The Regression-Discontinuity Design
关于(模糊)断点回归设计的100篇精选Articles专辑!!!

关于(模糊)断点回归设计的100篇精选Articles专辑邮箱:***********************所有计量经济圈方法论丛的code程序, 宏微观数据库和各种软件都放在社群里.欢迎到计量经济圈社群交流访问.上图为“coronavirus”在世界范围内谷歌搜索趋势.前些日,咱们圈子引荐了①“实证研究中用到的200篇文章, 社科学者常备toolkit”、②实证文章写作常用到的50篇名家经验帖, 学者必读系列、③过去10年AER上关于中国主题的Articles专辑、④AEA 公布2017-19年度最受关注的十大研究话题, 给你的选题方向,受到各位学者欢迎和热议,很多博士生导师纷纷推荐给指导的学生参阅。
继上次,腾讯公司相关部门与因果推断研究小组开展了还算友好的交流后(“BATJ巨头急需大批经济学博士, 望奔走相告”),最近,阿里巴巴相关部门人员也希望在因果推断研究小组交流访问(因果推断研究小组惊动了阿里巴巴!)。
经济学博士在BATJ公司有啥用呢? 难不成比IT程序员还有能耐,正如上文所讲,因果推断在将来很长一段时间里都是科技公司和社科学者使用的主流方法。
我们会一如既往地在小组和社群探讨主流的因果推断方法,同时也欢迎大型科技公司与咱们学者保持更紧密的互动。
之前,咱们小组引荐了1.断点回归设计RDD分类与操作案例,2.RDD断点回归, Stata程序百科全书式的宝典,3.断点回归设计的前沿研究现状, RDD,4.断点回归设计什么鬼?且听哈佛客解析,5.断点回归和读者的提问解答,6.断点回归设计RDD全面讲解, 教育领域用者众多,7.没有工具变量、断点和随机冲击,也可以推断归因,8.找不到IV, RD和DID该怎么办? 这有一种备选方法,9.2卷RDD断点回归使用手册, 含Stata和R软件操作流程,10.DID, 合成控制, 匹配, RDD 四种方法比较, 适用范围和特征,11.安神+克拉克奖得主的RDD论文, 断点回归设计,12.伊斯兰政府到底对妇女友不友好?RDD经典文献,13.PSM,RDD,Heckman,Panel模型的操作程序,14.RDD经典文献, RDD模型有效性稳健性检验,15.2019年发表在JDE上的有趣文章, 计量方法最新趋势与合成控制法(关于合成控制法SCM的33篇精选Articles专辑!)和双重差分法一样(关于双重差分法DID的32篇精选Articles 专辑!),断点回归设计RDD也是当下非常流行的因果推断方法,在英文和中文顶刊中频繁出现。
准实验研究的英语

准实验研究的英语IntroductionExperimental research is a type of research design that involves manipulating one or more variables to observe the effect on another variable. However, in some situations, experimental research may not be feasible or ethical. In such cases, researchers may opt for quasi-experimental research, which is a type of research design that lacks the random assignment of participants to groups. This article explores quasi-experimental research, its types, advantages, and disadvantages.Types of Quasi-Experimental Research1. Pre-Experimental DesignsPre-experimental designs are the simplest type of quasi-experimental designs, and they involve measuring the dependent variable before and after an intervention. There are three types of pre-experimental designs: one-shot design, one-group pretest-posttest design, and static group comparison design.a. One-shot design: In this design, the researcher measures the dependent variable after the intervention. However, there is no control group, and hence, it isdifficult to determine whether the observed change is dueto the intervention or other factors.b. One-group pretest-posttest design: In this design,the researcher measures the dependent variable before and after the intervention. However, there is no control group, and hence, it is difficult to determine whether theobserved change is due to the intervention or other factors.c. Static group comparison design: In this design, the researcher compares the dependent variable in a group that received the intervention and a group that did not receive the intervention. However, the groups are not randomly assigned, and hence, there may be differences between the groups that may affect the results.2. Quasi-Experimental DesignsQuasi-experimental designs involve the manipulation ofan independent variable, but the participants are not randomly assigned to groups. There are four types of quasi-experimental designs: nonequivalent control group design,time-series design, interrupted time-series design, and regression-discontinuity design.a. Nonequivalent control group design: In this design, the researcher compares the dependent variable in a group that received the intervention and a group that did not receive the intervention. However, the groups are not randomly assigned, and hence, there may be differences between the groups that may affect the results.b. Time-series design: In this design, the researcher measures the dependent variable at multiple time points before and after the intervention. However, there is no control group, and hence, it is difficult to determine whether the observed change is due to the intervention or other factors.c. Interrupted time-series design: In this design, the researcher measures the dependent variable at multiple time points before and after the intervention. However, there is a control group, which allows the researcher to determine whether the observed change is due to the intervention or other factors.d. Regression-discontinuity design: In this design, the researcher selects participants based on a cutoff score on a continuous variable. Participants who score above the cutoff score receive the intervention, while those who score below the cutoff score do not receive the intervention. This design allows the researcher to determine whether the observed change is due to the intervention or other factors.Advantages of Quasi-Experimental Research1. Ethical ConsiderationsIn some situations, experimental research may not be ethical. For example, it may not be ethical to manipulate an independent variable that may harm participants. Quasi-experimental research provides an alternative to experimental research, which allows researchers to study the effect of an intervention without compromising the ethical considerations.2. Real-World SettingsQuasi-experimental research is often conducted in real-world settings, which enhances the ecological validity ofthe research findings. This means that the researchfindings are more likely to be applicable to real-world situations.3. Cost-EffectiveQuasi-experimental research is often less costly than experimental research. This is because it does not involve random assignment of participants to groups, which can be time-consuming and costly.Disadvantages of Quasi-Experimental Research1. Lack of ControlQuasi-experimental research lacks the control associated with experimental research. This means that there may be other factors that may affect the results, which may make it difficult to determine whether the observed change is due to the intervention or other factors.2. Selection BiasQuasi-experimental research may suffer from selection bias. This is because participants are not randomly assigned to groups, which may result in differences between the groups that may affect the results.3. Internal ValidityQuasi-experimental research may suffer from internal validity issues. This is because there may be other factors that may affect the results, which may make it difficult to determine whether the observed change is due to the intervention or other factors.ConclusionQuasi-experimental research is a type of research design that lacks the random assignment of participants to groups. It is often used in situations where experimental research may not be feasible or ethical. Quasi-experimental research has advantages such as being ethical, conducted in real-world settings, and cost-effective. However, it also has disadvantages such as lack of control, selection bias, and internal validity issues. Researchers should carefully consider the advantages and disadvantages of quasi-experimental research before deciding on the research design to use.。
断点回归设计的步骤
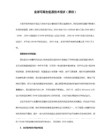
近在做一个需要利用断点回归设计的研究。
为了保证实践的规范性,并且避免未来审稿中可能面对的质疑,花了几天时间梳理了一下断点回归设计的标准操作,整理出来,供来人参考。
本文参考了三篇文献,先摆在这里,建议大家去读原文:第一篇:Lee, and Lemieux, 2010," Regression Discontinuity Designs in Economics ",Journal ofEconomic Literature, Vol. 48: 281–355.第二篇:Pinotti, Paolo. "Clicking on heaven's door: The effect of immigrant legalization oncrime." American Economic Review107.1 (2017): 138-68.第三篇:Thoemmes, Felix, Wang Liao, and Ze Jin. "The Analysis of the Regression-DiscontinuityDesign in R." Journal of Educational and Behavioral Statistics 42.3 (2017): 341-360.1.断点回归常规操作流程第1步检查配置变量(assignment variable,又叫running variable、forcing variable)是否被操纵。
这里的配置变量,其实就是RD中决定是否进入实验的分数(Score),是否被操纵的意思就是,是否存在某种跳跃性的变化。
在实际操作中有两种方式来检验,一是画出配置变量的分布图。
最直接的方法,是使用一定数量的箱体(bin),画出配置变量的历史直方图(histogrm)。
为了观察出分布的总体形状,箱体的宽度要尽量小。
“2+26”城市雾霾治理政策效果评估
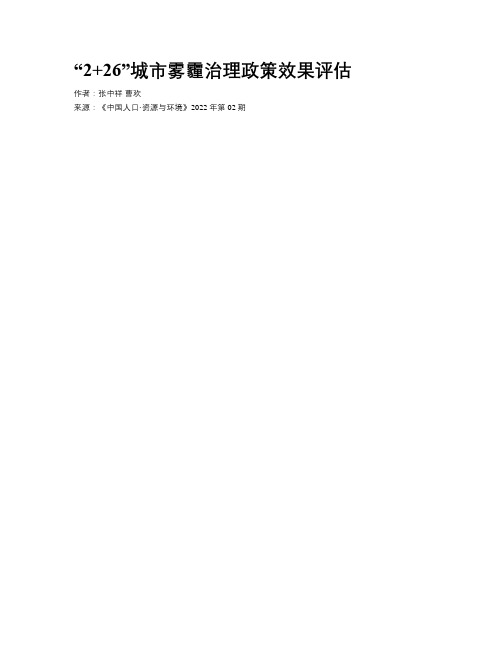
“2+26”城市雾霾治理政策效果评估作者:张中祥曹欢来源:《中国人口·资源与环境》2022年第02期摘要文章将《京津冀及周边地区2017年大气污染防治工作方案》和其后续“攻坚行动方案”的发布作为准自然实验,使用双重差分模型(DID)评估大气污染治理的政策效果。
回归结果发现:①“方案”的发布对于“2+26”城市的空气具有显著的改善作用,并通过了稳健性检验,构成雾霾的主要污染物PM2.5、PM10和AQI 的改善程度最明显,SO2、CO 和NO2的改善幅度次之,但O3浓度在政策处理期内不降反升,说明近年来O3污染程度加剧,亟须引起关注。
②长期视角下SO2和NO2的治理效果较短期情况下相比有所提升,说明有些大气污染物仍然具有进一步改善的潜力,印证了大气污染治理是一项长久的“攻坚战”。
③引入空间DID 分析,通过空间杜宾和双重差分的嵌套模型,放松个体相互独立的假设,从空间维度探讨“方案”的政策效果,对比空间视角下的直接效应与间接效应得出,区域联防联控大气治理手段相比单一地区空气质量改善政策而言能够使得治理效果事半功倍。
④使用中介效应模型,探讨了“方案”通过减少工业产值占GDP 的比重和减少能源消费总量达到空气质量改善的两种作用机制。
最后,文章为接下来进一步有效治理大气污染提出了相关的政策建议。
关键词“2+26”城市;双重差分模型;空间DID;机制分析中图分类号 X51;F061.5 文献标志码 A 文章编号1002-2104(2022)02-0026-11 DOI:10.12062/cpre20211126大气污染是中国经济不断快速发展的一项负外部公共品,在中国,受空气污染问题最多困扰的当属京津冀及周边地区[1-4]。
国务院发布的“十三五”生态环境保护规划中明确强调要“深化区域大气污染联防联控、显著削减京津冀及周边地区颗粒物浓度”,因此京津冀及周边地区成为大气污染防治的重点覆盖区域。
2017年2月17日,原环境保护部发布了《京津冀及周边地区2017年大气污染防治工作方案》(以下简称《2017方案》),形成了以京津冀及周边地区为主导的大气污染防治协作组。
RegressionDiscontinuity回归中断
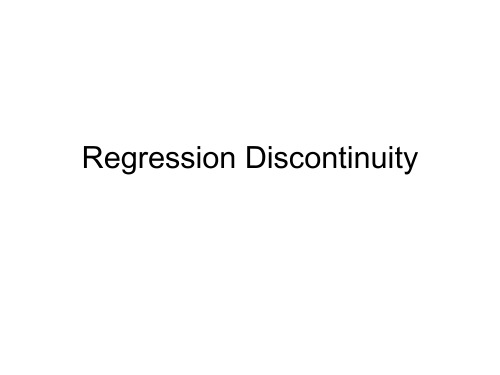
Regression DiscontinuityBasic Idea•Sometimes whether something happens to you or not depends on your …score‟ on a particular variable e.g–You get a scholarship if you get above a certain mark in an exam,–you get given remedial education if you get below acertain level,–a policy is implemented if it gets more than 50% ofthe vote in a ballot,–your sentence for a criminal offence is higher if youare above a certain age (an …adult‟)•All these are potential applications of the …regression discontinuity‟ designMore formally..•assignment to treatment depends in a discontinuous way on some observable variable W•simplest form has assignment to treatment being based on W being above some critical value w0-thediscontinuity•method of assignment to treatment is the very opposite to that in random assignment –it is a deterministicfunction of some observable variable.•But, assignment to treatment is as …good as random‟ in the neighbourhood of the discontinuity –this is hard to grasp but I hope to explain itBasics of RDD Estimator •Suppose average outcome in absence of treatment conditional on W is:•Suppose average outcome with treatment conditional on W is:•This is …full outcomes‟ approach.•Treatment effect conditional on W isg 1(W)-g(W):()(),0E y W X g W==()()1,1E y W X g W==How can we estimate this?•Basic idea is to compare outcomes just to the left and right of discontinuity i.e. to compare:•As δ→0 this comes to:•i.e. treatment effect at W=w 0()()0000E y w W w E y w W w δδ+≥>-≥≥-()()1000g w g w -Comments•the RDD estimator compares the outcome of people who are just on both sides of the discontinuity -difference in means between these two groups is an estimate of the treatment effect at the discontinuity•says nothing about the treatment effect away from the discontinuity -this is a limitation of the RDD effect.•An important assumption is that underlying effect on W on outcomes is continuous so only reason for discontinuity is treatment effectSome pictures –underlying relationship between y and W is linearE(y│W)w0WNow introduce treatment E(y│W)βw0WThe procedure in practice •If take process described above literally should choose a value of δthat is very small•This will result in a small number of observations•Estimate may be consistent but precision will be low•desire to increase the sample size leads one to choose a larger value of δDangers•If δis not very small then may not estimate just treatment effect –look at picture•As one increases δthe measure of the treatment effect will get larger. This is spurious so what should one do about it?•The basic idea is that one should control for the underlying outcome functions.If underlying relationship linear •If the linear relationship is the correct specification then one could estimate the ATE simply by estimating the regression:•But no good reason to assumerelationship is linear and this may cause problems01y X W γβγε=+++Suppose true relationship is:E(y│W)w 0Wg 0(W)g 1(W)Observed relationship between E(y)and WE(y│W)w0W g0(W) g1(W)•one would want to control for a different relationship between y and W for the treatment and control groups •Another problem is that the outcome functions might not be linear in W –it could be quadratic or something else.•The researcher then typically faces a trade-off:– a large value of δto get more precision from a larger sample size but run the risk of a misspecification of the underlying outcomefunction.–Choose a flexible underlying functional form at the cost of some precision (intuitively a flexible functional form can get closer toapproximating a discontinuity in the outcomes).In practice•it is usual for the researcher to summarize all the data in the graph of the outcome against W to get some idea of the appropriate functional forms and how wide a window should be chosen.•But its always a good idea to investigate the sensitivity of estimates to alternative specifications.An example•Lemieux and Milligan “Incentive Effects of Social Assistance: A regression discontinuity approach”, Journal of Econometrics, 2008•In Quebec before 1989 childless benefit recipients received higher benefits when they reached their 30th birthdayThe PictureThe EstimatesNote•Note that the more flexible is the underlying relationship between employment rate and age, the less precise is the estimate。
Regression Discontinuity Design - University of Chicago
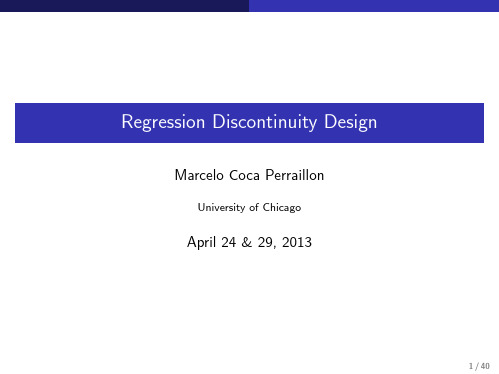
3 / 40
Introduction
Validity
Validity
Simple idea: assignment mechanism is completely known We know that the probability of treatment jumps to 1 if test score > c Assumption is that individuals cannot manipulate with precision their assignment variable (think about the SAT) Key word: precision. Consequence: comparable individuals near cutoff point If treated and untreated individuals are similar near the cutoff point then data can be analyzed as if it were a (conditionally) randomized experiment If this is true, then background characteristics should be similar near c (can be checked empirically) The estimated treatment effect applies to those near the cutoff point (external validity)
4 / 40
Introduction
Validity
Aside: validity doesn’t depend on assignment rule being “arbitrary” Hinges on assignment mechanism being known and free of manipulation with precision Manipulation example 1: Test with few questions and plenty of time Manipulation example 2: DMV test to get a driving license Again: some manipulation is fine (you can always study harder, for example). Precision is the key
准实验研究方法 - 下载,经济金融网,中国经济学教育科研网

前测
结果2
实验组 对照组
前测
后测
图3 带前后测的无实验处理对照组设计的第二种结果
结果3
实验组 对照组 前测
后测
图4 带前后测的无实验处理对照组设计的第三种结果
结果4
对照组 实验组
前测
后测
图5 带前后测的无实验处理对照组设计的第四种结果
结果5
实验组 对照组
前测
后测
图6 带前后测的无实验处理对照组设计的第五种结果
含多个前测的无实验处理对照组设计
O1 O2 X O3
O1
O2
O3
优点 可以较为容易地处理选择-成熟因素 要是O2在每一个组内都是非典型的观察值,并 且假如没有O1,那么由于统计回归的存在就会 使得我们推断出错误的实验处理效应。 可以使用实验组的O1-O2相关系数来估计实验 组的O2-O3相关系数,该估计值将会准确得多。
一项实验设计可被视为是对比较(对照)、
随机化和控制三者的权衡。 (Spector, 1981:21)
2 准实验法中的效度分析
内部效度、外部效度、构思效度与统计结
论效度
因子效度(factorial validity)、工作分析效度
(job-analytic validity)、合成效度(synthetic validity)、理性效度(rational validity)等。 (Cooper & Schindler, 1998:180, 注15)
2.2 内部效度及其影响因素
历史记录(history) 成熟(maturation) 测试效应(testing)
测试工具(instrumentation)
Regression Discontinuity Design v2
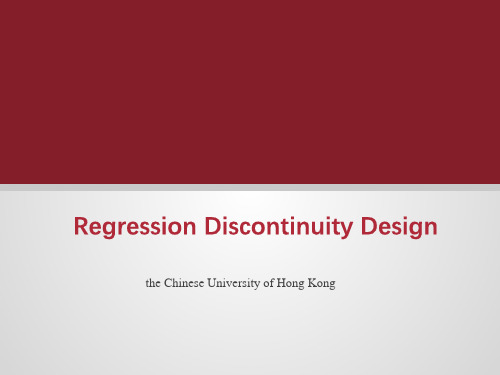
4
2.How to use RDD?
5
Problem 1: We want to study whether attending the first batch of undergraduate can raise a student's salary. If you directly compare the salary of two groups of students, the students of group No.1 attend the first batch of undergraduate while the students of group No.2 do not, you may draw a wrong conclusion because salary level is not only affected by academic qualifications, but also by the ability, family background, appearance and other factors, and the factors above are unobservable variables that can not be controlled.
10
《Evidence-on-the-impact-of-sustained-exposure-toair-pollution-on-life-expectancy》
N
Huai River
S
11
12
The estimated change in life expectancy just north of the Huai River is 5.04 years.
RD方法

RD方法(regression discontinuity design)什么是regression discontinuity design下面将用一篇文章来介绍:如何理解「由于使用燃煤取暖,中国 5 亿北方居民预期寿命将缩短年」7月8日发表在《美国国家科学院院刊》(PNAS)的一篇论文,向已经逐渐意识到空气污染危害的中国民众再次展现了残酷的现实:以淮河为界,烧煤供暖的中国北方地区空气污染水平高于中国南方,北方5亿居民因严重的空气污染,平均每人失去5年寿命。
这篇文章由清华大学的李宏彬、北京大学的陈玉宇和另外两位作者共同完成。
前两位完成了这篇论文的主要部分。
这个回答首先介绍他们的研究方法,再谈一谈研究者和媒体对这篇文章的引申。
科学家如何得出「使用燃煤取暖中国5亿北方居民预期寿命将缩短年」这个结论的首先要说的是这篇文章使用的RD方法(regression discontinuity design),即断点回归方法。
断点回归方法是最近的政策评估中非常重要的一个方法,他可以在没有随机性的情况下识别出政策的效果。
在早期的研究中,要识别一个处理(Treatment)的效果,我们必须拥有随机性,比如两组随机分开的小白鼠,一组加上某种处理,一组没有处理,最后观察两者的区别。
为什么我们那么需要随机性呢因为研究的基础需要几组十分类似的群体,他们的任何特征都服从一个相同的分布,无论是性别、年龄、教育、健康程度……这样,我们才能确定几组对象之间出现的差别是来自于实验处理的差异,而非某些个人特征。
从一个大样本中严格随机抽取的样本,正好满足这样的同分布假设。
但对于政策研究来说,我们不可能找到这样随机分开的两组人,而且也无法用实验的方法来获得结果——你能将随机分开的100人放在干净空气中,将另外100人放在肮脏空气中并观察一段时间吗一些研究者面对这种缺乏随机性的情况,采用了增加控制变量的方式。
比如,把性别年龄教育健康程度全部放进回归式中,然后声称,两组人之间由于性别年龄教育健康的不同而造成的差别都已经去掉了,剩下的就是这个政策的效果了。
政治关联英文文献索引

政治关联英文文献索引1.T he stock market implication of political connections: evidence from firms' dividend policy 政治关系的证券市场含义:来自公司股利政策的证据(Cao et al.2012)2.C orporate ownership, corporate governance reform and timeliness of earnings: Malaysian evidence公司所有权、公司治理改革与盈余及时性:马来西亚的证据3.P olitical connections, bank deposits, and formal deposit insurance: Evidence from an emerging economy政治关系、银行存款与正式的存款保险:来自新兴经济体的证据(June 18, 2013)4.P olitical connections and investment efficiency: Evidence from Chinese listed private firms 政治关系与投资效率:来自中国民营上市公司的证据(August 27, 2013)5.T he Political Determinants of the Cost of Equity: Evidence from Newly Privatized Firms权益资本成本的政治因素:来自刚刚私有化公司的证据(August 2008)6.C ronyism and Capital Controls: Evidence from Malaysia任人唯亲与资本管制:来自马来西亚的证据7.Capital Structure and Political Patronage: Evidence from China资本结构与政治庇护:来自中国的证据8.P olitical Connection and Government Patronage:Evidence from Chinese Manufacturing Firms政治关联与政府赞助:来自中国制造业企业的证据9.P olitical Connections and Firm V alue: Evidence from the Regression Discontinuity Design of Close Gubernatorial Elections政治关联与公司价值:来自临近州长竞选的不连续回归设计的证据10.How does state ownership affect tax avoidance? Evidence from China国家所有权如何影响避税?来自中国的证据11.Do Strong Corporate Governance Firms Still Require Political Connection? And Vice V ersa公司治理强的企业仍然需要政治关系吗?反之亦然12.Political Connections and Preferential Access to Finance: The Role of Campaign Contributions政治关联与优先获得融资:竞选捐款的作用13.Political Connections and the Cost of Equity Capital政治关联与权益资本成本(Boubakri et al. January 2012)14.The Impact of Political Connections on Firms' Operation Performance and Financing Decisions政治关联对公司经营业绩及融资决策的影响(Boubakri et al. November 29, 2011)15.Political Connections and the Cost of Bank Loans政治关系与银行贷款成本(Houston et al. February 15, 2012)16.Politicians and the IPO Decision: The impact of impending political promotions on IPO activity in China政治家与IPO决策:即将来临的政治晋升对中国IPO活动的影响17.Accounting Conservatism and Bankruptcy Risk会计稳健性与破产风险(Biddle et al. October 7, 2013)18.Accounting Conservatism and its Effects on Financial Reporting Quality:A Review of the Literature会计稳健性及其对影响财务报告质量:文献综述(September 9, 2011)19.Conservatism, Disclosure and the Cost of Equity Capital稳健性、披露与权益资本成本(Artiach et al. January 2012)20.Does Access to Finance Lower Firms’ Cost of Capital? Empirical Evidence from International Manufacturing Data获得融资降低了企业的资本成本吗?来自国际制造业数据的实证证据21.Political connections, founding family ownership and leverage decision of privately owned firms政治关联、创始家族所有权与私有企业杠杆决策22.The Impact of Political Connectedness on Firm V alue and Corporate Policies: Evidence from Citizens United政治关系对公司价值及公司政策的影响:来自美国公民的证据23.The Quality of Accounting Information in Politically Connected Firms政治关联企业的会计信息质量24.The political economy of corporate governance, cost of equity, and earnings quality: evidence from newly privatized firms公司治理的政治经济、权益资本成本与盈余质量:来自刚刚私有化公司的证据25.Do Political Connections Help Firms Gain Access to Bank Credit in Vietnam政治关系帮助越南企业获得银行信贷吗?26.Firm performance effects of nurturing political connections through campaign contributions通过竞选捐款培育政治关系的公司绩效效应27.The Chrysler Effect: The Impact of the Chrysler Bailout on Borrowing Cost克莱斯勒效应:克莱斯勒救助对借贷成本的影响28.Politically connected firms in Poland and their access to bank financing波兰的政治关联企业及他们获得银行融资29.Political Connections and Corporate Bailouts政治关系与企业救助30.The characteristics of politically connected firms政治关联企业的特征31.Rent Seeking Incentives, Political Connections and Organizational Structure: Empirical Evidence from Listed Family Firms in China寻租动机、政治关系与组织结构:来自中国上市家族企业的经验证据32.The V alue of Connections In Turbulent Times: Evidence from the United States在动荡的时代关系价值:来自美国的证据33.Malaysian Capital Controls: Macroeconomics and Institutions马来西亚的资本控制:宏观经济和制度34.Rent Seeking and Corporate Finance: Evidence from Corruption Cases寻租与公司融资:腐败案件的证据35.Political Motivation, Over-investment and Firm Performance政治动机、过度投资与公司绩效36.Political connections and earnings quality: evidence from malaysia政治关系与盈余质量——来自马来西亚的证据37.Political Connections and Minority-Shareholder Protection: Evidence from Securities-Market Regulation in China政治关系与少数股东保护:来自中国证券市场监管的经验证据38.Accounting Conservatism, Corporate Governance and Political Influence: Evidence from Malaysia会计稳健性、公司治理与政治影响:来自马来西亚的证据39.Internationalization and Capital Structure: Evidence from Malaysian Manufacturing Firm国际化和资本结构:来自马来西亚制造企业的证据40.Firm size and corporate financial leverage choice in a developing economy Evidence from Nigeria企业规模和企业财务杠杆的选择:来自尼日利亚发展中经济的证据41.Ownership and the V alue of Political Connections: Evidence from China所有权和政治关系的价值:来自中国的证据42.Ownership Types, CEO and Chairman Political Connections, and Long-run Post-IPO Performance: Evidence from China所有权类型、CEO和董事长的政治联系与IPO后长期绩效:来自中国的证据43.Theoretical Investigation on Determinants of Government-Linked Companies Capital Structure关于政府联系公司资本结构的影响因素的理论研究44.Auditor Choice in Privatized Firms: Empirical Evidence on the Role of State and Foreign Owners私有化企业的审计师选择:来自国家和外国所有者作用的经验证据45.The Political Economy of Residual State Ownership in Privatized Firms: Evidence from Emerging Markets私有化企业中剩余政府所有权的政治经济:从新兴市场的证据46.Political Connections and the Process of Going Public: Evidence from China政治关系和上市的过程:来自中国的证据47.Public policy, political connections,and effective tax rates: Longitudinal evidence from Malaysia公共政策、政治关系与有效税率:来自马来西亚的纵向证据48.Why do countries adopt International Financial Reporting Standards为什么各国采用国际财务报告准则49.Political Relationships, Global Financing and Corporate Transparency政治关系、全球融资与公司透明度50.Politically Connected CEOs and Corporate Outcomes: Evidence from France政治关系的首席执行官和公司的结果:来自法国的证据51.Corporate Lobbying, Political Connections, and the Bailout of Banks公司游说、政治关系与银行救助52.Corruption, Political Connections, and Municipal Finance腐败、政治关系与城市金融53.Political connections, corporate governance and preferential bank loans政治联系、公司治理与优惠的银行贷款54.Politically connected firms: an international event study政治关系的企业:一个国际事件研究55.Politicians at work:The private returns and social costs of political connections政客们在工作:私人收益与政治关系的社会成本56.Political Connection, Financing Frictions, and Corporate Investment: Evidence from Chinese Listed Family Firms政治联系、融资摩擦与企业投资——来自中国上市家族企业的证据57.The Role Political Connections Play in Access to Finance:Evidence from Cross-Listing 政治关系在获得融资中发挥的作用:来自交叉上市的证据58.Auditor Choice in Politically Connected Firms政治关联公司的审计师选择59.Financial liberalization, financing constraints and political connection: evidence from Chinese firms金融自由化、融资约束与政治联系:来自中国上市公司的经验证据60.Effects of Financial Liberalization and Political Connection on Listed Chinese Firms' Financing Constraints金融自由化与政治关系对中国上市公司融资约束的影响61.Auditor Tenure, Non-Audit Services and Earnings Conservatism: Evidence from Malaysia审计任期,非审计服务与盈余稳健性——来自马来西亚的证据62.Political Connections of Newly Privatized Firms新私有化企业的政治关系63.Social network, entertainment expenditures and bank lending decisions: Evidence from China’s nonSOE firms社会网络、娱乐支出和银行贷款决策:来自中国非国有企业的证据64.Bank connection, corruption and collateral in China银行联系、腐败和担保:来自中国的证据65.Red Capitalists: Political Connections and Firm Performance in China红色资本家:政治关系与中国公司的业绩66.Political connections, bank deposits, and formal deposit insurance: Evidence from an emerging economy政治关系、银行存款与正式存款保险:来自新兴经济体的证据67.Executive’s former banking experience, entertainment expenditures and bank lending decisions: Evidence from China’s non-SOE firms执行官以前的银行业经验、娱乐支出与银行信贷决策:来自中国的非国有企业的证据68.The effect of political connections on the level and value of cash holdings: International evidence政治关联对现金持有水平及其价值的影响:国际证据69.The Effect of Political Influence and Corporate Transparency on Firm Performance: Empirical Evidence From Indonesian Listed Companies政治影响力与企业透明度对企业绩效的影响:来自印尼股票上市公司的经验证据70.Going Public Process and Political Connections: Evidence from an Emerging Market上市过程与政治联系:来自新兴市场的证据71.Do IPOs Reduce Firms’ Cost of Bank Loans? Evidence from ChinaIPO降低企业的银行贷款成本?来自中国的证据72.Public governance and corporate finance: Evidence from corruption cases公共治理与公司融资:腐败案件的证据73.Dividends, ownership structure and board governance on firm value: empirical evidence from malaysian listed firms股利、股权结构和董事会治理与公司价值:来自马来西亚上市公司的经验证据74.Management Quality and the Cost of Debt: Does Management Matter to Lenders管理质量和债务成本:管理对贷款人重要吗75.Do Educational Ties with Politicians Increase Agency Problems教育与政客联结增加代理问题吗76.Red Capitalists: Political Connections and the Growth and survival of Start-up Companies in China红色资本家:政治关系、成长和中国创业企业的生存77.The Impact of Legal and Political Institutions on Equity Trading Costs: A Cross-Country Analysis法律和政治制度对股票交易成本的影响:一个跨国家分析78.Expropriation of minority shareholders in politically connected firms政治关系企业中侵占小股东利益79.The Political Economy of Residual State Ownership in Privatized Firms: Evidence from Emerging Markets私有化企业中剩余政府所有权的政治经济:来自新兴市场的证据80.Does Financial Globalization Discipline Politically Connected Firms金融全球化约束政治关联的企业吗81.Audit fees in malaysia: does corporate governance matter马来西亚的审计费用:公司治理重要吗82.The Costs of Political Influence: Firm-Level Evidence from Developing Countries政治影响的成本:来自发展中国家的企业层面的证据83.Corporate social responsibility disclosure and its relation on institutional ownership企业社会责任披露及其与机构所有权的关系84.Political Connections and the Process of Going Public: Evidence from China政治关系和上市过程:来自中国的证据85.The Economic Benefits of Political Connections in Late Victorian Britain英国维多利亚时代后期政治关系的经济效益86.Corporate Cash Holdings, Board Structure, and Ownership Concentration: Evidence from Singapore企业现金持有量、董事会结构与股权集中度:来自新加坡的证据Political Uncertainty and Corporate Investment Cycles政治的不确定性与企业投资周期The Chinese Corporate Savings Puzzle: A Firm-Level Cross-Country Perspective中国公司储蓄的困惑:一个企业层面跨国家的视角Chinese firms’ political connection, ownership, and financing constraints中国企业的政治联系,、所有权与融资约束Political Relations and Overseas Stock Exchange Listing: Evidence from Chinese StateownedEnterprises政治关系和海外证券交易所上市:来自中国国有企业的证据Determinants and Effects of Corporate Lobbying企业游说的影响因素及影响Tunneling or Propping: Evidence from Connected Transactions in China隧道还是支持:来自中国关联交易的证据Sheltering Corporate Assets from Political Extraction保护公司资产从政治的提取Bank Power and Cash Holdings: Evidence from Japan银行权利与现金持有:来自日本的证据OLIGARCHIC FAMIL Y CONTROL, SOCIAL ECONOMIC OUTCOMES, AND THE QUALITY OF GOVERNMENT寡头的家族控制、社会的经济成果与政府质量Government Ownership and Corporate Governance: Evidence from the EU政府所有权与公司治理:来自欧盟的证据The Political Economy of Financial Systems金融体系的政治经济Escaping Political Extraction: Political Participation, Institutions, and Cash Holdings in China逃避政治的提取:政治参与、制度与中国的现金持有The value of local political connections in a low-corruption environment地方政治关系在低的腐败环境中的价值Retained State Shareholding in Chinese PLCs: Does Government Ownership Reduce Corporate Value中国上市公司保留的国有股:政府所有权降低企业价值吗Ownership Structure, Institutional Development, and Political Extraction: Evidence from China所有权结构、制度发展与政治提取:来自中国的证据How Do Agency Costs Affect Firm Value? Evidence from China代理成本如何影响公司价值吗?来自中国的证据Corporate Lobbying and Financial Performance公司游说与财务绩效Rights Issues in China as Evidence for the Existence of Two Types of Agency Problems中国人权问题作为两类代理问题存在的证据Auditor Choice in Politically Connected Firms政治关联公司的审计师选择(Journal of Accounting Research,V ol. 52 No. 1 March 2014)Transparency in Politically Connected Firms: Evidence from Private Sector Firms in China政治关联公司的透明度:来自中国私营公司的证据Capital Structure and Political Patronage: Evidence from China资本结构与政治赞助:来自中国的证据Competitive Pressure and Corporate Policies竞争压力与公司政策Political Capital and Moral Hazard1政治资本与道德风险The Effects of Government Quality on Corporate Cash Holdings政府质量对企业现金持有量的影响Corruption in Developing Countries腐败在发展中国家Large investors, capital expenditures, and firm value: Evidence from the Chinese stock market大投资者、资本支出与企业价值:来自中国证券市场的经验证据Firm Investment & Credit Constraints in India, 1997 – 2006: A stochastic frontier approach印度公司的投资与信贷约束,1997–2006:随机前沿方法State Ownership, Soft-Budget Constraint and Cash Holdings:Evidence from China’s Privatized Firms政府所有权、软预算约束与现金持有:来自中国私有化企业的证据Why Do Firms Hold Less Cash为什么公司持有更少的现金Directors’ Political Conn ections and Compliance with Board of Directors Regulations: The Case of S&P/Tsx 300 Companies董事会的政治关系和董事会遵守:标准普尔/ TSX 300公司为例Political Connection and Firm Value政治联系与企业价值The Impact of Political Connectedness on Firm Value and Corporate Policies: Evidence from Citizens United政治关系对公司价值和公司政策的影响:来自美国公民的证据The Impact of Political Connectedness on Cash Holdings: Evidence from Citizens United政治关系对现金持有的影响:来自美国公民的证据Political power and blood-related firm performance政治权力与有血缘关系的公司绩效Politically-Connecte d Boards and the Structure of Chief Executive Officer Compensation Packages in Taiwanese Firms政治关联董事会和台湾公司CEO薪酬结构Government Ownership and Agency Problems in Equity Offerings in China政府所有权与中国股票发行的代理问题Political Uncertainty and Accounting Conservatism: Evidence from the U.S. Presidential Election Cycle 政治不确定性与会计稳健性:来自美国总统选举周期的证据Effect of political uncertainty and corporate investment cycles in Nepal政治不确定性对尼泊尔企业投资周期的影响CEOs’ Connectedness, Social Capital, and Corporate InvestmentCEO关联、社会资本与企业投资Sovereign Wealth Funds and Politically Connected Firms主权财富基金与政治关联企业Political reforms and family-related firm performance政治改革与家族企业绩效Family connections in a low-corruption environment: Evidence from revised municipality borders在一个较低的腐败环境中家族联系:来自修订市边界的证据Does Political Uncertainty Affect Capital Structure Choices政治不确定性影响资本结构的选择吗Corporate Political Connections and Tax Aggressiveness企业政治关系与税收激进性Executive Compensation vis-à-vis Firm Performance: Identifying Future Research Agenda经理薪酬相对于公司绩效:识别未来的研究议程,Does Organizational-level Affiliation of Internal Audit Influence Corporate Risk-Taking? -Evidences from Chinese Listed Companies内部审计风险水平影响企业组织的联系吗?——来自中国股票上市公司的证据Principal-Principal Conflicts under Weak Institutions: A Study of Corporate Takeovers in China较弱制度下的委托代理冲突:中国公司并购的研究Earnings Management Practices Between Government Linked and Chinese Family Linked Companies 政府关联公司与中国家族关联企业之间的盈余管理实践Managerial Agency Costs of Socialistic Internal Capital Markets: Empirical Evidence from China社会主义内部资本市场的经理人代理成本:来自中国的经验证据Firm Size, Sovereign Governance, and Value Creation公司规模、主权治理与价值创造Bank firm relationship and firm performance under a state-owned bank system: evidence from China银企关系与企业国有银行制度下的绩效:来自中国的证据Excess control rights and corporate acquisitions超额控制权与公司并购Bank loan and the agency costs of debt in indonesia; free cash flows and managerial perks perspective 银行贷款和印度尼西亚债务的代理成本:自由现金流与管理津贴的视角CEO Compensation and Political ConnectednessCEO薪酬与政治关联Enterprises, Political Connections and Public Procurement at a Time of Landmark企业、政治关系与公共采购:一次具有里程碑意义的The Political Determinants of the Cost of Equity: Evidence from Newly Privatized Firms股权成本的政治因素:来自刚刚私有化的公司的证据Managerial Attributes and Executive Compensation管理者特征与高管薪酬An Empirical Investigation into the Political Economy of the Firm in a Globalizing World Economy: How Domestic Political Connections Affect Cross-listing Choices实证研究在全球化的世界经济的坚定的政治经济:国内政治关系如何影响交叉上市的选择Capital Markets and Capital Allocation:Implications for Economies of Transition资本市场与资本配置:经济转型的影响Responding to Financial Crisis: The Rise of State Ownership and Implications for Firm Performance应对金融危机:国家所有权上升对企业绩效的影响Influential ownership and capital structure有影响力的所有权与资本结构The Strategic Role Firms’ Political Connections Play in Access to Finance: Coercion of Domestic Banks or Implicit Property Rights Protections企业政治关系在获得融资中的战略作用:国内银行或隐含产权保护的强制手段Corporate Bailouts: the Role of Costly External Finance and Operating Performance企业救助:昂贵的外部融资的作用与经营绩效Do Political Connections Matter? Empirical Evidence from Listed Firms in Pakistan政治联系重要吗?来自巴基斯坦上市公司的经验证据Tycoons Turned Leaders: Market Valuation of Political Connections富豪转身领导:政治联系的市场价值Political Contributions and CEO Pay政治捐款和首席执行官工资Valuing Changes in Political Networks: Evidence from Campaign Contributions to Close Congressional 重视政治网络的变化:从运动的贡献接近国会的证据Corruption in state asset sales – Evidence from China国有资产销售中的腐败–来自中国的证据Investor Protection and Interest Group Politics投资者保护与利益集团政治Privatization, Large Shareholders’ In centive to Expropriate, and Firm Performance私有化、大股东掠夺激励与公司绩效Advances in Measuring Corruption in the Field腐败测量领域的进展Macroeconomic Conditions and the Puzzles of Credit Spreads and Capital Structure宏观经济条件、信用利差的困惑与资本结构Family Ownership and the Cost of Under Diversification家族所有权与多元化的成本Political Geography and Corporate Political Strategy政治地理学与企业政治策略Evidence on the existence and impact of corruption in state asset sales in China中国国有资产出售中腐败现象的存在及影响的证据Stock versus cash dividends: signaling or catering股票与现金股利:信号或宴会The impact of corruption on state asset sales – Evidence from China腐败对国有资产出售的影响-来自中国的证据Executive Compensation and CEO Equity Incentives in China’s Listed Firms高管薪酬与中国上市公司首席执行官的股权激励Political Constraints, Organizational Forms, and Privatization Performance:Evidence from China政治约束、组织形式与民营化绩效:来自中国的证据State Ownership, Political Institutions, and Stock Price Informativeness: Evidence from Privatization政府所有权、政治制度与股价信息含量:来自私有化的证据Corporate Governance in Emerging Markets: A Survey新兴市场的公司治理:文献综述Politically Connected Boards and Top Executive Pay In Chinese Listed Firms1.Political connection and leverage: Some Malaysian evidence2.Do political connections affect the role of independent audit committees and CEO Duality? Someevidence from Malaysian audit pricing3.Board, audit committee and restatement-induced class action lawsuits4.The Political Determinants of the Cost of Equity: Evidence from Newly Privatized Firms5.The impact of political connections on firms’ operating performance and financing decisionsernment Connections and Financial Constraints:Evidence from a Large Representative Sampleof Chinese Firms7.Listing approach, political favours and earnings quality: Evidence from Chinese family firms8.Political connections and tax-induced earnings management: evidence from China9.Ownership Concentration, State Ownership, and Effective Tax Rates: Evidence from China's ListedContext: European Evidence10.Impact of financial reporting quality on the implied cost of equity capital: Evidence from theMalaysian listed firms公允价值会计准则在新兴市场实施的挑战:来自中国采用国际财务报告准则的证据制度环境、政治关系与融资约束——来自中国民营企业的证据(March 1, 2012)政治关联、终极控制人性质与权益资本成本政治关联、盈余质量与权益资本成本政治联系、市场化进程与权益资本成本——来自中国民营上市公司的经验证据政府干预、政治关联与权益资本成本民营企业的政治关联能降低权益资本成本吗。
断点回归RDD
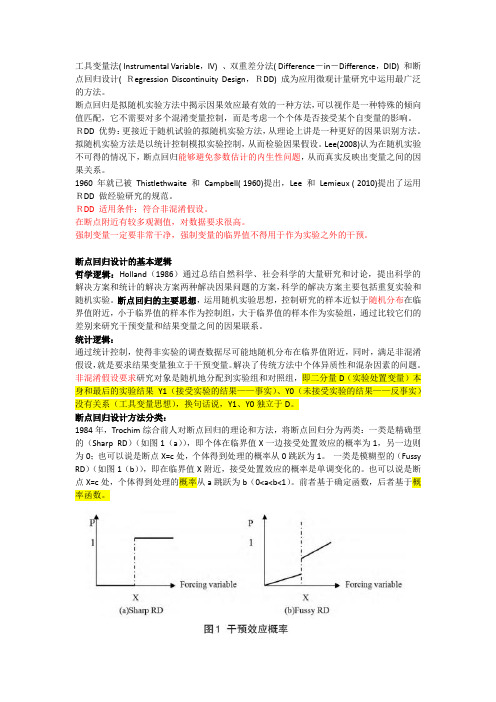
工具变量法( Instrumental Variable,IV) 、双重差分法( Difference-in-Difference,DID) 和断点回归设计( Regression Discontinuity Design,RDD) 成为应用微观计量研究中运用最广泛的方法。
断点回归是拟随机实验方法中揭示因果效应最有效的一种方法,可以视作是一种特殊的倾向值匹配,它不需要对多个混淆变量控制,而是考虑一个个体是否接受某个自变量的影响。
RDD 优势:更接近于随机试验的拟随机实验方法,从理论上讲是一种更好的因果识别方法。
拟随机实验方法是以统计控制模拟实验控制,从而检验因果假设。
Lee(2008)认为在随机实验不可得的情况下,断点回归能够避免参数估计的内生性问题,从而真实反映出变量之间的因果关系。
1960 年就已被Thistlethwaite 和Campbell( 1960)提出,Lee 和Lemieux ( 2010)提出了运用RDD 做经验研究的规范。
RDD 适用条件:符合非混淆假设。
在断点附近有较多观测值,对数据要求很高。
强制变量一定要非常干净,强制变量的临界值不得用于作为实验之外的干预。
断点回归设计的基本逻辑哲学逻辑:Holland(1986)通过总结自然科学、社会科学的大量研究和讨论,提出科学的解决方案和统计的解决方案两种解决因果问题的方案,科学的解决方案主要包括重复实验和随机实验。
断点回归的主要思想,运用随机实验思想,控制研究的样本近似于随机分布在临界值附近,小于临界值的样本作为控制组,大于临界值的样本作为实验组,通过比较它们的差别来研究干预变量和结果变量之间的因果联系。
统计逻辑:通过统计控制,使得非实验的调查数据尽可能地随机分布在临界值附近,同时,满足非混淆假设,就是要求结果变量独立于干预变量。
解决了传统方法中个体异质性和混杂因素的问题。
非混淆假设要求研究对象是随机地分配到实验组和对照组,即二分量D(实验处置变量)本身和最后的实验结果Y1(接受实验的结果——事实)、Y0(未接受实验的结果——反事实)没有关系(工具变量思想),换句话说,Y1、Y0独立于D。
使用地理边界进行断点回归设计
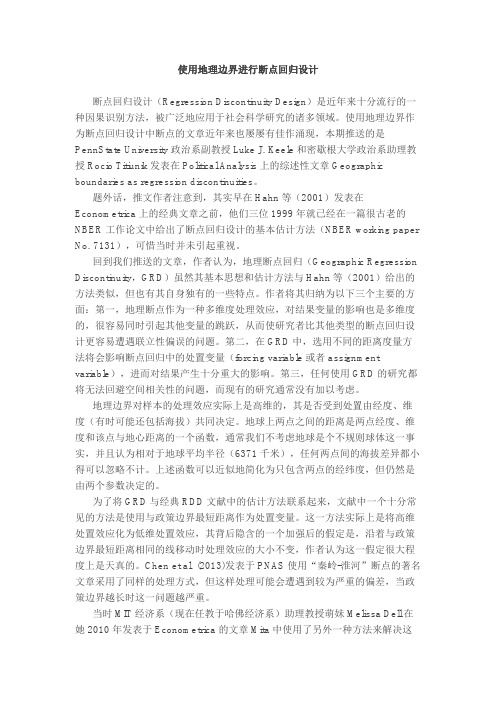
使用地理边界进行断点回归设计断点回归设计(Regression Discontinuity Design)是近年来十分流行的一种因果识别方法,被广泛地应用于社会科学研究的诸多领域。
使用地理边界作为断点回归设计中断点的文章近年来也屡屡有佳作涌现,本期推送的是PennState University政治系副教授Luke J. Keele和密歇根大学政治系助理教授Rocio Titiunik发表在Political Analysis上的综述性文章Geographic boundaries as regression discontinuities。
题外话,推文作者注意到,其实早在Hahn等(2001)发表在Econometrica 上的经典文章之前,他们三位1999年就已经在一篇很古老的NBER工作论文中给出了断点回归设计的基本估计方法(NBER working paper No. 7131),可惜当时并未引起重视。
回到我们推送的文章,作者认为,地理断点回归(Geographic Regression Discontinuity,GRD)虽然其基本思想和估计方法与Hahn等(2001)给出的方法类似,但也有其自身独有的一些特点。
作者将其归纳为以下三个主要的方面:第一,地理断点作为一种多维度处理效应,对结果变量的影响也是多维度的,很容易同时引起其他变量的跳跃,从而使研究者比其他类型的断点回归设计更容易遭遇联立性偏误的问题。
第二,在GRD中,选用不同的距离度量方法将会影响断点回归中的处置变量(forcing variable或者assignment variable),进而对结果产生十分重大的影响。
第三,任何使用GRD的研究都将无法回避空间相关性的问题,而现有的研究通常没有加以考虑。
地理边界对样本的处理效应实际上是高维的,其是否受到处置由经度、维度(有时可能还包括海拔)共同决定。
地球上两点之间的距离是两点经度、维度和该点与地心距离的一个函数,通常我们不考虑地球是个不规则球体这一事实,并且认为相对于地球平均半径(6371千米),任何两点间的海拔差异都小得可以忽略不计。
退休与城镇家庭消费基于断点回归设计的经验证据
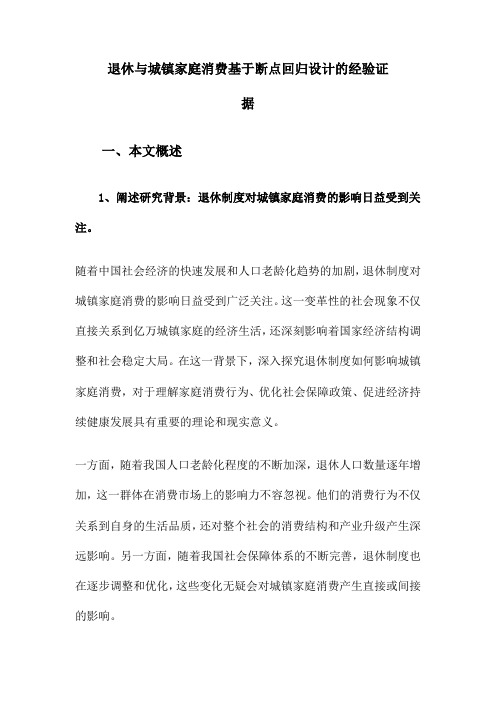
退休与城镇家庭消费基于断点回归设计的经验证据一、本文概述1、阐述研究背景:退休制度对城镇家庭消费的影响日益受到关注。
随着中国社会经济的快速发展和人口老龄化趋势的加剧,退休制度对城镇家庭消费的影响日益受到广泛关注。
这一变革性的社会现象不仅直接关系到亿万城镇家庭的经济生活,还深刻影响着国家经济结构调整和社会稳定大局。
在这一背景下,深入探究退休制度如何影响城镇家庭消费,对于理解家庭消费行为、优化社会保障政策、促进经济持续健康发展具有重要的理论和现实意义。
一方面,随着我国人口老龄化程度的不断加深,退休人口数量逐年增加,这一群体在消费市场上的影响力不容忽视。
他们的消费行为不仅关系到自身的生活品质,还对整个社会的消费结构和产业升级产生深远影响。
另一方面,随着我国社会保障体系的不断完善,退休制度也在逐步调整和优化,这些变化无疑会对城镇家庭消费产生直接或间接的影响。
因此,本文旨在通过断点回归设计等方法,实证探究退休制度对城镇家庭消费的影响,以期为相关政策制定提供科学依据和决策支持。
我们期望通过这一研究,不仅能够深化对退休制度与城镇家庭消费关系的理解,还能够为优化社会保障政策、促进消费升级提供有益参考。
2、提出研究问题:退休如何影响城镇家庭消费?是否存在断点回归现象?随着中国社会经济的持续发展,人口老龄化问题日益凸显,退休制度对城镇家庭消费的影响逐渐受到学术界的关注。
退休作为个体生命周期中的一个重要节点,标志着个体从职业劳动市场转向非劳动市场,这一转变可能会带来家庭消费结构和消费行为的变化。
因此,探讨退休对城镇家庭消费的具体影响及其内在机制,不仅对于理解个体和家庭在生命周期内的消费决策过程具有重要价值,也为政府制定相关政策以应对人口老龄化挑战提供了科学依据。
在经济学研究中,断点回归现象通常指的是某一变量在达到某一特定阈值后,其与其他变量之间的关系发生显著变化。
在退休与城镇家庭消费的背景下,断点回归现象可能表现为退休前后家庭消费模式的显著变化。
模糊断点回归

模糊断点回归在因果关系分析的实证方法中,最优的选择应当为随机实验,但是随机实验的时间成本和经济成本都比较高,而在随机实验不可得的情况下,需要考虑使用其它方法。
断点回归便是仅次于随机实验的, 能够有效利用现实约束条件分析变量之间因果关系的实证方法。
断点回归设计是由美国西北大学的心理学家campbell在1958年首先提出来的,到20世纪80年代,campbell及其同事一直从事断点回归的设计和研究工作。
断点回归设计(regressiondiscontinuity design)是一种仅次于随机实验的能够有效利用现实约束条件分析变量之间因果关系的实证方法。
断点回归可以分为两类,一类是模糊断点回归(fuzzy rd),其特征是断点x=c处,个体得到处理的概率从0跳跃到1;另一类是清晰断点回归(sharp rd),其特征是断点x=c 处,个体得到处理的概率从a跳跃到b,其中0<a<b<1。
rd的目的是选取其他特征相似的组,考察临界值区间上下不同比如考察进清华对收入的影响。
考687分的不能上清华,考689分的可以进去。
只差2分。
这两类人的基本能力其实没什么区别。
两组人,围绕688分的分割线,研究工资差异的内在效度很高,因为他们之间唯一的区别就是进不进清华。
其他都一样。
将这种想法扩展到控制其他变量。
数据分为1)688以下和2)688以上。
回归线应该斜率差不多,但截距明显不同。
截距项可以理解为招生带来的收入差。
rd需要数据更少,主要是考虑临界值附近的影响关于断点回归的基本逻辑、方法和应用,可参考中南财经政法大学罗胜博士在“统计与决策”上的《断点回归设计:基本逻辑、方法、应用评述》一文。
关于断点回归的操作,可参考三篇文献:第一是香樟经济学圈发表的基于lee,and lemieux, 2010,"regression discontinuity designs in economics ",journal of economic literature, vol. 48: 281–355.的推文,【香樟推文0620】运用断点回归设计做研究的规定动作()第二是2017年aer论文pinotti, paolo. "clicking on heaven's door: the effect of immigrant legalization on crime." american economicreview107.1(2017): 138-68.第三是一篇实际操作的比较thoemmes,felix, wang liao, and ze jin. "the analysis of the regression-discontinuity design in r." journal of educational and behavioral statistics 42.3 (2017): 341-360.以及史冬波梳理断点回归设计的标准操作()运用断点回归设计做研究的规定动作第1步检查配置变量(assignment variable,又叫running variable、forcing variable)是否被操纵。
Class-Size Caps, Sorting, and the Regression-Discontinuity Design

Class-Size Caps, Sorting, and the Regression-Discontinuity Design Author(s): Miguel Urquiola and Eric Verhoogen Source: The American Economic Review, Vol. 99, No. 1 (Mar., 2009), pp. 179-215 Published by: American Economic Association Stable URL: /stable/29730181 Accessed: 04-06-2015 15:11 UTC
a class-size
higher-income households sort intohigher-quality schools. The keyprediction, borne out in data from Chile's liberalized education market, is that schools at the class-size cap adjust prices (or enrollments) to avoid adding an addi? tional classroom, which generates discontinuities in the relationship between enrollment and household characteristics, violating the assumptions underly? ing regression-discontinuity research designs. (JEL D12,121,128, 015)
断点回归(RD)学习手册
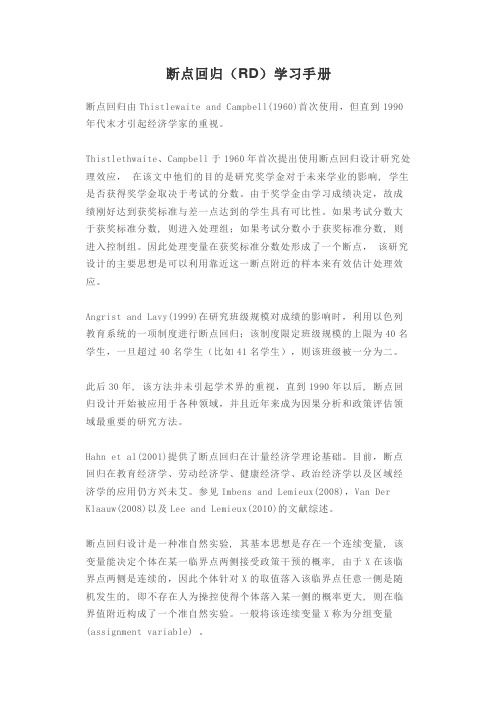
断点回归(RD)学习手册断点回归由Thistlewaite and Campbell(1960)首次使用,但直到1990年代末才引起经济学家的重视。
Thistlethwaite、Campbell于1960年首次提出使用断点回归设计研究处理效应, 在该文中他们的目的是研究奖学金对于未来学业的影响, 学生是否获得奖学金取决于考试的分数。
由于奖学金由学习成绩决定,故成绩刚好达到获奖标准与差一点达到的学生具有可比性。
如果考试分数大于获奖标准分数, 则进入处理组;如果考试分数小于获奖标准分数, 则进入控制组。
因此处理变量在获奖标准分数处形成了一个断点, 该研究设计的主要思想是可以利用靠近这一断点附近的样本来有效估计处理效应。
Angrist and Lavy(1999)在研究班级规模对成绩的影响时,利用以色列教育系统的一项制度进行断点回归;该制度限定班级规模的上限为40名学生,一旦超过40名学生(比如41名学生),则该班级被一分为二。
此后30年, 该方法并未引起学术界的重视,直到1990年以后, 断点回归设计开始被应用于各种领域,并且近年来成为因果分析和政策评估领域最重要的研究方法。
Hahn et al(2001)提供了断点回归在计量经济学理论基础。
目前,断点回归在教育经济学、劳动经济学、健康经济学、政治经济学以及区域经济学的应用仍方兴未艾。
参见Imbens and Lemieux(2008),Van Der Klaauw(2008)以及Lee and Lemieux(2010)的文献综述。
断点回归设计是一种准自然实验, 其基本思想是存在一个连续变量, 该变量能决定个体在某一临界点两侧接受政策干预的概率, 由于X在该临界点两侧是连续的,因此个体针对X的取值落入该临界点任意一侧是随机发生的, 即不存在人为操控使得个体落入某一侧的概率更大, 则在临界值附近构成了一个准自然实验。
一般将该连续变量X称为分组变量 (assignment variable) 。
断点回归设计方法应用的研究综述
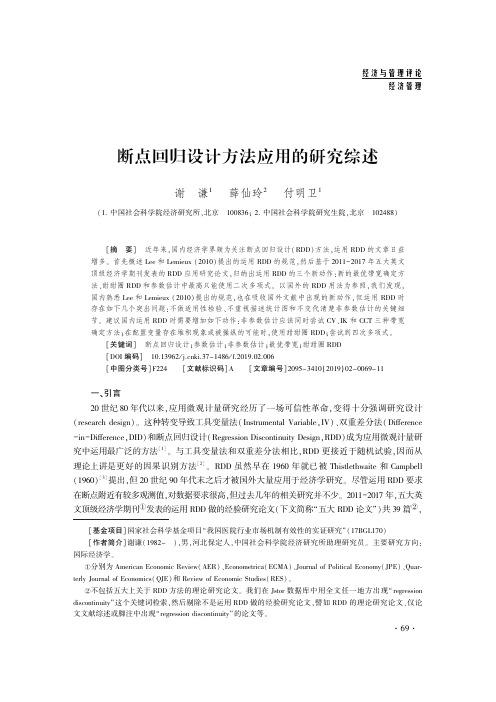
经济管理㊀㊀[基金项目]国家社会科学基金项目 我国医院行业市场机制有效性的实证研究 (17BGL170)㊀㊀[作者简介]谢谦(1982-㊀)ꎬ男ꎬ河北保定人ꎬ中国社会科学院经济研究所助理研究员ꎮ主要研究方向:国际经济学ꎮ①分别为AmericanEconomicReview(AER)㊁Econometrica(ECMA)㊁JournalofPoliticalEconomy(JPE)㊁Quar ̄terlyJournalofEconomics(QJE)和ReviewofEconomicStudies(RES)ꎮ②不包括五大上关于RDD方法的理论研究论文ꎮ我们在Jstor数据库中用全文任一地方出现 regressiondiscontinuity 这个关键词检索ꎬ然后剔除不是运用RDD做的经验研究论文ꎬ譬如RDD的理论研究论文㊁仅论文文献综述或脚注中出现 regressiondiscontinuity 的论文等ꎮ断点回归设计方法应用的研究综述谢㊀谦1㊀薛仙玲2㊀付明卫1(1.中国社会科学院经济研究所ꎬ北京㊀100836ꎻ2.中国社会科学院研究生院ꎬ北京㊀102488)㊀㊀[摘㊀要]㊀近年来ꎬ国内经济学界颇为关注断点回归设计(RDD)方法ꎬ运用RDD的文章日益增多ꎮ首先概述Lee和Lemieux(2010)提出的运用RDD的规范ꎬ然后基于2011-2017年五大英文顶级经济学期刊发表的RDD应用研究论文ꎬ归纳出运用RDD的三个新动作:新的最优带宽确定方法㊁甜甜圈RDD和参数估计中最高只能使用二次多项式ꎮ以国外的RDD用法为参照ꎬ我们发现ꎬ国内熟悉Lee和Lemieux(2010)提出的规范ꎬ也在吸收国外文献中出现的新动作ꎬ但运用RDD时存在如下几个突出问题:不做适用性检验㊁不重视描述统计图和不交代清楚非参数估计的关键细节ꎮ建议国内运用RDD时需要增加如下动作:非参数估计应该同时尝试CV㊁IK和CCT三种带宽确定方法ꎻ在配置变量存在堆积现象或被操纵的可能时ꎬ使用甜甜圈RDDꎻ尝试到四次多项式ꎮ[关键词]㊀断点回归设计ꎻ参数估计ꎻ非参数估计ꎻ最优带宽ꎻ甜甜圈RDD[DOI编码]㊀10.13962/j.cnki.37-1486/f.2019.02.006[中图分类号]F224㊀㊀[文献标识码]A㊀㊀[文章编号]2095-3410(2019)02-0069-11一㊁引言20世纪80年代以来ꎬ应用微观计量研究经历了一场可信性革命ꎬ变得十分强调研究设计(researchdesign)ꎮ这种转变导致工具变量法(InstrumentalVariableꎬIV)㊁双重差分法(Difference-in-DifferenceꎬDID)和断点回归设计(RegressionDiscontinuityDesignꎬRDD)成为应用微观计量研究中运用最广泛的方法[1]ꎮ与工具变量法和双重差分法相比ꎬRDD更接近于随机试验ꎬ因而从理论上讲是更好的因果识别方法[2]ꎮRDD虽然早在1960年就已被Thistlethwaite和Campbell(1960)[3]提出ꎬ但20世纪90年代末之后才被国外大量应用于经济学研究ꎮ尽管运用RDD要求在断点附近有较多观测值ꎬ对数据要求很高ꎬ但过去几年的相关研究并不少ꎮ2011-2017年ꎬ五大英文顶级经济学期刊①发表的运用RDD做的经验研究论文(下文简称 五大RDD论文 )共39篇②ꎬ96经济管理各年分别为6篇㊁3篇㊁3篇㊁11篇㊁5篇㊁7篇和4篇(如图1所示)ꎬ表明RDD在国际上已经成为一种主流的经验研究方法ꎮ根据在中国知网的检索ꎬ中文期刊发表的头两篇运用RDD做的经验研究论文是 退休会影响健康吗? [4]和 城市群落的崛起㊁经济绩效与区域收入差距 [5]ꎻ2011-2017年间ꎬ中文CSSCI期刊共发表运用RDD做的经验研究论文46篇ꎬ各年分别为1篇㊁1篇㊁0篇㊁5篇㊁8篇㊁8篇和23篇(如图1所示)ꎮ我们预计ꎬ大数据时代的来临会极大地打破运用RDD面临的数据限制①ꎬ运用RDD的相关研究会越来越多ꎮ图1㊀中文核心期刊和英文五大期刊上发表的断点回归设计经验研究论文数②Imbens和Lemieux(2008)[6]㊁vanderKlaauw(2008)[7]㊁Lee和Lemieux(2010)[2]㊁Skovron和Titiunik(2017)[8]㊁Hausman和Repson(2018)[9]专门综述了RDD的理论和应用研究ꎮ余静文和王春超(2011)[10]简要介绍了断点回归设计的发展历史㊁理论㊁实施步骤和应用情况ꎮ但是ꎬ由于RDD这种方法的理论研究仍很活跃ꎬ运用RDD涉及参数估计和非参数估计的选择㊁参数估计中多项式次数的选择和非参数估计最优带宽的确定等众多技术细节ꎬ文献中在RDD的具体用法上存在很大差别ꎬ特别是中文文献运用RDD的规范程度明显滞后于外文文献ꎬ有时还存在误用ꎮ因此ꎬ十分有必要归纳RDD应用的新进展ꎬ为国内学者规范运用RDD提供借鉴ꎮ由于本文强调被五大RDD论文运用过的新进展ꎬ侧重于应用ꎬ我们不会涉及多配置变量RDD(RDdesignswithassignmentvariables)[11]㊁分位数RDD[12]㊁拐点回归设计(regressionkinkdesigns)[13]㊁多断点RDD(RDdesignswithmultiplecutoffs)[14]㊁远离断点处的处理效应的识别方法(methodsforextrapolationawayfromthecutoff)[15][16]㊁离散型配置变量RDD[17]等五大RDD论文中未运用的新进展ꎮ本文综述Lee和Lemieux(2010)[2]以来RDD的新进展ꎮ选择以Lee和Lemieux07 ①②这方面的一个表现是ꎬ大量行政管理数据(administrativedata)被用于经济学经验研究ꎮ根据英国«经济学人»(TheEconomist)的报道ꎬNBER(NationalBureauofEconomicResearch)工作论文摘要里出现 administra ̄tivedata 的论文数ꎬ2000年是0篇ꎬ之后快速增长ꎬ2017年达到28篇ꎮ报道链接为:https://www.economist.com/international/2018/05/26/government-data-are-ever-more-important-to-economic-researchꎮ中文核心期刊论文数的检索方法如下:在中国知网检索出主题包含 断点回归 ㊁发表年度为2011年至2017年的中文社会科学引文索引(CSSCI)期刊论文ꎬ然后逐一检查㊁剔除非经验研究论文ꎬ最后剩下46篇ꎮ将检索限定在中文社会科学引文索引期刊ꎬ是为了保证检索到的论文的质量ꎮ英文五大期刊论文数的检索方法如下:在Jstor数据库中用全文任一地方出现 regressiondiscontinuity 这个关键词㊁发表年度为2011至2017年㊁期刊为五大期刊检索到50篇ꎬ然后逐一检查㊁剔除非经验研究论文ꎬ最后剩下39篇ꎮ经济管理(2010)[2]为基点的理由如下:首先ꎬ与Imbens和Lemieux(2008)[6]㊁vanderKlaauw(2008)[7]相比ꎬLee和Lemieux(2010)[2]的时间要晚ꎮ其次ꎬ与Skovron和Titiunik(2017)[8]综述政治学中断点回归设计的应用情况㊁Hausman和Repson(2018)[9]综述时间作为配置变量(也叫驱动变量)的RDD应用情况相比ꎬLee和Lemieux(2010)[2]综述的是经济学中的应用情况ꎬ包括各种类型的配置变量ꎬ更综合㊁更全面ꎮLee和Lemieux(2010)[2]提出了运用RDD做经验研究的规范ꎬ算是一个共识ꎮ但是ꎬ通过归纳2011-2017年的五大RDD论文ꎬ我们发现:一方面很多论文都没有遵守此规范ꎮ另一方面涌现出了很多被广泛应用的新进展ꎬ譬如非参数估计中确定带宽的IK法①[18]和CCT法[19]㊁参数估计中只应使用配置变量的低次项和甜甜圈RDD(DonutholeRDD)等②ꎮ通过归纳2011-2017年CSSCI期刊发表的RDD经验研究论文ꎬ我们发现ꎬ国内总体上了解运用RDD的规范ꎬ但仍然存在不少问题ꎬ譬如不做适用性检验㊁不重视描述统计图㊁不交代清楚非参数估计的关键细节等ꎮ二、运用断点回归设计的规范和新进展(一)运用断点回归设计做研究的既定规范Lee和Lemieux(2010)[2]提出了运用RDD做经验研究的规范ꎬ概述如下:1.利用配置变量检验RDD的适用性ꎮRDD的前提条件是个体不能精准操控(preciselymanipulate)配置变量ꎮ此条件可利用配置变量来检验ꎮ首先ꎬ选定一定数量的箱体画出配置变量的历史直方图ꎮ如果频数(frequencies)在邻近断点处的两个箱体间存在跳跃式变化ꎬ则很可能意味着前提条件不成立ꎮ其次ꎬ做配置变量的McCrary检验[20]ꎮ此检验的stata命令DCdensity是外部命令ꎬ可在下述网址下载后安装:https://eml.berkeley.edu/~jmccrary/DCden ̄sity/ꎮ2.利用前定变量检验RDD的适用性ꎮ首先ꎬ选定一定数量的箱体ꎬ求前定变量在每个箱体内的均值ꎬ画出均值对箱体中间点的散点图ꎻ接着再画出使用多项式模型对前定变量做出的拟合曲线ꎬ其中多项式一般是4次多项式③ꎮ如果前定变量的均值在断点处存在跳跃ꎬ则意味着前提条件很可能不成立ꎮ其次ꎬ用前定变量对常数项㊁处理变量㊁配置变量的多项式㊁处理变量和配置变量多项式的交互项做回归ꎮ如果前定变量比较多ꎬ那么随机因素也可能导致某个前定变量存在显著的断点ꎮ因此ꎬ有必要把众多检验合并为一个检验所有前定变量都不存在断点的统计量ꎮ这时ꎬ应该用似不相关回归(SeeminglyUnrelatedRegressionꎬSUR)ꎮ3.画结果变量的图ꎮ选定一定数量的箱体ꎬ求结果变量在每个箱体内的均值ꎬ画出均值对箱体中间点的散点图ꎻ接着再画出使用多项式模型对结果变量做出的拟合曲线ꎬ其中多项式一般是4次多项式ꎮ一定要求了局部平均值后再画图ꎮ如果直接画原始数据的散点图ꎬ那么噪音太大ꎬ看不出潜在函数的形状ꎮ如果结果变量的均值在断点处存在跳跃ꎬ则意味着处理变量有影响ꎮ用图来显示结果变量和配置变量之间的关系ꎬ已经成为运用RDD的标准作法ꎮLee17 ①②③Imbens和Kalyanaraman(2009)是工作论文ꎬ后来于2012年发表在«ReviewofEconomicStudies»上ꎮ用Stata软件实现这些新进展的操作命令可以参考Cattaneoetal.(2018)ꎬ更详细的操作命令细节可参考本文所引用文献的网上附件ꎮ这些网上附件可在发表各引用文献的期刊官网上下载ꎮ作者文中用的都是4次多项式ꎬ没解释不用其他次数多项式的原因ꎮ经济管理和Lemieux(2010)[2]指出ꎬ画图在RDD经验文章中是如此重要ꎬ以致没有图的文章会让人自然而然地怀疑图形证据对文章不利ꎮ4.全样本参数估计ꎮ使用全样本数据进行参数估计ꎮ以精确断点回归为例ꎬ估计模型如下:y=α+ρˑTreatment+ðKk=1βkˑ(x-c)k+ðKk=1γkˑTreatmentˑ(x-c)k+W+ε(1)其中y㊁x㊁treatment和W分别为结果变量㊁配置变量㊁处理变量(当xȡc时ꎬtreatment=1ꎬ否则treatment=0)和前定变量ꎮα㊁ρ㊁β和γ为待估计的参数ꎬε为随机扰动项ꎮρ显著不为0ꎬ表明处理对结果变量有影响ꎮ参数估计的要点是确定模型(1)中的多项式次数Kꎮ一般从一次尝试到八九次①ꎬ然后挑选赤池信息准则(AIC)取值最小的模型ꎮ另外一个比赤池信息准则更好的方法是ꎬ在多项式模型的自变量中先加入表示箱体的虚拟变量ꎬ然后不断加入配置变量的多次项ꎬ只到下述原假设成立:所有箱体虚拟变量的系数均等于零ꎮ5.子样本非参数估计ꎮ非参数估计就是对最优带宽内的子样本做加权局部线性回归ꎮ非参数估计的要点是确定最优带宽ꎮ确定最优带宽的方法有两种②:拇指规则法(ruleofthumbꎬROT)和交叉验证法(crossvalidationprocedureꎬCV)ꎮ加权可用矩形核密度函数㊁三角形核密度函数等ꎮ其中ꎬ使用矩形核密度函数加权的非参数估计ꎬ等于是基于子样本的参数估计ꎮ使用三角形核密度函数与使用矩形核密度函数的唯一区别是ꎬ前者给临近断点的观测值更大的权重ꎮ但是ꎬ要想给临近断点的观测值更大的权重ꎬ更透明的做法是在更小的带宽内使用矩形核密度函数ꎮ使用矩形核密度函数得到的结果也更易解释ꎮ值得庆幸的是ꎬ实践中不同核密度函数得到的结果一般是一致的ꎮ具体研究中ꎬ参数估计和非参数估计都要做ꎬ在两种方法下都稳定的结果更可信ꎮ(二)运用断点回归设计做研究的新进展Lee和Lemieux(2010)[2]之后的7年里(2011-2017年)ꎬ学者在运用RDD时出现了不少Lee和Lemieux(2010)[2]未提及的新做法ꎬ譬如非参数估计中确定带宽的IK法和CCT法㊁甜甜圈RDD(DonutholeRDD)㊁参数估计中只应使用配置变量的低次项和利用配置变量做适用性检验的Frandsen法[21]等ꎮ此处归纳比较重要的三种新做法如下:1.非参数估计中最优带宽的确定ꎮLee和Lemieux(2010)[2]介绍了ROT和CV两种确定最优带宽的方法ꎮ但是ꎬ2011-2017年间五大RDD论文使用得多的是IK法和CCT法ꎮstata中用非参数法做断点回归估计时的命令rdꎬ就是用IK法确定最优带宽ꎬ而命令rdrobust提供CV㊁IK㊁CCT三种不同的最优带宽计算方法选项ꎮImbens和Kalyanaraman(2012)[18]指出ꎬROT和CV两种方法对于在整个支撑集(supportset)里估计回归方程而言是最优的ꎬ但RDD只关心断点处的回归方程估计ꎬ故ROT和CV对于RDD而言不是最优的ꎬ而IK法对于RDD而言27 ①②作者说这是经验上的做法ꎬ没解释这样做的原因ꎮLee和Lemieux(2010)在脚注中提到了之后应用广泛的IK法(Imbens和Kalyanaramanꎬ2009)ꎬ但是文中举例只使用拇指规则法和交叉验证法ꎬ没使用IK法ꎮ经济管理是渐近最优的ꎻ另外ꎬCV法需要研究人员自己设定一个调节参数(tuningparameter)ꎬ而IK法是完全数据驱动的ꎮImbens和Kalyanaraman(2012)[18]进一步指出ꎬ尽管IK法具备这些优点ꎬ但研究人员不能只看这一个最优带宽下的估计结果ꎬ而应把这一带宽作为基准㊁检验结果对不同带宽的稳健性ꎮCalonico㊁Cattaneo和Titiunik(2014)[19]认为ꎬROT㊁CV和IK这些方法得出的最优带宽过大ꎬ导致相应的置信区间有偏ꎬ会过度拒绝 没有处理效应 的原假设ꎬ结果把 没有 处理效应说成 有 ꎮCCT法校正了过大带宽带来的偏误ꎮ2.甜甜圈RDD(DonutholeRDD)ꎮ甜甜圈RDD用来克服数据堆积(heaping)问题对估计结果的影响①ꎮ数据堆积指取配置变量某些值的观测值过多的现象ꎮ导致这一现象的原因包括受访者自报告某些信息时倾向于向某个数值近似ꎬ测量标尺的精读有限等ꎮ譬如ꎬ调查数据中受访者自报告的身高在160cm㊁170cm和180cm等取值上堆积ꎬ新生儿体重会在3000克㊁3500克和4000克等取值上堆积ꎮ数据操纵现象源自经济个体的逐利动机ꎬ只出现在断点处ꎮ然而ꎬ数据堆积不是源自经济个体的逐利动机ꎬ且可能出现在除断点之外的其他地方ꎮ如果结果变量受配置变量的堆积现象影响ꎬ那么RDD估计量可能是有偏的[22]ꎮ此时ꎬ可以去掉断点附近的某些观测值再做RDD估计ꎮ由于去掉断点附近的某些观测值后的数据就像一个 甜甜圈 ꎬ故称作 甜甜圈RDD ꎮ至于去掉断点附近多少观测值为适ꎬ文献中尚未达成共识ꎮ3.全样本参数估计的缺点ꎮ根据Lee和Lemieux(2010)[2]ꎬ配置变量多项式要尝试到八九次ꎮ但是ꎬGelman和Imbens(2014)[23]认为ꎬ不能尝试配置变量多项式的高次项ꎬ只能使用局部一次或局部二次多项式ꎬ理由有三点:第一ꎬ断点回归设计的估计量可以写成处理组结果的加权平均值和控制组结果的加权平均值之差ꎬ其中加权权重是配置变量的函数ꎮ运用全域高阶多项式方法时ꎬ当配置变量取值较大时ꎬ使用不同次数多项式得到的权重差别巨大ꎮ然而ꎬ局部线性回归方法给临近断点处的观测值赋予要大的权重ꎬ理论上讲更合理ꎮ第二ꎬ处理效应的估计值对全域高阶多项式的次数十分敏感ꎮ使用不同次数多项式得到的估计值差别很大ꎮ第三ꎬ在本身不存在断点的情况下ꎬ全域多项式方法得到错误结果(认为有断点)的概率高于实际水平ꎬ而局部一次(或二次)多项式方法得到错误结果的概率和实际水平差不多ꎮ三、断点回归设计的国外应用情况2011-2017年ꎬ五大英文顶级经济学期刊共发表39篇运用RDD做的经验研究论文ꎬ涉及公共经济学㊁新政治经济学㊁劳动经济学和教育经济学等众多领域ꎮ39篇论文中有33篇使用行政管理数据②ꎬ由此可见开放行政管理数据对于RDD应用的重要性ꎮ第二部分提到的三个新进展的应用情况如下:首先ꎬ使用非参数估计的26篇论文中ꎬ用IK法㊁CCT法确定最优带宽的分别为13篇㊁6篇ꎬ表明这两种最优带宽确定方法已被学界接受ꎻ其次ꎬ在甜甜圈RDD被提出的2011年之后ꎬ33篇论文中有5篇用了此方法ꎻ最后ꎬDell(2015)[24]和Pinotti(2017)[25]引用了Gelman和Imbens(2014)[23]ꎬ但还使用超过二次的配置变量多项式做稳健性检验ꎮ这些文献对RDD的运用与Lee和Lemieux(2010)[2]提出的规范大体一致ꎬ譬如20篇(占37 ①②实际上ꎬ甜甜圈RDD也可以克服数据操纵问题对估计结果的影响ꎮ分别来自美国㊁巴西㊁挪威㊁意大利等17个国家ꎮ经济管理51.28%)论文引用了Lee和Lemieux(2010)[2]ꎬ33篇(占84.62%)做了适用性检验ꎮ与Lee和Lemieux(2010)[2]提出的规范不一致的情况ꎬ包括如下几点:第一ꎬLee和Lemieux(2010)[2]建议参数估计和非参数估计均要做①ꎬ但这样做的只有16篇ꎮ只使用参数估计㊁只使用非参数估计的分别为13篇和10篇ꎮ在16篇同时使用了两种估计方法的文章中ꎬ将两种方法视为同等重要的有4篇ꎬ参数估计仅只是做稳健性检验㊁非参数仅只是做稳健性检验的分别为4篇和8篇ꎮ因此ꎬ总体来看ꎬ文献中偏重于参数估计ꎮ值得一提的是ꎬECMA上的4篇文章都只用非参数估计ꎬ而QJE上的5篇文章ꎬ除Malamud和Pop-Eleches(2011)[26]同时使用了两种方法外ꎬ其余4篇都只用参数估计ꎮECMA由国际计量经济学会主办ꎬQJE由哈佛大学经济系主办ꎮ二者在RDD应用上的差异可能体现了两个主办方不同的学术风格ꎮ第二ꎬLee和Lemieux(2010)[2]建议用前定变量做适用性检验时ꎬ应该做似不相关检验ꎬ但没有一篇文献这样做ꎮ第三ꎬLee和Lemieux(2010)[2]建议画描述统计图中的拟合曲线时ꎬ用配置变量的4次多项式ꎬ但仅有3篇论文用了4次多项式ꎮ39篇论文中ꎬ仅有Chettyetal(2014)[27]这一篇没在描述统计图中画上对散点的拟合曲线ꎮ在画了的38篇中ꎬ未说明用的什么方法㊁使用局部线性回归(locallinearregression)㊁使用局部线性平滑(locallinearsmoother)㊁移动平均法(running-meansmoothing)和多项式方法的分别为3篇㊁3篇㊁3篇㊁1篇和28篇ꎬ可见多项式方法是主流方法ꎮ在画拟合曲线所用方法上的差异具有明显的期刊特色:使用局部线性回归法的3篇文章有1篇来自QJE㊁2篇来自JPEꎬ使用局部线性平滑法的3篇文章均来自ECMAꎬ使用移动平均法的一篇文章来自RESꎬAER上的文章都使用多项式方法ꎮ在使用多项式方法的28篇论文中ꎬ使用1次㊁2次㊁3次㊁4次㊁8次及同时使用1次和2次多项式的分别为8篇㊁11篇㊁4篇㊁3篇㊁1篇和1篇ꎬ使用1次多项式和2次多项式的明显居多ꎮ值得一提的是ꎬMeng(2017)[28]在同一张图中同时画了1次多项式和2次多项式的拟合曲线ꎮ第四ꎬLee和Lemieux(2010)[2]建议参数估计时的配置变量多项式要尝试到八九次ꎬ然后挑选赤池信息准则(AIC)取值最小的模型ꎮ但是ꎬ使用过参数估计的29篇论文中ꎬ仅有Clark和Martorell(2014)[29]和Deshpande(2016)[30]根据AIC来挑选模型ꎮ在明确说明尝试过的多项式次数的论文中ꎬ仅有Auffhammer和Kellogg(2011)[31]㊁Pinotti(2017)[25]尝试过超过4次的多项式ꎬ绝大多数只尝试到4次多项式ꎮ第五ꎬLee和Lemieux(2010)[2]建议非参数估计时用矩形核密度函数ꎮ但是ꎬ在使用非参数估计且说明了所用核密度函数的16篇文献中ꎬ使用矩形核密度函数的为7篇ꎬ而使用三角形核密度函数的有8篇ꎬ还有一篇使用帐篷型核密度函数(tent-shapededgekernel)ꎮ综上所述ꎬLee和Lemieux(2010)[2]之后ꎬ运用RDD出现了IK和CCT两种最优带宽确定方法以及甜甜圈RDD两个新操作环节ꎮGelman和Imbens(2014)[23]不该使用超过两次的多项式的建议ꎬ尚未被学界普遍接受ꎮ同时ꎬ学界在Lee和Lemieux(2010)[2]涉及的RDD运用为方便讨论ꎬ使用矩形核密度函数的非参数估计虽然等同于参数估计ꎬ但此处只将其归为非参数估计ꎮ 47 ①经济管理环节上未达成共识ꎮLee和Lemieux(2010)[2]发表在权威经济学期刊«JournalofEconomicLit ̄erature»上ꎬ其两位作者均是国际上知名的经济学者ꎮ他们提出的RDD应用规范未被学界完全接受ꎬ并不因为他们的观点不准确㊁不权威ꎬ而是因为RDD的理论研究仍很活跃ꎬ故应用上达成的共识不多ꎻ因为RDD应用涉及众多技术环节ꎬ故更难达成共识ꎮ作为一个对比ꎬIV和DID的理论研究相对成熟㊁应用涉及的技术环节单一①ꎬ学者运用这两种方法做研究时的动作比较一致ꎮ四、断点回归设计的国内应用情况2011-2017年间ꎬCSSCI期刊共发表RDD经验研究论文46篇ꎬ涉及公共经济学㊁环境经济学㊁财政学等众多领域ꎬ其中使用行政管理数据的仅有王骏和孙志军(2015)[32]一篇ꎮ总体而言ꎬ对于两篇权威的RDD综述文章 Imbens和Lemieux(2008)[6]㊁Lee和Lemieux(2010)[2]ꎬ至少引用过其中一篇的中文论文有32篇ꎬ占69.57%ꎬ这意味着国内学者总体上了解运用RDD涉及的众多技术环节ꎮRDD应用的新进展也在被国内文献吸收ꎮ首先ꎬ确定最优带宽的IK法已被广泛应用ꎬCCT法也开始被使用ꎮ使用非参数估计且说明了带宽确定方法的24篇文章中ꎬ使用了IK法㊁CCT法的分别有10篇㊁3篇ꎮ其次ꎬ李宏彬等(2014)[33]㊁张川川等(2015)[34]和张明(2017)[35]都使用了甜甜圈RDDꎮ最后ꎬ马光荣等(2016)[36]㊁何文剑(2016)[37]㊁李江一和李涵(2017)[38]以及王家庭等(2017)[39]共4篇文章引用了Gelman和Im ̄bens(2014)[22]ꎮ与国外文献一样ꎬ国内文献运用RDD也存在很多与Lee和Lemieux(2010)[2]规范不一致的情况ꎬ具体如下:首先ꎬ同时使用参数估计和非参数估计的只有18篇(占39.13%)ꎮ只使用参数估计㊁只使用非参数估计的分别为17篇和11篇ꎮ在18篇同时使用了两种估计方法的文章中ꎬ将两种方法视为同等重要的有3篇ꎬ参数估计仅只是做稳健性检验㊁非参数仅只是做稳健性检验的分别为2篇和13篇ꎮ因此ꎬ总体来看ꎬ国内文献与国外文献一样ꎬ偏重于参数估计ꎮ其次ꎬ用前定变量做适用性检验时ꎬ仅有曹静等(2014)[40]㊁王骏和孙志军(2015)[32]使用了似不相关回归ꎮ再次ꎬ使用了参数估计的35篇文章中ꎬ只有杨小聪(2017)[41]㊁张英和陈绍志(2015)[42]㊁曹静等(2014)[40]3篇文章尝试到配置变量八九次的多项式ꎮ最后ꎬ在使用非参数估计且说明了所用核密度函数的11篇文章中ꎬ只使用矩形核密度函数㊁同时使用矩形和三角形核密度函数以及只使用三角形核密度函数的分别为2篇㊁5篇和4篇ꎮ由于运用RDD涉及众多技术环节ꎬ国内对RDD的运用还存在不少问题ꎬ主要表现在以下几个方面:首先ꎬ5篇文章未做适用性检验ꎬ2篇文章在适用性检验未通过时依然使用RDDꎮ张明(2017)[34]既没有用配置变量也没有用前定变量做适用性检验ꎮ陈强等(2017)[43]㊁黄新飞和杨丹(2017)[44]㊁高彦彦和王逸飞(2017)[45]以及王旭光(2017)[46]使用的配置变量不可能被操控ꎬ无须检验ꎬ但他们都没有检验前定变量的连续性ꎮ邹红和喻开志(2015)[47]㊁邓婷鹤和何秀荣(2016)[48]使用的配置变量 年龄 均在断点(60岁)处不连续ꎬ但依然运用RDDꎮ邹红57 ①运用IV必需的技术环节是报告第一阶段回归的F统计量取值ꎬ运用DID必需的技术环节是做平行趋势假设检验ꎮ经济管理和喻开志(2015)[47]做回归分析时去掉了60岁断点上的样本ꎬ但是出于男性实际停止工作的时间普遍是61岁这个考虑ꎬ并没有从RDD适用性这个角度深化㊁细化研究内容ꎬ譬如检验样本是否在50岁㊁60岁㊁70岁等年龄上存在堆积问题ꎮ其次ꎬ对描述统计图的运用不规范ꎮ如前所述ꎬ画图在RDD应用研究中十分必需ꎮ然而ꎬ有11篇没有画描述统计图ꎮ根据Lee和Lemieux(2010)[2]和英文顶级期刊的实际ꎬ画拟合曲线图以多项式方法居多ꎮ然而ꎬ在画有描述统计图的35篇文章中ꎬ15篇没有说明拟合曲线图所采用的方法ꎬ14篇采用局部多项式方法ꎬ6篇采用了平滑㊁多元回归等其他方法ꎮ未画描述统计图和未说明画拟合曲线所采用方法的文章合计达26篇(占56.52%)ꎬ表明国内学界对画图重要性的认识不够ꎮ最后ꎬ使用非参数估计时的关键技术细节未说明ꎮ在29篇使用了非参数估计的文章中ꎬ5篇没有报告带宽的确定方法ꎬ18篇(占62.07%)未说明使用了哪个核密度函数ꎮ五㊁总结和展望RDD在断点附近的局部等价于一个随机试验ꎬ这一特点使得其备受经验研究人员的青睐ꎮ在国外ꎬRDD从20世纪90年代末期开始被大量应用于经济学研究ꎮLee和Lemieux(2010)[2]提出了运用RDD的必备技术环节ꎬ相当于给出了RDD应用的规范ꎮ但是ꎬ由于RDD的理论研究仍在不断深入ꎬ至今尚未定型ꎬLee和Lemieux(2010)[2]之后应用RDD的文献出现了新的技术环节ꎬ譬如新的最优带宽确定方法㊁甜甜圈RDD和参数估计中最高只能使用二次多项式等ꎮ国内运用RDD做研究始于2010年ꎬ最近两年大幅增加ꎮ虽然国内熟悉Lee和Lemieux(2010)[2]提出的规范ꎬ但运用RDD时存在不少问题ꎬ譬如不做适用性检验㊁不重视描述统计图㊁不交代清楚非参数估计的关键细节等ꎮ为了使得研究结论更稳健ꎬ基于五大英文顶级期刊发表的论文ꎬ我们建议ꎬ国内运用RDD时ꎬ在完成Lee和Lemieux(2010)[2]提出的技术动作后ꎬ需要增加如下动作:考虑到学术界在最优带宽确定方法上尚未达成共识[26][49]ꎬ非参数估计时应该同时尝试CV㊁IK和CCT三种方法ꎻ在配置变量存在堆积现象或被操纵的可能时ꎬ使用甜甜圈RDDꎮ尝试到四次多项式ꎮRDD从理论上讲是更好的因果识别方法ꎬ但运用此方法要求在断点附近有较多观测值㊁对数据要求很高ꎮ可喜的是ꎬ最近几年ꎬ我国经济社会的数字化进程加速ꎬ越来越多的经济活动开始数据化ꎬ越来越多的中央政府部门和地方政府公开了行政管理数据ꎬ为运用RDD做研究提供了契机ꎮ特别值得指出的是ꎬ运用医疗卫生行业行政管理数据的研究已经成批量涌现ꎮ参考文献:[1]AngristJDꎬPischkeJ.Thecredibilityrevolutioninempiricaleconomics:Howbetterresearchdesignistak ̄ingtheconoutofeconometrics[J].JournalofEconomicPerspectivesꎬ2010ꎬ24(2):3-30.[2]LeeDSꎬLemieuxT.Regressiondiscontinuitydesignsineconomics[J].JournalofEconomicLiteratureꎬ2010ꎬ48(2):281-355.[3]ThistlethwaiteDLꎬCampbellDT.Regression-discontinuityanalysis:Analternativetotheexpostfactoex ̄periment[J].JournalofEducationalPsychologyꎬ1960ꎬ51(6):309-317.[4]雷晓燕ꎬ谭力ꎬ赵耀辉.退休会影响健康吗?[J].经济学(季刊)ꎬ2010ꎬ(04):1539-1558.[5]余静文ꎬ赵大利.城市群落的崛起㊁经济绩效与区域收入差距 基于京津冀㊁长三角和珠三角城市圈 67。
RD方法(regression discontinuity design)
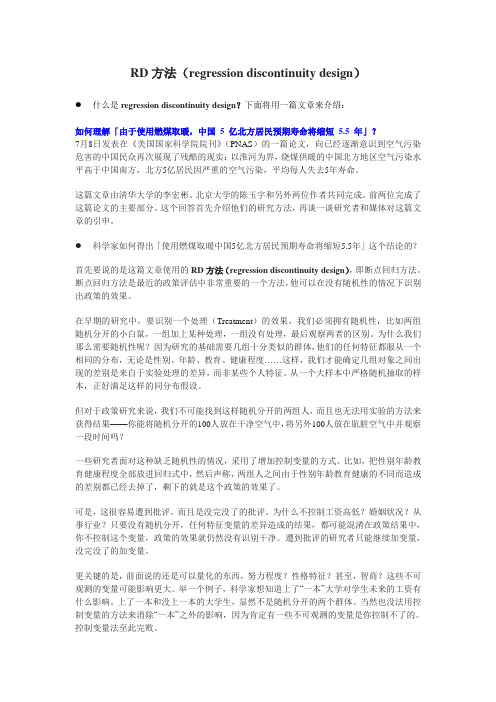
RD方法(regression discontinuity design)●什么是regression discontinuity design?下面将用一篇文章来介绍:如何理解「由于使用燃煤取暖,中国 5 亿北方居民预期寿命将缩短 5.5 年」?7月8日发表在《美国国家科学院院刊》(PNAS)的一篇论文,向已经逐渐意识到空气污染危害的中国民众再次展现了残酷的现实:以淮河为界,烧煤供暖的中国北方地区空气污染水平高于中国南方,北方5亿居民因严重的空气污染,平均每人失去5年寿命。
这篇文章由清华大学的李宏彬、北京大学的陈玉宇和另外两位作者共同完成。
前两位完成了这篇论文的主要部分。
这个回答首先介绍他们的研究方法,再谈一谈研究者和媒体对这篇文章的引申。
●科学家如何得出「使用燃煤取暖中国5亿北方居民预期寿命将缩短5.5年」这个结论的?首先要说的是这篇文章使用的RD方法(regression discontinuity design),即断点回归方法。
断点回归方法是最近的政策评估中非常重要的一个方法,他可以在没有随机性的情况下识别出政策的效果。
在早期的研究中,要识别一个处理(Treatment)的效果,我们必须拥有随机性,比如两组随机分开的小白鼠,一组加上某种处理,一组没有处理,最后观察两者的区别。
为什么我们那么需要随机性呢?因为研究的基础需要几组十分类似的群体,他们的任何特征都服从一个相同的分布,无论是性别、年龄、教育、健康程度……这样,我们才能确定几组对象之间出现的差别是来自于实验处理的差异,而非某些个人特征。
从一个大样本中严格随机抽取的样本,正好满足这样的同分布假设。
但对于政策研究来说,我们不可能找到这样随机分开的两组人,而且也无法用实验的方法来获得结果——你能将随机分开的100人放在干净空气中,将另外100人放在肮脏空气中并观察一段时间吗?一些研究者面对这种缺乏随机性的情况,采用了增加控制变量的方式。
财政学名词解释word英文版

财政学名词解释word英⽂版Chapter ONE1.public finance(public sector econmics/public economics): The field of econmics that analyzes government taxation and spending.2.unified budget:The document that includes all the federal government’s revenues and expenditures.3.regulatory budget:An annual statement of the costs imposed on the economy by government regulations.(xianzaimeiyoule)4.entitlement programs:Programs whose expenditures are determined by the number of people who qualify, rather than preset budget allocations.Chapter TWO5.substitution effect:The tendency of an individual to consume more of one goodand less of another because of a decrease in the price of theformer relative to the latter.6.normal good:A good for which demand increases as income increases anddemand decreases as income decreases, other things beingthe same.7.income effect:The effect of a price change on the quantity demanded dueexclusively to the fact that the consumer’s income haschanged.8.correlation:A measure of the extent to which two events move together.9.treatment group:The group of individuals who are subject to the intervention being studied.10.control group:The comparison group of individuals who are not subject to the intervention being studied.11.biased estimate:An estimate that conflates the true causal impact with the impact of outside factors.12.coumterfactual:The outcome for people in the treatment group had they not been treated.13.experimental study:An empirical study in which individuals are randomly assigned to the treatment and control groups.14.observational study:An empirical study that relies on observed data that are not obtained from an experimental setting.15.econometrics:The statistical tools for analyzing economic data.16.regression line:The line that provides the best fit through a scatter of data points.17.standard error:A statistical measure of how much an estimated regression coefficient might vary from itstrue value.18.cross-sectional data:Data that contain information on entities at a given point in time.19.time-series data:Data that contain information on an entity at different points in time.20.panel data:Data that contain information on individual entities at different point of time.21.quasi-experimental study:An observational study that relies on circumstances outside of the researcher’s control to mimic random assignment. 22.differerce-in-difference analysis:An analysis that compares changes over time in an outcome of the treatment group to changes over the same time period in the outcome of the control group.23.instrumental variables analysis:An analysis that relies on finding some variable that affects entry into thetreatment group, but in itself is not correlated with the outcome variable.24.regression-discontinuity analysis: An analysis that relies on a strict cut-off criterion for eligibility of the intervention under study in order to approximate an experimental design.Chapter THREE25.welfare economics:The branch of economic theory concerned with thesocial desirability of alternative economic states.26.edgeworth box:A device used to depict the distribution of goods in atwo good-two person world.27.pareto efficient:An allocation of resources such that no person can bemade better off without making another person worseoff.28.pareto improvement:A reallocation of resources that makes at least oneperson better off without making anyone else worse off.29.contract curve:The locus of all pareto efficient points.30.production possibilities curve:A graph that shows the maximum quantity of one output that can be produced, given the amount of the other output.31.marginal rate of transformation:The rate at which the economy can transform one good into another good; it is the absolute value of the slope of the production possibilities frontier.32.marginal cost:The incremental cost of producing one more unit of output.33.utility possibilities curve:A graph showing the maximum amount of one person’s utility given each level of utilityattained by the other person.34.social welfare function:A function reflecting society’s views on how the utilities of its members affect the well-beingof society as a whole.35.monopoly:A market with only one seller of a good.36.asymmetric information:A situation in which one party engaged in an economic transaction has better informationabout the good or service traded than the other party.37.externality:A cost or benefit that occurs when the activity of one entity directly affects the welfare ofanother in a way that is outside the market mechanism.38.public good:A good that is nonrival and nonexcludable in consumption.39.merit good:A commodity that ought to be provided even if people do not demand it.Chapter FOUR40.Pure public good:A commodity that is nonrival and nonexcludable inconsumption.41.Private good:A commodity that is rival and excludable inconsumption.42.Impure public good:A good that is rival and/or excludable to some extent.43.Publicly provided private goods:rival and excludable commodities that are provided bygovernments.44.Horizontal summation:The process of creating a market demand curve bysumming the quantities.45.Vertical summation:the process of aggregate demand curve for a public good by adding the prices each individual is willing to pay for a given quantity of the good.46.Free rider:the incentive to let other people pay for a public good while you enjoy the benefits.47.Perfect price discrimination:when a producer charges each person the maximum he or she is willing to pay the good.48.Privatization:The process of turning services that are supplied by the government over to the private sector for provision and/or production. /doc/f221ced2a7c30c22590102020740be1e640ecc41.html modity egalitarianism:the idea that some commodities ought to be made available to everybody.Chapter FIVE50.Externality:A cost or benefit that occurs when the activity of one entity directly affects the welfare ofanother in a way that is outside the market mechanism.51.Coase theorem:Provided that transaction costs are negligible, an efficient solution to an externality problem is achieved as long as someone is assigned property rights independent of who is assigned those rights52.Pigouvian tax:A tax levied on each unit of an externality generator’s output in an amount equal to themarginal damage at the efficient level of output.53.Emissions fee:A tax levied on each unit of pollution54.Cost effective:A policy that achieves a given outcome at the lowest cost possible.55.Congestion pricing:A tax levied on driving equal to the marginal congestion costs imposed on other drivers.56.Cap and trade:A policy of granting permits to pollute, with the number of permits set at the desiredpollution level, and allowing pollutes to trade the permits.57.Safety value price:Within a cap and trade system, a price set by government at which polluters can purchases additional permits beyond the cap.58.Incentive based regulations:Policies that provided polluters with financial incentives to reduce pollution./doc/f221ced2a7c30c22590102020740be1e640ecc41.html mand-and-control regulations: Policies that require a given amount of pollution reduction with limited or no flexibility to how it may be achieved,60.Technology standard:A type of command-and-control regulation that requires firms to use a particular technologyto reduce their pollution.61.Performance standard:A command-and-control regulation that sets an emissions goal for each individual polluterand allows some flexibility in meeting the goal62.Hot spots:Localized concentration of emission.Chapter SIX63.Political economy:The field that applies economics principles to the analysisof political decision making.64.Lindahl prices:The tax share an individual must pay par unit of publicgood65.Majority voting rule:One more than half voters muse favor a measure for it tobe approved66.V oting paradox:With majority voting community preferences can beinconsistent even though each individual’s preferencesare consistent67.Agenda manipulation:The process of organizing the order in which votes are taken to ensure a favorable outcome。
rdd(断点回归)名词解释
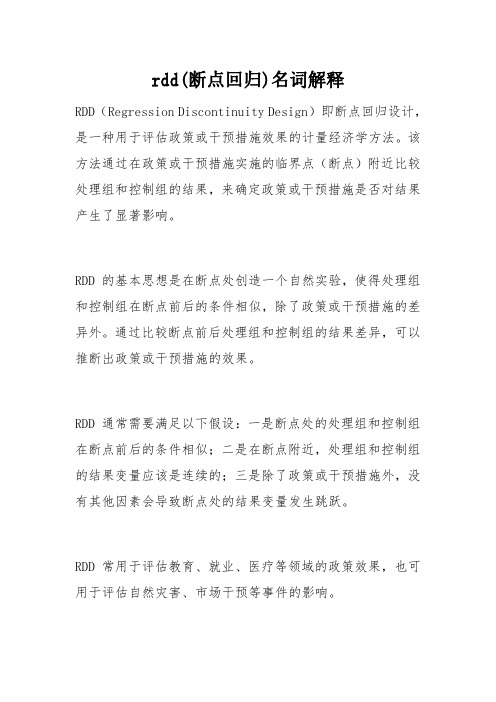
rdd(断点回归)名词解释
RDD(Regression Discontinuity Design)即断点回归设计,是一种用于评估政策或干预措施效果的计量经济学方法。
该方法通过在政策或干预措施实施的临界点(断点)附近比较处理组和控制组的结果,来确定政策或干预措施是否对结果产生了显著影响。
RDD的基本思想是在断点处创造一个自然实验,使得处理组和控制组在断点前后的条件相似,除了政策或干预措施的差异外。
通过比较断点前后处理组和控制组的结果差异,可以推断出政策或干预措施的效果。
RDD通常需要满足以下假设:一是断点处的处理组和控制组在断点前后的条件相似;二是在断点附近,处理组和控制组的结果变量应该是连续的;三是除了政策或干预措施外,没有其他因素会导致断点处的结果变量发生跳跃。
RDD常用于评估教育、就业、医疗等领域的政策效果,也可用于评估自然灾害、市场干预等事件的影响。
- 1、下载文档前请自行甄别文档内容的完整性,平台不提供额外的编辑、内容补充、找答案等附加服务。
- 2、"仅部分预览"的文档,不可在线预览部分如存在完整性等问题,可反馈申请退款(可完整预览的文档不适用该条件!)。
- 3、如文档侵犯您的权益,请联系客服反馈,我们会尽快为您处理(人工客服工作时间:9:00-18:30)。
Key Identifying Assumption
Key identifying assumption: E [Y0i jXi ] and E [Y1i jXi ] are continuos in Xi at X0 . This means that all other unobserved determinants of Y are continuously related to the running variable X . This allows us to use average outcomes of units just below the cuto¤ as a valid counterfactual for units right above the cuto¤. This assumption cannot be directly tested. But there are some tests which give suggestive evidence whether the assumption is satis…ed (see below).
1 2
Sharp RD: treatment is a deterministic function of a covariate X. Fuzzy RD: exploits discontinuities in the probability of treatment conditional on a covariate X (the discontinuity is then used as an IV).
10 / 48
Waldinger (Warwick)
Di¤erent Polynomials on the 2 Sides of the Discontinuity
To derive a regression model that can be used to estimate the causal e¤ect we use the fact that Di is a deterministic function of Xi : E [Yi jXi ] = E [Y0i jXi ] + (E [Y1i jXi ] E [Y0i jXi ])Di
Lecture 4: Regression Discontinuity Design
Fabian Waldinger
Waldinger ()
1 / 48
Topics Covered in Lecture
1 2 3 4 5
Sharp RD. Fuzzy RD. Practical Tips for Running RD Models. Example Fuzzy RD: Angrist & Lavy (1999) - Maimonides Rule. Regression Kink Design (very brie‡y).
1 2
Use a nonparametric kernel method (see more below). Use a pth order polynomial: i.e. estimate: Yi = α + β1 xi + β2 xi2 + ... + βp xip + ρDi + η i (1)
Sharp Regression Discontinuity - Nonlinear Case
Suppose the nonlinear relationship is E [Y0i jxi ] = f (Xi ) for some reasonably smooth function f (Xi ). In that case we can construct RD estimates by …tting: Yi = f (xi ) + ρDi + η i There are 2 ways of approximating f (xi ):
Waldinger (Warwick)
7 / 48
Sharp Regression Discontinuity - Nonlinear Case
Sometimes the trend relation E [Y0i jxi ] is nonlinear.
Waldinger (Warwick)
8 / 48
During the following introduction we will focus on the pth order polynomial approach but will discuss the other approach below.
Waldinger (Warwick)
9 / 48
(2)
Equation (1) above is a special case of (2) with β1 = β2 = βp = 0. The treatment e¤ect at Xo is ρ. The treatment e¤ect at Xi X0 = c > 0 is: ρ + β1 c + β2 c 2 + ...+ βp c p
Waldinger (Warwick) 11 / 48
Example Sharp RD: Lee (2008) Incumbency E¤ects
Lee (2008) uses a sharp RD design to estimate the probability that the incumbent wins an election. A large political science literature suggests that incumbents may use privileges and resources of o¢ ce to gain an advantage over potential challengers. An OLS regression of incumbency status on election success is likely to be biased because of unobserved di¤erences. Incumbents have already won an election so they may just be better. Lee analyzes the incumbency e¤ect using Democratic incumbents for US congressional elections. He analyzes the probability of winning the election in year t+1 by comparing candidates who just won compared to candidates who just lost the election in year t.
Di¤erent Polynomials on the 2 Sides of the Discontinuity
We can generalize the function f (xi ) by allowing the xi terms to di¤er on both sides of the threshold by including them both individually and interacting them with Di . As Lee and Lemieux (2010) note, allowing di¤erent functions on both sides of the discontinuity should be the main results in an RD paper (as otherwise we use values from both sides of the cuto¤ the estimate the function on each side). In that case we have: ei + β X e2 ep E [Y0i jXi ] = α + β01 X 02 i + ... + β0 p Xi ei + β X e2 ep E [Y1i jXi ] = α + ρ + β11 X 12 i + ... + β1 p Xi
RD captures the causal e¤ect by distinguishing the nonlinear and discontinuous function, 1(Xi Xo ) from the smooth function f (Xi ).
Waldinger (Warwick)
The regression model which you estimate is then: Yi = α + β01 e xi + β02 e xi2 + ... + β0p e xip
where β1 = β11
xi + β2 Di e xi2 + ... + βp Di e xip + η i + ρ Di + β 1 Di e β01 , β2 = β21 β21 and βp = β1p β0 p
ei = Xi where X
X0 Centering at X0 ensures that the treatment e¤ect at Xi = X0 is the coe¢ cient on Di in a regression model with interaction terms (because you do not have to add values of the Di interacted with X to get the treatment e¤ect at X0 ).