剩余容量估算的方法锂离子电池
修正参数的安时法估算锂离子电池剩余电量
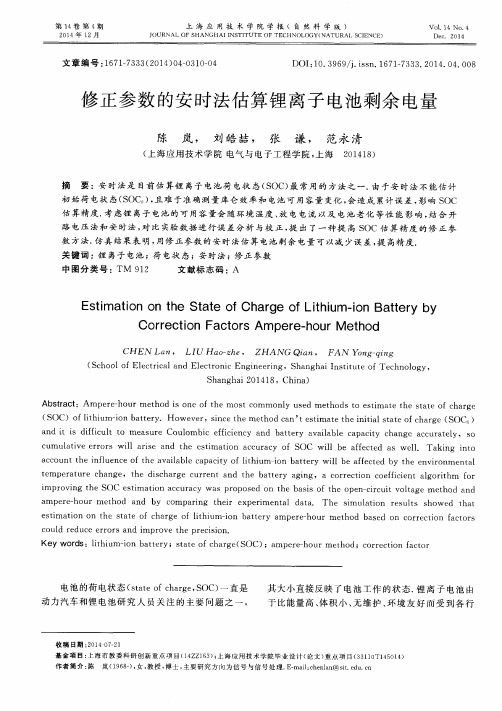
2 0 1 4年 1 2月
上 海 应 用 技 术 学 院 学 报 (自 然 科 学 版 )
J OURNAI OF S H ANGH AI I NS TI TUTE OF TE CH NOL OGY( NAT URAl S CI E NCE )
V0 L1 4 NO . 4
.
so
c umul a t i v e e r r or s wi l l a r i s e a nd t he e s t i ma t i o n a c c u r a c y o f SOC wi l l be a f f e c t e d a s we l 1 Ta ki n g i n t o a c c ou nt t h e i nf l u e n c e o f t he a v a i l a bl e c a pa c i t y of l i t h i um— i o n b a t t e r y wi l l be a f f e c t e d by t he e nv i r o nme nt a 1 t e mpe r a t u r e c ha ng e,t he di s c ha r g e c ur r e n t a nd t he ba t t e r y a gi ng,a c o r r e c t i on c oe f f i c i e nt a l g or i t hm f o r i mp r ov i n g t he SOC e s t i ma t i o n a c c u r a c y wa s p r op os e d o n t he ba s i s of t he o pe n — c i r c ui t vo l t a ge me t ho d a nd a mpe r e — h ou r me t ho d a n d by c o mp a r i ng t h e i r e x pe r i me nt a l da t a
锂离子电池组的SOC估计
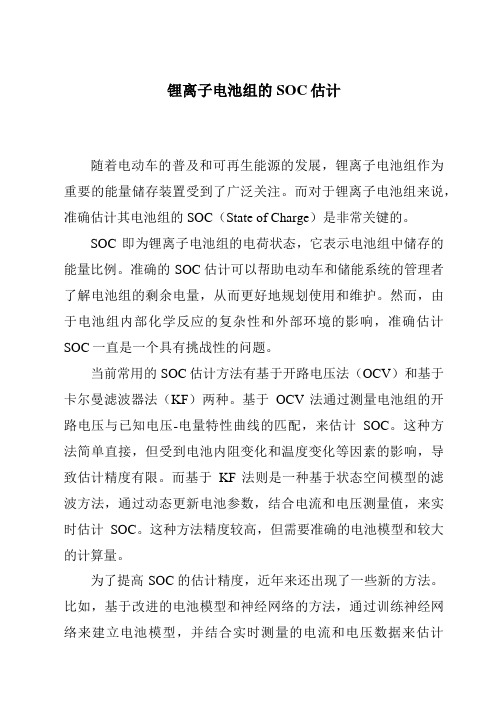
锂离子电池组的SOC估计随着电动车的普及和可再生能源的发展,锂离子电池组作为重要的能量储存装置受到了广泛关注。
而对于锂离子电池组来说,准确估计其电池组的SOC(State of Charge)是非常关键的。
SOC即为锂离子电池组的电荷状态,它表示电池组中储存的能量比例。
准确的SOC估计可以帮助电动车和储能系统的管理者了解电池组的剩余电量,从而更好地规划使用和维护。
然而,由于电池组内部化学反应的复杂性和外部环境的影响,准确估计SOC一直是一个具有挑战性的问题。
当前常用的SOC估计方法有基于开路电压法(OCV)和基于卡尔曼滤波器法(KF)两种。
基于OCV法通过测量电池组的开路电压与已知电压-电量特性曲线的匹配,来估计SOC。
这种方法简单直接,但受到电池内阻变化和温度变化等因素的影响,导致估计精度有限。
而基于KF法则是一种基于状态空间模型的滤波方法,通过动态更新电池参数,结合电流和电压测量值,来实时估计SOC。
这种方法精度较高,但需要准确的电池模型和较大的计算量。
为了提高SOC的估计精度,近年来还出现了一些新的方法。
比如,基于改进的电池模型和神经网络的方法,通过训练神经网络来建立电池模型,并结合实时测量的电流和电压数据来估计SOC。
这种方法能够在一定程度上克服电池模型不准确的问题,提高估计精度。
另外,还有基于电池内阻和电流功率的方法,通过测量电池内阻和电流功率的变化,来推测SOC的变化。
这种方法简单有效,但需要准确的传感器和较大的计算量。
综上所述,锂离子电池组的SOC估计是一个复杂而重要的问题,目前存在多种估计方法。
随着技术的发展和研究的深入,相信未来会出现更加准确和高效的SOC估计方法,为电动车和储能系统的管理者提供更好的支持。
锂离子电池荷电状态估算方案
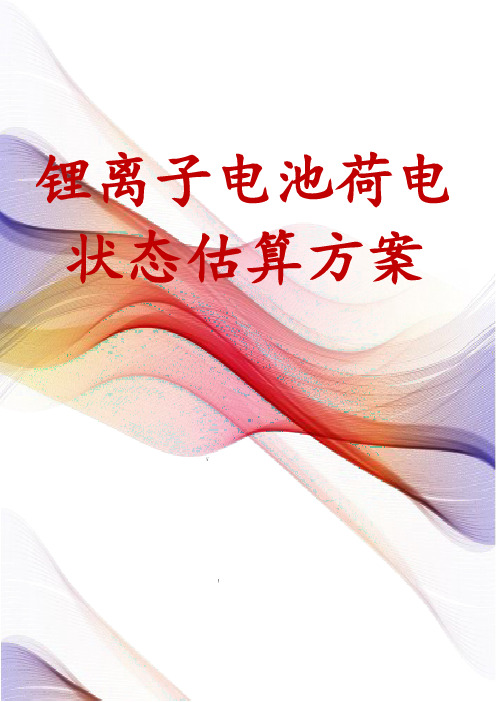
锂离子电池荷电状态估算方案锂离子电池荷电状态估算方案锂离子电池是目前最常见的电池类型之一,广泛应用于移动设备、电动车辆和可再生能源储存等领域。
为了正确估算锂离子电池的荷电状态,我们可以按照以下步骤进行思考。
第一步:确定锂离子电池的容量锂离子电池的容量是指在特定条件下电池可以存储的电荷量。
一般以安时(Ah)为单位进行表示。
通过查看电池的标识或使用特定仪器,我们可以确定电池的容量。
第二步:了解电池的初始荷电状态电池的初始荷电状态指的是在使用前电池的剩余电荷量。
可以通过电池管理系统或特定仪器读取电池的电量百分比来了解电池的初始荷电状态。
第三步:跟踪电池的充放电过程在使用过程中,我们可以通过跟踪电池的充放电过程来估算电池的荷电状态。
当电池充电时,电能被转化为化学能存储在电池内部,电池的荷电状态会增加;当电池放电时,化学能被转化为电能供电使用,电池的荷电状态会减少。
第四步:使用电池荷电状态算法进行估算根据电池的充放电特性,我们可以使用一些算法来估算电池的荷电状态。
常见的算法包括开路电压法、库仑计数法和卡尔曼滤波法等。
这些算法通过根据电池的电压、电流和时间等参数,计算出电池的荷电状态。
第五步:考虑电池的老化和环境因素锂离子电池在使用过程中会逐渐老化,导致其容量减少和荷电状态估算的不准确性增加。
此外,温度变化也会对电池的荷电状态估算产生影响。
因此,在进行荷电状态估算时,需要考虑电池的老化和环境因素对估算结果的影响。
综上所述,锂离子电池荷电状态估算包括确定容量、了解初始荷电状态、跟踪充放电过程、使用估算算法和考虑老化和环境因素等步骤。
通过正确估算锂离子电池的荷电状态,我们可以更好地管理和使用电池,延长其使用寿命并确保电池的可靠性和安全性。
电池剩余容量计算公式

电池剩余容量计算公式好的,以下是为您生成的文章:嘿,说起电池剩余容量计算公式,这可真是个有点复杂但又特别实用的玩意儿。
先给您讲讲我自己的一次经历吧。
有一回,我出门去露营,带了一堆电子设备,像手电筒、充电宝啥的。
晚上的时候,手电筒突然不太亮了,我心里就犯嘀咕,这电池到底还剩多少电啊?要是没电了,这黑灯瞎火的可咋办?这时候我就特别希望能有个准确的电池剩余容量计算公式来帮我搞清楚状况。
要弄明白电池剩余容量计算公式,咱们得先了解一些基本的概念。
电池的容量呢,通常用安时(Ah)或者毫安时(mAh)来表示,这就好比是一个大水桶能装多少水一样。
而电池剩余容量,就是这个“水桶”里还剩下多少“水”。
常见的电池剩余容量计算公式,会考虑电池的初始容量、放电电流、放电时间以及电池的内阻等因素。
比如说,对于一个简单的铅酸电池,我们可以用下面这个公式来估算剩余容量:剩余容量 = 初始容量 - 放电电流 ×放电时间 ×容量修正系数。
这里面的容量修正系数,就像是一个“调节小助手”,因为不同的电池在不同的使用条件下,性能会有所变化。
拿咱们手机里常用的锂离子电池来说,情况就稍微复杂一点啦。
它的剩余容量计算可能会涉及到电池的开路电压、温度、充放电循环次数等。
比如说,通过测量电池的开路电压,然后对照一个电压-容量的对应表格,就能大致估算出剩余容量。
但要注意哦,这个方法不是特别精确,因为电池的特性会随着使用时间慢慢变化。
在实际应用中,很多电子设备会内置一些智能的电池管理系统,它们通过复杂的算法和传感器来实时监测电池的状态,给咱们提供比较准确的剩余容量信息。
就像我那回露营,如果我的手电筒有这么个智能系统,我就不用那么心慌啦。
不过,这些计算公式和方法也不是万能的。
电池的实际使用情况会受到很多因素的影响,比如大电流放电会让电池容量下降得更快,低温环境会让电池性能变差等等。
所以,在使用电池的时候,咱们还是得心里有点数,别等到电池彻底没电了才着急。
三元电池剩余寿命的估算

三元电池剩余寿命的估算
三元电池是目前应用广泛的锂离子电池之一,其具有高能量密度、长寿命等优点,被广泛应用于电动汽车、储能系统、移动电源等领域。
然而,随着使用时间的增加,三元电池的容量和性能会逐渐下降,影响其使用寿命和性能。
因此,准确估算三元电池的剩余寿命非常重要。
目前,三元电池剩余寿命的估算方法主要有两种:一种是基于容量衰减的估算方法,另一种是基于内阻变化的估算方法。
容量衰减估算方法是通过对电池容量进行检测和分析来估算其
剩余寿命。
一般采用充电-放电测试法,即对电池进行一系列充放电
循环测试,通过对充放电数据进行分析,得出电池容量的变化曲线,通过对比变化曲线和标准曲线,可以估算电池的剩余寿命。
内阻变化估算方法是通过对电池内阻进行检测和分析来估算其
剩余寿命。
一般采用交流阻抗测试法,即对电池进行一系列小信号交流电测试,通过对测试数据进行分析,得出电池内阻的变化曲线,通过对比变化曲线和标准曲线,可以估算电池的剩余寿命。
总的来说,三元电池剩余寿命的估算方法需要综合考虑多个因素,如电池使用环境、充放电条件、内部结构等,同时需要对电池进行多次测试和分析,才能得出较为准确的估算结果。
- 1 -。
锂离子动力电池剩余容量估计算法研究与实现
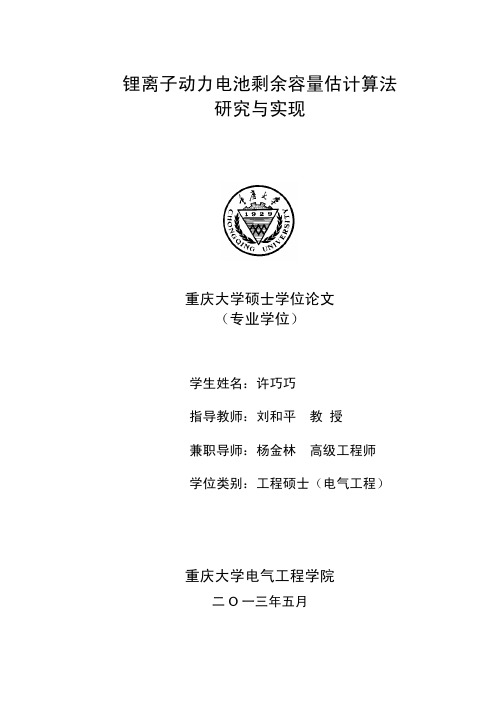
重庆大学硕士学位论文
英文摘要
4. The estimating performance of adaptive extended Kalman filter algorithm combined with battery two order equivalent circuit model to estimate SOC is perfect, so that it can satisfy engineering application requirement. On the basis of battery management and monitoring system of changed pure EVs, the SOC estimating algorithm was verified through vehicle road test. The battery management system was used to collect battery voltage, current, temperature and estimating SOC and the monitoring system was used to display the battery’s information as well as record historical data. Through the CAN bus, the battery management system and the monitoring system can exchange information. On the basis of the structural programming thought, software flow charts were designed. At last vehicle road tests were carried out to verify the practicable of the SOC estimation method. The simulation and vehicle road tests results show that the SOC estimation method in the paper can accurately estimate SOC, which can satisfy the national standard on SOC estimation of power batteries used on EVs and has guiding significance for engineering application. Keywords: Lithium Iron Phosphate Power Battery, SOC, online parameter identification, adaptive extended Kalman filter, vehicle test
如何算锂电池电量计算公式
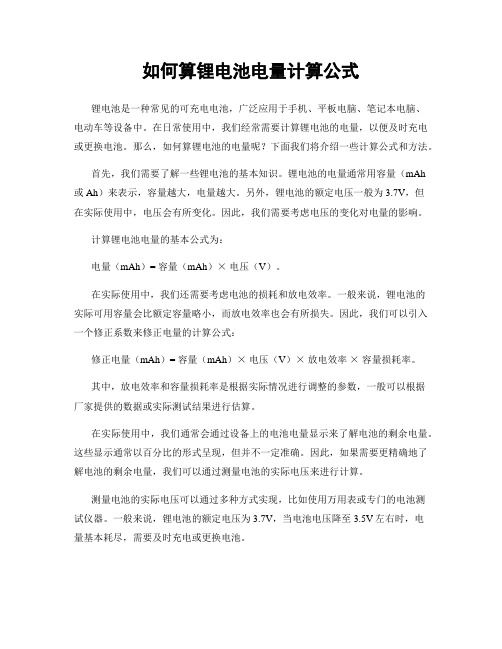
如何算锂电池电量计算公式锂电池是一种常见的可充电电池,广泛应用于手机、平板电脑、笔记本电脑、电动车等设备中。
在日常使用中,我们经常需要计算锂电池的电量,以便及时充电或更换电池。
那么,如何算锂电池的电量呢?下面我们将介绍一些计算公式和方法。
首先,我们需要了解一些锂电池的基本知识。
锂电池的电量通常用容量(mAh或Ah)来表示,容量越大,电量越大。
另外,锂电池的额定电压一般为3.7V,但在实际使用中,电压会有所变化。
因此,我们需要考虑电压的变化对电量的影响。
计算锂电池电量的基本公式为:电量(mAh)= 容量(mAh)×电压(V)。
在实际使用中,我们还需要考虑电池的损耗和放电效率。
一般来说,锂电池的实际可用容量会比额定容量略小,而放电效率也会有所损失。
因此,我们可以引入一个修正系数来修正电量的计算公式:修正电量(mAh)= 容量(mAh)×电压(V)×放电效率×容量损耗率。
其中,放电效率和容量损耗率是根据实际情况进行调整的参数,一般可以根据厂家提供的数据或实际测试结果进行估算。
在实际使用中,我们通常会通过设备上的电池电量显示来了解电池的剩余电量。
这些显示通常以百分比的形式呈现,但并不一定准确。
因此,如果需要更精确地了解电池的剩余电量,我们可以通过测量电池的实际电压来进行计算。
测量电池的实际电压可以通过多种方式实现,比如使用万用表或专门的电池测试仪器。
一般来说,锂电池的额定电压为3.7V,当电池电压降至3.5V左右时,电量基本耗尽,需要及时充电或更换电池。
通过测量电池的实际电压和使用上述的修正公式,我们可以比较准确地计算出电池的剩余电量。
当然,这种方法需要一定的专业知识和工具,不适合普通用户进行。
因此,对于普通用户来说,最简单的方法还是通过设备上的电池电量显示来了解电池的剩余电量。
总的来说,计算锂电池的电量并不复杂,但需要考虑多种因素的影响,包括容量、电压、放电效率和容量损耗率等。
锂电池剩余寿命预测方法
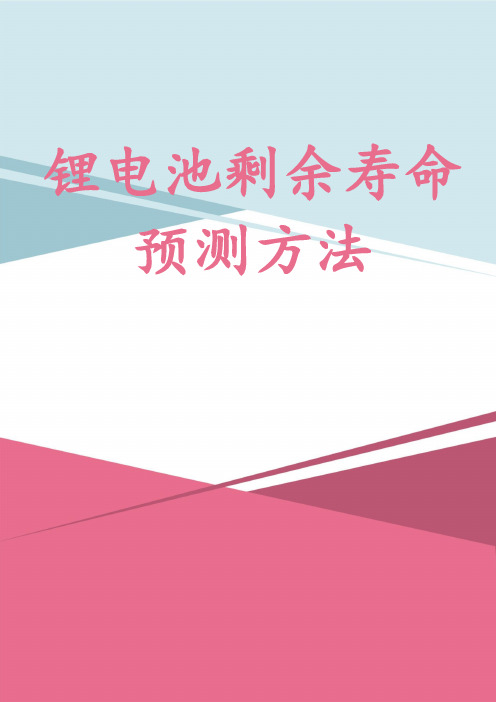
锂电池剩余寿命预测方法锂电池剩余寿命预测方法锂电池是一种常见的电池类型,被广泛应用于移动设备、电动车辆和储能系统等领域。
然而,随着使用时间的增加,锂电池的容量和性能会逐渐降低,最终导致电池寿命结束。
因此,预测锂电池的剩余寿命对于有效管理和维护电池至关重要。
以下是一种基于步骤思考的锂电池剩余寿命预测方法:第一步:数据收集首先,需要收集关于锂电池的历史运行数据。
这些数据可以包括电池的容量、充放电循环次数、充电速率、温度等信息。
此外,还应记录电池在实际使用环境中的运行状态和工作负载。
第二步:特征提取基于收集的数据,需要提取有意义的特征。
常用的特征包括电池容量衰减速率、充放电效率、内阻变化等。
这些特征可以通过统计分析或数据挖掘技术提取出来。
第三步:模型选择根据提取的特征,选择适合的预测模型。
常见的模型包括支持向量机、随机森林、神经网络等。
选择合适的模型需要考虑数据特点、模型复杂度和预测准确性等因素。
第四步:数据划分和训练将收集到的数据划分为训练集和测试集。
通常,将大部分数据用于训练模型,剩余的数据用于测试模型的准确性。
训练模型时,可以使用交叉验证和网格搜索等技术来选择最佳的超参数。
第五步:模型评估和优化使用测试集评估训练好的模型的性能。
通常使用常见的评估指标,如均方根误差(RMSE)、平均绝对百分比误差(MAPE)等。
如果模型表现不佳,则需要进一步优化模型,例如调整模型参数、增加特征数量等。
第六步:剩余寿命预测在经过训练和优化的模型上,输入当前的电池状态和特征数据,进行剩余寿命的预测。
预测的结果可以以时间或循环次数的形式呈现。
第七步:结果解释和应用根据预测结果,对电池的剩余寿命进行解释和分析。
如果剩余寿命较短,可能需要对电池进行维护或更换。
如果剩余寿命较长,可以采取相应的措施延长电池的使用寿命。
总结起来,通过以上步骤,我们可以根据锂电池的历史运行数据和特征,选择合适的模型来预测电池的剩余寿命。
这种方法可以帮助用户有效管理和维护锂电池,提前做出决策,避免因电池损坏而造成的不便。
怎样估计锂电池剩余电量SOC
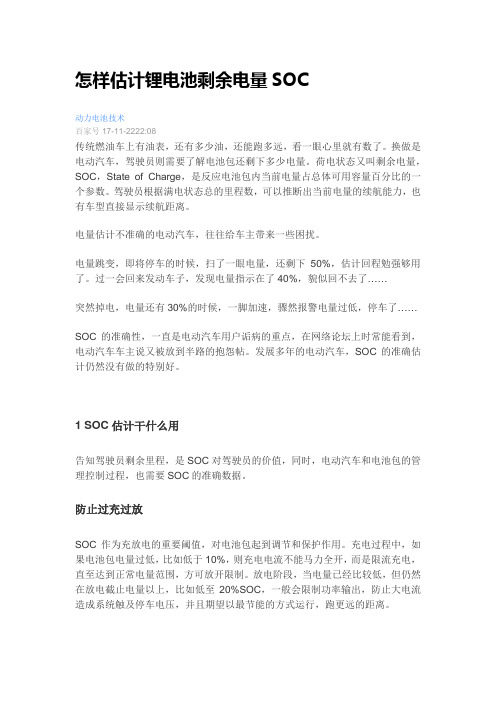
怎样估计锂电池剩余电量SOC动力电池技术百家号17-11-2222:08传统燃油车上有油表,还有多少油,还能跑多远,看一眼心里就有数了。
换做是电动汽车,驾驶员则需要了解电池包还剩下多少电量。
荷电状态又叫剩余电量,SOC,State of Charge,是反应电池包内当前电量占总体可用容量百分比的一个参数。
驾驶员根据满电状态总的里程数,可以推断出当前电量的续航能力,也有车型直接显示续航距离。
电量估计不准确的电动汽车,往往给车主带来一些困扰。
电量跳变,即将停车的时候,扫了一眼电量,还剩下50%,估计回程勉强够用了。
过一会回来发动车子,发现电量指示在了40%,貌似回不去了……突然掉电,电量还有30%的时候,一脚加速,骤然报警电量过低,停车了……SOC的准确性,一直是电动汽车用户诟病的重点,在网络论坛上时常能看到,电动汽车车主说又被放到半路的抱怨帖。
发展多年的电动汽车,SOC的准确估计仍然没有做的特别好。
1 SOC估计干什么用告知驾驶员剩余里程,是SOC对驾驶员的价值,同时,电动汽车和电池包的管理控制过程,也需要SOC的准确数据。
防止过充过放SOC作为充放电的重要阈值,对电池包起到调节和保护作用。
充电过程中,如果电池包电量过低,比如低于10%,则充电电流不能马力全开,而是限流充电,直至达到正常电量范围,方可放开限制。
放电阶段,当电量已经比较低,但仍然在放电截止电量以上,比如低至20%SOC,一般会限制功率输出,防止大电流造成系统触及停车电压,并且期望以最节能的方式运行,跑更远的距离。
作为低电量限流阈值为什么电池包电量较低的时候,需要设置限流策略?电量较低时,电池端电压较低,如果突然发生大电流放电,电池极化内阻会迅速增大,使得内阻占压上升,电池电势减去内阻占压后的电池端电压则会相应降低。
如果电流足够大,则端电压可能被拉低到停止供电电压以下。
如果低电压持续时间超过延时时间,电池管理系统判断电池电压过低,无法继续工作,需要断电。
锂电池剩余寿命的ELM间接预测方法
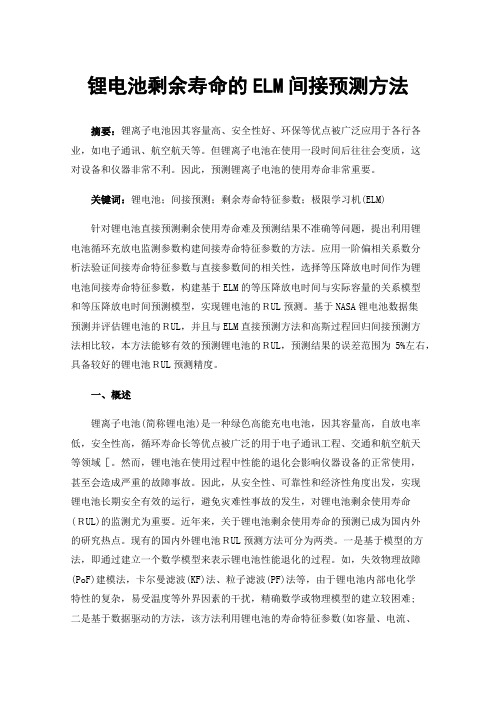
锂电池剩余寿命的ELM间接预测方法摘要:锂离子电池因其容量高、安全性好、环保等优点被广泛应用于各行各业,如电子通讯、航空航天等。
但锂离子电池在使用一段时间后往往会变质,这对设备和仪器非常不利。
因此,预测锂离子电池的使用寿命非常重要。
关键词:锂电池;间接预测;剩余寿命特征参数;极限学习机(ELM)针对锂电池直接预测剩余使用寿命难及预测结果不准确等问题,提出利用锂电池循环充放电监测参数构建间接寿命特征参数的方法。
应用一阶偏相关系数分析法验证间接寿命特征参数与直接参数间的相关性,选择等压降放电时间作为锂电池间接寿命特征参数,构建基于ELM的等压降放电时间与实际容量的关系模型和等压降放电时间预测模型,实现锂电池的RUL预测。
基于NASA锂电池数据集预测并评估锂电池的RUL,并且与ELM直接预测方法和高斯过程回归间接预测方法相比较,本方法能够有效的预测锂电池的RUL,预测结果的误差范围为5%左右,具备较好的锂电池RUL预测精度。
一、概述锂离子电池(简称锂电池)是一种绿色高能充电电池,因其容量高,自放电率低,安全性高,循环寿命长等优点被广泛的用于电子通讯工程、交通和航空航天等领域[。
然而,锂电池在使用过程中性能的退化会影响仪器设备的正常使用,甚至会造成严重的故障事故。
因此,从安全性、可靠性和经济性角度出发,实现锂电池长期安全有效的运行,避免灾难性事故的发生,对锂电池剩余使用寿命(RUL)的监测尤为重要。
近年来,关于锂电池剩余使用寿命的预测已成为国内外的研究热点。
现有的国内外锂电池RUL预测方法可分为两类。
一是基于模型的方法,即通过建立一个数学模型来表示锂电池性能退化的过程。
如,失效物理故障(PoF)建模法,卡尔曼滤波(KF)法、粒子滤波(PF)法等,由于锂电池内部电化学特性的复杂,易受温度等外界因素的干扰,精确数学或物理模型的建立较困难;二是基于数据驱动的方法,该方法利用锂电池的寿命特征参数(如容量、电流、电压、阻抗等),并借助智能算法模型(如神经网络、支持向量机、高斯过程回归等)进行检测、分析和预测,给出锂电池的剩余寿命分布、性能退化程度或失效概率,从而为维护和系统保障提供决策信息。
一种锂离子电池容量估计及剩余循环寿命预测方法[发明专利]
![一种锂离子电池容量估计及剩余循环寿命预测方法[发明专利]](https://img.taocdn.com/s3/m/38886bc24b73f242326c5fda.png)
专利名称:一种锂离子电池容量估计及剩余循环寿命预测方法专利类型:发明专利
发明人:刘大同,周建宝,王红,庞景月,彭宇,彭喜元
申请号:CN201310317219.X
申请日:20130725
公开号:CN103399276A
公开日:
20131120
专利内容由知识产权出版社提供
摘要:一种锂离子电池容量估计及剩余循环寿命预测方法,涉及一种电池容量估计及寿命的预测方法。
本发明解决了无法实现锂电池的容量估计及剩余寿命的预测的问题,本发明将采集到的电池的充放电周期次数x、每个充放电周期的放电电压及电池容量和每次充放电后的电池的剩余容量数据z的a/n和剩余的(n-a)/n的数据作为测试数据,利用分段三次Hermite插值法对训练数据进行扩展,利用扩展后获得的不同插值点的训练数据进行建模,利用获得具有不同参数的GPR模型进行外推预测,对锂电池的下N次充放电循环周期后的电池剩余容量预测,获得N次充放电周期后的电池剩余容量;本发明适用于电池容量估计及寿命的预测。
申请人:哈尔滨工业大学
地址:150001 黑龙江省哈尔滨市南岗区西大直街92号
国籍:CN
代理机构:哈尔滨市松花江专利商标事务所
代理人:岳泉清
更多信息请下载全文后查看。
锂离子电池soc估算方法
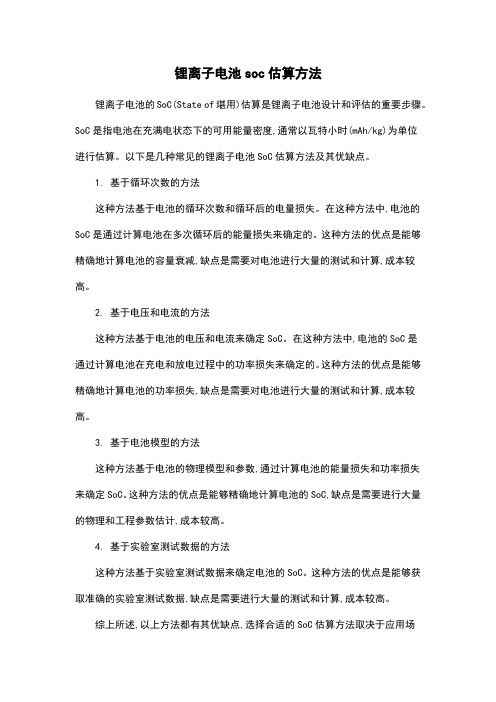
锂离子电池soc估算方法
锂离子电池的SoC(State of堪用)估算是锂离子电池设计和评估的重要步骤。
SoC是指电池在充满电状态下的可用能量密度,通常以瓦特小时(mAh/kg)为单位
进行估算。
以下是几种常见的锂离子电池SoC估算方法及其优缺点。
1. 基于循环次数的方法
这种方法基于电池的循环次数和循环后的电量损失。
在这种方法中,电池的SoC是通过计算电池在多次循环后的能量损失来确定的。
这种方法的优点是能够精确地计算电池的容量衰减,缺点是需要对电池进行大量的测试和计算,成本较高。
2. 基于电压和电流的方法
这种方法基于电池的电压和电流来确定SoC。
在这种方法中,电池的SoC是
通过计算电池在充电和放电过程中的功率损失来确定的。
这种方法的优点是能够精确地计算电池的功率损失,缺点是需要对电池进行大量的测试和计算,成本较高。
3. 基于电池模型的方法
这种方法基于电池的物理模型和参数,通过计算电池的能量损失和功率损失来确定SoC。
这种方法的优点是能够精确地计算电池的SoC,缺点是需要进行大量的物理和工程参数估计,成本较高。
4. 基于实验室测试数据的方法
这种方法基于实验室测试数据来确定电池的SoC。
这种方法的优点是能够获取准确的实验室测试数据,缺点是需要进行大量的测试和计算,成本较高。
综上所述,以上方法都有其优缺点,选择合适的SoC估算方法取决于应用场
景和预算。
锂电池SOC估计算法研究
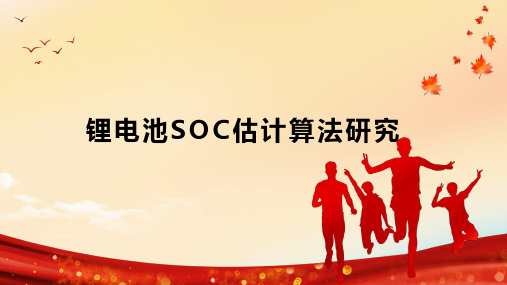
基本内容
在传统的SOC估计方法中,一般根据电池的电压、电流和温度等参数进行估算。 这些方法通常简单易行,但精度较低,且容易受到电池特性的变化和环境因素的 影响。随着现代控制理论和计算机技术的发展,一些现代估计方法逐渐被应用于 SOC估算,如基于神经网络、模糊逻辑和遗传算法等。这些方法通过建立电池模 型,能够提高估算精度,但需要大量的实验数据进行训练,且模型的适应性有待 进一步提高。
研究方法
研究方法
本次演示采用详细的数学建模和仿真方法进行研究。首先,建立磷酸铁锂电 池的详细数学模型,该模型考虑了电池的化学反应、电化学反应以及传热传质等 过程,并采用实测数据进行模型验证和校准。然后,利用所建立的模型进行电池 充放电仿真,分析不同充放电倍率、温度和老化程度等因素对电池性能的影响。
ቤተ መጻሕፍቲ ባይዱ
实验结果与分析
在SOC算法方面,本次演示所提出的混合法表现出较好的性能。在实验验证中, 该算法能够较为准确地估计电池的SOC,且稳定性较好。然而,在某些工况下, 如大电流充放电和快速温度变化时,算法的准确性可能会受到影响,这是后续研 究需要进一步改进和完善的地方。
结论与展望
结论与展望
本次演示对磷酸铁锂电池建模和SOC算法进行了研究,通过建立详细的数学模 型,分析电池的充放电性能和老化行为,并采用混合法进行SOC估计。实验结果 表明,所建立的电池模型能够较好地预测电池的性能,而混合法在SOC估计方面 也表现出较好的性能。然而,在某些特殊工况下,如大电流充放电和快速温度变 化时,算法的准确性仍需进一步提高。
相关技术综述
相关技术综述
磷酸铁锂电池是一种常见的锂离子电池,其正极材料为磷酸铁锂,负极材料 为石墨。电池的充放电过程是通过锂离子的迁移和嵌入实现的。在进行电池建模 时,需要考虑电池的化学反应、电化学反应以及传热传质等过程。常用的磷酸铁 锂电池建模方法包括物理建模、简化的数学建模和详细的数学建模等。
锂离子电池组可用剩余容量的估算方法
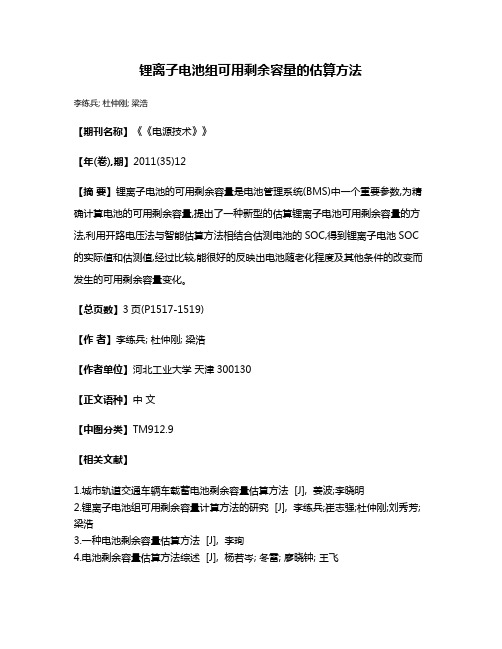
锂离子电池组可用剩余容量的估算方法
李练兵; 杜仲刚; 梁浩
【期刊名称】《《电源技术》》
【年(卷),期】2011(35)12
【摘要】锂离子电池的可用剩余容量是电池管理系统(BMS)中一个重要参数,为精确计算电池的可用剩余容量,提出了一种新型的估算锂离子电池可用剩余容量的方法,利用开路电压法与智能估算方法相结合估测电池的SOC,得到锂离子电池SOC 的实际值和估测值,经过比较,能很好的反映出电池随老化程度及其他条件的改变而发生的可用剩余容量变化。
【总页数】3页(P1517-1519)
【作者】李练兵; 杜仲刚; 梁浩
【作者单位】河北工业大学天津300130
【正文语种】中文
【中图分类】TM912.9
【相关文献】
1.城市轨道交通车辆车载蓄电池剩余容量估算方法 [J], 姜波;李晓明
2.锂离子电池组可用剩余容量计算方法的研究 [J], 李练兵;崔志强;杜仲刚;刘秀芳;梁浩
3.一种电池剩余容量估算方法 [J], 李珣
4.电池剩余容量估算方法综述 [J], 杨若岑; 冬雷; 廖晓钟; 王飞
5.基于特征处理与径向基神经网络的锂电池剩余容量估算方法 [J], 陈峥;李磊磊;舒星;沈世全;刘永刚;申江卫
因版权原因,仅展示原文概要,查看原文内容请购买。
- 1、下载文档前请自行甄别文档内容的完整性,平台不提供额外的编辑、内容补充、找答案等附加服务。
- 2、"仅部分预览"的文档,不可在线预览部分如存在完整性等问题,可反馈申请退款(可完整预览的文档不适用该条件!)。
- 3、如文档侵犯您的权益,请联系客服反馈,我们会尽快为您处理(人工客服工作时间:9:00-18:30)。
Hindawi Publishing CorporationAdvances in Mechanical EngineeringVolume2013,Article ID154831,7pages/10.1155/2013/154831Research ArticleA Method of Remaining Capacity Estimation forLithium-Ion BatteryJunfu Li,Lixin Wang,Chao Lyu,Weilin Luo,Kehua Ma,and Liqiang ZhangSchool of Electrical Engineering and Automation,Harbin Institute of Technology,Harbin150001,ChinaCorrespondence should be addressed to Lixin Wang;wlx@Received8September2013;Revised22October2013;Accepted22October2013Academic Editor:Xiaosong HuCopyright©2013Junfu Li et al.This is an open access article distributed under the Creative Commons Attribution License,which permits unrestricted use,distribution,and reproduction in any medium,provided the original work is properly cited.Combining particle filter(PF)with sample entropy feature of discharge voltage,a method of remaining capacity estimation for lithium-ion battery is proposed.The sample entropy calculated from discharge voltage curve can serve as an indicator for assessing the condition of battery.Under a certain working condition,a functional relationship between sample entropy and discharge capacity is created and estimations computed from the function are taken as observations to propagate particles in PF.The results indicate that the algorithm enhances the accuracy.Due to the establishment of functions at different discharge rates and temperature modification,prognostic accuracy of discharge capacity has been improved under multi-operating working conditions.1.IntroductionWith the rapid development of industrial technology,the exploration and utilization of new energy have been in urgent need.Electric vehicle occupies a pivotal position in new energy automobile.Battery management system(BMS)is specially designed to improve efficient utilization,to prevent overcharge or overdischarge,to prolong the service life,and to monitor the state of the battery.A more sophisticated prognostic of battery health state is much needed for high requirements of reliability,stability,and security of batteries. Consequently,the prediction of remaining battery life is considered as one of the promising research fields.Numerous papers have reported the studies on state of charge(SOC) and state of health(SOH)which are the focus of battery Prognostic and Health Management(PHM).Battery discharge capacity reaching its criteria without any omen leads to a disastrous failure in some cases.The accurate prediction of remaining useful life(RUL)of battery is essential for long-time efficient use.The causes of capacity fading are internal factors such as anodic and cathodic active material changes and SEI membrane incrassation [1,2].Accurate battery SOC estimation is of great signi-ficance to battery electric vehicles and hybrid electric vehi-cles.SOC estimation aims at the management of energy flows of electric vehicles and avoiding battery overcharge or undercharge.Lee et al.[3]proposed an Extended Kalman Filter(EKF)method along with a measurement noise model and data rejection of lithium-ion battery SOC estimation. The proposed algorithm and model approach were verified through several experiments.An adaptive unscented Kalman filtering method to estimate SOC of lithium-ion battery was presented[4].The proposed SOC estimation method had a better accuracy compared with previous works.Lee et al.[5] estimated the SOC and the capacity of a lithium-ion battery with a modified OCV-SOC model.The method overcame the variation in conventional OCV-SOC.Methods of battery capacity estimation are proposed based on the following two ideas.One method is feature-based.In one sense,as variations of voltage,current,and tem-perature characteristic curves could reflect the battery aging processes or internal resistance variations,some characters are often extracted from them.Salkind et al.[6]proposed a practical method that resistances obtained by electrochemical impedance spectroscopy(EIS)measurement and coulomb counting techniques were employed in predicting SOC and SOH.The advantage of the work was that there was no need to know previous discharge or cycling history.Gomez et al.[7]made a detailed analysis on EIS and pointed out that aging information could be extracted from the parameters of EIS equivalent circuit model.Pincus[8]firstly introduced the concept of approximate entropy mainly to compute thecomplexity of time series.Widodo et al.[9]took sample entropy features obtained from discharge voltage curves as inputs of support vector machine(SVM)and relevance vector machine(RVM)for SOH prediction.The results showed that the method proposed was plausible.The other is model-based.Generally,fault feature is closely related to the parameters of the model.Correction and adjustment of model parameters can enhance the pre-diction accuracy.The model-based techniques contribute to an in-depth understanding of the mechanism and have the advantage of real-time fault prediction.A model of battery system state is established to describe the discharge behavior or battery health state.Abbas et al.[10]introduced an integrated methodology based on both physics of failure models and Bayesian estimation methods for prognosis of electrical components.An empirical formula was proposed to depict discharging behavior of lithium-ion batteries[11–13].Simulation results indicated that PF algorithm was appropriate for the prediction of battery health state.Saha et al.[14]presented several algorithms including ARIMA, RVM,EKF,and PF.A RVM-PF framework had significant advantages over the conventional methods of RUL estimation like ARIMA and EKF.Some researchers have also established electrochemical numerical model and thermal model for the study on battery internal characteristics.Porous electrode model with liquid electrolyte was proposed by West et al.[15].That electrolyte depletion was the primary limiting factor of capacity was demonstrated.Park et al.[16]presented an electrochemical heat conduction phenomenal model.A better understanding of conduction phenomena of lithium-ion batteries was pre-sented.Kim et al.[17]extended one-dimensional modeling approach to three dimensions to capture geometrical features such as shapes and dimensions of cell components,to simulate oven tests and to determine how a local hot spot can propagate through the cell.Though some key behaviors of battery cells can be captured in these models,it is complex to deploy a large number of unknown parameters due to the memory and computation.Lumped battery models are likely to be the preferred choice with a relatively fewer parameters.A systematic comparative study of twelve lumped battery models was conducted[18].The developed cell voltage models could be used in SOC estimation in BMS.This work is conducted by the combination of the two ideas mentioned above.In the following section,we firstly introduce the theory about sample entropy and basic uti-lization of particle filter in terms of prognostics of lithium-ion battery RUL.Then,we present the detailed prediction procedure.2.Theory and Intelligent Prognostic Method 2.1.Sample Entropy.Sample entropy is defined as generation rate of new information by Richman and Moorman[19] for the calculation of complexity of time series.It can be expressed as SampEn(m,r,N),where N is a given total number of data,r is the tolerance for accepting matrices, and m is the dimension of vectors.The specific algorithm of sample entropy is as follows.For a given series{x i},we form N−m+1vectors asX(i)=[x(i),x(i+1),...,x(i+m−1)],for i=1to N−m+1.(1)The distance between vectors X(i)and X(j)is defined as d[X(i),X(j)]=max x(i+k)−x(j+k) ,for i,j=1to N−m+1,k=0to m−1.(2)For a given r,calculate the number when d[X(i),X(j)]< r,for i=j,and define the functionB m i(r)=1N−m num{d[X(i),X(j)]<r}.(3) Then,take the average of B m i(r).The result is expressed asB m(r)=1N−m+1N−m+1∑i=1B m i(r).(4)Similarly,replace m with m+1and repeat the steps from the beginning.Afterwards,we can determine the two values B m(r)and B m+1(r).As the sample length is always limited,the sample entropy is estimated bySampEn(m,r,N)=−ln[B m+1(r)B m(r)].(5)The value of SampEn(m,r,N)is closely correlated with m,r,and N.Thus,the proper selected parameters could result in more reasonable statistical properties.2.2.Particle Filter.PF is a Bayesian learning technique using Monte Carlo simulations.The idea is to describe the system state as a probability density function(PDF)approximated by particles that are generated from a priori distribution and updated from observations through a measurement model.Model parameters are included as a part of the state vector to be tracked[11].PF framework can be applied to RUL prediction of battery due to its good state tracking performance.Actual discharge capacity is associated with many factors. It is obvious that charging directly determines the discharge capacity in one cycle.Besides,reaction products forming up around the electrodes will decompose during rest or relaxation period,which lead to the increase of available capacity in next cycle.Primarily,considering the main influ-ence factors of battery capacity,the following state equations are cast to describe the model as follows:C k+1=β1C k+β2exp(β3ΔT k),(6)βi(k+1)=βi(k)+V i(k),i=1,2,3,(7)where k is cycle index,C k denotes the charge capacity,ΔT k is the relaxation period between the two adjacent cycles,C k+1 is the discharge capacity,β1,β2,andβ3are parameters of the state equation,and V1,V2,and V3are independent zero-mean Gaussian noise terms.Saha and Goebel[11]established a measurement model and regarded charging capacity as the observation to prop-agate particles.A reasonable observation for measuring the weights of particles and selectively propagating them plays an important role in prediction accuracy.In the case of our application,via the fitting method,a functional relationship of sample entropy and discharge capacity is established to obtain an appropriate observation.Particularly,sample entropy is calculated from the discharge voltage curve of the cycle number k.The corresponding output of the function is used as the observation in cycle k+1.It is worth mentioning that there is no need to take other experiments to obtain such features,for the discharge voltage curves can be easily obtained during the monitoring in each cycle.2.3.Intelligent Prognostic Method.The procedure comprises the following.(1)Data collection is as follows.(a)Extract battery discharge voltage curves fromtraining data and the selected parameters mand r are2and0.1,respectively.The functionalrelationship of discharge capacity and sampleentropy is created under the current operatingcondition.(b)Gain discharge current curves,charging capac-ity,and relaxation time of adjacent dischargecycles from validation test data.In addition,some historical capacity data are also needed.(2)Particle filter initialization is as follows.(a)Set the starting prediction point T in proportionto the number of historical capacity data.(b)Obtain initial parametersβi(i=1,2,3)viafitting.(c)500initial particles are generated with valuesobtained in(2)-(b)and the variances of noiseterm V i(i=1,2,3)are about10,000timessmaller thanβ.(3)Prediction is as follows.(a)Particles{x i k}N i=1are updated by(7)and thepriori discharge capacity values in cycle k+1are calculated through those updated particles{x i k+1}N i=1.(b)Take sample entropy feature as the input of thefunction and compute the weight of each parti-cle per deviation between the calculated obser-vation and previous discharge voltage value.Normalize the very particles using the followingformula:w k+1(x i k+1)=w k+1(x i k+1)∑N i=1w k+1(x i k+1).(8)(c)Through the method of random sampling,eachparticle{x i k+1}N i=1is copied or abandoned selec-tively according to its weight and then newsample{̃x i k+1}N i=1is obtained.(d)The average of the sample{̃x i k+1}N i=1representsthe probability density distribution expectationof each parameter in(6).Then,the final estima-tion C k+1can be easily figured up by(6).(e)Repeat the step from(3)-(a)to(3)-(d)until thecapacity reaches its criterion which is a30%fading of rated capacity.3.Experiment DataThe full set of aging data collected from commercially available18650-size lithium-ion cells provided by NASA Ames Prognostics Center of Excellence was taken as object of study.Battery anode and cathode materials are mostly LiNi0.8Co0.15Al0.05O2and MAG-10graphite,respectively.The electrolyte is1.2M LiPF6in EC:EMC(3:7wt%)and the separator is25μm thick PE.All testing batteries were run through different working profiles(charge,discharge,and impedance).Batteries No.6 and No.18were tested by the following steps:(1)charging was carried out in a constant current mode at1.5A until the battery voltage reached4.2V,(2)a constant voltage mode was then in operation until the charge current dropped to20mA,(3)batteries were put aside for a period of time,(4)impedance measurement was implemented with an electrochemical impedance spectroscopy frequency sweep from0.1Hz to5kHz,(5)at24∘C,discharging was carried out at a constant current level of2A until the battery voltage fell to2.5V,(6)the same step as(3),and(7)the same step as(4).Repeated charging and discharging resulted in an accelerated aging process.The experiments were stopped when the batteries reached the end-of-life criteria which was a30%fading in rated capacity(from2Ahr to1.4Ahr).4.Results and Discussion4.1.Single Working Condition.Figure1depicts the discharge voltage curves in different cycles.At a constant current of 2A,the voltage drops from4.2V to2.6V.Obviously,the curves vary from cycle to cycle in the aging processes.It can be seen from Figure1that the lowest voltage point bounces back instantly at the end of discharge and subsequently rises slowly until it comes to a stop.The two arrows point out the processes mentioned above.Observing the definition of sample entropy,we can find that when the maximum distance computed from the adjacent vectors constituted by the sequential samples is greater than r,the complexity number of the corresponding vector in(3)will not changeV o l t a g e (V )4.23.83.432.6First cycle Second cycle Third cycle TimeFourth cycleFigure 1:Battery voltage curves in different cycles and the two voltage variation processes were pointed out by thearrows.2.11.91.71.51.3Actual discharge capacityEstimated value with observation obtained from sample entropyC a p a c i t y (A h r )Cycle (—)Figure 2:Prediction of battery No.6.E r r o r (%)0.080.060.040.02020406080100120140Cycle (—)Figure 3:Relativeerrors.2.11.91.71.51.31.1Actual discharge capacityEstimated value with observation obtained from sample entropy C a p a c i t y (A h r )Cycle (—)Estimated value with observation obtained from charging capacityFigure 4:Comparative simulation results through different meth-ods.in statistical calculations.Otherwise,if the noise signal is added to the samples with larger amplitude,it will be ignored by detection,for the distance between the disturbed vectors is longer than others.In that sense,sample entropy could capture the features of voltage variance in a constant current mode.As battery is aging gradually during the usage period,we find an interesting connection between the sample entropy feature and the discharge capacity.In consequence,sample entropy could serve as an indicator for assessing the condition of battery.With training data of battery No.18,a cubic polynomial fitting is introduced to find out the functional relationship between them.When the parameter m and r are deployed to 2and 0.1,respectively,a better fitting effect is obtained with a reasonable statistical result.The starting point T and predicting length are 25and 115.Figures 2and 3show the prediction result of battery No.6and its errors.From the actual discharge capacity curve,it is evident that battery No.6has faded to its limit 1.405Ahr when it cycles at cycle number 108.Observing Figure 3,apart from several points,most relative errors are within 5%.The early prediction has higher precision and errors of some rebound points are less than 2%.To illustrate the superiority of this work compared with Saha and Goebel [11],Figure 4shows the comparative pre-diction result.As is showed in Figure 4,some key points of prediction are pointed out by seven arrows on the graph and the contrastive prediction apparently engenders a greater error.Prediction accuracy is measured by the root-mean-squared (RMS)error and peak error.The statistical figures reveal that RMS errors of both predictions are 8.64%and 4.30%,respectively,and the peak errors are 37.86%and 8.28%.The discharge capacity is not only directly related to charge capacity and rest time of adjacent cycles but is also affected by actual working conditions.When the forecasting and training conditions,such that ambient temperature and discharge rate are inconsistent,it can be easily expected that the estimation points will deviate from the actual ones in each cycle.4.2.Multioperating Working Condition.Without knowing of aging mechanism,it is hard to make a specific illustration that how the aging process inside the battery is influenced by environmental factors.But,it is certain that as battery aging processes,different operational conditions accounts for the discharge capacity fading behaviors.It is required to update or revise the aforementioned function properly to satisfy the requirement of high accuracy when facing a multioperating working condition.The datasets provided by NASA only include several discharge rates.Thus,the paper builds three functions taking different C -rates under each ambient temperature into account summarized in Table 1,where x is sample entropy and F is the estimation capacity used as observation in algorithm PF in our method.Suppose that the operating ambient temperature is 24∘C.It is interesting to find that the relative mean deviations between estimation values and discharge capacities at actualTable 1:Capacity estimation functions under different optional conditions.Discharge rate Ambient temperatureCapacity estimation function0.5C 4∘C F =(9.6169x 3−0.4326x 2+0.0035x +0.0001)×1041C 24∘C F =(−1.1240x 3+0.0154x 2−0.0166x +0.0018)×1032C24∘C F =(−7.2590x 3+0.3225x 2−0.0044x +0.00003)×105O ff s e t (A h r )0.1−0.1−0.3−0.551015202530354045Temperature (deg)0−0.4−0.200.2Figure 5:Offsets at different temperature.Actual EstimatedC a p a c i t y (A h r )1.41.31.21.110.90.80.701020304050607080Cycle (—)Figure 6:Prediction of battery No.55.Actual EstimatedC a p a c i t y (A h r )1.821.781.741.71.66510152025303540Cycle (—)Figure 7:Prediction of battery No.31.C a p a c i t y (A h r )1.61.20.4005152025303540Actual Estimated0.810Cycle (—)Figure 8:Prediction of battery No.39.Table 2:RMS errors and peak errors of battery No.55.Starting point T RMS error (%)Peak error (%)10 3.2613.2215 2.647.0720 2.30 6.75252.245.63Table 3:RMS errors and peak errors of battery No.31.Starting point T RMS error (%)Peak error (%)10 2.17 5.2712 1.64 4.22151.373.12temperature 4∘C and 43∘C are around −0.38and 0.02.As a matter of fact,higher or lower temperature affects the actual discharge capacity.On account of the higher ambi-ent temperature,the internal substances are more active resulting in a larger discharge capacity.On the contrary,the lower temperatures slow down the physicochemical reactions inside the battery leading to the fact that the actual capacity cannot reach the maximum.In a constant discharge current mode,it is reasonable and essential to modify the capacity observations in PF algorithm.Thus,according to the previous calculations,a functional relationship between ambient temperatures and estimation offsets is established through quadratic curve fitting.The fitting result is given in Figure 5.The selected offset benchmark is zero at 24∘C.Figures 6and 7show the prediction results of battery No.55(4∘C,1C )and No.31(43∘C,2C ).Both two offsets are separately −0.38and 0.02.As is expected,the prediction curves are basically consistent with the actual ones.Tables 2and 3show the RMS errors and peak errors at different prediction starting points.The results indicate that as the number of historical capacity data is increasing,errors have the downward trends.Battery No.39is tested under a multioperating working condition.The first several discharge cycles are tested at 24∘C,2C and the others at 44∘C,0.5C .The corresponding capacity estimation function should be selected in accordance with the operating condition.As one of the relevant functions is built at 4∘C,0.5C ,the actual offset at 44∘C should be increased to 0.398rather than 0.018in Figure 5.The prediction result of battery No.39is presented in Figure 8and the RMS error is 5.78%.Figure 9shows the contrastive prediction result.Without the consideration of C -rate and ambient temperature,the estimation performs much worse with 27.56%RMS error.Actual EstimatedC a p a c i t y (A h r )1.61.20.400.8515202530354010Cycle (—)Figure 9:Comparative prediction.A large amount of discharge datasets will be required mainly for establishment of a set of capacity estimation functions.The choice of a proper function in accordance with the working condition is necessary for the implement of algorithm.Otherwise,it takes about a period of 200ms proportional to the number of estimation points to complete prediction for each cycle.5.ConclusionsThis paper focuses on developing an intelligent prediction method of battery capacity through particle filter and sample entropy.Under a certain optional condition,a functional relationship of sample entropy and discharge capacity is cre-ated.The estimations computed from the function are taken as observations to propagate particles in PF.When facing a multioperating working condition,this paper builds three functions considering different C -rates under different ambi-ent temperatures.It is a key point to select a corresponding capacity estimation function and to modify the observation by temperature.On account of good tracking capabilities,PF algorithm is applied to determine the unknown parameters and fulfill the prediction with better statistical calculations.The prediction result can reflect the capacity fading behaviors and has a higher accuracy with not more than 5%RMS error of battery pared with other methods,prognostic accuracy has been greatly improved under a large range of cycling conditions with less than 6%RMS error.In addition,though the prediction results have been satisfactory,there still leaves considerable room for improve-ments.Our method is not fit for practical application now,for the ambient temperature and C -rates are constants in one cycle in our work.When facing a dynamic cycle,such as a complex current,its impact on capacity could be equivalently seen as a constant one,which seems to be a considerable solution.With an improving understanding of these impacts on battery capacity,the prognostic performance can be further refined.Conflict of InterestsThe authors declare that there is no conflict of interests regarding the publication of this paper.AcknowledgmentsThis research is financially supported by the National Nat-ural Science Foundation of China (no.51107021)and the Fundamental Research Funds for the Central Universities (Grant no.HIT.NSRIF.2014021).We sincerely appreciate the significant help on translation by Miss.Han Wang.References[1]Q.Zhang and R.E.White,“Capacity fade analysis of a lithium ion cell,”Journal of Power Sources ,vol.179,no.2,pp.793–798,2008.[2]M.Dubarry and B.Y.Liaw,“Identify capacity fading mechanismin a commercial LiFePO 4cell,”Journal of Power Sources ,vol.194,no.1,pp.541–549,2009.[3]J.Lee,O.Nam,and B.H.Cho,“Li-ion battery SOC estimationmethod based on the reduced order extended Kalman filtering,”Journal of Power Sources ,vol.174,no.1,pp.9–15,2007.[4]F.Sun,X.Hu,Y.Zou,and S.Li,“Adaptive unscented Kalmanfiltering for state of charge estimation of a lithium-ion battery for electric vehicles,”Energy ,vol.36,no.5,pp.3531–3540,2011.[5]S.Lee,J.Kim,J.Lee,and B.H.Cho,“State-of-charge and cap-acity estimation of lithium-ion battery using a new open-circuit voltage versus state-of-charge,”Journal of Power Sources ,vol.185,no.2,pp.1367–1373,2008.[6]A.J.Salkind,C.Fennie,P .Singh,T.Atwater,and D.E.Reisner,“Determination of state-of-charge and state-of-health of batter-ies by fuzzy logic methodology,”Journal of Power Sources ,vol.80,no.1,pp.293–300,1999.[7]J.Gomez,R.Nelson,E.E.Kalu,M.H.Weatherspoon,and J.P .Zheng,“Equivalent circuit model parameters of a high-power Li-ion battery:thermal and state of charge effects,”Journal of Power Sources ,vol.196,no.10,pp.4826–4831,2011.[8]S.M.Pincus,“Approximate entropy as a measure of systemcomplexity,”Proceedings of the National Academy of Sciences of the United States of America ,vol.88,no.6,pp.2297–2301,1991.[9]A.Widodo,M.-C.Shim,W .Caesarendra,and B.-S.Yang,“Int-elligent prognostics for battery health monitoring based on sample entropy,”Expert Systems with Applications ,vol.38,no.9,pp.11763–11769,2011.[10]M.Abbas,A.A.Ferri,M.E.Orchard,and G.J.Vachtsevanos,“An intelligent diagnostic/prognostic framework for automo-tive electrical systems,”in Proceedings of the IEEE Intelligent Vehicles Symposium (IV ’07),pp.352–357,Istanbul,Turkey,June 2007.[11]B.Saha and K.Goebel,“Modeling Li-ion battery capacity dep-letion in a particle filtering framework,”in Proceedings of the Annual Conference of the Prognostics and Health Management Society ,2009.[12]K.Goebel,B.Saha,A.Saxena,J.R.Celaya,and J.P .Christopher-sen,“Prognostics in battery health management,”IEEE Instru-mentation and Measurement Magazine ,vol.11,no.4,pp.33–40,2008.[13]S.Saha,B.Saha,and K.Goebel,“Distributed prognostic healthmanagement with Gaussian process regression,”in Proceedings of the Conference of the Society for Machinery Failure Prevention Technology (MFPT ’09),April 2009.[14]B.Saha,K.Goebel,and J.Christophersen,“Comparison of pro-gnostic algorithms for estimating remaining useful life of bat-teries,”Transactions of the Institute of Measurement and Control ,vol.31,no.3-4,pp.293–308,2009.[15]K.West,T.Jacobsen,and S.Atlung,“Modeling of porous inser-tion electrodes with liquid electrolyte,”Journal of the Electro-chemical Society,vol.129,no.7,pp.1480–1485,1982.[16]M.Park,X.Zhang,M.Chung,G.B.Less,and A.M.Sastry,“Areview of conduction phenomena in Li-ion batteries,”Journal of Power Sources,vol.195,no.24,pp.7904–7929,2010.[17]G.H.Kim,A.Pesaran,and R.Spotnitz,“A three-dimensionalthermal abuse model for lithium-ion cells,”Journal of Power Sources,vol.170,no.2,pp.476–489,2007.[18]X.Hu,S.Li,and H.Peng,“A comparative study of equivalentcircuit models for Li-ion batteries,”Journal of Power Sources,vol.198,pp.359–367,2012.[19]J.S.Richman and J.R.Moorman,“Physiological time-seriesanalysis using approximate and sample entropy,”American Jour-nal of Physiology:Heart and Circulatory Physiology,vol.278,no.6,pp.H2039–H2049,2000.Impact Factor 1.73028 Days Fast Track Peer Review All Subject Areas of Science Submit at Hindawi Publishing Corporation Volume 2013The Scientific World Journal。