国内外股票市场财富效应研究文献综述
[证券论文]我国证券市场“财富效应”分析汇总
![[证券论文]我国证券市场“财富效应”分析汇总](https://img.taocdn.com/s3/m/d6669e014b35eefdc8d333de.png)
[证券论文]我国证券市场“财富效应”分析本文通过对我国证券市场“财富效应”的传导机制及主要制约因素的分析,指出目前我国证券市场的“财富效应”还不太明显。
因此,为了充分发挥证券市场的“财富效应”,使之发挥在内需强约束情况下,对经济增长的促进作用,政府应该在有关方面作出适当的安排。
[关键词]证券市场;财富效应;经济增长“财富效应”是指资产价格的上升使资产所有者财富增加,并导致消费支出增长的现象。
能够带来“财富效应”的既可以是证券资产,也可以是实物资产,本文主要研究证券资产价格上升带来的“财富效应”。
从国外的经验看,股市上涨造成的财富效应能够有效扩大消费需求,推动经济增长。
传统经济理论认为,股票市场是一个赌场,是“零和博弈”,有输必有赢,财富总量不变。
果真如此,股票市场就不会为所有股票持有者带来财富增加,只能是既有财富在各投资者之间的重新分配。
这种财富重新分配能否产生正财富效应取决于财富增加者的消费倾向。
这里的问题是股票市场上会不会出现大部分乃至所有投资者都赚钱,即财富总量增加的情形?回答是肯定的。
从短期来看,如果股市的繁荣发展不能持续,则其财富效应是有限的,因为财富总量增加或者结构调整,即使存在,也仅仅是暂时的,它会很快被随后而来的股市萧条引致的财富减少所抵销。
但是,如果股市繁荣能够持续,即从长期来看,财富总量肯定会随之而增加。
1929年以前的美国股市、80年代的日本股市以及目前的美国股市持续了多年的繁荣,具有的财富效应,均证明了这一点。
我国股市也带来一定的财富效应,但是由于种种原因,这种效应在当前条件下还比较有限。
一、证券市场财富效应的传导机制分析证券市场主要通过以下途径影响居民的消费支出:1、通过影响居民实际收入来扩大消费。
在一个不太长的时期内,居民的消费倾向是个定量,消费中最具决定性的因素是可支配收入。
证券市场的价格上扬使证券投资者获得资本利得,而资本利得虽然不计入国民经济资产中的居民收入这一指标,但它事实上构成了居民的可支配收入的一部分,对居民消费发生影响。
关于股利政策理论文献综述
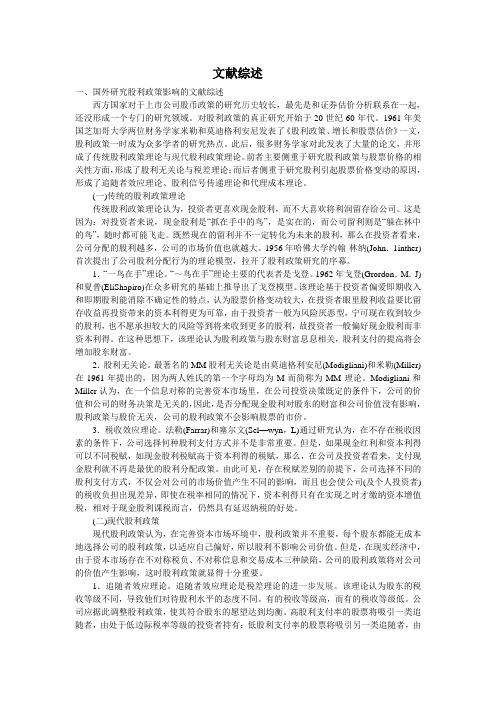
文献综述一、国外研究股利政策影响的文献综述西方国家对于上市公司股币政策的研究历史较长,最先是和证券估价分析联系在一起,还没形成一个专门的研究领域。
对股利政策的真正研究开始于20世纪60年代。
1961年美国芝加哥大学两位财务学家米勒和莫迪格利安尼发表了《股利政策、增长和股票估价》一文,股利政策一时成为众多学者的研究热点。
此后,很多财务学家对此发表了大量的论文,并形成了传统股利政策理论与现代股利政策理论。
前者主要侧重于研究股利政策与股票价格的相关性方面,形成了股利无关论与税差理论;而后者侧重于研究股利引起股票价格变动的原因,形成了追随者效应理论、股利信号传递理论和代理成本理论。
(一)传统的股利政策理论传统股利政策理论认为,投资者更喜欢现金股利,而不大喜欢将利润留存给公司。
这是因为:对投资者来说,现金股利是“抓在手中的鸟”,是实在的,而公司留利则是“躲在林中的鸟”,随时都可能飞走。
既然现在的留利并不一定转化为未来的股利,那么在投资者看来,公司分配的股利越多,公司的市场价值也就越大。
1956年哈佛大学约翰·林纳(John.1inther)首次提出了公司股利分配行为的理论模型,拉开了股利政策研究的序幕。
1.“一鸟在手”理论。
“~鸟在手”理论主要的代表者是戈登。
1962年戈登(Grordon.M.J)和夏普(EliShapiro)在众多研究的基础上推导出了戈登模型。
该理论基于投资者偏爱即期收入和即期股利能消除不确定性的特点,认为股票价格变动较大,在投资者眼里股利收益要比留存收益再投资带来的资本利得更为可靠,由于投资者一般为风险厌恶型,宁可现在收到较少的股利,也不愿承担较大的风险等到将来收到更多的股利,故投资者一般偏好现金股利而非资本利得。
在这种思想下,该理论认为股利政策与股东财富息息相关,股利支付的提高将会增加股东财富。
2.股利无关论。
最著名的MM股利无关论是由莫迪格利安尼(Modigliani)和米勒(Miller)在1961年提出的,因为两人姓氏的第一个字母均为M而简称为MM理论。
探析如何完善股票市场财富负效应证券论文范文

探析如何完善股票市场财富负效应证券论文范文财富及财富创造一直都是经济学研究的重要命题之一。
财富的多少、变动以及对财富的期望都影响人们的行为,从而引起经济的波动,这就是财富效应。
自从20 世纪90 年代以来,无论从世界经济还是从国内经济发展来看,资本市场尤其是股票市场,对于国民经济的作用越来越凸现,股市的长期繁荣或大幅下跌,对于国民经济的整体影响越来越大。
由于股市下跌对宏观经济的整体发展带来一系列负面影响,远远大于股市上升时为经济增长所带来的积极作用,因此,国内外许多理论研究者、政策制定者和投资者都对股票市场负财富效应进行了大量的研究。
一、资本市场负财富效应的的原因。
(一)我国的上市公司投资效率低下,破坏了财富效应发挥的前提。
长期以来,我国上市公司一直存在重融资、轻使用的不良现象,相当数量的上市公司进行后续融资时,并不是出于发展主业的考虑,而是编造项目以图“圈钱”,结果造成募集资金投资不足,效率低下,这主要表现在3 个方面:(l) 募集资金大量闲置;(2)募集资金大量用于委托理财;(3)随意更改资金用途。
据全国人大常委会证券法执行检查组披露,上市公司经常随意改变资金方向,并造成一定的损失。
(二)我国股市功能严重扭曲,从根本上限制了股市财富效应的发挥。
在我国,股市的主力是A 股市场,而A股市场的定位是为国企改革服务,并且发行方式的限制使股市无法满足中小企业的融资需要。
股市的这种定位,使得散户们长期处于弱势地位,他们承担了本不该承担的大部分代价,其整体盈亏状况令人堪忧。
这种功能定位的偏差,很重要的一点与我们现行干部体制下低效率干部考评制度有关,即把股票市场上每年的融资额当成一个重要考核指标。
因此,在我国股市中,长期存在不考虑投资者,不考虑这个市场怎么为优化配置资源服务的问题。
在我国股市中,个人投资者中月均收入3000 元以下的中低收入人群达91.56%,其中更有6.35%的失业人员和22.9%的离退休人员。
中国股市财富效应的实证分析的开题报告

中国股市财富效应的实证分析的开题报告一、选题的背景和意义随着中国经济的高速发展和金融改革的不断深化,中国股市作为重要的金融市场,其影响力日益增强,被视为反映经济发展情况的一个重要指标。
同时,随着人们投资理念的不断转变和风险意识的加强,越来越多的人开始投资股市,对中国股市的表现和发展情况产生了广泛关注。
其中,股市财富效应作为衡量股市对于经济和社会的贡献的重要指标,一直备受关注。
股市财富效应指根据股价波动变化所形成的财富变化,对经济或社会发展所产生的积极或消极影响的程度。
特别是在当前中国股市高速发展的背景下,进一步研究股市财富效应,探讨其实证分析,对于社会和经济的发展具有重要的意义和实际应用价值。
二、研究的目的和内容目的:本文旨在实证分析中国股市财富效应的情况,探讨其对于经济和社会发展的积极或消极影响。
通过对于历史数据的分析,总结和分析中国股市财富效应的变化和趋势,为未来的股市投资和政策调控提供科学的参考和建议。
内容:文章主要涉及以下内容:1. 研究股市财富效应的相关概念和理论。
2. 通过对于历史数据的分析,总结和分析中国股市财富效应的变化和趋势,以及不同股市变化对于社会和经济的影响。
3. 探讨中国股市财富效应的影响因素,包括经济发展水平、政府宏观政策、投资者心态等因素。
4. 提出针对不同情况下股市财富效应的政策建议,为未来的股市投资和政策调控提供科学的参考。
三、研究方法和步骤本文将采用以下方法和步骤:1. 文献综述法。
回顾国内外股市财富效应的研究现状,总结和分析已有研究成果,了解相关概念和理论。
2. 统计分析法。
通过对于历史数据的分析,采用图表等形式展示中国股市财富效应的变化和趋势,以及不同股市变化对于社会和经济的影响。
3. 影响因素分析法。
结合经济发展水平、政府宏观政策、投资者心态等因素,对股市财富效应的影响因素进行探讨。
4. 政策建议法。
根据分析结果,提出针对不同情况下股市财富效应的政策建议。
《股权结构与盈利能力关系研究国内外文献综述3500字》

股权结构与盈利能力关系研究国内外文献综述目录股权结构与盈利能力关系研究国内外文献综述 (1)(一)国外文献综述 (1)1.资本结构与盈利能力的关系。
(1)2.股权结构和盈利能力的关系 (1)(二)国内文献综述 (2)(三)文献述评 (3)参考文献 (4)(一)国外文献综述1.资本结构与盈利能力的关系。
Titman(2009)根据400多家制造业上市公司的相关数据,分析企业在不同负债阶段下的盈利能力,得出如果公司负债过高,将影响公司的盈利能力[1]。
S. Ouchene等(2013)主要分析了美国银行优化资本结构的途径是提高二级资本,并提高了盈利能力2]。
Lepetit L等(2014)在研究影响银行盈利能力的因素时,深入分析了股东控制和美国次贷危机的影响。
研究发现,当股东控制相对集中时,银行的利润最大。
风险也较高,在一定程度上会影响公司的盈利能力[3]。
Daskalakis N等(2017)重点研究了外部环境、内部流动性和中小企业长期债务负债率的变化。
短期负债随外部环境的变化变化更为明显,而本案例中短期负债变化不大[4]。
Vecchiato M等(2018)以美国金融业为研究对象。
基于美国金融业的相关金融数据,将研究对象限定在金融业。
了解行业不同变化下的资本结构将影响其盈利能力,最终发现在美国金融行业资产收益率越低,公司经营业绩越好的研究结论[5]。
2.股权结构和盈利能力的关系Welch(2003)为了研究股权结构与盈利能力的关系,选取澳大利亚上市公司作为研究样本,同时选取了股权结构作为内生变量,实证结果表明,公司股权结构与盈利能力相关,且内部人持股与盈利能力有着非线性相关的关系[6]。
Andersson等(2004)选取了瑞典87家上市公司,以1999-2003年的数据为样本,以资产收益率和产权净利率为盈利能力指标,对公司的股权结构与盈利能力之间的关系进行实证研究。
研究结果表明,当内部股东表决权为5%、10%和20%时,表决权对盈利能力的影响也各不相同[7]。
《货币政策对证券市场的影响研究国内外文献综述6300字》

货币政策对证券市场的影响研究国内外文献综述目录货币政策对证券市场的影响研究国内外文献综述 (1)1.1国外研究动态 (1)1.2国内研究动态 (1)1.3货币政策效应 (2)1)货币政策对股票市场影响的相关文献 (2)2)货币政策溢出效应的相关文献 (3)1.4研究述评 (5)参考文献 (7)1.1国外研究动态国外关于货币政策传导至股票市场的文献中采用的政策代理变量主要是利率、汇率、货币数量,股价的代理变量是股票市场的价格指数,分析采用的模型大多是V AR模型。
此模型在研究多个相关经济指标的相互影响以及进行测试时,为了解决变量的内生性问题,被学者广泛运用。
为了说明货币政策对于股市影响的普遍性,以下文献包括发达国家的研究和发展中国家的研究。
Asaolu, Ogunmuyiwa(2017)〔1〕研究了分析美国货币政策对股票市场的影响,结果显示相比货币供应量,利率的变化对股票市场的波动起到了更大的作用,且两者呈现负相关关系。
Chatziantoniou与Duffy David(2018)〔2〕用向量自回归模型检验联邦基金利率与股价之间的关系,认为在样本区间内,货币政策对股价的影响不明显,而且发现较低迷股市而言货币政策对于活跃的股市影响更大。
Brian Muroyiwa(2019)分析货币政策对于股市的影响时,引入了汇率这一变量,得到汇率是影响股价变动的因素的结论。
1.2国内研究动态虽然我国的股票市场建立时间不长,但是发展迅速,因此有关我国货币政策股价传导渠道的研究不算少。
根据研究侧重点的不同,有关这一渠道的研究分成三个方面:一是研究货币政策是如何影响股市的;二是研究股市是如何影响经济实体的;三是研究整个传导渠道任永平与李伟(2020)根据应用向量自回归模型分析货币政策对股市的影响时,发现信贷供应量对股市的影响逐渐变弱,利率、货币数量对股市的影响逐渐增强。
任永平与李伟(2020)建立滞后期为5 的向V AR模型研究货币政策对股市的影响,研究发现货币政策会影响股价,利率对股价的影响小于货币数量对股价的影响。
股利政策相关国内外研究文献综述_谢婉玲
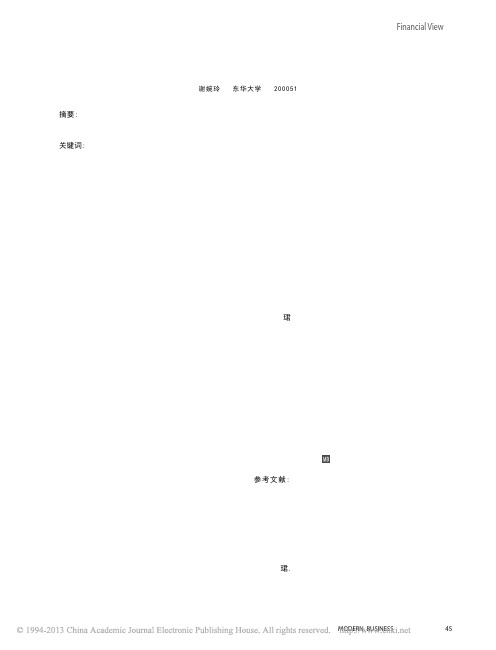
Financial View金融视线 |MODERN BUSINESS 现代商业45股利政策相关国内外研究文献综述谢婉玲 东华大学 200051摘要:股利政策在上市公司财务经营活动中处于重要地位。
国内外文献的对股利研究重点往往集中于现金股利分配水平的影响因素分析。
近年来则有所创新,有关学者提出运用判别模型对不同股利政策的选择动因进行研究。
然而到目前为止,该类研究仅将现金股利和股票股利进行了对比,对双重股利的研究较少。
关键词:股利政策;现金股利;股票股利;双重股利一、引言股利政策作为上市公司经营状况成果的分配它一直是证券市场和广大投资者关心的问题,在上市公司财务经营活动中处于重要地位。
上市公司通过选择最优股利支付,向市场传递有关公司现在状态和未来前景的信息,来达到内部管理层和外部投资者的信息均衡。
因此投资者可以根据公司的股利政策和市场反应来判断公司的发展状况和质量。
二、国外股利政策研究综述国外关于公司股利政策的研究,最早是由Lintner(1956)提出的公司股利分配行为的理论模型。
该理论认为当前股利收入比留存收益所带来的未来资本利得更为可靠,那么对于那些厌恶风险的投资者来说,他们可能宁愿获得较少的股利,也不愿意将留存收益给未来不确定的高额资本利得。
Miller和Modigliani(1961)的股利无关论则成为股利政策研究的基础。
该理论认为,在一个信息对称的完美资本市场里,在公司投资决策既定的条件下,公司的价值和公司的财务决策是无关的。
该理论的缺陷在于忽视了资本市场的交易费用、资本市场的信息不对称等种种现实。
但Miller和Modigliani后来认为,未预期到的股利变化会向市场提供有关公司未来盈余的信息,因此会导致股票价格的变化,当公司提高股利时,就是向市场传递出“盈利能力佳”的良好信号;反之,就会向市场传递出“盈利能力差”的不良信号。
这就是股利政策的信号传递理论假说。
客户效应理论最先也是由Miller(1961)和Modigliani(1961)提出!的。
股票市场财富效应及货币政策规则选择
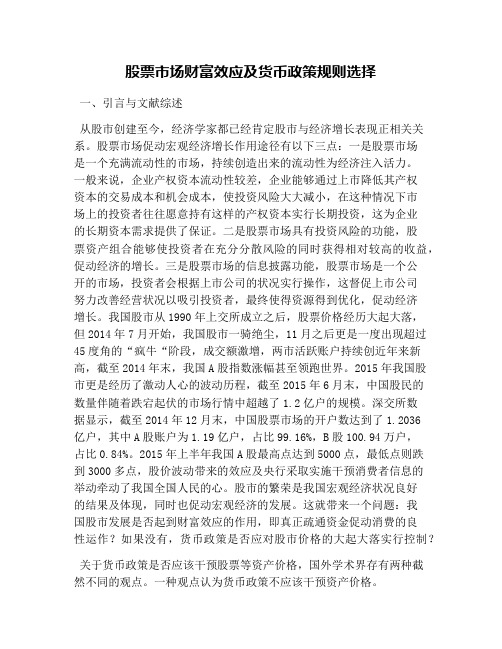
股票市场财富效应及货币政策规则选择一、引言与文献综述从股市创建至今,经济学家都已经肯定股市与经济增长表现正相关关系。
股票市场促动宏观经济增长作用途径有以下三点:一是股票市场是一个充满流动性的市场,持续创造出来的流动性为经济注入活力。
一般来说,企业产权资本流动性较差,企业能够通过上市降低其产权资本的交易成本和机会成本,使投资风险大大减小,在这种情况下市场上的投资者往往愿意持有这样的产权资本实行长期投资,这为企业的长期资本需求提供了保证。
二是股票市场具有投资风险的功能,股票资产组合能够使投资者在充分分散风险的同时获得相对较高的收益,促动经济的增长。
三是股票市场的信息披露功能,股票市场是一个公开的市场,投资者会根据上市公司的状况实行操作,这督促上市公司努力改善经营状况以吸引投资者,最终使得资源得到优化,促动经济增长。
我国股市从1990年上交所成立之后,股票价格经历大起大落,但2014年7月开始,我国股市一骑绝尘,11月之后更是一度出现超过45度角的“疯牛“阶段,成交额激增,两市活跃账户持续创近年来新高,截至2014年末,我国A股指数涨幅甚至领跑世界。
2015年我国股市更是经历了激动人心的波动历程,截至2015年6月末,中国股民的数量伴随着跌宕起伏的市场行情中超越了1.2亿户的规模。
深交所数据显示,截至2014年12月末,中国股票市场的开户数达到了1.2036亿户,其中A股账户为1.19亿户,占比99.16%,B股100.94万户,占比0.84%。
2015年上半年我国A股最高点达到5000点,最低点则跌到3000多点,股价波动带来的效应及央行采取实施干预消费者信息的举动牵动了我国全国人民的心。
股市的繁荣是我国宏观经济状况良好的结果及体现,同时也促动宏观经济的发展。
这就带来一个问题:我国股市发展是否起到财富效应的作用,即真正疏通资金促动消费的良性运作?如果没有,货币政策是否应对股市价格的大起大落实行控制?关于货币政策是否应该干预股票等资产价格,国外学术界存有两种截然不同的观点。
美国与中国股票市场财富效应对比研究——基于2013-2018年数据
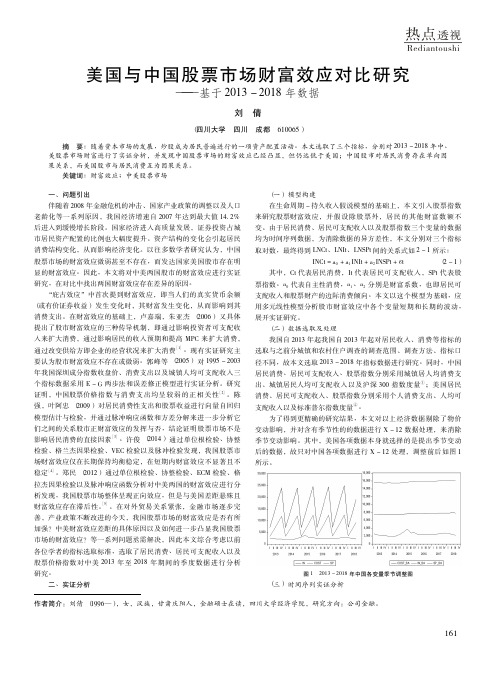
一、问题引出 伴随着 2008 年金融危机的冲击、国家产业政策的调整以及人口 老龄化等一系列原因,我国经济增速自 2007 年达到最大值 14. 2% 后进入到缓慢增长阶段。国家经济进入高质量发展,证券投资占城 市居民资产配置的比例也大幅度提升。资产结构的变化会引起居民 消费结构变化,从而影响经济变化。以往多数学者研究认为,中国 股票市场的财富效应微弱甚至不存在,而发达国家美国股市存在明 显的财富效应。因此,本文将对中美两国股市的财富效应进行实证 研究,在对比中找出两国财富效应存在差异的原因。 “庇古效应” 中首次提到财富效应,即当人们的真实货币余额 ( 或有价证券收益) 发生变化时,其财富发生变化,从而影响到其 消费支出。在财富效应的基础上,卢嘉瑞,朱亚杰 ( 2006) 又具体 提出了股市财富效应的三种传导机制,即通过影响投资者可支配收 入来扩大消费,通过影响居民的收入预期和提高 MPC 来扩大消费, 通过改变供给方即企业的经营状况来扩大消费[1]。现有实证研究主 要认为股市财富效应不存在或微弱。郭峰等 ( 2005) 对 1995 - 2003 年我国深圳成分指数收盘价、消费支出以及城镇人均可支配收入三 个指标数据采用 E - G 两步法和误差修正模型进行实证分析。研究 证明,中国股票价格 指 数 与 消 费 支 出 均 呈 较 弱 的 正 相 关 性[2]。陈 强,叶阿忠 ( 2009) 对居民消费性支出和股票收益进行向量自回归 模型估计与检验,并通过脉冲响应函数和方差分解来进一步分析它 们之间的关系股市正财富效应的发挥与否,结论证明股票市场不是 影响居民消费的直接因素[3]。许俊 ( 2014) 通过单位根检验、协整 检验、格兰杰因果检验、VEC 检验以及脉冲检验发现,我国股票市 场财富效应仅在长期保持均衡稳定,在短期内财富效应不显著且不 稳定[4]。郑民 ( 2012) 通过单位根检验、协整检验、ECM 检验、格 拉杰因果检验以及脉冲响应函数分析对中美两国的财富效应进行分 析发现,我国股票市场整体呈现正向效应,但是与美国差距悬殊且 财富效应存在 滞 后 性。[5] 。 在 对 外 贸 易 关 系 紧 张, 金 融 市 场 逐 步 完 善,产业政策不断改进的今天,我国股票市场的财富效应是否有所 加强? 中美财富效应差距的具体原因以及如何进一步凸显我国股票 市场的财富效应? 等一系列问题丞需解决,因此本文综合考虑以前 各位学者的指标选取标准,选取了居民消费、居民可支配收入以及 股票价格指数对中美 2013 年 至 2018 年 期 间 的 季 度 数 据 进 行 分 析 研究。 二、实证分析
股票市场财富效应研究
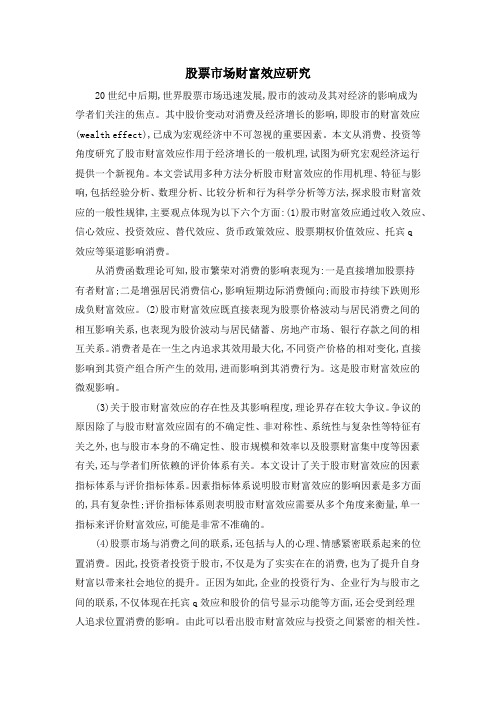
股票市场财富效应研究20世纪中后期,世界股票市场迅速发展,股市的波动及其对经济的影响成为学者们关注的焦点。
其中股价变动对消费及经济增长的影响,即股市的财富效应(wealth effect),已成为宏观经济中不可忽视的重要因素。
本文从消费、投资等角度研究了股市财富效应作用于经济增长的一般机理,试图为研究宏观经济运行提供一个新视角。
本文尝试用多种方法分析股市财富效应的作用机理、特征与影响,包括经验分析、数理分析、比较分析和行为科学分析等方法,探求股市财富效应的一般性规律,主要观点体现为以下六个方面:(1)股市财富效应通过收入效应、信心效应、投资效应、替代效应、货币政策效应、股票期权价值效应、托宾q效应等渠道影响消费。
从消费函数理论可知,股市繁荣对消费的影响表现为:一是直接增加股票持有者财富;二是增强居民消费信心,影响短期边际消费倾向;而股市持续下跌则形成负财富效应。
(2)股市财富效应既直接表现为股票价格波动与居民消费之间的相互影响关系,也表现为股价波动与居民储蓄、房地产市场、银行存款之间的相互关系。
消费者是在一生之内追求其效用最大化,不同资产价格的相对变化,直接影响到其资产组合所产生的效用,进而影响到其消费行为。
这是股市财富效应的微观影响。
(3)关于股市财富效应的存在性及其影响程度,理论界存在较大争议。
争议的原因除了与股市财富效应固有的不确定性、非对称性、系统性与复杂性等特征有关之外,也与股市本身的不确定性、股市规模和效率以及股票财富集中度等因素有关,还与学者们所依赖的评价体系有关。
本文设计了关于股市财富效应的因素指标体系与评价指标体系。
因素指标体系说明股市财富效应的影响因素是多方面的,具有复杂性;评价指标体系则表明股市财富效应需要从多个角度来衡量,单一指标来评价财富效应,可能是非常不准确的。
(4)股票市场与消费之间的联系,还包括与人的心理、情感紧密联系起来的位置消费。
因此,投资者投资于股市,不仅是为了实实在在的消费,也为了提升自身财富以带来社会地位的提升。
《上市公司市盈率影响因素研究国内外文献综述2700字》

上市公司市盈率影响因素研究国内外文献综述1 国外文献综述国外有关市盈率的理论研究和实证分析已经相对比较完善和全面,国外学者从各个方面对市盈率进行了相关的理论或者实证分析。
最早提出市盈率概念的是“现代证券分析奠基人”本杰明·格雷厄姆(Benjamin Graham)。
早在1934年,他与大卫·多德合著的《证券分析》一书中首次对市盈率作了表述,在他们看来,股票的价值是其当期收益的一定倍数,而这个倍数由两个方面决定,一部分由当时的人气决定,也就是投资者预期;另一部分由企业的性质和记录决定,即企业的盈利能力和模式等特点决定,而这个一定倍数正是表示市盈率的大小【1】。
在市盈率的内在含义方面,Fairfield(1994)从经济含义的角度分析了市盈率和市净率,认为市盈率是未来盈利能力预期变化函数,市净率是未来盈利能力预期水平函数。
而且,通过利用美国1970-1984 年的数据建立模型发现,从账面价值角度看,市净率与预期回报呈正相关关系,对未来超额盈余的增长率有正面的影响,市净率与市盈率合在一起对未来股价有比较强的预测能力,此外,根据市盈率与市净率进行分组的不同的组合对未来的盈利能力也会不同【2】。
Kane, Marcus 和Noh(1996)认为市盈率是十分易变的,实证研究结果表明,市场波动增加百分之一能够引起市盈率1.8%的降低,因此,任何对于市场价值的评估,如果不考虑市盈率的变动将犯根本上的错误【3】。
Sujata Behera(2020)通过对大中小盘236 家公司的股票市值,进行了相关信息含量分析。
结果表明,在正常市场收益率变化的情况下,基于经济增加值的估值模型优于需求收益率不变的基于经济增加值的估值模型【5】。
Adil Pasha等(2019)通过对13 个行业的70 家非金融类巴基斯坦证券交易所上市公司10 年的数据,进行研究发现经济增加值与股票收益率之间存在显著的关系【6】。
中国股票市场价值投资分析文献综述
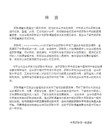
场国家已经成为主导流派的价值投资理念,在这样的情况下,价值投资成为投资
策略的热点。
从长远来看,它有助于逐渐改变目前中国证券市场上中小投资者跟风炒
作、希望在短期内获得高额回报的投机理念和过度炒作的现象,有助于规范我国
证券市场的秩序,促进中国证券市场的健康发展,充分发挥股票市场功能。
安迪?基尔伯特里认为价值投资的真谛在于通过对股票基本面的经济分
析,使用金融资产定价模型估计股票的内在价值,并通过对股价和内在价值的比
较去发现并投资那些市场价格低于其他内在价值的潜力个股,以期获得超过大盘
指数增长率的超额收益[2]。 格雷厄姆的价值投资源自念核心思想主要包括两点[3] :
第一,内在价值Intrinsic Value原则。格雷厄姆证券分析的核心是价值
对未来增长幅度和持续时间的预测之上的。与此对立的是空中楼阁理论[6]。
周贵银著??炒赢中长线:价值投资必须要考虑的八大因素是:价值理论、
安全边际、内在价值、集中持股、复利增长、预测市场、投资心态和休息策略[7]。
其中,价值理论、安全边际和内在价值是选股策略方面的因素,就是如何去评估上
市公司的价值和是否值得投资;而集中持股、复利增长和休息策略是投资风格问
中国股票市场在过去的十多年中获得了长足的发展,我国市场上主流的
股票投资理念和方法也随着市场的发展阶段不断转换。一个最突出的表现就是随
着股票市场规模的不断扩大,各类投资者的数量飞速增长。投资者的高度投资热
情为各种投资理念在中国的应用提供了契机。从基本面分析到技术分析,从成长
股投资到价值股投资,投资者总是运用各种投资策略以希望取得超额收益。随着
股票市场与经济增长文献综述及外文文献资料
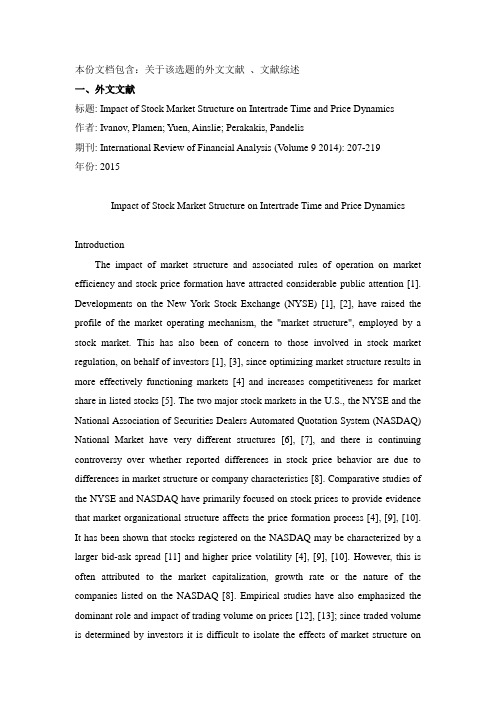
本份文档包含:关于该选题的外文文献、文献综述一、外文文献标题: Impact of Stock Market Structure on Intertrade Time and Price Dynamics作者: Ivanov, Plamen; Yuen, Ainslie; Perakakis, Pandelis期刊: International Review of Financial Analysis (V olume 9 2014): 207-219年份: 2015Impact of Stock Market Structure on Intertrade Time and Price DynamicsIntroductionThe impact of market structure and associated rules of operation on market efficiency and stock price formation have attracted considerable public attention [1]. Developments on the New York Stock Exchange (NYSE) [1], [2], have raised the profile of the market operating mechanism, the "market structure", employed by a stock market. This has also been of concern to those involved in stock market regulation, on behalf of investors [1], [3], since optimizing market structure results in more effectively functioning markets [4] and increases competitiveness for market share in listed stocks [5]. The two major stock markets in the U.S., the NYSE and the National Association of Securities Dealers Automated Quotation System (NASDAQ) National Market have very different structures [6], [7], and there is continuing controversy over whether reported differences in stock price behavior are due to differences in market structure or company characteristics [8]. Comparative studies of the NYSE and NASDAQ have primarily focused on stock prices to provide evidence that market organizational structure affects the price formation process [4], [9], [10]. It has been shown that stocks registered on the NASDAQ may be characterized by a larger bid-ask spread [11] and higher price volatility [4], [9], [10]. However, this is often attributed to the market capitalization, growth rate or the nature of the companies listed on the NASDAQ [8]. Empirical studies have also emphasized the dominant role and impact of trading volume on prices [12], [13]; since traded volume is determined by investors it is difficult to isolate the effects of market structure onprice formation. As the influence of market structure on stock prices may be obscured by exogenous factors such as demand and supply [12], [13], we hypothesize that modulation of the flow of transactions due to market operations may carry a stronger imprint of the internal market mechanism.Here we analyse times between consecutive transactions for a diverse group of stocks registered on the NYSE and NASDAQ markets, and we relate the dynamical properties of the intertrade times with those of the corresponding price fluctuations. To understand how market structure may affect stock prices, we study the information contained in the times between consecutive stock transactions. As market-specific operations may modulate the flow of transactions, we hypothesize that dynamical features of transaction timing reflect the underlying market mechanism. Specifically, we ask if stocks of companies with diverse characteristics registered on a given market exhibit common features in their transaction timing, which may be associated with the particular market structure. Further, we investigate how the dynamical properties of transaction timing over a range of time scales relate to stock price dynamics and whether market structure affects the temporal organisation of price fluctuations.To probe how market structure influences the trading of stocks, we consider the two major U.S. stock markets, the NYSE and the NASDAQ. All transactions on the NYSE of a given stock are centralised and are controlled by a single human operator called a "specialist", whose primary role is to match together public buy and sell orders on the basis of price, in an auction-like setting [6]. The NYSE specialist is under obligation to maintain both price continuity and a "fair and orderly market" [6], as well as to intervene, using his own firm's inventory of available stock, to provide liquidity in the event of an order imbalance, thus preventing sharp changes in the stock price [6]. The NYSE regulations allow for considerable flexibility within the specialist's operations [2].In contrast, trading on the NASDAQ is decentralised, with trading in a given stock managed by a number of dealers called "market makers". These market makers maintain a stock inventory, posting their best prices at which they are prepared toimmediately buy and sell stock [7]. Market makers compete with each other for orders, so in theory competition ensures that investors get the best prices. Alternatively, an order can be placed into an Alternative Trading System (ATS), operated by NASD members or NASD-member affiliates and designed to allow two subscribers to meet directly on the system under the regulation of a third party. The most commonly used form of ATS is the Electronic Communication Network (ECN), a facility that matches customer buy and sell orders directly through a computer network.A third alternative, in case the order placed is very small, is to enter the order into the Small Order Execution System (SOES), which is an electronic network designed to allow fast automatic routing, execution and reporting of orders of 500 shares or less. Orders are automatically routed to market makers whose quotes are currently identical to the highest bid (buy) and the lowest offer (sell) prices. Participation in the SOES system was made mandatory [7] after the market crash of October 1987, as one of the reported problems on the NASDAQ during the crash was the inability to reach market makers by the phone during periods of rapid price movement.To summarize the differences between the two market structures, each market maker on the NASDAQ maintains his own inventory of stock in order to buy and sell [7]. In comparison, the NYSE specialist rarely uses his own firm's inventory: such transactions involve less than 15% of trading volume [14]. Although several regional exchanges may trade NYSE listed stocks, price formation has primarily been attributed to NYSE trading [15]. In contrast, the NASDAQ market relies on competition between multiple dealers for public orders to facilitate the price formation process [11]. Moreover, a substantial fraction of share volume on the NASDAQ is not handled by dealers, but is traded electronically via networks for small public orders and for institutional investors [7]. Such fragmentation of the NASDAQ stock market has been associated with higher price volatility [4].Here we ask to what extent such structural and operational differences between the NYSE and NASDAQ markets affect the flow of transactions. It is difficult to answer whether differences in intertrade times are due to individual companycharacteristics or external market influences (Fig. 1). Two empirical studies have considered only a single company stock over a short period of a few months [16], [17]. Studies which considered a larger group of stocks either did not find common features in the intertrade times [18], [19] or did not compare between markets [20]-[22]. The only comparative study considered a single NYSE and a single Paris stock, finding some differences in their intertrade times, but those may well be due to a different culture of trading [23]. To probe for evidence of the impact of market structure on the trading of stocks, we employ concepts and methods from statistical physics to investigate the correlation properties of transaction timing for diverse companies, over time scales ranging from seconds up to a year.Figure 1. Relationship between stock price and trading activity.Representative example of time series derived from the Trades and Quotes (TAQ) database for transactions of stock in Compaq Computer Corp. (CPQ) registered on the NYSE. (a) Price of CPQ stock over a three week period from 20 Feb.- 8 Mar. 1996 (42606 trades). On 1 Mar. 1996 Compaq reported that it would cut product prices in order to meet sales targets, leading to a drop in the stock price. (b) Intertrade times (ITT) of CPQ stock over the same period. Data exhibit complex fluctuations, a daily pattern of trading activity (with short ITT at the open and close of a trading day and longer ITT in between), and highly heterogeneous structure, as seen in the flurry of trades following the price drop. The relaxation time of the ITT response following the price drop extends over several days, suggesting that information may be contained in the temporal structure of trading activity. Data include transactions occurring between 9.30am and 4pm EST, excluding weekends and holidays.DataWe examine one hundred stocks listed on the NYSE, from eleven industry sectors: Technology-Hardware(5), Semiconductors(2), Pharmaceutical & Medical Equipment(10), Financial(8), Automotive(9), Defense/Aerospace(9), Mining, Metals & Steel Works(8), Chemicals & Plastics(7), Retail & Food(17), Petroleum, Gas & Heavy Machinery(10), Telephone Service Providers(7), Electric & Power Services(8). We study the time intervals between successive stock trades, over a period of fouryears-4 Jan. 1993 to 31 Dec. 1996-as recorded in the Trades and Quotes (TAQ) database from the NYSE (Table 1).Table 1. Characteristics of one hundred NYSE stocks studied over the period 4 Jan. 1993-31 Dec. 1996.We also analyse one hundred NASDAQ stocks from fourteen industry sectors: Technology-Hardware(28), Technology-Software(16), Semiconductors(7), Pharmaceutical, Biotechnology & Medical Equipment(12), Financial(5), Automotive(1), Steel Works(1), Chemicals(1), Retail & Food(16), Petroleum, Gas & Heavy Machinery (2), Telephone & Cable Television Service Providers(5), Services(2), Transportation(3), Electrical Apparatus(1). We study the time intervals between successive stock trades as recorded in the TAQ database, for twenty-nine companies over the period 4 Jan. 1993-31 Dec. 1996, and seventy one companies over the period 3 Jan. 1994-30 Nov. 1995 (marked with (*) in Table 2). For both markets, we select companies with average market capitalisations ranging over three decades, and varying levels of trading activity with average values of intertrade time between 11 and 640 seconds for NYSE stocks, and between 5 and 680 seconds for NASDAQ stocks. In parallel with the intertrade times, we analyse the prices for both sets of stocks over the same periods.Table 2. Characteristics of one hundred NASDAQ stocks studied; data covers twenty nine companies over the period 4 Jan. 1993-31 Dec. 1996, and seventy one companies (marked with *) over the period 3 Jan. 1994-30 Nov. 1995.MethodLike many financial time series the intertrade times (ITT) are inhomogeneous and nonstationary, with statistical properties changing with time, e.g. ITT data exhibit trends superposed on a pattern of daily activity [24]. While ITT fluctuate in an irregular and complex manner on a trade-by-trade basis, empirical observations reveal that periods of inactive trading are often followed by periods of more active trading (Fig. 1). Such patterns can be seen at scales of observation ranging from minutes to months, suggesting that there may be a self-similar, fractal structure in the temporal organisation of intertrade times, independent of the average level of trading activity ofa given stock [24].To probe for scale-invariant features in the fluctuations of intertrade times, we apply the detrended fluctuation analysis (DFA) method, which has been shown to detect and accurately quantify long-range power-law correlations embedded in noisy non-stationary time series with polynomial trends [25]. We choose this method because traditional techniques such as power spectral, autocorrelation and Hurst analyses are not suited to nonstationary data [26]. The DFA method (DFA-) quantifies the root-mean-square fluctuations of a signal at different time scales , after accounting for nonstationarity in the data by subtracting underlying polynomial trends of order ().A power-law functional form indicates self-similarity and fractal scaling in the ITT time series. The scaling exponent quantifies the strength of correlations in the ITT fluctuations: if there are no correlations, and the signal is uncorrelated random noise; if the signal is anti-correlated, meaning that large values are more likely to be followed by small values; if there are positive correlations and the signal exhibits persistent behaviour, where large values are more likely to be followed by large values and small values by small values. The higher the value of , the stronger the correlations. The DFA method avoids the spurious detection of apparent long-range correlations that are an artifact of polynomial trends and other types of nonstationarities [27]-[30].ResultsWe find that the ITT series for all stocks on both markets exhibit long-range power-law correlations over a broad range of time scales, from several trades to hundreds of thousands of trades, characterised by a scaling exponent (Fig. 2 and Fig.3). For all stocks on both markets we observe a crossover in the scaling curve from a scaling regime with a lower exponent over time scales less than a trading day, to a scaling regime with an exponent (stronger positive correlations) over time scales from days to almost a year.[Figure omitted, see PDF]Figure 2. Root-mean-square fluctuation function obtained using DFA-2 analysis, for the intertrade times (ITT) of stock in NASDAQ company Sun Microsystems(SUNW) and NYSE company Compaq Computer Corp. (CPQ).Here indicates the time scale in number of trades. We normalize the time scale by the daily average number of trades for each stock, so that a unit normalized scale indicates one trading day (marked by a dashed line). The scaling curves are vertically offset for clarity. While both companies have similar market capitalisations, industry sectors and average levels of trading activity (average ITT) and exhibit long-range power-law correlations over a broad range of scales, the scaling behaviour of the intertrade times for the two stocks is quite different. For CPQ we find a pronounced crossover from weaker correlations over time scales smaller than a day, to stronger correlations over time scales larger than a trading day (). In contrast, the scaling function for SUNW does not exhibit such a crossover, and we find much stronger correlations over time scales smaller than a trading day compared with CPQ ().[Figure omitted, see PDF]Figure 3. Different correlation properties in intertrade times for stocks registered on the NYSE and NASDAQ markets.Correlation exponents and characterising the temporal structure in ITT for (a) one hundred NYSE stocks and (b) one hundred NASDAQ stocks, of companies with a broad range of market capitalisations and industry sectors. Stocks are ranked in order of decreasing average value of ITT () (as in Tables 1 and 2), and are split into subsets (marked by vertical dashed lines) of companies with matching , and with approximately equal number of stocks in each subset. We estimate over scales from 8 trades to half of the daily average number of trades (for stocks with fewer than trades/year), and to a third of the daily average number of trades (for stocks with more than trades/year). We estimate over scales from 3 to 100 times the daily average number of trades. Group averages and standard deviations of and are shown to the right of the panel for each market. Systematically higher values of for the NASDAQ stocks as compared to the NYSE stocks (statistically significant difference with p-value by Student's t-test), suggest an underlying influence of market structure on the temporal organisation of intertrade times over scales within a trading day. In contrast, no systematic differences between the two markets are observed in the values of ,characterising correlation properties of intertrade times over scales above a trading day ( by Student's t-test). We find similar results when we analyse trading activity at high resolution in terms of the number of trades per minute: a crossover at one trading day and stronger correlations for NASDAQ stocks compared to NYSE stocks over time scales less than a day (features which were not observed in previous studies [52], [50]). We further observe an increasing trend in the values of and with decreasing and increasing company capitalisation for the companies on both markets.Further, we find that this crossover is systematically more pronounced for NYSE stocks compared to NASDAQ stocks (Fig. 2 and Fig. 3). Characterising ITT fluctuations over time scales less than a day, we find that NASDAQ stocks exhibit statistically stronger correlations than NYSE stocks as indicated by Student's t-test (, ), with significantly higher average value of the exponent (group mean std. dev.) as compared to (Fig. 3). In contrast, over time horizons above a trading day, we find that the correlation properties of ITT on both markets are statistically similar (, ), with average scaling exponent comparable with (Fig. 3). Values for the scaling exponents and for the companies on the NYSE and NASDAQ markets are shown in Table 1 and Table 2 respectively.We next investigate how the correlation properties of ITT depend on the average level of trading activity, and if this dependence differs with market structure. Since both sets of a hundred stocks that we study on the NYSE and NASDAQ markets encompass a range of average trading activity spanning over two decades, we split both sets into six subsets with matching average ITT () and approximately equal numbers of stocks in each subset (Fig. 3a,b). Within each market we find that over time scales less than a day, the correlation exponent characterising the trading dynamics is larger for stocks with higher trading activity (lower ) and correspondingly higher market capitalisation (Fig. 3a,b and Fig. 4a). Surprisingly, this dependence persists also for , characterizing the dynamics over much longer time scales, ranging from days to months (Fig. 4b). For NYSE stocks we find a logarithmic dependence of and on (subsequent to posting this manuscript on the Los Alamos archive [31], this logarithmic dependence was later confirmed in [32] on a different set of NYSEstocks). This dependence does not appear to hold for NASDAQ stocks (Fig. 4).[Figure omitted, see PDF]Figure 4. Comparing long-range correlations in ITT for groups of stocks with varying average levels of trading activity on the NYSE and the NASDAQ.(a) Dependence of exponent , characterizing the strength of correlations in ITT over scales from seconds up to a trading day, on the average level of trading activity. Each datapoint represents the group average over a subset of stocks, with a matching range of average intertrade times for the two markets. Stocks are grouped into subsets as indicated by vertical dashed lines in Fig. 3a,b. The consistent difference in the scaling exponent between NYSE and NASDAQ stocks suggests that independent of company characteristics such as market capitalization and industry sector, the temporal organization of ITT within a trading day carries an imprint of market structure. (b) Dependence of exponent characterizing correlations in ITT over time scales from a trading day to several months, on the average level of trading activity. On both markets we observe similar behavior with no systematic difference in the values of between NYSE and NASDAQ subsets of stocks with matching ranges of . These results suggest that over time horizons longer than a trading day, the impact of market structure on trading dynamics is less pronounced as more information is available to investors over longer time scales, driving their trading activity. The resulting more coherent behavior of investors is reflected in stronger correlations over longer time scales.We then compare the scaling behaviour of ITT for each subset of NASDAQ stocks with the corresponding subset of NYSE stocks with matching . We find that for each subset the average correlation exponent for the NASDAQ stocks is significantly higher compared to the NYSE stocks (all values ; Fig. 4a). We also find that there is no significant correlation between the differences in each subset and the , as indicated by Pearson's test (, ). These observations show that within a trading day the difference in the correlation properties of intertrade times of NYSE and NASDAQ stocks is independent of the average level of trading activity. In contrast to , there is no systematic difference in the values of the average for NASDAQ and NYSE stocks forsubsets with matching (all values ; Fig. 4b) except for the subset of companies with the highest frequency of trading (; Fig. 4b).Since for both NYSE and NASDAQ stocks we have chosen companies representing eleven industry sectors with a broad range of market capitalisations and average levels of trading activity spanning over more than two decades, our findings of (i) a crossover in the scaling behaviour of ITT that is more pronounced for NYSE stocks, and (ii) stronger correlations over intraday time scales of NASDAQ stocks with higher values for compared to NYSE stocks, support our hypothesis that market structure affects the dynamics of transaction timing. However, more established companies listed on the NYSE may be subject to different trading patterns when compared with the younger and more rapidly growing companies on the NASDAQ. To verify that the stronger correlations in ITT over time scales less than a day for NASDAQ stocks are indeed due to market structure, we ask if the scaling properties of ITT systematically change for companies that transfer from the NASDAQ to the NYSE. In particular, we investigate the trading dynamics of ten companies that moved from the NASDAQ to the NYSE around the end of 1994 and the beginning of 1995 (Table 3). For each company, we analyse the ITT time series while the company was registered on the NASDAQ, and then repeat the analysis when the company was on the NYSE.Table 3. Characteristics of ten stocks that moved from the NASDAQ to the NYSE during the period 3 Jan. 1994-30 Nov. 1995.For all ten companies we find a significant change in the scaling properties of intertrade times: a marked decrease in the strength of the power-law correlations within a trading day (lower ) associated with the transfer from the NASDAQ to the NYSE (average difference ; Fig. 5b). There is however, no corresponding systematic change in the correlations over time scales above a trading day (average difference ; Fig. 5c), consistent with our findings of statistically similar values of scaling exponent for the two groups of one hundred stocks registered on the NYSE and NASDAQ (Fig.2 and Fig. 3). Thus, our results indicate that market structure impacts not only trading dynamics on a trade-by-trade basis [19], but also the fractal temporal organisation oftrades over time scales from a few seconds up to a day. The presence of stronger intraday correlations in transaction timing for NASDAQ stocks may be attributed to the multiplicity of dealers (ranging from 2 to 50 per stock during 1994 [11]) and electronic methods of trading (Electronic Communication Networks and the Small Order Execution System [7]), allowing the NASDAQ to efficiently absorb fluctuations in trading activity in almost real time [5]. In contrast, for each stock on the NYSE, while there is the electronic SuperDOT routing system, each order has to be exposed to and compared with outstanding orders, as the single NYSE specialist finds the best bid to match an offer with [6]. This may lead to interruptions in the execution of a rapid succession of trades on the NYSE, resulting in weaker correlations in intertrade times within a trading day.[Figure omitted, see PDF]Figure 5. Correlation properties of intertrade times of companies that moved from the NASDAQ to the NYSE.(a) Fluctuation function , obtained using DFA-2 analysis on ITT of stock in the company Mid-Atlantic Medical Services Inc. while it was on the NASDAQ (3 Jan. 1994-29 Sep. 1994) and then after it moved to the NYSE (30 Sep. 1994-30 Nov. 1995). Here indicates the scale in number of trades and the vertical dashed lines indicate the average daily number of trades while on the NYSE or the NASDAQ. The two scaling curves are vertically offset for clarity. After the move to the NYSE there is a decrease in the correlation exponent at time scales within a trading day and a pronounced crossover to stronger correlations with a higher exponent at larger time scales. (b) characterising fluctuations over time scales less than a trading day in ITT of stock in ten companies that moved from the NASDAQ to the NYSE. Companies are ranked in order of decreasing while on the NYSE (as in Table 3) and the scaling range for is the same as for the hundred NYSE and NASDAQ stocks (Fig. 3a,b). For all companies there is a decrease in after the move to the NYSE, indicating that the transition to weaker correlations in ITT over time scales less than a day is due to the NYSE market structure and not to company-specific characteristics. (c) over time scales extending from a trading day to almost a year. In this case we do not observeany systematic change when companies move to the NYSE, which is consistent with the finding of statistically similar values of scaling exponent for the two groups of the one hundred stocks registered on the NYSE and on the NASDAQ (Fig. 3a,b).On the other hand, our finding of stronger power-law correlations for both markets over time horizons from a trading day to several months () suggests that investors' behaviour is more coherent over longer time scales, as information driving trading activity takes time to disseminate. Moreover, this can account for the similar values of for subsets of NYSE and NASDAQ stocks with matched (Fig. 4b), since news and information driving trading activity are exogenous to market structure.Finally, we investigate if the market-mediated differences in long-range power-law correlations in ITT translate into differences in the scaling behaviour of price fluctuations of stocks registered on the NASDAQ and NYSE markets. To this end, in parallel with ITT we analyse the absolute price returns for each company in our database for both markets. For all stocks we observe a crossover at a trading day in the scaling function of price fluctuations [33], [24], from weaker to stronger correlations, corresponding to the crossover we observe for intertrade times. In addition we find that over time scales less than a day, stocks with stronger correlations in ITT exhibit stronger correlations in absolute price returns (Fig. 6a), as indicated by Pearson's test (, ). In particular, we find that the stronger correlations in ITT associated with the NASDAQ market structure (), are accompanied by stronger correlations in price fluctuations () over time scales within a trading day (Fig. 6a).[Figure omitted, see PDF]Figure 6. Relation between correlations in intertrade times and stock price dynamics.(a) Dependence of exponent characterising power-law correlations in absolute logarithmic price return fluctuations, on correlation exponent characterising intertrade times within a trading day. Data represent one hundred NYSE (Table 1) and one hundred NASDAQ (Table 2) stocks. We calculate price returns over 1-minute intervals and over time scales from 8 to 180 minutes ( half a trading day, which is 390 minutes). The positive relationship between and indicates that stronger correlations inITT are coupled with stronger correlations in price fluctuations. This finding suggests that price fluctuations are not merely a response to short-term bursts of trading activity [34], [16]: rather the fractal organisation of price fluctuations over a broad range of time scales is linked to the observed underlying scaling features in the series of intertrade times. (b) Strong relationship between correlations in ITT and correlations in price fluctuations over time scales larger than a trading day for NASDAQ stocks. In contrast, there is no corresponding positive relationship for NYSE stocks. This suggests a weaker coupling between trading dynamics and price formation under the NYSE market structure, over time horizons above a trading day. Dependence of stock price volatility on (c) the correlation exponent and (d) the average value of ITT for the same stocks as in (a). We calculate as the standard deviation of daily logarithmic price returns over six-month periods, averaging over all six-month periods throughout the entire record of each stock. Our results show no strong dependence between stock price volatility and average level of trading activity, rather the volatility appears sensitive to the strength of the temporal correlations in ITT. These findings suggest that scale-invariant features in transaction times may play an important role in price formation. Furthermore, both dynamic and static properties of stock prices appear to be influenced by market-specific features in transaction timing: stronger power-law correlations in ITT (higher values of ) for NASDAQ stocks are matched by stronger power-law correlations in price fluctuations (higher values of ) and higher volatility (), compared with NYSE stocks.We also find evidence of a positive relationship between correlations in ITT and correlations in price fluctuations over time scales larger than a trading day for NASDAQ stocks (Pearson's test shows statistically significant correlation between with with , ; Fig. 6b). In contrast, there is no corresponding positive relationship between with for NYSE stocks (Pearson's test: , ), suggesting a weaker coupling between trading dynamics and price formation under the NYSE market structure, over time horizons above a trading day. While previous work has suggested that bursts of trading activity have an instantaneous impact on stock prices [19], [34], our results show that the interaction between trading times and price formation is more complex,。
我国股票市场的财富效应研究

我国股票市场的财富效应研究我国股票市场的财富效应研究引言自改革开放以来,我国股票市场逐渐发展壮大,吸引了大量投资者的关注和参与。
股票市场作为重要的资本市场组成部分,对于国民经济的发展和个人财富的增长具有重要意义。
然而,股票市场是否真正带来了财富效应,在实践中备受争议。
本文将探讨我国股票市场的财富效应,并分析其中的原因。
一、财富效应的定义和衡量指标财富效应指的是股票市场的繁荣对广大投资者财富的影响程度。
在衡量财富效应时,可以从以下几个方面考虑:1. 股票市场规模的增长:股票市场规模的扩大常常与财富效应的增加相对应。
当市场规模增长时,投资者的选择余地也相应增加。
2. 股票市场的涨跌幅度:股票市场的涨跌幅度直接影响投资者的收益。
当市场呈现较大的涨跌幅度时,投资者有机会获得更高的回报。
3. 投资者参与程度:投资者参与股票市场的投资决策程度直接关系到财富效应的实现。
如果投资者参与市场的程度较高,他们更有机会分享到市场繁荣的红利。
二、我国股票市场的发展及财富效应1. 我国股票市场的发展历程作为社会主义市场经济体制下的资本市场,我国股票市场经历了多次体制改革和发展。
自1990年代初以来,我国的股票市场经历了从股票配售制到实行发行上市制,再到现在的以市场竞价方式交易的股票市场改革。
这一系列的改革,逐步推动了我国股票市场的繁荣与发展。
2. 股票市场规模的增长我国股票市场规模在改革开放以来以几何形式的增长。
作为全球最大的股票市场之一,我国股票市场规模不断扩大,证券交易所的设立和改革进一步提升了市场的规模。
市场规模的增长为投资者带来了更多的选择机会,也为财富效应的实现提供了基础。
3. 股票市场的涨跌幅度随着市场规模的扩大,我国股票市场的涨跌幅度也显著增加。
尤其是在1990年代以来的股市繁荣期间,股票市场呈现出较大的涨幅。
这些涨幅使得投资者有机会获得丰厚的收益,带来了较为明显的财富效应。
4. 投资者参与程度我国股票市场的参与程度逐步增加。
股票市场财富效应研究文献综述
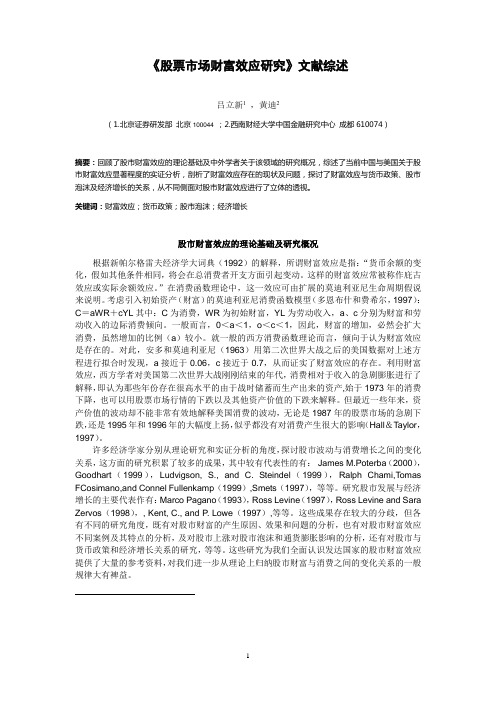
《股票市场财富效应研究》文献综述吕立新1,黄迪2(1.北京证券研发部北京100044 ;2.西南财经大学中国金融研究中心成都610074)摘要:回顾了股市财富效应的理论基础及中外学者关于该领域的研究概况,综述了当前中国与美国关于股市财富效应显著程度的实证分析,剖析了财富效应存在的现状及问题,探讨了财富效应与货币政策、股市泡沫及经济增长的关系,从不同侧面对股市财富效应进行了立体的透视。
关键词:财富效应;货币政策;股市泡沫;经济增长股市财富效应的理论基础及研究概况根据新帕尔格雷夫经济学大词典(1992)的解释,所谓财富效应是指:“货币余额的变化,假如其他条件相同,将会在总消费者开支方面引起变动。
这样的财富效应常被称作庇古效应或实际余额效应。
”在消费函数理论中,这一效应可由扩展的莫迪利亚尼生命周期假说来说明。
考虑引入初始资产(财富)的莫迪利亚尼消费函数模型(多恩布什和费希尔,1997):C=aWR+cYL其中:C为消费,WR为初始财富,YL为劳动收入,a、c分别为财富和劳动收入的边际消费倾向。
一般而言,0<a<1,o<c<1,因此,财富的增加,必然会扩大消费,虽然增加的比例(a)较小。
就一般的西方消费函数理论而言,倾向于认为财富效应是存在的。
对此,安多和莫迪利亚尼(1963)用第二次世界大战之后的美国数据对上述方程进行拟合时发现,a接近于0.06,c接近于0.7,从而证实了财富效应的存在。
利用财富效应,西方学者对美国第二次世界大战刚刚结束的年代,消费相对于收入的急剧膨胀进行了解释,即认为那些年份存在很高水平的由于战时储蓄而生产出来的资产,始于1973年的消费下降,也可以用股票市场行情的下跌以及其他资产价值的下跌来解释。
但最近一些年来,资产价值的波动却不能非常有效地解释美国消费的波动,无论是1987年的股票市场的急剧下跌,还是1995年和1996年的大幅度上扬,似乎都没有对消费产生很大的影响(Hall&Taylor,1997)。
国内外上市公司股利政策文献综述

国内外上市公司股利政策研究综述张洪芳王颖(教授)(杭州电子科技大学管理学院杭州310018)【摘要】本文对国内外上市公司股利政策研究进行了综述,指出了我国学者在上市公司股利政策研究方面存在的不足,建议应进一步加强对上市公司股利政策的选择动因及投资偏好的研究。
【关键词】股利政策股利无关论税差理论股利政策是上市公司理财的三大核心内容之一,合理的股利政策不仅可以使上市公司树立良好的形象,而且能激发广大投资者对上市公司的投资热情,从而使上市公司长期、稳定地发展。
因此,制定合理的股利政策对上市公司的未来发展至关重要,上市公司的管理层必须慎重对待。
1956 年,Lintner 对股利政策进行了开创性研究。
此后,对于股利政策的研究开始受到人们的关注。
国内外众多学者潜心钻研,取得了许多有价值的研究成果,并在上市公司股利政策的实施中发挥了重要的作用。
一、国外相关研究综述1.“一鸟在手”理论。
国外的股利政策研究开展得比较早。
早在1938 年,Williams 就揭开了股利政策研究的序幕,提出了股利重要论。
1956 年,哈佛大学教授Lintner 对股利政策进行了开创性的研究,首次提出公司股利分配行为的理论模型,认为公司管理者在制定股利政策时会考虑三个因素:①目标股利支付率,据此可确定公司愿意支付的股利比例。
②根据长期可持续的变动收益调整股利,公司管理者只有觉得在长期内能够维持更高的收益时才提高股利支付率。
一般来说,股利的增长总是落后于收益的增长且平滑得多。
③股利的变动。
公司管理者更加关注股利的变动而非股利的发放水平。
加利福尼亚大学教授Walter 研究了股利、每股利润、投资收益率及基准折现率同股票价值的关系,建立了华特模型,用数学公式证明了股利政策与股票价值有关。
Gordon 对当时流行的盈利假说和股利假说做了精辟的分析,认为购买股票像购买其他资产一样,是因为它在将来能创造收入,并通过分析证明投资者购买股票是为了获得股利,股利的分配确实能够影响公司的价值。
中国股票市场价值投资分析文献综述

中国股票市场价值投资分析文献综述中国股票市场在过去的十多年中获得了长足的发展,我国市场上主流的股票投资理念和方法也随着市场的发展阶段不断转换。
一个最突出的表现就是随着股票市场规模的不断扩大,各类投资者的数量飞速增长。
投资者的高度投资热情为各种投资理念在中国的应用提供了契机。
从基本面分析到技术分析,从成长股投资到价值股投资,投资者总是运用各种投资策略以希望取得超额收益。
随着中国资本市场的日益开放,各种投资者和证券界的从业人员逐渐接受了在成熟市场国家已经成为主导流派的价值投资理念,在这样的情况下,价值投资成为投资策略的热点。
从长远来看,它有助于逐渐改变目前中国证券市场上中小投资者跟风炒作、希望在短期内获得高额回报的投机理念和过度炒作的现象,有助于规范我国证券市场的秩序,促进中国证券市场的健康发展,充分发挥股票市场功能。
价值投资理念的产生源于对1929年经济危机的反思,其策略共同点是摒弃技术分析,按照股票的内在价值来确定其是否有投资价值。
价值投资理念是由埃利奥特?吉尔德最先提出。
约翰?B?威廉斯在其著《投资价值理论》的一书中,论证了股票的内在价值等于其日后获得的全部股息的现值,给出一个计算内在价值的公式,特别提到“贴现”这一概念。
1934年,本杰明?格雷厄姆和戴维?多德合著的《证券分析》一书,正式把这一理论推广开来。
后来沃伦?巴菲特,马里奥?加比拉,格伦?格林伯格,罗伯特?H?海布伦等投资家按照价值投资理论在实践中都取得了丰厚的收益,从而使价值投资理念的影响大增。
格雷厄姆??证券分析:投资价值理念,即通过对股票内在价值的分析,并比较股票价格来决定股票买卖策略的一种投资理念[1]。
安迪?基尔伯特里认为价值投资的真谛在于通过对股票基本面的经济分析,使用金融资产定价模型估计股票的内在价值,并通过对股价和内在价值的比较去发现并投资那些市场价格低于其他内在价值的潜力个股,以期获得超过大盘指数增长率的超额收益[2]。
股票证券投资方法的文献综述

股票证券投资方法的文献综述随着金融市场的发展,股票证券投资逐渐成为人们投资收益的重要途径。
针对股票证券投资,研究者们提出了许多投资方法和策略,本文将对其中的代表性文献进行综述。
1.技术分析技术分析是一种通过研究股票价格和成交量等走势来预测未来股票价格变化的方法。
其中比较有代表性的文献是John J. Murphy所写的《技术分析之圣经》。
本书详细介绍了技术分析中的各种图表、指标和形态,并对它们的应用进行了解释。
例如,按照趋势来分,技术分析可以分为上升趋势、下降趋势和盘整。
针对不同时期的趋势,可以采用不同的分析方法,如移动平均线、相对强弱指标等。
2.基本面分析基本面分析是另一种预测股票价格变化的方法,它通过研究上市公司的基本经济数据,如利润、收入、资产负债表等来评估其价值。
Benjamin Graham和David Dodd在《证券分析》中详细介绍了这种方法。
该书强调了公司的内在价值和股票价格的关系,认为价值投资应该以公司的基本面为基础,而不是单纯追求短期的价格波动。
该书还介绍了一些评估股票价值的方法,如股利折现模型、盈利能力分析等。
3.量化投资量化投资是一种利用计算机模型进行投资决策的方法。
其中很有代表性的工作是Andrea Unger在《量化投资系统之道》中的研究。
Andrea Unger提出了一种基于趋势追踪的量化投资策略,他通过分析股票价格历史数据,发现了一些趋势规律,并将它们编码成计算机程序。
这种投资策略在过去几年中取得了不少成功,他也因此获得了多个投资比赛的冠军。
该书详细介绍了这种方法的原理和实践应用,以及如何建立自己的量化投资系统。
4.资产配置资产配置是指投资者在不同资产类别(如股票、债券、房地产等)之间分配资金的过程。
Gary P. Brinson等人在《资产配置》中对这种方法进行了详细的讨论。
该书认为资产配置是投资组合管理中最重要的环节,它决定了整个投资组合的风险和收益水平。
作者介绍了一些资产配置的原则和实践方法,如资产类别的选择、权重的分配等。
- 1、下载文档前请自行甄别文档内容的完整性,平台不提供额外的编辑、内容补充、找答案等附加服务。
- 2、"仅部分预览"的文档,不可在线预览部分如存在完整性等问题,可反馈申请退款(可完整预览的文档不适用该条件!)。
- 3、如文档侵犯您的权益,请联系客服反馈,我们会尽快为您处理(人工客服工作时间:9:00-18:30)。
现 代 商 贸 工 业 Mo d e r n B u s i n e s s T r a d e I n d u s t r y
2 0 1 3 年第 O 4期
国 内外股票市场财富效应研究文献综述
叶茂 升
( 武 汉纺 织大学经 济学院 , 湖 北 武汉 4 3 0 0 7 7 ) 摘 要 : 从 三 个 不 同 角 度 回 顾 了 国 内外 股 票 市 场 财 富 效 应 研 究 的 总 体 状 况 。研 究 结 果 表 明 , 发 达 国 家股 市 财 富 效 应 显 著 于发展 中国家 ; 长期股 市财富效应 强于短期 股 市财富效应 ; 间接 财 富 效 应 显 著 于 由收 入 分 配 引 起 的 直接 财 富 效 应 。 关键词 : 股票 市场 ; 直接 财富效应 ; 间接 财 富 效 应 ; 次 贷 危 机
证券价 格升值 对消 费刺激 作 用更加 显著 , 财 大; T a n n e r ( 1 9 9 8 ) 和B r a y 等( 1 9 9 9 ) 运用 消费 C AP M 模 型 研 现 了 长 期 繁 荣 , 借 助 统 计 思 想 和 计 量 工 具 的 不 究发 现 , 股 东 在 消 费 和 证 券 收 益 关 联 性 方 面 明 显 高 于 非 股 富效 应 的 研 究 也 不 断 深 入 , 股 市 财 富 效 应 研 究 的 深 度 和 广 度 也 不 断 提 东, 这 意 味 着 股 市 直 接 财 富 效 应 更 为 显 著 。 Me h r a ( 2 0 0 1 ) 运 断 更 新 和 发 展 , 真 对 股 市 财 富 效 应 的 研 究 大 致 可 以 划 分 为 以 下 用 时间序列数据 分 析分 析得 出, 消费 和 证券 收益 在短 期 内 高 。 目前 , 呈负 相关 , 但在长期 内二者存在显著 的正相关 关系 。
市 场 可 能 产 生 的 潜 在 冲击 。本 文 的 研 究 主 要 从 以 下 三 个 层 心 的影 响 。
面 对 股 市 财 富效 应 的 相 关 文 献 进 行 总 结 和 回顾 。
3 股市 财富 效应 形成 机 制研究
An d o和 Mo d i g l i a n i ( 1 9 6 3 ) 最早对 股票市 场财 富效 应 形 成机 制展开理论 化 分析 , 他们 引入家 庭 消 费行为 的生命 周
中图分类号 : F 8 3
文献标 识码 : A
文章 编号 : 1 6 7 2 - 3 1 9 8 ( 2 0 1 3 ) 0 4 — 0 1 0 9 — 0 2 行等特 点 , 尽 管存在一 定程度 的财 富效应 , 但 财富增 长 对 消
1 引 言
2 0 0 5 ) 利 用协 整方 程模 型研 有关股票市 场财 富 效应 , 一直 以来 都是 学 术 界一 个 热 费拉动 效应 较为 有限 。郭峰 等 ( 究 发 现 , 我 国 居 民 消 费 支 出 与 股 票 价 格 指 数 存在 长 期 均衡 点 研 究 领 域 。近 年 来 , 随着统计 理论 和计 量 工具 的发 展 , 股 误差修 正模 型也 显 示两 者 之间 在短 期 也存 在 较 为微 市财 富效应领域 研究 形 成 了不少 新 的成 果 , 特 别 是 伴 随 新 关 系 , 中 国 股 票 市 场 与 西 方 发 达 国 家 经 济 周 期 出现 的 资 本 市 场 持 续 繁 荣 , 以 及 随 后 美 国次 贷 危 弱 的正 相 关 关 系 。 这 表 明 , 存在较 为有 限 的财 富 效 应 。陈 红等 ( 2 0 0 7 ) 研究 也 发 机 引发 的全球 股市 持续 动荡 , 各 国股 票 市场 都 经 历 了过 山 类似 , 中 国股 市 财 富 效 应 较 为 微 弱 , 其 表 现 形 式 为 股 市 市 值 增 车 一 样 的大 幅 震 荡 。在 这 种 背 景 下 , 股 票 市 场 价 格 波 动 不 现 , 并 扩大 居 民收入预算 约束 , 最终 仅导 致上市公 司市 值 的改变 , 更 引 起 股 票 持 有 者 个 人 财 富 长导致 证券资产 价值升值 ,
的大 起大落 。随着 中 国股票 市 场 的大 发 展 , 个 人 财 富证 券 刺 激 居 民消 费 支 出 。
. 3 股 市 无 财 富 效 应 化 已达到相 当水平 。股 票 、 债 券等 证 券 价格 的涨 跌将 直 接 2 Ot o o ( 1 9 9 9 ) 的研 究 认 为 , 消 费 与 股 票 价 格 之 间 没 有 明 影 响 我 国广 大 居 民财 富 的 积 累 。 当 股 票 在 居 民 个 人 财 富 份
2 股票 市场是 否存 在显 著的 财富 效应
2 . 1 股 市 存 在 显 著 的 财 富 效 应
阐述 了消 费 和财 富 之 间的影 响机 制 和 以及 传 导 路 D y n a n和 Ma k i ( 2 0 0 1 ) 利 用家 庭 消费 支 出 调查 数 据 , 发 期模 型 , 0年代 美 国新经 济 的爆发 , 股 票市 场 出 现股 市直接财 富效 应快 速增 长 , 而 间 接 效 应 变 化 却 变 化 不 径 。伴 随着上世 纪 9
股市 收益 是 影 响消 费 者信 国消 费 、 投资、 以 及 最 终 经 济 增 长 产 生 重 要 影 响 。正 是 基 于 在 2个 星期 到一 个月 的时 间 内 , r a n g e 原因, 也就是说 股市 并不 是通过 财 富效应 对 消 上述 原 因, 股票市 场 的涨跌 一 直 以来 都 受 到宏 观 经 济决 策 心 的 G 消费 变化 不 是 源于 财富 效应 而 是受 到 消 费信 者 的高度关 注 , 任 何宏 观政 策 的 出 台都 必须 考 虑 其对 股 票 费 产 生 影 响 ,
居 民 消 费 不 会 因 为 股 票 价 格 波 动 而 有 所 调 额 中 占有较 大 比重 时 , 股票 就 不 能简 单 地列 入 虚 拟经 济 的 显 的 相 关 关 系 , a n s e n和 J a h u i s ( 2 0 0 3 ) 对 欧洲 1 1个 国 家 1 9 8 6年 ~ 研究 范畴 , 它将直接 影响社会居 民 的财 富再 分配 , 通 过 收 入 整 。J 2 0 0 1年期 间 的 股 票 价 格 和 消 费 关 系 进 行 了检 验 , 结 果 发现, 效应 、 消费效应 以及 投资效应等 机制 作用 于 实体 经济 , 对一