伍德里奇计量经济学讲义-文档资料
伍德里奇 计量经济学导论
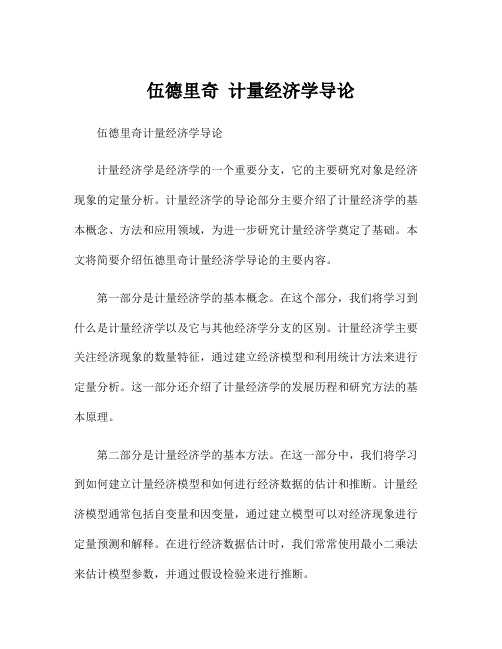
伍德里奇计量经济学导论伍德里奇计量经济学导论计量经济学是经济学的一个重要分支,它的主要研究对象是经济现象的定量分析。
计量经济学的导论部分主要介绍了计量经济学的基本概念、方法和应用领域,为进一步研究计量经济学奠定了基础。
本文将简要介绍伍德里奇计量经济学导论的主要内容。
第一部分是计量经济学的基本概念。
在这个部分,我们将学习到什么是计量经济学以及它与其他经济学分支的区别。
计量经济学主要关注经济现象的数量特征,通过建立经济模型和利用统计方法来进行定量分析。
这一部分还介绍了计量经济学的发展历程和研究方法的基本原理。
第二部分是计量经济学的基本方法。
在这一部分中,我们将学习到如何建立计量经济模型和如何进行经济数据的估计和推断。
计量经济模型通常包括自变量和因变量,通过建立模型可以对经济现象进行定量预测和解释。
在进行经济数据估计时,我们常常使用最小二乘法来估计模型参数,并通过假设检验来进行推断。
第三部分是计量经济学的应用领域。
计量经济学的应用非常广泛,包括宏观经济学、微观经济学、劳动经济学、财政经济学等诸多领域。
我们将学习到如何应用计量经济学的方法和模型来进行实证研究,比如估计宏观经济模型的参数、评估政策效果等。
在学习计量经济学导论的过程中,我们也需要掌握一些数学和统计学知识。
计量经济学主要运用了数理统计学、矩阵代数和微积分等数学工具,并使用了一些基本的统计学方法,比如假设检验、置信区间等。
因此,我们需要具备一定的数学和统计学基础,才能更好地理解和应用计量经济学。
伍德里奇计量经济学导论的教材内容很全面,结构条理清晰,同时也提供了大量的实证案例和应用案例,使我们能更好地理解和应用计量经济学的理论和方法。
通过学习这门课程,我们可以更好地理解和分析经济现象,并为实际问题的研究提供科学的分析工具。
总之,伍德里奇计量经济学导论是一门非常重要的课程,它为我们进一步研究和应用计量经济学提供了扎实的基础。
通过学习这门课程,我们可以更好地理解和分析经济现象,并运用计量经济学的方法进行实证研究。
计量经济学 伍德里奇 第一章

The main challenge of an impact evaluation is the construction of a suitable counterfactual situation.
An ideal experiment can be conducted to obtain the causal effect of fertilizer amount on yield when the levels of fertilizer are assigned to plots independently of other plot features that affect yield.
.12
.1
.08
unemployment rate
.06
.04
1976 1980
1985
1990
1995
2000
2005
Note: Shaded areas are times of recession following the definition of Elsby et al. (2009).
2010
Dandan Zhang (NSD)
Sep.-Dec. 2014 1 / 37
1. Introduction
Course Structure
1. Introduction (We4 Chapter 1) 2. Mathematical Foundations,Probability Theory (We4 Appendix B & C) 3. The Bivariate Linear Regression Model (We4 Chapter 2) 4. The Multivariate Linear Regression Model (We4 Chapter 3) 5. Inference (We4 Chapter 4) 6. Further Issues (We4 Chapter 6) 7. Multiple Regression Analysis with Qualitative Information (We4 Chapter 7) 8. Heteroscedasticity (We4 Chapter 8) 9. Specification and Data Issues (We4 Chapter 9) 10. Instrument variables (We4 Chapter 15) 11. Panel Data (We4 Chapter 14)
伍德里奇计量经济学绪论共54页文档
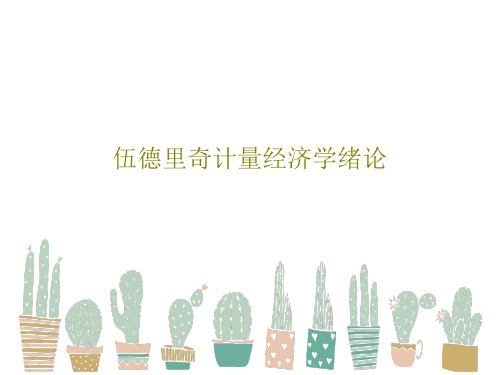
46、法律有权打破平静。——马·格林 47、在一千磅法律里,没有一盎司仁 爱。— —英国
48、法律一多,公正就少。——托·富 勒 49、犯罪总是以惩罚相补偿;只有处 罚才能 使犯罪 得到偿 还。— —达雷 尔
50、弱者就是财富 ❖ 丰富你的人生
71、既然我已经踏上这条道路,那么,任何东西都不应妨碍我沿着这条路走下去。——康德 72、家庭成为快乐的种子在外也不致成为障碍物但在旅行之际却是夜间的伴侣。——西塞罗 73、坚持意志伟大的事业需要始终不渝的精神。——伏尔泰 74、路漫漫其修道远,吾将上下而求索。——屈原 75、内外相应,言行相称。——韩非
伍德里奇计量经济学课件 (1)
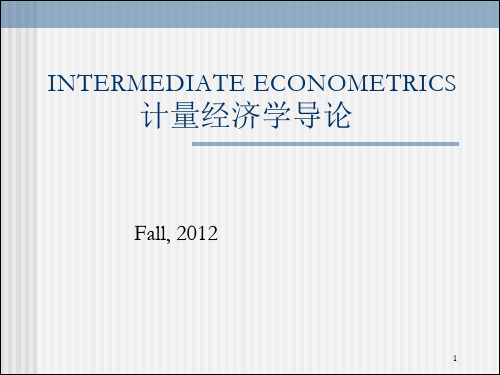
18
计量经济学
n
若贝尔经济学奖获奖名单
2004 Finn Kydland , Edward Prescott 2003 Robert F. Engle, Clive W. J. Granger 2002 Daniel Kahneman, Vernon L. Smith 2001 George A. Akerlof, A. Michael Spence, Joseph E. Stiglitz 2000 James J Heckman, Daniel L McFadden 1999 Robert A. Mundell 1998 Amartya Sen 1997 Robert C. Merton, Myron S. Scholes 1996 James A. Mirrlees, William Vickrey
INTERMEDIATE ECONOMETRICS
计量经济学导论
Fall, 2012
1
Outline
有关信息 n 什么是计量经济学 n 计量经济学的作用 n 数据: 输入数据 n 经验分析的步骤 n 本课程涵盖的内容
n
2
信息:课程——计量经济学
金融计量学 课号:01663 学分:4 课程性质:教育部规定核心课程
△诺贝尔经济学奖与计量经济学
77位获奖者中10位直接因为对计量经济学发展的贡献而获奖 1969 R. Frish J. Tinbergen 1973 W. Leotief 1980 L. R. Klein 1984 R. Stone 1989 T. Haavelmo 2000 J. J. Heckman D. L. McFadden 2003 R. F. Engle C. W. J. Granger
伍德里奇计量经济学导论
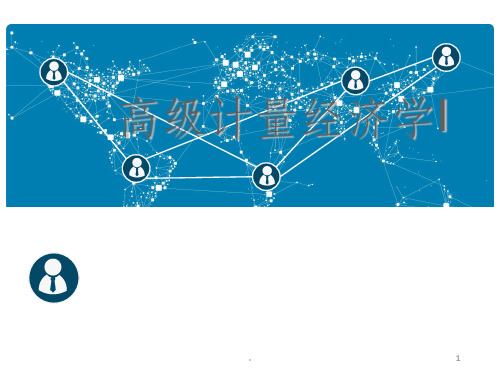
(3)因此,给定收入X的值Xi,可得消费支出Y的条件均值(conditional mean)或条件期望(conditional expectation): E(Y|X=Xi)
(4)该例中: E(Y | X=80)=65
.
描出散点图发现:随着收入的增加,消费“平均地说”也在增加,且Y的 条件均值均落在一根正斜率的直线上。这条直线称为总体回归线。
. E(y|x) = 0 + 1x
x1=5
x2 =10
.
34
对于所研究的经济问题,通常总体回归直线 E(Yi|Xi) = 0 + 1Xi 是观测不到
的。可以通过收集样本来对总体(真实的)回归直线做出估计。
样本回归模型: Yˆi ˆ0ˆ1Xi
或: Yi ˆ0ˆ1Xiei
② y = 0 + 1 x + u
u 为误差项或扰动项,它代表了除了x之外可以影响y的因素。
l 线性回归的含义: y 和x 之间并不一定存在线性关系,但是,只 要通过转换可以使y的转换形式和x的转换形式存在相对于参数的 线性关系,该模型即称为线性模型。
.
19 19
Ø 总体回归函数的随机设定
l 对于某一个家庭,如何描述可支配收入和消费支出的关系?
l 等式右边的变量被称为解释变量(Explanaiory Variable)或自 变量(Independent Variable)、右边变量、回归元,协变量,或控制变量。
l 等式y = b0 + b1x + u只有一个非常数回归元。我们称之为简单回归模型, 两
变量回归模型或双变量回归模型.
.
Ø 回归分析的目的
a. 函数形式:可以是线性或非线性的。
伍德里奇计量经济学讲义7
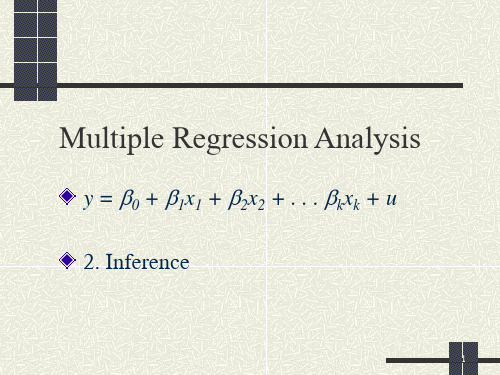
~ Normal
0,1
because it
ˆ b j is distribute d normally is a linear combinatio
n of the errors
5
The t Test
Under the CLM assumption s ˆ se b j
bˆ
j
b
j
ˆ c se b , where c is the 1 - a percentile ˆ bj j 2
in a t n k 1 distributi on
16
Computing p-values for t tests
An alternative to the classical approach is to ask, “what is the smallest significance level at which the null would be rejected?” So, compute the t statistic, and then look up what percentile it is in the appropriate t distribution – this is the p-value p-value is the probability we would observe the t statistic we did, if the null were true
3
The homoskedastic normal distribution with a single explanatory variable
y
f(y|x)
伍德里奇 计量经济学导论

伍德里奇计量经济学导论摘要:I.计量经济学的性质与经济数据A.计量经济学的定义B.经济数据的特点和来源II.简单回归模型A.回归模型的基本概念B.线性回归模型的建立与估计C.线性回归模型的检验III.多元回归分析A.多元回归模型的基本概念B.多元回归模型的建立与估计C.多元回归模型的检验IV.回归模型的应用与拓展A.回归模型在经济学研究中的应用B.回归模型的拓展与修正正文:伍德里奇在《计量经济学导论》一书中,对计量经济学的基本概念、方法和应用进行了系统性的介绍。
首先,他明确了计量经济学的定义,即在一定的经济理论基础之上,采用数学与统计学的工具,通过建立计量经济模型对经济变量之间的关系进行定量分析的学科。
为了更好地进行计量分析,书中详细阐述了经济数据的特点和来源,以及如何有效地利用这些数据。
在简单回归模型部分,伍德里奇介绍了回归模型的基本概念,以及如何建立和估计线性回归模型。
他详细地说明了最小二乘法(Least Squares Method)在回归模型估计中的运用,并通过实例展示了线性回归模型的检验方法。
在多元回归分析部分,伍德里奇进一步阐述了多元回归模型的基本概念,以及如何建立和估计多元回归模型。
他详细地介绍了矩阵代数在多元回归模型估计中的应用,并通过实例展示了多元回归模型的检验方法。
此外,他还介绍了如何通过回归模型对经济变量之间的关系进行解释和预测。
在回归模型的应用与拓展部分,伍德里奇通过实例展示了回归模型在经济学研究中的具体应用,包括对产出、消费、投资等经济变量的分析。
他还介绍了如何对回归模型进行拓展和修正,以更好地反映现实经济中的复杂关系。
伍德里奇:计量经济学导论chapter12

CHAPTER 12SOLUTIONS TO PROBLEMS12.1 We can reason this from equation (12.4) because the usual OLS standard error is anestimate of /σthe AR(1) parameter, , tends to be positive in time series regression models. Further, the independent variables tend to be positive correlated, so (x t - x )(x t +j - x ) – which is what generally appears in (12.4) when the {x t } do not have zero sample average – tends to be positive for most t and j . With multiple explanatory variables the formulas are more complicated but have similar features.If ρ < 0, or if the {x t } is negatively autocorrelated, the second term in the last line of (12.4)could be negative, in which case the true standard deviation of 1ˆβ is actually less than /σ12.3 (i) Because U.S. presidential elections occur only every four years, it seems reasonable to think the unobserved shocks – that is, elements in u t – in one election have pretty much dissipated four years later. This would imply that {u t } is roughly serially uncorrelated.(ii) The t statistic for H 0: ρ = 0 is -.068/.240 ≈ -.28, which is very small. Further, theestimate ˆρ= -.068 is small in a practical sense, too. There is no reason to worry about serial correlation in this example.(iii) Because the test based on ˆt ρ is only justified asymptotically, we would generally beconcerned about using the usual critical values with n = 20 in the original regression. But any kind of adjustment, either to obtain valid standard errors for OLS as in Section 12.5 or a feasible GLS procedure as in Section 12.3, relies on large sample sizes, too. (Remember, FGLS is not even unbiased, whereas OLS is under TS.1 through TS.3.) Most importantly, the estimate of ρ ispractically small, too. With ˆρso close to zero, FGLS or adjusting the standard errors would yield similar results to OLS with the usual standard errors.12.5 (i) There is substantial serial correlation in the errors of the equation, and the OLS standard errors almost certainly underestimate the true standard deviation in ˆE Z β. This makes the usualconfidence interval for βEZ and t statistics invalid.(ii) We can use the method in Section 12.5 to obtain an approximately valid standard error.[See equation (12.43).] While we might use g = 2 in equation (12.42), with monthly data we might want to try a somewhat longer lag, maybe even up to g = 12.SOLUTIONS TO COMPUTER EXERCISESC12.1 Regressingˆu on 1ˆt u-, using the 69 available observations, givesˆρ≈ .292 andtse(ˆρ) ≈ .118. The t statistic is about 2.47, and so there is significant evidence of positive AR(1) serial correlation in the errors (even though the variables have been differenced). This means we should view the standard errors reported in equation (11.27) with some suspicion.C12.3 (i) The test for AR(1) serial correlation gives (with 35 observations) ˆρ≈–.110,se(ˆρ)≈ .175. The t statistic is well below one in absolute value, so there is no evidence of serial correlation in the accelerator model. If we view the test of serial correlation as a test of dynamic misspecification, it reveals no dynamic misspecification in the accelerator model.(ii) It is worth emphasizing that, if there is little evidence of AR(1) serial correlation, there is no need to use feasible GLS (Cochrane-Orcutt or Prais-Winsten).C12.5 (i) Using the data only through 1992 givesdem w ins= .441 -.473 partyWH+ .479 incum+ .059 partyWH⋅gnews(.107) (.354) (.205) (.036)- .024 partyWH⋅inf(.028)n = 20, R2 = .437, 2R = .287.The largest t statistic is on incum, which is estimated to have a large effect on the probability of winning. But we must be careful here. incum is equal to 1 if a Democratic incumbent is running and –1 if a Republican incumbent is running. Similarly, partyWH is equal to 1 if a Democrat is currently in the White House and –1 if a Republican is currently in the White House. So, for an incumbent Democrat running, we must add the coefficients on partyWH and incum together, and this nets out to about zero.The economic variables are less statistically significant than in equation (10.23). The gnews interaction has a t statistic of about 1.64, which is significant at the 10% level against a one-sided alternative. (Since the dependent variable is binary, this is a case where we must appeal to asymptotics. Unfortunately, we have only 20 observations.) The inflation variable has the expected sign but is not statistically significant.(ii) There are two fitted values less than zero, and two fitted values greater than one.(iii) Out of the 10 elections with demwins = 1, 8 of these are correctly predicted. Out of the 10 elections with demwins = 0, 7 are correctly predicted. So 15 out of 20 elections through 1992 are correctly predicted. (But, remember, we used data from these years to obtain the estimated equation.)(iv) The explanatory variables are partyWH = 1, incum = 1, gnews = 3, and inf = 3.019. Therefore, for 1996,dem w ins = .441 - .473 + .479 + .059(3) - .024(3.019) ≈ .552.Because this is above .5, we would have predicted that Clinton would win the 1996 election, as he did.(v) The regression of ˆu on 1ˆt u- produces ˆρ≈ -.164 with heteroskedasticity-robust standardterror of about .195. (Because the LPM contains heteroskedasticity, testing for AR(1) serial correlation in an LPM generally requires a heteroskedasticity-robust test.) Therefore, there is little evidence of serial correlation in the errors. (And, if anything, it is negative.)(vi) The heteroskedasticity-robust standard errors are given in [⋅] below the usual standard errors:dem w ins = .441 -.473 partyWH + .479 incum + .059 partyWH⋅gnews(.107) (.354) (.205) (.036)[.086] [.301] [.185] [.030]– .024 partyWH⋅inf(.028)[.019]n = 20, R2 = .437, 2R = .287.In fact, all heteroskedasticity-robust standard errors are less than the usual OLS standard errors, making each variable more significant. For example, the t statistic on partyWH⋅gnews becomes about 1.97, which is notably above 1.64. But we must remember that the standard errors in the LPM have only asymptotic justification. With only 20 observations it is not clear we should prefer the heteroskedasticity-robust standard errors to the usual ones.C12.7 (i) The iterated Prais-Winsten estimates are given below. The estimate of ρis, to three decimal places, .293, which is the same as the estimate used in the final iteration of Cochrane-Orcutt:chnimp = -37.08 + 2.94 log(chempi) + 1.05 log(gas) + 1.13 log(rtwex) log()(22.78) (.63) (.98) (.51)-.016 befile6- .033 affile6- .577 afdec6(.319) (.322) (.342)n = 131, R2 = .202(ii) Not surprisingly, the C-O and P-W estimates are quite similar. To three decimal places,they use the same value of ˆρ(to four decimal places it is .2934 for C-O and .2932 for P-W). The only practical difference is that P-W uses the equation for t = 1. With n = 131, we hope this makes little difference.C12.9 (i) Here are the OLS regression results:log()avgprc = -.073 - .0040 t - .0101 mon - .0088 tues + .0376 wed + .0906 thurs (.115) (.0014) (.1294) (.1273) (.1257) (.1257)n = 97, R 2 = .086The test for joint significance of the day-of-the-week dummies is F = .23, which gives p -value = .92. So there is no evidence that the average price of fish varies systematically within a week.(ii) The equation islog()avgprc = -.920 - .0012 t - .0182 mon - .0085 tues + .0500 wed + .1225 thurs (.190) (.0014) (.1141) (.1121) (.1117) (.1110)+ .0909 wave2 + .0474 wave3(.0218) (.0208)n = 97, R 2 = .310Each of the wave variables is statistically significant, with wave2 being the most important. Rough seas (as measured by high waves) would reduce the supply of fish (shift the supply curve back), and this would result in a price increase. One might argue that bad weather reduces the demand for fish at a market, too, but that would reduce price. If there are demand effects captured by the wave variables, they are being swamped by the supply effects.(iii) The time trend coefficient becomes much smaller and statistically insignificant. We can use the omitted variable bias table from Chapter 3, Table 3.2 to determine what is probably going on. Without wave2 and wave3, the coefficient on t seems to have a downward bias. Since we know the coefficients on wave2 and wave3 are positive, this means the wave variables arenegatively correlated with t . In other words, the seas were rougher, on average, at the beginning of the sample period. (You can confirm this by regressing wave2 on t and wave3 on t .)(iv) The time trend and daily dummies are clearly strictly exogenous, as they are just functions of time and the calendar. Further, the height of the waves is not influenced by past unexpected changes in log(avgprc ).(v) We simply regress the OLS residuals on one lag, getting ˆ垐.618,se().081,7.63.t ρρρ=== Therefore, there is strong evidence of positive serial correlation.(vi) The Newey-West standard errors are 23垐se().0234 and se().0195.wave wave ββ== Given the significant amount of AR(1) serial correlation in part (v), it is somewhat surprising that these standard errors are not much larger compared with the usual, incorrect standard errors. In fact,the Newey-West standard error for 3ˆwave βis actually smaller than the OLS standard error.(vii) The Prais-Winsten estimates arelog()avgprc = -.658 - .0007 t + .0099 mon + .0025 tues + .0624 wed + .1174 thurs (.239) (.0029) (.0652) (.0744) (.0746) (.0621)+ .0497 wave2 + .0323 wave3(.0174) (.0174)n = 97, R 2 = .135The coefficient on wave2 drops by a nontrivial amount, but it still has a t statistic of almost 3. The coefficient on wave3 drops by a relatively smaller amount, but its t statistic (1.86) is borderline significant. The final estimate of ρ is about .687.C12.11 (i) The average of 2ˆi uover the sample is 4.44, with the smallest value being .0000074 and the largest being 232.89.(ii) This is the same as C12.4, part (ii):2ˆi u = 3.26 - .789 return t-1 + .297 21t return - + residual t (0.44) (.196) (.036)n = 689, R 2 = .130.(iii) The graph of the estimated variance function isThe variance is smallest when return -1 is about 1.33, and the variance is then about 2.74.(iv) No. The graph in part (iii) makes this clear, as does finding that the smallest variance estimate is 2.74.(v) The R -squared for the ARCH(1) model is .114, compared with .130 for the quadratic in return -1. We should really compare adjusted R -squareds, because the ARCH(1) model contains only two total parameters. For the ARCH(1) model, 2R is about .112; for the model in part (ii), 2R = .128. Therefore, after adjusting for the different df , the quadratic in return -1 fits better than the ARCH(1) model.(vi) The coefficient on 22ˆt uis only .042, and its t statistic is barely above one (t = 1.09). Therefore, an ARCH(2) model does not seem warranted. The adjusted R -squared is about .113, so the ARCH(2) fits worse than the model estimated in part (ii).。
伍德里奇计量经济学讲义9
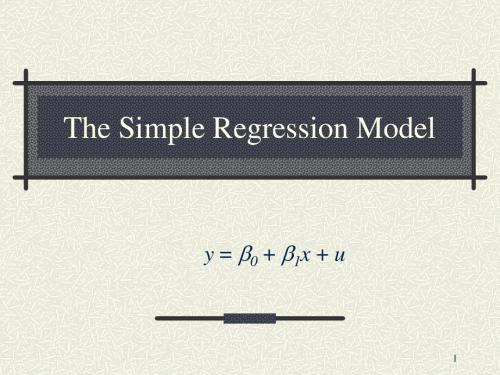
11
More Derivation of OLS
We want to choose values of the parameters that will ensure that the sample versions of our moment restrictions are true The sample versions are as follows:
n
2
providedthat xi x 0
2
15
Summary of OLS slope estimate
The slope estimate is the sample covariance between x and y divided by the sample variance of x If x and y are positively correlated, the slope will be positive If x and y are negatively correlated, the slope will be negative Only need x to vary in our sample
伍德里奇计量经济学讲义8PPT课件
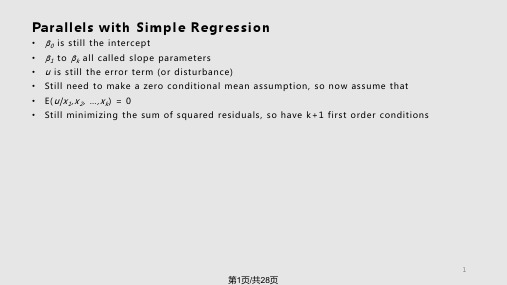
18
第18页/共28页
Variance of the at the sampling distribution of our estimate is centered around the true parameter
Want to think about how spread out this distribution is
第12页/共28页
Omitted Variable Bias (cont)
Recall thetruemodel,so that
yi b0 b1xi1 b2 xi2 ui , so t he
n um erat o rbeco m es
xi1 x1 b0 b1xi1 b2xi2 ui b1 xi1 x1 2 b2 xi1 x1 xi2 xi1 x1 ui
1
第1页/共28页
Interpreting Multiple Regression
yˆ bˆ0 bˆ1x1 bˆ2x2 ... bˆk xk , so yˆ bˆ1x1 bˆ2 x2 ... bˆk xk ,
so holdingx2,...,xk fixedimpliesthat
伍德里奇《计量经济学导论--现代观点》
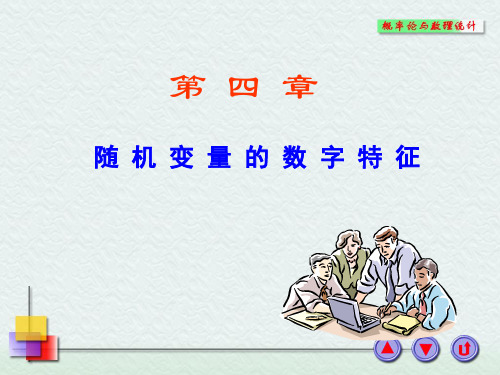
X
22
3
1.
15
p
0.2 0.1 0.1 0.1 0.1 0.3 0.1
( X ,Y ) (1,1) (1,0) (1,1) (2,1) (2,1) (3,0) (3,1)
( X Y )2 4 1 0 9 1 9 4
得 E[(X Y )2] 4 0.3 1 0.2 0 0.1 9 0.4 5.
( X ,Y ) (1,1) (1,0) (1,1) (2,1) (2,1) (3,0) (3,1) Y X 1 0 1 1 2 1 2 0 1 3
于是
E Y 1 0.2 0 0.1 1 0.1 1 0.1 1 0.1 0 0.3 1 0.1
(2) 级数的绝对收敛性保证了级数的和不 随级数各项次序的改变而改变 , 之所以这样要 求是因为数学期望是反映随机变量X 取可能值 的平均值,它不应随可能值的排列次序而改变.
(3) 随机变量的数学期望与一般变量的算 术平均值不同.
例1 谁的技术比较好? 甲,乙两个射手,他们的射击技术分别为
甲射手
击中环数 8 9 10 概率 0.3 0.1 0.6
第四章
随机变量的数字特征
第一节 数学期望
一、随机变量的数学期望 二、随机变量函数的数学期望 三、数学期望的性质 四、小结
一、随机变量的数学期望
1. 离散型随机变量的数学期望
定义4.1设离散型随机变量 X 的分布律为
P{ X xk } pk , k 1,2,.
若级数
xk pk 绝对收敛,则称级数
故甲射手的技术比较好.
例2 如何确定投资决策方向?
某人有10万元现金, 想投资
伍德里奇 计量经济学导论
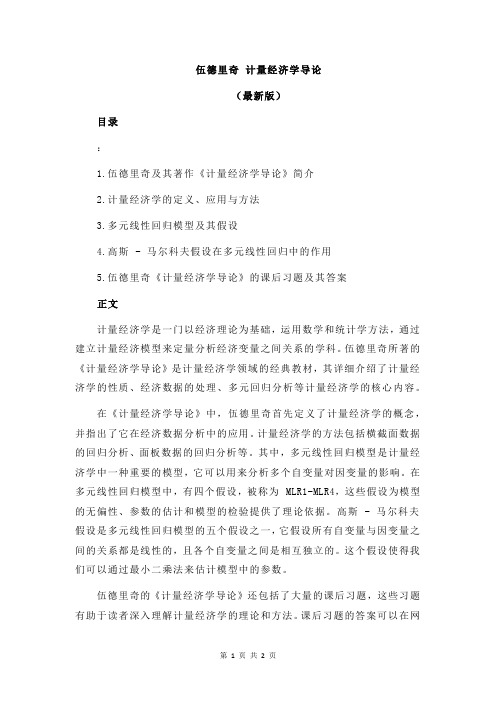
伍德里奇计量经济学导论
(最新版)
目录
:
1.伍德里奇及其著作《计量经济学导论》简介
2.计量经济学的定义、应用与方法
3.多元线性回归模型及其假设
4.高斯 - 马尔科夫假设在多元线性回归中的作用
5.伍德里奇《计量经济学导论》的课后习题及其答案
正文
计量经济学是一门以经济理论为基础,运用数学和统计学方法,通过建立计量经济模型来定量分析经济变量之间关系的学科。
伍德里奇所著的《计量经济学导论》是计量经济学领域的经典教材,其详细介绍了计量经济学的性质、经济数据的处理、多元回归分析等计量经济学的核心内容。
在《计量经济学导论》中,伍德里奇首先定义了计量经济学的概念,并指出了它在经济数据分析中的应用。
计量经济学的方法包括横截面数据的回归分析、面板数据的回归分析等。
其中,多元线性回归模型是计量经济学中一种重要的模型,它可以用来分析多个自变量对因变量的影响。
在多元线性回归模型中,有四个假设,被称为 MLR1-MLR4,这些假设为模型的无偏性、参数的估计和模型的检验提供了理论依据。
高斯 - 马尔科夫假设是多元线性回归模型的五个假设之一,它假设所有自变量与因变量之间的关系都是线性的,且各个自变量之间是相互独立的。
这个假设使得我们可以通过最小二乘法来估计模型中的参数。
伍德里奇的《计量经济学导论》还包括了大量的课后习题,这些习题有助于读者深入理解计量经济学的理论和方法。
课后习题的答案可以在网
络上找到,如 Daisy-Tung 的博客、word 版伍德里奇所著的《计量经济学导论》等。
这些答案为读者提供了自我检验和巩固知识的机会,是学习计量经济学的重要资源。
伍德里奇 计量经济学导论
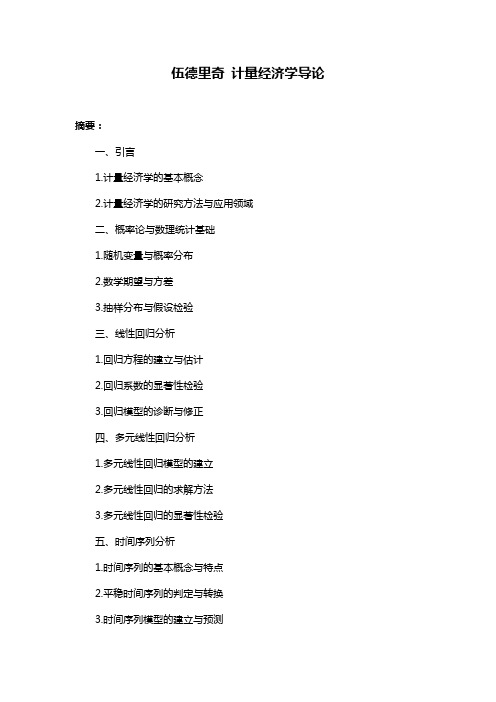
伍德里奇计量经济学导论摘要:一、引言1.计量经济学的基本概念2.计量经济学的研究方法与应用领域二、概率论与数理统计基础1.随机变量与概率分布2.数学期望与方差3.抽样分布与假设检验三、线性回归分析1.回归方程的建立与估计2.回归系数的显著性检验3.回归模型的诊断与修正四、多元线性回归分析1.多元线性回归模型的建立2.多元线性回归的求解方法3.多元线性回归的显著性检验五、时间序列分析1.时间序列的基本概念与特点2.平稳时间序列的判定与转换3.时间序列模型的建立与预测六、非参数统计方法1.非参数检验的基本思想与方法2.非参数回归与插值方法3.非参数统计方法的优缺点及应用场景七、计量经济学在实践中的应用1.我国经济发展中的计量经济学应用案例2.计量经济学在国际贸易、金融、环境等领域的应用3.计量经济学在政策评估与制定中的作用八、伍德里奇计量经济学导论的评价与启示1.教材的结构与内容特点2.伍德里奇计量经济学导论在我国的影响力3.对我国计量经济学教育的启示正文:计量经济学是一门运用概率论、统计学、数学等方法研究经济现象及其规律的科学。
在当今经济学领域,计量经济学已成为一门重要的分支学科,广泛应用于科研、教学和实践。
伍德里奇《计量经济学导论》一书,系统地阐述了计量经济学的基本原理、方法及应用,为读者提供了宝贵的理论指导和实践经验。
本书首先介绍了计量经济学的基本概念和研究方法。
计量经济学的研究方法主要包括实证分析、理论分析及实证与理论相结合的分析方法。
研究范围涉及宏观、微观及政策评估等多个领域。
此外,本书还简要介绍了概率论和数理统计的基本知识,为后续章节的学习奠定了基础。
在概率论和数理统计基础部分,本书详细讲解了随机变量、概率分布、数学期望、方差等概念,以及抽样分布、假设检验等统计方法。
这些知识为后续的回归分析提供了理论支持。
线性回归分析是计量经济学的重要内容之一。
本书介绍了回归方程的建立与估计、回归系数的显著性检验以及回归模型的诊断与修正方法。
伍德里奇计量经济学讲义3
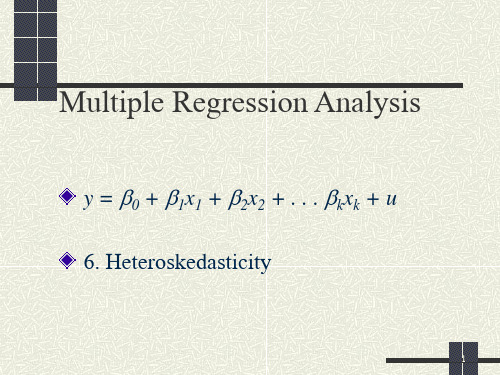
Alternate form of the White test
Consider that the fitted values from OLS, ŷ, are a function of all the x’s Thus, ŷ2 will be a function of the squares and crossproducts and ŷ and ŷ2 can proxy for all of the xj, xj2, and xjxh, so Regress the residuals squared on ŷ and ŷ2 and use the R2 to form an F or LM statistic Note only testing for 2 restrictions now
7
Robust Standard Errors (cont)
Important to remember that these robust standard errors only have asymptotic justification – with small sample sizes t statistics formed with robust standard errors will not have a distribution close to the t, and inferences will not be correct In Stata, robust standard errors are easily obtained using the robust option of reg
13
Weighted Least Squares
While it’s always possible to estimate robust standard errors for OLS estimates, if we know something about the specific form of the heteroskedasticity, we can obtain more efficient estimates than OLS The basic idea is going to be to transform the model into one that has homoskedastic errors – called weighted least squares
伍德里奇 计量经济学导论
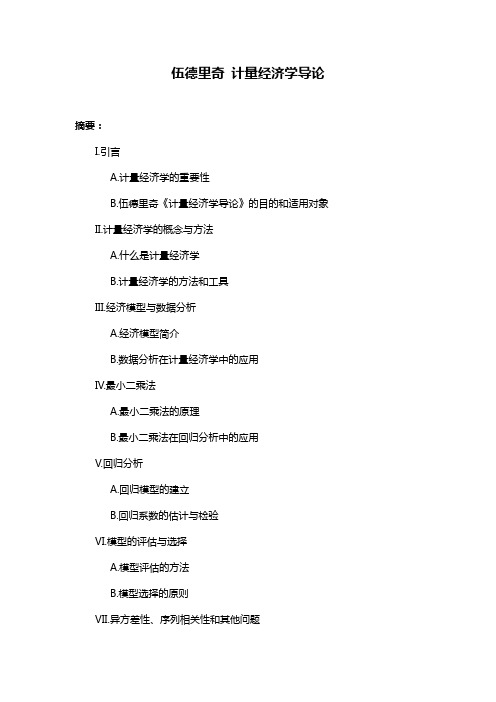
伍德里奇计量经济学导论摘要:I.引言A.计量经济学的重要性B.伍德里奇《计量经济学导论》的目的和适用对象II.计量经济学的概念与方法A.什么是计量经济学B.计量经济学的方法和工具III.经济模型与数据分析A.经济模型简介B.数据分析在计量经济学中的应用IV.最小二乘法A.最小二乘法的原理B.最小二乘法在回归分析中的应用V.回归分析A.回归模型的建立B.回归系数的估计与检验VI.模型的评估与选择A.模型评估的方法B.模型选择的原则VII.异方差性、序列相关性和其他问题A.异方差性的处理B.序列相关性的检验与处理C.其他可能出现的问题及解决方法VIII.实证分析与应用A.实证分析的步骤B.计量经济学在实际问题中的应用IX.总结与展望A.计量经济学导论的主要内容回顾B.计量经济学的发展趋势和前景正文:在当今经济学的各个领域中,计量经济学发挥着越来越重要的作用。
它不仅为经济理论提供了实证依据,而且为政策制定者提供了决策依据。
伍德里奇所著的《计量经济学导论》旨在为初学者提供一个关于计量经济学的全面认识,帮助他们掌握计量经济学的基本概念、方法和应用。
在本书中,伍德里奇首先介绍了计量经济学的重要性,以及本书的目的和适用对象。
接着,他对计量经济学的基本概念和方法进行了详细阐述,包括经济模型、数据分析、最小二乘法等。
此外,他还深入讲解了回归分析的原理和方法,以及如何对回归模型进行评估和选择。
在本书的后续章节中,伍德里奇对可能出现的问题,如异方差性、序列相关性等进行了讨论,并介绍了处理这些问题的方法。
他还通过实证分析,展示了计量经济学在实际问题中的应用,例如在通货膨胀、失业、贸易政策等方面的应用。
总之,《计量经济学导论》是一本很好的入门教材,它系统地介绍了计量经济学的基本概念、方法和应用。
1伍德里奇计量经济学绪论

• 模型:是对现实的描述和模拟。
对现实的各种不同的描述和模拟方法,就构成 了各种不同的模型,例如,物理模型、几何模 型、数学模型和计算机模拟模型等。
• 经济数学模型是用数学方法描述经济活动,根 据所采用的数学方法不同,对经济活动揭示的 程度不同,构成各类不同的经济数学模型。
• 近20位担任过世界计量经济学会会长 • 30余位左右在获奖成果中应用了计量经济学
创立
Frisch
经 典
建立第1个应用模型
Tinbergen
计
建立概率论基础
Haavelmo
量
经
发展数据基础
Stone
济
学
发展应模型
Klein
建立投入产出模型
Leontief
The Bank of Sweden Prize in Economic Sciences in Memory of Alfred Nobel 2000
△ 在经济学科中占据极重要的地位
克莱因(R.Klein):“计量经济学已经在经 济学科中居于最重要的地位”,“在大多数大 学和学院中,计量经济学的讲授已经成为经济 学课程表中最有权威的一部分”。
萨缪尔森(P.Samuelson) :“第二次大战后 的经济学是计量经济学的时代”。
二、计量经济学模型
本,L表示劳动。
• 计量经济模型揭示经济活动中各个因素之间的 定量关系,用随机性的数学方程加以描述。上
述生产活动中因素之间的关系,用随机数学方 程描述为
•
QAKL
• 其中μ为随机误差项。这就是计量经济学模型 的理论形式。
三、计量经济学的内容体系
△ 广义计量经济学和狭义计量经济学 △ 初、中、高级计量经济学 △ 理论计量经济学和应用计量经济学 △ 经典计量经济学和非经典计量经济学 △ 微观计量经济学和宏观计量经济学
伍德里奇计量经济学讲义4
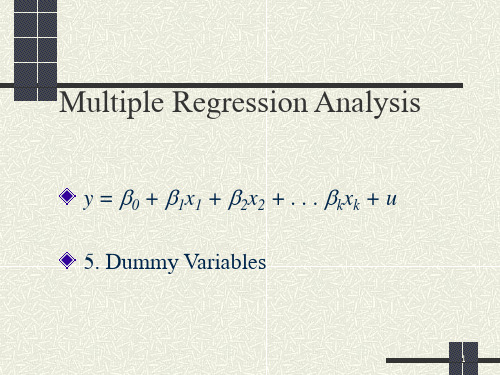
Multiple Categories (cont)
Any categorical variable can be turned into a set of dummy variables Because the base group is represented by the intercept, if there are n categories there should be n – 1 dummy variables If there are a lot of categories, it may make sense to group some together Example: top 10 ranking, 11 – 25, etc.
16
Self-selection Problems
If we can control for everything that is correlated with both participation and the outcome of interest then it’s not a problem Often, though, there are unobservables that are correlated with participation In this case, the estimate of the program effect is biased, and we don’t want to set policy based on it!
9
Example of d0 > 0 and d1 < 0 y
y = b0 + b1= 0 dx d=1 y = (b0 + d0) + (b1 + d1) x x
- 1、下载文档前请自行甄别文档内容的完整性,平台不提供额外的编辑、内容补充、找答案等附加服务。
- 2、"仅部分预览"的文档,不可在线预览部分如存在完整性等问题,可反馈申请退款(可完整预览的文档不适用该条件!)。
- 3、如文档侵犯您的权益,请联系客服反馈,我们会尽快为您处理(人工客服工作时间:9:00-18:30)。
More difficult if one model uses y and the other uses ln(y) Can follow same basic logic and transform predicted ln(y) to get ŷ for the second step In any case, Davidson-MacKinnon test may reject neither or both models rather than clearly preferring one specification
2
Functional Form (continued)
First, use economic theory to guide you Think about the interpretation Does it make more sense for x to affect y in percentage (use logs) or absolute terms? Does it make more sense for the derivative of x1 to vary with x1 (quadratic) or with x2 (interactions) or to be fixed?
7
Proxy Variables
What if model is misspecified because no data is available on an important x variable? It may be possible to avoid omitted variable bias by using a proxy variable A proxy variable must be related to the unobservable variable – for example: x3* = d0 + d3x3 + v3, where * implies unobserved Now suppose we just substitute x3 for x3*
Multiple Regression Analysis
y = b0 + b1x1 + b2x2 + . . . bkxk + u
7. Specification and Data Problems
1
Functional Form
We’ve seen that a linear regression can really fit nonlinear relationships Can use logs on RHS, LHS or both Can use quadratic forms of x’s Can use interactions of x’s How do we know if we’ve gotten the right functional form for our model?
3
Functional Form (continued)
We already know how to test joint exclusion restrictions to see if higher order terms or interactions belong in the model It can be tedious to add and test extra terms, plus may find a square term matters when really using logs would be even better A test of functional form is Ramsey’s regression specification error test (RESET)
4
Ramsey’s RESET
RESET relies on a trick similar to the special form of the White test Instead of adding functions of the x’s directly, we add and test functions of ŷ So, estimate y = b0 + b1x1 + … + bkxk + d1ŷ2 + d1ŷ3 +error and test H0: d1 = 0, d2 = 0 using F~F2,n-k-3 or LM~χ22
5
Nonnested Alternative Tests
If the models have the same dependent variables, but nonnested x’s could still just make a giant model with the x’s from both and test joint exclusion restrictions that lead to one model or the other An alternative, the Davidson-MacKinnon test, uses ŷ from one model as regressor in the second model and tests for significance
8
Proxy Variables (continued)
What do we need for for this solution to
give us consistent estimates of b1 and b2?
E(x3* | x1, x2, x3) = E(x3* | x3) = d0 + d3x3 That is, u is uncorrelated with x1, x2 and x3* and v3 is uncorrelated with x1, x2 and x3