(x i g);x ig,其它.2改进的差分进化算法差分进化算法通过两个主要的操作变异和交叉来对种群个体进行扰动,从而保持种群内个体的多样性,实现在整个搜索空间的开发;通过选择操作引导个体走向全局最优解,实现算法在局部区域的探索。
这种扰动(开发)与选择(探索)过程的实现与其它的进化算法类似。
在扰动过程中,首先在父代中,按照某种规则选择一个个体加上两个随机选择个体的差向量,得到一个新个体,DE算法的个体以一定的概率被新个体取代。
选择过程中,通过适者生存的竞争机制保留新个体与原个体中的优势个体。
DE算法中至少存在三种不同性质的收敛类型:全局收敛:算法在要求的代数内达到了全局最优解。
此时表明,算法在开发和探索之间达到了一个较好的平衡。
早熟收敛:算法在搜索过程中陷入了某个非最优解点。
表明算法的开发能力较弱,没有有效地保持种群的多样性。
慢速收敛:算法具有找到全局最优解的能力,但速度较慢没有在要求的代数内达到全局最优解。
表明与探索能力相比,开发(扰动)能力过强。
影响DE算法收敛性质的两个最为重要的因素为控制参数和进化策略。
目前对控制参数的研究较多,控制参数包括种群规模、交叉概率和缩放因子。
大量研究的实验结果表明,DE算法的性能对控制参数的取值极为敏感。
已有的经验表明,对应于三种不同的收敛性质可以把参数空间分解为三部分。
假设已经得到了参数空间的这种分解,将很容易的对算法的参数进行选择。
然而不幸的是,这种参数空间的分解是很难得到的。
鉴于此,学者对自适应参数的DE算法进行了大量的研究,并取得了一定进展。
结果表明,自适应参数的DE算法在保持种群的多样性同时具有较好的探索能力。
另一方面,DE 算法的进化策略众多,不同的进化策略具有不同的寻优性能。
最近,学者对DE的策略进行了自适应方面的研究,提出了自适应策略的DE算法[4],取得了一定的进展并提高了DE的性能。
这也说明良好的策略对于保持开发与探索之间的平衡具有重要的意义。
由于优化问题本身性质(如单峰或者多峰)的不同,不可能找到一个统一组合策略与参数的DE算130第四期曲福恒,等:改进差分进化算法及其在模糊聚类分析中的应用法来有效地处理不同类型的优化问题;而对于一个具体的优化问题,不同策略和参数组合的DE算法的寻优能力也会有差异[4,7]。
对于一个具体的问题,某些组合具有更高的寻优能力,说明它在进化的过程中较好的保持了个体的多样性,并能够在开发和探索上达到了较好的平衡。