《数据、模型与决策(第13版)》第13章 决策分析
数据模型与决策决策分析教案
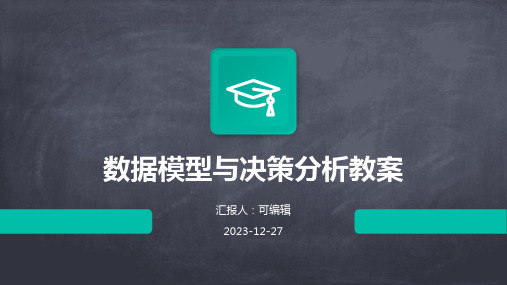
决策分析的步骤
02 确定问题、收集数据、建立模型、分析结果、制定决
策。
决策分析的分类
03
定量决策分析、定性决策分析、结构化决策分析、非
结构化决策分析。
决策分析的常用方法
概率决策分析
基于概率和期望值进行决策的方 法,包括期望值法、概率排序法 等。
多目标决策分析
处理多个相互冲突的目标的决策 问题,常用的方法有层次分析法 、多属性效用函数等。
,提高销售业绩。
案例二:基于数据模型的金融风险评估
总结词:通过数据模型识别和评估潜在 的金融风险
根据模型结果制定风险管理策略,如资 产配置、止损点设置等,以降低潜在损 失。
利用数据模型分析市场波动、相关性等 风险因素。
详细描述
收集各类金融数据,包括股票价格、债 券收益率、汇率等。
案例三:基于数据模型的企业战略规划
练习1
练习2
利用SPSS软件,对给定的市场调查数据进行分析 ,建立分类数据模型,预测目标市场的客户群体。
利用Excel或其他数据分析工具,对给定的 销售数据建立数据模型,并基于该模型进行 销售预测。
练习3
利用Python编程语言,对给定的股票价格 数据建立时间序列模型,预测股票价格的走 势。
思考题
思考题1
在决策分析中,如何选择合适的数据模型? 需要考虑哪些因素?
思考题2
数据模型在决策分析中的作用是什么?如何 评估数据模型的有效性?
思考题3
如何将数据模型与实际业务场景相结合,提 高决策的准确性和效率?
思考题4
在决策分析中,如何处理不确定性因素?如 何利用数据模型进行风险评估?
THANKS
感谢观看
物理数据模型
数据模型与决策ppt课件
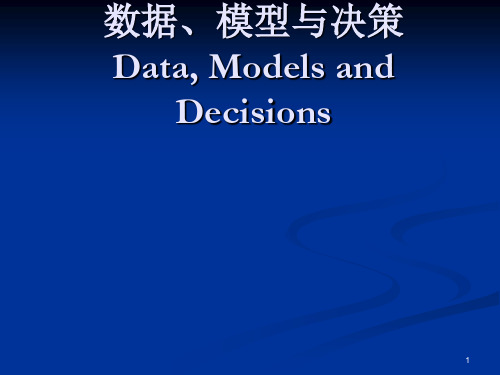
2. 选择目标数据,用鼠标选定B4:D8 ,单击 “系列”卡片,点击“下一步”。
28
3. 输入系列名称,用鼠标选定“分类(X)轴标志” A5:A8 ,单击“下一步”
29
4. 输入或修改“标题”、“坐标轴”、“网 格线”、“图例”、“数据标志”、“数 据表”等属性,单击“下一步”。
30
5. 选择图表位置,单击“完成”。
例3.2 博客调查 (/blogsurvey/thebloggi
ngiceberg.html) Perseus Development 公司在其网页上发布了
一项关于博客的调查报告。该调查根据8个 博客服务商提供的博客用户资料,得到了 各年龄段的人创建的博客数,频数频率分 布表3显示了调查结果。
判断失误 察觉得晚 判断失误 驾驶错误
察觉得晚 判断失误 酒后或疲劳驾驶 判断失误 察觉得晚
偏离规定的行驶 路线 判断失误 察觉得晚 驾驶错误 酒后或疲劳驾驶
察觉得晚 察觉得晚 察觉得晚 驾驶错误 察觉得晚
判断失误
判断失误 驾驶错误 驾驶错误 察觉得晚
判断失误 判断失误 判断失误 察觉得晚 察觉得晚
数据、模型与决策 Data, Models and
Decisions
1
1、主要讲授课程: 计量经济学、运筹学、经济预测与决策技
术 2、主要研究方向: 多元统计 半参数统计中估计问题 因果效应推断
2
本课程的主要内容
数据分析的基本内容 计量模型的基本方法 预测与决策的基本技术 软件的简单应用
18
我们从表1很容易看出: 哪些因素是比较主要的因素? 各因素之间频率的差异有多大? 等等。 有时,累积频率也需要在频数频率分布表
数据模型与决策管理科学篇原书第十三版课程设计
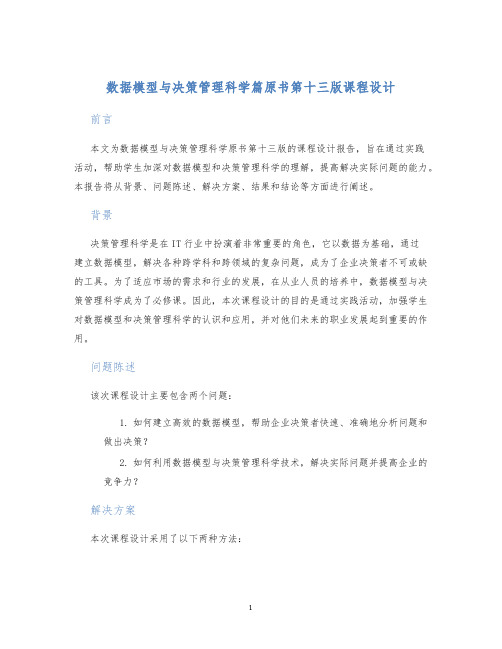
数据模型与决策管理科学篇原书第十三版课程设计前言本文为数据模型与决策管理科学原书第十三版的课程设计报告,旨在通过实践活动,帮助学生加深对数据模型和决策管理科学的理解,提高解决实际问题的能力。
本报告将从背景、问题陈述、解决方案、结果和结论等方面进行阐述。
背景决策管理科学是在IT行业中扮演着非常重要的角色,它以数据为基础,通过建立数据模型,解决各种跨学科和跨领域的复杂问题,成为了企业决策者不可或缺的工具。
为了适应市场的需求和行业的发展,在从业人员的培养中,数据模型与决策管理科学成为了必修课。
因此,本次课程设计的目的是通过实践活动,加强学生对数据模型和决策管理科学的认识和应用,并对他们未来的职业发展起到重要的作用。
问题陈述该次课程设计主要包含两个问题:1.如何建立高效的数据模型,帮助企业决策者快速、准确地分析问题和做出决策?2.如何利用数据模型与决策管理科学技术,解决实际问题并提高企业的竞争力?解决方案本次课程设计采用了以下两种方法:理论授课理论授课是本次课程设计的基础,其中包括了数据模型的定义、分类、建模方法以及相关软件的应用等。
这些知识将为学生在实践活动中提供必要的理论支持。
项目实践项目实践是本次课程设计的重点。
课程设计中既包括了实际案例的分析,又包括了实际生产应用以及各种实验模拟。
项目实践的具体内容包括以下三个部分:案例分析学生需要从实际生产、市场、网站等来源中选取一个实际问题进行分析。
通过了解和收集资料,学生需要对问题进行概括和描述,建立数学模型,并利用相应的软件进行模拟和验证,得出准确的结果和决策建议。
同时,学生还需分析影响因素,制定最优解决方案,并考虑可行性和实施状况。
实验模拟课程设计中包括了一系列实验模拟,旨在让学生对课程内容有更深入、更全面的理解。
具体实验包括:•构建数学模型•建立数据表、查询和报表•利用图表展示数据•模拟决策流程生产应用在课程设计中,学生将利用现有的软件工具对实际生产问题进行分析,以提高企业的竞争力。
数据、模型与决策(运筹学)课后习题和案例答案013
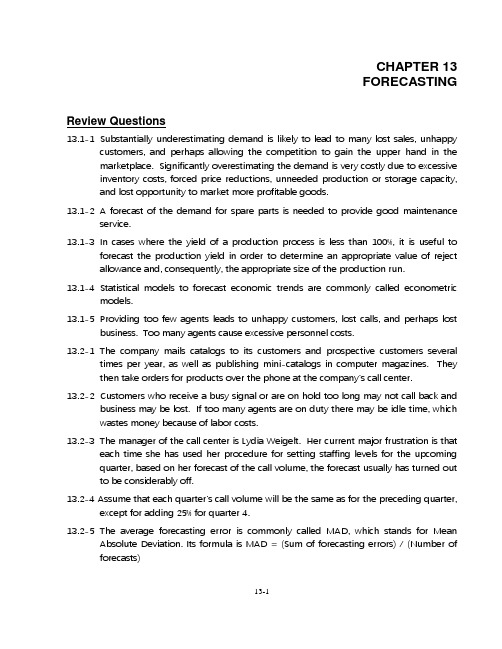
CHAPTER 13FORECASTINGReview Questions13.1-1 Substantially underestimating demand is likely to lead to many lost sales, unhappycustomers, and perhaps allowing the competition to gain the upper hand in the marketplace. Significantly overestimating the demand is very costly due to excessive inventory costs, forced price reductions, unneeded production or storage capacity, and lost opportunity to market more profitable goods.13.1-2 A forecast of the demand for spare parts is needed to provide good maintenanceservice.13.1-3 In cases where the yield of a production process is less than 100%, it is useful toforecast the production yield in order to determine an appropriate value of reject allowance and, consequently, the appropriate size of the production run.13.1-4 Statistical models to forecast economic trends are commonly called econometricmodels.13.1-5 Providing too few agents leads to unhappy customers, lost calls, and perhaps lostbusiness. Too many agents cause excessive personnel costs.13.2-1 The company mails catalogs to its customers and prospective customers severaltimes per year, as well as publishing mini-catalogs in computer magazines. They then take orders for products over the phone at the company’s call center.13.2-2 Customers who receive a busy signal or are on hold too long may not call back andbusiness may be lost. If too many agents are on duty there may be idle time, which wastes money because of labor costs.13.2-3 The manager of the call center is Lydia Weigelt. Her current major frustration is thateach time she has used her procedure for setting staffing levels for the upcoming quarter, based on her forecast of the call volume, the forecast usually has turned out to be considerably off.13.2-4 Assume that each quarter’s cal l volume will be the same as for the preceding quarter,except for adding 25% for quarter 4.13.2-5 The average forecasting error is commonly called MAD, which stands for MeanAbsolute Deviation. Its formula is MAD = (Sum of forecasting errors) / (Number of forecasts)13.2-6 MSE is the mean square error. Its formula is (Sum of square of forecasting errors) /(Number of forecasts).13.2-7 A time series is a series of observations over time of some quantity of interest.13.3-1 In general, the seasonal factor for any period of a year measures how that periodcompares to the overall average for an entire year.13.3-2 Seasonally adjusted call volume = (Actual call volume) / (Seasonal factor).13.3-3 Actual forecast = (Seasonal factor)(Seasonally adjusted forecast)13.3-4 The last-value forecasting method sometimes is called the naive method becausestatisticians consider it naive to use just a sample size of one when additional relevant data are available.13.3-5 Conditions affecting the CCW call volume were changing significantly over the pastthree years.13.3-6 Rather than using old data that may no longer be relevant, this method averages thedata for only the most recent periods.13.3-7 This method modifies the moving-average method by placing the greatest weighton the last value in the time series and then progressively smaller weights on the older values.13.3-8 A small value is appropriate if conditions are remaining relatively stable. A largervalue is needed if significant changes in the conditions are occurring relatively frequently.13.3-9 Forecast = α(Last Value) + (1 –α)(Last forecast). Estimated trend is added to thisformula when using exponential smoothing with trend.13.3-10 T he one big factor that drives total sales up or down is whether there are any hotnew products being offered.13.4-1 CB Predictor uses the raw data to provide the best fit for all these inputs as well asthe forecasts.13.4-2 Each piece of data should have only a 5% chance of falling below the lower line and a5% chance of rising above the upper line.13.5-1 The next value that will occur in a time series is a random variable.13.5-2 The goal of time series forecasting methods is to estimate the mean of theunderlying probability distribution of the next value of the time series as closely as possible.13.5-3 No, the probability distribution is not the same for every quarter.13.5-4 Each of the forecasting methods, except for the last-value method, placed at leastsome weight on the observations from Year 1 to estimate the mean for each quarter in Year 2. These observations, however, provide a poor basis for estimating the mean of the Year 2 distribution.13.5-5 A time series is said to be stable if its underlying probability distribution usuallyremains the same from one time period to the next. A time series is unstable if both frequent and sizable shifts in the distribution tend to occur.13.5-6 Since sales drive call volume, the forecasting process should begin by forecastingsales.13.5-7 The major components are the relatively stable market base of numerous small-niche products and each of a few major new products.13.6-1 Causal forecasting obtains a forecast of the quantity of interest by relating it directlyto one or more other quantities that drive the quantity of interest.13.6-2 The dependent variable is call volume and the independent variable is sales.13.6-3 When doing causal forecasting with a single independent variable, linear regressioninvolves approximating the relationship between the dependent variable and the independent variable by a straight line.13.6-4 In general, the equation for the linear regression line has the form y = a + bx. Ifthere is more than one independent variable, then this regression equation has a term, a constant times the variable, added on the right-hand side for each of these variables.13.6-5 The procedure used to obtain a and b is called the method of least squares.13.6-6 The new procedure gives a MAD value of only 120 compared with the old MADvalue of 400 with the 25% rule.13.7-1 Statistical forecasting methods cannot be used if no data are available, or if the dataare not representative of current conditions.13.7-2 Even when good data are available, some managers prefer a judgmental methodinstead of a formal statistical method. In many other cases, a combination of the two may be used.13.7-3 The jury of executive opinion method involves a small group of high-level managerswho pool their best judgment to collectively make a forecast rather than just the opinion of a single manager.13.7-4 The sales force composite method begins with each salesperson providing anestimate of what sales will be in his or her region.13.7-5 A consumer market survey is helpful for designing new products and then indeveloping the initial forecasts of their sales. It is also helpful for planning a marketing campaign.13.7-6 The Delphi method normally is used only at the highest levels of a corporation orgovernment to develop long-range forecasts of broad trends.13.8-1 Generally speaking, judgmental forecasting methods are somewhat more widelyused than statistical methods.13.8-2 Among the judgmental methods, the most popular is a jury of executive opinion.Manager’s opinion is a close second.13.8-3 The survey indicates that the moving-average method and linear regression are themost widely used statistical forecasting methods.Problems13.1 a) Forecast = last value = 39b) Forecast = average of all data to date = (5 + 17 + 29 + 41 + 39) / 5 = 131 / 5 =26c) Forecast = average of last 3 values = (29 + 41 + 39) / 3 = 109 / 3 = 36d) It appears as if demand is rising so the average forecasting method seemsinappropriate because it uses older, out-of-date data.13.2 a) Forecast = last value = 13b) Forecast = average of all data to date = (15 + 18 + 12 + 17 + 13) / 5 = 75 / 5 =15c) Forecast = average of last 3 values = (12 + 17 + 13) / 3 = 42 / 3 = 14d) The averaging method seems best since all five months of data are relevant indetermining the forecast of sales for next month and the data appears relativelystable.13.3MAD = (Sum of forecasting errors) / (Number of forecasts) = (18 + 15 + 8 + 19) / 4 = 60 / 4 = 15 MSE = (Sum of squares of forecasting errors) / (Number of forecasts) = (182 + 152 + 82 + 192) / 4 = 974 / 4 = 243.513.4 a) Method 1 MAD = (258 + 499 + 560 + 809 + 609) / 5 = 2,735 / 5 = 547Method 2 MAD = (374 + 471 + 293 + 906 + 396) / 5 = 2,440 / 5 = 488Method 1 MSE = (2582 + 4992 + 5602 + 8092 + 6092) / 5 = 1,654,527 / 5 = 330,905Method 2 MSE = (3742 + 4712 + 2932 + 9062 + 3962) / 5 = 1,425,218 / 5 = 285,044Method 2 gives a lower MAD and MSE.b) She can use the older data to calculate more forecasting errors and compareMAD for a longer time span. She can also use the older data to forecast theprevious five months to see how the methods compare. This may make her feelmore comfortable with her decision.13.5 a)b)c)d)13.6 a)b)This progression indicatesthat the state’s economy is improving with the unemployment rate decreasing from 8% to 7% (seasonally adjusted) over the four quarters.13.7 a)b) Seasonally adjusted value for Y3(Q4)=28/1.04=27,Actual forecast for Y4(Q1) = (27)(0.84) = 23.c) Y4(Q1) = 23 as shown in partb Seasonally adjusted value for Y4(Q1) = 23 / 0.84 = 27 Actual forecast for Y4(Q2) = (27)(0.92) = 25Seasonally adjusted value for Y4(Q2) = 25 / 0.92 = 27 Actual forecast for Y4(Q3) = (27)(1.20) = 33Seasonally adjusted value for Y4(Q3) = 33/1.20 = 27Actual forecast for Y4(Q4) = (27)(1.04) = 28d)13.8 Forecast = 2,083 – (1,945 / 4) + (1,977 / 4) = 2,09113.9 Forecast = 782 – (805 / 3) + (793 / 3) = 77813.10 Forecast = 1,551 – (1,632 / 10) + (1,532 / 10) = 1,54113.11 Forecast(α) = α(last value) + (1 –α)(last forecast)Forecast(0.1) = (0.1)(792) + (1 –0.1)(782) = 783 Forecast(0.3) = (0.3)(792) + (1 –0.3)(782) = 785 Forecast(0.5) = (0.5)(792) + (1 – 0.5)(782) = 78713.12 Forecast(α) = α(last value) + (1 –α)(last forecast)Forecast(0.1) = (0.1)(1,973) + (1 –0.1)(2,083) = 2,072 Forecast(0.3) = (0.3)(1,973) + (1 –0.3)(2,083) = 2,050 Forecast(0.5) = (0.5)(1,973) + (1 – 0.5)(2,083) = 2,02813.13 a) Forecast(year 1) = initial estimate = 5000Forecast(year 2) = α(last value) + (1 –α)(last forecast)= (0.25)(4,600) + (1 –0.25)(5,000) = 4,900 Forecast(year 3) = (0.25)(5,300) + (1 – 0.25)(4,900) = 5,000b) MAD = (400 + 400 + 1,000) / 3 = 600MSE = (4002 + 4002 + 1,0002) / 3 = 440,000c) Forecast(next year) = (0.25)(6,000) + (1 – 0.25)(5,000) = 5,25013.14 Forecast = α(last value) + (1 –α)(last forecast) + Estimated trendEstimated trend = β(Latest trend) + (1 –β)(Latest estimate of trend) Latest trend = α(Last value – Next-to-last value) + (1 –α)(Last forecast – Next-to-last forecast)Forecast(year 1) = Initial average + Initial trend = 3,900 + 700 = 4,600Forecast (year 2) = (0.25)(4,600) + (1 –0.25)(4,600)+(0.25)[(0.25)(4,600 –3900) + (1 –0.25)(4,600 –3,900)] + (1 –0.25)(700) = 5,300Forecast (year 3) = (0.25)(5,300) + (1 – 0.25)(5,300) + (0.25)[(0.25)(5,300 – 4,600) + (1 – 0.25)(5,300 – 4,600)]+(1 – 0.25)(700) = 6,00013.15 Forecast = α(last value) + (1 –α)(last forecast) + Estimated trendEstimated trend = β(Latest trend) + (1 –β)(Latest estimate of trend) Latest trend = α(Last value – Next-to-last value) + (1 –α)(Last forecast – Next-to-last forecast)Forecast = (0.2)(550) + (1 – 0.2)(540) + (0.3)[(0.2)(550 – 535) + (1 – 0.2)(540 –530)] + (1 – 0.3)(10) = 55213.16 Forecast = α(last value) + (1 –α)(last forecast) + Estimated trendEstimated trend = β(Latest trend) + (1 –β)(Latest estimate of trend) Latest trend = α(Last value – Next-to-last value) + (1 –α)(Last forecast – Next-to-last forecast)Forecast = (0.1)(4,935) + (1 – 0.1)(4,975) + (0.2)[(0.1)(4,935 – 4,655) + (1 – 0.1) (4,975 – 4720)] + (1 – 0.2)(240) = 5,21513.17 a) Since sales are relatively stable, the averaging method would be appropriate forforecasting future sales. This method uses a larger sample size than the last-valuemethod, which should make it more accurate and since the older data is stillrelevant, it should not be excluded, as would be the case in the moving-averagemethod.b)c)d)e) Considering the MAD values (5.2, 3.0, and 3.9, respectively), the averagingmethod is the best one to use.f) Considering the MSE values (30.6, 11.1, and 17.4, respectively), the averagingmethod is the best one to use.g) Unless there is reason to believe that sales will not continue to be relatively stable,the averaging method should be the most accurate in the future as well.13.18 Using the template for exponential smoothing, with an initial estimate of 24, thefollowing forecast errors were obtained for various values of the smoothing constant α:use.13.19 a) Answers will vary. Averaging or Moving Average appear to do a better job thanLast Value.b) For Last Value, a change in April will only affect the May forecast.For Averaging, a change in April will affect all forecasts after April.For Moving Average, a change in April will affect the May, June, and July forecast.c) Answers will vary. Averaging or Moving Average appear to do a slightly better jobthan Last Value.d) Answers will vary. Averaging or Moving Average appear to do a slightly better jobthan Last Value.13.20 a) Since the sales level is shifting significantly from month to month, and there is noconsistent trend, the last-value method seems like it will perform well. Theaveraging method will not do as well because it places too much weight on olddata. The moving-average method will be better than the averaging method butwill lag any short-term trends. The exponential smoothing method will also lagtrends by placing too much weight on old data. Exponential smoothing withtrend will likely not do well because the trend is not consistent.b)Comparing MAD values (5.3, 10.0, and 8.1, respectively), the last-value method is the best to use of these three options.Comparing MSE values (36.2, 131.4, and 84.3, respectively), the last-value method is the best to use of these three options.c) Using the template for exponential smoothing, with an initial estimate of 120, thefollowing forecast errors were obtained for various values of the smoothingconstant α:constant is appropriate.d) Using the template for exponential smoothing with trend, using initial estimates of120 for the average value and 10 for the trend, the following forecast errors wereobtained for various values of the smoothing constants α and β:constants is appropriate.e) Management should use the last-value method to forecast sales. Using thismethod the forecast for January of the new year will be 166. Exponentialsmoothing with trend with high smoothing constants (e.g., α = 0.5 and β = 0.5)also works well. With this method, the forecast for January of the new year will be165.13.21 a) Shift in total sales may be due to the release of new products on top of a stableproduct base, as was seen in the CCW case study.b) Forecasting might be improved by breaking down total sales into stable and newproducts. Exponential smoothing with a relatively small smoothing constant canbe used for the stable product base. Exponential smoothing with trend, with arelatively large smoothing constant, can be used for forecasting sales of each newproduct.c) Managerial judgment is needed to provide the initial estimate of anticipated salesin the first month for new products. In addition, a manger should check theexponential smoothing forecasts and make any adjustments that may benecessary based on knowledge of the marketplace.13.22 a) Answers will vary. Last value seems to do the best, with exponential smoothingwith trend a close second.b) For last value, a change in April will only affect the May forecast.For averaging, a change in April will affect all forecasts after April.For moving average, a change in April will affect the May, June, and July forecast.For exponential smoothing, a change in April will affect all forecasts after April.For exponential smoothing with trend, a change in April will affect all forecastsafter April.c) Answers will vary. last value or exponential smoothing seem to do better than theaveraging or moving average.d) Answers will vary. last value or exponential smoothing seem to do better than theaveraging or moving average.13.23 a) Using the template for exponential smoothing, with an initial estimate of 50, thefollowing MAD values were obtained for various values of the smoothing constantα:Choose αb) Using the template for exponential smoothing, with an initial estimate of 50, thefollowing MAD values were obtained for various values of the smoothing constantα:Choose αc) Using the template for exponential smoothing, with an initial estimate of 50, thefollowing MAD values were obtained for various values of the smoothing constantα:13.24 a)b)Forecast = 51.c) Forecast = 54.13.25 a) Using the template for exponential smoothing with trend, with an initial estimatesof 50 for the average and 2 for the trend and α = 0.2, the following MAD values were obtained for various values of the smoothing constant β:Choose β = 0.1b) Using the template for exponential smoothing with trend, with an initial estimatesof 50 for the average and 2 for the trend and α = 0.2, the following MAD valueswere obtained for various values of the smoothing constant β:Choose β = 0.1c) Using the template for exponential smoothing with trend, with an initial estimatesof 50 for the average and 2 for the trend and α = 0.2, the following MAD valueswere obtained for various values of the smoothing constant β:13.26 a)b)0.582. Forecast = 74.c) = 0.999. Forecast = 79.13.27 a) The time series is not stable enough for the moving-average method. Thereappears to be an upward trend.b)c)d)e) Based on the MAD and MSE values, exponential smoothing with trend should beused in the future.β = 0.999.f)For exponential smoothing, the forecasts typically lie below the demands.For exponential smoothing with trend, the forecasts are at about the same level as demand (perhaps slightly above).This would indicate that exponential smoothing with trend is the best method to usehereafter.13.2913.30 a)factors:b)c) Winter = (49)(0.550) = 27Spring = (49)(1.027) = 50Summer = (49)(1.519) = 74Fall = (49)(0.904) = 44d)e)f)g) The exponential smoothing method results in the lowest MAD value (1.42) and thelowest MSE value (2.75).13.31 a)b)c)d)e)f)g) The last-value method with seasonality has the lowest MAD and MSE value. Usingthis method, the forecast for Q1 is 23 houses.h) Forecast(Q2) = (27)(0.92) = 25Forecast(Q3) = (27)(1.2) = 32Forecast(Q4) = (27)(1.04) = 2813.32 a)b) The moving-average method with seasonality has the lowest MAD value. Using13.33 a)b)c)d) Exponential smoothing with trend should be used.e) The best values for the smoothing constants are α = 0.3, β = 0.3, and γ = 0.001.C28:C38 below.13.34 a)b)c)d)e) Moving average results in the best MAD value (13.30) and the best MSE value(249.09).f)MAD = 14.17g) Moving average performed better than the average of all three so it should beused next year.h) The best method is exponential smoothing with seasonality and trend, using13.35 a)••••••••••0100200300400500600012345678910S a l e sMonthb)c)••••••••••0100200300400500600012345678910S a l e sMonthd) y = 410.33 + (17.63)(11) = 604 e) y = 410.33 + (17.63)(20) = 763f) The average growth in sales per month is 17.63.13.36 a)•••01000200030004000500060000123A p p l i c a t i o n sYearb)•••01000200030004000500060000123A p p l i c a t i o n sYearc)d) y (year 4) = 3,900+ (700)(4) = 6,700 y (year 5) = 3,900 + (700)(5) = 7,400 y (year 6) = 3,900 + (700)(6) = 8,100 y (year 7) = 3,900 + (700)(7) =8,800y (year 8) = 3,900 + (700)(8) = 9,500e) It does not make sense to use the forecast obtained earlier of 9,500. Therelationship between the variable has changed and, thus, the linear regression that was used is no longer appropriate.f)•••••••0100020003000400050006000700001234567A p p l i c a t i o n sYeary =5,229 +92.9x y =5,229+(92.9)(8)=5,971the forecast that it provides for year 8 is not likely to be accurate. It does not make sense to continue to use a linear regression line when changing conditions cause a large shift in the underlying trend in the data.g)Causal forecasting takes all the data into account, even the data from before changing conditions cause a shift. Exponential smoothing with trend adjusts to shifts in the underlying trend by placing more emphasis on the recent data.13.37 a)••••••••••50100150200250300350400450500012345678910A n n u a l D e m a n dYearb)c)••••••••••50100150200250300350400450500012345678910A n n u a l D e m a n dYeard) y = 380 + (8.15)(11) = 470 e) y = 380 = (8.15)(15) = 503f) The average growth per year is 8.15 tons.13.38 a) The amount of advertising is the independent variable and sales is the dependentvariable.b)•••••0510*******100200300400500S a l e s (t h o u s a n d s o f p a s s e n g e r s )Amount of Advertising ($1,000s)c)•••••0510*******100200300400500S a l e s (t h o u s a n d s o f p a s s e n g e r s )Amount of Advertising ($1,000s)d) y = 8.71 + (0.031)(300) = 18,000 passengers e) 22 = 8.71 + (0.031)(x ) x = $429,000f) An increase of 31 passengers can be attained.13.39 a) If the sales change from 16 to 19 when the amount of advertising is 225, then thelinear regression line shifts below this point (the line actually shifts up, but not as much as the data point has shifted up).b) If the sales change from 23 to 26 when the amount of advertising is 450, then the linear regression line shifts below this point (the line actually shifts up, but not as much as the data point has shifted up).c) If the sales change from 20 to 23 when the amount of advertising is 350, then the linear regression line shifts below this point (the line actually shifts up, but not as much as the data point has shifted up).13.40 a) The number of flying hours is the independent variable and the number of wingflaps needed is the dependent variable.b)••••••024*********100200W i n g F l a p s N e e d e dFlying Hours (thousands)c)d)••••••024*********100200W i n g F l a p s N e e d e dFlying Hours (thousands)e) y = -3.38 + (0.093)(150) = 11f) y = -3.38 + (0.093)(200) = 1513.41 Joe should use the linear regression line y = –9.95 + 0.10x to develop a forecast forCase13.1 a) We need to forecast the call volume for each day separately.1) To obtain the seasonally adjusted call volume for the past 13 weeks, we firsthave to determine the seasonal factors. Because call volumes follow seasonalpatterns within the week, we have to calculate a seasonal factor for Monday,Tuesday, Wednesday, Thursday, and Friday. We use the Template for SeasonalFactors. The 0 values for holidays should not factor into the average. Leaving themblank (rather than 0) accomplishes this. (A blank value does not factor into theAVERAGE function in Excel that is used to calculate the seasonal values.) Using thistemplate (shown on the following page, the seasonal factors for Monday, Tuesday,Wednesday, Thursday, and Friday are 1.238, 1.131, 0.999, 0.850, and 0.762,respectively.2) To forecast the call volume for the next week using the last-value forecasting method, we need to use the Last Value with Seasonality template. To forecast the next week, we need only start with the last Friday value since the Last Value method only looks at the previous day.The forecasted call volume for the next week is 5,045 calls: 1,254 calls are received on Monday, 1,148 calls are received on Tuesday, 1,012 calls are received on Wednesday, 860 calls are received on Thursday, and 771 calls are received on Friday.3) To forecast the call volume for the next week using the averaging forecasting method, we need to use the Averaging with Seasonality template.The forecasted call volume for the next week is 4,712 calls: 1,171 calls are received on Monday, 1,071 calls are received on Tuesday, 945 calls are received on Wednesday, 804 calls are received on Thursday, and 721 calls are received onFriday.4) To forecast the call volume for the next week using the moving-average forecasting method, we need to use the Moving Averaging with Seasonality template. Since only the past 5 days are used in the forecast, we start with Monday of the last week to forecast through Friday of the next week.The forecasted call volume for the next week is 4,124 calls: 985 calls are received on Monday, 914 calls are received on Tuesday, 835 calls are received on Wednesday, 732 calls are received on Thursday, and 658 calls are received on Friday.5) To forecast the call volume for the next week using the exponential smoothing forecasting method, we need to use the Exponential with Seasonality template. We start with the initial estimate of 1,125 calls (the average number of calls on non-holidays during the previous 13 weeks).The forecasted call volume for the next week is 4,322 calls: 1,074 calls are received on Monday, 982 calls are received on Tuesday, 867 calls are received onWednesday, 737 calls are received on Thursday, and 661 calls are received on Friday.b) To obtain the mean absolute deviation for each forecasting method, we simplyneed to subtract the true call volume from the forecasted call volume for each day in the sixth week. We then need to take the absolute value of the five differences.Finally, we need to take the average of these five absolute values to obtain the mean absolute deviation.1) The spreadsheet for the calculation of the mean absolute deviation for the last-value forecasting method follows.This method is the least effective of the four methods because this method depends heavily upon the average seasonality factors. If the average seasonality factors are not the true seasonality factors for week 6, a large error will appear because the average seasonality factors are used to transform the Friday call volume in week 5 to forecasts for all call volumes in week 6. We calculated in part(a) that the call volume for Friday is 0.762 times lower than the overall average callvolume. In week 6, however, the call volume for Friday is only 0.83 times lower than the average call volume over the week. Also, we calculated that the call volume for Monday is 1.34 times higher than the overall average call volume. In Week 6, however, the call volume for Monday is only 1.21 times higher than the average call volume over the week. These differences introduce a large error.。
数据模型与决策完整

数据,模型和决策第一章决策分析一、比尔.桑普拉斯的夏季打工决策一个决策树模型及分析比尔. 桑普拉斯(bill Sampras) 在麻省理工学院的斯隆管理学院就读第一学期,已经是第三周了。
除了花在准备功课上的时间外,bill开场认真考虑有关明年夏季打工的事情,特别是该决策在几周后必须做出。
8月底,在bill飞往波士顿的途中,他坐在vanessa Parker 的旁边,并与她就双方感兴趣的问题进展了交谈。
vanessa 是一个重要的商业投资银行有关资产预算的副总裁。
在飞机到达波士顿后,vanessa坦率地告诉bill,她愿意考虑明年夏季雇佣bill的可能性,并希望在她的公司于11月中旬开场进展的夏季招聘方案时,请bill直接与她联系。
bill感觉到自己的经历和所具有的风度给vanessa留下了很深的印象〔bill曾经在一个财富500强公司的金融部门就来自税收业务的额外现金的短期投资工作过4年〕。
当bill 8月离开公司去攻读MBA时,他的老板john Mason把他叫到一边,对他许诺,到第二年夏季可以雇佣他。
夏季回到公司进展为期12个星期的打工薪水将是12000美元。
但john也告诉bill夏季工作招聘期限仅到10月底有效。
因此,bill在得到vanessa提供夏季工作的细节之前,必须决定是否承受john的工作。
vanessa已经解释,她的公司在11月中旬之前不愿意讨论夏季工作方案的细节。
如果bill回绝john的好意,bill要么承受vanessa的提供〔如果vanessa承受bill的申请〕,要么通过参加斯隆管理学院在1月和2月举办的公司夏季招聘方案中,寻找另一个夏季工作时机。
决策准那么假设bill认为所有的夏季工作时机〔为john工作,为vanessa工作和参加斯隆学院的夏季打工方案〕都将会给bill提供类似的学习、交流以及丰富经历的时机。
那么,bill判断夏季工作时机的优劣的唯一标准就是工作的薪水,以薪水越高越好。
《数据模型与决策》课件
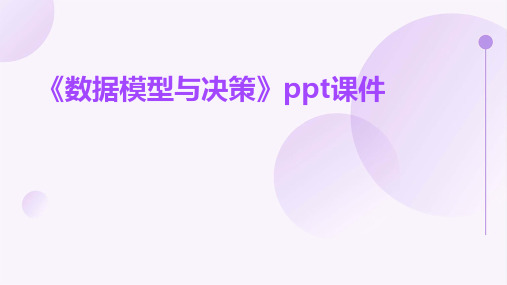
04
实际案例分析
案例一:基于数据模型的营销决策
总结词
通过数据模型分析市场趋势,制定有效的营销策略。
详细描述
利用大数据和统计模型分析消费者行为和市场趋势,预 测未来市场需求,制定个性化的营销策略,提高销售业 绩和市场占有率。
总结词
优化营销预算分配。
详细描述
通过数据分析确定各营销渠道的投资回报率,合理分配 营销预算,提高营销效果和投资回报率。
03
未来还需要加强数据安全和隐 私保护等方面的研究,以保障 数据的安全性和可靠性。
数据模型与决策的实际应用价值
数据模型与决策在企业管理 中具有重要的应用价值,可 以帮助企业进行科学决策和
优化资源配置。
数据模型与决策还可以帮助 企业提高市场竞争力,如通 过数据分析发现市场趋势和 消费者需求,制定更加精准
总结词
提升客户满意度和忠诚度。
详细描述
通过数据模型分析客户反馈和行为数据,了解客户需求 和期望,优化产品和服务,提高客户满意度和忠诚度。
案例二:基于数据模型的金融风险评估
总结词
利用数据模型评估贷款违约风险。
01
02
详细描述
通过分析历史数据和信贷信息,利用统计模 型和机器学习算法评估贷款违约风险,为金 融机构提供风险预警和决策支持。
数据模型在决策中的作用
数据模型为决策提供数据支持
通过建立数据模型,将原始数据转化为有价值的信息,帮助决策者 更好地理解数据,从而做出更准确的决策。
数据模型提高决策效率
数据模型可以对大量数据进行处理和分析,快速得出结果,提高决 策效率。
数据模型降低决策风险
通过数据模型的预测和模拟功能,可以预测未来趋势,帮助决策者 提前做好准备,降低决策风险。
《决策分析模型》课件

,并建立相应的数学模型。
这些模型可以用来预测结果、评估风险和不确定性,以及制定
03
最优的决策策略。
决策分析模型的作用
01 帮助决策者更好地理解和分析问题,明确 问题的结构和关键因素。
02
提供一种系统化的方法来比较不同方案和 结果,以便做出更明智的决策。
03
通过预测和评估,减少决策中的风险和不 确定性。
多目标决策模型的步骤
确定目标
明确决策需要优化的目标,并确 保目标的可衡量性和可比较性。
收集数据
收集与各目标相关的数据和信息 ,为决策提供依据。
权重分配
根据各目标的相对重要性,为其 分配相应的权重。
结果评估
对求解结果进行评估,分析各目 标的权衡和取舍。
求解模型
运用适当的求解方法,得出最优 解或满意解。
04
促进团队内部的沟通和协作,达成共识和 支持决策的实施。
决策分析模型的分类
01
根据所涉及的决策类型,决策 分析模型可以分为确定型、风 险型和不确定型。
02
根据所处理问题的性质,决策 分析模型可以分为静态和动态 模型。
03
根据所使用的数学方法和技术 ,决策分析模型可以分为线性 规划、非线性规划、动态规划 、概率论和统计方法等。
市场营销
在市场营销中,风险决策模型可用于市场调查、预测销售 量、制定营销策略等方面,帮助企业更好地把握市场机遇 和应对竞争挑战。
项目管理
在项目管理中,风险决策模型可以帮助项目经理识别项目 潜在的风险,制定相应的应对措施,确保项目的顺利实施 。
生产管理
在生产管理中,风险决策模型可用于生产计划的制定、库 存管理、质量控制等方面,提高生产效率和降低生产成本 。
数据模型与决策决策分析ppt教案

难点1:选择合适的数据模 型
难点4:结合实际情况调整 模型和参数
05
教学方法与手段
讲授法:对概念进行讲解
定义:讲授法是 一种以教师讲授 为主,学生被动 接受知识的教学 方法。
特点:讲授法具 有传授知识效率 高、系统性强、 易于控制等优点, 但也存在学生参 与度低、缺乏实 践操作等缺点。
应用场景:在数 据模型与决策分 析课程中,讲授 法可以用于对概 念、原理、方法 等进行讲解,帮 助学生建立基础 知识和理论框架。
YOUR LOGO
THANK YOU
汇报人:
案例分析:对案例进行深入的分析,包括数据的处理、模型的选择、决策的制定等
案例总结:总结案例的主要观点和结论,以及案例对决策分析的启示和意义
04
教学重点与难点
重点:数据模型ห้องสมุดไป่ตู้决策分析的关系
数据模型是决策分 析的基础:介绍数 据模型的概念、作 用和重要性,以及 如何建立有效的数 据模型。
决策分析是数据模 型的应用:介绍决 策分析的概念、方 法和应用场景,以 及如何利用数据模 型进行决策分析。
注意事项:在采 用讲授法时,需 要注意控制讲授 时间,避免过于 冗长或过于简略, 同时要注重与学 生的互动,及时 了解学生的掌握 情况。
讨论法:小组讨论案例分析
讨论法:小组讨论案例分析
案例法:通过实际案例来讲解 数据模型与决策分析
互动式教学法:鼓励学生提问, 进行课堂互动
实践法:通过实践操作来加深 对数据模型与决策分析的理解
提问导入:什么 是数据模型?
引导学生思考: 数据模型与决策 的关系是什么?
介绍数据模型的 概念和分类。
探讨如何利用数 据模型进行决策 分析。
《数据模型与决策》案例分析报告-劳动力安排
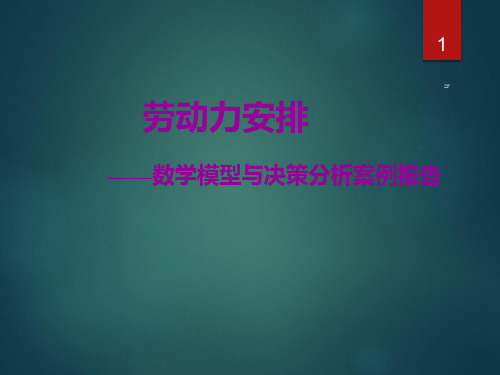
基本思路
6
问题界定: 戴维斯仪器公司劳动力分配问题属于典型的线 性规划问题
解决方案 确定目标函数,采用线性模型求解
模型与使用工具 成本最小化模型 Excel中线性规划求解功能求解
分析过程
7
目标函数:
MinY=2000*Xi1+4800*Xi2+7500*Xi3+875(Xi1+Xi2+Xi3) Xi1:雇用一个月临时工数目 Xi2:雇用二个月临时工数目 Xi3:雇用三个月临时工数目 Y:总花费
0
0
10
0
23
0
19
0
26
20
14
0
112
分析过程
12
项目 费用 人数 合计 总费用
费用统计表 1个月工资/人 2个月工资/人 3个月工资/人
培训费 用
2000
4800
7500
700
112
0
0
112
224000
0
302400
0
78400
分析过程
13
总结:
1、总费用为$302400,比培训费用为$875/人, $减少11125
2000 7
费用统计表
2个月工资/ 3个月工资/
人
人
4800 3
7500 13
全职工 资
15840 10
培训费用 /人
875 33
合计
14000
14400
97500 158400 28875
总费用 313175
从计算结果可以看出,总费用会比全部雇用临时工少$350,因为培训 费用虽然可以减少$ 8750,但是工资却增加$ 8400,所以在培训费用 较高的情况下,多雇用全职员工可减少总费用;在培训费用较低的情 况下,就尽量少雇用全职员工。例如:当培训费用减少至$ 700时, 若雇用10名全职工,总费用将增加$ 5000。
清华大学《数据模型与决策》DMD(孙静) - 课程精髓及案例分析流程
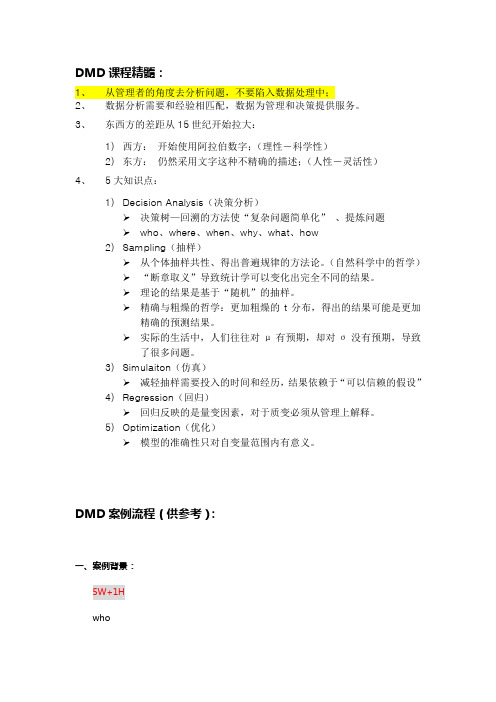
DMD课程精髓:1、从管理者的角度去分析问题,不要陷入数据处理中;2、数据分析需要和经验相匹配,数据为管理和决策提供服务。
3、东西方的差距从15世纪开始拉大:1)西方:开始使用阿拉伯数字;(理性-科学性)2)东方:仍然采用文字这种不精确的描述;(人性-灵活性)4、5大知识点:1)Decision Analysis(决策分析)➢决策树—回溯的方法使“复杂问题简单化”、提炼问题➢who、where、when、why、what、how2)Sampling(抽样)➢从个体抽样共性、得出普遍规律的方法论。
(自然科学中的哲学)➢“断章取义”导致统计学可以变化出完全不同的结果。
➢理论的结果是基于“随机”的抽样。
➢精确与粗燥的哲学:更加粗燥的t分布,得出的结果可能是更加精确的预测结果。
➢实际的生活中,人们往往对μ有预期,却对σ没有预期,导致了很多问题。
3)Simulaiton(仿真)➢减轻抽样需要投入的时间和经历,结果依赖于“可以信赖的假设”4)Regression(回归)➢回归反映的是量变因素,对于质变必须从管理上解释。
5)Optimization(优化)➢模型的准确性只对自变量范围内有意义。
DMD案例流程(供参考):一、案例背景:5W+1Hwhowhenwherewhat (要干什么)why (待分析的原始数据或者解决途径)how (怎样做,D.T)P25-规范的决策树key point:(---总体框架)➢有用的信息和数据(why);➢提炼问题(what:Unkown information and question);二、初步分析:根据决策树建模,即通常是分析框架、一个公式,或者一个目标key point:(清晰分析思路―注意不要陷在数据里,有些可能无解,但要写明原因。
)➢决策思路(D.T)说明是否做敏感性分析,是否另行设计决策树找出其他的解决办法,或从其他角度重新看这个问题-把复杂的问题分解成若干问题,简化问题;➢列出具体的分析思路和步骤;➢在思路基础上,找出相关需要的变量、函数和相互间的关系;例子:(最后一个书商案例)决策变量:P书Q页数Q印刷Q销售目标函数:∏=销售收入-总成本=P书×Q销售-f总成本(Q页数,Q印刷)约束:1 毛利率=1-直接成本/销售收入=1-g直接成本(Q页数,Q印刷)/(P书×Q印刷)>= 40%2 25<= P书<=353 Q销售<= Q印刷4 所有变量>=0P书―――需优化求解Q页数―――已知条件Q印刷―――需回归或仿真Q销售―――需回归或仿真f总成本(Q页数,Q印刷)―――需回归g直接成本(Q页数,Q印刷)―――需回归三、数据处理:key point:(根据初步分析思路,进行数据处理,找出可以符合管理者角度意愿的证据。
《数据、模型与决策》教学大纲.doc
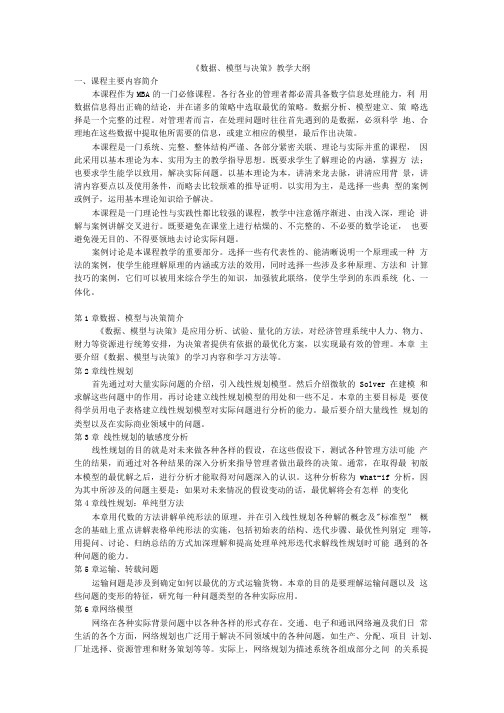
《数据、模型与决策》教学大纲一、课程主要内容简介本课程作为MBA的一门必修课程。
各行各业的管理者都必需具备数字信息处理能力,利用数据信息得出正确的结论,并在诸多的策略中选取最优的策略。
数据分析、模型建立、策略选择是一个完整的过程。
对管理者而言,在处理问题时往往首先遇到的是数据,必须科学地、合理地在这些数据中提取他所需要的信息,或建立相应的模型,最后作出决策。
本课程是一门系统、完整、整体结构严谨、各部分紧密关联、理论与实际并重的课程,因此采用以基本理论为本、实用为主的教学指导思想。
既要求学生了解理论的内涵,掌握方法;也要求学生能学以致用,解决实际问题。
以基本理论为本,讲清来龙去脉,讲清应用背景,讲清内容要点以及使用条件,而略去比较烦难的推导证明。
以实用为主,是选择一些典型的案例或例子,运用基本理论知识给予解决。
本课程是一门理论性与实践性都比较强的课程,教学中注意循序渐进、由浅入深,理论讲解与案例讲解交叉进行。
既要避免在课堂上进行枯燥的、不完整的、不必要的数学论证,也要避免漫无目的、不得要领地去讨论实际问题。
案例讨论是本课程教学的重要部分。
选择一些有代表性的、能清晰说明一个原理或一种方法的案例,使学生能理解原理的内涵或方法的效用,同时选择一些涉及多种原理、方法和计算技巧的案例,它们可以被用来综合学生的知识,加强彼此联络,使学生学到的东西系统化、一体化。
第1章数据、模型与决策简介《数据、模型与决策》是应用分析、试验、量化的方法,对经济管理系统中人力、物力、财力等资源进行统筹安排,为决策者提供有依据的最优化方案,以实现最有效的管理。
本章主要介绍《数据、模型与决策》的学习内容和学习方法等。
第2章线性规划首先通过对大量实际问题的介绍,引入线性规划模型。
然后介绍微软的Solver在建模和求解这些问题中的作用,再讨论建立线性规划模型的用处和一些不足。
本章的主要目标是要使得学员用电子表格建立线性规划模型对实际问题进行分析的能力。
自考30447数据、模型与决策【优质PPT】
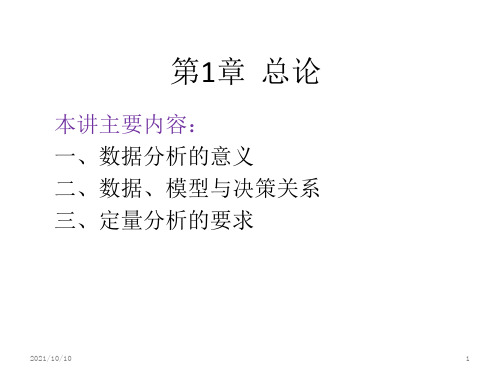
先分层或分类,然后从各层中分别抽取一定数量的个体单位
整群抽样
将总体分成若干群,以群为单位抽样,对抽中的群实行全面调查。
系统抽样
先将总体单位按某种标识进行排列,在规定的范围内随机抽取第一个样本单位,此后按一套规则确定其他样本单位。
阶段抽样
分几个阶段进行,第一阶段抽取一级样本单位,对抽中的一级样本单位从中抽取二级样本单位等。
第2章 数据资料的来源、分类与变换
二、数据资料的分类 2、时空要素分类
时空要素
特点
作用
截面数据
同一时刻上搜集的有关总体各个单位的资料
分析总体内部的构成,了解现象空间范围内差异。
时间序列
按时间先后顺序排列在一起的观察资料
考察同一现象不同时间发展变化过程及其动态变化趋势
面板数据
截面资料和时间序列资料交织在一起的资料
第2章 数据资料的来源、分类与变换
一、数据资料的来源 4、数据资料搜集途径
第2章 数据资料的来源、分类与变换
一、数据资料的来源 4、数据资料搜集途径
第2章 数据资料的来源、分类与变换
一、数据资料的来源 5、不同抽样方式下的样本单位确定
抽样调查组织方式
样本单位的确定
简单抽样
每个单位都有等同的可能性被抽到
第3章 统计资料描述分析
三、数据分析的特征数字 (二)定量数据的特征数字 2、定量数据集中趋势特征数字的综合应用 (3)马尔可夫不等式 为一组非负观察值, 为算术平均数, k为大于1常系数,则有 表明某个数值不小于算术平均数k倍的概率不会超过1/k 。
第3章 统计资料描述分析
第1章 总论
本讲主要内容: 一、数据分析的意义 二、数据、模型与决策关系 三、定量分析的要求
《数据、模型与决策》PPT
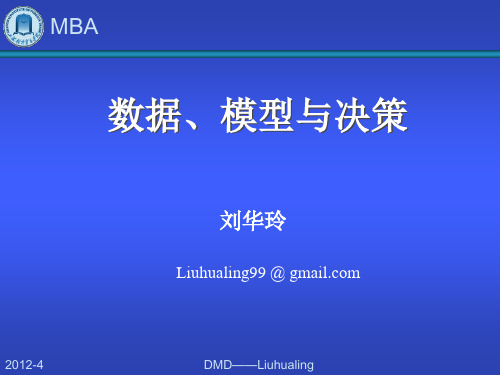
2012-4
DMD——Liuhualing
MBA
经典管理科学获奖应用
联合航空公司(1-2/1986,$600万) 满足乘客需求以最低成本进行订票处和机场工作班次排程 Citgo石油公司(1-2/1987,$7000万) 优化炼油运作以及产品的供应、配送和营销 旧金山警署(1-2/1989,$1100万) 用计算机系统最优排程和巡警设置 荷玛特发展公司(1-2/1987,$4000万) 商业区和办公楼销售的最优化安排 AT&T(1-2/1990,$4.06亿,更多的销售) 为公司商业用户的电话销售中心的优化选址
如何来预测客户的需求量呢?
2012-4
DMD——Liuhualing
MBA
供应链网络设计
上海国美电器商场有限公司在上海的商场为
什么是圆形布点?
围绕上海市外环线内部圆形均匀分布着9家商
场,为什么只有一个配送中心,为什么要建在
外环线的外面?
2012-4
DMD——Liuhualing
MBA
管理科学就是帮助你 解决管理中的决策难题的!
6)帮助实施被管理者采纳的建议
2012-4
DMD——Liuhualing
MBA
计划 组织
管理工作的内容
制定组织目标,并指明如何实现目标 为组织制定出可以达到目标的组织结构 保证有人完成所有的工作 告诉员工应该做什么 鼓励员工做好工作 确保有足够的资源来完成工作 检查实现目标的进度 采取行动,确保组织朝着目标运转 使每个人都了解进度并相互激励
问题的确定 分析问题 建立模型 软件求解 实施方案
确定解决方案
控制
结果分析
2012-4
DMD——Liuhualing
《数据、模型与决策》第4部分_决策分析_学生
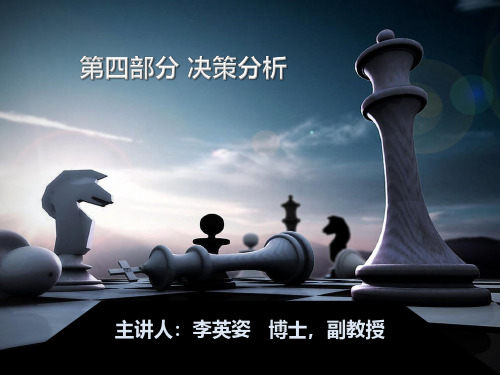
2019/12/1 3
北京科技大学 经济管理学院 张晓冬
22
2.2 不确定决策案例分析
销量 方案
A1: 建立新车间 A2: 改造现有车间
A3: 部分生产 部分外购
某企业生产新产品方案备选表
销量状态
较高
一般
较低
850
420
-150
600
400
-109/12/1 3
很低
-400 -350 -50
• 由于管理系统一般具有属性及目标多样化的特点,在管理决策 时通常要考虑多个目标(如TQCSE),但它们在很多情况下又是 相互消长或矛盾的,这就使得多目标决策分析在管理决策分析 中具有了日益重要的作用
• 目前多目标决策问题的常用方法有:化多目标为单目标的方法 (含系统评价中的加权和及各种确定目标权重的方法)、重排 次序法、目标规划法及层次分析(AHP )方法等
23
2.2 不确定决策案例分析– 乐观法
乐观准则(Max-Max准则)
这种准则又称为大中取大准则,是冒险型决策。该准则对于每个行 动方案Ai,都考虑最有利的情况,认为将是最好的状态发生,即益损值 最大的状态发生,然后,比较各行动方案实施后的结果,取具有最大益 损值的行动为最优行动,也称为最大-最大准则。设Mi为方案Ai的准则值, Vij为方案Ai在j状态的损益值,则其步骤为:
• 取各方案折衷值中的最大值,可得: max{350,220,220} = 350
• 对应实施方案为A1 ,即新建车间生产
2019/12/1 3
29
2.2 不确定决策案例分析 – 等概率法
等可能准则(Laplace准则)
该准则又称为Laplace准则、等概率准则,是平均主义决策。在该准则下, 认为各种状态出现的概率相同,于是根据益损值的最大平均数做出决策。求 出每个行动方案Ai各状态下的益损值算术平均值。然后,比较各行动方案实施 后的结果,取具有最大平均值的行动为最优行动的决策原则。其步骤为:
数据模型与决策决策分析教案

课程展望
进一步深入研究数据模型和决策分析的理论和方法,探索新的应用领域和应用场景
加强与各行业的合作,结合实际需求开发更加贴近实际的数据模型和决策分析工具
培养更加高素质的数据科学家和决策分析师,为社会提供更加准确、高效、科学的 决策支持和服务。
感谢观看
THANKS
详细描述
客户关系管理是企业与客户之间的重要纽带,通过运用数据模型和决策分析工具,企业可以更好地了解客户需求 、行为和偏好,优化客户服务,提高客户满意度和忠诚度。同时,通过分析客户反馈和市场变化,企业可以及时 调整产品和服务策略,增强市场竞争力。
06
课程总结与展望
课程总结
掌握数据模型的基本概念、分类和构建过程
。
03
决策分析基础
决策分析定义
定义
决策分析是一种过程,旨在帮助 决策者识别问题、收集信息、评 估选项、选择最佳方案以及实施 和评估结果。
重要性
决策分析可以帮助决策者做出更 明智、更有效的决策,减少风险 并增加成功的机会。
决策分析流程
收集信息
收集与问题相关的信息,包括 数据、事实、意见等。
选择最佳方案
05
决策分析在实践中的应用案
例
商业智能与决策支持案例
总结词
利用数据模型和决策分析为商业决策提供支持和优化方案。
详细描述
商业智能和决策支持系统利用数据模型和决策分析工具,帮助企业进行数据驱动 的决策,提高运营效率和盈利能力。通过实时分析销售数据、库存数据、市场趋 势等,企业可以制定更加明智的商业决策,调整产品策略、营销策略等。
基于评估结果,选择一个或多 个最佳的解决方案。
识别问题
确定需要解决的问题,了解问 题的背景和相关因素。