MODIS Land Cover Date数据说明
modis土地覆盖分类
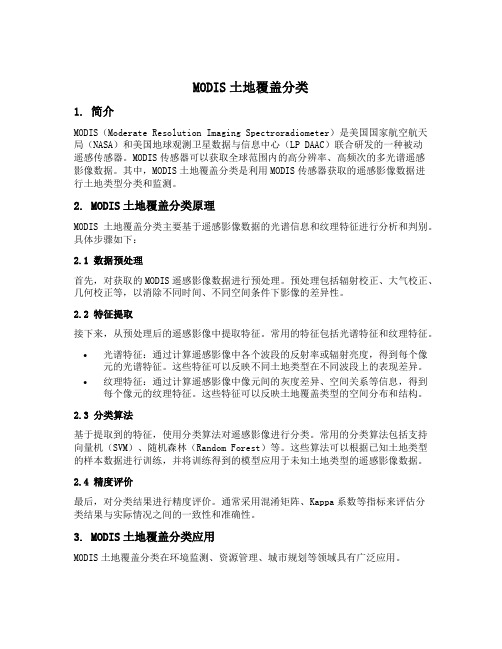
MODIS土地覆盖分类1. 简介MODIS(Moderate Resolution Imaging Spectroradiometer)是美国国家航空航天局(NASA)和美国地球观测卫星数据与信息中心(LP DAAC)联合研发的一种被动遥感传感器。
MODIS传感器可以获取全球范围内的高分辨率、高频次的多光谱遥感影像数据。
其中,MODIS土地覆盖分类是利用MODIS传感器获取的遥感影像数据进行土地类型分类和监测。
2. MODIS土地覆盖分类原理MODIS土地覆盖分类主要基于遥感影像数据的光谱信息和纹理特征进行分析和判别。
具体步骤如下:2.1 数据预处理首先,对获取的MODIS遥感影像数据进行预处理。
预处理包括辐射校正、大气校正、几何校正等,以消除不同时间、不同空间条件下影像的差异性。
2.2 特征提取接下来,从预处理后的遥感影像中提取特征。
常用的特征包括光谱特征和纹理特征。
•光谱特征:通过计算遥感影像中各个波段的反射率或辐射亮度,得到每个像元的光谱特征。
这些特征可以反映不同土地类型在不同波段上的表现差异。
•纹理特征:通过计算遥感影像中像元间的灰度差异、空间关系等信息,得到每个像元的纹理特征。
这些特征可以反映土地覆盖类型的空间分布和结构。
2.3 分类算法基于提取到的特征,使用分类算法对遥感影像进行分类。
常用的分类算法包括支持向量机(SVM)、随机森林(Random Forest)等。
这些算法可以根据已知土地类型的样本数据进行训练,并将训练得到的模型应用于未知土地类型的遥感影像数据。
2.4 精度评价最后,对分类结果进行精度评价。
通常采用混淆矩阵、Kappa系数等指标来评估分类结果与实际情况之间的一致性和准确性。
3. MODIS土地覆盖分类应用MODIS土地覆盖分类在环境监测、资源管理、城市规划等领域具有广泛应用。
3.1 环境监测MODIS土地覆盖分类可以用于监测和评估生态环境的变化。
通过对不同时间段的遥感影像数据进行分类,可以获得土地覆盖类型的时空变化信息。
MODIS大数据说明书(经典)
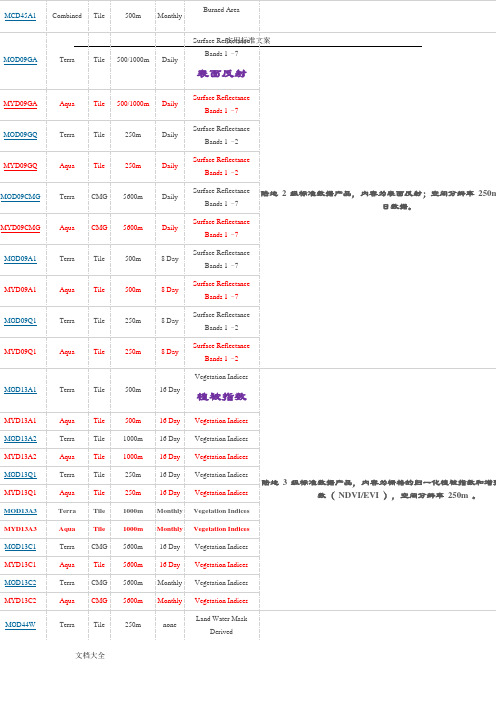
Aqua CMG 5600m
Daily
Terra CMG 5600m
8 Day
文档大全
Land Surface Temperature &
Emissivity
Land Surface Temperature &
Emissivity
Land Surface Temperature &
Emissivity
500m
Nadir BRDF-Adjusted Reflectance
16 Day
纳迪尔
BRDF 的调
文档大全
实用标准文案
整后反射
MCD43B4 MCD43C4
MOD12Q1
Combined Tile Combined CMG
Terra
Tile
1000m 5600m
1000m
Nadir BRDF-Adjusted 16 Day
Terra
Tile
500 m 5600m 5600m
500m
Yearly Land Cover Dynamics
Yearly Land Cover Type
Yearly Land Cover Type
Yearly
Vegetation Continuous Fields
连续场植被
MODIS 数据简介及具体编号说明情
陆地 2 级标准数据产品,内容为热异常 - 火灾和生物量燃烧, 率 1km ,确定火灾发生的位置、火灾等级以及暗火与燃烧
Thermal Anomalies & Daily
Fire
Thermal Anomalies & 8 Day
Fire
基于 MODIS 数据的秸秆焚烧遥感监测
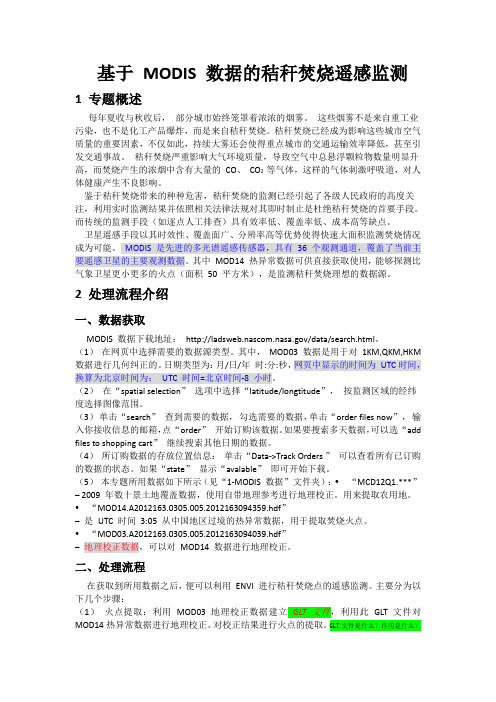
基于MODIS 数据的秸秆焚烧遥感监测1 专题概述每年夏收与秋收后,部分城市始终笼罩着浓浓的烟雾。
这些烟雾不是来自重工业污染,也不是化工产品爆炸,而是来自秸秆焚烧。
秸秆焚烧已经成为影响这些城市空气质量的重要因素,不仅如此,持续大雾还会使得重点城市的交通运输效率降低,甚至引发交通事故。
秸秆焚烧严重影响大气环境质量,导致空气中总悬浮颗粒物数量明显升高,而焚烧产生的浓烟中含有大量的CO、CO2 等气体,这样的气体刺激呼吸道,对人体健康产生不良影响。
鉴于秸秆焚烧带来的种种危害,秸秆焚烧的监测已经引起了各级人民政府的高度关注,利用实时监测结果并依照相关法律法规对其即时制止是杜绝秸秆焚烧的首要手段。
而传统的监测手段(如逐点人工排查)具有效率低、覆盖率低、成本高等缺点。
卫星遥感手段以其时效性、覆盖面广、分辨率高等优势使得快速大面积监测焚烧情况成为可能。
MODIS 是先进的多光谱遥感传感器,具有36 个观测通道,覆盖了当前主要遥感卫星的主要观测数据。
其中MOD14 热异常数据可供直接获取使用,能够探测比气象卫星更小更多的火点(面积50 平方米),是监测秸秆焚烧理想的数据源。
2 处理流程介绍一、数据获取MODIS 数据下载地址:/data/search.html。
(1)在网页中选择需要的数据源类型。
其中,MOD03 数据是用于对1KM,QKM,HKM 数据进行几何纠正的。
日期类型为:月/日/年时:分:秒,网页中显示的时间为UTC时间,换算为北京时间为:UTC 时间=北京时间-8 小时。
(2)在“spatial selection” 选项中选择“latitude/longtitude”,按监测区域的经纬度选择图像范围。
(3)单击“search” 查到需要的数据,勾选需要的数据,单击“order files now”,输入你接收信息的邮箱,点“order” 开始订购该数据。
如果要搜索多天数据,可以选“add files to shopping cart” 继续搜索其他日期的数据。
modis数据格式及使用方法
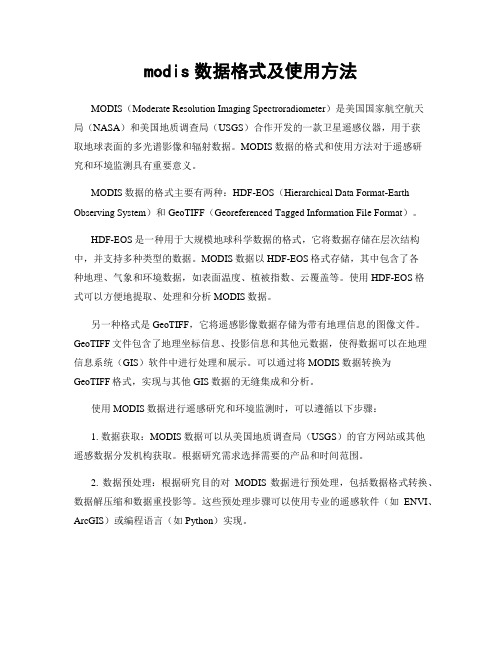
modis数据格式及使用方法MODIS(Moderate Resolution Imaging Spectroradiometer)是美国国家航空航天局(NASA)和美国地质调查局(USGS)合作开发的一款卫星遥感仪器,用于获取地球表面的多光谱影像和辐射数据。
MODIS数据的格式和使用方法对于遥感研究和环境监测具有重要意义。
MODIS数据的格式主要有两种:HDF-EOS(Hierarchical Data Format-Earth Observing System)和GeoTIFF(Georeferenced Tagged Information File Format)。
HDF-EOS是一种用于大规模地球科学数据的格式,它将数据存储在层次结构中,并支持多种类型的数据。
MODIS数据以HDF-EOS格式存储,其中包含了各种地理、气象和环境数据,如表面温度、植被指数、云覆盖等。
使用HDF-EOS格式可以方便地提取、处理和分析MODIS数据。
另一种格式是GeoTIFF,它将遥感影像数据存储为带有地理信息的图像文件。
GeoTIFF文件包含了地理坐标信息、投影信息和其他元数据,使得数据可以在地理信息系统(GIS)软件中进行处理和展示。
可以通过将MODIS数据转换为GeoTIFF格式,实现与其他GIS数据的无缝集成和分析。
使用MODIS数据进行遥感研究和环境监测时,可以遵循以下步骤:1. 数据获取:MODIS数据可以从美国地质调查局(USGS)的官方网站或其他遥感数据分发机构获取。
根据研究需求选择需要的产品和时间范围。
2. 数据预处理:根据研究目的对MODIS数据进行预处理,包括数据格式转换、数据解压缩和数据重投影等。
这些预处理步骤可以使用专业的遥感软件(如ENVI、ArcGIS)或编程语言(如Python)实现。
3. 数据提取:根据研究需求,从MODIS数据中提取出感兴趣的地理、气象或环境参数,比如植被指数、着火点数据等。
esri 10m land cover和modis土地利用分类

esri 10m land cover和modis土地利用分类Esri 10m Land Cover ClassificationEsri 10m Land Cover Classification is a dataset that provides detailed information about the land cover classes present in a geographic area. This dataset is widely used in various fields including urban planning, environmental management, and natural resource analysis. The classification scheme used in Esri 10m Land Cover Classification includes the following classes:1.Water:–Category: Water bodies such as oceans, lakes,rivers, and reservoirs.–Purpose: Identifying and analyzing water resources, hydrology, and aquatic ecosystem management.2.Forest:–Category: Areas covered with trees and densevegetation.–Purpose: Studying forest ecosystems, biodiversity, and monitoring deforestation.3.Agricultural land:–Category: Land used for cultivating crops, raising livestock, and other agricultural purposes.–Purpose: Analyzing agricultural practices, crop yield estimation, and land management strategies. 4.Grassland:–Category: Areas dominated by grass or herbaceous vegetation.–Purpose: Monitoring changes in grassland ecosystems, grazing patterns, and wildlife habitat analysis.5.Urban areas:–Category: Areas characterized by human-madestructures and infrastructure.–Purpose: Urban planning, land use change analysis, and understanding the impacts of urbanization.6.Wetland:–Category: Land that is permanently or temporarily covered with water.–Purpose: Wetland conservation, studying waterresource management, and habitat assessment.7.Barren land:–Category: Areas devoid of vegetation or with sparse vegetation cover.–Purpose: Studying desertification, land degradation, and identifying areas suitable for afforestation. 8.Snow and ice:–Category: Areas covered with snow, glaciers, or ice.–Purpose: Monitoring changes in snow cover, glacial retreat, and analyzing the impacts of climatechange.MODIS Land Use ClassificationMODIS (Moderate Resolution Imaging Spectroradiometer) Land Use Classification is another widely used dataset that provides information about the various land use classes in a specific region. This dataset has a coarser resolution compared to Esri 10m Land Cover Classification but covers a larger area. The land use classes in MODIS Land Use Classification include the following:1.Cropland:–Category: Land used for agricultural purposes, including cultivation of crops.–Purpose: Monitoring agricultural practices,analyzing crop patterns, and estimating cropproductivity.2.Grassland:–Category: Land predominantly covered with grasses or herbaceous vegetation.–Purpose: Evaluating grazing practices, studying grassland dynamics, and wildlife habitat analysis.3.Urban and built-up:–Category: Areas characterized by human-madestructures, urban development, and infrastructure.–Purpose: Urban planning, understanding urban expansion patterns, and analyzing the impacts ofurbanization.4.Forest and woodland:–Category: Areas covered with trees and forests.–Purpose: Studying forest ecosystems, monitoring deforestation, and assessing biodiversity.5.Water bodies:–Category: Lakes, rivers, oceans, and other water bodies.–Purpose: Analyzing water resources, hydrological processes, and aquatic ecosystem management.6.Shrubland:–Category: Land covered with shrubs or low-lying vegetation.–Purpose: Studying shrubland ecology, wildlifehabitat analysis, and land management strategies. 7.Desert:–Category: Barren land or areas with sparsevegetation cover.–Purpose: Understanding desertification, landdegradation, and identifying suitable areas forvegetation restoration.8.Snow and ice:–Category: Areas covered with snow, glaciers, or ice.–Purpose: Monitoring changes in snow cover,analyzing glacial retreat, and studying the impactsof climate change.These are just a few examples of the land cover and land use classifications provided by Esri 10m Land Cover and MODIS datasets. Both datasets offer valuable insights into the composition and distribution of land cover classes, allowing researchers, policymakers, and planners to make informed decisions for sustainable land management.Sure, here are more classifications from Esri 10m Land Cover and MODIS Land Use datasets:Esri 10m Land Cover Classification9.Shrubland:–Category: Land covered with shrubs or low-lying vegetation.–Purpose: Studying shrubland ecology, wildlifehabitat analysis, and land management strategies.10.Mangroves:–Category: Coastal wetlands dominated by salt-tolerant trees or shrubs.–Purpose: Monitoring and conservation of mangrove ecosystems, coastal management.11.Swamp/Marshes:–Category: Wetlands characterized by saturated soil and emergent vegetation.–Purpose: Studying wetland biodiversity, water quality, and carbon storage.12.Bare Ground:–Category: Areas devoid of vegetation or withminimal vegetation cover.–Purpose: Monitoring land degradation, erosion, and assessing soil health.13.Rock and Scree:–Category: Areas predominantly covered by rocks, stones, or loose debris.–Purpose: Studying geomorphology, landscapeevolution, and land stability analysis.MODIS Land Use Classification9.Wetland:–Category: Areas of marsh, peatland, or other wetland environments.–Purpose: Wetland conservation, water resource management, and habitat assessment.10.Plantations:–Category: Extensively managed areas with single-species plantations, such as tree plantations.–Purpose: Monitoring and managing plantation resources, evaluating land use change.11.Open Space:–Category: Land used for recreational purposes, public parks, or open areas.–Purpose: Urban planning, urban green spaces analysis, and promoting outdoor activities.12.Mining:–Category: Areas used for extraction of minerals, including open-pit mines and quarries.–Purpose: Monitoring mining activities, assessing environmental impacts, and land reclamation. 13.Built-up/Paved:–Category: Areas covered with impervious surfaces, such as buildings, roads, and parking lots.–Purpose: Urban planning, analyzing urban heatisland effect, and assessing land use changes.These additional classifications provide a more comprehensive understanding of the land cover and land use patterns in a given area. Detailed analysis of these datasets enables researchers and decision-makers to address various environmental, social, and economic challenges.。
MODIS数据介绍、下载及处理
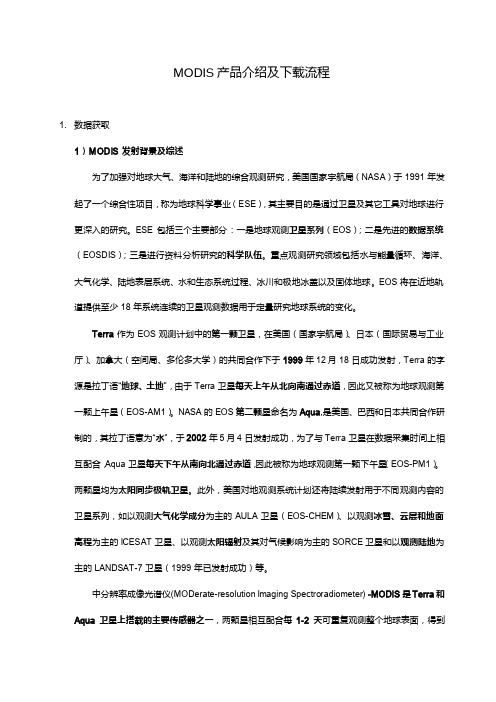
MODIS产品介绍及下载流程1.数据获取1)MODIS 发射背景及综述为了加强对地球大气、海洋和陆地的综合观测研究,美国国家宇航局(NASA)于1991年发起了一个综合性项目,称为地球科学事业(ESE),其主要目的是通过卫星及其它工具对地球进行更深入的研究。
ESE包括三个主要部分:一是地球观测卫星系列(EOS);二是先进的数据系统(EOSDIS);三是进行资料分析研究的科学队伍。
重点观测研究领域包括水与能量循环、海洋、大气化学、陆地表层系统、水和生态系统过程、冰川和极地冰盖以及固体地球。
EOS将在近地轨道提供至少18年系统连续的卫星观测数据用于定量研究地球系统的变化。
Terra作为EOS观测计划中的第一颗卫星,在美国(国家宇航局)、日本(国际贸易与工业厅)、加拿大(空间局、多伦多大学)的共同合作下于1999年12月18日成功发射,Terra的字源是拉丁语“地球、土地”,由于Terra卫星每天上午从北向南通过赤道,因此又被称为地球观测第一颗上午星(EOS-AM1)。
NASA的EOS第二颗星命名为Aqua,是美国、巴西和日本共同合作研制的,其拉丁语意为“水”,于2002年5月4日发射成功,为了与Terra卫星在数据采集时间上相互配合,Aqua卫星每天下午从南向北通过赤道,因此被称为地球观测第一颗下午星(EOS-PM1)。
两颗星均为太阳同步极轨卫星。
此外,美国对地观测系统计划还将陆续发射用于不同观测内容的卫星系列,如以观测大气化学成分为主的AULA卫星(EOS-CHEM)、以观测冰雪、云层和地面高程为主的ICESAT卫星、以观测太阳辐射及其对气候影响为主的SORCE卫星和以观测陆地为主的LANDSAT-7卫星(1999年已发射成功)等。
中分辨率成像光谱仪(MODerate-resolution Imaging Spectroradiometer) -MODIS是Terra和Aqua卫星上搭载的主要传感器之一,两颗星相互配合每1-2天可重复观测整个地球表面,得到36个波段的观测数据,这些数据将有助于我们深入理解全球陆地、海洋和低层大气内的动态变化过程,因此,MODIS在发展有效的、全球性的用于预测全球变化的地球系统相互作用模型中起着重要的作用,其精确的预测将有助于决策者制定与环境保护相关的重大决策。
MODIS数据说明
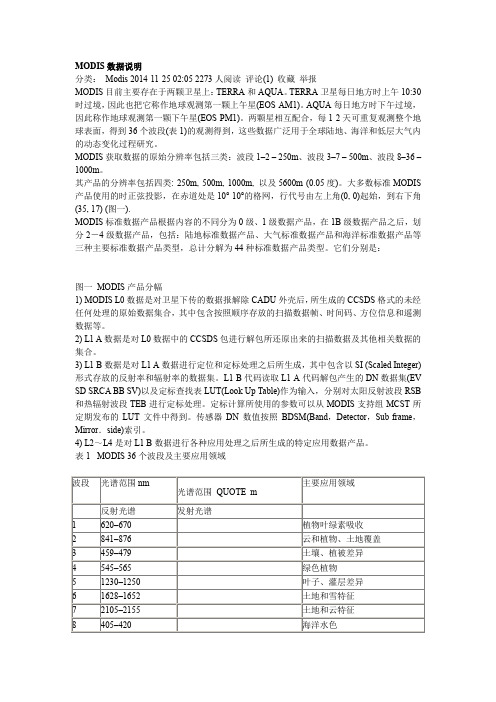
MODIS数据说明分类:Modis 2014-11-25 02:05 2273人阅读评论(1) 收藏举报MODIS目前主要存在于两颗卫星上:TERRA和AQUA。
TERRA卫星每日地方时上午10:30时过境,因此也把它称作地球观测第一颗上午星(EOS-AM1)。
AQUA每日地方时下午过境,因此称作地球观测第一颗下午星(EOS-PM1)。
两颗星相互配合,每1-2天可重复观测整个地球表面,得到36个波段(表1)的观测得到,这些数据广泛用于全球陆地、海洋和低层大气内的动态变化过程研究。
MODIS获取数据的原始分辨率包括三类:波段1–2 – 250m、波段3–7 – 500m、波段8–36 –1000m。
其产品的分辨率包括四类: 250m, 500m, 1000m, 以及5600m (0.05度)。
大多数标准MODIS 产品使用的时正弦投影,在赤道处是10° 10°的格网,行代号由左上角(0, 0)起始,到右下角(35, 17) (图一).MODIS标准数据产品根据内容的不同分为0级、1级数据产品,在1B级数据产品之后,划分2-4级数据产品,包括:陆地标准数据产品、大气标准数据产品和海洋标准数据产品等三种主要标准数据产品类型,总计分解为44种标准数据产品类型。
它们分别是:图一MODIS产品分幅1) MODIS L0数据是对卫星下传的数据报解除CADU外壳后,所生成的CCSDS格式的未经任何处理的原始数据集合,其中包含按照顺序存放的扫描数据帧、时间码、方位信息和遥测数据等。
2) L1 A数据是对L0数据中的CCSDS包进行解包所还原出来的扫描数据及其他相关数据的集合。
3) L1 B数据是对L1 A数据进行定位和定标处理之后所生成,其中包含以SI (Scaled Integer)形式存放的反射率和辐射率的数据集。
L1 B代码读取L1 A代码解包产生的DN数据集(EV SD SRCA BB SV)以及定标查找表LUT(Look Up Table)作为输入,分别对太阳反射波段RSB 和热辐射波段TEB进行定标处理。
定量遥感-MODIS遥感影像数据介绍

1000
1000 1000 1000 1000 1000 1000 1000
6.535-6.895
1000
11
12 13h 13l
526-536
546-556 662-672 662-672
1000
1000 1000 1000
28
29 30 31
7.175-7.475
8.400-8.700 9.580-9.880 10.78011.280
所获取的MODIS数据,是特定卫星(Terra, Aqua)、 特定产品(44种之一)、特定版本的数据。 通常所说的MODIS一般为1B,只是44种产品之一。 按照等级, 44种产品分别采用不同数据格式,需要 不同处理软件。
标定产品
大气产品
陆地产品
冰雪产品
海洋产品
MODIS L0数据是对卫星下传的数据报解除外壳后, 所生成的CCSDS格式的未经任何处理的原始数据集合 ,其中包含按照顺序存放的扫描数据帧、时间码、 方位信息和遥测数据等。 LlA数据是对L0数据中的CCSDS包进行解包所还原出 来的扫描数据及其他相关数据的集合。 LlB数据是对LlA数据进行定位和定标处理之后所生成 ,其中包含以SI(Scaled Integer)形式存放的反射率和 辐射率的数据集。
545-565 1230-1250 1628-1652 2105-2155 405-420 438-448 483-493
500
500 500 500 500 1000 1000 1000
20
21 22 23 24 25 26 27 1360-1390
3.660-3.840
3.929-3.989 3.929-3.989 4.020-4.080 4.433-4.498 4.482-4.549
MODIS土地覆盖数据下载
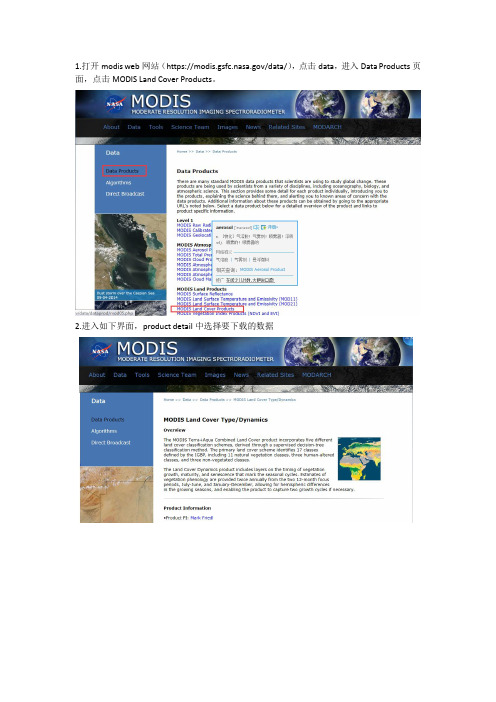
4.进入如下页面,设置搜索项、时间范围、空间范围,选择需要的数据集,点击
5.进入筛选的数据界面后,添加需要的数据到cart,进入下一页面,如下图所示,点击download,保存所需的数据链接,打开下载的txt文件,进行土地覆盖数据的下载
6.数据的信息可参照/s/blog_764b1e9d0100pz8i.html
进入如下页面设置搜索项时间范围空间范围选择需要的数据集点击进入筛选的数据界面后添加需要的数据到cart进入下一页面如下图所示点击download保存所需的数据链接打开下载的txt文件进行土地覆盖数据的下载6
1.打开modis web网站(https:///data/),点击data,进入Data Products页面,点击MODIS Land Cover Products。
第一次全国水利普查技术细则
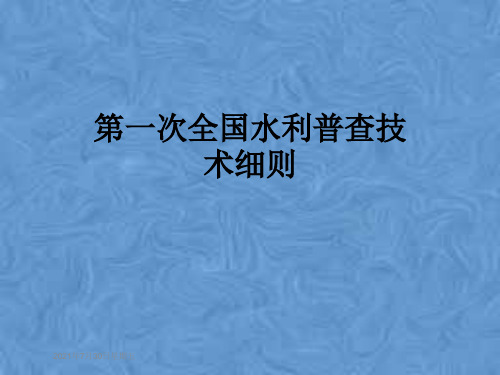
(2)MODIS辅助法
技术流程:
利用已经进行大气纠正的现成遥感图像数据
产品对卫星影像进行大气纠正得到地表反射率。
1)采用MODIS星上定标产品得到MODIS图像获取时 太阳在大气顶的辐照度,将定标好的MODIS L1B辐亮度 产品MOD02转换为大气顶的表观反射率;
2.1.2 MODIS数据
MODIS数据校正,利用头文件信息直接将地理 坐标信息赋给图像,采用ENVI软件完成。
2.1.3 Aqua/AMSR-E亮温数据
Aqua/AMSR-E数据校正,利用头文件信息直 接将地理坐标信息赋给图像,在ENVI软件完成。
L0
2.2 大气效应纠正
2.2.1 图像信息获取
2. 遥感数据预处理
2.1 几何精校正
2.1.1 HJ-1-A、HJ-1-B数据
几何精校正流程图
(1) 数据挑选
数据源选取需能有效的覆盖普查范围,保证数据质量和 方便色调处理。具体标准有:
1)相邻区域时相一致或相近的图像;
2)图幅方正,图像清晰,地物层次分明,色调均一;
3)图像没有坏行缺带,没有条带、斑点噪声和耀斑;
多源空间数据整合
多要素整合
➢ 气象 ➢ 地形 ➢ 土壤 ➢ 植被 ➢ 水土保持措施
多数据类型整合
➢ 矢量:土地利用图、土壤图 ➢ 栅格:DEM、遥感影像 ➢ 属性:气象、土壤属性、水保措施
土壤侵蚀量
降雨 +
措施 +
植被 +
地形 +
土壤
MODIS 1km
HJ-1 30m BJ-1 32m 中巴02B 19.5m
MODIS植被指数数据产品
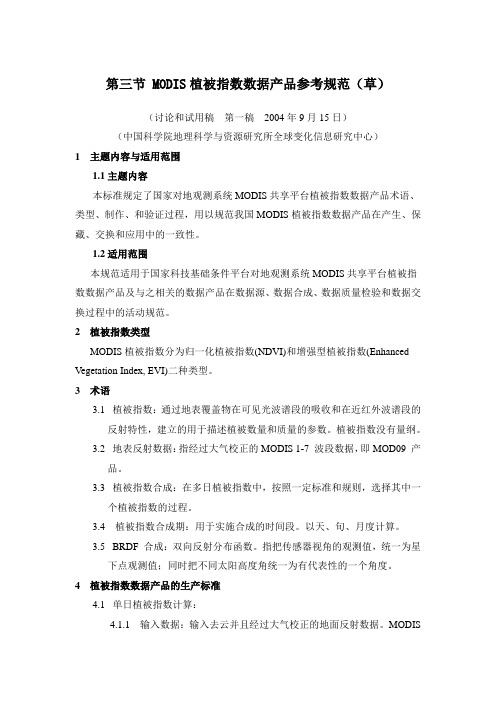
第三节 MODIS植被指数数据产品参考规范(草)(讨论和试用稿第一稿2004年9月15日)(中国科学院地理科学与资源研究所全球变化信息研究中心)1主题内容与适用范围1.1主题内容本标准规定了国家对地观测系统MODIS共享平台植被指数数据产品术语、类型、制作、和验证过程,用以规范我国MODIS植被指数数据产品在产生、保藏、交换和应用中的一致性。
1.2适用范围本规范适用于国家科技基础条件平台对地观测系统MODIS共享平台植被指数数据产品及与之相关的数据产品在数据源、数据合成、数据质量检验和数据交换过程中的活动规范。
2植被指数类型MODIS植被指数分为归一化植被指数(NDVI)和增强型植被指数(Enhanced Vegetation Index, EVI)二种类型。
3术语3.1植被指数:通过地表覆盖物在可见光波谱段的吸收和在近红外波谱段的反射特性,建立的用于描述植被数量和质量的参数。
植被指数没有量纲。
3.2地表反射数据:指经过大气校正的MODIS 1-7 波段数据,即MOD09 产品。
3.3植被指数合成:在多日植被指数中,按照一定标准和规则,选择其中一个植被指数的过程。
3.4植被指数合成期:用于实施合成的时间段。
以天、旬、月度计算。
3.5BRDF合成:双向反射分布函数。
指把传感器视角的观测值,统一为星下点观测值;同时把不同太阳高度角统一为有代表性的一个角度。
4植被指数数据产品的生产标准4.1单日植被指数计算:4.1.1输入数据:输入去云并且经过大气校正的地面反射数据。
MODIS1-7 波段。
其中:1-2波段空间分辨率250m,3-7波段空间分辨率500m。
4.1.2植被指数定义及计算公式:NDVI =(B2-B1)/(B2+B1)EVI =2.5*(B2-B1)/(B2+6*B1–7.5*B3 + 1)其中:NDVI:归一化植被指数EVI:增强型植被指数B1:MODIS第1波段B2:MODIS第2波段B3:MODIS第3波段4.1.3输出数据:NDVI和EVI,日数据,空间分辨率250米。
全球30m地表覆盖产品Global Landcover datset 30-2010数据说明
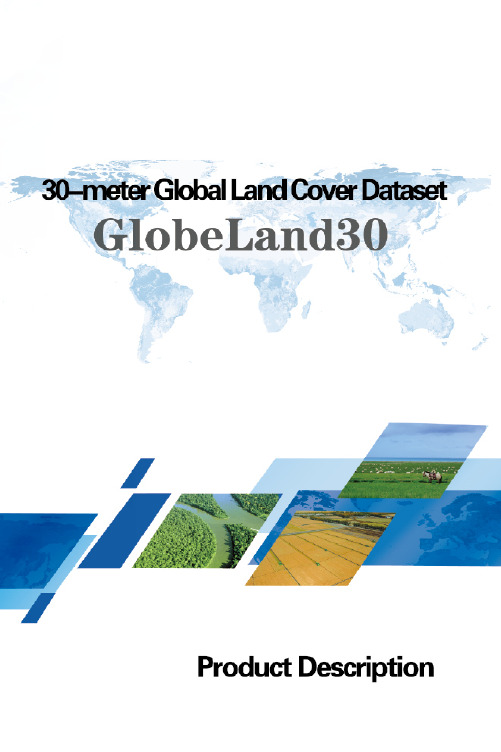
全球30米地表覆盖数据(GlobeLand30)产品说明国家基础地理信息中心二○一四年五月目录1.项目简介 (1)2. 分类影像和参考资料 (1)2.1 分类影像 (1)2.2 辅助数据 (4)3.类型定义与赋值 (7)3.1类型定义 (7)3.2类型赋值 (8)4分类技术指标 (9)5. 分类策略与方法 (10)5.1分类策略 (10)5.2分类方法 (11)6. GlobeLand30产品 (12)6.1 参考坐标系 (12)6.2 数据分幅 (13)6.3数据组成及格式 (13)6.4文件命名与组织 (15)7. 精度自评估 (16)7.1抽样方案 (16)7.2 自评估精度 (16)8. 致谢 (18)附录:全球地表覆盖数据产品元数据表结构 (19)i1.概述地表覆盖是指地球表面各种物质类型及其自然属性与特征的综合体,其空间分布反映着人类社会经济活动过程,决定着地表的水热和物质平衡,其变化直接影响到生物地球化学循环,改变着陆地-大气的水分、能量和碳循环,甚至影响气候变化。
科学准确地测定全球地表覆盖的空间分布与动态变化,对于全球变化研究,如地球系统的能量平衡、碳循环及其它生物地球化学循环、气候变化等,有着十分重要的意义。
为了有效地支撑全球变化研究和地球系统模式发展,科技部在2010年启动了 863计划“全球地表覆盖遥感制图与关键技术研究”重点科研项目。
项目由国家基础地理信息中心牵头,来自测绘局、中科院、教育部、农业部、林业局等7个部门的18家单位共同参与。
2013年底, 2010基准年的30米全球地表覆盖遥感制图数据产品(GlobeLand30-2010)研制完成。
该数据覆盖南北纬80度的陆地范围,包括耕地、森林、草地、灌木地、湿地、水体、苔原、人造地表、裸地、冰川和永久积雪等10种地表覆盖类型。
本手册是对GlobeLand30-2010产品进行简要介绍的材料,包括所用的分类影像、参考资料、影像处理方法、分类方法、数据产品组织和精度评估情况等,供国内外学者和相关人员参考使用。
MODIS土地利用数据在CALPUFF中的应用

MODIS土地利用数据在CALPUFF中的应用下垫面( underlying surface) 是指与大气下层直接接触的地球表面。
大气圈以地球的水陆表面为其下界,称为大气层的下垫面。
在模拟区域和污染源确定的情况下,下垫面参数是影响大气扩散最重要的参数,对污染物浓度预测结果影响很大。
各大气质量模型都需要土地利用数据。
1. AERMOD土地利用数据介绍在AERMOD模型中,下垫面参数通过粗糙度、反照率和波文比表示,共分有8中不同的土地利用类型,还可以根据气候情况干燥、半湿润和湿润来选取不同的参数,这些参数在导则附录中已按春夏秋冬四季给出明确数值。
在使用AERMOD模型预测中,由于一般情况下预测范围较小,我们手动设定其地表参数即可,将预测区域以原点为中心划分扇区,最多划分12个扇区,每个扇区的下垫面特征可以根据半径3km的情况进行确定。
2. CALPUFF模型下垫面参数介绍CALPUFF模型中使用更多的参数来表述下垫面,下垫面参数采用粗糙度、反照率、反照率、土壤热通量、人类活动热通量和叶面积指数表示。
土地利用类型基于美国地表土地利用分类系统,分为14种,土地利用类型及其参数见下表。
2.1. GLCC土地利用数据介绍CALPUFF模型中所有参数均为网格化的,下垫面参数也不例外,故需要给每个网格赋值一个下垫面参数,CALPUFF考虑到我们给每个网格设置参数,在模拟网格数很多时,实在很不方便,而且在大多情况下我们也难于判断每个网格的下垫面,故它提供了一个前处理程序,程序可以读取预定格式的土地利用数据,给出每个网格的下垫面参数,CALPUFF官方怕我们找不到预定格式的土地利用数据更是贴心的给我们提供全球范围的土地利用数据,而且数据还是免费的,数据如下:石家庄庄为例,坐标采用UTM(北半球50区),范围60km*40km。
采用CALPUFF提供的预处理程序CTGPROC V2.684版本,得出结果如下:2.2MODIS数据介绍鉴于以上情况。
esri 10m land cover和modis土地利用分类
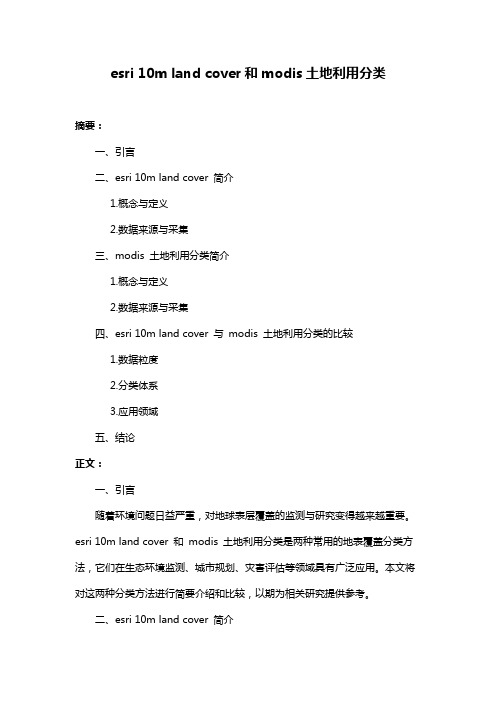
esri 10m land cover和modis土地利用分类摘要:一、引言二、esri 10m land cover 简介1.概念与定义2.数据来源与采集三、modis 土地利用分类简介1.概念与定义2.数据来源与采集四、esri 10m land cover 与modis 土地利用分类的比较1.数据粒度2.分类体系3.应用领域五、结论正文:一、引言随着环境问题日益严重,对地球表层覆盖的监测与研究变得越来越重要。
esri 10m land cover 和modis 土地利用分类是两种常用的地表覆盖分类方法,它们在生态环境监测、城市规划、灾害评估等领域具有广泛应用。
本文将对这两种分类方法进行简要介绍和比较,以期为相关研究提供参考。
二、esri 10m land cover 简介1.概念与定义esri 10m land cover 是一种基于地理信息系统(GIS)的高分辨率地表覆盖分类方法,数据分辨率为10 米。
它将地球表面的覆盖类型划分为多个类别,如森林、草地、水域、城市等。
2.数据来源与采集esri 10m land cover 的数据来源于多个遥感平台和传感器,如Landsat、Sentinel 等。
数据采集方式包括卫星遥感、航空遥感等。
三、modis 土地利用分类简介1.概念与定义modis 土地利用分类是基于MODIS(Moderate Resolution Imaging Spectroradiometer)遥感数据的地球表面覆盖分类方法。
它将地表覆盖划分为多个类别,如森林、草地、水域、城市等。
2.数据来源与采集modis 土地利用分类的数据来源于美国国家航空航天局(NASA)的Terra 和Aqua 卫星上的MODIS 传感器。
数据采集方式为卫星遥感。
四、esri 10m land cover 与modis 土地利用分类的比较1.数据粒度esri 10m land cover 的数据分辨率为10 米,而modis 土地利用分类的数据分辨率为500 米。
esri 10m land cover和modis土地利用分类

esri 10m land cover和modis土地利用分类
(原创实用版)
目录
1.介绍 ESRI 10m 土地覆盖和 MODIS 土地利用分类
2.ESRI 10m 土地覆盖的特点
3.MODIS 土地利用分类的特点
4.两者的比较
5.应用领域
正文
ESRI 10m 土地覆盖和 MODIS 土地利用分类是两种常用的遥感数据
产品,它们在土地利用和覆盖研究中扮演着重要的角色。
ESRI 10m 土地覆盖数据是由 ESRI 公司开发的,其空间分辨率为 10 米,涵盖了全球范围内的陆地地区。
该数据集包含了 15 种主要的土地覆盖类型,如森林、草地、水体、城市等,能够较为准确地反映出地球表面的土地利用状况。
MODIS 土地利用分类数据则是由美国宇航局(NASA)开发的,其空间分辨率也为 10 米。
该数据集包含了 16 种土地利用类型,除了常见的森林、草地、水体等类型外,还包括了一些特殊类型的土地利用,如灌木地、裸地等。
尽管 ESRI 10m 土地覆盖和 MODIS 土地利用分类的空间分辨率相同,但它们在数据类型、分类方式等方面存在一些差异。
例如,ESRI 10m 土
地覆盖数据更注重土地覆盖的类型,而 MODIS 土地利用分类数据则更注
重土地的利用方式。
在应用领域方面,ESRI 10m 土地覆盖和 MODIS 土地利用分类都广泛应用于环境研究、城市规划、农业管理等领域。
例如,科研人员可以利用
这些数据研究全球范围内的土地利用变化,或者通过比较不同地区的土地利用类型和方式,寻找出最适合某种农作物生长的土地。
modis土地覆盖分类
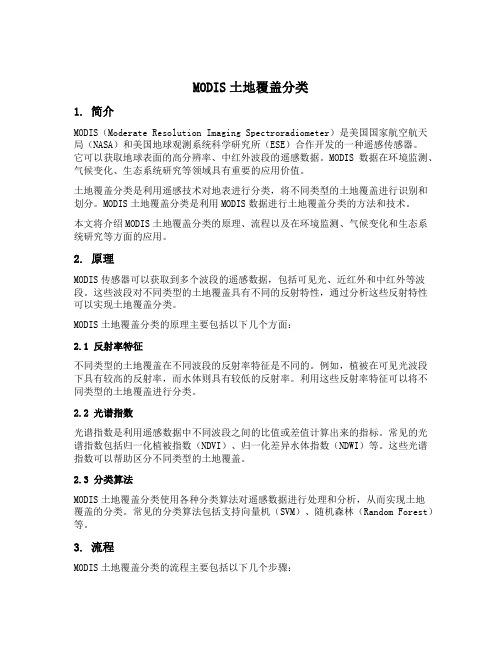
MODIS土地覆盖分类1. 简介MODIS(Moderate Resolution Imaging Spectroradiometer)是美国国家航空航天局(NASA)和美国地球观测系统科学研究所(ESE)合作开发的一种遥感传感器。
它可以获取地球表面的高分辨率、中红外波段的遥感数据。
MODIS数据在环境监测、气候变化、生态系统研究等领域具有重要的应用价值。
土地覆盖分类是利用遥感技术对地表进行分类,将不同类型的土地覆盖进行识别和划分。
MODIS土地覆盖分类是利用MODIS数据进行土地覆盖分类的方法和技术。
本文将介绍MODIS土地覆盖分类的原理、流程以及在环境监测、气候变化和生态系统研究等方面的应用。
2. 原理MODIS传感器可以获取到多个波段的遥感数据,包括可见光、近红外和中红外等波段。
这些波段对不同类型的土地覆盖具有不同的反射特性,通过分析这些反射特性可以实现土地覆盖分类。
MODIS土地覆盖分类的原理主要包括以下几个方面:2.1 反射率特征不同类型的土地覆盖在不同波段的反射率特征是不同的。
例如,植被在可见光波段下具有较高的反射率,而水体则具有较低的反射率。
利用这些反射率特征可以将不同类型的土地覆盖进行分类。
2.2 光谱指数光谱指数是利用遥感数据中不同波段之间的比值或差值计算出来的指标。
常见的光谱指数包括归一化植被指数(NDVI)、归一化差异水体指数(NDWI)等。
这些光谱指数可以帮助区分不同类型的土地覆盖。
2.3 分类算法MODIS土地覆盖分类使用各种分类算法对遥感数据进行处理和分析,从而实现土地覆盖的分类。
常见的分类算法包括支持向量机(SVM)、随机森林(Random Forest)等。
3. 流程MODIS土地覆盖分类的流程主要包括以下几个步骤:3.1 数据获取和预处理首先需要获取MODIS传感器获取到的遥感数据,包括不同波段的反射率数据。
获取到的数据需要进行预处理,包括辐射校正、大气校正等,以提高数据的质量。
MODIS Land Cover Dynamics (MCD12Q2) 产品用户手册说明书
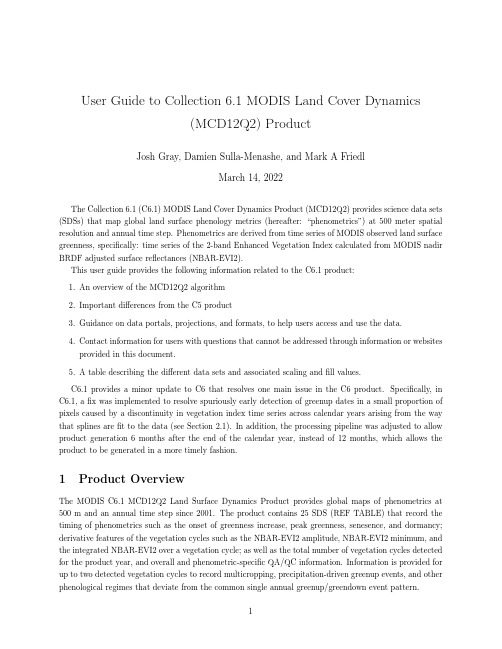
User Guide to Collection6.1MODIS Land Cover Dynamics(MCD12Q2)ProductJosh Gray,Damien Sulla-Menashe,and Mark A FriedlMarch14,2022The Collection6.1(C6.1)MODIS Land Cover Dynamics Product(MCD12Q2)provides science data sets (SDSs)that map global land surface phenology metrics(hereafter:“phenometrics”)at500meter spatial resolution and annual time step.Phenometrics are derived from time series of MODIS observed land surface greenness,specifically:time series of the2-band Enhanced Vegetation Index calculated from MODIS nadir BRDF adjusted surface reflectances(NBAR-EVI2).This user guide provides the following information related to the C6.1product:1.An overview of the MCD12Q2algorithm2.Important differences from the C5product3.Guidance on data portals,projections,and formats,to help users access and use the data.4.Contact information for users with questions that cannot be addressed through information or websitesprovided in this document.5.A table describing the different data sets and associated scaling and fill values.C6.1provides a minor update to C6that resolves one main issue in the C6product.Specifically,in C6.1,a fix was implemented to resolve spuriously early detection of greenup dates in a small proportion of pixels caused by a discontinuity in vegetation index time series across calendar years arising from the way that splines are fit to the data(see Section2.1).In addition,the processing pipeline was adjusted to allow product generation6months after the end of the calendar year,instead of12months,which allows the product to be generated in a more timely fashion.1Product OverviewThe MODIS C6.1MCD12Q2Land Surface Dynamics Product provides global maps of phenometrics at 500m and an annual time step since2001.The product contains25SDS(REF TABLE)that record the timing of phenometrics such as the onset of greenness increase,peak greenness,senesence,and dormancy; derivative features of the vegetation cycles such as the NBAR-EVI2amplitude,NBAR-EVI2minimum,and the integrated NBAR-EVI2over a vegetation cycle;as well as the total number of vegetation cycles detected for the product year,and overall and phenometric-specific QA/QC rmation is provided for up to two detected vegetation cycles to record multicropping,precipitation-driven greenup events,and other phenological regimes that deviate from the common single annual greenup/greendown event pattern.1.1Differences from Collection5ProductThe C6.1methodological approach and SDSs differ substantially from the previous C5product.Changes were designed to better capture phenometrics in systems with multiple vegetation cycles per year,to increase the reliability of retrieved phenometrics in tropical,arid,and semi-arid ecosystems,to more accurately represent phenometrics in systems where NBAR-EVI2time series do not closely resemble logistic growth patterns,to provide working QA/QC SDS,and to deliver phenometrics in a more usable and intuitive fashion when vegetation cycles cross calendar boundaries,especially in the Southern ers of C5products should note the additional SDS,the provision of overall and bit-packed,phenometric-specific QA/QC SDSs,the change to a days since1-1-1970standard for date delivery,and the adoption of a new standard for assigning vegetation cycles to product years:all phenometrics are delivered for vegetation cycles where the date of peak NBAR-EVI2is within the product’s calendar year.This particular change should ease difficulties in collating phenometrics across product years when vegetation cycles cross calendar year boundaries by ensuring that all phenometrics for a vegetation cycle are fully contained in a single product year.2MethodologyThe C6.1MCD12Q2product is created by assembling time series of NBAR-EVI2,eliminating outliers and filling dormant period values,fitting a QA/QC-weighted penalized cubic smoothing spline to the time series, identifying valid vegetation cycles within the time series,and then extracting and recording phenometrics for each vegetation cycle.All subsequent functions are applied to a time series of three consecutive years: the product year and preceding and subsequent years.2.1Spline FitNBAR-EVI2observations identified as being snow contaminated by the MCD432product,or where the corresponding NBAR-NDSI>−0.2,are filled with a dormant period value.The dormant NBAR-EVI2is taken to be the5th percentile snow-free value across the product year and its preceding and subsequent year,unless this differs by more than25%from the10th percentile snow-free value for the central year,in which case only the central year’s data are used to compute the background NBAR-EVI2value.This is to accommodate land cover changes that may alter the true dormant period NBAR-EVI2value.Missing NBAR-EVI2values between snow-contaminated observations are also filled with the dormant NBAR-EVI2 value.Finally,a penalized cubic smoothing spline is fit to the value with the weights proportional to the MCD43A2QA/QC flags.2.2Identifying Valid Vegetation CyclesValid vegetation phases in the time series are identified as periods of sustained increase in NBAR-EVI2 followed by sustained periods of decrease,subject to ecologically meaningful heuristics concerning the length of these periods and magnitude of the NBAR-EVI2amplitude.First,“peaks”in the NBAR-EVI2time seres are identified as points where the first derivative(numerically evaluated)changes sign(Fig.1A).These candidate peaks are sorted by NBAR-EVI2magnitude and then analyzed with a recursive function that attempts to identify associated start and end dates for the greenup/down segment.Figure1:Three years of a hypothetical splined NBAR-EVI2time series with potential peaks(upper-case letters),troughs(lower-case letters),and search windows for start/end of the segment corresponding to peak F marked(A).Diagram of phenometrics retrieved for a single hypothetical vegetation cycle(B).Illustration of the methodology used to determine the phenometric-specific QA/QC score(C).Starting with the smallest peak,the start of the greenup segment is sought as the date of the minimum NBAR-EVI2value closest to the candidate peak between30days prior to peak and the maximum of:the start of the time series,the closest of any preceding candidate peaks,or the candidate peak date minus a maximum greenup period length parameter(185days).This search windows are depicted in Fig.1A for the segment associated with peak F.If the NBAR-EVI2amplitude between this minimum value and the peak is greater than or equal to0.1,and greater than or equal to35%of the time series maximum NBAR-EVI2 minus the minimum NBAR-EVI2,then the identified greenup period is considered valid and an associated greendown period is sought.If these conditions are not met,then the candidate peak is removed from the list of candidate peaks.The end of the greendown period is sought in an identical manner,but in the opposite temporal direction.A candidate end date is identified as the date of the closest minimum NBAR-EVI2 value between30days after the candidate peak and the minimum of:the end of the time series,the closest of any following peaks,or the candidate peak date plus a maximum greendown length parameter(set to 185days;Fig.1A).The entire greenup and greendown segment is considered a valid vegetation cycle ifthe NBAR-EVI2amplitude between the candidate segment end date and candidate peak is greater than orequal to0.1.Otherwise,the candidate peak is eliminated.This process is repeated,each time updating the candidate peak list,until all candidate peaks are either eliminated or identified as belonging to a valid vegetation cycle.The full3-year time series is thus partitioned into valid vegetation cycles.For example,in Fig.1A,the potential peaks would first be sorted by NBAR-EVI2magnitude:D,C,A, G,F,H,E,and B.A segment start for potential peak D would be identified as trough d and the NBAR-EVI2 amplitude between points d and D calculated.Because this amplitude is less than the global minimum of0.1,peak D would be eliminated from the list of potential peaks.Similarly,the segment associated with peakC would be eliminated because although the NBAR-EVI2magnitude between points c and C exceeds0.1, it is less than35%of the maximum NBAR-EVI2variation exhibited over the three-year interval(minimum point d and maximum point B depict an amplitude of0.67,so the35%threshold is0.234,but c to C is0.2). Likewise,the potential segment associated with peak A would be eliminated because while the NBAR-EVI2 amplitude between a and A exceeds0.1and the35%threshold,the greendown portion(points A to b)does not.The segment start/end search windows shown in Fig.1A are for the segment associated with peak F.A segment start is sought as a NBAR-EVI2minimum point between the preceding peak E and30days prior to F.The segment end date is sought between a period30days after F and the next valid peak(G).Valid vegetation cycles would be retrieved for the segments associated with peaks B,E,F,G,and H.2.3Phenometric ExtractionIdentification of phenophase transition dates is performed on each of the identified vegetation cycles for which the peak date falls within the calendar year of the product year(e.g.phenometrics associated with peak F in Fig.1A would be delivered with product year2004).The first metric:number of vegetation cycles, is determined by simply counting the number of such peaks.Subsequent phenophase date retrieval,and quantification of associated QA,are performed only for up to two of the valid segments with highest segment NBAR-EVI2amplitude.That is,if there are three cycles,the number of vegetation cycles metric will reflect the correct number,but phenophase transitions will not be retrieved for the cycle with the lowest NBAR-EVI2amplitude(e.g.product year2004for the time series represented in Fig.1A would have3for number of cycles,but only phenometrics for segments associated with peaks F and H would be delivered).The start of greenup,greenup midpoint,and maturity dates are retrieved as the first date within the greenup segment where the NBAR-EVI2time series crosses15,50,and90%of the greenup segment NBAR-EVI2amplitude (peak NBAR-EVI2-segment start EVI;Fig.1B).Similarly,start of senescence,senescence midpoint,and dormancy are retrieved as the last date within the greendown segment where the NBAR-EVI2time series crosses90,50,and15%of the greendown segment NBAR-EVI2amplitude(peak NBAR-EVI2-segment end NBAR-EVI2;Fig.1B).All dates are converted to UNIX-epoch time:days since Jan1,1970.Segment integrated NBAR-EVI2is calculated as the sum of daily NBAR-EVI2values,minus the greenup segment minimum value,between the segment start and end dates(not from the start of greenup and dormancy;Fig. 1B).NBAR-EVI2amplitude and NBAR-EVI2minimum values are also recorded as distinct product layers (NBAR-EVI2maximum can be retrieved as NBAR-EVI2amplitude-NBAR-EVI2minimum).Quality assurance(QA)scores are calculated for each phenometric(detailed QA),and for the entire greenup/down segment(overall QA).These scores are a weighted combination of the fraction of not missing (e.g.due to snow)and not filled(e.g.with dormant NBAR-EVI2value)values(80%weight),and the spline goodness-of-fit(R2;20%weight),in the vicinity of each phenometric(2weeks before and after;detailed QA;Fig.1C)or within the entire segment(overall QA).These0–1scores are mapped to categories as:[1, 0.75)=0(best),[0.75,0.5)=1(good),[0.5,0.25)=2(fair),and[0.25,0]=3(poor).Detailed QA scores are thenbit-packed into a single16-bit integer value,whereas the overall QA score is retained as a separate product layer(details on detailed QA below).3Data Formats and ProjectionMCD12Q2data are provided as tiles that are approximately10◦x10◦at the Equator using a Sinusoidal grid in HDF4file rmation related to the MODIS sinusoidal projection and the HDF4file format can be found at:•MODIS tile grid:/MODLAND_grid.html•MODIS HDF4:/products/hdf4/Several parameters are needed to reproject the Sinusoidal HDF4files to other projections using widely used software such as GDAL.Here we provide the values used for the upper left corner of the grid,the size of a single pixel,and the Sinusoidal projection string in Cartographic Projections Library(PROJ4)and Well-Known Text(WKT)formats.•ULY Grid=10007554.677,ULX Grid=-20015109.354•Pixel Size(m)=463.312716525•Number of Pixels per Tile=2400•Projection InformationPROJ4:‘+proj=sinu+a=6371007.181+b=6371007.181+units=m’WKT:PROJCS["Sinusoidal",GEOGCS["GCS_unnamed ellipse",DATUM["D_unknown",SPHEROID["Unknown",6371007.181,0]],PRIMEM["Greenwich",0],UNIT["Degree",0.017453292519943295]],PROJECTION["Sinusoidal"],PARAMETER["central_meridian",0],PARAMETER["false_easting",0],PARAMETER["false_northing",0],UNIT["Meter",1]3.1Accessing MODIS Data ProductsSeveral ways to access the MODIS data products are listed below.More info about the data sets,data formats,and quality information are available from the Land Processes DAAC.For MCD12Q2the link is https:///dataset_discovery/modis/modis_products_table/mcd12q2_v006•Bulk download:LP DAAC Data Pool and DAAC2Disk.•Search and browse:USGS EarthExplorer and NASA Earthdata Search.3.2Known Issues and Sources of Uncertainty•Greenup and Dormancy phenometrics are anomalously early/late in some high-latitude regions with low-amplitude NBAR-EVI2variation.This likely results from the splining approach assuming a dor-mant period NBAR-EVI2value that is too low.The cause and a fix are being investigated,but until a fix is devised,users are encouraged to use the more realistic and stable MidGreenup and MidGreendown metrics to capture season start/end in these regions(e.g.high-latitude evergreen forests).•In some semi-arid and arid environments exhibiting low-amplitude NBAR-EVI2variation,there are large differences in filled pixels between the Collection5and Collection6products.Collection6 typically retrieves fewer pixels because it applies a global minimum NBAR-EVI2amplitude requirement of0.1in addition to the relative amplitude requirements in Collection5(valid segments must have NBAR-EVI2amplitude that is at least35%of the3-year max-min.)•For pixels with more than two valid vegetation cycles,the two cycles that are documented in the product may not be temporally adjacent.Instead,they are the two cycles with the largest NBAR-EVI2amplitudes.That is,the two cycles may have a temporally intervening cycle of lower amplitude.•Because heterogeneous land cover/use results in NBAR-EVI2time series that are a mixture of perhaps distinct phenologies,users should be careful in interpreting multi-cycle results.They are not,for example,guaranteed to represent two distinct cropping cycles,but may instead capture two different sub-pixel scale fields with very different sow/harvest dates.•Users may notice substantial differences in patterns of missing data between Collections5and6in the Southern Hemisphere.This is mostly a result of the difference in methodology whereby phenometrics are assigned to particular product years.Collection5phenometrics are delivered with the product year associated with the year of the particular phenometric,and so the phenometrics associated witha particular vegetation cycle may be spread across multiple product years.In Collection6,the pheno-metrics for a particular cycle are always kept together,and delivered with the product year associated with the calendar year in which the Peak phenometric falls.4Contact InformationUser Contact:•Josh Gray(****************)•Mark Friedl(*************)5Science Data SetsTable1describes the Science Data Sets contained in the MCD12Q2Collection6product.All SDS have a 16-bit integer data type.All except”NumCycles”contain two data layers corresponding to up to two valid vegetation cycles.Overall QA can be interpreted directly as an integer,but Detailed QA is bit-packed and so must be unpacked for proper interpretation(details and examples below).The QA Overall SDS provides a simple representation of the quality of retrievals for a particular vegeta-tion cycle.As previously described,it is calculated from a weighted combination of the fraction of missingT a b l e 1:M C D 12Q 2S c i e n c e D a t a S e t s .S D S N a m e D e s c r i p t i o n L a y e r s D a t a T y p e U n i t s V a l i d R a n g e F i l l V a l u e S c a l e F a c t o r N u m C y c l e sT o t a l n u m b e r o f v a l i d v e g -e t a t i o n c y c l e s w i t h p e a k i n p r o d u c t y e a r 1I N T 16n o n e 1–7327671G r e e n u p D a t e w h e n E V I 2fi r s t c r o s s e d 15%o f t h e s e g -m e n t E V I 2a m p l i t u d e 2I N T 16d a y s s i n c e 1-1-197011138–32766327671M i d G r e e n u p D a t e w h e n E V I 2fi r s t c r o s s e d 50%o f t h e s e g -m e n t E V I 2a m p l i t u d e 2I N T 16d a y s s i n c e 1-1-197011138–32766327671M a t u r i t y D a t e w h e n E V I 2fi r s t c r o s s e d 90%o f t h e s e g -m e n t E V I 2a m p l i t u d e 2I N T 16d a y s s i n c e 1-1-197011138–32766327671P e a kD a t e w h e nE V I 2r e a c h e d t h e s e g m e n t m a x i m u m 2I N T 16d a y s s i n c e 1-1-197011138–32766327671S e n e s c e n c eD a t e w h e nE V I 2l a s t c r o s s e d 90%o f t h e s e g -m e n t E V I 2a m p l i t u d e 2I N T 16d a y s s i n c e 1-1-197011138–32766327671M i d G r e e n d o w n D a t e w h e n E V I 2l a s t c r o s s e d 50%o f t h e s e g -m e n t E V I 2a m p l i t u d e 2I N T 16d a y s s i n ce 1-1-197011138–32766327671D o r m a n c y D a t e w h e nE V I 2l a s t c r o s s e d 15%o f t h e s e g -m e n t E V I 2a m p l i t u d e 2I N T 16d a y s s i n ce 1-1-197011138–32766327671E V I M i n i m u m S e g m e n t m i n i m u m E V I 2v a l u e 2I N T 16N B A R -E V I 20–10000327670.0001E V I A m p l i t u d eS e g m e n t m a x i m u m -m i n -i m u m E V I 22I N T 16N B A R -E V I 20–10000327670.0001E V I A r e aS u m o f d a i l y i n t e r p o l a t e d E V I 2f r o m G r e e n u p t o D o r m a n c y 2I N T 16N B A R -E V I 20–3700327670.1Q A O v e r a l l Q A c o d e f o r e n t i r e s e g -m e n t 2I N T 16n o n e0–3327671Q A D e t a i l e dB i t -p a c k e d ,S D S -s p e c i fi c Q A c o d e s2I N T 16n o n e0–16383327671or filled NBAR-EVI2data in the cycle,and the goodness of fit of the smoothing spline.Values are in the range0–3corresponding to“best”,“good”,“fair”,and“poor”.The QA Detailed SDS contains bit-packed QA information for each date phenometric(e.g.Greenup,MidGreenup,etc.).Individual QA values have an identical interpretation as QA Overall.However,accessing these scores requires the user to convert the 16-bit integer value to binary,and then retrieve specific bit pairs that may then be converted to an integerin the range0–3.Figure2provides a visual example of this conversion,and Table2provides some examplesof arbitrary QA scores and their16-bit integer value to aid users in verifying their interpretation.Figure2:An example QA Detailed16-bit integer value,its binary representation,and associated phenometric-specific bit pair interpretation.Table2:Detailed QA example valuesGreenup MidGreenup Maturity Peak Senescence MidGreendown Dormancy Value 00000000 11111115461 321123315963 120102314409 333333316383Here is an example R function that converts raw values of QA Detailed to a vector of0–3quality scoresin the order:Greenup,MidGreenup,Maturity,Peak,Senescence,MidGreendown,Dormancy:UnpackDetailedQA<-function(x){bits<-as.integer(intToBits(x))quals<-sapply(seq(1,16,by=2),function(i)sum(bits[i:(i+1)]*2^c(0,1)))[1:7]return(quals)}。
- 1、下载文档前请自行甄别文档内容的完整性,平台不提供额外的编辑、内容补充、找答案等附加服务。
- 2、"仅部分预览"的文档,不可在线预览部分如存在完整性等问题,可反馈申请退款(可完整预览的文档不适用该条件!)。
- 3、如文档侵犯您的权益,请联系客服反馈,我们会尽快为您处理(人工客服工作时间:9:00-18:30)。
MOD12Q1三级数据土地覆盖类型产品(Land Cover data)是根据一年的Terra和Aqua 观测所得的数据经过处理,描述土地覆盖的类型。
该土地覆盖数据集中包含了17个主要土地覆盖类型,根据国际地圈生物圈计划(IGBP),其中包括11个自然植被类型,3个土地开发和镶嵌的地类和3个非草木土地类型定义类。
MODIS Terra + Aqua三级土地覆盖类型年度全球500米产品MCD12Q1采用五种不同的土地覆盖分类方案,信息提取主要技术是监督决策树分类。
下面是该数据中包含的五个数据集,五个分类方案如下:
土地覆盖分类1:IGBP的全球植被分类方案
土地覆盖分类2:美国马里兰大学(UMD格式)方案
土地覆盖分类3:基于MODIS叶面积指数/光合有效辐射方案
土地覆盖分类4:基于MODIS衍生净初级生产力(NPP)方案
土地覆盖分类5:植物功能型(肺功能)方案
MCD12Q1是HDF格式的,包含16个数据集
前五个就是五个分类方案的分类图,接下来是分类评估图、其中只有type1有产品,还有质量控制数据集
各种分类数据中,数值代表类别如下表:
(以上数据均出自https:///products/modis_products_table)。