基于数据挖掘技术的学生成绩分析系统之欧阳家百创编
基于数据挖掘技术的成绩分析系统研究
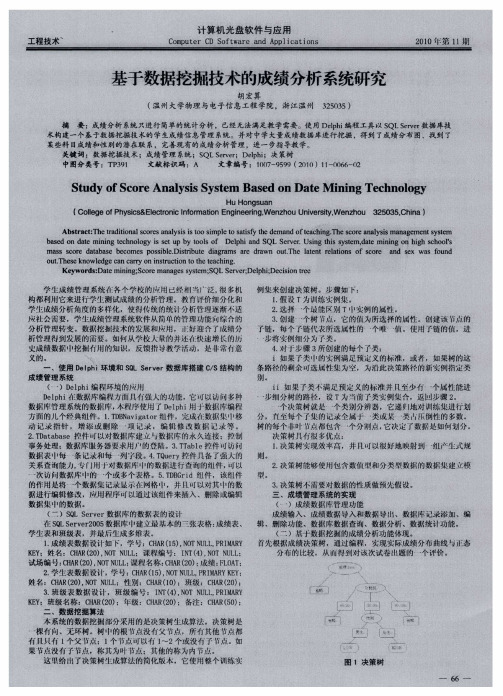
一
例 来 创建 决策树 。步骤如 : I假 设 T 训练实 例 。 _ 为 2 选拌 个蛙 能 别 T・ 例n 属性 。 . I t 勺 3 创建 ,个 树 1点 ,它的 值为所 选样 的属 性 。创建 该 1点的 . ‘ y 了链 ,每个 了链代 表 所选 属性 的 ’ 性 值 。使用 了链 的值 ,进 个I 步将 实例 分 为 了类 。 4 对十 步骤 3 . 所创 建 的每 个 了类 : i如果 了类 ・ 的实例 满足 预定 义的标 准 ,或 .如 果树 的这 l -
ma s c r d tbae e o e os il. srbue dig a a e rw n s s o e aa s b c m s p sbeDiti t a r ms r d a ou. e ae t eains of s o e a d e wa f u d t Th ltn rlto c r n s x s on o tT e ek owld ec n ar o i tu to ot e c n . u . s n h e g a c ry n r c int ta hig ns he
Ab ta tTh a iina c r sa ayssi o i et aif hede a dof e c i gTh c r n l i n g me ts tm s r c : et dto ls o e n l i st osmpl s tsy t m n ta h n . e s oea ayssma a e n yse r o
Ke wod : t nn ;c r n g ssse S evrDep i cso e y r sDaemiigS oema a e ytm;QLS re; lh; i nt e De i r
物联网考试答案之欧阳体创编
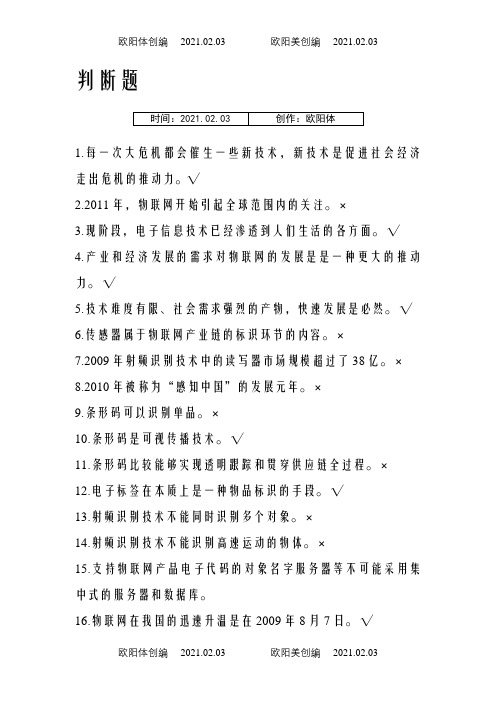
判断题1.每一次大危机都会催生一些新技术,新技术是促进社会经济走出危机的推动力。
√2.2011年,物联网开始引起全球范围内的关注。
×3.现阶段,电子信息技术已经渗透到人们生活的各方面。
√4.产业和经济发展的需求对物联网的发展是是一种更大的推动力。
√5.技术难度有限、社会需求强烈的产物,快速发展是必然。
√6.传感器属于物联网产业链的标识环节的内容。
×7.2009年射频识别技术中的读写器市场规模超过了38亿。
×8.2010年被称为“感知中国”的发展元年。
×9.条形码可以识别单品。
×10.条形码是可视传播技术。
√11.条形码比较能够实现透明跟踪和贯穿供应链全过程。
×12.电子标签在本质上是一种物品标识的手段。
√13.射频识别技术不能同时识别多个对象。
×14.射频识别技术不能识别高速运动的物体。
×15.支持物联网产品电子代码的对象名字服务器等不可能采用集中式的服务器和数据库。
16.物联网在我国的迅速升温是在2009年8月7日。
√17.物联网提出已逾10年,业已形成统一明晰的定义。
×18.物联网是独立于互联网的存在。
×19.物联网是在互联网基础上的延伸和拓展。
√20.物联网中的“物”可在网络中共享被识别的编号。
√21.自动化不属于物联网的基本特征。
×22.人物相联、物物相联是物联网的基本要求之一。
√23.RFID是一种目前比较先进的接触式识别技术。
×24.电子标签具有各种形状,且任意形状都能满足阅读距离的要求。
×25.无源标签的工作电源完全有其内部电池供给。
×26.微波电子标签的工作频段在125KHz-134KHz之间。
×27.超高频电子标签的工作原理一般是电磁耦合。
×28.无源电子标签的作用距离一般是3-10m。
×29.超高频UHF以上频段的系统一般采用无源标签。
基于数据挖掘技术的中学成绩分析系统研究

基于数据挖掘技术的中学成绩分析系统研究
郝尚富;王志辉
【期刊名称】《电脑知识与技术》
【年(卷),期】2010(006)029
【摘要】现有的中学教学管理软件中,很少涉及深层次的成绩分析,不利于充分挖掘这些数据所隐含的信息,无法进行科学的教学评价.利用数据挖掘技术可以精确地展现学生成绩分布的多个方面,找出影响成绩变化的规律性因素.系统采用标准分数作为数学模型,选用SOA架构标准模范,以JAVAJSP为开发语言,研发出一套科学、高效的成绩分析系统,为学校教务部门提供决策支持信息,促使教学工作更好地开展,最终提高教学质量.
【总页数】2页(P8164-8165)
【作者】郝尚富;王志辉
【作者单位】河北北方学院,信息科学与工程学院,河北,张家口,075000;河北北方学院,信息科学与工程学院,河北,张家口,075000
【正文语种】中文
【中图分类】TP311
【相关文献】
1.基于数据挖掘技术的中职学校成绩分析应用 [J], 袁炜文
2.基于数据挖掘技术的成绩分析系统研究 [J], 胡宏算
3.基于数据挖掘技术的学生成绩分析 [J], 狄晓娇
4.基于数据挖掘技术的大学生英语四级考试成绩分析方法探讨 [J], 梁玮
5.数据挖掘技术在教学管理中的应用——以对某中学高中文科综合考试成绩进行聚类分析为例 [J], 吕岚;林玉连
因版权原因,仅展示原文概要,查看原文内容请购买。
基于数据挖掘技术的学生成绩分析
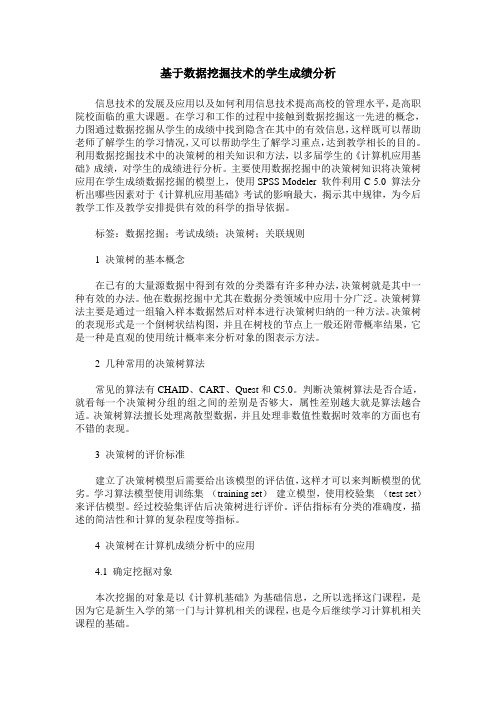
基于数据挖掘技术的学生成绩分析信息技术的发展及应用以及如何利用信息技术提高高校的管理水平,是高职院校面临的重大课题。
在学习和工作的过程中接触到数据挖掘这一先进的概念,力图通过数据挖掘从学生的成绩中找到隐含在其中的有效信息,这样既可以帮助老师了解学生的学习情况,又可以帮助学生了解学习重点,达到教学相长的目的。
利用数据挖掘技术中的决策树的相关知识和方法,以多届学生的《计算机应用基础》成绩,对学生的成绩进行分析。
主要使用数据挖掘中的决策树知识将决策树应用在学生成绩数据挖掘的模型上,使用SPSS Modeler 软件利用C 5.0 算法分析出哪些因素对于《计算机应用基础》考试的影响最大,揭示其中规律,为今后教学工作及教学安排提供有效的科学的指导依据。
标签:数据挖掘;考试成绩;决策树;关联规则1 决策树的基本概念在已有的大量源数据中得到有效的分类器有许多种办法,决策树就是其中一种有效的办法。
他在数据挖掘中尤其在数据分类领域中应用十分广泛。
决策树算法主要是通过一组输入样本数据然后对样本进行决策树归纳的一种方法。
决策树的表现形式是一个倒树状结构图,并且在树枝的节点上一般还附带概率结果,它是一种是直观的使用统计概率来分析对象的图表示方法。
2 几种常用的决策树算法常见的算法有CHAID、CART、Quest和C5.0。
判断决策树算法是否合适,就看每一个决策树分组的组之间的差别是否够大,属性差别越大就是算法越合适。
决策树算法擅长处理离散型数据,并且处理非数值性数据时效率的方面也有不错的表现。
3 决策树的评价标准建立了决策树模型后需要给出该模型的评估值,这样才可以来判断模型的优劣。
学习算法模型使用训练集(training set)建立模型,使用校验集(test set)来评估模型。
经过校验集评估后决策树进行评价。
评估指标有分类的准确度,描述的简洁性和计算的复杂程度等指标。
4 决策树在计算机成绩分析中的应用4.1 确定挖掘对象本次挖掘的对象是以《计算机基础》为基础信息,之所以选择这门课程,是因为它是新生入学的第一门与计算机相关的课程,也是今后继续学习计算机相关课程的基础。
教育数据挖掘技术在学生成绩分析中的应用研究
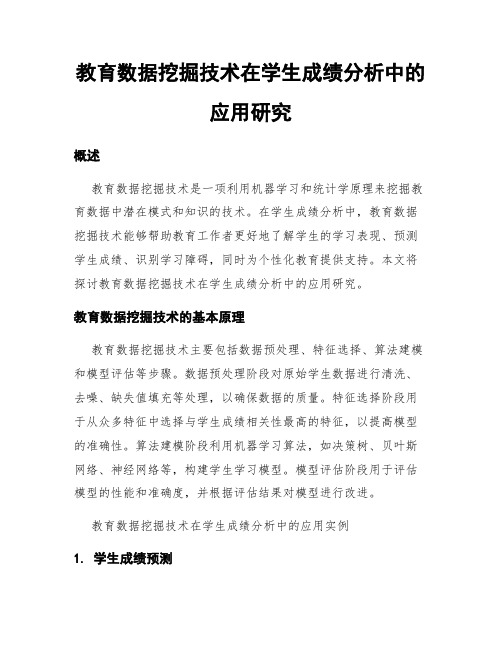
教育数据挖掘技术在学生成绩分析中的应用研究概述教育数据挖掘技术是一项利用机器学习和统计学原理来挖掘教育数据中潜在模式和知识的技术。
在学生成绩分析中,教育数据挖掘技术能够帮助教育工作者更好地了解学生的学习表现、预测学生成绩、识别学习障碍,同时为个性化教育提供支持。
本文将探讨教育数据挖掘技术在学生成绩分析中的应用研究。
教育数据挖掘技术的基本原理教育数据挖掘技术主要包括数据预处理、特征选择、算法建模和模型评估等步骤。
数据预处理阶段对原始学生数据进行清洗、去噪、缺失值填充等处理,以确保数据的质量。
特征选择阶段用于从众多特征中选择与学生成绩相关性最高的特征,以提高模型的准确性。
算法建模阶段利用机器学习算法,如决策树、贝叶斯网络、神经网络等,构建学生学习模型。
模型评估阶段用于评估模型的性能和准确度,并根据评估结果对模型进行改进。
教育数据挖掘技术在学生成绩分析中的应用实例1. 学生成绩预测通过教育数据挖掘技术,可以根据学生的历史学习数据,预测其未来的学习成绩。
例如,可以利用学生的课堂表现、作业提交情况、课程参与度等数据,结合机器学习算法,建立学生学习模型来预测他们的未来成绩。
这种预测模型可以帮助教师及时发现学习较为薄弱的学生,及早采取干预措施,提高学生的学习效果和成绩。
2. 学习行为分析教育数据挖掘技术可以通过分析学习过程中学生的行为数据,了解学生的学习行为特征和学习策略。
例如,可以分析学生学习时长、课程参与度、访问频率等指标,进而评估学生的学习态度和学习动力。
这样的分析结果对于教师来说,可以提供有针对性的指导建议,帮助学生改进学习策略,提高学习效果。
3. 学习障碍识别教育数据挖掘技术还可以通过挖掘学生学习数据中的潜在模式,识别学生的学习障碍。
例如,可以根据学生的作业得分、测试成绩等数据,利用机器学习算法,建立学生学习挑战的模型。
这样的模型可以帮助教师及时发现学生的学习障碍,针对性地提供帮助和支持,促进学生成绩的提升。
基于数据挖掘的学生评价系统设计与实现

基于数据挖掘的学生评价系统设计与实现随着信息技术的普及和发展,数据挖掘技术正逐渐应用到学生评价系统中,为学生提供更加全面、科学的评价服务。
在本文中,我们将探讨基于数据挖掘的学生评价系统的设计与实现。
一、数据挖掘技术在学生评价系统中的应用数据挖掘技术是一种可以从大量数据中自动提取出有用信息的技术。
在学生评价系统中,可以应用数据挖掘技术对学生的课堂表现、作业完成情况、考试得分等信息进行分析和挖掘。
这种分析和挖掘不仅可以提供给学生科学的评价结果,还可以为老师提供科学的教学反馈和指导。
二、基于数据挖掘的学生评价系统设计方案1. 数据采集:首先需要采集学生的各种数据,包括学生基本信息、课堂表现、作业完成情况、考试得分等。
这些数据可以通过各个系统自动采集,也可以由学生或老师手动输入。
2. 数据预处理:采集到的数据需要进行预处理,包括数据清洗、数据过滤、数据转换等。
这样可以保证数据的准确性和真实性,避免造成错误的评价结果。
3. 数据分析:利用数据挖掘技术对采集到的数据进行分析和挖掘,得出学生的评价结果。
分析和挖掘的方法包括聚类分析、分类分析、关联规则分析等。
4. 评价结果展示:通过可视化的方式将学生的评价结果展示出来,方便学生和老师查看和理解。
展示方式可以包括柱状图、折线图、雷达图等等。
5. 反馈和指导:除了展示评价结果外,还可以为学生和老师提供相应的反馈和指导。
例如,针对学生的评价结果,可以推荐相应的学科课程;对于老师的教学反馈,可以提供相应的教学改进建议。
三、基于数据挖掘的学生评价系统的实现基于数据挖掘的学生评价系统的实现需要依赖于相关的技术和工具。
以下是关键技术和工具的说明:1. 数据库技术:学生评价系统需要用到数据库技术来存储和管理采集到的数据。
例如,可以使用MySQL、Oracle等关系型数据库。
2. 数据挖掘工具:对采集到的数据进行分析和挖掘需要使用相应的数据挖掘工具。
例如,可以使用Weka、RapidMiner等数据挖掘软件。
数据挖掘技术在学生成绩预测中的应用研究
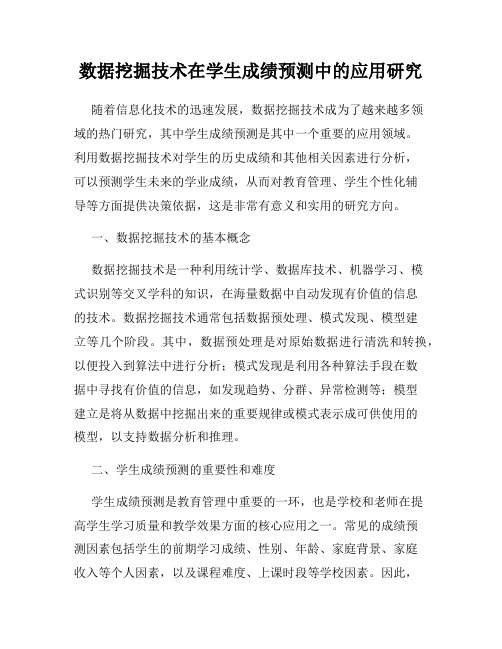
数据挖掘技术在学生成绩预测中的应用研究随着信息化技术的迅速发展,数据挖掘技术成为了越来越多领域的热门研究,其中学生成绩预测是其中一个重要的应用领域。
利用数据挖掘技术对学生的历史成绩和其他相关因素进行分析,可以预测学生未来的学业成绩,从而对教育管理、学生个性化辅导等方面提供决策依据,这是非常有意义和实用的研究方向。
一、数据挖掘技术的基本概念数据挖掘技术是一种利用统计学、数据库技术、机器学习、模式识别等交叉学科的知识,在海量数据中自动发现有价值的信息的技术。
数据挖掘技术通常包括数据预处理、模式发现、模型建立等几个阶段。
其中,数据预处理是对原始数据进行清洗和转换,以便投入到算法中进行分析;模式发现是利用各种算法手段在数据中寻找有价值的信息,如发现趋势、分群、异常检测等;模型建立是将从数据中挖掘出来的重要规律或模式表示成可供使用的模型,以支持数据分析和推理。
二、学生成绩预测的重要性和难度学生成绩预测是教育管理中重要的一环,也是学校和老师在提高学生学习质量和教学效果方面的核心应用之一。
常见的成绩预测因素包括学生的前期学习成绩、性别、年龄、家庭背景、家庭收入等个人因素,以及课程难度、上课时段等学校因素。
因此,在成绩预测中,需要对海量、复杂的学生和课程数据进行处理和分析,从而得出准确的预测结果。
然而,学生学习过程和成绩受到众多因素的影响,既包括实际学习因素如知识掌握程度、学业热情等,也包括非学习因素如兴趣爱好、家庭背景、心理状态等。
这些复杂因素之间相互作用,增加了成绩预测的难度。
三、数据挖掘技术在学生成绩预测中的应用1.数据预处理在成绩预测中,数据预处理是非常重要的,它往往可以决定模型建立的质量。
数据挖掘技术通常包括数据清理、数据集成、数据转换和数据归约等几个方面的处理。
首先,进行数据清理,包括去重、筛选、填充空值等操作,以保证数据完整性和可靠性。
然后,进行数据集成,将来自不同数据源的数据集成为一个整体数据集。
数据挖掘技术在高校学生成绩分析中的应用
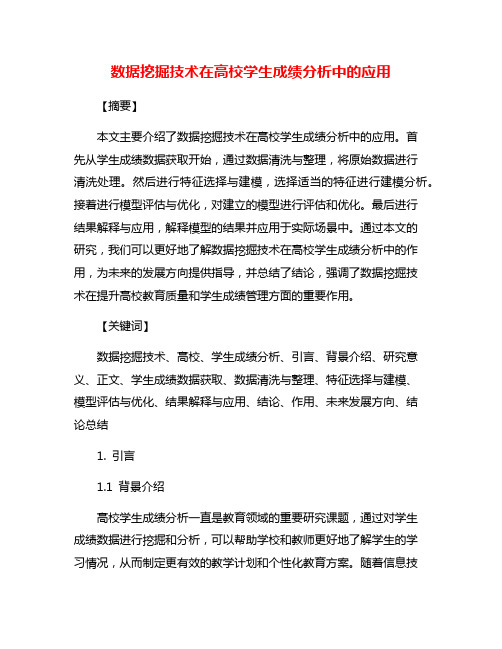
数据挖掘技术在高校学生成绩分析中的应用【摘要】本文主要介绍了数据挖掘技术在高校学生成绩分析中的应用。
首先从学生成绩数据获取开始,通过数据清洗与整理,将原始数据进行清洗处理。
然后进行特征选择与建模,选择适当的特征进行建模分析。
接着进行模型评估与优化,对建立的模型进行评估和优化。
最后进行结果解释与应用,解释模型的结果并应用于实际场景中。
通过本文的研究,我们可以更好地了解数据挖掘技术在高校学生成绩分析中的作用,为未来的发展方向提供指导,并总结了结论,强调了数据挖掘技术在提升高校教育质量和学生成绩管理方面的重要作用。
【关键词】数据挖掘技术、高校、学生成绩分析、引言、背景介绍、研究意义、正文、学生成绩数据获取、数据清洗与整理、特征选择与建模、模型评估与优化、结果解释与应用、结论、作用、未来发展方向、结论总结1. 引言1.1 背景介绍高校学生成绩分析一直是教育领域的重要研究课题,通过对学生成绩数据进行挖掘和分析,可以帮助学校和教师更好地了解学生的学习情况,从而制定更有效的教学计划和个性化教育方案。
随着信息技术的不断发展和普及,数据挖掘技术在高校学生成绩分析中的应用也变得日益重要。
在传统的学生成绩分析中,往往只能利用少量的简单统计方法来对学生成绩进行评估和分析,无法深入挖掘和发现隐藏在数据中的规律和规律。
而数据挖掘技术通过利用大数据处理和分析的方法,可以对学生成绩数据进行全面深入的挖掘,发现不同学生之间的学习特点和规律,为教育工作者提供更多的参考和决策依据。
借助数据挖掘技术进行高校学生成绩分析具有非常重要的意义,能够为学校和教师提供更加全面准确的学生学习情况信息,帮助他们更好地制定教学方案和教育政策,促进学生成绩的提升和个性化教育的实施。
1.2 研究意义高校学生成绩一直是教育领域的重要研究课题,通过对学生成绩进行分析可以帮助学校更好地了解学生的学习情况,发现问题并制定合理的解决方案。
数据挖掘技术的应用为高校学生成绩分析提供了新的思路和方法。
基于数据挖掘的学生成绩分析与预测研究
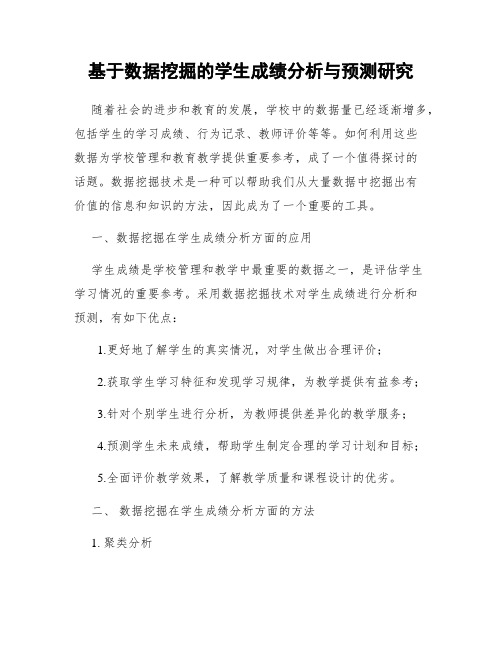
基于数据挖掘的学生成绩分析与预测研究随着社会的进步和教育的发展,学校中的数据量已经逐渐增多,包括学生的学习成绩、行为记录、教师评价等等。
如何利用这些数据为学校管理和教育教学提供重要参考,成了一个值得探讨的话题。
数据挖掘技术是一种可以帮助我们从大量数据中挖掘出有价值的信息和知识的方法,因此成为了一个重要的工具。
一、数据挖掘在学生成绩分析方面的应用学生成绩是学校管理和教学中最重要的数据之一,是评估学生学习情况的重要参考。
采用数据挖掘技术对学生成绩进行分析和预测,有如下优点:1.更好地了解学生的真实情况,对学生做出合理评价;2.获取学生学习特征和发现学习规律,为教学提供有益参考;3.针对个别学生进行分析,为教师提供差异化的教学服务;4.预测学生未来成绩,帮助学生制定合理的学习计划和目标;5.全面评价教学效果,了解教学质量和课程设计的优劣。
二、数据挖掘在学生成绩分析方面的方法1. 聚类分析聚类分析是一种对数据进行分类和分组的方法,通过找到不同数据的相似之处,将它们分组,进而了解这些数据的共性和特征。
在学生成绩分析中,聚类分析可以根据学生的成绩、性别、专业等特征,将学生分成不同的群组,从而指导教师制定分组教学方案。
2. 关联分析关联分析可以找到数据之间的关联关系,比如学生的物理成绩和数学成绩之间的关系。
在学生的学习中,不同科目之间存在着一定的关联关系,理解这些关系可以帮助教师更好地设计科目课程,提高学生学习效率。
3. 分类预测分类预测是利用已有的数据,通过挖掘有用信息,对新数据进行分类预测的技术。
在学生成绩方面,可以根据历史数据预测学生未来的成绩,为教学管理提供有益的参考和指导。
三、学生成绩分析与预测的案例研究为了更好地了解数据挖掘在学生成绩分析与预测方面的应用,以下列举一些案例研究的实例:1. 基于学生成绩的聚类分析通过对学生的成绩数据进行聚类分析,可以将学生分成不同的群组,方便教师进行个性化、差异化的教学。
基于数据挖掘技术的学生成绩预测研究

基于数据挖掘技术的学生成绩预测研究随着教育信息化的不断发展,学校大量的学生学习信息、考试成绩等数据将产生。
借助数据挖掘技术对这些数据进行分析和利用,可以为学校提供科学的帮助和决策支持,特别是对学生成绩预测的研究。
一、学生成绩预测的意义学生成绩预测是指通过定量方法对学生的学习情况进行预测,包括预测学生将获得的考试成绩。
对于教育机构来说,学生成绩预测是一项具有重要意义的工作。
首先,它可以帮助教育机构及时了解学生的学习情况,指导学校在教学方面做进一步调整和优化;其次,对个别学生来说,学生成绩预测还可以预警可能存在的问题,使得学生及时采取措施做好学习计划。
二、数据挖掘技术在学生成绩预测中的应用数据挖掘是一种从大量数据中提取有用信息和知识的技术。
在学生成绩预测领域,数据挖掘技术可以针对学生的历史学习数据及其他相关数据,进行复杂计算和分析,提取出学生成绩与其他因素之间的关系和规律。
常用的数据挖掘技术包括分类、聚类、关联规则学习和序列挖掘。
其中,分类技术是指根据学生成绩与其他因素的关系,将学生分为不同的类别,以判断学生今后会属于哪种类别;聚类技术是指根据学生成绩和其他因素的共同特征,将学生分为不同的组,以了解学生的差异;关联规则学习是指根据学生成绩和其他因素之间的联系,找出其中的因果关系;序列挖掘则是指按照时间顺序找出学生成绩和其他因素的演变规律。
三、学生成绩预测的方法学生成绩预测方法包括传统的回归分析,以及基于数据挖掘技术的预测方法。
1. 传统回归预测方法传统回归分析是一种建立数学模型的方法,通过回归方程来预测学生的考试成绩。
它的具体操作方法是,先确定参考因素,然后将参考因素与学生成绩进行回归计算,得出平均绩点预测值。
传统回归分析的优点是易于操作,模型可解释性好,但缺点也非常明显:回归模型需要人为选定参考因素和回归方程,过度依赖人的贡献。
此外,回归模型对数据的要求很高,对数据的异常值比较敏感,容易导致预测结果不准确。
基于数据挖掘的学生成绩查询系统

基于数据挖掘的学生成绩查询系统作者:何云峰来源:《电脑知识与技术》2013年第01期摘要:开发基于的学生成绩挖掘系统。
一方面使学校教学资源得到优化,减轻教师对于学生成绩查询的工作量。
另一方面,通过数据挖掘技术进行系统开发,使大量的数据能得到有效利用。
挖掘出的隐藏规则对学校各方面工作的指导以及学生的培养有着重要意义。
关键词:学生成绩查询;系统设计;数据挖掘中图分类号:TP311 文献标识码:A 文章编号:1009-3044(2013)01-0017-03信息社会的高科技,商品经济化的高效益,使计算机的应用已普及到经济和社会生活的各个领域。
为了满足现今社会人们的时间观念,成绩查询系统软件成为教学办公的一项重要工具。
该软件的功能在系统内部有源代码直接完成。
通过操作手册,使用者可以了解本软件的基本工作原理。
操作人员只需输入一些简单的汉字、数字,即可达到自己的目标[1]。
1 系统现状与解决方案目前该系统主要是完成3类用户的需求。
学生:对各科成绩的查询及查看本班成绩排名等情况。
任课老师:输入并修改所教科目的学生成绩,能够计算成绩排名,输出成绩及排名情况;其中班主任则拥有更多的权利,如输入并维护本班的基本信息,对本班的各科成绩汇总,计算各科成绩的总分,排名,平均分等需求;并可输出上述结果。
教务处(管理员):学校全体成员的信息管理,对考试科目,时间及对所考科目的编号等进行具体的管理,并对任课老师,班主任等输入的信息进行存库,对学生的信息进增加、删除、修改等,可打印学生的所有信息,可添加用户等权限。
本研究应用框架,采用流行的三层架构开发数据挖掘系统。
技术是典型基于B/S结构开发模式的技术,它提供了为建立和部署企业级 Web 应用程序所必需的服务。
可以使用.NET平台快速方便的部署三层架构[2]。
最具革命性的变化在于制作网页中使用了基于事件的处理,可以指定处理的后台代码文件。
.NET中可以方便的实现组件的装配,后台代码通过命名控件可以方便的使用自己定义的组件。
基于数据挖掘的联大学生成绩查询系统的设计与实现的开题报告

基于数据挖掘的联大学生成绩查询系统的设计与实现的开题报告一、项目背景随着高校教育的普及,人们的需求越来越多地聚焦于学生成绩的查询与分析。
传统的学生成绩查询系统存在很多的限制,例如仅能查询到某个学期的成绩,不能进行多种条件的筛选等等,导致学生和教师的查询效率低下,不利于教学和管理的全面推进。
针对这些问题,本项目旨在基于数据挖掘技术,构建一个联大学生成绩查询系统,使查询更加方便、快捷和多样化。
二、项目目的本项目的主要目的,是建立并实现一个基于数据挖掘的联大学生成绩查询系统,该系统具有较高的查询效率和准确性,同时,也能够对学生的历史成绩进行分析和预测,为学生制定更明智的学习计划以及教师更好地开展教学提供数据支持。
三、项目任务(1)数据库设计:设计学生信息、课程信息、成绩信息等关键数据表,确保数据表结构合理,且满足系统需求;(2)系统架构规划:明确系统整体框架,包括后台数据库和前端用户界面的设计与实现;(3)系统功能实现:①查询功能:实现基本的成绩信息查询,包括按课程名称、学生ID、成绩等多种条件查询方式;②统计分析功能:利用数据挖掘技术对历史成绩数据进行分析与挖掘,提供学生学习情况的横向和纵向比较等功能;③成绩预测功能:基于历史数据,通过数据挖掘技术构建预测模型,对学生目前的学习情况进行分析和预测,帮助学生制定更好的学习计划;(4)系统测试:对系统进行充分的测试和调试,确保系统的稳定性和性能足够好,同时也结合实际使用体验进行优化。
四、项目技术和工具本项目主要采用以下技术和工具:(1)MySQL数据库:作为后台数据库用于存储学生、课程和成绩等关键数据信息;(2)Python语言:作为数据挖掘和后端服务器开发的主要语言;(3)Scikit-Learn:Python常用的机器学习框架,用于实现数据挖掘和成绩预测功能;(4)Flask框架:Python的Web后端开发框架,用于实现查询和分析功能;(5)HTML/CSS/JavaScript:用于实现前端UI界面的设计和构建。
基于数据挖掘技术的学生成绩系统的设计与实现
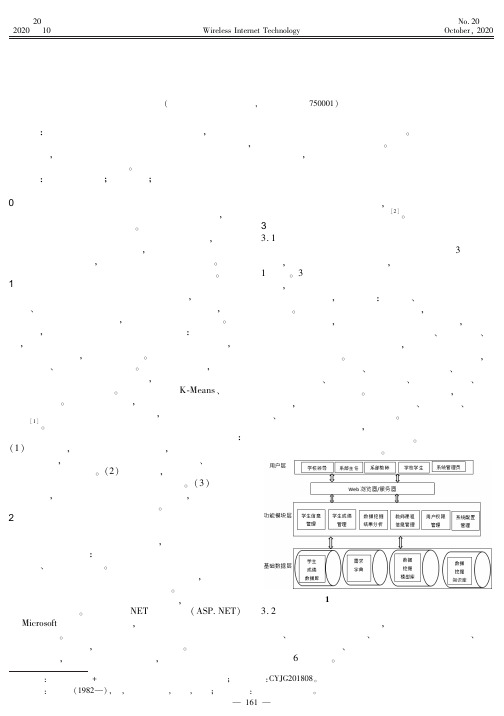
基金项目:基于互联网+时代的网络营销课程教学改革研究;项目编号:CYJG201808㊂作者简介:刘思皖(1982—),女,宁夏银川人,讲师,硕士;研究方向:计算机应用技术㊂刘思皖(宁夏财经职业技术学院,宁夏㊀银川㊀750001)摘㊀要:数据挖掘技术是一种数据处理的技术,其可以从海量数据中提取潜在的有用数据信息㊂基于数据挖掘技术的学生成绩系统可以实现对学生成绩的自动分类与分析,达到对学生多方位预测的目的㊂文章通过对数据挖掘技术的分析,引导出基于数据挖掘技术的学生成绩系统设计的内在要求,最后提出基于数据挖掘技术的学生成绩系统的具体设计方案与实现策略㊂关键词:数据挖掘技术;学生成绩;设计0㊀引言学生成绩不仅是反映学生学习效果的重要指标,也是高校改进教学质量的重要依据㊂传统的学生成绩管理模式主要是利用计算机技术对学生的成绩进行排名,难以有效的挖掘出潜在的有用数据信息,而数据挖掘技术则可以利用关联规则等元素,实现对学生成绩的自动分析㊂因此本文详细阐述基于大数据技术的学生成绩分析系统㊂1㊀数据挖掘技术的概述数据挖掘技术是一种知识发现的技术,从大量的不确定㊁模糊的数据中寻找数据内在的特征和规律,从而发现潜在的有价值的信息,为决策者提供数据依据㊂一般而言,数据挖掘技术主要包括以下几种:一是分类技术,即从特定的数据群中找出特定类别的描述方法,以此将其进行分类,构造分类模型㊂例如我们常用的数理统计方法㊁神经网络模型等等㊂二是聚类技术,即将数据库中的数据集划分为若干子集,使得每个数据子集内部都具有较强的相关性㊂例如常见的K-Means㊁数据统计方法等㊂三是关联分析,即用于发现数据集合中数据项之间的某种关联和联系,发现其内在的规律性[1]㊂数据挖掘技术的使用流程主要包括以下几个方面:(1)数据准备,它是数据挖掘的基础,主要是将数据进行集中汇总,该环节主要是消除数据中的噪声㊁消除数据间的不一致和模糊性㊂(2)数据发现,即选择合适的算法和恰当的分析方法将影响数据挖掘的结果㊂(3)结果表达和解释,它是数据挖掘的最后一个环节,也就是将挖掘的结果以可视化的方式进行展示㊂2㊀基于数据挖掘技术的学生成绩系统设计的内在需求构建基于数据挖掘技术的学生成绩系统设计必须要清晰地了解系统设计的内在要求,结合实践调查其内在需求主要表现为:一是传统的学生成绩统计模式存在时间长㊁效率低的问题㊂例如传统的学生统计模式主要是利用各种表格方式将学生的成绩进行排名,而没有对学生成绩的深层次问题进行准确分析㊂而利用数据挖掘技术则可以构建出学生成绩分析系统,实现对学生成绩的深层次分析㊂例如采取NET 技术路线()和Microsoft 数据库进行开发,从而设计支持多人协作开发的系统㊂二是数据挖掘技术可以实现对学生成绩数据的自动化分析,挖掘出潜在有用信息㊂学生成绩来源渠道不同,而且差异性比较突出,因此在数据采集时需要考虑到数据的变量问题,而对于数据变量的分析则必须要通过数据挖掘技术实现[2]㊂3㊀基于数据挖掘技术的学生成绩系统设计3.1㊀体系结构设计基于数据挖掘的高校学生成绩系统采用3层体系结构,结合上述学生成绩模型,三层体系结构设计如图1所示㊂3层体系结构将数据和业务逻辑以及系统实现分开,使得系统用户只需专注数据分析结果而无须理会数据的操作过程,具体分为:用户层㊁功能模块层和基础数据层㊂基础数据层为最底层的结构,它将基础数据存储于数据仓库中,对数据进行集中管理和处理,数据库中存储的数据包括学生成绩及相关数据㊁需求字典㊁数据挖掘方法模型库以及知识库等,系统通过数据库对数据进行读取和操作㊂功能模块层又称为业务逻辑层,主要由数据挖掘流程管理㊁数据挖掘需求管理㊁数据挖掘模型方法管理㊁数据挖掘结果分析㊁系统配置管理㊁数据源配置等功能模块构成㊂用户层为顶层结构,是系统的展示层,系统用户主要包括学校领导㊁系部主任㊁系部教师㊁在校学生以及系统管理员等㊂而系部主任和系部教师则是系统的主要用户,他们负责学生成绩数据挖掘并将结果展示给学校领导或相关用户㊂用户角色和用户权限通过系统配置管理实现㊂图1 数据挖掘学生成绩系统结构3.2㊀功能模块设计按照功能需求分析结果,系统划分为学生信息管理模块㊁学生成绩管理模块㊁数据挖掘结果分析模块㊁教师课程信息管理模块㊁用户权限管理模块和系统配置管理模块等6个模块㊂161第20期2020年10月无线互联科技㊃技术应用No.20October,20203.3㊀数据库表设计数据库是一个系统所有数据的集合,这些数据按特定的组织方式存储在一起,通过通用的存取方式合理而高效地完成系统所需要的各类功能㊂系统信息表主要由系统所有基本编码表组成,这些系统编码表是系统赖以运行的基本;教务信息表包含教学计划表㊁课程表㊁班级表㊁教学资源表等;人员信息表包括教师信息表㊁学生信息表和教职工信息表㊂3.4㊀应用Apriori算法分析学生成绩3.4.1㊀数据挖掘过程数据挖掘过程是对相关数据进行预处理的过程,主要包括:(1)明确数据挖掘对象与目标㊂数据挖掘技术使用的关键就是要确定具体的挖掘数据,基于本文设计目标,数据挖掘的对象主要是学生的成绩,因此需要相关人员将涉及学生成绩的所有数据纳入到数据库系统中,为下一步的数据提取㊁清理工作打下基础㊂(2)数据预处理㊂数据预处理就是去除数据中的无关信息,即去除与学生成绩无关或者无效成绩的数据㊂(3)对数据进行挖掘㊂对数据库中的数据进行深入分析㊁挖掘,得出相应的分析结果,为用户提供有用信息㊂3.4.2㊀学生成绩数据采集为了更好地对学生成绩进行分析,本文以我院计算机专业学生4个学期的所有课程成绩数据作为研究对象,并结合学生的学习兴趣,对这些数据进行清洗㊁转换等,通过关联规则的算法挖掘出影响学生成绩的关键因素㊂依据学生的培养方案,学生在学习 必修 限选 任选 类课程时必须遵照培养方案中的学分下限要求㊂由于 限选 和 必修 类的课程囊括了在校学生的学科内部专业课程和基础课程两个方面,同时高校学生的专业课程成绩与学生最终的成绩联系最紧密,即: 限选 和 必修 类课程的重要程度比 任选 类课程高,因此,借助对学生 必修 和 限选 两类课程的成绩数据挖掘分析,忽略 任选 类课程㊂高校里不同专业开设的课程每学期都小幅度调整㊁更新,但是 必修 课程和 限选 课程变动情况却非常少,因此,数据库当中这两种类型的成绩出现率也是非常高的,数据存储的时间跨度最大㊂综合上述,把 限选 和 必修 类课程的成绩作为研究对象,采集数据预期分析效果较为理想,可以有效揭示学生考试成绩所蕴含的关联[3]㊂3.4.3㊀数据预处理考虑到学生成绩的差异性特点,本次设计将学生的每门成绩按照不合格率㊁合格率㊁中等率以及优秀率的等级进行划分,对原始数据进行离散化处理,对学生成绩当中的较高成绩与较低成绩进行深入分析㊂一方面分析学生考试成绩之间所隐含的影响因素,另一方面分析不同课程之间的关联㊂数据挖掘过程中应用的数据采集自高校的教学管理的成绩数据仓库:将数据存储在表格内的可以直接导出到CSV等数据集去,预处理阶段处理成绩缺失值等问题㊂3.4.4㊀关联规则挖掘实施关联规则的挖掘实施是数据挖掘算法实施的关键,本文选择的是关联规则挖掘Apriori算法,因此根据系统设计的原则要求,设置的最小支持度为0.2,最小置信度是0.5㊂首先需要建立健全Grade数据库,据库中的Course表是用来存储课程信息,Special畸Inf.o用来存储学籍信息,而且ade表是用来存储学生的考试成绩信息的;其次对数据库中的所有信息进行分析,并且对成绩超过80分的进行总结,同时将课程的支持度和课程名称的计数信息存放到频繁1项集的数据表格Frequentl中,Frequentl有两个关键的字段nem和SupCount㊂再次得到频繁项集之后,就可以计算出相应的候选项集生成相应的频繁项集㊂最后算出最终的频繁项目集中的非空子集所包含的置信度和支持度,并且拿它们与最小支持度和最小置信度进行比较,比较后删除那些小于最小置信度的记录,并且最终会产生关联规则㊂4㊀系统的测试为检验系统的各项性能在系统规定允许的软硬件环境下(包括服务器㊁客户机的各类机器指标如CPU主频㊁机器结构㊁硬盘速度㊁网络带宽㊁实际传输速率等)是否符合预期给定的指标,需要进行性能测试,主要测试软件在特定环境下的处理速度㊂而环境要尽量考虑实际运行状态下的环境,根据实际的测试结果分析可知,未发现本系统中存在严重等级较高的异常或错误,从整体上来讲通过了本次测试㊂[参考文献][1]周凌.浅析大数据时代的数据挖掘技术与应用[J].中小企业管理与科技,2018(5):189-190.[2]吴修国,孙涛.基于教育大数据挖掘的大学生学业预警研究[J].中国教育信息化,2020(7):55-57,62.[3]丁国勇,程晋宽.基于教育数据挖掘的高校学生学业表现建模研究[J].黑龙江高教研究,2020(2):81-86.(编辑㊀傅金睿) Design and implementation of student achievement system basedon data mining technologyLiu Siwan(Ningxia College of Finance and Economics,Yinchuan750001,China)Abstract:Data mining technology extracts potentially useful data information from massive data.The student achievement system based on data mining technology can realize the automatic classification and analysis of students achievement and achieve the purpose of multi-directional prediction of students.This paper analyzes the data mining technology,guides the internal requirements of student achievement system design,and finally puts forward the specific design scheme and implementation strategy.Key words:data mining technology;student performance;design261。
基于数据挖掘技术的学生成绩预测文献研究(IJEME-V7-N6-5)
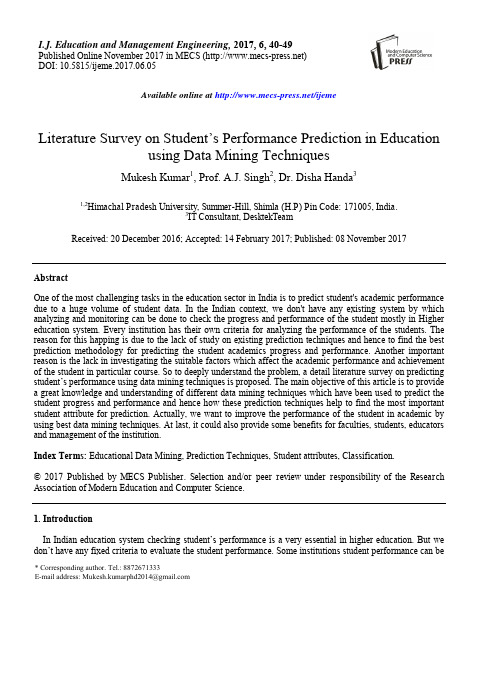
I.J. Education and Management Engineering, 2017, 6, 40-49Published Online November 2017 in MECS ()DOI: 10.5815/ijeme.2017.06.05Available online at /ijemeLiterature Survey on Student’s Performance Prediction in Educationusing Data Mining TechniquesMukesh Kumar1, Prof. A.J. Singh2, Dr. Disha Handa31,2Himachal Pradesh University, Summer-Hill, Shimla (H.P) Pin Code: 171005, India.3IT Consultant, DesktekTeamReceived: 20 December 2016; Accepted: 14 February 2017; Published: 08 November 2017AbstractOne of the most challenging tasks in the education sector in India is to predict student's academic performance due to a huge volume of student data. In the Indian context, we don't have any existing system by which analyzing and monitoring can be done to check the progress and performance of the student mostly in Higher education system. Every institution has their own criteria for analyzing the performance of the students. The reason for this happing is due to the lack of study on existing prediction techniques and hence to find the best prediction methodology for predicting the student academics progress and performance. Another important reason is the lack in investigating the suitable factors which affect the academic performance and achievement of the student in particular course. So to deeply understand the problem, a detail literature survey on predicting student’s performance using data mining techniques is proposed. The main objective of this article is to provide a great knowledge and understanding of different data mining techniques which have been used to predict the student progress and performance and hence how these prediction techniques help to find the most important student attribute for prediction. Actually, we want to improve the performance of the student in academic by using best data mining techniques. At last, it could also provide some benefits for faculties, students, educators and management of the institution.Index Terms: Educational Data Mining, Prediction Techniques, Student attributes, Classification.© 2017 Published by MECS Publisher. Selection and/or peer review under responsibility of the Research Association of Modern Education and Computer Science.1. IntroductionIn Indian education system checking student’s pe rformance is a very essential in higher education. But we don’t have any fixed criteria to evaluate the student performance. Some institutions student performance can be * Corresponding author. Tel.: 8872671333E-mail address: Mukesh.kumarphd2014@observed by using internal assessment and co-curriculum. In the Indian context, an institution with the higher degree of reputation using the good academic record as its basic criteria for their admissions [1]. There are lots of definitions of student academic performance prediction should be given in the literature. Different authors are using different student factors/attributes for analyzing student performance. Most of the author used CGPA, Internal assessment, External assessment, Examination final score and extra co-circular activities of the student as prediction criteria.Most of the Indian institution and universities using final examination grade of the student as the student academic performance criteria. The final grades of any student depend on different attributes like internal assessment, external assessment, laboratory file work and viva-voce, sessional test. The performance of the student depends upon how many grades a student score in the final examination. Norlida Buniyamin, Pauziah Mohd Arsad et al. (2013) stated that what are the significance of academic analytics for an educational institution and how they work for the improvement of education. They also proposed an intelligent recommendation intervention system to improve the student’s performance and achievement in education.This system uses two different student attribute to measure the achievement and that is student grade and student information [2]. Zaidah Ibrahim and Daliela Rusli et al. (2007) stated that predicting student's performance is very critical for any educational institution because it is important for the formation of new rule and standards for the improvement of the education and reputation. They used CGPA and demographic attributes of the first year student to predict their result in the first year of education in engineering [3].Data mining techniques which are used in mostly education are known as Educational data mining. There are lots of data mining techniques are available to predict the student performance. Education data mining help to find the hidden information from a huge database of education setting, because at present lots of data are generated in educational institution related to student [4]. Further, this hidden information can be used for performance, dropout and final result prediction of the student. It also helps the educator, management and faculties to work according to the learning standards of the students. Actually data mining help in the different field of education sector [5]. So to properly understand the real meaning of the data mining in education we need to do a systematic literature review on different work done by the different researcher. Our main objectives to this proposed work are:i.To understand, analyse and then find the difference between different prediction techniques of datamining in education.ii.To identify and understand different student attributes which are mainly used for the predicting the student performance.iii.To identify and understand the different prediction techniques which are mainly used for predicting the student performance.The above points are the main focus of our study. In section 2, the main focus will be given on the methodology adopted for the formation of research questions for this paper and literature survey. In section 3-4, the main focus of the study is to find or identify the important factors on Predicting Student's performance and prediction methods used for student performance. In section 5-6, the main focus is one the overall discussion on the result of the study and in the last conclusion and future work scope is given.2. Research Questions Formation and Search Strategy for Literature ReviewThe main purpose of literature survey is to find out new techniques to work on the old data set and then find out some new information form that. To do some relational survey, the literature of more than 10 years should be taken into consideration and then find out some knowledge gaps between works done by the researcher. It helps to justify your research questions and gave some direction for future research.Formulation of Research Questions: Research question formation is one of the essential tasks when going for written any research paper. Before the formation of any research question try to understand and following the Kitchenhams steps. B. Kitchenhams, R Pretorius et al. (2010), stated that PIOC (Population, Intervention, Outcome, Context) are the most critical factors which are considered when going to frame research question for you research paper [6].Table 1. Research Question Formation CriteriaCriteria Detail of targeted organisationIntervention Data Mining Techniques/ method used for prediction of student performance and progress in educationOutcome Student performance prediction accuracy, finalise prediction techniquesContext University, Schools and colleges ( Private and Government)From the above table, everything is clear about the research target organisation, techniques undertaken during the review, related outcome and affected organisation. Considering the above criteria in mind when framing the research question, we restricted the scope of this study with these research questions.i.Try to identify those student attributes which are helpful for predicting student academic performance.ii.Try to identify those data mining techniques which are mostly used for predicting student academic performance.After research questions formation we need to do the pilot study on the related topic and then need to find out the research gaps between different works done the different researcher by using data mining techniques. Before start the literature survey, everything should be clear in the mind of the researcher that what they want to search and how the search can be done.Search strategy for literature review:Searched databases: Springer Link, Researchgate, IEEE Xplore, ACM Digital library, Elsevier, Science Direct other computer science journals. Searching sentences and keywords: Predicting student performance, Predicting student performance uses data mining techniques, Application of data mining in education, Educational Data Mining methodology or techniques, Prediction of student result using data mining techniques. Publication periods are taken into consideration: 2007 to July 2016. Types of text searched: Documents, PDF, Full-length paper with abstract and keywords. Search Items: Journal articles, Conferences paper, Workshop papers, Expert lectures or talks, topics related blogs, Topic related communities (like Educational data miningcommunity).3. Important Factors of Students Used for Predicting Student’s PerformanceThe prediction of SAP is based on different factors of student’s like an individual, community, psychological and environmental variables. During last few years lots of resea rches have been carried out to predict students’ academic performance. So in this section, we are taking few research articles into consideration and then analyse them for different student's factors which affect the student academics prediction. Almost 30-40 research papers, article, book chapters are considered for review. Farhana Sarker and Hugh C Davis (2013) in his research showed that the institutional internal data sources (IDS) and external data source (EDS) gave the best result than the model based on only institutional internal student databases [4]. In another study, D. M. D. Angeline (2013) used Internal Assessment Test grade, Assignment submission and Grade, Correct Response, Self-Confidence, Interest in the particular course and Degree ambition for prediction of student's academic performance [5]. Abeer Badr El Din Ahmed et. al. (2014) in his study used the course of the student, HSD, mid-term marks, Lab test grade, seminar performance, assignment, attendance, homework, student participation for prediction SAP [6]. Fadhilah Ahmad and Azwa Abdul Aziz (2015) collected data from the database of Academic Department, UniSZA that stored in Informix Database Management System (DBMS). They further used nine different parameters like gender, race and hometown, GPA, family income, university entry mode, grades Malay Language, English, and Mathematics [7]. Mashael A. Al-Barrak and Mona S. Al-Razgan (2015) collected dataset of student's from the Information Technology department at Kin Saud University, Saudi Arabia for their analysis. They further used the different attribute for the prediction like student ID, student name, student grades in three different quiz's, midterm1, midterm2, project, tutorial, final exam, and total points obtained in Data structure course of computer science department [8]. Edin Osmanbegović and Mirza Suljic (2012) collected data from surveys in the midst of first-year students and the data taken during the enrollment at the University of Tuzla. They further used the different attribute for the prediction like Gender, Family, Distance, High School, GPA, Entrance exam, Scholarships, Time, Materials, the Internet, Grade importance, Earnings [9]. Raheela Asif and Mahmood K. Pathan (2014) in his study they used four academic batches of Computer Science & Information Technology (CS&IT) department at NED University, Pakistan. They used HSC marks, marks in MPC, Maths marks in HSC, marks in various subject studied in the regular course of a programming language, CSA, Logic design, OOP, DBMS, ALP, FAM, SAD, Data Structure etc for their analysis [10]. Mohammed M. Abu Tair and Alaa M. El-Halees (2012) in his study tried to extract some useful information from student's data of Science and Technology College – Khan Younis. They initially selected different attributes like Gender, date of Birth, Place of Birth, Speciality, Enrollment year, Graduation year, City, Location, Address, Telephone number, HSC Marks, SSC school type, HSC obtained the place, HSC year, College CGPA for analysis. But after preprocessing of the data they found that attribute like Gender, Speciality, City, HSC Marks, SSC school type, College CGPA are most significant [11]. Azwa Abdul Aziz and H.I.F Ahmad (2014) used first-semester student data of Bachelor of Computer Science from University Sultan ZainalAbidin (UniSZA) for analysis. They used the attributes like Gender, race, Hometown Location, University Entry Mode, Family Income for data collection [12]. K.D Kolo and J.K Alhassan (2015) collected computer science student's data of Nigerian Colleges of Education. In his study, they considered Data Structure course of computer science is one of the most important subjects and hence collect data respective to this subject. They considered student attributes like Student's grade, Student's status, Students gender, financial strength, Attitude to learning as important factors for the prediction of SAP [13]. Jyoti Bansode (2016) for predicting student academics performance collected data from Shah and Anchor Kutchhi Polytechnic, Chembur, Mumbai. They considered student attributes like parent's education, parent' s occupation, category, SSC board, admission type, SSC medium, SSC class, first-semester result, second-semester, third-semester, forth-semester, the fifth-semester and sixth-semester result as most important attributes [15]. R. Sumitha and E.S. Vinoth Kumar (2016) for his research collected data of around 350, BE (CSE) students of KLN College of Information Technology. Initially, they selected 24 attributes for analysis, but finally attributes with the higher ranking aretaken into consideration for the classification purpose. The selected attribute are CGPA, arrears, attendance, SSC marks, Engineering Cut-off, medium-of-education and type of Board [16]. Mrinal Pandey and S. Taruna (2016) for this study used datasets from an engineering Institution. They included the data related to the student’s academics attributes as well as their demographics information [18]. Maria Goga, Shade Kuyoro, Nicolae Goga (2015) used student data from Babcock University, Nigeria. On the basis of reviewed literature, they considered age, gender, parent's marital status, parent's qualification, parent's occupations, SSC score, HSC score, CGPA first year [19]. Maria Koutina and Katia Lida Kermanidis (2011) they tried to find out the best techniques for predicting the final grade of the postgraduate students of Ionian University Informatics, Greece. On the basis of reviewed literature, they considered Gender, Age, Marital Status, Number of children, Occupation, Job associated with computers, Bachelor, Another master, Computer literacy, Bachelor in informatics [24].After reviewed almost 20-25 research paper, we found that in most of the cases, student's factors which affect the SAP are gender, high school grade, student's parental education, financial background, living location, medium of teaching, student's family status, students' previous semester marks, class test grade, seminar performance, assignment performance, general proficiency, attendance in class and lab work, Interest in particular course, Study Behaviour, Engage Time and Family Support for study, admission type, previous schools marks, accommodation type, parent's qualification, parent's occupation. All these attributes fall into different categories like personal, family, Academic, Institutional and Social.The most important personal attributes of the student like gender, age, interested in the study, admission type, Study Behaviour are taken into consideration [7, 8, 9, 11, 12, 13, 18, 19, 24]. The family attributes like parent’s qualification, parent’s occupation, family income, family status, Family Support for study are also taken as important for the academics prediction [7, 9, 15, 19, 24]. Whereas for academic attributes like high school grade, students’ previous semester mark s, class test grade, seminar performance, assignment performance, attendance in class and lab work, previous schools marks are taken into consideration [5, 6, 7, 8, 9, 10, 15, 16, 18, 19, 24] and for institutional attributes most the researcher are taken medium of teaching, accommodation type, infrastructure, water and toilet facilities, teaching methodology, transportation facilities into consideration[4, 7, 9, 12, 16, 18, 24].4. Different Data Mining Techniques used for Predicting Student’s PerformanceIn Educational data mining field, making a prediction about student academic performance is usually done. To build a predictive modelling we need to take different data mining techniques into consideration like classification, clustering association rule mining and regression analysis. In almost every research paper, the only classification algorithm is taken into consideration for predicting student academic performance. There are so many classification techniques available for prediction but we are taking into consideration only decision tree, Naive Bayes, Support Vector Machine (SVM), Artificial Neural Networks (ANN), K-Nearest Neighbor, SMO, Linear Regression, Random Forest, Random Tree, REPTree, LADTree, J48 etc. Table-2 gave a brief finding of different r esearch papers with their author’s name, main attributes helpful for prediction accuracy with different data mining algorithm used.Table 2. Different Data Mining Techniques used for Predicting Student’s PerformanceAuthor’s Attributes which affect prediction accuracy DT NB RB KNN ANNF Sarker et. al. Internal attributes + students’ first semestermark ( Model1)-- -- -- -- 74.5F Sarker et. al. Int + Ext attributes + students’ first semestermark ( Model2)-- -- -- -- 76.5F Ahmad et. al Gender, race, hometown, GPA, family income,uni. mode entry, SPM grades-- 67.0 71.3 -- 68.8Mashael A.et. al. first midterm exam (Predict Students Failure) -- 91 55.0 -- 89.8 M Suljic et. al. GPA, URK , MAT, VRI -- 76.6 -- 73.9R Asif et. al. HSC, MPC and HSC marks, pre-uni marks,marks in different courses73 83.6 56.9 74 67.6El-Halees et. al. SS_Type, HSC marks, City,Gender, Speciality-- 67.5 71.2 -- --A Aziz et. al. Gender, race, Hometown Location, Uni EntryMode, Family Income68.8 63.3 68.8 -- --K D Kolo et.al. status, gender 66.8 -- -- -- --Jyoti Bansode SSC marks, SSC medium, Admission type,mother‘s occupation85 -- -- -- --R. Sumitha et. al. TWM, MOE, TOB, ATD ECUT, CGPA,arrears,97.2 85.9 96.1 -- --S V. Shinde et. al Student’s Internal Assessment 97.5M Pandey et. al. Academic information’s, Demographicinformation98.8 91.5 84.1 -- --N Goga et. al. family, PEP, EES, end of the first sessionresult99.9 -- 96.7 -- --G. S Josan et. al. Sex, INS-High, TOB, MOI, TOS, PTUI, S-Area, Mob, Com-HM, Netacs, Int-GR, Atdn69.7 65.1 -- -- --M Koutina et. al. Gender, Age, Marital Status, No of children,Occu., Job associated with PC, Bachelor,Another master, Comp literacy, Bachelor ininformatics68.5 100 90.9 100 --From the above table, we find that Maria Koutina and Katia Lida Kermanidis, In his research found the 100% accuracy with Naive Bayes and K-Nearest Neighbor algorithm [24]. They represented their result in Table 6 under “Total accuracy (%) of re-sample data and fea ture selection”. For prediction student academic performance they used attributes like Gender, Age, Marital Status, Number of children, Occupation, Job associated with the computer, Bachelor, Another master, Computer literacy, Bachelor in informatics.5. Discussion on This Predicting Student’s SurveyIn this particular section, we will discuss the main finding of our meta-analysis. In this meta-analysis, we find that mostly used data mining algorithm for SAP is Decision Tree (DT), Naive Bayes (NB), Artificial Neural Networks (ANN), Rule-based (RB) and K-Nearest Neighbor (KNN). In Decision tree algorithm the maximum and minimum accuracy for predicting student’s academic performance are 99.9% and 66.8% respectively. To find the maximum prediction accuracy Maria Goga, Shade Kuyoro and Nicolae Goga used the combination of student’s attribute like family, PEP, EES, end of first session result [19]. In Naive Bayes algorithm, the maximum and minimum accuracy for predicting student's academic performance are 100% and 63.3% respectively. Maria Koutina et. al. used the different combination of student's attribute like Gender, Age, Marital Status, Number of children, Occupation, Job associated with the computer, Bachelor, Another master,Computer literacy, Bachelor in informatics for getting maximum accuracy [24]. In rule-based algorithm, the maximum and minimum accuracy for predicting student's academic performance are 96.7% and 55.0% respectively. To find the maximum prediction accuracy Maria Goga et al. used a combination of student's attribute like family, PEP, EES, end of first session result [19]. In K-Nearest Neighbor algorithm the maximum and minimum accuracy for predicting student’s academic performance are 100% and 74% respectively [24]. In Artificial Neural Netwo rks (ANN) the maximum and minimum accuracy for predicting student’s academic performance are 89.8% and 67.6% respectively. To find the maximum prediction accuracy Mashael A. Al-Barrak and Mona S. Al-Razgan used a combination of student's attribute like first mid-term examination in their first-year course [8]. Table-3 gave a brief representation of result analysis.Table 3. Student Academic Performance Prediction Techniques with Their AccuracyData Mining Techniques DT NB RB KNN NNLowest Accuracy66.8% 63.3% 55.0% 74% 67.6%Fig. 1 shows the prediction accuracy that uses classification method grouped by algorithms for predicting student’s performance since 2012 to 2016.Fig.1. Student Academic Performance Prediction Grouped By Algorithm used6. Conclusion and Future WorkAt present research in educational data mining create lots of interest in the research community. Because predicting student academic performance, predicting educational dropout student in near future, predicting institute placement and admission in a new academic year is most useful for educators and management and educational policy maker. It also used for improving the teaching-learning process in the institution as well. This paper has reviewed lots of research papers, the article on predicting student's academic performance withselected attribute and an analytical algorithm used. In most of the cases, CGPA and the internal marks of the student in academic are important attributes for prediction of result. In one of the research paper author's find 100% accuracy for their prediction with a combination of different attributes like Gender, Age, Marital Status, Number of children, Occupation, Job associated with the computer, Bachelor, Another master, Computer literacy, Bachelor in informatics. In the case of data mining prediction, classification is frequently used technique. Most of the researchers used Decision Tree, Naive Bayes and Rule- Based algorithm for predicting student’s academic performance. At the end, we conclude that the meta-analysis on predicting student's academic performance motivated us to do further research work in our own educational environment. It will really help to improve our education system to check the regular performance of the student. AcknowledgementsI am grateful to my guide Prof. A.J. Singh and Dr Disha Handa for all help and valuable suggestion provided by them during the study.References[1]Mi hai Dascalu and Elvira Popescu et. al., Predicting Academic Performance Based on Students’ Blogand Microblog Posts, Springer International Publishing Switzerland 2016 K. Verbert et al. (Eds.): EC-TEL 2016, LNCS 9891, pp. 370–376, 2016. DOI: 10.1007/978-3-319-45153-4_29.[2]U. bin Mat, N. Buniyamin, P. M. Arsad, R. Kassim, An overview of using academic analytics to predictand improve students’ achievement: A proposed proactive intelligent intervention, in: Engineering Education (ICEED), 2013 IEEE 5th Conference on, IEEE, 2013, pp. 126–130.[3]Randa Kh. Hemaid and Alaa M. El-Halees, Improving Teacher Performance using Data Mining,International Journal of Advanced Research in Computer and Communication Engineering Vol. 4, Issue 2, February 2015.[4]Farhana Sarker, Thanassis Tiropanis and Hugh C Davis, Students’ Performance Prediction by UsingInstitutional Internal and External Open Data Sources, /353532/1/Students' mark prediction model.pdf, 2013.[5] D. M. D. Angeline, Association rule generation for student performance analysis using an apriorialgorithm, The SIJ Transactions on Computer Science Engineering & its Applications (CSEA) 1 (1) (2013) p12–16.[6]Abeer Badr El Din Ahmed and Ibrahim Sayed Elaraby, Data Mining: A prediction for Student'sPerformance Using Classification Method, World Journal of Computer Application and Technology 2(2): 43-47, 2014.[7]Fadhilah Ahmad, Nur Hafieza I smail and Azwa Abdul Aziz, The Prediction of Students’ AcademicPerformance Using Classification Data Mining Techniques, Applied Mathematical Sciences, Vol. 9, 2015, no. 129, 6415 - 6426HIKARI Ltd, /10.12988/ams.2015.53289.[8]Mashael A. Al-Barrak and Mona S. Al-Razgan, predicting students’ performance through classification:a case study, Journal of Theoretical and Applied Information Technology 20th May 2015. Vol.75. No.2.[9]Edin Osmanbegović and Mirza Suljic, DATA MINING APPROACH FOR PREDICTING STUDENTPERFORMANCE, Economic Review – Journal of Economics and Business, Vol. X, Issue 1, May 2012.[10]Raheela Asif, Agathe Merceron, Mahmood K. Pathan, Predicting Student Academic Performance atDegree Level: A Case Study, I.J. Intelligent Systems and Applications, 2015, 01, 49-61 Published Online December 2014 in MECS (/) DOI: 10.5815/ijisa.2015.01.05.[11]Mohammed M. Abu Tair, Alaa M. El-Halees, Mining Educational Data to Improve Students’Performance: A Case Study, International Journal of Information and Communication Technology Research, ISSN 2223-4985, Volume 2 No. 2, February 2012.[12]Azwa Abdul Aziz, Nor Hafieza Ismailand Fadhilah Ahmad, First S emester Computer Science Students’Academic Performances Analysis by Using Data Mining Classification Algorithms, Proceeding of the International Conference on Artificial Intelligence and Computer Science(AICS 2014), 15 - 16 September 2014, Bandung, INDONESIA. (e-ISBN978-967-11768-8-7).[13]Kolo David Kolo, Solomon A. Adepoju, John Kolo Alhassan, A Decision Tree Approach for PredictingStudents Academic Performance, I.J. Education and Management Engineering, 2015, 5, 12-19 Published Online October 2015 in MECS () DOI: 10.5815/ijeme.2015.05.02.[14]Dr Pranav Patil, a study of student’s academic performance using data mining techniques, internationaljournal of research in computer applications and robotics, ISSN 2320-7345, vol.3 issue 9, pg.: 59-63 September 2015.[15]Jyoti Bansode, Mining Educational Data to Predict Student‘s Academic Performance, InternationalJournal on Recent and Innovation Trends in Computing and Communication ISSN: 2321-8169, Volume:4 Issue: 1, 2016.[16]R. Sumitha and E.S. Vinoth kumar, Prediction of Students Outcome Using Data Mining Techniques,International Journal of Scientific Engineering and Applied Science (IJSEAS) – Volume-2, Issue-6,June 2016 ISSN: 2395-3470.[17]Karishma B. Bhegade and Swati V. Shinde, Student Performance Prediction System with EducationalData Mining, International Journal of Computer Applications (0975 – 8887) Volume 146 – No.5, July 2016.[18]Mrinal Pandey and S. Taruna, Towards the integration of multiple classifiers pertaining to the Student'sperformance prediction, /10.1016/j.pisc.2016.04.076 2213-0209/© 2016 Published by Elsevier GmbH. This is an open access article under the CC BY-NC-ND license (/ licenses/by-nc-nd/4.0/).[19]Maria Goga, Shade Kuyoro, Nicolae Goga, A recommender for improving the student academicperformance, Social and Behavioural Sciences 180 (2015) 1481 – 1488.[20]Anca Udristoiu, Stefan Udristoiu, and Elvira Popescu, Predicting Students’ Results Using Rough SetsTheory, E. Corchado et al. (Eds.): IDEAL 2014, LNCS 8669, pp. 336–343, 2014. © Springer International Publishing Switzerland 2014.[21]Parneet Kaur, Manpreet Singh, Gurpreet Singh Josan, Classification and prediction based data miningalgorithms to predict slow learners in education sector, Procedia Computer Science 57 (2015) 500 – 508.[22]M. Durairaj and C. Vijitha, Educational Data mining for Prediction of Student Performance UsingClustering Algorithms, International Journal of Computer Science and Information Technologies, Vol. 5(4), 2014, 5987-5991.[23]Mohammed I. Al-Twijri and Amin Y. Noaman, A New Data Mining Model Adopted for HigherInstitutions, Procedia Computer Science 65 ( 2015 ) 836 – 844, doi: 10.1016/j.procs.2015.09.037. [24]Maria Koutina and Katia Lida Kermanidis, Predicting Postgraduate Students’ Performance UsingMachine Learning Techniques, L. Iliadis et al. (Eds.): EANN/AIAI 2011, Part II, IFIP AICT 364, pp.159–168, 2011. © IFIP International Federation for Information Processing 2011.Authors’ ProfilesMukesh Kumar (10/04/1982) has pursuing PhD in Computer Science from Himachal PradeshUniversity, Summer-Hill Shimla-5. India. My research interest includes Data Mining,Educational Data Mining, Big Data and Image Cryptography.。
R语言数据挖掘之欧阳语创编
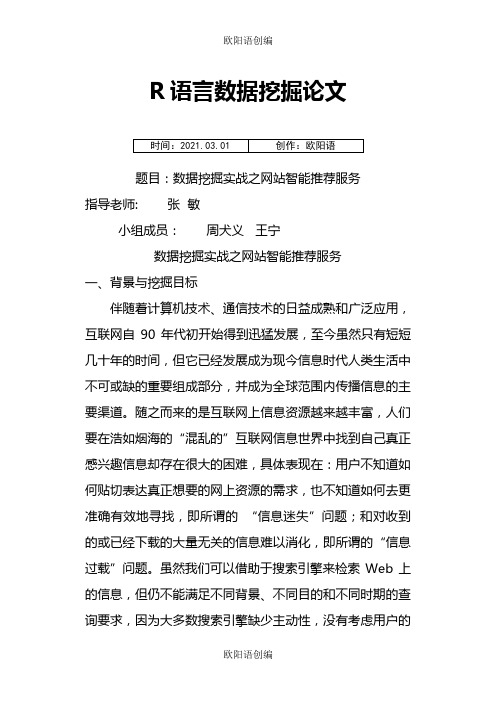
R语言数据挖掘论文题目:数据挖掘实战之网站智能推荐服务指导老师: 张敏小组成员:周犬义王宁数据挖掘实战之网站智能推荐服务一、背景与挖掘目标伴随着计算机技术、通信技术的日益成熟和广泛应用,互联网自90年代初开始得到迅猛发展,至今虽然只有短短几十年的时间,但它已经发展成为现今信息时代人类生活中不可或缺的重要组成部分,并成为全球范围内传播信息的主要渠道。
随之而来的是互联网上信息资源越来越丰富,人们要在浩如烟海的“混乱的”互联网信息世界中找到自己真正感兴趣信息却存在很大的困难,具体表现在:用户不知道如何贴切表达真正想要的网上资源的需求,也不知道如何去更准确有效地寻找,即所谓的“信息迷失”问题;和对收到的或已经下载的大量无关的信息难以消化,即所谓的“信息过载”问题。
虽然我们可以借助于搜索引擎来检索Web上的信息,但仍不能满足不同背景、不同目的和不同时期的查询要求,因为大多数搜索引擎缺少主动性,没有考虑用户的兴趣偏好和用户的不同,无法有效地解决信息过载和信息迷失的问题。
为了适应用户不断增长的信息需求,有效地解决信息过载和信息迷失给人们带来的种种问题,研究人员纷纷从人工智能中寻找突破口。
在许多探索性研究中,个性化推荐服务作为一种崭新的智能信息服务方式,应用前景广泛,十分引人注目。
与搜索引擎不同,推荐系统并不需要用户提供明确的需求,而是通过分析用户的历史行为,根据用户的行为主动推荐能够满足他们兴趣和需求的信息。
因此,对于用户而言推荐系统和搜索引擎是两个互补的工具。
搜索引擎满足有明确目的的用户需求,而推荐系统能够帮助用户发现感兴趣的内容。
因此,在电子商务领域中推荐技术可以起到以下作用:第一,帮助用户发现其感兴趣的物品,节省用户时间、提升用户体验;第二,提高用户对电子商务网站的忠诚度,如果推荐系统能够准确地发现用户的兴趣点,并将合适的资源推荐给用户,用户就会对该电子商务网站产生依赖,从而建立稳定的企业忠实顾客群,提高用户满意度。
基于数据挖掘的学生成绩查询系统
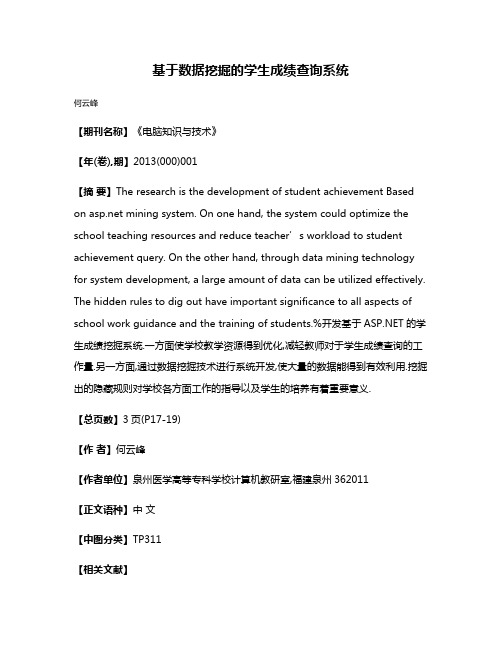
基于数据挖掘的学生成绩查询系统何云峰【期刊名称】《电脑知识与技术》【年(卷),期】2013(000)001【摘要】The research is the development of student achievement Based on mining system. On one hand, the system could optimize the school teaching resources and reduce teacher’s workload to student achievement query. On the other hand, through data mining technology for system development, a large amount of data can be utilized effectively. The hidden rules to dig out have important significance to all aspects of school work guidance and the training of students.%开发基于的学生成绩挖掘系统.一方面使学校教学资源得到优化,减轻教师对于学生成绩查询的工作量.另一方面,通过数据挖掘技术进行系统开发,使大量的数据能得到有效利用.挖掘出的隐藏规则对学校各方面工作的指导以及学生的培养有着重要意义.【总页数】3页(P17-19)【作者】何云峰【作者单位】泉州医学高等专科学校计算机教研室,福建泉州362011【正文语种】中文【中图分类】TP311【相关文献】1.基于ASP技术的学生成绩查询系统设计 [J], 李承遥;2.基于Android系统的学生成绩查询系统设计 [J], 王祥凤;李波;李丰鹏;范一航3.基于区块链的学生课程成绩查询系统 [J], 陈永强;颜廷秦;丁雯丽4.基于Java语言的学生成绩查询系统的设计 [J], 邹洁;冒绮5.基于Java语言的学生成绩查询系统的设计 [J], 邹洁; 冒绮因版权原因,仅展示原文概要,查看原文内容请购买。
- 1、下载文档前请自行甄别文档内容的完整性,平台不提供额外的编辑、内容补充、找答案等附加服务。
- 2、"仅部分预览"的文档,不可在线预览部分如存在完整性等问题,可反馈申请退款(可完整预览的文档不适用该条件!)。
- 3、如文档侵犯您的权益,请联系客服反馈,我们会尽快为您处理(人工客服工作时间:9:00-18:30)。
本科毕业设计(论文)欧阳家百(2021.03.07)题目: 基于数据挖掘技术的学生成绩分析系统的设计与实现姓名张宇恒学院软件学院专业软件工程班级 2010211503学号 10212099班内序号 01指导教师牛琨2014年5月基于数据挖掘技术的学生成绩分析系统的设计与实现摘要随着科技的不断发展和中国教育制度的日趋完善,各大高校对教务管理工作提出了越来越高的要求。
各大高校不再满足于传统的成绩管理方式,开始运用数据挖掘领域的先进方法对学生成绩进行分析和研究。
教务工作人员使用关联规则挖掘算法分析课程间的内在联系,可为学校的改进教学工作提供依据,并为学生的选课和学业规划提供指导;对学生进行分类,让学生能够对自己在校期间所学课程的成绩有一个全面而清晰的了解,方便学生扬长避短选择选修课程,及时对可能在学习上遇到困难的学生进行预警;运用聚类算法对学生进行聚类,找出具有共同特征的学生,并对不同学生群体分别采取不同的教学方法,初步体现因材施教的教育理念,最终探索出适合中国国情和教育制度的个性化培养模式。
本系统采用Eclipse作为开发平台,以Java作为开发语言。
通过对高校学生成绩分析系统的需求分析,本系统设计实现了可以使用关联规则挖掘算法分析课程间的内在联系,使用分类算法对学生进行分类,使用聚类算法对学生进行聚类。
希望本系统能对今后高校学生成绩分析系统的开发提供一定的参考价值。
关键词成绩分析关联规则分类聚类Design and implementation of student achievement analysis system based on data mining technologyABSTRACTWith the continuous development of technology and the Chinese education system maturing, Universities have put higher requirements to their academic administration.Universities are no longer satisfied with traditional performance management, began to apply advanced data mining methods to analyze and study students’ achievement.Staffof academic affairs use association rule mining algorithm to analysis intrinsic link between courses, which can provide the basis for improving the teaching of the school andguidance for the student's enrollment and academic ing classification algorithm to classify the students, so that students can have a clear understanding in their academic performance, and facilitate students in selecting courses.Warning students who probably face difficulties in the ing clustering algorithm to cluster the students to identify students with common characteristics, so that teachers can teach different students in different way,embodies the concept of individualized education, finally discover a personalized education model, which is suitable for China's national conditions and education system.The system was developedinEclipse, with java as a development language.By analyzing the need of student achievementanalysis system, this system uses association rule mining algorithm to analysis intrinsic link between courses, uses classification algorithm to classify the students, uses clustering algorithm to cluster the students to identify students.I hope this system can provide some reference value to the future development of college students’ achievement analysis system.KEYWORDS achievement analysisassociation rulesclassificationclustering目录第一章引言11.1选题的背景和意义11.2个性化培养的重要意义11.3国内外个性化培养的现状21.3.1国外个性化培养现状21.3.2 国内个性化培养现状31.4成绩分析系统的现状和存在的问题31.4.1成绩分析系统开发使用的现状31.4.2成绩分析系统建设存在的问题4第二章相关技术42.1相关数据挖掘知识理论42.1.1数据挖掘42.1.2 关联规则52.1.3 分类62.1.4 聚类62.2开发工具的选择72.2.1 Eclipse简介72.2.2 Eclipse的优势7第三章系统分析83.1软件过程模型83.2需求分析93.2.1 用例图93.2.2 需求的结构化描述10第四章系统设计与实现144.1系统概要设计144.1.1系统体系结构144.1.2 系统数据结构154.2系统详细设计164.2.1 文件导入数据164.2.2 数据预处理164.2.3 关联规则184.2.4 分类194.2.5 聚类204.2.6 导出文件224.3系统实现234.3.1 文件导入数据234.3.2 数据预处理234.3.3 关联规则234.3.4 分类244.3.5 聚类244.3.6 导出文件254.4系统应用26第五章结论30参考文献31致谢32第一章引言1.1 选题的背景和意义进入新世纪以来,我国的高等教育事业正在快速发展,各个领域的重大科研成果不断涌现,各知名院校的国际排名和知名度也不断攀升。
然而在这些光鲜靓丽的学术成果之下,各大高校对学生的管理工作却并没有跟上时代的步伐。
其实对于给大高校来说,学生的考试成绩是一笔非常宝贵的财富。
学生的成绩是反映学校教学水平的最直接的第一手资料,这些数据可以为学校改进招生和教学工作提供重要依据。
然而,学生成绩的管理工作并没有引起高校足够的重视,尤其是在对学生成绩的分析处理方面,绝大部分高校还停留在较为原始的数据库管理和查询阶段,没有对学生的成绩进行横向和纵向的对比研究,也缺乏对各学科之间成绩内在联系的挖掘。
学科成绩间的内在联系是广泛存在于各个专业的各门课程中的,学科成绩间内在联系的分析和研究对对学生和学校都有着十分重要的意义。
通过了解学科成绩间存在的内在联系,学生可以清楚地认识到基础课程、先导课程的重要性,并且在选择选修课的时候,做到扬长避短,通过更多地选择与自己优势课程成绩正相关的课程来帮助提高成绩。
而对于学校来说,分析学科成绩间的内在联系可以为各个专业的课程设置提供重要的参考依据。
在教务工作人员进行排课工作时,可参考对往届学生成绩的分析结果来调整课程的排布顺序,达到不断优化课程设置的目的。
个性化培养和发展是当今高等教育发展的主流方向,以往填鸭式、工厂式的教学方式已经被先进的教育理念所替代,而中国教育制度中流水线式的培养模式一直是一个被广泛诟病、急需解决的问题。
在这一方面,我们可以借鉴发达国家的先进经验,结合我国高等教育发展现状,探索并逐步建立由中国特色的、适合我国国情的个性化培养体系。
目前可以通过对学生的成绩进行挖掘和分析,对学生进行分群,为具有一定共同特征的学生制定个性化培养方案。
基于上述情况,本题目以完善高校培养制度和制定学生个性化培养方案为背景,通过运用数据挖掘及相关专业技术,设计并实现学生成绩分析系统。
1.2 个性化培养的重要意义个性化培养其实并不是一个新颖的概念,2000多年前,我国著名教育家孔子就提出了因材施教的教育理念,并且身体力行地用因材施教的方法教育自己的弟子。
在2000多年后的今天,多元化人才培养是高等教育发展的必然趋势。
个性化培养不仅是实现多元化人才培养最重要的方式,更是我国建立创新型国家战略对高等教育提出的必然需求。
只有摒弃传统的流水线式培养模式,在高等院校中全面推行个性化培养,我国高等教育才能真正完成人才培养方式的革命。
(1)个性化培养是高等教育发展的现实需要个性化教育,是社会对大学生的预期变化的结果。
在当今时代,社会要求每个人都能发挥自己独特而不可替代的作用,教育的使命也悄然转变为激发每一名学生的内在潜能。
在高等院校推行个性化教育是中国高等教育的发展方向,更是世界大多数国家的共识。
随着大学的扩招,原本曲高和寡的高等教育已经走进了普罗大众的生活。
但是大众化的高等教育不等于流水线式的培养,相反普及高等教育的意义正在于让更多的人进入大学,按照自己的兴趣和自身特点选择专业进行学习,成为独特的人才。
(2)个性化培养是创新型国家战略的必然要求2006年,国家制定了建设创新型国家的重大决策。
增强自主创新能力是建设创新型国家战略中最重要的一环,而作为高端人才培养基地的众多高等院校毫无疑问成为了自主创新的排头兵。
受制于特殊的国情和几千年的科举传统,我国目前的教育模式仍然是以死记硬背为主要学习手段,以分数作为评价学生优劣的主要甚至是唯一标准。
在这种教育模式中培养出来的学生中,相当一部分是与社会脱节的、毫无创新能力可言的“考试机器”。