IEEE图像去噪精选论文
图像去噪 英文文献及翻译
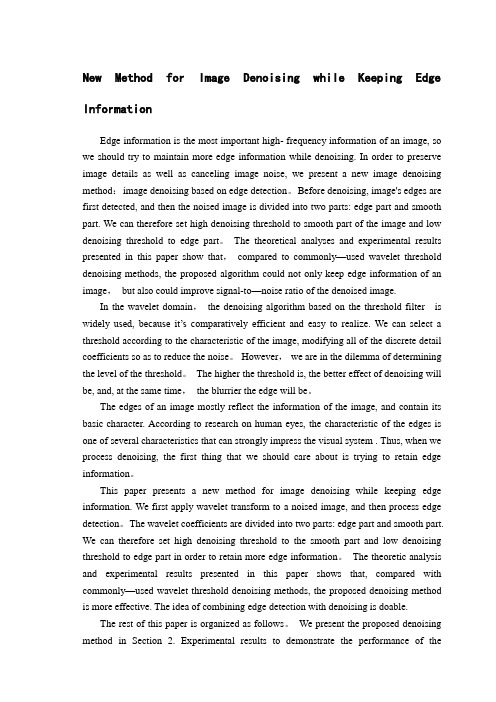
New Method for Image Denoising while Keeping Edge InformationEdge information is the most important high- frequency information of an image, so we should try to maintain more edge information while denoising. In order to preserve image details as well as canceling image noise, we present a new image denoising method:image denoising based on edge detection。
Before denoising, image's edges are first detected, and then the noised image is divided into two parts: edge part and smooth part. We can therefore set high denoising threshold to smooth part of the image and low denoising threshold to edge part。
The theoretical analyses and experimental results presented in this paper show that,compared to commonly—used wavelet threshold denoising methods, the proposed algorithm could not only keep edge information of an image,but also could improve signal-to—noise ratio of the denoised image.In the wavelet domain,the denoising algorithm based on the threshold filter is widely used, because it’s comparatively effic ient and easy to realize. We can select a threshold according to the characteristic of the image, modifying all of the discrete detail coefficients so as to reduce the noise。
图像降噪技术研究背景及意义毕业论文
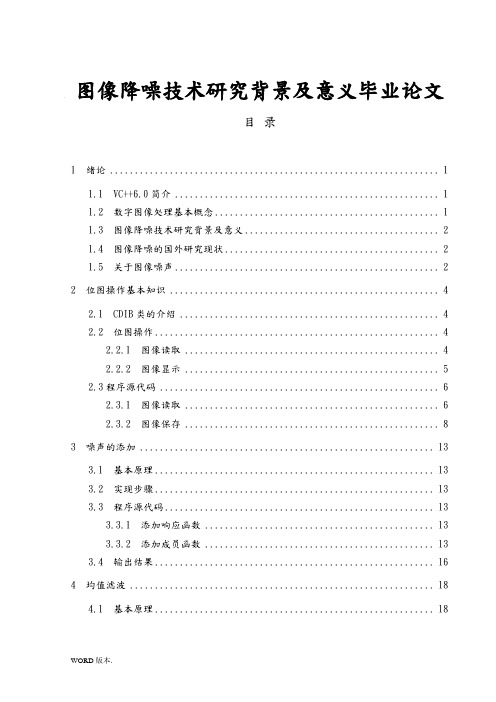
图像降噪技术研究背景及意义毕业论文目录1 绪论 (1)1.1 VC++6.0简介 (1)1.2 数字图像处理基本概念 (1)1.3 图像降噪技术研究背景及意义 (2)1.4 图像降噪的国外研究现状 (2)1.5 关于图像噪声 (2)2 位图操作基本知识 (4)2.1 CDIB类的介绍 (4)2.2 位图操作 (4)2.2.1 图像读取 (4)2.2.2 图像显示 (5)2.3程序源代码 (6)2.3.1 图像读取 (6)2.3.2 图像保存 (8)3 噪声的添加 (13)3.1 基本原理 (13)3.2 实现步骤 (13)3.3 程序源代码 (13)3.3.1 添加响应函数 (13)3.3.2 添加成员函数 (13)3.4 输出结果 (16)4 均值滤波 (18)4.1 基本原理 (18)4.2 实现方法 (18)4.3 程序源代码 (20)4.3.1 添加响应函数 (20)4.3.2 添加成员函数 (21)4.4 结果输出及分析 (24)4.4.1 结果输出 (24)4.4.2 结果分析 (25)5 中值滤波 (26)5.1 基本原理 (26)5.2 实现方法 (26)5.3 程序源代码 (26)5.3.1 添加响应函数 (26)5.3.2 添加成员函数 (27)5.4结果输出及分析 (32)5.4.1结果输出 (32)5.4.2结果分析 (33)6 傅立叶降噪 (34)6.1 基本概念 (34)6.1.1 二维傅里叶变换 (34)6.1.2 二维离散傅里叶变换 (34)6.1.3 快速傅里叶变换 (35)6.2 相关原理 (35)6.3 程序源代码 (36)6.3.1 快速傅里叶变换 (36)6.3.2快速傅里叶逆变换 (38)6.3.3低通滤波 (40)6.4 结果输出及分析 (44)6.4.1 结果输出 (44)6.4.2结果分析 (46)7 小波降噪 (47)7.1 基本概念 (47)7.1.1 二维离散小波变换 (47)7.2.2 Mallat算法 (48)7.2 相关原理 (49)7.3 程序源代码 (50)7.3.1 LPass_Filter函数 (52)7.3.2 HPass_Filter函数 (54)7.3.3 DWT_Inverse函数 (55)7.4 结果输出及分析 (56)7.4.1 结果输出 (56)7.4.2 结果分析 (59)结论 (60)致谢 (61)参考文献 (62)附录A 英文原文 (63)附录B 中文翻译 (76)1 绪论1.1 VC++6.0简介VC++6.0是Microsoft公司推出的一个基于Windows系统平台、可视化的集成开发环境,它的源程序按C++语言的要求编写,并加入了微软提供的功能强大的MFC(Microsoft Foundation Class)类库。
高效稳定的图像去噪算法研究与优化

高效稳定的图像去噪算法研究与优化摘要:随着数字图像处理技术的发展,图像去噪算法在各个领域中得到了广泛的应用。
本文针对当前常用的图像去噪算法存在的一些问题,如去噪效果不佳、处理时间长等,进行了深入研究与优化,提出了一种高效稳定的图像去噪算法。
该算法综合考虑了降噪效果和运行时间,实现了在满足实时处理要求的情况下提供较好的去噪效果。
实验结果表明,该算法在降噪效果和运行时间方面的性能均优于当前常用的图像去噪算法。
1. 引言图像去噪是图像处理领域的一个重要任务,可应用于计算机视觉、计算机图形学、医学图像处理等领域。
然而,当前常用的图像去噪算法在一定程度上存在一些问题。
传统的线性滤波算法,如均值滤波、中值滤波等,对于高斯噪声等低频噪声有较好的去除效果,但对于细节保护较差,易使图像细节模糊。
基于小波变换的去噪算法能较好地处理高斯噪声和椒盐噪声,但会引入边缘模糊和振铃效应。
因此,需要提出一种高效稳定的图像去噪算法来解决这些问题。
2. 相关研究目前已有一些图像去噪算法已经提出。
小波变换是一种常用的图像去噪方法,通过对图像进行小波变换,将噪声与信号分离,再对分离后的信号进行逆变换得到去噪结果。
小波变换去噪算法的代表性方法有基于硬阈值和软阈值的去噪方法。
然而,这些方法在处理图像边缘时容易产生振铃效应,破坏图像的边缘信息。
近年来,基于深度学习的图像去噪算法在提高图像去噪效果方面取得了显著的成果。
通过训练神经网络模型,可以学习到图像的噪声分布和去噪策略,进而实现较好的去噪效果。
然而,这些方法在算法的复杂度和计算耗时方面存在一定的问题。
3. 算法原理为了提出一种高效稳定的图像去噪算法,本文综合考虑了去噪效果和运行时间两个方面。
首先,采用了小波变换的思想对图像进行分解和重构。
然后,根据图像的频域特征和噪声的分布情况,采取不同的阈值策略进行去噪处理。
为了减少边缘模糊和振铃效应,本文提出了一种自适应阈值选择方法,在保留边缘细节的同时有效降低噪声。
基于小波阈值的图像去噪-毕业论文
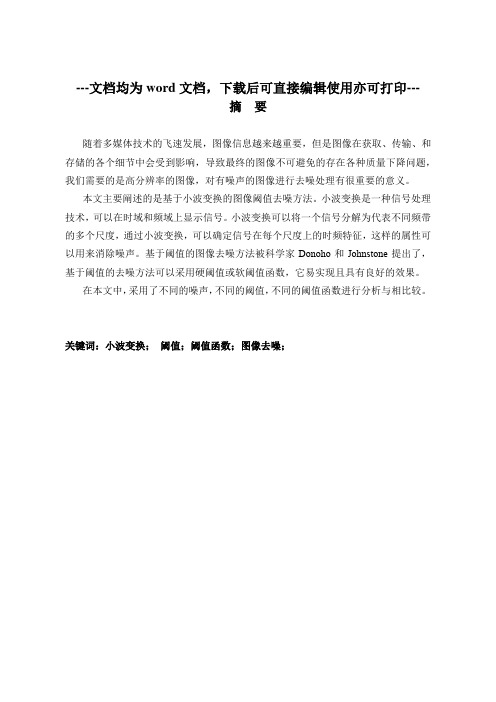
---文档均为word文档,下载后可直接编辑使用亦可打印---摘要随着多媒体技术的飞速发展,图像信息越来越重要,但是图像在获取、传输、和存储的各个细节中会受到影响,导致最终的图像不可避免的存在各种质量下降问题,我们需要的是高分辨率的图像,对有噪声的图像进行去噪处理有很重要的意义。
本文主要阐述的是基于小波变换的图像阈值去噪方法。
小波变换是一种信号处理技术,可以在时域和频域上显示信号。
小波变换可以将一个信号分解为代表不同频带的多个尺度,通过小波变换,可以确定信号在每个尺度上的时频特征,这样的属性可以用来消除噪声。
基于阈值的图像去噪方法被科学家Donoho和Johnstone提出了,基于阈值的去噪方法可以采用硬阈值或软阈值函数,它易实现且具有良好的效果。
在本文中,采用了不同的噪声,不同的阈值,不同的阈值函数进行分析与相比较。
关键词:小波变换;阈值;阈值函数;图像去噪;A b s t r a c tWith the rapid development of multimedia technology and network technology, image information becomes more and more important in people's work, study and life. But the image in the acquisition, transmission, and storage process sections will be affected seriously, which leads to the final image effected by all kinds of inevitable quality problems. but, which we need is the image with clearity and high resolution. Therefore, to deal with the noise of noisy images has very important meaning in practical application and life.There are a lot of methods for image de-noising. This paper mainly describes the image de-noising method based on wavelet transform. It is well known that wavelet transform is a signal processing technique which can display the signals on in both time and frequency domain. In this paper, we use several threshold based on wavelet transform to provide an enhanced approach for eliminating noise.Wavelet transforms can decompose a signal into several scales that represent different frequency band. The position of signal's instantaneous at each scale can be determined approximately by wavelet transform.Such a property can be used to denoise. Threshold-based de-noising method was proposed by Donoho. Threshold-based de-noising method is used hard-threshold or soft-threshold. It is very simple and has good performance. This paper uses the threshold techniques which applied threshold according to each band characteristic of image.In this paper, the results will be analyzed and compared for different noises, different thresholds, different threshold functions. It has a superior performance than traditional image de-noising method.Keyword:Wavelet Transform; Threshold; Threshold Function; Image De-noising第一章绪论1.1研究目的和意义当今各种信息充斥于我们的日常生活中,图像信息成为人类获取信息的重要信息,因为图像具有传输速度快,信息量大等一系列的强势[1]。
基于小波分析的图像去噪研究

文章 编 号 :079 1(0 0 .100 10 —4 62 1)80 2 —2 1
在图像 的采集和传输过程 中 , 常常受到 图像 采集设备和外 波基 条件 下对 图像去噪的仿真效果 比对 , 图像 在不 同的噪 声强度下 , 分 部噪声的干扰而降低质量 , 使得实 际的得到的数字图像包含 了噪声 解层数对 图像去噪效果 的影 响以及在选择不同的阈值条件下 , 对小 再对高低频系数重构 , 实现 图像的去噪 , 成分。 噪声 的存在 , 破坏 了图像 问在结构、 纹理和内容 等方 面的相关 波分解系数进行阈值量 化, 最后运用Malb t 仿真平 台对 图像信 号去噪进行仿真 效果 比对 。 a 性, 使得 图像失真 , 这些给 图像的理解带来困难 , 所以必须 首先对 图 像进行 消噪 , 以提高 图像 的质量 。
t1 0一
Hale Waihona Puke ( 4 ) 本 文是基于Malb ta 的二维 图像信号 的小 波降噪 , 运用小 波分 析 算法对 图像进行消 噪处理 。 以Ma a 提供的小波 工具 为基础 , l fb 利 用小波变换对二维含噪图像进 行小波多尺度分解 , 在选取不 同的小
I _ ■一
第 二层 嘲值处 理去 嗓图像 第三 层 阉值处理 去嗓 瞄像
重构公 式如式 ( ) 4 所示为 :
9, - Z,一 )-1( () Z , 2h 2c, e r n { m( 1 k k z) D j
+ Zg-m{ 2d( Z ( 2h n+. k ) - )。0 l  ̄k
+ ∑J一 )f 。D ∑ l 2譬一 嚷 m (2
图像去噪论文
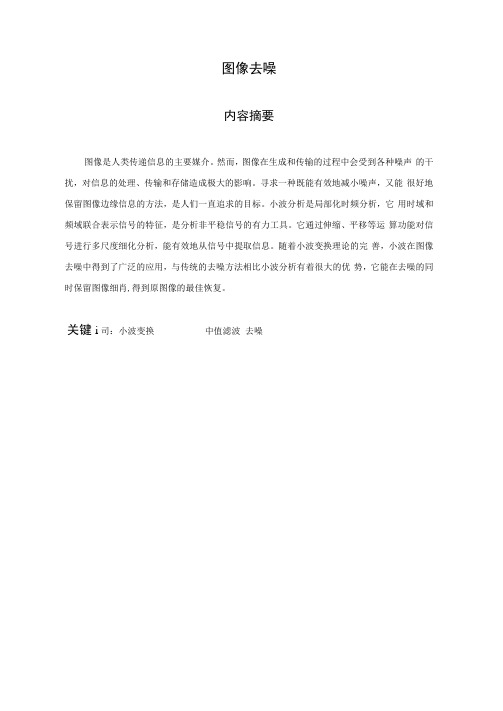
图像去噪内容摘要图像是人类传递信息的主要媒介。
然而,图像在生成和传输的过程中会受到各种噪声的干扰,对信息的处理、传输和存储造成极大的影响。
寻求一种既能有效地减小噪声,又能很好地保留图像边缘信息的方法,是人们一直追求的目标。
小波分析是局部化时频分析,它用时域和频域联合表示信号的特征,是分析非平稳信号的有力工具。
它通过伸缩、平移等运算功能对信号进行多尺度细化分析,能有效地从信号中提取信息。
随着小波变换理论的完善,小波在图像去噪中得到了广泛的应用,与传统的去噪方法相比小波分析有着很大的优势,它能在去噪的同时保留图像细肖,得到原图像的最佳恢复。
关键i司:小波变换中值滤波去噪序言 (1)—、小波分析 (1)(一)小波分析的发展 (1)(二) .......................................... 小波变换1(1) ............................................................................................ 小波函数2(2) ................................................................................. 小波函数的性质3(三)................................................. 均值滤波与中值滤波 (3)(1 )均值滤波 (3)(2)中值滤波 (3)二、数学基础 (4)(一) ...................................... 希尔伯特变换4(二) ........................................ 傅里叶变换5三、小波去噪与中值滤波去噪.. (6)(一)M A T L A B 介11绍 (6)(二)小波去噪与中值滤波去噪 (7)四、总结 (1)参考文献11图像是人类视觉的基础,给人具体而直观的作用。
《基于神经网络的图像去噪》论文

写一篇《基于神经网络的图像去噪》论文《基于神经网络的图像去噪》摘要:图像去噪是深度学习领域中一个具有挑战性的问题,主要目标是从受损图像中重建出有效信息,以恢复最高可能的图像质量。
本文所提出的图像去噪方法是基于深度神经网络(DNN)的,旨在提高图像去噪的性能,以获得更好的去噪效果。
我们提出使用DNN进行图像去噪的思想,该方法的基本流程是:(1)将输入图像预处理以获得用于训练的样本数据集;(2)训练深度神经网络用于恢复去噪后的图像;(3)将训练得出的网络应用于输入图像,得到去噪后的图像。
我们还提出使用自适应学习率来提高DNN的性能,并评估了两个常见的熵衡量指标,包括平均偏差和熵,两者都可以评估DNN的图像去噪性能。
实验结果表明,与传统图像去噪方法相比,所提出的DNN-based图像去噪方法可以获得更好的结果。
关键词:图像去噪;深度神经网络;自适应学习率;平均偏差;熵1. 引言近年来,随着深度学习(DL)技术的发展,图像去噪也变得越来越重要。
图像去噪的目的是从受损的输入图像中重建出有意义的信息,以恢复最高可能的图像质量。
目前,已经有许多研究工作提出了各种图像去噪方法来提高图像质量。
但是,大多数方法都不能在复杂环境下达到理想的图像去噪性能。
2. 相关工作传统的图像去噪方法基于图像处理、图像增强和统计图像处理技术,主要包括中值滤波、均值滤波等。
最近,越来越多的学者把深度学习(DL)的技术应用到图像去噪任务中,以改善传统图像处理技术的图像去噪性能。
例如,Xie等人使用卷积神经网络(CNN)来提高图像去噪的性能,并且取得了不错的结果[1]。
Guo等人提出了一种基于局部近邻搜索的自适应滤波器,用于图像去噪[2]。
3. 方法在本文中,我们提出一种基于深度神经网络(DNN)的图像去噪方法,以改善常规图像处理技术的图像去噪性能。
该方法的基本流程如下:(1)图像预处理,以获得用于训练的样本数据集;(2)训练深度神经网络,即训练网络以恢复去噪后的图像;(3)将训练得出的网络应用于输入图像,以获得去噪后的图像。
图像去噪毕业设计论文
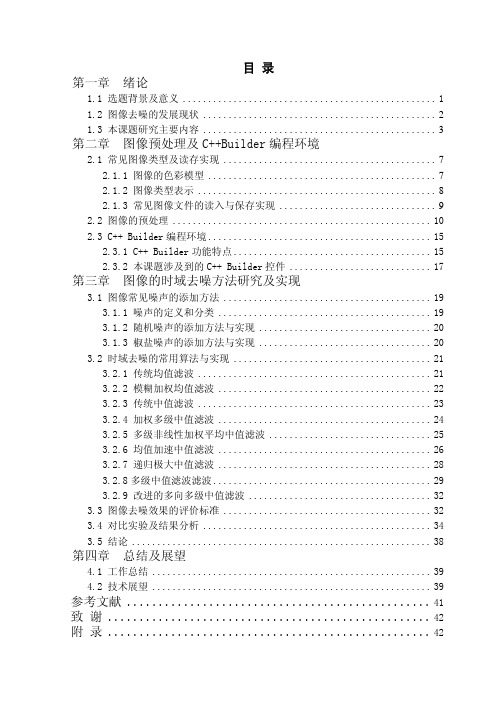
目 录第一章 绪论1.1 选题背景及意义 (1)1.2 图像去噪的发展现状 (2)1.3 本课题研究主要内容 (3)第二章 图像预处理及C++Builder编程环境2.1 常见图像类型及读存实现 (7)2.1.1 图像的色彩模型 (7)2.1.2 图像类型表示 (8)2.1.3 常见图像文件的读入与保存实现 (9)2.2 图像的预处理 (10)2.3 C++ Builder编程环境 (15)2.3.1 C++ Builder功能特点 (15)2.3.2 本课题涉及到的C++ Builder控件 (17)第三章 图像的时域去噪方法研究及实现3.1 图像常见噪声的添加方法 (19)3.1.1 噪声的定义和分类 (19)3.1.2 随机噪声的添加方法与实现 (20)3.1.3 椒盐噪声的添加方法与实现 (20)3.2 时域去噪的常用算法与实现 (21)3.2.1 传统均值滤波 (21)3.2.2 模糊加权均值滤波 (22)3.2.3 传统中值滤波 (23)3.2.4 加权多级中值滤波 (24)3.2.5 多级非线性加权平均中值滤波 (25)3.2.6 均值加速中值滤波 (26)3.2.7 递归极大中值滤波 (28)3.2.8多级中值滤波滤波 (29)3.2.9 改进的多向多级中值滤波 (32)3.3 图像去噪效果的评价标准 (32)3.4 对比实验及结果分析 (34)3.5 结论 (38)第四章 总结及展望4.1 工作总结 (39)4.2 技术展望 (39)参考文献 (41)致谢 (42)附 录 (42)第一章 绪 论1.1 选题背景及意义人类通过眼、耳、鼻、舌、身接受信息,感知世界,并进而认识世界和改造世界。
据统计,人类约有70%的信息是通过视觉系统获取的。
粗略地说,图像是二维或三维景物呈现在人心目中的影像。
如果接受并加工识别这种视觉信息的是电子计算机,则称之为计算机图像处理和识别。
近年来,由于计算机技术的迅猛发展,计算机的速度越来越快,图像处理系统的价格日益下降,从而使图像处理得以广泛应用于众多的科学与工程领域,如遥感、工业检测、医学、气象、侦察、通信、智能机器人等。
基于小波及其统计特性的图像去噪方法研究(精)
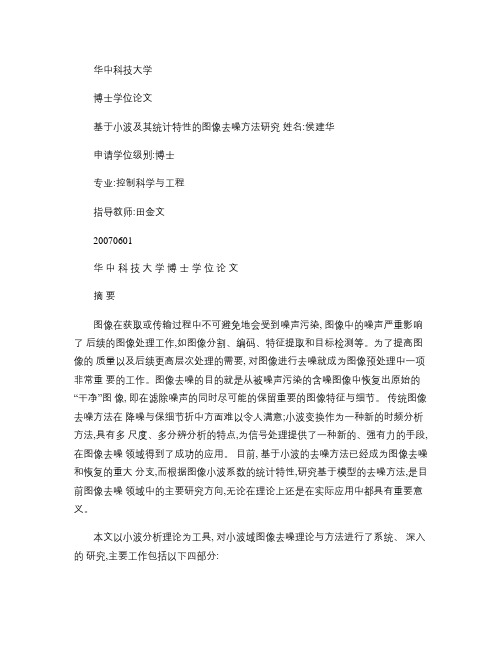
华中科技大学博士学位论文基于小波及其统计特性的图像去噪方法研究姓名:侯建华申请学位级别:博士专业:控制科学与工程指导教师:田金文20070601华中科技大学博士学位论文摘要图像在获取或传输过程中不可避免地会受到噪声污染, 图像中的噪声严重影响了后续的图像处理工作,如图像分割、编码、特征提取和目标检测等。
为了提高图像的质量以及后续更高层次处理的需要, 对图像进行去噪就成为图像预处理中一项非常重要的工作。
图像去噪的目的就是从被噪声污染的含噪图像中恢复出原始的“干净”图像, 即在滤除噪声的同时尽可能的保留重要的图像特征与细节。
传统图像去噪方法在降噪与保细节折中方面难以令人满意;小波变换作为一种新的时频分析方法,具有多尺度、多分辨分析的特点,为信号处理提供了一种新的、强有力的手段,在图像去噪领域得到了成功的应用。
目前, 基于小波的去噪方法已经成为图像去噪和恢复的重大分支,而根据图像小波系数的统计特性,研究基于模型的去噪方法,是目前图像去噪领域中的主要研究方向,无论在理论上还是在实际应用中都具有重要意义。
本文以小波分析理论为工具, 对小波域图像去噪理论与方法进行了系统、深入的研究,主要工作包括以下四部分:1、小波图像去噪方法研究综述本文前两章作为全文的基础,对基于小波的图像去噪方法进行了全面的研究总结。
首先综述了图像去噪技术的发展现状,特别是小波图像去噪方法的研究进展。
针对目前小波图像去噪领域尚未有一个较全面的分类方法, 本文以该领域发展的三个阶段为线索, 将小波图像去噪算法进行了新的分类并划分为四类, 并对每种类型中代表性的算法做了分析讨论。
阈值去噪是小波去噪研究中一类非常重要的方法, 对此进行了系统、深入的分析,在阈值选择这一核心问题上,对最具代表性的阈值结合具体的算法在原理与方法上做了清晰的阐述, 并对这些典型算法分别在正交小波变换基和平移不变小波基下进行了全面的实验仿真和分析讨论。
从实验结果和性能分析中得到了一些有意义的结论。
图像噪声分类及去噪方法综述论文.doc

图像噪声分类及去噪⽅法综述论⽂.doc图像噪声分类及去噪⽅法综述数字图像中,噪声主要来源于图像的获取或传输过程。
成像传感器的性能受各种因素的影响,如图像获取过程中的环境条件和传感元器件⾃⾝的质量。
例如,在使⽤CCD摄像机获取图像时,光照⽔平和传感器温度是影响结果图像中噪声数量的主要因素。
图像在传输中被污染主要是由于传输信道中的⼲扰。
例如,使⽤⽆线⽹络传输的图像可能会因为光照或其他⼤⽓因素⽽污染。
图像噪声的分类图像噪声是图像在摄取或传输时所受的随机信号⼲扰,是图像中各种妨碍⼈们对其信息接受的因素。
很多时候将图像噪声看成是多维随机过程,因⽽描述噪声的⽅法完全可以借⽤随机过程的描述,即⽤其概率分布函数和概率密度分布函数。
图像噪声是多种多样的,其性质也千差万别,所以了解噪声的分类是很有必要的。
⼀.按产⽣的原因分类1.外部噪声,即指系统外部⼲扰以电磁波或经电源串进系统内部⽽引起的噪声。
如电⽓设备,天体放电现象等引起的噪声。
2.内部噪声,⼀般有四个源头:a)由光和电的基本性质所引起的噪声。
如电流的产⽣是由电⼦或空⽳粒⼦的集合,定向运动所形成。
因这些粒⼦运动的随机性⽽形成的散粒噪声;导体中⾃由电⼦的⽆规则热运动所形成的热噪声;根据光的粒⼦性,图像是由光量⼦所传输,⽽光量⼦密度随时间和空间变化所形成的光量⼦噪声等。
b)电器的机械运动产⽣的噪声。
如各种接头因抖动引起电流变化所产⽣的噪声;磁头、磁带等抖动或⼀起的抖动等。
c)器材材料本⾝引起的噪声。
如正⽚和负⽚的表⾯颗粒性和磁带磁盘表⾯缺陷所产⽣的噪声。
随着材料科学的发展,这些噪声有望不断减少,但在⽬前来讲,还是不可避免的。
d)系统内部设备电路所引起的噪声。
如电源引⼊的交流噪声;偏转系统和箝位电路所引起的噪声等。
这种分类⽅法有助于理解噪声产⽣的源头,有助于对噪声位置定位,对于降噪算法只能起到原理上的帮助。
⼆.按噪声频谱分类频谱均匀分布的噪声称为⽩噪声;频谱与频率成反⽐的称为1/f噪声;⽽与频率平⽅成正⽐的称为三⾓噪声等等。
图像去噪算法的优化与改进研究
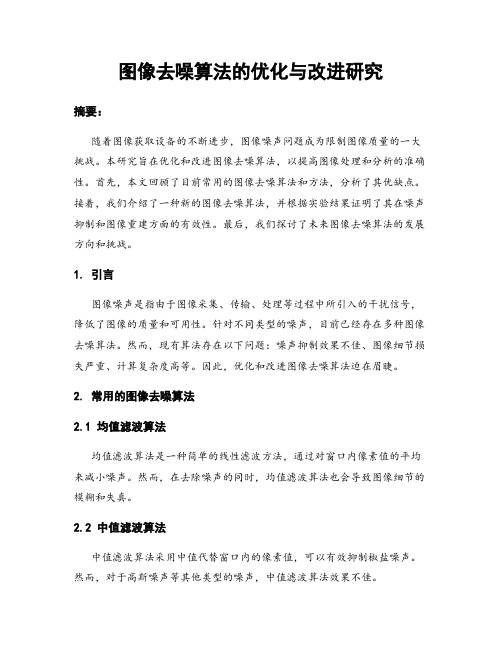
图像去噪算法的优化与改进研究摘要:随着图像获取设备的不断进步,图像噪声问题成为限制图像质量的一大挑战。
本研究旨在优化和改进图像去噪算法,以提高图像处理和分析的准确性。
首先,本文回顾了目前常用的图像去噪算法和方法,分析了其优缺点。
接着,我们介绍了一种新的图像去噪算法,并根据实验结果证明了其在噪声抑制和图像重建方面的有效性。
最后,我们探讨了未来图像去噪算法的发展方向和挑战。
1. 引言图像噪声是指由于图像采集、传输、处理等过程中所引入的干扰信号,降低了图像的质量和可用性。
针对不同类型的噪声,目前已经存在多种图像去噪算法。
然而,现有算法存在以下问题:噪声抑制效果不佳、图像细节损失严重、计算复杂度高等。
因此,优化和改进图像去噪算法迫在眉睫。
2. 常用的图像去噪算法2.1 均值滤波算法均值滤波算法是一种简单的线性滤波方法,通过对窗口内像素值的平均来减小噪声。
然而,在去除噪声的同时,均值滤波算法也会导致图像细节的模糊和失真。
2.2 中值滤波算法中值滤波算法采用中值代替窗口内的像素值,可以有效抑制椒盐噪声。
然而,对于高斯噪声等其他类型的噪声,中值滤波算法效果不佳。
2.3 小波去噪算法小波去噪算法是一种基于小波变换的非局部方法,其基本思想是通过将图像转换到频域,然后根据不同频率的系数进行阈值处理。
然而,小波去噪算法对图像细节的保护效果较差,对均匀噪声的去除效果也不理想。
3. 新的图像去噪算法本研究提出了一种基于深度学习的图像去噪算法,利用卷积神经网络(CNN)进行噪声模式的学习和噪声特征的提取。
具体而言,我们设计了一个多层的CNN网络,其中包括卷积层、池化层和全连接层。
通过训练大量的带有噪声的图像样本,我们的算法可以自动学习到噪声的特征,并将其应用于去噪处理。
4. 实验与结果分析我们使用了多个常见的图像数据集进行实验,将我们的新算法与现有的算法进行了比较。
实验结果表明,我们的算法在不同类型的噪声下均取得了良好的去噪效果,并成功保护了图像的细节。
毕业设计--基于双边滤波的图像去噪的方法
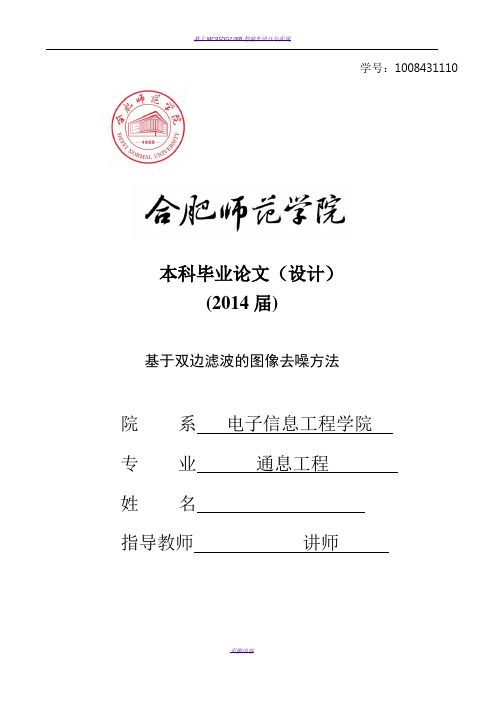
学号:1008431110本科毕业论文(设计)(2014届)基于双边滤波的图像去噪方法院系电子信息工程学院专业通息工程姓名指导教师讲师2014年4月摘要双边滤波是非线性的滤波方法,是结合图像的像素值相似度空间邻近度和空间领近度的一种折衷处理,同时考虑灰度相似性和空域信息,达到保边去噪的目的。
双边滤波具有简单、非迭代、局部的特点。
双边滤波器的好处是可以做边缘保存,一般过去用的维纳滤波或者高斯滤波去降噪,都会较明显地模糊边缘,对于高频细节的保护效果并不明显。
双边滤波比高斯滤波多了一个高斯方差,它是基于空间分布的高斯滤波函数,所以在边缘附近,离的较远的像素不会影响到边缘上的像素值,这样就保证了边缘附近像素值的保存。
但是由于保存了过多的高频信息对于彩色图像里的高频噪声,双边滤波器不能够彻底的滤掉,只能够对于低频信息进行较好的滤波。
其具体的操作方法有两个,第一个是高斯模版,用个模板对图像中的每一个像素值进行扫描,然后把某一点和其邻域内像素的加权平均值代替那一个中心的值高斯滤波器是根据高斯函数的形状来选择其权值的线性平滑滤波器,高斯滤波是线性平滑滤波的一种,最适合去除的噪声类型是服从正态分布的噪声。
第二个是以灰度级的差值作为函数系数生成的模板。
然后这两个模板点乘就得到了最终的双边滤波模板,最后得到双边滤波处理后的图像。
关键词:图像;去噪;双边滤波;高斯滤波AbstractThe bilateral filter is a nonlinear filtering method, is the combination of image pixel value similarity space proximity and space brought a compromise approach degree, considering the gray similarity and spatial information, to achieve the purpose of edge preserving denoising. The bilateral filter has the advantages of simple, non iterative, local. The bilateral filter is good to do edge preservation,generally used Wiener filtering or Gauss filter to denoise, will obviously fuzzy edge, for the protection of high frequency detail is not obvious. Bilateral filtering than Gauss filter has a Gauss variance, it is Gauss filter function based on the spatial distribution, so near the edge, the pixel will not affect the farther to the pixel on the edge of the value, thus ensuring the preservation of edge pixel values. But because of the high frequency information saved too much for the high frequency noise in the color image, the bilateral filter can not be completely filtered out, can only be better filtering for the low frequency information. The specific operation method has two, the first is Gauss template, scanning for each pixel in the image with a template, and then the weighted one point and its neighborhood pixels instead of the average value of a central value Gauss filters are linear smoothing filter to select the weights based on the Gauss function the shape, the Gauss filter is a linear smoothing filter for noise removal, the type is subject to normally distributed noise. The second is the difference of gray level as function coefficients generated templates. Then the two template dot get bilateral filtering template final, finally get the image after bilateral filtering.Key words: Image ;Denoising;Bilateral Filtering;Gauss Filtering目录摘要 (I)ABSTRACT (II)1 引言 (1)1.1 课题的研究背景及意义 (1)1.2 国内外研究现状 (1)1.3 图像噪声及图像去噪方法 (2)1.4 图像质量评价方法 (5)1.5论文研究目标及结构安排 (9)2 双边滤波理论 (7)2.1双边滤波定义 (7)2.2双边滤波器的设计 (7)3 图像去噪的方法 (9)3.1 中值滤波介绍 (9)3.2 高斯滤波介绍 (11)4 双边滤波实验结果 (17)4.1结果图片 (17)5 论文总结 (18)参考文献 (19)1 引言1.1 课题的研究背景及意义当今社会已经进入了一个高度信息化的阶段,人们对信息的需求越来越多。
数字图像处理论文,图像去噪
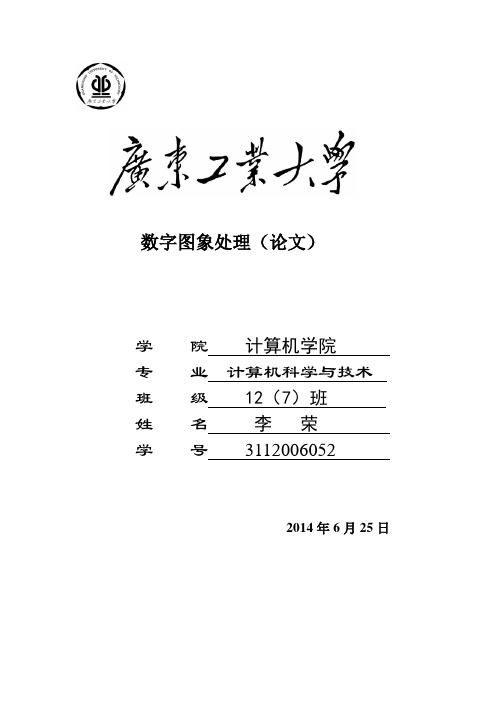
数字图象处理(论文)学院计算机学院专业计算机科学与技术班级 12(7)班姓名李荣学号**********2014年6月25日图像去噪算法论文图像在生成或传输过程中常常因受到各种噪声的干扰和影响而使图像爱那个的质量下降,对后续的图像处理(如分割、理解等)产生不利影响。
因此,图像爱那个去噪是图像处理中的一个重要环节。
而对图像去噪的方法又可以分为两类,一种是在空间域内对图像进行去噪,一种是将图像变换到频域进行去噪的处理。
一般数字图像系统中的常见噪声主要有高斯噪声和椒盐噪声,还有加性、乘性噪声等,如上,减少噪声的方法,可以在图像空间域或在图像频率域完成。
在空间域对图像处理主要有均值滤波算法和中值滤波算法。
图像频率域去噪方法是对图像进行某种变换,将图像从空间域转换到频率域,对频率域中的变换系数进行处理,再进行反变换将图像从频率域转换到空间域来达到去除图像噪声的目的。
将图像从空间转换到变换域的变换方法很多,常用的有傅立叶变换、小波变换等。
在这节课上我学习的是借助Matlab软件对图像进行处理。
在图像去噪方面,在Matlab 中常用的去噪函数有imfilter( ), wiener2( ), medfilt2( ), ordfilt2( )以及小波分析工具箱提供的wrcoef2( )和wpdencmp( )等,好像随着Matlab的发展,有些函数变了,不过早大致上变化不大,也有可能是我下载的Matlab不完整吧,总之在实践过程中有些错误让我很纠结。
因为我是刚接触到这类知识,所以很多都还不懂,虽然从课上有了一些了解,但我觉得还远远不够,然而最近实在时间不多,只能等以后再去详细的学习了。
我不敢说我以后会有多熟悉它,只能尽量,因为每一款出名的软件都有各自的市场,而我很难涉及到每个市场,只能当作业余去了解如今有这么一款什么软件可以做什么,如果在以后在这方面有需要时不至于两眼一抹黑。
现在我只能照搬看到的代码,观察比较各个算法对于图像去噪的效果。
(完整版)图像去噪算法研究毕业设计论文
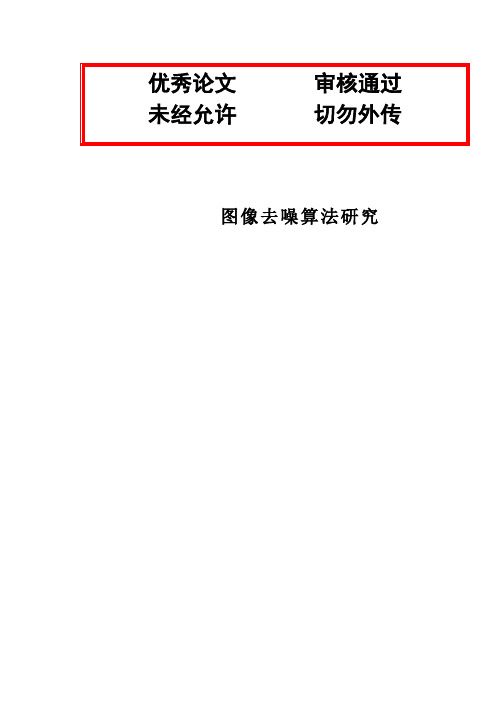
优秀论文审核通过未经允许切勿外传图像去噪算法研究摘要图像是一种重要的信息源,通过图像处理可以帮助人们了解信息的内涵。
数字图像噪声去除涉及光学系统、微电子技术、计算机科学、数学分析等领域,是一门综合性很强的边缘科学,如今其理论体系已十分完善,且其实践应用很广泛,在医学、军事、艺术、农业等都有广泛且成熟的应用。
MATLAB是一种高效的工程计算语言,在数值计算、数据处理、图像处理、神经网络、小波分析等方面都有广泛的应用。
MATLAB是一种向量语言,它非常适合于进行图像处理。
本文概述了小波阈值去噪的基本原理。
对常用的几种阈值去噪方法进行了分析比较和仿真实现。
最后结合理论分析和实验结果,讨论了一个完整去噪算法中影响去噪性能的各种因素。
为实际的图像处理中,小波阈值去噪法的选择和改进提供了数据参考和依据。
关键字:小波变换;图像去噪;阈值;MATLABAbstractImage is one kind of important information source, may the connotation. The digital image de-noise involves domains and so on optical system, microelectronic technology, computer science,mathematical analysis, it’s a very comprehensive interdisciplinary science, now its practice application is very widespread. In the medicine, the military, art, the agriculture and all . MATLAB is one kind of language, in aspects and so on value computation, data processing, imagery processing, neural network, wavelet analysis all .This article done comparing experiments using several good threshold denoising methods. Finally according to the theory analysis and simulation results, the paper discusses several kinds of factors which affect the denoising capability in a complete denoisingalgorithm. That provides the date reference of threshold denoising methods in actual image process.Keywords: Wavelet transformation; Image denoising; Wavelet threshold; MATLAB目录第一章绪论 .............................................................................................................1.1引言 .......................................................................................................................1.2数字图像的基本概念...........................................................................................1.3数字图像处理的基本理论...................................................................................1.4问题的产生 ...........................................................................................................1.5文各章节的安排...................................................................................................第二章图像去噪基本方法研究...................................................................................2.1图像噪声的基本概念...........................................................................................2.2图像去噪方法基本方法.......................................................................................2.2.1 均值滤波 ........................................................................................................2.2.2 中值滤波 ........................................................................................................2.3实验结果 ...............................................................................................................2.3.1 均值滤波 ........................................................................................................2.3.2 中值滤波 ........................................................................................................第三章小波变换的图像去噪 .......................................................................................3.1小波变换 ...............................................................................................................3.2小波去噪问题的描述...........................................................................................3.3小波变换的图像去噪原理...................................................................................3.4阈值的选取 ...........................................................................................................3.5小波去噪基于MATLAB的实现............................................................................3.6实验结果 ...............................................................................................................3.7几种算法的比较...................................................................................................第四章总结与展望 .......................................................................................................参考文献 .........................................................................................................................致谢 ...........................................................................................................................第一章绪论1.1引言近些年来,随着数码产品及各类数字产品的普及,数字图像处理已成为数学技术和计算机技术交叉领域的一个研究热点。
图像去噪去噪算法研究论文开题报告

图像去噪去噪算法研究论文开题报告(1)选题的目的、意义目的:由于成像系统、传输介质和记录设备等的不完善,数字图像在其形成、传输记录过程中往往会受到多种噪声的污染,影响了图像的视觉效果,甚至妨碍了人们正常识别。
另外,在图像处理的某些环节当输入的对象并不如预想时也会在结果图像中引入噪声。
这些噪声在图像上常表现为—引起较强视觉效果的孤立象素点或象素块[1]。
一般,噪声信号与要研究的对象不相关它以无用的信息形式出现,扰乱图像的可观测信息。
要构造一种有效抑制噪声的滤波必须考虑两个基本问题能有效地去除目标和背景中的噪声;同时,也要能很好的保护图像目标的形状、大小及特定的几何和拓扑结构特征。
意义:噪声的污染直接影响着对图像边缘检测、特征提取、图像分割、模式识别等处理,使人们不得不从各种角度进行探索以提高图像的质量[2] [3]。
所以采用适当的方法尽量消除噪声是图像处理中一个非常重要的预处理步骤。
现在图像处理技术已深入到科学研究、军事技术、工农业生产、医学、气象及天文学等领域。
科学家利用人造卫星可以获得地球资源照片、气象情况;医生可以通过X射线或C T对人体各部位的断层图像进行分析。
但在许多情况下图像信息会受到各种各样噪声的影响,严重时会影响图像中的有用信息,所以对图像的噪声处理就显得十分重要[4] [5]。
图像去噪作为图像处理的一个重要环节,可以帮助人们更加准确地获得我们所需的图像特征,使其应用到各个研究领域,帮助解决医学、物理、航天、文字等具体问题。
如何改进图像去噪算法,以有效地降低噪声对原始图像的干扰程度,并且增强视觉效果,提高图像质量,使图像更逼真,仍存在继续研究的重要意义。
(2)国内外对本课题涉及问题的研究现状针对图像去噪的经典算法,科学工作者通过努力,提出了一些的改进算法,比如模拟退火法[6]。
图像去噪 SCI论文
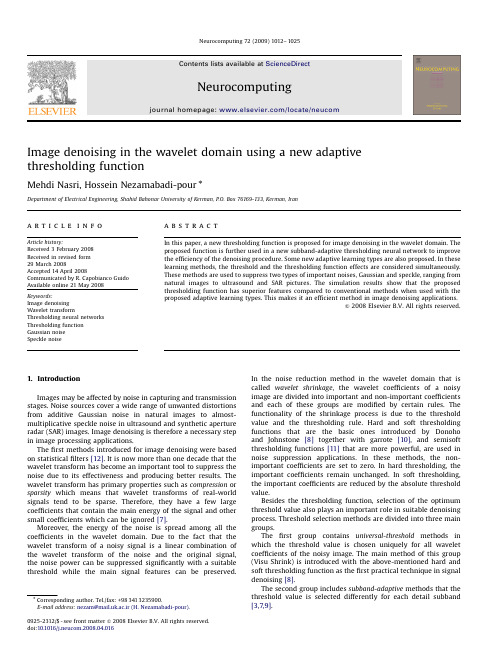
article info
Article history: Received 3 February 2008 Received in revised form 29 March 2008 Accepted 14 April 2008 Communicated by R. Capobianco Guido Available online 21 May 2008
The second group includes subband-adaptive methods that the threshold value is selected differently for each detail subband [3,7,9].
ARTICLE IN PRESS
M. Nasri, H. Nezamabadi-pour / Neurocomputing 72 (2009) 1012–1025
The first methods introduced for image denoising were based on statistical filters [12]. It is now more than one decade that the wavelet transform has become an important tool to suppress the noise due to its effectiveness and producing better results. The wavelet transform has primary properties such as compression or sparsity which means that wavelet transforms of real-world signals tend to be sparse. Therefore, they have a few large coefficients that contain the main energy of the signal and other small coefficients which can be ignored [7].
- 1、下载文档前请自行甄别文档内容的完整性,平台不提供额外的编辑、内容补充、找答案等附加服务。
- 2、"仅部分预览"的文档,不可在线预览部分如存在完整性等问题,可反馈申请退款(可完整预览的文档不适用该条件!)。
- 3、如文档侵犯您的权益,请联系客服反馈,我们会尽快为您处理(人工客服工作时间:9:00-18:30)。
ABSTRACT
The paper addresses the problem of image denoising using adaptive wavelet transfoniis. Two scale-adaptive versions o f the wavelet transform are implemented and experimentally tested: the classical and the lifting one. In both o f them exemplary test images are contaminated with noise, decomposed into several multiresolution levels, modified via soft thresholding (only detail subimages) and inversely synthesized. On each level the LL image from the previous stage is decomposed into LL, LH, HL and HH subimages using different wavelet or predicdupdate lifting filters (L-lowpass, H-highpass). At each level as the best filters are chosen such ones, for which the normalized energy o f the detail HH subimage is the lowest. Image denoising is realized by soft thresholding o f all detail HH subimages only. Several test images are processed in the paper. Explicit equations for the design of lifting filters are presented.
-
+ 2Nd +3)+ = 1;
N=l
Nd=O, N 2 2
0lNdlN-2
பைடு நூலகம்
(6)
n = l,2,...,K
calculation of filtersp'M.') and
y ( M . M ) (M.1) = g(M.1)
itN. from: I'
(7)
(8)
- 1 : g ( n )= (-l)"+lh(n);
IMAGE DENOISING USING SCALE-ADAPTIVE LIFTING SCHEMES
Jacek Stepien”, Tomasz ZieZinski*’, Roman Rumian”
’ ) Department of Electronics, *) Department of lnstrumentation and Measurement University of Mining and Metallurgy, al. Mickiewicza 30, 30-059 Krakbw, Poland tel: +(4812) 6172700, fax: +(4812) 6338565, e-mail: stepien@.pl
2. DESIGN OF LIFTING FILTERS
In this section we will extend results presented in [2, 61 and propose the explicit solution for the update filter U(.) as well as equations for the corresponding wavelet transform filters. Different choice o f parameters M, h f d , N and Nd leads to different pairs of lifting filters.
2.1 ,,Predict first” version of the forward lifting
Msamples long predict filterp‘M.I):
288
0-7803-6297-7/00/$10.0002000 IEEE
2.3 Corresponding wavelet transform filters
Let us denote the high-pass and low-pass analysis filters of the wavelet transform as g ( n ) and h(n), and the dual synthesis filters as g ( n ) and z ( t 7 ) . After the choice of lifting filters P(.) and U(.) the corresponding wavelet ones can be easily found from the tree-like signal flow diagrams presented in figure 2 or from the following equations:
updated on the base of d(n) and a smoothed signal approximation c(n) is obtained. Filters P(.) and U(.) can be chosen arbitrary. They can be even non-linear (e.g. median) and data-adaptive. Since the signal is reconstructed exactly in reversed order (see fig. I), the transform is always invertable. When the update filter is followed by the predict filter, the ”update-first” lifting scheme is get. Situation wHen both filters are linear has been discussed in detail in [3+4]. Some fruitful graphical illustrations and constraints for filters P(.) and U(.) have been derived and presented there. In particular, relations which should fulfil the filters P ( . ) and U(.) have been given. They assure exact decomposition of the signal x(n) into its low-pass and high-pass components (in figure 1 c(n) and d(n), respectively). In the lifting implementation of the wavelet transform standard high-pass and low-pass decomposition filters g(n) and h(n), respectively, are replaced with the predict and update filters P( .) and U( .) (figure 2). The design problem o f adaptive and non-linear ,,lifted wavelet transforms has been already discussed in literature [3+7]. It has been suggested there that in case of linear filters P(.) and U(.) the length of prediction one can change according to signal characteristics. For instance, the prediction filter should become shorter before and after abrupt change of data. With the help of the presented procedure one can design several pairs of predict & update filters and switch them according to the nature of decomposed data.
n=1,2, ...,2 M - 1 :
n = l,2,...,M
g(n)=O; g ( 2 + 2 M d ) = I ;
g ( 2 n - 1) = - p ( n ) ;
h ( K )= C ( K ' N,)u ( ~ " ) ;h(2Md
n=1,2, ...,2 M - 1 :
h(n)=(-l)"g(n); h"(2+2Md)=1;
1. INTRODUCTION
Second-generation wavelet transforms have been introduced recently [l]. They are obtained using the socalled lifting construction. Lifting implementations of wavelet transform offers several advantages in comparison to the traditional filter bank approach, e.g. faster, in-place calculations and possibility o f designing adaptive, nonlinear, irregular-sampled and integer-to-integer wavelet transforms. It has been shown that any wavelet transfonn can be factored into lifting steps [2]. Block diagrams of the direct and inverse lifting schemes are presented in figure 1. The analyzed signal x(n) is first split into even and odd samples: x,(n) and odd xo(n). Then the odd samples are predicted from the even ones using any function, in particular a linear one. Predicted values are subtracted from x,(n). The resultant signal d(n) represents high-frequency content of x(n) - the so-called detail signal. Finally, the even samples xe(n) are