不可重复GRR研究
GRR卡尺重复性和再现性分析报告
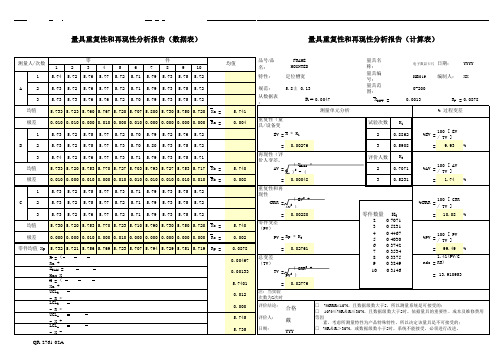
0.000
0.000
0.000
0.000
0.000
0.000
0.000
0.000
0.000
0.000
0.000
0.000
0.000
0.000
0.000
0.000
0.000
0.000
0.000
0.000
0.000
0.000
0.000
0.000
R-Chart
0.014 0.012 0.010 0.008 0.006 0.004 0.002 0.000
7
5.7933
8
5.7267
9
5.7533
10
5.7167
1
5.7300
2
5.7200
3
5.7533
4
5.7700
5
5.7233
6
5.7100
7
5.7900
8
5.7300
9
5.7500
10
5.7200
5.745
5.745
5.745
5.745
5.745
5.745
5.745
5.745
5.745
5.745
5.741 0.004
重复性(量具/设备变差,EV) EV = R * K1 = 0.00276
试验次数 2 3 评价人数 2 3
K1 0.8862 0.5908 K2 0.7071 0.5231 %AV = 100 [ AV / TV ] = 1.74 % %EV = 100 [ EV / TV ] = 9.93 %
0.012
0.012
0.012
grr指标 -回复
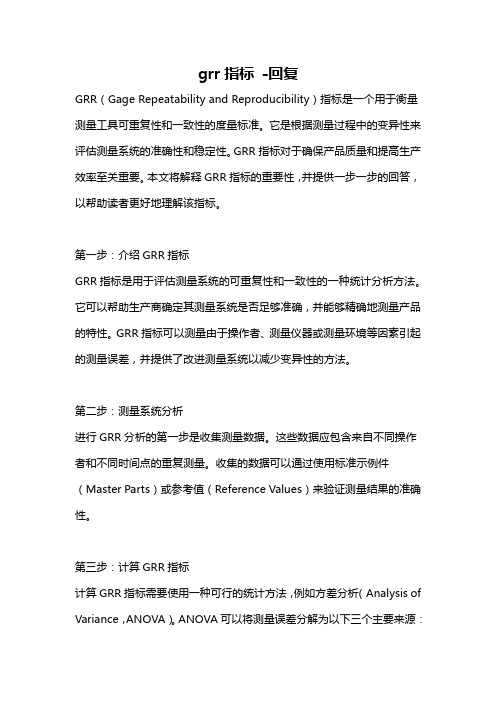
grr指标-回复GRR(Gage Repeatability and Reproducibility)指标是一个用于衡量测量工具可重复性和一致性的度量标准。
它是根据测量过程中的变异性来评估测量系统的准确性和稳定性。
GRR指标对于确保产品质量和提高生产效率至关重要。
本文将解释GRR指标的重要性,并提供一步一步的回答,以帮助读者更好地理解该指标。
第一步:介绍GRR指标GRR指标是用于评估测量系统的可重复性和一致性的一种统计分析方法。
它可以帮助生产商确定其测量系统是否足够准确,并能够精确地测量产品的特性。
GRR指标可以测量由于操作者、测量仪器或测量环境等因素引起的测量误差,并提供了改进测量系统以减少变异性的方法。
第二步:测量系统分析进行GRR分析的第一步是收集测量数据。
这些数据应包含来自不同操作者和不同时间点的重复测量。
收集的数据可以通过使用标准示例件(Master Parts)或参考值(Reference Values)来验证测量结果的准确性。
第三步:计算GRR指标计算GRR指标需要使用一种可行的统计方法,例如方差分析(Analysis of Variance,ANOVA)。
ANOVA可以将测量误差分解为以下三个主要来源:重复性误差(Repeatability Error)、操作者误差(Operator Error)和重复性操作者误差(Reproducibility Operator Error)。
通过计算这些误差的方差可以得到每个组件的测量系统的稳定性。
第四步:解释GRR结果通过分析GRR结果,可以确定测量系统的可接受性。
一般来说,可接受的GRR指标应小于或等于总变异性的10。
具体而言,重复性和操作者误差应小于于总变异性的5,而重复性操作者误差应小于总变异性的2。
如果GRR指标超过这些阈值,测量系统需要进行改进以提高其稳定性。
第五步:改进测量系统如果GRR指标超过了可接受的阈值,需要采取措施来改善测量系统的可靠性和准确性。
GRR目视系统与CPK制程能力指数

GRR目视系统与CPK制程能力指数GRR(Gauge Repeatability and Reproducibility)是一种评估测试设备的可重复性和再现性的方法,而CPK(Capability Process Index)是一种衡量制程能力的指标。
两者在质量管理中起着重要作用,并且经常同时使用。
GRR目视系统是一种测试设备,用于检测和记录产品特征的值。
它通常由一个操作员使用,通过目视观察来判断产品是否符合要求。
GRR目视系统的可重复性是指同一个操作员在相同条件下进行多次测试,得到相似结果的能力。
而再现性是指不同操作员在相同条件下进行测试,得到相似结果的能力。
通过进行GRR测试,可以评估目视系统的可靠性和稳定性,确保其能够准确地记录产品特征的值。
CPK制程能力指数用于衡量制程的稳定性和一致性。
它统计了制程的分布情况,比较了制程上限和下限与规范上限和下限之间的差异。
CPK指数越高,表示制程越稳定,能够产生符合规范要求的产品。
CPK制程能力指数是一种客观地判断制程是否稳定并且能够达到产品质量要求的方法。
GRR目视系统和CPK制程能力指数在质量管理中通常同时使用。
首先,通过进行GRR测试,评估测试设备的可重复性和再现性,确保测试结果的准确性。
然后,使用CPK制程能力指数来判断制程的稳定性和一致性,确保制造出的产品符合规范要求。
这两种方法互相补充,帮助企业提高产品质量,减少不良品率,并确保产品的一致性和可靠性。
总之,GRR目视系统和CPK制程能力指数是质量管理中两种重要的评估方法。
GRR目视系统评估测试设备的可重复性和再现性,CPK制程能力指数评估制程的稳定性和一致性。
两者的使用可以确保产品质量的一致性,并帮助企业提高生产效率和竞争力。
GRR(Gauge Repeatability and Reproducibility,即测量设备的重复性和再现性)和CPK(Capability Process Index,即制程能力指数)是两种在质量管理中常用的评估方法。
GRR测量系统分析报告范例
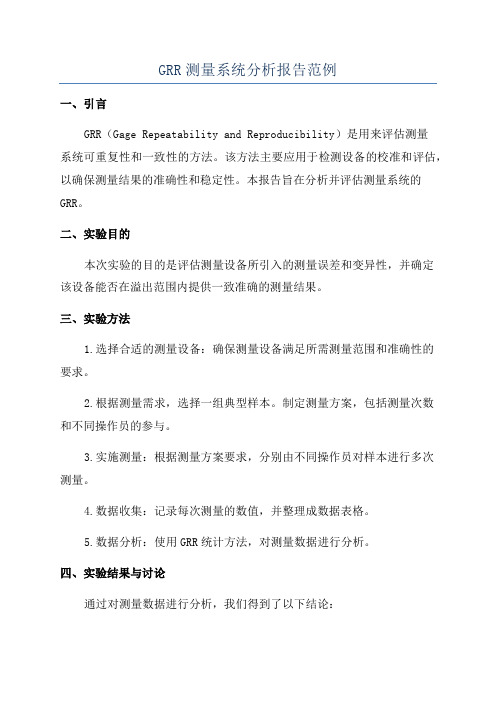
GRR测量系统分析报告范例一、引言GRR(Gage Repeatability and Reproducibility)是用来评估测量系统可重复性和一致性的方法。
该方法主要应用于检测设备的校准和评估,以确保测量结果的准确性和稳定性。
本报告旨在分析并评估测量系统的GRR。
二、实验目的本次实验的目的是评估测量设备所引入的测量误差和变异性,并确定该设备能否在溢出范围内提供一致准确的测量结果。
三、实验方法1.选择合适的测量设备:确保测量设备满足所需测量范围和准确性的要求。
2.根据测量需求,选择一组典型样本。
制定测量方案,包括测量次数和不同操作员的参与。
3.实施测量:根据测量方案要求,分别由不同操作员对样本进行多次测量。
4.数据收集:记录每次测量的数值,并整理成数据表格。
5.数据分析:使用GRR统计方法,对测量数据进行分析。
四、实验结果与讨论通过对测量数据进行分析,我们得到了以下结论:1. 测量设备的可重复性(Repeatability):根据GRR方法的定义,可重复性是指在同一操作员对样本进行多次测量时,测量结果的变异性。
可重复性通过测量系统内部误差来衡量。
经过分析,我们得到了测量设备的可重复性为X%。
根据测量标准的要求,此可重复性符合要求。
2. 测量设备的一致性(Reproducibility):一致性是指在不同操作员对同一样本进行测量时,测量结果之间的变异性。
一致性通过测量系统间误差来衡量。
经过分析,我们得到了测量设备的一致性为X%。
根据测量标准的要求,此一致性符合要求。
3.单次测量误差:通过计算测量系统的稳定性指标,我们得到了单次测量误差为X。
根据测量标准的要求,此误差在可接受范围内。
五、结论与建议根据我们对测量系统的分析,结合测量标准的要求,我们得出以下结论:1.所评估的测量系统的可重复性和一致性符合要求,能够满足预期的测量准确性和稳定性。
2.单次测量误差也在可接受的范围内。
3.根据实验结果,我们建议对测量系统进行定期的校准和维护,以确保其性能的稳定性和准确性。
GRR(重复性和再现性)简单介绍
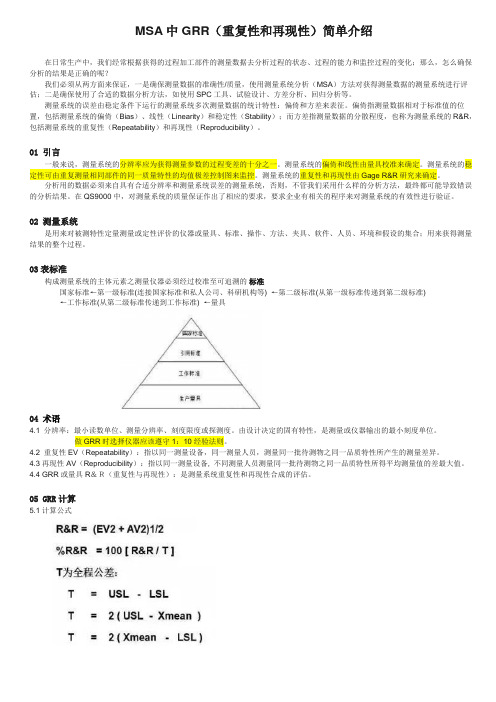
MSA中GRR(重复性和再现性)简单介绍在日常生产中,我们经常根据获得的过程加工部件的测量数据去分析过程的状态、过程的能力和监控过程的变化;那么,怎么确保分析的结果是正确的呢?我们必须从两方面来保证,一是确保测量数据的准确性/质量,使用测量系统分析(MSA)方法对获得测量数据的测量系统进行评估;二是确保使用了合适的数据分析方法,如使用SPC工具、试验设计、方差分析、回归分析等。
测量系统的误差由稳定条件下运行的测量系统多次测量数据的统计特性:偏倚和方差来表征。
偏倚指测量数据相对于标准值的位置,包括测量系统的偏倚(Bias)、线性(Linearity)和稳定性(Stability);而方差指测量数据的分散程度,也称为测量系统的R&R,包括测量系统的重复性(Repeatability)和再现性(Reproducibility)。
01 引言一般来说,测量系统的分辨率应为获得测量参数的过程变差的十分之一。
测量系统的偏倚和线性由量具校准来确定。
测量系统的稳定性可由重复测量相同部件的同一质量特性的均值极差控制图来监控。
测量系统的重复性和再现性由Gage R&R研究来确定。
分析用的数据必须来自具有合适分辨率和测量系统误差的测量系统,否则,不管我们采用什么样的分析方法,最终都可能导致错误的分析结果。
在QS9000中,对测量系统的质量保证作出了相应的要求,要求企业有相关的程序来对测量系统的有效性进行验证。
02测量系统是用来对被测特性定量测量或定性评价的仪器或量具、标准、操作、方法、夹具、软件、人员、环境和假设的集合;用来获得测量结果的整个过程。
03表标准构成测量系统的主体元素之测量仪器必须经过校准至可追溯的标准国家标准←第一级标准(连接国家标准和私人公司、科研机构等)←第二级标准(从第一级标准传递到第二级标准)←工作标准(从第二级标准传递到工作标准)←量具04 术语4.1 分辨率:最小读数单位、测量分辨率、刻度限度或探测度。
测量系统分析---5 重复性和再现性 GRR
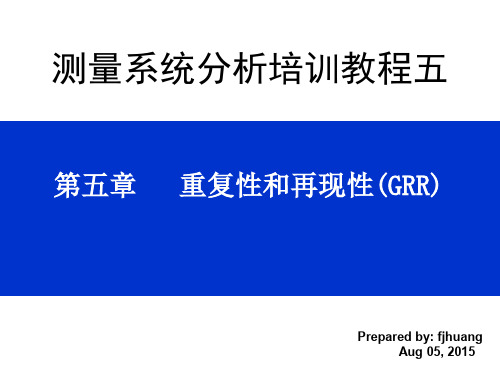
EV---Equipment Variation 设备变差----重复性: AV---Appraiser Variation 评价者变差---再现性: PV---Part Variation 零件的变差--------产品偏差:
与评价人之间的交互作用和由于量具造成的重复误差。但 计算复杂,需掌握一定程度的统计学知识。
-7-
第五章
重复性和再现性
GRR分析方法---极差法
例:2个评价人对5个零件进行测量。在研究中,两个评价人各将每 个零件测量一次。每个零件的极差是评价人A获得测量值和B获得 测量值之间的绝对差值。计算极差的和与平均极差。通过将平均极 差均值乘以1/d2*得到标准偏差.
计算A评价者测试数据的平均值 计算B评价者测试数据的平均值
计算C评价者测试数据的平均值 计算全部评价者所测数据的平均值 计算单个零件的平均值 计算零件全距Rp 计算最大与最小量测值班的差异 计算零件全距的极差R的平均值
-12-
6 7
8 9 10 11
=Max(Xa,Xb, Xc)-Min(Xa,Xb,Xc) =( Ra + Rb + Rc ) / 3
第五章
重复性和再现性
GRR分析方法
● 极差法 (全距法) 特点:简单快捷,能提供整体大概概况 ● 均值极差法(全距及平均值法)(包括控制图法) 特点:可将测量系统的变差分成两个部分-----重复性和再
现性,而不是他们的交互作用
● ANOVE法--方差分析法(变异数分析法) 特点:是一种标准统计技术,可算出零件、评价人、零件
GRR再现性和重复性
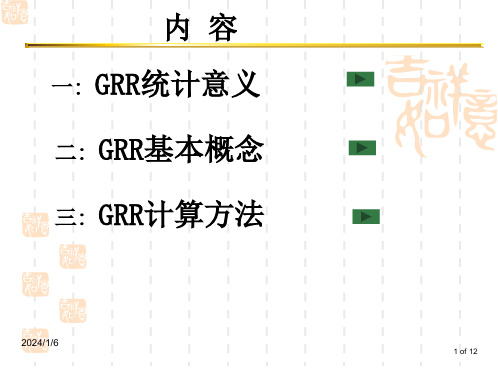
2024/1/6
8 of 12
3 GRR计算(二)
有3种方法:
➢ 极差法 (Range Method)
➢均值-极差法 (Average and Range Method
➢方差分析法
(ANOVA)
2024/1/6
9 of 12
量测系统的判定
GRR=<10% 量具系统可接受
可接受.可不接受,决定于该量具系
5 of 12
再生性(Reproducibility)
➢ 再生性又称作业者变异,指不同作业者以相同量具量测相同产品 的同一特性时,量测平均值的变异(3同一异)
➢ 在量测的条件有所变化下,重复的量测值之间的变异(操作者,装 夹,位置,环境条件,较长的时间段)
➢ 为外在因素引起的量测系统的变异
主值
检查员 A 检查员 B
内容 一: GRR统计意义 二: GRR基本概念 三: GRR计算方法
2024/1/6
1 of 12
1 GRR统計意义
➢ 测量系统变异概述
实际值
实际产品变异
实际值
测量值
量测系统
量测变异
量检具造成的变异 操作员造成的变异
观察到的产品变异
2024/1/6
2 of 12
测量系统精确度与准确度
准确度:平均值
2024/1/6
4 of 12
重复性(Repeatability)
➢ 重复性又称为量具变异,是指用同一种量具,同一位作业者, 多次量测相同零件的相同特性时的变异(四同)
➢ 在完全相同的量测条件下,多次量测值间的差异
➢ 为量测系统本身产生的差异,随机误差范畴
良好重复性
主值
主值
GRR再现性和重复性

量测系统分析 Gauge Repeatability and Reproducibility
2019/5/3
1 of 12
内容 一: GRR统计意义 二: GRR基本概念 三: GRR计算方法
2019/5/3
2 of 12
1 GRR统計意义
测量系统变异概述
实际值
实际产品变异
实际值
测量值
可接受.可不接受,决定于该量具系
10%<GRR<30% 统之重要性,修理所需之费用等因
素
GRR>=30%
量具系统不能接受, 须予以改进
2019/5/3
11 of 12
2019/5/3
12 of 12
总变异:TV TV = GR&R 2+ PV 2
2019/5/3
9 of 12
3 GRR计算(二)
有3种方法:
极差法 (Range Method)
均值-极差法 (Average and Range Method
方差分析法
(ANOVA)
2019/5/3
10 of 12
量测系统的判定
GRR=<10% 量具系统可接受
精确度:变动性 观察到的变动性 = 产品变动 + 衡量的变动
实际值
测量值
2 总量
=
2 产品
+
2 测量
衡量系统变动性- 通过 “GR&R 研究”决定
2019/5/3
5 of 12
重复性(Repeatability)
重复性又称为量具变异,是指用同一种量具,同一位作业者, 多次量测相同零件的相同特性时的变异(四同)
GRR原理及计算
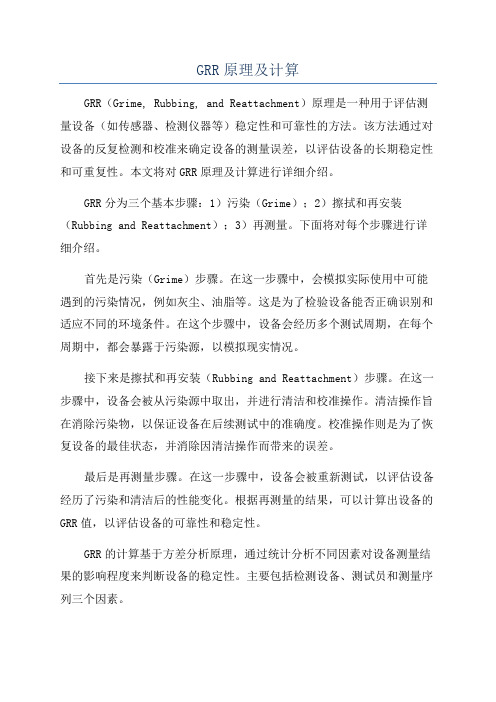
GRR原理及计算GRR(Grime, Rubbing, and Reattachment)原理是一种用于评估测量设备(如传感器、检测仪器等)稳定性和可靠性的方法。
该方法通过对设备的反复检测和校准来确定设备的测量误差,以评估设备的长期稳定性和可重复性。
本文将对GRR原理及计算进行详细介绍。
GRR分为三个基本步骤:1)污染(Grime);2)擦拭和再安装(Rubbing and Reattachment);3)再测量。
下面将对每个步骤进行详细介绍。
首先是污染(Grime)步骤。
在这一步骤中,会模拟实际使用中可能遇到的污染情况,例如灰尘、油脂等。
这是为了检验设备能否正确识别和适应不同的环境条件。
在这个步骤中,设备会经历多个测试周期,在每个周期中,都会暴露于污染源,以模拟现实情况。
接下来是擦拭和再安装(Rubbing and Reattachment)步骤。
在这一步骤中,设备会被从污染源中取出,并进行清洁和校准操作。
清洁操作旨在消除污染物,以保证设备在后续测试中的准确度。
校准操作则是为了恢复设备的最佳状态,并消除因清洁操作而带来的误差。
最后是再测量步骤。
在这一步骤中,设备会被重新测试,以评估设备经历了污染和清洁后的性能变化。
根据再测量的结果,可以计算出设备的GRR值,以评估设备的可靠性和稳定性。
GRR的计算基于方差分析原理,通过统计分析不同因素对设备测量结果的影响程度来判断设备的稳定性。
主要包括检测设备、测试员和测量序列三个因素。
其中,检测设备因素是指设备的测量误差和稳定性,可以通过GRR来评估。
测试员因素是指不同测试员使用相同的设备进行测试时产生的误差。
测量序列因素是指相同测试员在不同时间、不同顺序下测量同一样品时产生的误差。
根据方差分析原理,可以通过计算不同因素的占比来评估各个因素对总方差的贡献度。
具体来说,GRR的计算方法包括方差分析计算、方差分解和可重复性计算。
方差分析计算是将测量数据进行分组,计算组间方差和组内方差。
重复性和再现性
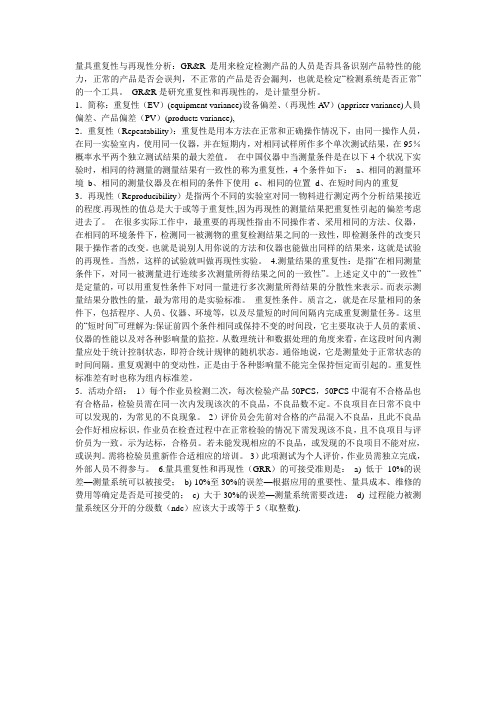
量具重复性与再现性分析:GR&R 是用来检定检测产品的人员是否具备识别产品特性的能力,正常的产品是否会误判,不正常的产品是否会漏判,也就是检定“检测系统是否正常”的一个工具。
GR&R是研究重复性和再现性的,是计量型分析。
1.简称:重复性(EV)(equipment variance)设备偏差、(再现性AV)(appriser variance)人員偏差、产品偏差(PV)(products variance),2.重复性(Repeatability):重复性是用本方法在正常和正确操作情况下,由同一操作人员,在同一实验室内,使用同一仪器,并在短期内,对相同试样所作多个单次测试结果,在95%概率水平两个独立测试结果的最大差值。
在中国仪器中当测量条件是在以下4个状况下实验时,相同的待测量的测量结果有一致性的称为重复性,4个条件如下:a、相同的测量环境b、相同的测量仪器及在相同的条件下使用c、相同的位置d、在短时间内的重复3.再现性(Reproducibility)是指两个不同的实验室对同一物料进行测定两个分析结果接近的程度.再现性的值总是大于或等于重复性,因为再现性的测量结果把重复性引起的偏差考虑进去了。
在很多实际工作中,最重要的再现性指由不同操作者、采用相同的方法、仪器,在相同的环境条件下,检测同一被测物的重复检测结果之间的一致性,即检测条件的改变只限于操作者的改变。
也就是说别人用你说的方法和仪器也能做出同样的结果来,这就是试验的再现性。
当然,这样的试验就叫做再现性实验。
4.测量结果的重复性:是指“在相同测量条件下,对同一被测量进行连续多次测量所得结果之间的一致性”。
上述定义中的“一致性”是定量的,可以用重复性条件下对同一量进行多次测量所得结果的分散性来表示。
而表示测量结果分散性的量,最为常用的是实验标准。
重复性条件。
质言之,就是在尽量相同的条件下,包括程序、人员、仪器、环境等,以及尽量短的时间间隔内完成重复测量任务。
GRR简单介绍
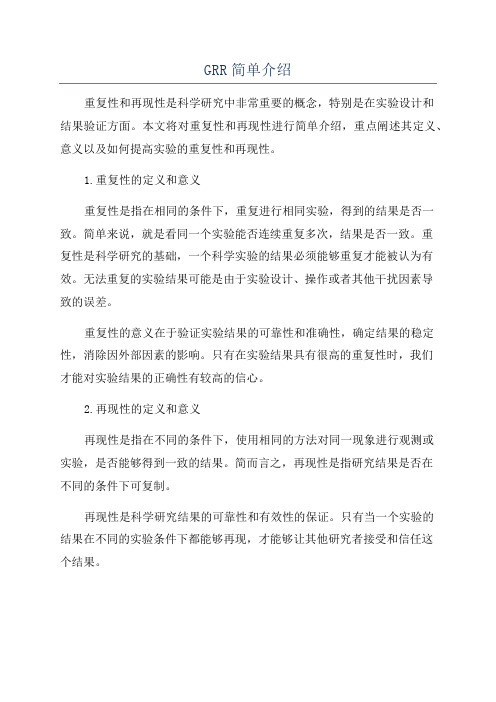
GRR简单介绍重复性和再现性是科学研究中非常重要的概念,特别是在实验设计和结果验证方面。
本文将对重复性和再现性进行简单介绍,重点阐述其定义、意义以及如何提高实验的重复性和再现性。
1.重复性的定义和意义重复性是指在相同的条件下,重复进行相同实验,得到的结果是否一致。
简单来说,就是看同一个实验能否连续重复多次,结果是否一致。
重复性是科学研究的基础,一个科学实验的结果必须能够重复才能被认为有效。
无法重复的实验结果可能是由于实验设计、操作或者其他干扰因素导致的误差。
重复性的意义在于验证实验结果的可靠性和准确性,确定结果的稳定性,消除因外部因素的影响。
只有在实验结果具有很高的重复性时,我们才能对实验结果的正确性有较高的信心。
2.再现性的定义和意义再现性是指在不同的条件下,使用相同的方法对同一现象进行观测或实验,是否能够得到一致的结果。
简而言之,再现性是指研究结果是否在不同的条件下可复制。
再现性是科学研究结果的可靠性和有效性的保证。
只有当一个实验的结果在不同的实验条件下都能够再现,才能够让其他研究者接受和信任这个结果。
再现性的意义在于验证研究结果的普适性和泛化能力,即是否可以在更广泛的条件下得到相同的结论。
一个研究结果只有在多个实验中都能够再现才能够真正具有可靠性和可信度。
3.如何提高实验的重复性和再现性3.1.实验设计良好的实验设计是保证实验重复性和再现性的基础。
合理的实验设计应具备以下特点:-控制变量:控制实验过程中其他可能影响结果的变量,保证实验稳定性;-随机分组:将实验对象随机分组,减少不确定性;-复盲实验:对于需要主观评估的实验,采用复盲实验,避免主观因素的干扰;-样本量计算:通过统计学方法计算样本量,确保结果的可信度。
3.2.数据处理和统计分析良好的数据处理和统计分析方法是提高实验重复性和再现性的关键。
以下是一些常用的方法:-统计分析:通过合适的统计学方法对实验数据进行分析,减少随机误差;- 内部一致性分析:例如Cronbach's α值用于评估测试的内部一致性;-外部效度分析:例如与已有研究结果进行对比,检验实验结果的一致性和稳定性。
grr指标 -回复
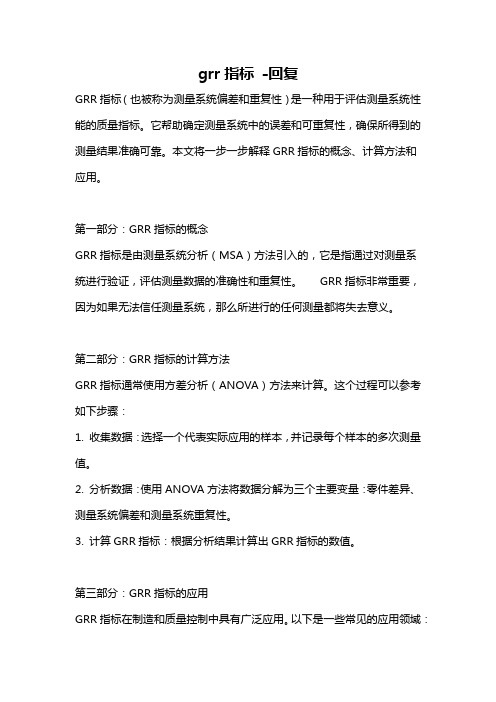
grr指标-回复GRR指标(也被称为测量系统偏差和重复性)是一种用于评估测量系统性能的质量指标。
它帮助确定测量系统中的误差和可重复性,确保所得到的测量结果准确可靠。
本文将一步一步解释GRR指标的概念、计算方法和应用。
第一部分:GRR指标的概念GRR指标是由测量系统分析(MSA)方法引入的,它是指通过对测量系统进行验证,评估测量数据的准确性和重复性。
GRR指标非常重要,因为如果无法信任测量系统,那么所进行的任何测量都将失去意义。
第二部分:GRR指标的计算方法GRR指标通常使用方差分析(ANOVA)方法来计算。
这个过程可以参考如下步骤:1. 收集数据:选择一个代表实际应用的样本,并记录每个样本的多次测量值。
2. 分析数据:使用ANOVA方法将数据分解为三个主要变量:零件差异、测量系统偏差和测量系统重复性。
3. 计算GRR指标:根据分析结果计算出GRR指标的数值。
第三部分:GRR指标的应用GRR指标在制造和质量控制中具有广泛应用。
以下是一些常见的应用领域:1. 测量设备选择:通过对不同测量系统进行GRR测试,可以选择最合适的测量设备,并确定其是否满足特定的测量要求。
2. 制造过程控制:通过定期进行GRR测试,可以监控测量系统的稳定性和准确性,并及时进行校准或调整。
3. 生产线优化:通过识别测量系统中的误差来源,可以采取措施降低这些误差,从而提高整个生产线的效率和质量。
4. 供应商评估:通过对供应商提供的测量系统进行GRR测试,可以评估其可靠性,并选择最合适的供应商。
第四部分:GRR指标解读和改善对于GRR指标的解读,一般认为指标值越小越好。
根据常见的行业标准,GRR指标为10以下被认为是良好的,20以下则被认为是可接受的。
如果GRR指标高于20,则可能需要采取改善措施,例如优化测量方法或设备,增加员工培训等。
改善GRR指标的方法通常包括以下步骤:1. 定期校准和维护测量设备,确保其准确性和稳定性。
2. 培训操作人员,提高其测量技巧和标准化程度。
不可重复的测量系统如何进行GRR分析(用minitab进行分析)

大家好!今天我们谈谈:“不可重复的测量系统如何进行测量系统分析”。我们用minitab软件进行分析
就是经过检测后,受检部件的完整性遭到破 坏或部分破坏,而不能重复测量
什么是不可重复的测量系统?
就是经过检测后,受检部件的完整性遭到破 坏或部分破坏,而不能重复测量
零件 序号
1
2
3
4
5
获取的方式
6
7
8
9
10
不同批号 不同炉号 不同批原料 不同班次
确保这10个零件有较大差异
零件 序号
1 2 3 4 5 6 7 8
9
评价人一
第一次试验 第二次试验 第三次试验 第一次试
验
34
35
35
35
评价人二
第二次试验 第三次试验
34
36
10
在可重复测量中,每个零件可以重复测量
选择:统计>质量工具>量具研究>创建量具R&R研究工作表 接下来使用Minitab软件进行分析。启动Minitab
创建量具R&R研究工作表
创建量具R&R研究工作表 在这个研究工作表中
运行“研究工作表”获得数据表格 我们根据“部件”以及“操作员”的顺序,分别实施试验工作
交叉性研究 这里先说一个交叉型研究和嵌套型研究。当测量的对象是相同时候,我们用交叉型研究
7组 7A—7F 36.5 36.4 36.6 36.7 36.6 36.5
8组 8A—8F 34.3 34.4 34.3 34.4 34.4 34.5
9组 9A—9F 35.6 35.6 35.7 35.5 35.6 35.6
grr指标 -回复
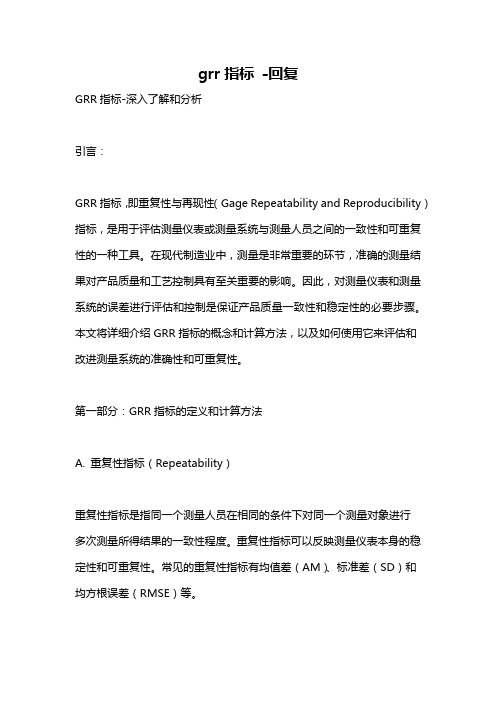
grr指标-回复GRR指标-深入了解和分析引言:GRR指标,即重复性与再现性(Gage Repeatability and Reproducibility)指标,是用于评估测量仪表或测量系统与测量人员之间的一致性和可重复性的一种工具。
在现代制造业中,测量是非常重要的环节,准确的测量结果对产品质量和工艺控制具有至关重要的影响。
因此,对测量仪表和测量系统的误差进行评估和控制是保证产品质量一致性和稳定性的必要步骤。
本文将详细介绍GRR指标的概念和计算方法,以及如何使用它来评估和改进测量系统的准确性和可重复性。
第一部分:GRR指标的定义和计算方法A. 重复性指标(Repeatability)重复性指标是指同一个测量人员在相同的条件下对同一个测量对象进行多次测量所得结果的一致性程度。
重复性指标可以反映测量仪表本身的稳定性和可重复性。
常见的重复性指标有均值差(AM)、标准差(SD)和均方根误差(RMSE)等。
计算公式如下:AM = ∑(测量值-平均值)/测量次数SD = √[∑(测量值-平均值)^2 / (测量次数-1)]RMSE = √(∑(测量值-平均值)^2 / 测量次数)B. 再现性指标(Reproducibility)再现性指标是指在相同的条件下,由不同的测量人员对同一个测量对象进行多次测量所得结果的一致性程度。
再现性指标主要反映测量人员之间的差异和不稳定性。
与重复性指标类似,常见的再现性指标也包括均值差、标准差和均方根误差等。
计算公式与重复性指标相同。
C. GRR指标(Gage Repeatability and Reproducibility)GRR指标综合考虑了重复性和再现性的指标,用于评估整个测量系统的一致性和可靠性。
GRR指标可基于不同的测量设计方法进行计算,如ANOVA方法、Shainin方法和平均移动范围(MMR)方法等。
其中,ANOVA方法是应用最广泛和较为常用的一种计算方法。
GRR指标的计算步骤如下:1. 进行测量。
grr指标 -回复
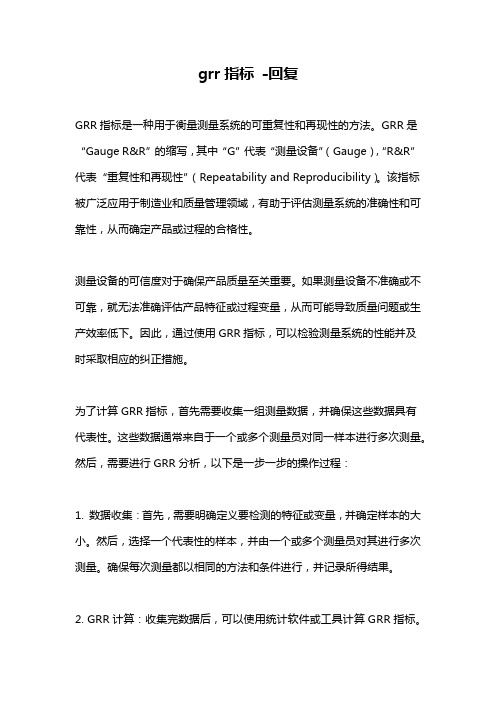
grr指标-回复GRR指标是一种用于衡量测量系统的可重复性和再现性的方法。
GRR是“Gauge R&R”的缩写,其中“G”代表“测量设备”(Gauge),“R&R”代表“重复性和再现性”(Repeatability and Reproducibility)。
该指标被广泛应用于制造业和质量管理领域,有助于评估测量系统的准确性和可靠性,从而确定产品或过程的合格性。
测量设备的可信度对于确保产品质量至关重要。
如果测量设备不准确或不可靠,就无法准确评估产品特征或过程变量,从而可能导致质量问题或生产效率低下。
因此,通过使用GRR指标,可以检验测量系统的性能并及时采取相应的纠正措施。
为了计算GRR指标,首先需要收集一组测量数据,并确保这些数据具有代表性。
这些数据通常来自于一个或多个测量员对同一样本进行多次测量。
然后,需要进行GRR分析,以下是一步一步的操作过程:1. 数据收集:首先,需要明确定义要检测的特征或变量,并确定样本的大小。
然后,选择一个代表性的样本,并由一个或多个测量员对其进行多次测量。
确保每次测量都以相同的方法和条件进行,并记录所得结果。
2. GRR计算:收集完数据后,可以使用统计软件或工具计算GRR指标。
最常用的是通过方差分析(ANOVA)方法来计算GRR指标。
3. 统计模型建立:使用一个统计模型来分解总变异为各个分量。
这包括部件内变异(Part-to-Part Variation)、重复性(Repeatability)和再现性(Reproducibility)。
- 部件内变异是由所测特征或变量本身引起的变异,是被测对象自身固有的变异。
- 重复性是由于测量员内部的变异引起的,即同一测量员在测量同一个样本时的变化。
- 再现性是由于不同测量员之间的变异引起的,即不同测量员在测量同一个样本时的差异。
4. GRR指标计算:通过统计模型,可以计算出每个分量的方差,并以百分比的形式表示GRR指标。
GRR讲解及分析公式
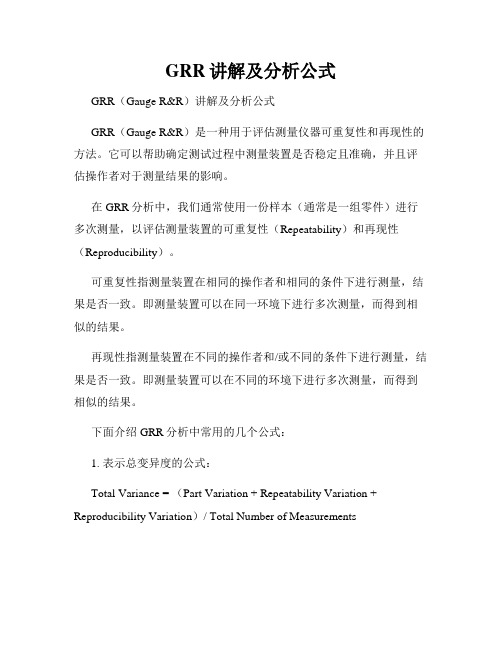
GRR讲解及分析公式GRR(Gauge R&R)讲解及分析公式GRR(Gauge R&R)是一种用于评估测量仪器可重复性和再现性的方法。
它可以帮助确定测试过程中测量装置是否稳定且准确,并且评估操作者对于测量结果的影响。
在GRR分析中,我们通常使用一份样本(通常是一组零件)进行多次测量,以评估测量装置的可重复性(Repeatability)和再现性(Reproducibility)。
可重复性指测量装置在相同的操作者和相同的条件下进行测量,结果是否一致。
即测量装置可以在同一环境下进行多次测量,而得到相似的结果。
再现性指测量装置在不同的操作者和/或不同的条件下进行测量,结果是否一致。
即测量装置可以在不同的环境下进行多次测量,而得到相似的结果。
下面介绍GRR分析中常用的几个公式:1. 表示总变异度的公式:Total Variance = (Part Variation + Repeatability Variation + Reproducibility Variation)/ Total Number of Measurements总变异度公式中,考虑了零件本身的变异度(Part Variation)、测量装置的可重复性变异度(Repeatability Variation)和测量装置的再现性变异度(Reproducibility Variation)。
2. 表示测量装置可重复性的公式:Repeatability = (Part Variation + Repeatability Variation) / Total Number of Measurements可重复性公式中,只考虑了零件本身的变异度和测量装置的可重复性变异度。
3. 表示测量装置再现性的公式:Reproducibility = Reproducibility Variation / Total Number of Measurements再现性公式中,只考虑了测量装置的再现性变异度。
GRR测量系统分析报告
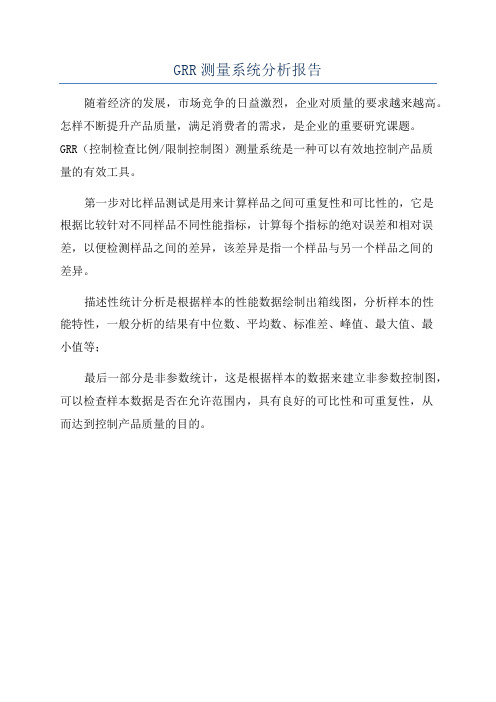
GRR测量系统分析报告
随着经济的发展,市场竞争的日益激烈,企业对质量的要求越来越高。
怎样不断提升产品质量,满足消费者的需求,是企业的重要研究课题。
GRR(控制检查比例/限制控制图)测量系统是一种可以有效地控制产品质
量的有效工具。
第一步对比样品测试是用来计算样品之间可重复性和可比性的,它是
根据比较针对不同样品不同性能指标,计算每个指标的绝对误差和相对误差,以便检测样品之间的差异,该差异是指一个样品与另一个样品之间的
差异。
描述性统计分析是根据样本的性能数据绘制出箱线图,分析样本的性
能特性,一般分析的结果有中位数、平均数、标准差、峰值、最大值、最
小值等;
最后一部分是非参数统计,这是根据样本的数据来建立非参数控制图,可以检查样本数据是否在允许范围内,具有良好的可比性和可重复性,从
而达到控制产品质量的目的。
品质GRR考核是什么意思

品质GRR考核是什么意思1. 引言品质GRR考核是一种质量管理中常用的评估方法。
GRR代表重复性与再现性(Gage Repeatability and Reproducibility),主要用于评估测量设备的可靠性和准确性。
通过GRR考核,我们可以了解测量设备是否能够在重复测量中产生一致结果,并且是否能够在不同操作员之间产生可比较的结果。
本文将详细介绍品质GRR考核的意义、目的和步骤。
2. 品质GRR考核的意义品质GRR考核对于产品质量管理至关重要。
以下是品质GRR考核的主要意义:•评估测量设备的可靠性:通过GRR考核,我们可以了解测量设备的重复性和再现性。
重复性是指同一操作员在相同条件下多次重复测量获得的结果的一致性,再现性是指不同操作员在相同条件下进行测量获得的结果的可比性。
如果测量设备有良好的重复性和再现性,那么其测量结果则可以可靠地用于产品质量控制。
•发现测量偏差:GRR考核可以帮助我们发现测量偏差。
测量偏差是指测量结果与真实值之间的差异。
通过GRR考核,我们可以评估测量设备的准确性,并根据偏差情况进行校正,以提高测量结果的准确性。
•优化测量设备:GRR考核结果可以提供优化测量设备的依据。
如果GRR考核结果较差,我们可以通过调整或更换测量设备,进一步提高测量结果的可靠性和准确性。
3. 品质GRR考核的目的品质GRR考核的主要目的是评估测量设备的可行性和准确性。
以下是品质GRR考核的具体目的:•评估重复性:通过GRR考核,我们可以评估同一测量设备在相同条件下多次重复测量的一致性。
这有助于判断测量设备是否稳定,以及是否能够产生可靠的测量结果。
•评估再现性:GRR考核还可以评估不同操作员在相同条件下使用相同测量设备时产生的结果的可比性。
这有助于判断测量设备是否可靠地产生一致的结果,无论是由谁来操作。
•评估测量偏差:GRR考核可以帮助我们评估测量设备的准确性,即测量结果与真实值之间的偏差。
通过评估测量偏差,我们可以发现并纠正测量设备的问题,以提高测量结果的准确性。
GR&R分析共有三种方法
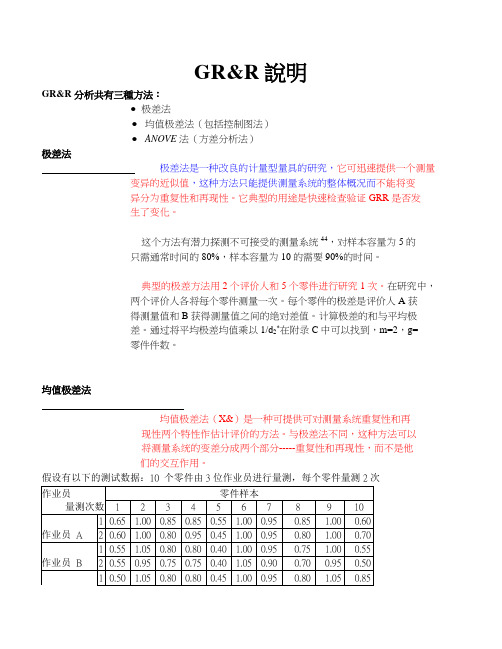
GR&R說明GR&R分析共有三種方法:●极差法●均值极差法(包括控制图法)●ANOVE法(方差分析法)极差法极差法是一种改良的计量型量具的研究,它可迅速提供一个测量变异的近似值,这种方法只能提供测量系统的整体概况而不能将变异分为重复性和再现性。
它典型的用途是快速检查验证GRR是否发生了变化。
这个方法有潜力探测不可接受的测量系统44,对样本容量为5的只需通常时间的80%,样本容量为10的需要90%的时间。
典型的极差方法用2个评价人和5个零件进行研究1次。
在研究中,两个评价人各将每个零件测量一次。
每个零件的极差是评价人A获得测量值和B获得测量值之间的绝对差值。
计算极差的和与平均极差。
通过将平均极差均值乘以1/d2*在附录C中可以找到,m=2,g=零件件数。
均值极差法均值极差法(X&)是一种可提供可对测量系统重复性和再现性两个特性作估计评价的方法。
与极差法不同,这种方法可以将测量系统的变差分成两个部分-----重复性和再现性,而不是他们的交互作用。
假设有以下的测试数据:10 个零件由3位作业员进行量测,每个零件量测2次计算步骤:(1) 计算出每个作业员对所有零件的量测平均值 (a x ,b x ,c x ) 以及量测全距平均值 (a R ,b R ,c R )(2) 计算零件平均值 (p x )、零件全距(R p ) 、总量测平均值(x )、总量测全距平均值(R )以及最大与最小量测平均值的差异(DIFF x )(3) 计算量测全距的上、下管制界线 (UCL R , LCL R )(4) 依上述数值计算出各变异值Repeatability - Equipment Variation 量具变异 EV = R * K 1 = 0.0383 * 4.566 = 0.1749K 1 = 5.15/d 2 = 4.566查附件二d 2 = 1.128 (当量测次数m = 2 , 零件数乘以作业员人数g = 10*3 = 30 )EV=5.15σe =5.15R / d 2σe =R / d 2(極差法計算標準差)σe 為量測過程中由於重復量測引起的標準差。
MSA-测量系统分析-GRR资料

内容
一、 测量系统的定义 二、 认识误差 三、 GR&R 四、 稳定性 五、 不确定度 六、 测量系统分析
1、什么是误差
由于测量系统的输出值用于做出关于产品和过程的决 策,所有变差源的累积影响通常为测量系统误差,或 有时称为“误差”。
测量系统误差可以分成五种类型:偏倚、重复性、再现性、稳 定性和线性。
3、如何“减小”误差?
- 多次测量取平均值 - 多人测量取平均值 - 使用更“高级”的测量设备 - 改进测量方法 - 使用辅助工具 - 向更高级别的标准看齐 - 培训 - 测量环境的要求 - 改进被测工件,方便测量
……
—避免失误 —“立场”问题
—设备的能力 —方法 —方法 —方法(规范) —人的能力 —环境因素 —被测对象
思考题 2
如果让你来评估本公司的一套测量系统(板测或终 测的自动测试),你会选择以下哪些指标:
A. FOR B. CpK C. NFF D. GRR
测量系统: 是用来对被测特性定量测量或定性评价的仪器或量具、
标准、操作、方法、夹具、软件、人员、环境和假设的 集合;用来获得测量结果的整个过程。
根据定义,一个测量过程可以看成是一个制造过程, 它产生数值(数据)作为输出。这样看待测量系统是有 用的,因为这可以使用权我们运用那些早已在统计过程 控制领域证明了有效性的所有概念、原理和工具。
√ 对于产品和过程条件,可能是评价人、环境(时间) 或方法的误差
√ 通常指AV(Appraiser Variant)- 评价人变差
√ 系统间(条件)变差
√ ASTM E456-96 包括重复性、实验室、环境及评价人 影响
2、重复性与再现性的差异
- 1、下载文档前请自行甄别文档内容的完整性,平台不提供额外的编辑、内容补充、找答案等附加服务。
- 2、"仅部分预览"的文档,不可在线预览部分如存在完整性等问题,可反馈申请退款(可完整预览的文档不适用该条件!)。
- 3、如文档侵犯您的权益,请联系客服反馈,我们会尽快为您处理(人工客服工作时间:9:00-18:30)。
NON-REPLICABLE GRR CASE STUDY David Benham, DaimlerChrysler Corporationwith input from the MSA workgroup:Peter Cvetkovski, Ford Motor CompanyMichael Down, General Motors CorporationGregory Gruska, The Third Generation, Inc.ABSTRACTGage studies provide an estimate of how much of the observed process variation is due to measurement system variation. This is typically done by a methodical procedure of measuring, then re-measuring the same parts by different appraisers. This cannot be done with a non-replicable (destructive) measurement system because the measurement procedure cannot be replicated on a given part after it has been destroyed. Here a method using ANOVA is used in a case study which demonstrates one possible way to determine measurement variation in a non-replicable system.This paper is intended to serve as additional guidelines for the analysis of measurement systems.Non-Replicable GRR Case StudyGage studies provide an estimate of how much of the observed processvariation is due to measurement system variation. This is typically doneby a methodical procedure of measuring, then re-measuring the sameparts by different appraisers. With a standard 10-3-31GRR, anassumption is made that between the trials and when handing offbetween appraisers, no physical change has occurred to the part. Allappraisers in the study have the opportunity to examine the same parts.The measurements can be replicated between appraisers and betweentrials. With most situations this is a safe assumption to make.In Figure 1, there are 10 parts to be measured, numbered from 1 to 10.As can be seen, the same part is measured by each appraiser and for eachtrial. A 10-2-3 format is shown for the sake of brevity and as a lead-in tothe case study which follows; randomization is not shown for the sake ofclarity.Appraiser 1 Appraiser 2Part # Trial 1 Trial 2 Trial 3 Trial 1 Trial 2 Trial 31 1 1 1 1 1 12 2 2 2 2 2 23 3 3 3 3 3 34 4 4 4 4 4 45 5 5 5 5 5 56 6 6 6 6 6 67 7 7 7 7 7 78 8 8 8 8 8 89 9 9 9 9 9 910 10 10 10 10 10 10Figure 1: Example of a Standard GRR Layout (10-2-3)However, there are certain instances where the measurements cannot bereplicated between trials or appraisers. The part is destroyed orsomehow physically changed when it is measured – that characteristiccannot be measured again. That measurement characteristic is said to benon-replicable. An example might be a destructive weld test where aweld nut is pushed off a part and the peak amount of pushout forcebefore destruction is measured; the weld is destroyed in the process, so itcannot be measured again. So, how can a measurement systems analysisbe conducted when the part is destroyed2 during its measurement?110 parts, 3 appraisers, 3 trials.2Not all non-replicable MSA studies necessarily involve destroying parts. In fact, the measurands need not be parts per se, either. For example, when tests are done on chemical processes, the chemical sample (“part”) used for testing may have been altered by the test itself and the solution it was drawn from may have been from a dynamic process where the solution is in constant motion – therefore it cannot be precisely re-sampled.Non-Replicable GRR Case StudyJune 5, 2002The first thing that must be done before tackling a non-replicable GRR study is to ensure that all the conditions surrounding the measurement testing atmosphere are defined, standardized and controlled – appraisers should be similarly qualified and trained, lighting should be adequate and consistently controlled, work instructions should be detailed and operationally defined, environmental conditions should be controlled to an adequate degree, equipment should be properly maintained and calibrated, failure modes understood, etc. Figure 2 in the MSA manual, Measurement System Variability Cause and Effect Diagram, p. 15, and the Suggested Elements for a Measurement System Development Checklist, pp. 36 – 38, may assist in this endeavor. Second, there is a good deal of prerequisite work that must be done before doing a non-replicable study. The production process must be stable and the nature of its variation understood to the extent that units may be appropriately sampled for the non-replicable study – where is the process homogeneous and where is it heterogeneous? Another consideration: if the overall process appears to be stable AND CAPABLE, and all the surrounding pre-requisites have been met, it may not make sense to spend the effort to do a non-replicable study since the overall capability includes measurement error – if the total product variation and location is OK, the measurement system may be considered acceptable. Standard GRR procedures and analysis methods must be changed and certain other assumptions must be made before conducting a non-replicable measurement systems analysis. The plan for sampling parts to be used in a non-replicable GRR needs some structure. Since the original part cannot be re-measured due to its destruction, other similar (homogeneous) parts must be chosen for the study (for the other trials and other appraisers) and an assumption must be made that they are “duplicate” or identical parts. In other words, as the “duplicate” parts are re-measured across other trials and by other appraisers, we will pretend that the same part is being measured. Refer to Figure 2. “Part 1” is now Part 1-1, 1-2, 1-3, 1-4, 1-5, 1-6, for this 10-2-3 layout. Six very similar, assumed to be identical, parts are used to represent Part 1, and so on for all 10 parts. The assumption must be made that all the parts sampled consecutively (within one batch) are identical enough that they can be treated as if they are the same. If the particular process of interest does not satisfy this assumption, this method will not work .Non-Replicable GRR Case StudyJune 5, 2002Appraiser 1 Appraiser 2Part # Trial 1 Trial 2 Trial 3 Trial 1 Trial 2 Trial 31A…1F 1-1 1-2 1-3 1-4 1-5 1-62A…2F 2-1 2-2 2-3 2-4 2-5 2-63A…3F 3-1 3-2 3-3 3-4 3-5 3-64A…4F 4-1 4-2 4-3 4-4 4-5 4-65A…5F 5-1 5-2 5-3 5-4 5-5 5-66A…6F 6-1 6-2 6-3 6-4 6-5 6-67A…7F 7-1 7-2 7-3 7-4 7-5 7-68A…8F 8-1 8-2 8-3 8-4 8-5 8-69A…9F 9-1 9-2 9-3 9-4 9-5 9-610A…10F 10-1 10-2 10-3 10-4 10-5 10-6Figure 2: Non-Replicable GRR Layout3Care must be taken in choosing these “duplicate” parts. Typically for theparts that represent part number 1 in a study, each “duplicate” is selectedin a way that it is as much alike the original part as possible. Likewisefor part number 2, and number 3, 4, 5, etc. These parts should beproduced under production conditions as similar as possible. Considerthe “5 M’s +E”4 and make them all as alike as possible. Generally, ifparts are taken from production in a consecutive manner, thisrequirement is met.However, the parts chosen to represent part number 2, for example, mustbe chosen to be unlike part number 1, part number 3, 4, 5, etc. Sobetween part numbers, the 5 M’s +E must be unlike each other. Thesedifferences must be forced to be between part numbers. The totalnumber of duplicate parts selected for each row must equal the numberof appraisers times the number of trials.5 In Figure 2, groups of partswithin each row are assumed to be identical, but groups of parts betweenrows are assumed to be different.Part variation may be expressed as part-to-part, shift-to-shift, day-to-day,lot-to-lot, batch-to-batch, week-to-week, etc. With parts the minimumvariation would be part-to-part – this represents the minimum possibleamount of time between each part. When parts are not sampledconsecutively (i.e., part-to-part), there is more opportunity for variationto occur – different production operators, different raw material, differentcomponents, changes in environment, etc.So, within a row it is desirable to minimize variation by taking partsconsecutively, thus representing part-to-part variation. Between rows itis desirable to maximize variation by taking parts from different lots,batches, etc. There may be economic, time or other constraints involvedwhich will impose limits on the length of time we can wait to take3The necessary randomized presentation to the appraiser is not shown here for the sake of clarity.4Man, Machine, Material, Method, Measurement plus Environment. Measurement may seem redundant here, but there may be times where two or more “identical” measurement systems are used to gain the same information and this should be considered in any study.5When the source of measurement variation is thought to be due to equipment only, using different appraisers may not be required.Non-Replicable GRR Case StudyJune 5, 2002samples for the between-row data – the process must be run, the PPAPmust be submitted, etc. When constraints arise and interfere with doingthings the “right” way, the results may be subject to modifiedinterpretation.Another statistical assumption that must be made for this type of study isthat the measurement error is normally distributed. This is a prerequisitefor any ANOVA (Analysis of Variance).ANOVA is a better analysis tool for a non-replicable measurementsystems analysis than the average and range method. ANOVA has thepower to examine interactions that the average range method will notcatch.As a precautionary note, the results from this type of study willcontain some process variation because the “identical” parts arenot really identical. This may come into play when interpreting theresults in terms of the error percentage related to process variationor tolerance. The better the methodology used to achieve anunderstanding of the production process and its correspondingmeasurement system, the more meaningful this non-replicablemeasurement study will be.It is critical in a non-replicable GRR study that the parts be clearlyidentified and saved after testing. If any issues arise after the standardanalysis, these parts may be needed for further exploration – e.g.,microscopic examination.A case study may serve to better illustrate this methodology.CASE STUDYA stamped part goes through a critical weld assembly process whichmust be destructively tested on an ongoing basis. The process has an in-house progressive stamping die which produces the steel stampings.This process is followed by robotic MIG welding (attaching an outsidepurchased steel rod to the stamping) in one of 6 different weld stationseach of which has 4 parallel weld fixtures (only one of which will beused per assembly). Each weld fixture is assigned a letter designation, Athrough X. This process has been in production long enough that it hasbeen studied and analyzed for stability and capability. Each of the 24weld stations is providing a stable and capable process, however someare better than others. In an effort to improve the overall process, themeasurement system would be analyzed using this non-replicable MSAmethodology which had been recently introduced to the supplier.This study used the 10-2-3 format – 10 parts, 2 appraisers, 3 – thus Study Formatrequiring 60 parts total for the study. Given the complexity of theprocess, it was felt that the 10-2-3 would be more manageable than a 10-3-3. Although there are 24 weld fixtures, only 10 of them were used forthis study and they were chosen using previously gathered data toNon-Replicable GRR Case StudyJune 5, 2002 represent the full range of the process. There was enough earlyconfidence in the measurement system to make this judgment.Similarity (homogeneity) within each row was created by taking 6consecutively produced stampings (6 is chosen to meet the 2 appraisers x3 trials requirement), then welding those 6 parts consecutively throughthe same weld fixture. Dissimilarity (heterogeneity) between rows wascreated by taking groups of 6 consecutive stampings from different coilsof steel at a time separated by a few hours, then running themconsecutively through a different weld fixture at a different time.The rod component which is welded to the stamping is received in bulkand has already been determined to not play a major role in pull testvariation. Therefore, in this study there was no effort made to maintainsimilarity and dissimilarity issues with the rod component.Previous studies using common problem solving tools had shown that amanual positioning and clamping system used on the testing machinewas appraiser dependent, so a new and better positioning system withhydraulic clamps was installed. Parts are located into the machine withpositive locators and hydraulic clamps. A hook on the testing machinegrabs the rod and mechanically pulls on the rod to destruction. A digitalreadout on the machine displays the peak pulloff force in pounds andreads to one decimal place. From this readout, the data is recorded andthe failure mode noted (weld must pull metal from the stamping).Although the appraiser dependency was assumed to be resolved, thisstudy still used two appraisers to verify that assumption.A total of 60 parts were required to do this study. There were 10 groupsof similar parts, 2 appraisers and 3 trials; 10 x 2 x 3 = 60. Parts werefirst gathered off the stamping operation, carefully numbered andquarantined until all 60 parts had been collected. These 10 groups ofparts were selected at 3 hour intervals, over 3 days of production, inorder to force some difference between each group of parts.Then, each similar stamped group of parts was run through a differentweld fixture. Parts introduced to each weld fixture were presented inrandom order within each group of 6.Appraiser 1 Appraiser 2Part # Trial 1 Trial 2 Trial 3 Trial 1 Trial 2 Trial 31-1R…1-6R 1-6R531-5R251-3R71-4R401-1R341-2R322-1P…2-6P 2-2P272-6P352-5P572-4P122-3P432-1P173-1H…3-6H 3-1H213-2H363-6H13-5H563-4H103-3H264-1G…4-6G 4-4G464-6G424-3G84-2G284-1G554-5G305-1E…5-6E 5-4E55-3E205-1E135-6E545-2E395-5E506-1F…6-6F 6-1F526-3F36-4F376-5F296-2F516-6F457-1M…7-6M 7-6M167-4M117-1M237-2M67-3M157-5M148-1O…8-6O 8-6O498-3O608-1O338-5O418-2O448-4O199-1Q…9-6Q 9-5Q319-6Q599-3Q249-2Q49-4Q99-1Q210-1T…10-6T 10-2T2210-5T1810-3T4710-4T5810-1T4810-6T38Figure 3: Layout Used for Case StudyNon-Replicable GRR Case StudyJune 5, 2002Referring to Figure 3, each row shows the “similar” parts. “1-1R” standsfor Stamping #1 of the first group of 6 stampings, which was run throughweld fixture R. “1-2R” stands for Stamping #2 of the first group ofstampings, which was run through weld fixture R. “10-1T” stands forStamping #1 of the tenth group of stampings, which was run throughweld fixture T.6The parts were numbered with the identification shown in Figure 3.Parts within each row were presented in a random order to both the weldassembly operation and to the weld test operation, each with a differentrandom order. The order shown above is for the weld test operation; theorder for the weld assembly operation within each row was a differentrandom order and is not shown here. Such randomization reduces thepossibility of any bias present in the order of manufacturing and/ortesting.Once all assembly was completed, the parts were presented to theappraisers for destructive testing and the data were recorded. Parts weresaved, preserving the original part numbers, in case any post-analysisneeded to be done.RESULTSThe data were put into a Minitab® “Gage R&R (nested)” routine whichgenerated a nested ANOVA. A nested (vs. crossed) ANOVA is requiredfor this type of study because all parts are not tested by (crossed with) allappraisers across all trials – they cannot be because they are destroyedafter one test. Each appraiser cannot be crossed with each part. Othercharts were also generated by Minitab.The first thing to look at is the Gage Run Chart.6For the sake of clarity here, the randomized order of total presentation to the appraisers running the test machine is shown as a subscript.Non-Replicable GRR Case StudyJune 5, 2002Figure 4: Gage Run Chart – Weld Tester, Rod PulloffThe Gage Run Chart in Figure 4 shows plotted points that representindividual measurement values made on each “part” by each appraiserduring each trial. The data is grouped by appraiser and presented in theorder in which the trials occurred. The horizontal dashed line representsthe overall mean of all the individual values displayed on the chart. Theabscissa shows each “part” number (a group of 6 parts in this case).This type of chart provides visual clues as to the presence of any patternsin the data. It is desirable that there be no particular pattern within“parts.” If undesirable patterns are present, then further, moresophisticated investigation may be required. It is also desirable that therebe differences shown between “parts”. If these differences do not occurthen the measurement system cannot distinguish between the parts usedin the study. Since there are no control limits or other statistical guidesdisplayed on this chart, some guesswork and common sense may berequired when reviewing it.In this particular study, there are no significant patterns within “parts”and there is some difference between “parts.”Next the summary of the GRR graphics is reviewed.Non-Replicable GRR Case StudyJune 5, 2002Figure 5: GRR Summary Graphics – GRR Study (Nested), Weld Tester, Rod PulloffA graphical summary, generated by Minitab, of this study is presented inFigure 5. Five graphs are shown and are numbered from 1 to 5.Presentation and analysis of these charts falls in line with a standardGRR.Graph No. 1 shows the components of variation. Four sources aredisplayed here• Gage R&R – is the variation due to the measurement system.• Repeatability – is the variation due to the measuring equipment.• Reproducibility – is the variation due to differences in the appraisers.• Part-to-Part – is the variation due to measurements taken acrossdifferent parts.Two bars are shown for each source of variation7:• % Contribution – is 100 times that variance component sourceσ).divided by the total variance (2• % Study Variation – is 100 times that study variation source dividedby the total study variation (σ).7It is possible in Minitab to also display “% Tolerance” and “% Process” but these options were not chosen here.Non-Replicable GRR Case StudyJune 5, 2002Graph No. 2 is a Range (R) Chart showing the range of the readings foreach appraiser on each “part.” Note that there is an upper control limit(UCL) and lower control limit (LCL) and that to be acceptable all pointsshould be within these limits. Out of control conditions means there issome sort of inconsistency occurring and should be investigated beforegoing further.The data in this case study is all within the control limits and isconsidered acceptable with respect to the Range Chart.Graph No. 3 is an Xbar (X) Chart showing the average of eachappraiser’s 3 readings on each “part.” Note that there is an upper controllimit (UCL) and lower control limit (LCL) and that to be acceptable,approximately 50% of the points should be outside these control limits.The distance between the control limits represents the band ofmeasurement system variation. If all points were within the controllimits it would mean that no significant distinction can be made betweenany of the parts in the study.In the data for this case study, 11 of 20 points (55%) are out of controland this is considered acceptable for the Xbar Chart.Graph No. 4 is a chart of the part-to-part variation across the study. Forthis particular study, Graph 4 does not add much value.Graph No. 5 shows the grand average for each appraiser as well aspoints representing the individual readings. The horizontal line betweenthese points indicates a visual reference for the difference in the grandaverage – the flatter this line the less difference there is between thesegrand averages.In the data for this case study, the reference line is quite flat indicatingvery little difference in the grand average for each appraiser.Graphs 4 and 5 may be used as clue generators for further analysis.Also, other graphics, such as histograms, may be used for more detailedanalysis.While reviewing the graphics it is a good idea to at the same time reviewthe ANOVA summary of the GRR data. Again, this summary is thesame as a standard ANOVA table and what would be generated by astandard GRR study.Gage R&R Study - Nested ANOVANested ANOVA TableSOURCE DF SS MS F PAppraiser 1 15636 15636 0.0202 0.88869Part(Appraiser) 18 13965615775868 16.7447 0 Repeatability 40 1853410 46335Total 5915834661Non-Replicable GRR Case StudyJune 5, 2002Gage R&RSOURCE VARCOMP % CONTRIBUTION(OF VARCOMP)Total Gage R&R 46335 16.00 Repeatability 46335 16.00 Reproducibility 0 0 Part-To-Part 243177 84.00 Total Variation 289513 100.00SOURCE STD DEVIATION(SD) STUDY VARIATION(6 * SD)% STUDY VARIATION(% SV)Total Gage R&R 215.256 1291.54 40.01Repeatability 215.256 1291.54 40.01Reproducibility 0 0 0Part-To-Part 493.130 2958.78 91.65Total Variation 538.064 3228.38 100.00Given all the above information one must make some decisions about themeasurement system – is it acceptable, is it useable, is more studyneeded, does an appraiser need better training, is customer approvalrequired for use, etc? For acceptability, generally the GRR% is reviewedfor suitability8.In this particular case study example the overall GRR% = 40.01% whichdoes not make for a clean interpretation. Is this acceptable given that thetraditional upper limit for even a marginally acceptable measurementsystem is 30%?Consider:1. The overall process to which the GRR% is compared is actuallyrepresented only by the parts (and weld fixtures) chosen for thisstudy. Only 10 of the 24 weld fixtures were included in thisstudy. Not all of the process is necessarily represented here. Ifthe process variation due to all the fixtures is much larger thanthat of the 10 selected for this study, then the measurementsystem may be acceptable based on MSA guidelines.2. The data in any non-replicable study such as this will necessarilyinclude SOME process variation. So some portion of the 40.01GRR% is actually process variation. It is impossible to separateall process variation from measurement system variation withthis scheme.3. The machine used to do the destruct pulloffs is a relativelysophisticated and expensive piece of equipment. How much 8ndc is also typically reviewed at this time, however for the sake of brevity it will not be shown here.Non-Replicable GRR Case StudyJune 5, 2002more could it be improved and would it be cost effective? Thesewould be questions for a possible cost study.Determining the acceptability of results of a non-replicable study Acceptability Issuesinvolves many other possible issues which go beyond the immediatesubject of this paper.• Production process stability: In order to properly analyze andinterpret a non-replicable measurement system study, the productionprocess must be statistically stable. Since the measurement systemstability is contained within the production process data, if theoverall data demonstrates stability, then the measurement system istheoretically stable. Data collection frequency and sample size mustbe balanced between Average Run Length (ARL)9 and cost ofinspection.• Production process capability: If the production process ismarginally capable, there is not much breathing room between itsnatural process limit(s) and the measurement system’s “gray area”(see discussion in Chapter III, Section C, of MSA-3). Acceptabilitylimits (for the process data) might be used when the non-replicablemeasurement system analysis shows marginal or questionableacceptability. These acceptability limits would be calculated byσ, then adding that valueusing a 3 or 4 sigma multiplier10 of theGRRto the lower specification limit and subtracting it from the upperspecification limit. However, for this to work with any degree ofconfidence, there must be adequate breathing room between theproduction process and these limits. In the example provided, wherethere is only a lower specification limit, the resultant value fromusing this multiplier would be added to the lower specification limitonly; for a process with only an upper specification limit, theresultant value from using the multiplier would be subtracted fromthe upper specification limit only.• Long-term measurement system stability: Some effort should bemade to study the measurement system’s stability on a long-termbasis using techniques similar to what has been described above.These techniques may be scaled down to “mini” studies and used onan ongoing basis.• Ironically, the better the process Cp11, the more difficult it may be toestablish acceptable non-replicable measurement system analysisresults using the methods here. Recall that a major requirement forthis method to work successfully is that one can knowingly producesimilar (homogeneous) parts (to be used within “parts” and operatoracross trials) and dissimilar (heterogeneous) parts (to be usedbetween “parts” and operators). If the production process has a very9The average run length (ARL) at a given quality level is the average number of samples (subgroups) taken before an action signal is given.10This multiplier value would be chosen depending on the amount of risk one chooses to accept.11Technically a process with unilateral tolerance (such as demonstrated in the case study here) has no Cp. What is meant here is a process with a relatively small amount of variation.Non-Replicable GRR Case StudyJune 5, 2002tight Cp, it may not be possible to meet this requirement. However,if such a process were to demonstrate stability and a high Cpk, thenthe measurement system is probably acceptable (provided there areno bias or linearity issues) because the data that generated this highCpk would include measurement variation.• Bias and linearity are not evaluated by this method. As with anyGRR study, only repeatability and reproducibility are considered.The measurement equipment calibration plan becomes critical to theoverall acceptability of the non-replicable measurement system.As can be seen, a non-replicable measurement study may raise as many Summaryquestions as it resolves. Due to the relative sophistication and expense ofthe measurement equipment used in this non-replicable measurementprocess, and due to the factors mentioned above, it was decided that thismeasurement process was suitable for gaging continuous improvement ofthe manufacturing process. At the same time, opportunities forimproving the measurement system would also be evaluated in thefuture.This case study is a single event and therefore has been termed a non-replicable GRR study. The bigger picture with any measurement systemis the long-term, cradle-to-grave concept. The study here may be used toinitially qualify a system, but more work is required to control thatmeasurement system over time to ensure its stability and usefulness inmaking the appropriate process control and/or capability decisions andcontinuous improvement. To keep tabs on this measurement process overtime, a control chart could be used to record the results of special,consecutive samples taken periodically and those results used todetermine the system’s stability.Finally, the methodology above was what was chosen for this particularsituation. There are other methods which will serve to determine non-replicable measurement error and each must be carefully selected to suitthe particular situation. The methods shown here merely illustrate asingle approach for a single situation and hopefully it will spur the readerto consider developing an approach for their own non-replicablemeasurement error situation._____________________________________________________________________________________________Dave Benham works at DaimlerChrysler Corporation in Auburn Hills, Michigan, as a Senior Consultant in Supplier Development. He has a B.S. in Psychology and M.A. in Education from Michigan State University. Dave is an ASQ Certified Quality Engineer and Certified Reliability Engineer. He has worked in the automotive industry in the field of quality for 25 years.。