基于模糊滑模的卫星姿态控制系统故障诊断
基于模糊控制的航天器姿态控制系统设计
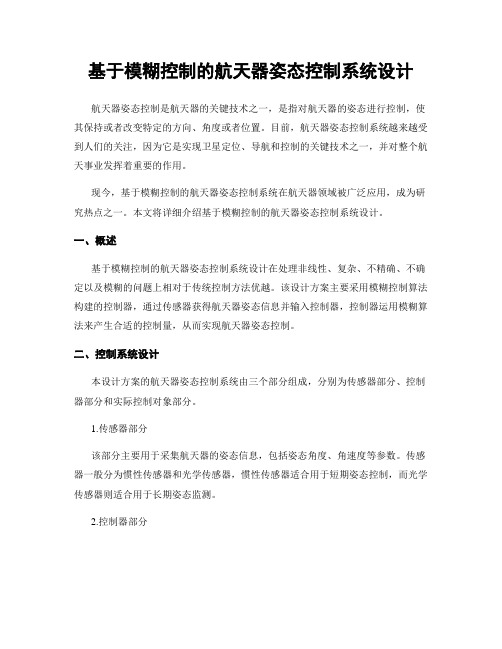
基于模糊控制的航天器姿态控制系统设计航天器姿态控制是航天器的关键技术之一,是指对航天器的姿态进行控制,使其保持或者改变特定的方向、角度或者位置。
目前,航天器姿态控制系统越来越受到人们的关注,因为它是实现卫星定位、导航和控制的关键技术之一,并对整个航天事业发挥着重要的作用。
现今,基于模糊控制的航天器姿态控制系统在航天器领域被广泛应用,成为研究热点之一。
本文将详细介绍基于模糊控制的航天器姿态控制系统设计。
一、概述基于模糊控制的航天器姿态控制系统设计在处理非线性、复杂、不精确、不确定以及模糊的问题上相对于传统控制方法优越。
该设计方案主要采用模糊控制算法构建的控制器,通过传感器获得航天器姿态信息并输入控制器,控制器运用模糊算法来产生合适的控制量,从而实现航天器姿态控制。
二、控制系统设计本设计方案的航天器姿态控制系统由三个部分组成,分别为传感器部分、控制器部分和实际控制对象部分。
1.传感器部分该部分主要用于采集航天器的姿态信息,包括姿态角度、角速度等参数。
传感器一般分为惯性传感器和光学传感器,惯性传感器适合用于短期姿态控制,而光学传感器则适合用于长期姿态监测。
2.控制器部分该部分是本系统的核心部分,主要负责产生控制量来控制航天器姿态。
本设计方案的控制器主要采用模糊控制算法,该算法具有处理不确定因素和非线性因素的特点,能够适应实际控制对象的动态特性。
为了保证该控制器的精度和稳定性,需要对其进行模糊规则库的建立和调整。
首先,需要根据航天器的姿态参数构建模糊规则库,然后对模糊规则库进行优化和调整,以满足控制器的精度和稳定性要求。
3.实际控制对象部分该部分主要是实际控制对象,也就是要控制的航天器。
本设计方案的控制对象为六自由度的刚体,可以采用非线性动力学模型来描述。
基于上述三个部分,本设计方案的姿态控制系统可以实现航天器的姿态控制,具有精度高、响应速度快、适应性强等优点。
三、实验结果基于模糊控制的航天器姿态控制系统设计的实验结果表明,该系统具有很好的姿态控制效果。
基于滑模观测器的故障检测与重构方法
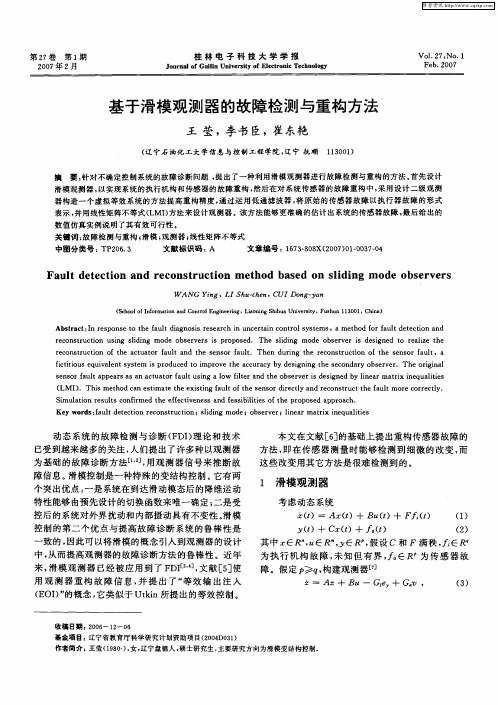
中 图 分 类 号 :TP206.3
文 献 标 识 码 :A
文 章 编 号 :1673—808X(2007)01—0037—04
Fault detection and reconstruction m ethod based on sliding m ode observers
W A N G Ying ,£, Shu-chen,CU I D ong—yah
维普资讯
第 27卷 第 1期 2007年 2月
桂 林 电 子 科 技 大 学 学 报
Journal of Guilin University of Electronic Technology
V o1.27,No.1 Feb.2007
基于滑模观 测器的故障检测与重构方法
(2)
其 中 X∈R ,U∈R ,Y∈Rp,假 设 c和 F 满秩 , ∈
为执 行 机 构 故 障 ,未 知 但 有 界 , ∈R 为 传 感 器 故
障 。假 定 ≥g,构 建观 测器[
之 : Az+ Bu — G e + G 7. 2,
(3)
收 稿 日期 :2OO6— 12一O6 基 金项 耳:辽宁省教育厅科学研究计划资助项 目(2OO4DO31) 作者简介 :王莹(1980一),女,辽宁盘锦人 ,硕士研 究生,主要研究方向为滑模变结构控制.
动态 系统 的故 障 检测 与 诊 断 (FDI)理 论 和 技 术 已受 到越来 越多 的关注 ,人们提 出 了许多种 以观 测器 为 基础 的 故障 诊 断方 法rh ,用观 测 器 信号 来 推 断故 障信 息 。滑模控 制是 一种特 殊 的变 结构 控制 。它有两 个 突 出优 点 :一 是系统 在到达 滑动 模态后 的降 维运 动 特性 能够 由预先 设计 的切换 函数 来唯一 确定 ;二是 受 控后 的系统对外 界扰 动和 内部摄 动具有 不变性 。滑 模 控 制 的第 二个 优 点与提 高 故 障诊 断 系统 的鲁棒 性 是 一 致 的 ,因此 可 以将 滑模 的概念 引入到 观测 器的设 计 中 ,从而 提高 观测器 的故 障诊断 方法 的鲁棒 性 。近 年 来 ,滑 模观 测 器 已经 被 应 用到 了 FDI[。“],文 献 rs]使 用 观 测 器 重 构 故 障 信 息 ,并 提 出 了 “等 效 输 出 注 入 (EOI)”的概念 ,它类 似于 Utkin所提 出的等 效控 制 。
基于深度神经网络的航天器姿态控制系统故障诊断与容错控制研究
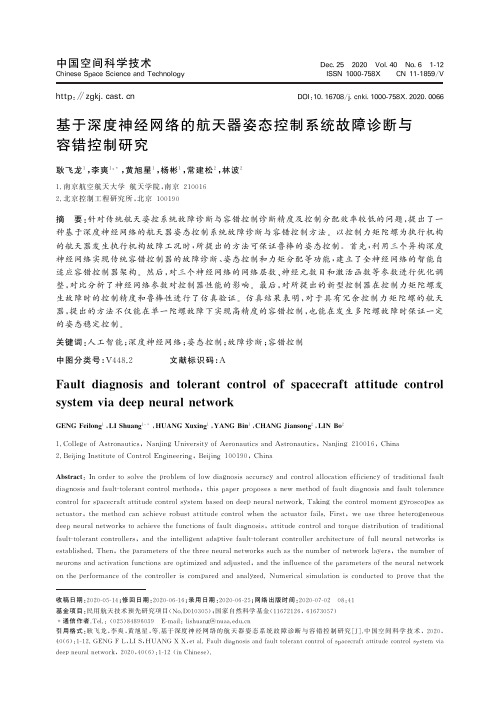
n
t
e
l
l
i
tadap
t
i
vef
au
l
t
G
t
o
l
e
r
an
tc
on
t
r
o
l
l
e
ra
r
ch
i
t
e
c
t
u
r
eo
ff
u
l
lneu
r
a
lne
two
r
ksi
s
gen
e
s
t
ab
l
i
shed.Then,t
hepa
r
ame
t
e
r
so
ft
het
hr
e
eneu
r
a
lne
two
r
kssucha
st
henumbe
ro
fne
的姿态稳定控制.
关键词:人工智能;深度神经网络;姿态控制;故障诊断;容错控制
中图分类号:
V448.
2 文献标识码:
A
Fau
l
td
i
agno
s
i
sandt
o
l
e
r
an
tc
on
t
r
o
lo
fs
c
e
c
r
a
f
ta
t
t
i
t
ud
ec
on
t
r
o
l
pa
s
s
t
基于模糊贝叶斯风险和T-S模糊模型的故障诊断
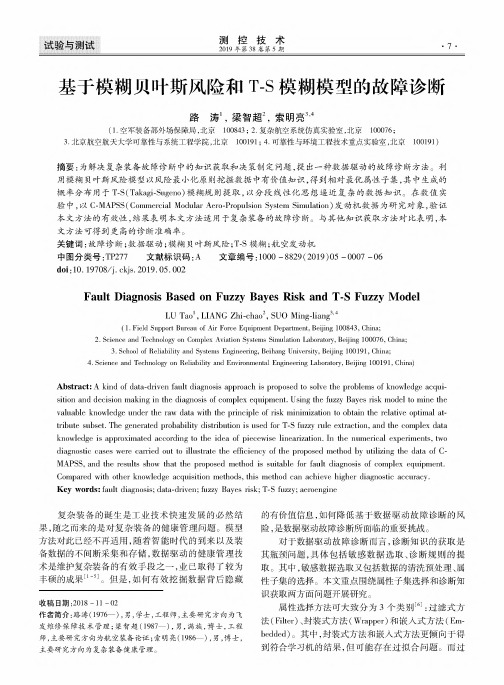
测控技术2019年第38卷第5期・7・试验与测试基于模糊贝叶斯风险和T-S模糊模型的故障诊断路涛J梁智超2,索明亮3,(1.空军装备部外场保障局,北京100843;2.复杂航空系统仿真实验室,北京100076;3.北京航空航天大学可靠性与系统工程学院,北京100191;4.可靠性与环境工程技术重点实验室,北京100191)摘要:为解决复杂装备故障诊断中的知识获取和决策制定问题,提出一种数据驱动的故障诊断方法。
利用模糊贝叶斯风险模型以风险最小化原则挖掘数据中有价值知识,最,其中的概率分布用于T-S(Takagi-Sugeno)模糊规则提取,以分段线性化思想逼近复杂的数据知识。
在数值实验中,以C-MAPSS(Commercial Modular Aero-Propulsion System Simulation)发动机数据为研究对象,验证方法的有,结果表明本文方法适用于复杂装备的故障诊断。
知识获取方法明,本文方法的诊断准确率。
关键词:故障诊断;数据驱动;模糊贝叶斯风险;T-S模糊;航空发动机中图分类号:TP277文献标识码:A文章编号:1000-8829(2019)05-0007-06doi:10.19708/j.ckjs.2019.05.002Fault Diagnosis Based on Fuzzy Bayes Risk and T-S Fuzzy ModelLU Tao1,LIANG Zhi-chao2,SUO Ming-liang3,4(1.Field Support Bureau of Air Force Equipment Department,Beijing100843,China;2.Science and Technology on Complex Aviation Systems Simulation Laboratory,Beijing100076,China;3.School of Reliability and Systems Engineering,Beihang University,Beijing100191,China;4.Science and Technology on Reliability and Environmental Engineering Laboratory,Beijing100191,China)Abstract:A kind of data-driven fault diagnosis approach is proposed to solve the problems of knowledge acquisition and decision making in the diagnosis of complex ing the fuzzy Bayes risk model to mine the valuable knowledge under the raw data with the principle of risk minimization to obtain the relative optimal attribute subset.The generated probability distribution is used for T-S fuzzy rule extraction,and the complex data knowledge is approximated according to the idea of piecewise linearization.In the numerical experiments,two diagnostic cases were carried out to illustrate the efficiency of the proposed method by utilizing the data of C-MAPSS,and the results show that the proposed method is suitable for fault diagnosis of complex equipment.Compared with other knowledge acquisition methods,this method can achieve higher diagnostic accuracy.Key words:fault diagnosis;data-driven;fuzzy Bayes risk;T-S fuzzy;aeroengine复杂装备的诞生是工业技术快速发展的必然结果,随之而来的是对复杂装备的健康管理问题。
《电液位置伺服控制系统的模糊滑模控制方法研究》
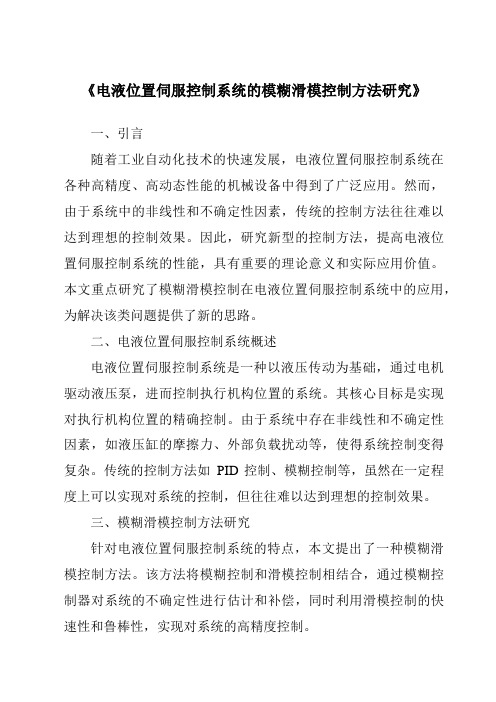
《电液位置伺服控制系统的模糊滑模控制方法研究》一、引言随着工业自动化技术的快速发展,电液位置伺服控制系统在各种高精度、高动态性能的机械设备中得到了广泛应用。
然而,由于系统中的非线性和不确定性因素,传统的控制方法往往难以达到理想的控制效果。
因此,研究新型的控制方法,提高电液位置伺服控制系统的性能,具有重要的理论意义和实际应用价值。
本文重点研究了模糊滑模控制在电液位置伺服控制系统中的应用,为解决该类问题提供了新的思路。
二、电液位置伺服控制系统概述电液位置伺服控制系统是一种以液压传动为基础,通过电机驱动液压泵,进而控制执行机构位置的系统。
其核心目标是实现对执行机构位置的精确控制。
由于系统中存在非线性和不确定性因素,如液压缸的摩擦力、外部负载扰动等,使得系统控制变得复杂。
传统的控制方法如PID控制、模糊控制等,虽然在一定程度上可以实现对系统的控制,但往往难以达到理想的控制效果。
三、模糊滑模控制方法研究针对电液位置伺服控制系统的特点,本文提出了一种模糊滑模控制方法。
该方法将模糊控制和滑模控制相结合,通过模糊控制器对系统的不确定性进行估计和补偿,同时利用滑模控制的快速性和鲁棒性,实现对系统的高精度控制。
1. 模糊控制器设计模糊控制器是本方法的核心部分。
通过对系统的不确定性因素进行观察和学习,模糊控制器可以自动调整其参数,以适应系统状态的变化。
在电液位置伺服控制系统中,模糊控制器通过接收系统的位置、速度等信息,利用模糊推理机制对系统的不确定性进行估计和补偿。
2. 滑模控制器设计滑模控制是一种变结构控制方法,其核心思想是根据系统状态的变化,实时调整系统的控制策略。
在电液位置伺服控制系统中,滑模控制器通过设计适当的滑模面和滑模控制律,使系统在受到外部扰动时,能够快速地回到预设的滑模面上,从而实现高精度的位置控制。
四、实验验证与分析为了验证本文提出的模糊滑模控制方法的有效性,我们进行了大量的实验。
实验结果表明,与传统的控制方法相比,模糊滑模控制方法在电液位置伺服控制系统中具有更好的控制性能。
基于干扰观测器的卫星姿态误差四元数模糊滑模控制
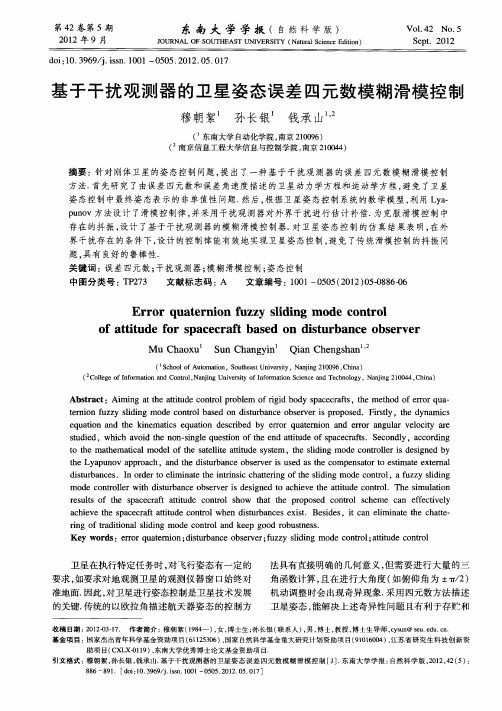
s did,whih a i e no -i l ue to ft e e d at ud fs c c a t. S c n y,a c r i g u t e c vod t n sng eq si n o h n ti e o pa e r fs e o dl h t c odn t e mah mai a d lo e s tli ti d yse ,t e si i g mo e c nr le sde i n d b o t t e tc lmo e ft ae l e a t u e s t m h h t t h ld n d o to lri sg e y
标姿态 四元数 Q =[c 。 q q r, q q。 以 系统初 o , 始姿态四元数 Q= q q q q r, [ 。 。 。 。 则卫星本体
坐标系 到轨道坐标 系的姿态误差 四元 数 Q。 定义为
d su b n e .I r e o ei n t h nt nsc c atrn ft e si i g mo e c n r l uz y si i g it r a c s n o d rt l i m ae t e i r i h te g o ld n d o to ,a f z ld n i i h mo e c n o lrwih d su b n e o e v ri sg e o a h e e t e at u e c n o .The smulto d o t l t it r a c bs r e sde i n d t c iv h ti d o t 1 r e t r i ain r s ls o t e p c c at t t d c n o s ow t a t e r p e c n o s h me a e f c i l e u t f h s a e r f a t u e o t l h i r h t h p o os d o t l c e c n fe t y r ve
深空探测航天器姿态控制系统故障定位

1 引言
深空探测航天器研发周期长、成本高, 而深空探测 具有面临环境复杂、任务周期长以及任务多样化等特 点, 提高星上控制系统可靠性和容错运行能力是深空
探测任务迫切而具有挑战性的研究重点. 姿态控制系 统(attitude control system, ACS)是卫星最关键的分系 统之一, 却是卫星故障易发的分系统, 而一旦姿控系 统发生故障, 将影响卫星任务执行效率, 严重时甚至
第 36 卷第 12 期 2019 年 12 月
控制理论பைடு நூலகம்应用
Control Theory & Applications
Vol. 36 No. 12 Dec. 2019
深空探测航天器姿态控制系统故障定位
叶正宇1, 程月华2†, 韩笑冬3, 姜 斌2
(1. 南京航空航天大学 航天学院, 江苏 南京 211100; 2. 南京航空航天大学 自动化学院, 江苏 南京 211100; 3. 中国空间技术研究院 通信卫星事业部, 北京 100830)
3. Communications Satellite Division, China Academy of Space Technology, Beijing 100830, China) Abstract: Fault detection and location is the prerequisite and guarantee for the reliability of the satellite for deep space exploration missions. Combined with the analysis of the attitude control system (ACS) mechanism, we present a datadriven fault diagnosis (FD) method based on neural networks and support vector machines (SVM) to detect and locate fault. The FD scheme is composed of 3 steps. Firstly, the sensor signals of the ACS are collected, and a neural network is applied to model the unknown dynamic characteristic of closed-loop ACS and make predictions; Then, ACS sensor signals are compared with the neural network predictions to generate residuals and extract the fault features; Finally, an SVM is applied to identify the fault features to realize fault detection. And combined with kinematic analysis, fault location is realized. The simulation results show that the proposed method can effectively extract and identify the fault features and is capable of realizing the fault detection and location of actuator and sensor. Key words: fault location; neural networks; support vector machines; attitude control system Citation: YE Zhengyu, CHENG Yuehua, HAN Xiaodong, et al. Fault location for attitude control systems of deep space exploration satellites. Control Theory & Applications, 2019, 36(12): 2093 – 2099
卫星姿态控制系统的故障诊断研究
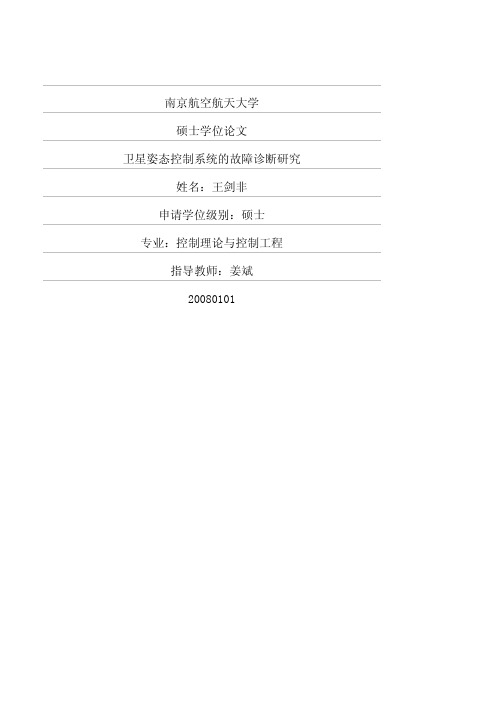
规律:失、升、氧、得、降、还
问题六 :氧化复原反响与四大根本反响的关系?
学 生
①
2Mg + O2 = 2MgO
〔化合,氧还〕
练 习
②
CaO
+
CO2
=
CaCO3
〔化合,非氧还〕
③ H2CO3 = H2O + CO2
〔分解,非氧还〕
④ 2H2O = 2H2↑+ O2↑
〔分解,氧还〕
教材分析
1、教材的地位和作用
只有让学生掌握氧化复原反响的根本概念才能 使他们理解这些反响的本质。学生对本节教材 掌握的好坏直接影响着其以后对化学的学习。 本节教材安排在这里是承前启后、符合情理。 它既涉及了初中的根本反响类型及氧化反响、 复原反响的重要知识并以此为铺垫展开对氧化 复原反响的较深层次的学习,又还将是今后联 络元素化合物知识的重要纽带。所以这样的编 排充分表达了教学大纲“螺旋式上升〞的根本 思想。
教学难点
理解氧化复原反响的本质是发生了电子转移
教法分析
“将课堂还给学生,让课堂焕发出生命的活力〞是进 展教学的指导思想,充分发挥老师的主导作用和学生 的主体作用。结合教材及学生的实际将采取以下教学 方法:
1、以激发学生的学习动机为主线,通过提问,讨论 等形式,调动学生学习的积极性。
2、以知识有层次构造为根底,通过分析,讲解,让 老师的认识转化为学生的认识。 3、以思维训练为核心,通过提问,讨论到达使学生多 种感官同时合作,多项信息综合反响的作用,充分发 挥学生的主动作用
⑤
NaOH + HCl = NaCl
〔复分解,非氧还〕
+
H2O
iData_基于新型终端滑模的航天器执行器故障容错姿态控制_胡庆雷
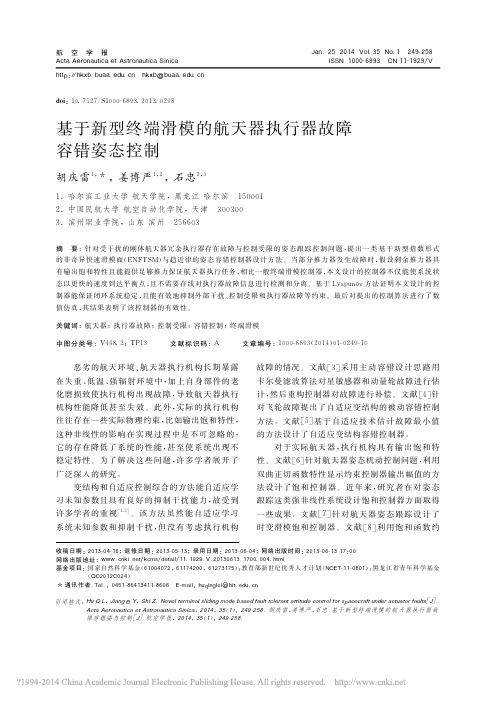
航天器执行机构长期暴露 恶劣的航天 环 境 、 在失 重 、 低 温、 强 辐 射 环 境 中, 加上自身部件的老 化磨损致使执行 机 构 出 现 故 障 , 导致航天器执行 机构性能降 低 甚 至 失 效 。 此 外 , 实际的执行机构 往往存在一些实际物理约束 , 比如输出饱和特性 , 这种非线性的影 响 在 实 现 过 程 中 是 不 可 忽 略 的 , 它的存在降低了 系 统 的 性 能 , 甚至使系统出现不 稳定特性 。 为了 解 决 这 些 问 题 , 许多学者展开了 广泛深入的研究 。 变结构和自适应控制综合的方法能自适应学 故受到 习未知参数且具 有 良 好 的 抑 制 干 扰 能 力 , 许多学者的 重 视
J a n . 2 5 2 0 1 4V o l . 3 5N o . 1 2 4 9 2 5 8 - / I S S N 1 0 0 0 6 8 9 3 N 1 1 1 9 2 9 V - C -
基于新型终端滑模的航天器执行器故障 容错姿态控制
2 3 ,石忠2, 胡庆雷1,* ,姜博严1,
2 5 0
航 空 学 报
×
J a n . 2 5 2 0 1 4V o l . 3 5N o . 1
束控制器输出 , 并定义了一个饱和系数作为饱和 再通过 对 这 个 系 数 的 自 适 应 学 习 消 除 度的指标 , 了系统中的非线 性 项 。 文 献 [ 也通过饱和函数 9] 约束控制器输出 , 但是控制器中并没有直接对饱 和系数进行自适 应 , 而是通过对非线性增益和饱 和系数混 合 项 进 行 自 适 应 来 消 除 系 统 的 非 线 性 部分 。 基于终端滑模的设计方法综合了有限时间收 敛和滑模鲁棒性 强 的 优 点 , 既能保证系统状态在 有限时间内收敛 到 平 衡 点 , 又具有较好的鲁棒性
基于自适应模糊滑模的卫星姿态控制方法
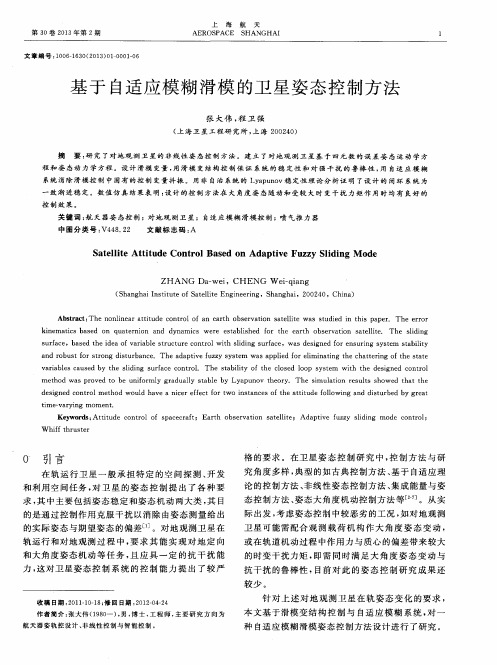
a n d r o b u s t f o r s t r o n g d i s t u r b a n c e . Th e a d a p t i v e f u z z y s y s t e m wa s a p p l i e d f o r e l i mi n a t i n g t h e c h a t t e r i n g o f t h e s t a t e v a r i a b l e s c a u s e d b y t h e s l i d i n g s u r f a c e c o n t r o 1 . Th e s t a b i l i t y o f t h e c l o s e d l o o p s y s t e m wi t h t h e d e s i g n e d c o n t r o l
S a t e l l i t e At t i t u d e Co nt r o l Ba s e d o n Ad a pt i v e Fu z z y S l i di n g Mo d e
ZH A N G Da — we i , CH EN G We i — q i a n g
( 上 海 卫 星 工 程 研 究所 , 上海 2 0 0 2 4 0 )
摘 要 : 研 究 了 对地 观 测 卫 星 的 非 线 性 姿 态控 制 方 法 。 建 立 了对 地 观 测 卫 星 基 于 四 元 数 的误 差 姿 态 运 动 学方
程 和姿 态 动 力 学 方 程 。设 计 滑 模 变量 , 用 滑 模 变结 构 控 制 保 证 系统 的 稳 定 性 和 对 强 干 扰 的 鲁 棒 性 , 用 自适 应 模 糊 系统 消 除 滑模 控 制 中 固有 的 控 制 变量 抖 振 。 用 非 自治 系统 的 L y a p u n o v 稳 定性 理论 分 析 证 明 了设 计 的 闭环 系统 为
故障诊断论文:基于聚类分析的卫星姿态控制系统故障诊断方法研究
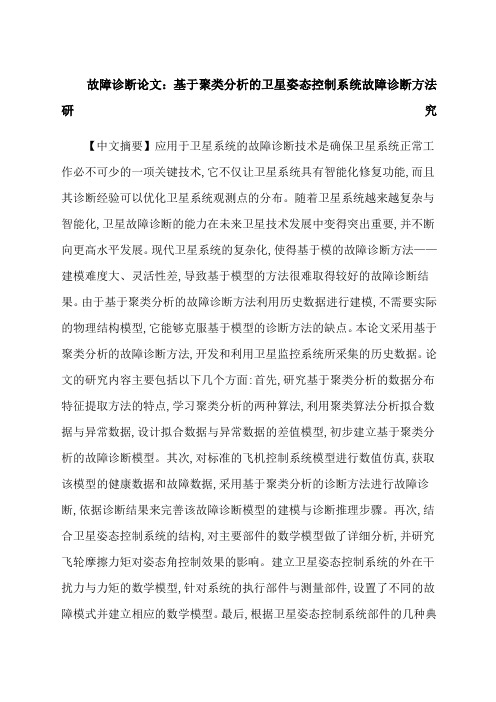
故障诊断论文:基于聚类分析的卫星姿态控制系统故障诊断方法研究【中文摘要】应用于卫星系统的故障诊断技术是确保卫星系统正常工作必不可少的一项关键技术,它不仅让卫星系统具有智能化修复功能,而且其诊断经验可以优化卫星系统观测点的分布。
随着卫星系统越来越复杂与智能化,卫星故障诊断的能力在未来卫星技术发展中变得突出重要,并不断向更高水平发展。
现代卫星系统的复杂化,使得基于模的故障诊断方法——建模难度大、灵活性差,导致基于模型的方法很难取得较好的故障诊断结果。
由于基于聚类分析的故障诊断方法利用历史数据进行建模,不需要实际的物理结构模型,它能够克服基于模型的诊断方法的缺点。
本论文采用基于聚类分析的故障诊断方法,开发和利用卫星监控系统所采集的历史数据。
论文的研究内容主要包括以下几个方面:首先,研究基于聚类分析的数据分布特征提取方法的特点,学习聚类分析的两种算法,利用聚类算法分析拟合数据与异常数据,设计拟合数据与异常数据的差值模型,初步建立基于聚类分析的故障诊断模型。
其次,对标准的飞机控制系统模型进行数值仿真,获取该模型的健康数据和故障数据,采用基于聚类分析的诊断方法进行故障诊断,依据诊断结果来完善该故障诊断模型的建模与诊断推理步骤。
再次,结合卫星姿态控制系统的结构,对主要部件的数学模型做了详细分析,并研究飞轮摩擦力矩对姿态角控制效果的影响。
建立卫星姿态控制系统的外在干扰力与力矩的数学模型,针对系统的执行部件与测量部件,设置了不同的故障模式并建立相应的数学模型。
最后,根据卫星姿态控制系统部件的几种典型的故障模式,建立相应的simulink仿真模型,设置卫星姿态控制系统仿真参数与设定该系统的状态观测点,对这几种仿真模型进行数值仿真模拟并采集系统的健康数据与故障数据。
利用聚类分析的方法对该姿态控制系统进行故障诊断。
【英文摘要】The Fault Diagnosis Technique (FDT) applied to satellite systems is a special technique adopted to ensure the proper functions of the systems. It can not only realize the intelligent repaire, but the diagnosis experiences can also optimize the observation points of the satellite systems. With the developing tendency of more complex and intelligent of satellite systems, FDT plays a more important role and is pushed to reach a higher of the complexity of the modern satellite systems, the model-based FDT, which has a complicated modeling process and poor flexibility, can not always obtain a good result of fault diagnosis; while the cluster-analysis-based on history process data FDT (CBHD-FDT), which makes use of historical data and does not need the physical structure of systems, can overcome those short comings. Therefore, the research in this paper is using the CBHD-FDT, and exploring and applying the historical data gathered by the satellite monitering system. The main content of this research is as follows:Firstly, the characteristics of the extraction method into the data distrubuted features of CBHD-FDT are taken into deep research. Then two kindscluster analysis algorithms are studied, using which the expected fitting data and the actual abnormal data are analyzed, a variance model is built, and the initial CBHD-FDT model is , the numerical simulation is performed on a standard aircraft control system, from which both the healthy and faulty data are obtained. Further, CBHD-FDT is applied to diagnose this system, based on the results of which, the modeling process and diagnosis reasoning steps can be ’s more, combined with the actural structure of the satellite attitude controling system, the mathematical models of the main components are analyzed in details, with the influence of the flywheel friction torque on the attitude angle controling effects. The analytical models of the external disturbance forces and torques loading on the satellite attitude controling system are established. In addition, in view of specific operating and measuring components, the corresponding mathematical models are set under different faulure , based on several typical failure modes of a satellite attitude controling system, after the parameters of the simulation models and the state-observation points of the system are set, the simulation model is to be built in SIMULINK. From the running of the simulation, both healthy and faulty data of the system can be gathered, and CBHD-FDT is applied to perform the fault diagnosis of this controling system.【关键词】故障诊断控制系统仿真历史数据聚类分析【英文关键词】fault diagnosis simulation of controling system historical data cluster analysis【目录】基于聚类分析的卫星姿态控制系统故障诊断方法研究摘要4-5 ABSTRACT 5 第1章绪论 8-20 课题背景和意义8-9 故障诊断技术国内外进展 9-17 基于定性模型的故障诊断方法 10-12 基于定量模型的故障诊断方法 12-13 基于历史数据的故障诊断方法 13-14 基于历史数据的定量模型故障诊断方法 14-17 发展趋势 17-18 本文工作总结 18-20 第2章基于聚类分析的故障诊断方法 20-33 引言 20 聚类算法的选取标准 20-22 聚类算法可行性 21 聚类质量评估21-22 聚类分析的数据结构和数据类型 22-24 聚类分析的数据结构 22-23 聚类分析的数据类型 23-24 聚类算法模型建立 24-26 基于划分的方法 24-25 基于密度的方法 25两种方法的比较 25-26 建立基于聚类分析的故障诊断模型 26-32 健康状态的聚类学习 28-30 系统异常状态监测 30-32 本章小结 32-33 第3章基于聚类分析的控制系统故障诊断 33-46引言 33 数据矢量的属性对异常检测结果的影响 33-40 输入为常值时 34-36 输入为正弦波时 36-38 输入为方波时38-40 健康数据的选择对故障检测结果的影响 40-43 输入为常值 40-41 输入为方波 41-42 输入为正弦波 42-43基于聚类算法的复合故障检测 43-44 本章小结 44-46 第4章卫星姿态控制系统仿真模型的建立 46-57 引言 46 数学模型 46-55 卫星姿态控制系统的数学模型 46-53 空间环境力与力矩 53-55 控制系统的故障建模 55-56 本章小结56-57 第5章卫星控制系统的故障诊断 57-65 引言 57 卫星姿态控制系统的数值模拟 57-64 系统仿真模型 57-58 系统仿真参数 58 系统健康状态学习 58-59 故障分类与建模 59-60 基于聚类算法的故障检测 60-62 测量部件的故障检测 62-64 本章小结 64-65 结论 65-66 参考文献66-70 致谢 70。
基于模型的航天器推进系统故障诊断
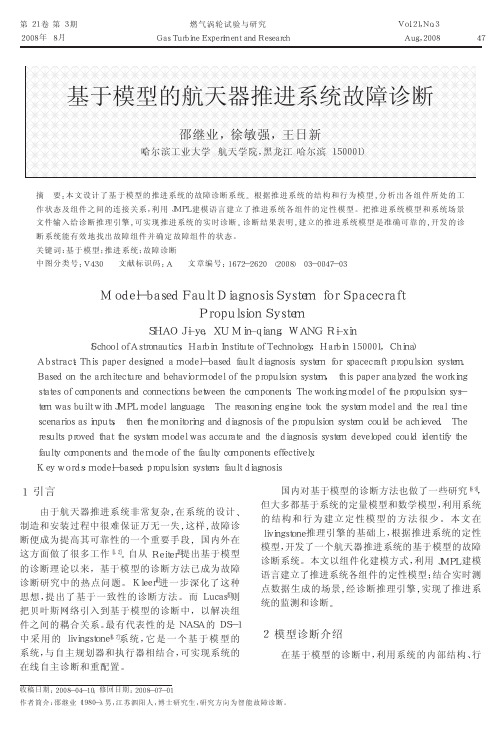
第21卷第3期燃气涡轮试验与研究Vol.21,No.32008年8月GasTurbineExperimentandResearchAug.,2008基于模型的航天器推进系统故障诊断邵继业,徐敏强,王日新(哈尔滨工业大学航天学院,黑龙江哈尔滨150001)摘要:本文设计了基于模型的推进系统的故障诊断系统。
根据推进系统的结构和行为模型,分析出各组件所处的工作状态及组件之间的连接关系,利用JMPL建模语言建立了推进系统各组件的定性模型。
把推进系统模型和系统场景文件输入给诊断推理引擎,可实现推进系统的实时诊断。
诊断结果表明,建立的推进系统模型是准确可靠的,开发的诊断系统能有效地找出故障组件并确定故障组件的状态。
关键词:基于模型;推进系统;故障诊断中图分类号:V430文献标识码:A文章编号:1672-2620(2008)03-0047-03Model-basedFaultDiagnosisSystemforSpacecraftPropulsionSystemSHAOJi-ye,XUMin-qiang,WANGRi-xin(SchoolofAstronautics,HarbinInstituteofTechnology,Harbin150001,China)Abstract:Thispaperdesignedamodel-basedfaultdiagnosissystemforspacecraftpropulsionsystem.Basedonthearchitectureandbehaviormodelofthepropulsionsystem,thispaperanalyzedtheworkingstatesofcomponentsandconnectionsbetweenthecomponents.Theworkingmodelofthepropulsionsys-temwasbuiltwithJMPLmodellanguage.Thereasoningenginetookthesystemmodelandtherealtimescenariosasinputs,thenthemonitoringanddiagnosisofthepropulsionsystemcouldbeachieved.Theresultsprovedthatthesystemmodelwasaccurateandthediagnosissystemdevelopedcouldidentifythefaultycomponentsandthemodeofthefaultycomponentseffectively.Keywords:model-based;propulsionsystem;faultdiagnosis1引言由于航天器推进系统非常复杂,在系统的设计、制造和安装过程中很难保证万无一失,这样,故障诊断便成为提高其可靠性的一个重要手段,国内外在这方面做了很多工作[1,2]。
应用自组织模糊神经网络估计卫星姿态系统执行机构故障
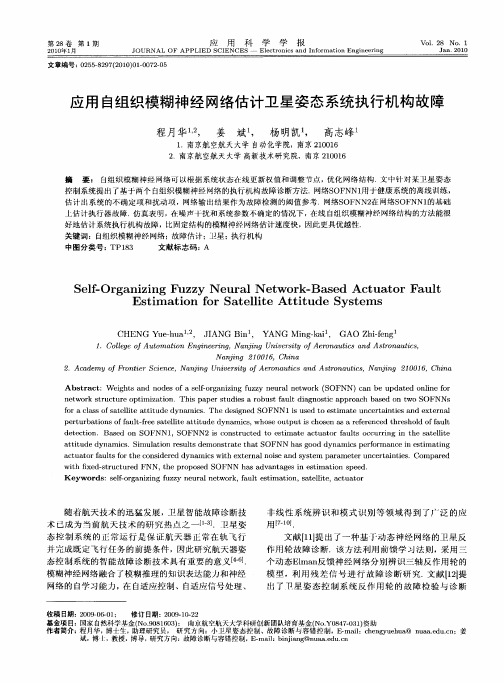
文章编号:0 5— 2 7 2 1 )10 7 —5 2 58 9 (0 00 —0 20
应 用 自组织模糊神经 网络估 计卫星姿 态系统执行机构 故障
程月华 , 姜 斌 杨 明凯 高志峰 , ,
1 .南京航 空航天 大学 自动化学 院,南京 2 0 1 106 2 .南京航 空航 天大学 高新技 术研 究院,南京 2 0 1 106
a tt ded n m is i l t o e u t e o s r t h tS t iu y a c .S mu a i n r s lsd m n t a et a OFNN a o d d n mi sp ro m a c n e tm a i h g o y a c e f r n e i s i tng s
Ab ta t sr c :Weg t n o e f e -ra i n u z e r l ew r S NN) al eu d tdo l efr ih s d n d so slog nz gfzyn u a t o k( oF a a f i n c ib p ae ni n o
好 地 估 计 系 统 执 行 机 构 故 障 ,比同 定 结 构 的模 糊神 经 网络 估 计速 度 快 ,因此 史 具 优 越 性 . 关 键 词 :自组 织 模 糊 神 经 网络 ; 障 估 计 ;_星 ; 行 机 构 故 『 J 执
《基于数据驱动的卫星姿控系统故障诊断研究》
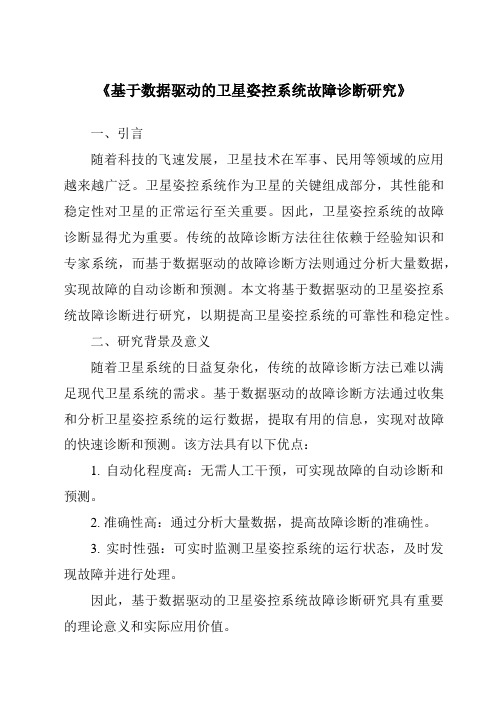
《基于数据驱动的卫星姿控系统故障诊断研究》一、引言随着科技的飞速发展,卫星技术在军事、民用等领域的应用越来越广泛。
卫星姿控系统作为卫星的关键组成部分,其性能和稳定性对卫星的正常运行至关重要。
因此,卫星姿控系统的故障诊断显得尤为重要。
传统的故障诊断方法往往依赖于经验知识和专家系统,而基于数据驱动的故障诊断方法则通过分析大量数据,实现故障的自动诊断和预测。
本文将基于数据驱动的卫星姿控系统故障诊断进行研究,以期提高卫星姿控系统的可靠性和稳定性。
二、研究背景及意义随着卫星系统的日益复杂化,传统的故障诊断方法已难以满足现代卫星系统的需求。
基于数据驱动的故障诊断方法通过收集和分析卫星姿控系统的运行数据,提取有用的信息,实现对故障的快速诊断和预测。
该方法具有以下优点:1. 自动化程度高:无需人工干预,可实现故障的自动诊断和预测。
2. 准确性高:通过分析大量数据,提高故障诊断的准确性。
3. 实时性强:可实时监测卫星姿控系统的运行状态,及时发现故障并进行处理。
因此,基于数据驱动的卫星姿控系统故障诊断研究具有重要的理论意义和实际应用价值。
三、研究内容与方法1. 数据收集与预处理首先,收集卫星姿控系统的运行数据,包括姿态、速度、加速度等参数。
然后,对数据进行清洗、去噪、归一化等预处理操作,为后续的分析提供高质量的数据。
2. 特征提取与选择通过分析预处理后的数据,提取与故障相关的特征,如峰值、谷值、均值等。
利用特征选择算法,选择对故障诊断有重要影响的特征,降低数据的冗余性。
3. 故障诊断模型构建采用机器学习、深度学习等算法,构建基于数据驱动的故障诊断模型。
通过对模型的训练和优化,提高模型的诊断准确性和泛化能力。
4. 实验与结果分析利用实际卫星姿控系统的运行数据对模型进行测试,分析模型的诊断准确率、误诊率等指标。
通过对比传统方法和基于数据驱动的方法,评估基于数据驱动的故障诊断方法的效果。
四、实验结果与分析1. 实验数据与设置本实验采用某型卫星姿控系统的实际运行数据。
基于切换模糊化的滑模变结构auv姿态控制
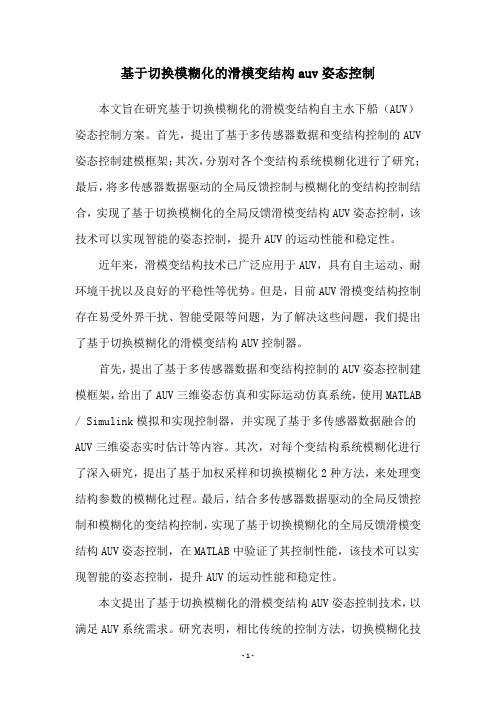
基于切换模糊化的滑模变结构auv姿态控制本文旨在研究基于切换模糊化的滑模变结构自主水下船(AUV)姿态控制方案。
首先,提出了基于多传感器数据和变结构控制的AUV 姿态控制建模框架;其次,分别对各个变结构系统模糊化进行了研究;最后,将多传感器数据驱动的全局反馈控制与模糊化的变结构控制结合,实现了基于切换模糊化的全局反馈滑模变结构AUV姿态控制,该技术可以实现智能的姿态控制,提升AUV的运动性能和稳定性。
近年来,滑模变结构技术已广泛应用于AUV,具有自主运动、耐环境干扰以及良好的平稳性等优势。
但是,目前AUV滑模变结构控制存在易受外界干扰、智能受限等问题,为了解决这些问题,我们提出了基于切换模糊化的滑模变结构AUV控制器。
首先,提出了基于多传感器数据和变结构控制的AUV姿态控制建模框架,给出了AUV三维姿态仿真和实际运动仿真系统,使用MATLAB / Simulink模拟和实现控制器,并实现了基于多传感器数据融合的AUV三维姿态实时估计等内容。
其次,对每个变结构系统模糊化进行了深入研究,提出了基于加权采样和切换模糊化2种方法,来处理变结构参数的模糊化过程。
最后,结合多传感器数据驱动的全局反馈控制和模糊化的变结构控制,实现了基于切换模糊化的全局反馈滑模变结构AUV姿态控制,在MATLAB中验证了其控制性能,该技术可以实现智能的姿态控制,提升AUV的运动性能和稳定性。
本文提出了基于切换模糊化的滑模变结构AUV姿态控制技术,以满足AUV系统需求。
研究表明,相比传统的控制方法,切换模糊化技术可以改善AUV系统的性能,实现智能化的姿态控制。
然而,本文仍有一些局限性,包括未考虑AUV变结构系统模糊化过程中的复杂物理约束,以及模糊化参数的精确选择问题,未来的工作将是解决这些问题。
本文探讨了基于切换模糊化的滑模变结构AUV姿态控制方案,提出了基于多传感器数据驱动和模糊化变结构控制的AUV控制器,并通过模拟验证,从而实现智能的姿态控制,提高AUV的系统稳定性和可控性。
电子操纵器自动化故障诊断方法——基于模糊聚类的类型自动化火箭发射器的故障诊断说明书
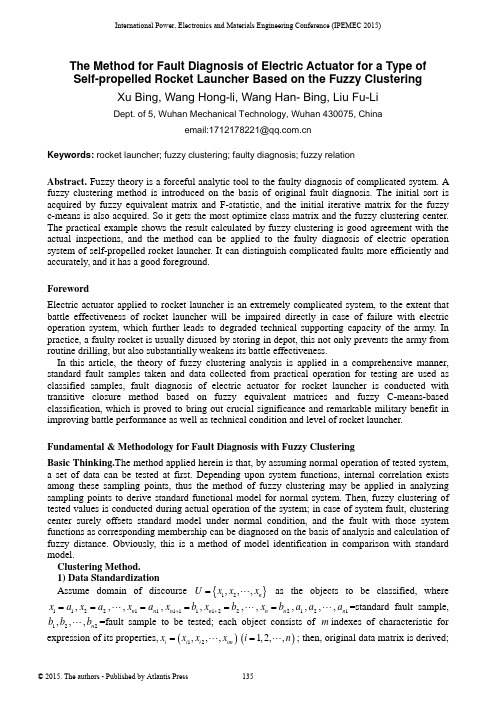
The Method for Fault Diagnosis of Electric Actuator for a Type ofSelf-propelled Rocket Launcher Based on the Fuzzy ClusteringXu Bing, Wang Hong-li, Wang Han- Bing, Liu Fu-LiDept. of 5, Wuhan Mechanical Technology, Wuhan 430075, Chinaemail:*****************.cnKeywords: rocket launcher; fuzzy clustering; faulty diagnosis; fuzzy relationAbstract. Fuzzy theory is a forceful analytic tool to the faulty diagnosis of complicated system. Afuzzy clustering method is introduced on the basis of original fault diagnosis. The initial sort isacquired by fuzzy equivalent matrix and F-statistic, and the initial iterative matrix for the fuzzyc-means is also acquired. So it gets the most optimize class matrix and the fuzzy clustering center.The practical example shows the result calculated by fuzzy clustering is good agreement with theactual inspections, and the method can be applied to the faulty diagnosis of electric operationsystem of self-propelled rocket launcher. It can distinguish complicated faults more efficiently andaccurately, and it has a good foreground.ForewordElectric actuator applied to rocket launcher is an extremely complicated system, to the extent thatbattle effectiveness of rocket launcher will be impaired directly in case of failure with electricoperation system, which further leads to degraded technical supporting capacity of the army. Inpractice, a faulty rocket is usually disused by storing in depot, this not only prevents the army fromroutine drilling, but also substantially weakens its battle effectiveness.In this article, the theory of fuzzy clustering analysis is applied in a comprehensive manner,standard fault samples taken and data collected from practical operation for testing are used asclassified samples, fault diagnosis of electric actuator for rocket launcher is conducted withtransitive closure method based on fuzzy equivalent matrices and fuzzy C-means-basedclassification, which is proved to bring out crucial significance and remarkable military benefit inimproving battle performance as well as technical condition and level of rocket launcher.Fundamental & Methodology for Fault Diagnosis with Fuzzy ClusteringBasic Thinking.The method applied herein is that, by assuming normal operation of tested system,a set of data can be tested at first. Depending upon system functions, internal correlation existsamong these sampling points, thus the method of fuzzy clustering may be applied in analyzingsampling points to derive standard functional model for normal system. Then, fuzzy clustering oftested values is conducted during actual operation of the system; in case of system fault, clusteringcenter surely offsets standard model under normal condition, and the fault with those systemfunctions as corresponding membership can be diagnosed on the basis of analysis and calculation offuzzy distance. Obviously, this is a method of model identification in comparison with standardmodel.Clustering Method.1) Data StandardizationAssume domain of discourse {}12,,,n U x x x = as the objects to be classified, where11x a =,22x a =, ,11n n x a =,111n x b +=,122n x b +=, ,2n n x b =,1a ,2a , ,1n a =standard fault sample,1b ,2b , ,2n b =fault sample to be tested; each object consists of m indexes of characteristic forexpression of its properties,()12,,,i i i im x x x x = ()1,2,,i n = ; then, original data matrix is derived;International Power, Electronics and Materials Engineering Conference (IPEMEC 2015)and then, standardization processing is performed with original data matrix derived. Usually, standard data is compressed to interval[0,1], with range transformation: {}{}{}'111min max min ik ik i n ik ik ik i ni n x x x x x ≤≤≤≤≤≤−=− (1) 2) Rating (Construct Fuzzy Similar Matrix)Construct fuzzy similar matrix ()ij R γ=,where ij γ=similar coefficient, to describe similaritybetween samples i and j . Angle cosine is applied:22111()/(()())m mm ij ik jk ik jk k k k x x x x γ====∑∑∑ (2)3) ClusteringClustering requires fuzzy relation R to be fuzzy equivalent relation, fuzzy equivalent relationmatrix R can be derived from square root calculation,i.e. Derivation in sequence 2R ,4R , , 2k R ,until 122k k R R −=, then 2k R R =. Determination of Best Threshold λ. Best value λ is determined by F - statistics; assume c as number of classification corresponding to value λ, number of samples class j is nj , and samplesclass j are expressed as ()()()12,,,j j j njx x x , clustering center of samples class j is vector ()()()()()12,,,j j j j mx x x x = , where ()j k x =mean value of number k characteristics, namely, ()()11nj j j k ik i x x nj ==∑(1,2,,)k m = . F -statistics: ()21()()211||||()||||()nj j j njr j j i i j j nj x x r c F nj x x n r ===−−=−−∑∑∑ (3) Where ()||||j x x −=()()21()m j j k k xx =−∑=distance between ()j xand x , ()()||||j j i i x x −=distance between ()j i x of samples class j and center ()j x .F -Statistics is subjected to F-distribution with DOF of c -1,n -; numerator means distancebetween classes, and denominator means distance of samples in the same class; therefore, bigger F value suggests bigger distance and bigger difference between classes for better classification.Fuzzy C-mean Clustering Algorithm.⑴ Class C of samples can be derived from value λ corresponding to F maximum, thenclassification is transformed to corresponding initial membership matrix (0)c A before iterative algorithm.⑵ Calculate clustering center ()q iV from 11()()n m i ik k ik k i V r x r αα===∑∑ ()q i V (q =iterative times). ⑶ Calculate *ij r based on ()q i V 1*2(1)1||||||||m ij j i j k k r x V x V α−−= =−−∑ to derive new classification matrix *(1)q c A +, where α is taken to 1<α<+∞.⑷ Randomly given a small positive number ε, and assume (1)||||q q A A ε+−<, iteration closes,otherwise, assume 1q q =+ and go back to ⑵ for further iteration, and finally best classification matrix A and clustering center V are derived.Analysis on the Conclusion of Fault Diagnosis.One best classification is derived after fuzzyclustering analysis. If a given sample to be tested belongs to the same class as standard sample of a fault, indicating occurrence of such fault; however, when samples to be tested belong to the sameclass as several classes of standard fault samples, indicating similarity of samples to be tested to several classes of standard fault samples, namely, possibility of concurrence of these faults. If samples to be tested do not belong to the same class as any class of standard fault, it indicates a new class of fault. By case analysis and diagnosis, standard fault samples can be improved further to increase confidence of fault diagnosis.Fault Diagnosis of Electric Actuator for Self-propelled Rocket LauncherFor various systems of rocket launcher, and in occurrence of one phenomenon, it’s likely to be a fault by many causes; likewise, multiple fault causes are likely to result in as much fault phenomena; for example, poor contact of moving contact for manual resistance controller is one cause of the fault that could lead to a number of faults with rocket, including unstable operation, abrupt shutdown, enlargement of motor output voltage surge, and etc; therefore, relation between fault and phenomenon is intricate. To determine main cause of certain phenomenon, the extent of relation between the cause of fault and phenomenon of fault should have to be identified at first, i.e. a weighing coefficient to be assessed based upon empirical and statistic results; working process of whole fuzzy diagnosis is shown in the chart below:Chart 1 Fuzzy Diagnosis ProcessTable 1 contains original data used for fault diagnosis based on fuzzy clustering.Table 1. Original Data Standard fault sample (SFS)Sample Characteristic Distribution Type of FaultStandard fault sample 1 Standard fault sample 2 Standard fault sample 3 Standard fault sample 4 Standard fault sample 5 Standard fault sample 6 Standard fault sample 7 Sample a to be tested Sample b to be tested Sample c to be tested 4 2 2 0 0 3 3 1 1 0 0 2 4 0 0 2 2 0 0 1 3 0 4 3 3 1 10 0 2 0 0 0 0 0 2 2 0 0 00 0 0 4 0 0 0 4 0 31 0 0 32 1 13 1 00 2 1 1 1 2 00 2 02 0 03 3 1 13 0 00 2 4 0 0 3 30 0 10 3 6 0 0 3 30 0 2 Controller faultControl panel faultPolarized relay failureIntermediary relayfailure Variable resistor short-circuit Angle retainer malfunction Enlarged motor fault Type of fault TBD Type of fault TBD Type of fault TBDCollection of data on removed faults by the army Conclusion & analysis of practice experience Determinat ion of weighing coefficient Determination of one fuzzy matrix Computer-based diagnosis Interactive man-mach ine dialogue Output of diagnosis resultA =R = R λ=0.9559=R =By standardization and calculation of similarity coefficient from range transformation and anglecosine of original data contained in Table 1, fuzzy matrix and fuzzy equivalent matrix are derived, based on which F-statistic value corresponding to best λ value is derived.Fuzzy matrix:1.0000 0.6719 0.5599 0.6396 0.0942 0.4139 0.5449 0.4461 0.7242 0.66300.6719 1.0000 0.5836 0.5252 0.0870 0.1457 0.5754 0.1293 0.9813 0.99820.5599 0.5836 1.0000 0.2020 0.4016 0.5603 0.4426 0.6466 0.5490 0.59340.6396 0.5252 0.2020 1.0000 0.0000 0.2774 0.3651 0.2462 0.6794 0.51830.0942 0.0870 0.4016 0.0000 1.0000 0.7351 0.1613 0.6525 0.0750 0.11450.4139 0.1457 0.5603 0.2774 0.7351 1.0000 0.4557 0.9559 0.1884 0.14380.5449 0.5754 0.4426 0.3651 0.1613 0.4557 1.0000 0.3596 0.5788 0.56780.4461 0.1293 0.6466 0.2462 0.6525 0.9559 0.3596 1.0000 0.1672 0.12760.7242 0.9813 0.5490 0.6794 0.0750 0.1884 0.5788 0.1672 1.0000 0.97810.6630 0.9982 0.5934 0.5183 0.1145 0.1438 0.5678 0.1276 0.9781 1.0000 Fuzzy equivalent matrix:1.0000 0.7242 0.5934 0.6794 0.5934 0.5934 0.5788 0.5934 0.7242 0.72420.7242 1.0000 0.5934 0.6794 0.5934 0.5934 0.5788 0.5934 0.9813 0.99820.5934 0.5934 1.0000 0.5934 0.6466 0.6466 0.5788 0.6466 0.5934 0.59340.6794 0.6794 0.5934 1.0000 0.5599 0.5603 0.5788 0.5934 0.6794 0.67940.5934 0.5934 0.6466 0.5599 1.0000 0.7351 0.5754 0.7351 0.5934 0.59340.5934 0.5934 0.6466 0.5603 0.7351 1.0000 0.5754 0.9559 0.5934 0.59340.5788 0.5788 0.5788 0.5788 0.5754 0.5754 1.0000 0.5788 0.5788 0.57880.5934 0.5934 0.6466 0.5934 0.7351 0.9559 0.5788 1.0000 0.5934 0.59340.7242 0.9813 0.5934 0.6794 0.5934 0.5934 0.5788 0.5934 1.0000 0.98130.7242 0.9982 0.5934 0.6794 0.5934 0.5934 0.5788 0.5934 0.9813 1.0000F statistic value and corresponding λ value:λ 0.7242 0.5934 0.6794 0.9813 0.9982 0.6466 0.7351 0.9559F 11.1157 1.4751 11.6086 32.2437 32.4937 10.9798 8.7882 34.5249Through analysis of fuzzy equivalent matrix and F statistic value, when λ=0.9559, bestclassification matrix is obtained, original data are classified into 7 types, namely, {1},{2,b,c},{3},{4},{5},{6,a},{7}; in this case, classification matrix is expressed as follows:1 0 0 0 0 0 0 0 0 00 1 0 0 0 0 0 0 1 10 0 1 0 0 0 0 0 0 00 0 0 1 0 0 0 0 0 00 0 0 0 1 0 0 0 0 00 0 0 0 0 1 0 1 0 00 0 0 0 0 0 1 0 0 00 0 0 0 0 1 0 1 0 00 1 0 0 0 0 0 0 1 10 1 0 0 0 0 0 0 1 1 By further calculation of above classification results, final classification matrix and finalclustering center are derived, as follows: Final classification matrix: 0.0841 0.1089 0.0060 0.0072 0.0090 0.1068 0.0891 0.0638 0.1048 0.11690.3832 0.2871 0.5928 0.2950 0.3694 0.3786 0.3861 0.2908 0.3905 0.0130 0.1028 0.1089 0.0659 0.2086 0.0991 0.1068 0.1089 0.2057 0.1048 0.2468 0.0374 0.0396 0.0240 0.0288 0.0360 0.1553 0.1584 0.0284 0.0381 0.0519 0.2243 0.0396 0.0240 0.1151 0.1441 0.1359 0.1386 0.0426 0.0571 0.2078 0.0467 0.2475 0.1497 0.2518 0.2252 0.0485 0.0495 0.2482 0.1429 0.32470.1215 0.1683 0.1377 0.0935 0.1171 0.0680 0.0693 0.1206 0.1619 0.0390In final clustering:V =0.0001 0.0000 0.9993 0.0342 0.0000 0.0000 0.12830.0000 0.0000 0.0001 0.0096 0.0001 1.0000 0.03000.0001 0.0000 0.0002 0.0233 0.9998 0.0000 0.07800.0001 0.0000 0.0002 0.8928 0.0000 0.0000 0.6539 0.9996 0.0000 0.0002 0.0371 0.0001 0.0000 0.1028 0.0000 1.0000 0.0000 0.0030 0.0000 0.0000 0.00700.0001 0.0000 0.0000 0.0000 0.0000 0.0000 0.0000 Diagnosis outcome based on above analysis is that, data a to be tested indicates angle retainermalfunction, while data b and c to be tested indicate control panel fault, base on which engineeringtechnician can work out method and steps for removal of such faults, so as to eliminate any faultsdiscovered in a timely manner. It’s obvious that this fuzzy clustering is easy for realization ofreal-time and intelligent fault diagnosis. Case examination suggests consistent theoreticalcalculation and on-site inspection. This is the evidence that fuzzy clustering is potential for practicalapplication by providing a desirable approach in fault diagnosis of complex system.ConclusionIn this discourse, a new method for fault diagnosis of electric actuator for self-propelled rocketlauncher is expounded, which, based on fuzzy clustering, helps realizing application of uncertain orinsufficient knowledge of human in subjective and practical experiences, so as to succeed in themost accurate classification determination for types of fault thereon. During rocket launcher drillingof the army, type of fault can be determined accurately and quickly with this method in case of fault,and the operator can remove such fault quickly by adopting appropriate method in terms of type offault, which greatly improves technical supporting capacity of rocket launcher and generatesremarkable economic and military benefits.References[1] Brandt M,Kharas Y .An error convergence simulation study of hardvs.fuzzy C-means clustering. In:IEEEInter-NationalConference on Fuzzy System.Orlando:The IEEE Neural NetworksCouncil and the IEEE Orlando Section,1994.1835-1839[2] Sam J,Chan Laiwan.Alternative membership function for sequential fuzzy clustering.In:IEEEInternational Con-Ferenc on Fuzzy System.Orlando:The IEEE Neural Networks Council and theIEEE Orlando Section,1994.1846-1851[3] Wang Shuhai et al, Mechanism of Rocket Launcher [M]; Beijing: Chinese People's LiberationArmy Publishing House, 2000[4] Xu Zhangsui, Fang Liqing, Wang Xiwu et al, Fundamental & Application of Fault InformationDiagnosis, [M]; Beijing: National defense Industry Press, 2000[5] Li Hongxing, Wang Qun, Duan Qinzhi et al, Methodology & Application of Engineering FuzzyMathematics [M]; Tianjin: Tianjin Science and Technology Press, 1993[6] Wu Jinpei, Fuzzy Diagnosis Theory and Application [M]; Beijing: Science Press, 1995[7] Gao Xinbo, Fuzzy Clustering Analysis and Application [M]; Xi’an: Xi'an University ofElectronic Science and Technology Press, 2004。
卫星姿态控制系统执行器的故障诊断方法研究
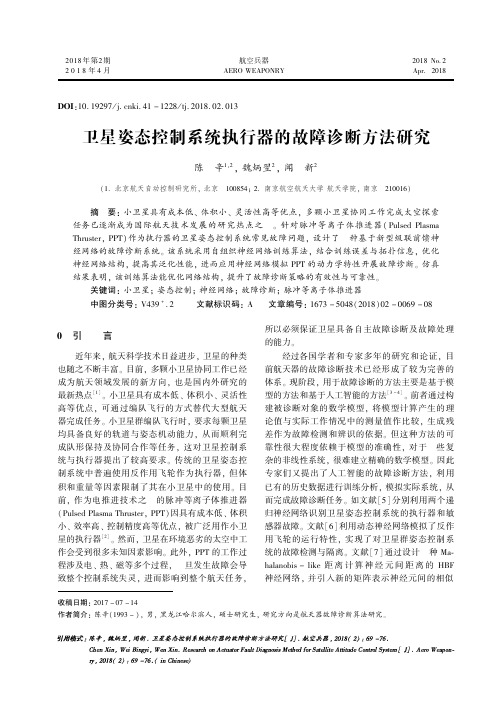
收稿日期院 圆园员苑 原 园苑 原 员源 作者简介院 陈辛渊员怨怨猿 原 冤 袁 男袁 黑龙江哈尔滨人袁 硕士研究生袁 研究方向是航天器故障诊断算法研究遥
窑苑园窑
航空兵器摇 圆园员愿 年第 圆 期
度袁 用于航天器执行机构的故障重构遥 而神经网络 的 跟 踪 拟 合 能 力 很 大 程 度 上 取 决
员摇 卫星姿态系统
员援 员摇 卫星姿态运动方程 卫星的姿态 运 动 方 程 可 以 描 述 其 绕 自 身 质 心
的运动状态袁 通常由两部分组成院 一部分是通过坐
标变换关系得到的运动学方程袁 另一部分是通过
牛顿运动定律得出的动力学方程咱员源暂 遥 首先通过四
元数的表示方法给出卫星的运动学方程遥 四元数
法主要依据欧拉旋转定理袁 即两个坐标系间的相
对方向可以通过一个轴的旋转来表示袁 同时也可避
免欧拉角表示方法中出现的奇异点问题遥 四元数通
关键词院 小卫星曰 姿态控制曰 神经网络曰 故障诊断曰 脉冲等离子体推进器 中图分类号院 灾源猿怨 垣 援 圆摇 摇 文献标识码院 粤 摇 文章编号院 员远苑猿 原 缘园源愿渊圆园员愿冤园圆 原 园园远怨 原 园愿
园摇 引摇 摇 言
近年来袁 航天科学技术日益进步袁 卫星的种类 也随之不断丰富遥 目前袁 多颗小卫星协同工作已经 成为航天领域发展的新方向袁 也是国内外研究的 最新热点咱员暂 遥 小卫星具有成本低尧 体积小尧 灵活性 高等优点袁 可通过编队飞行的方式替代大型航天 器完成任务遥 小卫星群编队飞行时袁 要求每颗卫星 均具备良好的轨道与姿态机动能力袁 从而顺利完 成队形保持及协同合作等任务袁 这对卫星控制系 统与执行器提出了较高要求遥 传统的卫星姿态控 制系统中普遍使用反作用飞轮作为执行器袁 但体 积和重量等因素限制了其在小卫星中的使用遥 目 前袁 作为电推进技术之一的脉冲等离子体推进器 渊孕怎造泽藻凿 孕造葬泽皂葬 栽澡则怎泽贼藻则袁 孕孕栽冤 因具有成本低尧 体积 小尧 效率高尧 控制精度高等优点袁 被广泛用作小卫 星的执行器咱圆暂 遥 然而袁 卫星在环境恶劣的太空中工 作会受到很多未知因素影响遥 此外袁 孕孕栽 的工作过 程涉及电尧 热尧 磁等多个过程袁 一旦发生故障会导 致整个控制系统失灵袁 进而影响到整个航天任务袁
基于神经网络的卫星姿控系统故障预测(英文)
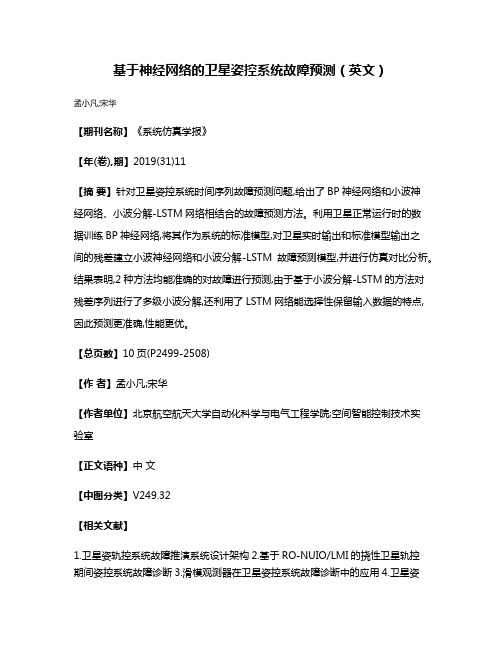
基于神经网络的卫星姿控系统故障预测(英文)
孟小凡;宋华
【期刊名称】《系统仿真学报》
【年(卷),期】2019(31)11
【摘要】针对卫星姿控系统时间序列故障预测问题,给出了BP神经网络和小波神
经网络、小波分解-LSTM网络相结合的故障预测方法。
利用卫星正常运行时的数
据训练BP神经网络,将其作为系统的标准模型,对卫星实时输出和标准模型输出之
间的残差建立小波神经网络和小波分解-LSTM故障预测模型,并进行仿真对比分析。
结果表明,2种方法均能准确的对故障进行预测,由于基于小波分解-LSTM的方法对残差序列进行了多级小波分解,还利用了LSTM网络能选择性保留输入数据的特点,因此预测更准确,性能更优。
【总页数】10页(P2499-2508)
【作者】孟小凡;宋华
【作者单位】北京航空航天大学自动化科学与电气工程学院;空间智能控制技术实
验室
【正文语种】中文
【中图分类】V249.32
【相关文献】
1.卫星姿轨控系统故障推演系统设计架构
2.基于RO-NUIO/LMI的挠性卫星轨控
期间姿控系统故障诊断3.滑模观测器在卫星姿控系统故障诊断中的应用4.卫星姿
控系统基于模糊基函数网络与自回归模型的故障预测5.基于嵌入式仿真的卫星姿控系统故障模拟平台
因版权原因,仅展示原文概要,查看原文内容请购买。
- 1、下载文档前请自行甄别文档内容的完整性,平台不提供额外的编辑、内容补充、找答案等附加服务。
- 2、"仅部分预览"的文档,不可在线预览部分如存在完整性等问题,可反馈申请退款(可完整预览的文档不适用该条件!)。
- 3、如文档侵犯您的权益,请联系客服反馈,我们会尽快为您处理(人工客服工作时间:9:00-18:30)。
姜
斌
1
( 1 南京航空航天大学自动化学院, 南京 210016 ) ( 南京航空航天大学高新技术研究院, 南京 210016 )
S 模糊方法和滑模观测器的 摘要: 针对卫星姿态控制系统的执行机构故障 , 提出了一种基于 TS 模糊模型的卫星姿态控制系统模型 , 故障诊断方法. 首先, 建立了基于 T并在此基础上, 设计了 一种用于状态检测的模糊滑模观测器 . 该观测器在执行机构发生故障的情况下 , 仍然保持滑模模 态, 可以利用等效输出注入实现故障的直接估计 . 在故障和干扰上界未知的情况下, 采用迭代学 习算法在线计算并调节滑模项的切换增益大小 , 避免了不必要的高频抖振现象; 同时利用 H ∞ 思 通过求解 LM I 获得观测器参数, 将干扰对故障估计的影响最小化. 计算机仿真结果表明, 该 想, , . 故障诊断方法能够对执行机构故障进行较为精确的估计 从而验证了方法的有效性 S 模糊模型; 滑模观测器 关键词: 卫星; 姿态控制; 故障诊断; T023806 中图分类号: TB277 文献标识码: A 文章编号: 1001 - 0505 ( 2010 ) 增刊( I) -
THEN
x ( t) = A i x( t) + Bu( t) y( t) = Cx( t)
i = 1, 2, …, r
}
( 4)
i i z1 , z2 , …, z s 为先验变量; M 1 , M2 , …, M is 为隶属度函数; r 为模糊规则数; s 为先验变量数; A i 通过对 式中, 式( 3 ) 在工作点线性化得到. 考虑执行机构故障, 整个系统的状态方程可表示为 r
x =
h i ( z ) ( A i x + Bu + Df a ∑ i =1
+ Ed)
y = Cx
}
( 5)
240
东南大学学报( 自然科学版)
r r
第 40 卷 w i( z) ∑ i =1 ≥ 0, 且∑ hi ( z ) = 1, 其中
i =1
Hale Waihona Puke f a 为执行机构故障; d 为外部干扰; D = B ; h i ( z ) = w i ( z ) 式中,
1
1. 1
系统描述
卫星姿态的动力学和运动学方程 三轴配置反作用飞轮的刚体卫星姿态动力学方程可表示为 Jω + ω * ( Jω + h ω ) = L c + L d
T
( 1)
J 为卫星的惯量矩阵; ω = { ω x , 式中, ωy , ω z } 为星体相对与惯性空间的角速度在本体坐标系中的投影矢 量; h ω 为飞轮角动量; L c 为飞轮控制力矩; L d 为各种干扰力矩总和. 0 定义[ ω ]= ω z - ωy
T 令 ω r = { ω1 , ω2 , ω3 } 为卫星姿态相对轨道坐标系的转动角速度在星体坐标系下的投影 . ω 与 ω r 的关 b b 系为 ω r = ω - C o ω o , 其中 C o 为轨道坐标系到星体坐标系的转换矩阵 , ω o 为轨道角速度. T T 定义状态变量 x = { , ωx , θ, ωy , ψ, ωz } , 输出 y = { ω x , θ, ωy , ω z } . 根据式( 1 ) 和 ( 2 ) , 可定义如下的系 统方程:
增刊( I)
樊雯, 等: 基于模糊滑模的卫星姿态控制系统故障诊断
239
Takagi 等[1]提出的 TS 模糊建模方法, 利用多个局部线性模型模糊逼近非线性系统模型 , 既考虑了 , . 系统本身的非线性特征 又降低了观测器设计问题的困难性与复杂性 该方法已被用于解决许多非线性系 统故障观测器的设计问题
作者简介: 樊雯( 1989 —) ,女, 硕士生; 姜斌( 联系人) ,男,博士,教授,博士生导师,binjiang@ nuaa. edu. cn. 基金项目: 江苏省自然科学基金重点资助项目( BK2010072 ) 、 南京航空航天大学研究生创新实验室基金资助项目 、 南京航空航天大学大 学生创新基金资助项目 . 0510. 收稿日期: 2010-
[2 ]
.
滑模观测器因其对系统中的干扰 、 未建模动态等不确定因素具有鲁棒性而被越来越多地应用于故障 [3 ~ 6 ] [3 ] . Edw ards 等 提出了一种基于滑模观测器的故障诊断策略 : 在故障发生时, 诊断中 观测器仍然保持 滑动模态, 故障信号可通过构造等效输出注入项得到重构 . 这一方法避免了产生评价残差的复杂性. 使用 这种策略时, 需要已知故障与外部干扰的上界 . 在实际应用中, 过大的切换增益会引起不必要的高频抖振 .
s
w i( z) =
M ij ( z j ) . ∏ j =1
2
2. 1
模糊滑模观测器设计
观测器设计
B ∈R n × q ; C ∈R p × n ; E ∈R n × k ; n > p > q. 考虑式( 5 ) 所示的不确定动态系统, 其中, C ) 可观测, 2, …, r ; ② 存在已知函数 α( t, u) 和已知常数 β, 对于此系统, 假设满足: ① ( A i , 且 i = 1, 使得 t) ‖≤α( t, u) , D, C ) 的任意不变零点都位于左半平面. ‖f a ( x, ‖d‖≤β; ③ rank( CD ) = rank( D ) , 且( A i , PDC , : 根据 原则 提出如下形式的滑模观测器
Fault diagnosis in attitude control system of satellite based on fuzzy sliding mode
Fan Wen1
(
1
2 Cheng Yuehua1,
Jiang Bin1
College of Automation Engineering ,Nanjing University of Aeronautics and Astronautics,Nanjing 210016 ,China) (
x = f( x, t) + Bu y = Cx u 为控制力矩. 式中, 1. 2 TS 模糊模型
}
( 3)
TS 模糊模型通过一系列 IFTHEN 规则下的多个局部线性模型模糊逼近非线性系统 , 本文给出的 TS 模糊模型描述如下:
i IF z 1 ( t) is M 1 ( t) , …, z s ( t) is M is ( t)
i IF z 1 ( t) is M 1 ( t) , …, z s ( t) is M is ( t) · r
THEN + G ni v i )
x ^ =
hi ( z ) ( A i x ^ + Bu + G li ( y - y ^) ∑ i =1
r
y =
hi ( z ) C x ^ ∑ i =1
2
Academy of Frontier Science,Nanjing University of Aeronautics and Astronautics,Nanjing 210016 ,China)
Abstract : A fault diagnosis approach based on the TakagiSugeno ( TS ) fuzzy and the sliding mode observer is proposed for actuator faults in the attitude control systems of a satellite. First,an attitude control system model of the satellite is constructed based on the TS fuzzy and a sliding mode observer is proposed for state detection. The observer can maintain a sliding motion even in the presence of actuator faults; thus,the estimation of the faults can be directly achieved by equivalent output injection. When the upper bounds of the faults and disturbances are unknow n,an iterative learning algorithm is used to calculate and update the sw itching gain of the sliding mode term ,avoiding unnecessary chattering. And by using the concepts of H ∞ ,a linear matrix inequality ( LM I) is calculated to minimize the effects of disturbances on the estimation of the faults. The results of the numerical simulation show that the accurate estimation of the actuator faults can be obtained,w hich proves the effectiveness of this approach. Key words: satellite; attitude control; fault diagnosis; TakagiSugeno ( TS ) fuzzy model; sliding mode observer 近年来, 随着空间技术的发展, 对卫星姿态控制系统的可靠性和自主运行能力有了更高的要求 . 卫星 姿态控制系统的故障诊断技术研究 , 对切实保障其可靠性与安全性具有重要意义 . 作为故障诊断方法的一 种, 基于模型的故障诊断方法应用较多 , 但需要以精确的数学模型为基础 , 构造观测器产生残差, 继而进行 故障的评价与决策. 大多基于模型的故障诊断方法都是针对线性系统的 , 对于非线性系统, 缺乏统一可行 的方法.