OgreUserSurvey2011[1]
2011年全球雇员指数报告
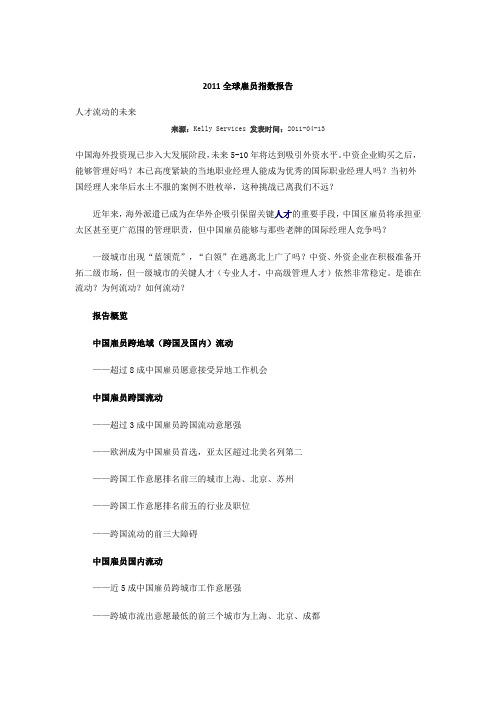
2011全球雇员指数报告人才流动的未来来源:Kelly Services 发表时间:2011-04-13中国海外投资现已步入大发展阶段,未来5-10年将达到吸引外资水平。
中资企业购买之后,能够管理好吗?本已高度紧缺的当地职业经理人能成为优秀的国际职业经理人吗?当初外国经理人来华后水土不服的案例不胜枚举,这种挑战已离我们不远?近年来,海外派遣已成为在华外企吸引保留关键人才的重要手段,中国区雇员将承担亚太区甚至更广范围的管理职责,但中国雇员能够与那些老牌的国际经理人竞争吗?一级城市出现“蓝领荒”,“白领”在逃离北上广了吗?中资、外资企业在积极准备开拓二级市场,但一级城市的关键人才(专业人才,中高级管理人才)依然非常稳定。
是谁在流动?为何流动?如何流动?报告概览中国雇员跨地域(跨国及国内)流动——超过8成中国雇员愿意接受异地工作机会中国雇员跨国流动——超过3成中国雇员跨国流动意愿强——欧洲成为中国雇员首选,亚太区超过北美名列第二——跨国工作意愿排名前三的城市上海、北京、苏州——跨国工作意愿排名前五的行业及职位——跨国流动的前三大障碍中国雇员国内流动——近5成中国雇员跨城市工作意愿强——跨城市流出意愿最低的前三个城市为上海、北京、成都——跨城市流动意愿前五强的行业及职位——跨职级、跨年代比较分析——一级城市雇员对生活与平衡的观点分析超过8成(82%)的中国雇员愿意接受异地工作机会,超过全球平均水平(77%)超过3成(34%)的中国雇员跨国工作意愿强,超过全球平均水平(30%)而近五成(48%)中国雇员倾向国内跨城市工作。
分析人才流动的意义——人才短缺已成为在华企业当前首要的业务挑战,人才跨区流动列入人才短缺的前三大原因:1、本地劳动力供给不足 2、企业业务及规模扩展 3、人才流动与竞争企业的高薪挖角并列第三- Kelly Services 中国区PT业务总经理Mark Hall先生指出影响因素——跨区域工作的雇员通常关注薪酬、被派遣地、所属行业、职位层级、派遣时间、回国后工作安排等问题,以下影响因素明显:海外扩张推动人才跨国流动。
OGRE软件技术简介
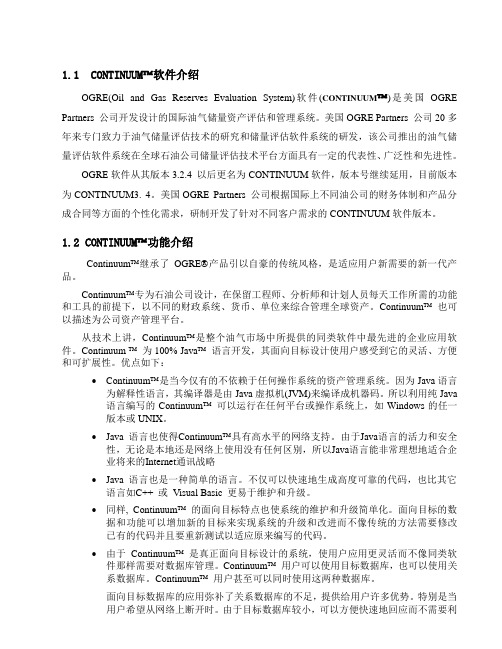
1.1 CONTINUUM™软件介绍OGRE(Oil and Gas Reserves Evaluation System)软件(CONTINUUM™)是美国OGREPartners 公司开发设计的国际油气储量资产评估和管理系统。
美国OGRE Partners 公司20多年来专门致力于油气储量评估技术的研究和储量评估软件系统的研发,该公司推出的油气储量评估软件系统在全球石油公司储量评估技术平台方面具有一定的代表性、广泛性和先进性。
OGRE软件从其版本3.2.4 以后更名为CONTINUUM软件,版本号继续延用,目前版本为CONTINUUM3. 4。
美国OGRE Partners 公司根据国际上不同油公司的财务体制和产品分成合同等方面的个性化需求,研制开发了针对不同客户需求的CONTINUUM软件版本。
1.2 CONTINUUM™功能介绍Continuum™继承了OGRE®产品引以自豪的传统风格,是适应用户新需要的新一代产品。
Continuum™专为石油公司设计,在保留工程师、分析师和计划人员每天工作所需的功能和工具的前提下,以不同的财政系统、货币、单位来综合管理全球资产。
Continuum™也可以描述为公司资产管理平台。
从技术上讲,Continuum™是整个油气市场中所提供的同类软件中最先进的企业应用软件。
Continuum ™为100% Java™语言开发,其面向目标设计使用户感受到它的灵活、方便和可扩展性。
优点如下:∙Continuum™是当今仅有的不依赖于任何操作系统的资产管理系统。
因为Java语言为解释性语言,其编译器是由Java虚拟机(JVM)来编译成机器码。
所以利用纯Java语言编写的Continuum™可以运行在任何平台或操作系统上,如Windows的任一版本或UNIX。
∙Java 语言也使得Continuum™具有高水平的网络支持。
由于Java语言的活力和安全性,无论是本地还是网络上使用没有任何区别,所以Java语言能非常理想地适合企业将来的Internet通讯战略∙Java 语言也是一种简单的语言。
Microsoft Business Solutions-Axapta 问卷模块文档说明书
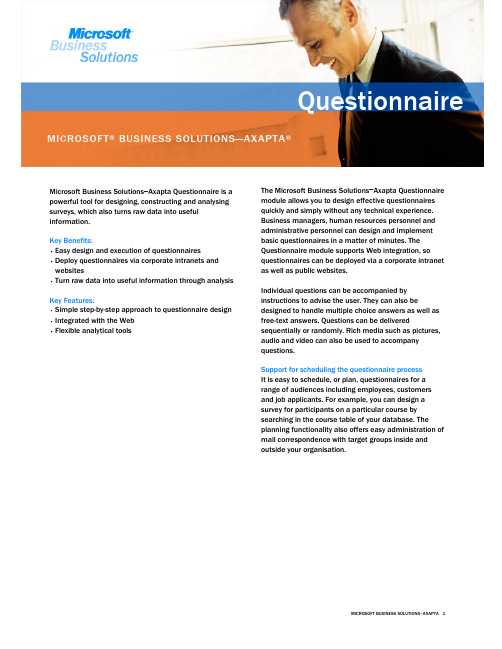
The Microsoft Business Solutions −Axapta Questionnaire module allows you to design effective questionnaires quickly and simply without any technical experience. Business managers, human resources personnel and administrative personnel can design and implement basic questionnaires in a matter of minutes. The Questionnaire module supports Web integration, so questionnaires can be deployed via a corporate intranet as well as public websites.Individual questions can be accompanied by instructions to advise the user. They can also bedesigned to handle multiple choice answers as well as free-text answers. Questions can be deliveredsequentially or randomly. Rich media such as pictures, audio and video can also be used to accompany questions.Support for scheduling the questionnaire process It is easy to schedule, or plan, questionnaires for a range of audiences including employees, customers and job applicants. For example, you can design a survey for participants on a particular course by searching in the course table of your database. The planning functionality also offers easy administration of mail correspondence with target groups inside and outside your organisation.Microsoft Business Solutions −Axapta Questionnaire is a powerful tool for designing, constructing and analysing surveys, which also turns raw data into useful information.Key Benefits: • Easy design and execution of questionnaires • Deploy questionnaires via corporate intranets and websites • Turn raw data into useful information through analysis Key Features: • Simple step-by-step approach to questionnaire design • Integrated with the Web • Flexible analytical toolsDesign effective questionnaires quickly and simplyMultiple usesThe Questionnaire module can be used for a range of activities including customer or employee-satisfaction surveys, job development dialogue, ethical and environmental measurements and management and staff testing.Store all your data in one placeThe Questionnaire module allows you to store your knowledge from surveys in the same system that you store your daily business interaction knowledge. This simplifies retrieval and reduces transaction costs. You no longer have to search through a number of spreadsheets or data conversions from other survey systems.As the questionnaire module is an integral part of Axapta the system provides extensive help with finding and addressing target audiences for questionnaires, as long as they are already listed in the system. Customers, vendors, participants in courses, your own employees and job applicants can be selected from your system. You don’t have to pick out specific contact people or course participants but you can search everybody who has ‘Quality Assurance Manager’ as their job title, for example.Analysis of resultsAnalysis is mandatory for large volume evaluations so the Questionnaire module supports a large number of statistical tools such as calculation and graphical functions, including Pivot Graphics.Analyse results from questionnaires immediatelyYou can make a calculation on any data set in a questionnaire, anywhere in a response hierarchy. There is demographic support for all employees, via the Axapta Human Resource (HRM) module, so that you can cross-reference employee groups across organisational dimensions such as gender, age, length of employment in company, working place, role, salary level and so on.For respondents outside of your organisation, demographic data can be cross-tabbed with respondent master data from the Axapta Customer Relationship Management (CRM) module. The information in Axapta is also integrated with Microsoft Excel, which allows for even greater analysis.Compare new data with earlier resultsIf surveys are repeated, you can compare results, as earlier responses are stored as business transactions in Axapta. This makes it easier to compare results and enables variance and development tracking.Questionnaires can also be used as tracking for management. Whether it is leadership/manager evaluation or business excellence surveys, the results can be easily measured in the Balanced Scorecard module. Data is easy to analyse from within the module or via an On-Line Analytical Processing (OLAP) interface in third party software.One evaluation toolThe questionnaire system supports all business functions that are represented in Axapta, and works closely with modules such as CRM – Telemarketing, HRM, Employee Development for Enterprise Portal and Balanced Scorecard. This means that the module can be used to communicate with your customers, your vendors or your suppliers. You have one evaluation tool across your entire business, and it is ready to cross analyse with your existing business information.Easy to useTraining is simple and inexpensive. As your employees are already familiar with the user interface and terminology, they only need one training course. At the same time, employees across the organisation can share knowledge on how to design and execute surveys.Microsoft Business Solutions−Axapta Enterprise Portal is a Web solution which seamlessly connects your employees, customers and vendors with your business while reducing information overload and making tasks less complex.• Anytime, anywhere access to data• Connect instantly with only Web access• Intuitive Web layout and browser functionality for walk-up usage• Greater visibility for everyone• High ROI - deploy Intranet, Extranet, and Web solution as needed without hassle• No need to buy third party softwareContact your partnerShould you wish to find out more about Microsoft Business Solutions—Axapta, please contact our Internal Sales Team on 0870 60 10 100 where they will be pleased to put you in contact with a certified Microsoft Business Solutions Partner. If you are already a Microsoft Business Solutions customer please contact your Certified Microsoft Business Solutions Partner.About Microsoft Business SolutionsMicrosoft Business Solutions, which includes the businesses of Great Plains®, Microsoft bCentral™ and Navision a/s, offers a wide range of business applications designed to help small and midmarket businesses become more connected with customers, employees, partners and suppliers. Microsoft Business Solutions applications automate end-to-end business processes across financials, distribution, project accounting, electronic commerce, human resources and payroll, manufacturing, supply chain management, business intelligence, sales and marketing management and customer service and support. More information about Microsoft Business Solutions can be found at:/uk/businesssolutionsAddress:Microsoft Business SolutionsMicrosoft CampusThames Valley ParkReadingBerkshire RG6 IWG***********Key Features DescriptionEASY TO USE • Intuitive layout and structure• User-adjustable menu• User-adjustable layout of master files and journals• Windows commands incl. ‘copy and paste’ from and to Axapta• Direct access to master files from journals• Advanced sorting and filter options• Built-in user help including an integrated manual• Option to mail and fax directly from Axapta• Application can be run in different languagesDESIGN AND EXECUTION • Rapid design and deployment of surveys• Individual questions can be accompanied by instructions to advise the user• Each question is linked to an answer mode identified by text, date, numeric value oran answer collection defined by the questionnaire administrator• It is possible to enable free-text answers to any type of question• Response options can be openly defined• Multiple choice or data types• When designing a questionnaire, the questions can be delivered sequentially or inrandom order• Possible to show the percentage of questions in a specific questionnaire that need tobe answered in order to obtain a valid result• Questions can be accompanied by rich media (pictures, audio video, etc.)• Hierarchical questions can be validated• Response groups can be presented sequentially or randomly• Management of access control and user profilesSCHEDULING • Management of questionnaire planning• Easy planning of employees and other individuals in surveys related to applicants,business partners, course participants, networks or organisations• Mail correspondence with all respondents before, during and after response• Online tracking of respondents and responsesREPORTS AND QUESTIONNAIRE ANALYSIS • Response history by questionnaire and individual• Advanced statistical analysis tool supporting: SUM, AVG., MIN., MAX. COUNT, variance and standard deviations• Statistics for % points or number of correct answers• View statistics on individuals including age, geography, etc.• Graphical support and use of pivot table and pivot graphics, integrated to Microsoft Excel• Feedback analysis for 360 degree feedback and other evaluations• Result report, answer report and ‘wrong answers’ reportQUESTIONNAIRE WEB PORTAL • Web execution• Viewing and analysing results onlineQUESTIONNAIRE AND ERP IN ONE • Unique planning and tracking with questionnaires linked to all Axapta processes• Specific integration to CRM – Tele-Marketing• Specific integration to HRM and Employee Development for Enterprise Portal• Specific integration to Balanced ScorecardData summary sheetSystem RequirementsTO OBTAIN ALL OF THE FEATURES MENTIONED IN THIS FACT SHEET, THE FOLLOWING MODULES AND TECHNOLOGIES ARE REQUIRED: • Microsoft Business Solutions−Axapta 3.0• Microsoft Business Solutions−Axapta Questionnaire I• Microsoft Business Solutions−Axapta Questionnaire II• Microsoft Business Solutions−Axapta Enterprise Portal Framework• Microsoft Business Solutions−Axapta Employee role• Microsoft Business Solutions−Axapta Questionnaire for Enterprise Portal07/04/2003© 2003 Microsoft Corporation. All rights reserved.Microsoft Business Solutions includes the business of Great Plains, Microsoft bCentral™ and Navision A/S。
finale2011
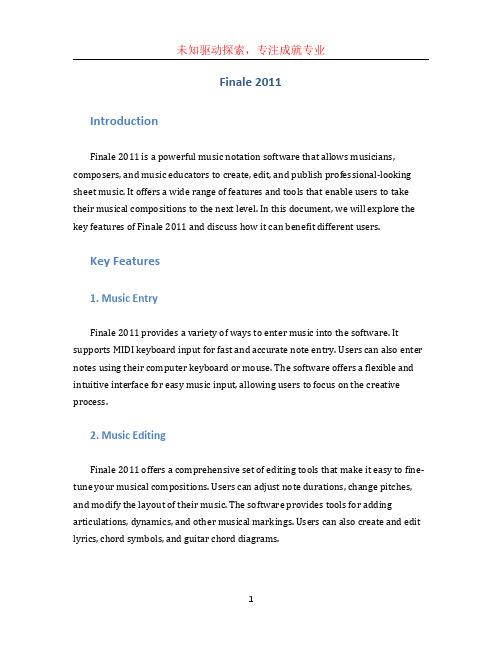
Finale 2011IntroductionFinale 2011 is a powerful music notation software that allows musicians, composers, and music educators to create, edit, and publish professional-looking sheet music. It offers a wide range of features and tools that enable users to take their musical compositions to the next level. In this document, we will explore the key features of Finale 2011 and discuss how it can benefit different users.Key Features1. Music EntryFinale 2011 provides a variety of ways to enter music into the software. It supports MIDI keyboard input for fast and accurate note entry. Users can also enter notes using their computer keyboard or mouse. The software offers a flexible and intuitive interface for easy music input, allowing users to focus on the creative process.2. Music EditingFinale 2011 offers a comprehensive set of editing tools that make it easy to fine-tune your musical compositions. Users can adjust note durations, change pitches, and modify the layout of their music. The software provides tools for adding articulations, dynamics, and other musical markings. Users can also create and edit lyrics, chord symbols, and guitar chord diagrams.3. Playback and Sound LibrariesOne of the standout features of Finale 2011 is its powerful playback functionality. Users can play back their compositions using a wide variety of sounds and instruments. The software includes a vast collection of high-quality sound libraries, covering orchestral instruments, guitars, pianos, drums, and more. This allows users to get a realistic preview of how their music will sound when performed.4. Music EngravingFinale 2011 excels in music engraving, ensuring that your sheet music looks professional and polished. The software provides extensive control over the layout and formatting of your music. Users can adjust spacing, margins, staff size, and more. Finale 2011 supports a wide range of musical symbols and notation styles, allowing users to create sheet music that adheres to their desired aesthetic.5. PublishingFinale 2011 makes it easy to publish your music in various formats. Users can print their compositions directly from the software or export them as PDF files. The software also supports MusicXML, allowing users to share their music with other notation software. Furthermore, users can create audio files of their compositions in various formats, making it easy to share their music online or burn it onto a CD.Benefits for Different UsersMusiciansFor musicians, Finale 2011 provides a platform for creating and editing their musical compositions. The software offers a variety of tools for notation and playback, enabling musicians to transcribe their ideas onto paper and hear them come to life. Finale 2011 allows musicians to explore different musical possibilities, experiment with arrangements, and share their work with others.ComposersComposers can greatly benefit from Finale 2011’s extensive set of features. The software provides composers with a flexible and intuitive environment for composing their music. It offers tools for creating arrangements, orchestrations, and harmonizations. Finale 2011 allows composers to easily experiment with different musical ideas, make revisions, and refine their compositions to perfection.Music EducatorsFinale 2011 is a valuable tool for music educators. The software allows educators to create lesson materials, exercises, and musical examples for their students. It simplifies the process of creating custom worksheets and study materials. Finale 2011 also offers tools for creating accompaniments and play-along tracks, making it a valuable resource for music instruction.ConclusionFinale 2011 is a powerful music notation software that offers a wide range of features and tools. Whether you are a musician, composer, or music educator, Finale2011 provides a flexible and intuitive platform for creating, editing, and publishing music. With its comprehensive set of features, high-quality sound libraries, and extensive control over music engraving, Finale 2011 is a valuable tool for anyone involved in music creation and education.。
用户体验UE:用户体验友好的量化法

导读:信息构建师Peter Morville对用户体验(User Experience)设计进行总结,并设计出了一个描绘用户体验(User Experience)要素的蜂窝图,从而可以量化网站用户体验的效果,为网站设计师提供更多理论依据。
新时代的信息构建师(包括网站设计师、架构师等)应当特别掌握好网站的用户体验(User Experience)设计方法,以给用户提供积极丰富的体验,为网站提高利益。
在用户体验方面,信息构建师Peter Morville由于长期从事信息构建和用户体验(User Experience)设计的工作,对此深有体会,他对用户体验(User Experience)设计进行总结,并设计出了一个描绘用户体验(User Experience)要素的蜂窝图,如图1所示。
用户体验要素蜂窝图该蜂窝图很好的描述了用户体验的组成元素,信息构建师在设计网站或其他信息系统时应当参照这个进行。
这个蜂窝图也说明,良好的用户体验不仅仅指是可用性,而是在可用性方面还有其他一些很重要的东西。
比如:•有用性(useful):它表示设计的网站产品应当是有用的,而不应当局限于上级的条条框框去设计一些对用户来说根本毫无用处的东西;•可找到性(findable):网站应当提供良好的导航和定位元素,使用户能很快的找到所需信息,并且知道自身所在的位置,不至于迷航;•可获得性(accessible):它要求网站信息应当能为所有用户所获得,这个是专门针对于残疾人而言的,比如盲人,网站也要支持这种功能。
•满意度(desirable):是指网站元素应当满足用户的各种情感体验,这个是来源于情感设计的;•可靠性(credible):是指网站的元素要是能够让用户所信赖的,要尽量设计和提供使用户充分信赖的组件;•价值性(valuable):它是指网站要能盈利,而对于非赢利性网站,也要能促使实现预期目标。
这个模型告诉我们用户体验包含多方面的因素,在网站设计时如参照这几个方面进行将会大大提高网站设计和用户体验水平。
EXECUTIVE SUMMARY..........................................................................
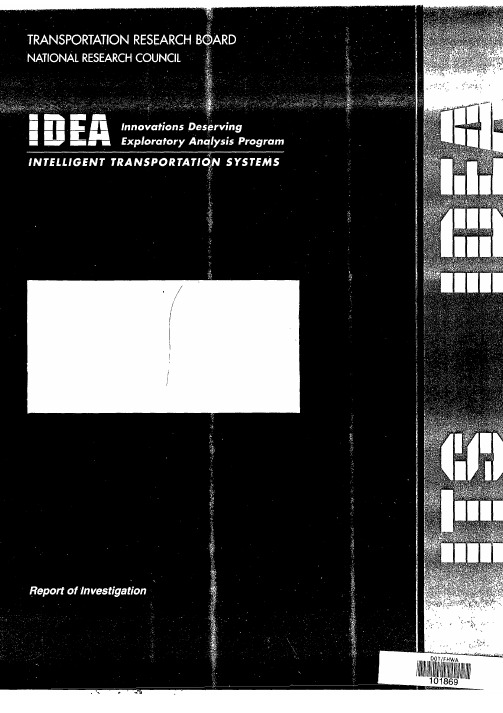
The ITS-IDEA program is jointly funded by the U.S. Department of Transportation’s Federal Highway Administration, National Highway Traffic Safety Administration, and Federal Railroad Administration. For information on the IDEA Program contact Dr. K. Thirumalai, IDEA Program Manager, Transportation Research Board, 2101 Constitution Avenue N.W., Washington, DC 20418 (phone 202-334-3568 fax 202-334-3471).IDEA PROJECT FINAL REPORTContract ITS-6IDEA ProgramTransportation Research BoardNational Research CouncilNovember 28, 1995LASER VEHICLEPrepared by:Richard Wangler Schwartz Electra-Optics, Inc.Orlando, FloridaINNOVATIONS DESERVING EXPLORATORY ANALYSIS (IDEA) PROGRAMS MANAGED BY THETRANSPORTATION RESEARCH BOARD (TRB)This investigation was completed as part of the ITS-IDEA Program, which is one of three IDEA programs managed by the Transportation Research Board (TRB) to foster innovations in surface transportation. It focuses on products and results for the development and deployment of intelligent transportation systems (ITS), in support of the U.S. Department of Transportation’s national ITS program plan. The other two IDEA programs areas are TRANSIT-IDEA, which focuses on products and results for transit practice in support of the Transit Cooperative Research Program (TCRP), and NCHRP-IDEA, which focuses on products and results for highway construction, operation, and maintenance in support of the National Cooperative Highway Research Program (NCHRP). The three IDEA program areas are integrated to achieve the development and testing of nontraditional and innovative concepts, methods, and technologies, including conversion technologies from the defense, aerospace, computer, and communication sectors that are new to highway, transit, intelligent, and intermodal surface transportation systems.The publication of this report does not necessarily indicate approval or endorsement of the findings, technical opinions, conclusions, or recommendations, either inferred or specifically expressed therein, by the National Academy of Sciences or the sponsors of the IDEA program from the United States Government or from the American Association of State Highway and Transportation Officials or its member states.TABLE OF CONTENTSEXECUTIVE SUMMARY. . . . . . . . . . . . . . . . . . . . . . . . . . . . . . . . . . . . . . . . . . . . . . . . . . . . . . . . . . . . . . . . . . . . . . . . . . . . . . . . . . . . . . . . . . . . . . . . . . . . . . . . . . . . . . . . . . . . . . . . 1PROBLEM STATEMENT. . . . . . . . . . . . . . . . . . . . . . . . . . . . . . . . . . . . . . . . . . . . . . . . . . . . . . . . . . . . . . . . . . . . . . . . . . . . . . . . . . . . . . . . . . . . . . . . . . . . . . . . . . . . . . . . . . . . . . . . 2 VEHICLE-SENSOR SURVEY. . . . . . . . . . . . . . . . . . . . . . . . . . . . . . . . . . . . . . . . . . . . . . . . . . . . . . . . . . . . . . . . . . . . . . . . . . . . . . . . . . . . . . . . . . . . . . . . . . . . . . . . . . . . . . . . . . . . . . . . 3 PRODUCT DESIGN SPECIFICATION. . . . . . . . . . . . . . . . . . . . . . . . . . . . . . . . . . . . . . . . . . . . . . . . . . . . . . . . . . . . . . . . . . . . . . . . . . . . . . . . . . . . . . . . . . . . . . . . . . . . . . . . . . . . . . . . . . . . . . . . 4RESEARCH APPROACH. . . . . . . . . . . . . . . . . . . . . . . . . . . . . . . . . . . . . . . . . . . . . . . . . . . . . . . . . . . . . . . . . . . . . . . . . . . . . . . . . . . . . . . . . . . . . . . . . . . . . . . . . . . . . . . . . . . . . . . . 4 RESULTS (8)CONCLUSION (9)GLOSSARY (10)REFERENCES (10)APPENDIX A: VEHICLE-SENSOR SURVEY (11)APPENDIX B:VEHICLE SPEED AND LENGTH MEASUREMENT ACCURACY (14)EXECUTIVE SUMMARYThis report describes a diode-laser-based vehicle detector and classifier (VDAC) developed by Schwartz Electro-Optics (SEO) under the Transportation Research Board (TRB) Intelligent Vehicle-Highway Systems (IVHS), now Intelligent Transportation Systems (ITS), Innovations Deserving Exploratory Analysis (IDEA) Program. The VDAC uses a scanning laser rangefinder to measure three-dimensional vehicle profiles that can be used for accurate vehicle classification. The narrow laser beam width permits the detection of closely spaced vehicles moving at high speed; even a 2-in.-wide tow bar can be detected. The VDAC shows great promise for applications involving electronic toll collection from vehicles at freeway speeds, where very high detection and classification accuracy is mandatory.The extensive network of modem highways in the United States today offers a fast, safe, convenient means of transporting goods and people within and between the major cities of the country. However, the U.S. highway system is under considerable stress. The traffic congestion that currently pervades metropolitan areas threatens future gridlock if mitigating steps are not soon taken. According to ITS America (I), “The percent of peak hour travel on urban interstates that occurred under congested conditions reached 70 percent in 1989, up from 41 percent in 1975 .”If this trend continues, all peak-hour traffic will be congested by 2000; there is good reason to believe that the trend will continue. FHWA data show that since about 1965 the number of vehicle miles traveled has been increasing at a faster rate than expenditures on highway maintenance and that total capital spending for highways, streets, roads, and bridges has declined by more than 50 percent. It is assumed that the growth in traffic and decline in new roadway construction will continue and that a worsening traffic congestion problem can be expected.One of the goals for ITS in the United States is to reduce congestion. Through areawide traffic management, ITS can use existing facilities to improve traffic-flow efficiency. Advanced sensor technology is needed to provide accurate, real-time traffic-parameter data, such as volume, occupancy, speed, and classification, which are required to optimize the performance of areawide traffic management systems. Information on real-time traffic conditions can be used for rapid incident detection and en-route driver navigation.The sensors of choice for many future ITS applications will undoubtedly be mounted overhead. Although inductive loops are simple, low-cost devices, they are not as easily installed or maintained because of their in-pavement location. Several types of overhead vehicle detectors are being developed (2), including video detection systems, microwave radar detectors, ultrasonic detectors, passive infrared sensors, and active infrared sensors. Of these, only the active infrared sensor, using a laser rangefmder, has the capability for accurate vehicle profiling as a result of the narrow angular beam width of the laser.This profiling capability, a dual-beam configuration that permits speed measurement, and efficient vehicle-recognition software combine to produce a sensor that can classify vehicles as well as measure their presence and speed. The outstanding utility of such a sensor became good motivation for its development as a practical device.VDAC relies on an inherent laser characteristic-narrow angular beam width--to provide the high resolution required for accurate vehicle, profiling. The VDAC beam-scan geometry is shown in Figure 1. The SCANNING BEAMSFIGURE 1 VDAC beam-scan geometry.1system scans two narrow laser beams, at a fixed angularseparation, across the width of a lane at a rate of up to 720scans/sec. Pulsed time-of-flight range measurementsprovide accurate _ (+ 3 in.) transverse height profiles of avehicle on each scan. The vehicle speed, determined fromthe time interval between the interceptions of the two laserbeams by the vehicle, is used to space the transverseprofiles appropriately to obtain the full three-dimensionalvehicle profile. An algorithm similar to those developedfor military target recognition is applied to the three-dimensional profile for vehicle-classification purposes.An example of the VDAC three-dimensional profilingcapability is provided by the range image shown in Figure2. This range image of a van pulling a boat traveling at aspeed of 45 mph was obtained by the VDAC operatingwith a scan rate of 360 scans/sec. The pixel spacingresulting from the l-degree scan resolution is more thanadequate for vehicle identification.VDAC uses a rotating polygon as shown in Figure 3 toline scan a diode-laser rangefmder across a 12-ft-widelane of highway. The polygon scanner rotatescontinuously in one direction at a constant speed. Theangle between each facet and the base of the polygonalternates between 87.5 and 92.5 degrees for adjacentfacets; as a result, successive scans are made with anangular separation of 10 degrees, which provides the twoseparate beams needed for speed measurements. Asshown in Figure 4, the 0.5- by 12-mrad laser beamilluminates a 5- by 120-mm spot on the pavement thatprovides good m-lane resolution and optimum cross-lanecoverage when the laser is pulsed once per degree of scanangle.Applications for VDAC are many and include thefollowing:l Vehicle classification for toll charging.l Use with wireless smart cards to prevent cheating byverifying vehicle classification.l Vehicle road location and timing determination forlicense plate photography.l Wide-area real-time surveillance for signalizedintersections and freeway monitoring.l Traffic parameter measurement such as average speed, road occupancy, traffic count by type of vehicle, and queue length at lights.l Very accurate vehicle presence detection.l Vehicle height measurement for bridge, tunnel, or overpass warning.l Road and freeway accident detection by traffic speed measurement.l Temporary emergency replacement for disabled in-pavement inductive loops.l Operation where inductive loops are impractical:bridges, parking garages, or cobblestone or brick streets.PROBLEM STATEMENT Because ITS is such a new program, a set of precise requirements for VDAC does not exist. The first several months of the project were used to establish these requirements through the aid of a vehicle sensor survey and phone conversations with potential users. After the survey results were analyzed, a detailed product design specification was generated.FIGURE 3 VDAC hardware showing rotating polygon.FIGURE 2 Three-dimensional range image of a van pulling a boat.23 6 9 12 15 18NUMBER OF VEHICLE CLASSESFIGURE 5 Example histogram showing number of vehicle classes required.The survey revealed that the most common VDAC requirements not satisfied by current sensors are vehicle separation and classification, particularly under high-volume, high-speed traffic conditions. Survey responses indicated interest in the following areas of application (in order of interest): (a) traffic data collection, (b) traffic signal control, (c) temporary installations, and (d) electronic toll collection. For the most part, it was not possible to categorize questionnaire response according to application area because respondents indicated an interest in more than one area. This was not true for the electronic toll collection area, however, which was of singular interest in three of four cases (e.g., Hughes Transportation Management Systems, Amtech Systems Corporation, and MFS Network Technologies). These potential VDAC users want sensors that are very accurate (99.9 to 99.9999 percent detection accuracy, 95 to 99.95 percent classification accuracy), highly reliable, and have a long lifetime (2.3 to 5 years). They are concerned about the effect of environmental conditions on sensor performance, particularly weather (rain, fog, snow) and temperature (minus 40o to 85o C). On the basis of their need for high detection and classification accuracy, the electronic toll collection companies appear to be prime customers for VDAC systems.4PRODUCT DESIGN SPECIFICATIONThe product design specification presented in Table 1 was established on the basis of (a) the results of a vehicle-sensor survey implemented via questionnaires mailed to potential VDAC users, (b) discussions with major ITS companies (e.g., MFS Network Technologies and Hughes Transportation Management Systems), and (c) previous SEO experience in developing diode-laser-based vehicle sensors.RESEARCH APPROACHA schematic diagram of the VDAC system is shown in Figure 6. The VDAC’s laser rangefmder uses an InGaAs diode-laser transmitter and a silicon avalanche photodiode (APD) receiver in a side-by-side configuration. The transmitter consists of the diode laser and its driver circuit and a collimating lens. The optical receiver is composed of an objective lens, narrow-band optical filter, detector-amplifier, and threshold detector.The laser diode used in the VDAC is an InGaAs injection laser diode having 12-W output at 10 A pulsed current drive. The laser driver produces a 10-A peak current pulse with a 3-nsec rise time and an 8-nsec pulseTABLE 1 VDAC SpecificationsSCAN RATEFIELD-OF-REGARDSCAN RESOLUTIONBEAM SEPARATIONRANGE MEASUREMENTS PER SCAN MAXIMUM RANGEMINIMUM RANGERANGE ACCURACYRANGE RESOLUTIONINTERFACELASER BEAM GEOMETRYLASER WAVELENGTHLASER EYE SAFETYPOWER SUPPLY VOLTAGE TEMPERATURE RANGEVEHICLE CLASSIFICATIONSPEED ACCURACY 360 SCANS / SEC / BEAM30”1”IO”3050 FT5 FT3 IN3 INRS422, RS232SOLID STATE RELAY- PRESENCE LOGIC-LEVEL (l-l-L) PRESENCEIN-LANE AXIS - 0.5 mradCROSS-LANE AXIS - 16 mrad904 nm“EYE SAFE”IN COMPLIANCE WlTH 21 CFR 1040 CDRH115VAC, 24VDAC-40o C TO 60o C11 CLASSESSPEED DEPENDENT (see Appendix B)width. A trigger pulse from the scanner control circuit triggers the laser at the proper scan angles. The 904-nm laser emission is at an ideal wavelength for the silicon APD receiver used.The optical detection circuitry converts optical radiation reflected from the vehicle and road to, first, an equivalent electrical analog of the input radiation and, finally, a logic-level signal. The logic-level signals are processed within the range counter logic to yield analog range data, which are read by the microprocessor.An analog range-measurement technique was chosen for VDAC because of its better resolution, smaller size, simpler circuitry, lower power consumption, and lower cost when compared with digital techniques. The analog range measurement circuit, know as a time-to-amplitude converter (TAC), has an accuracy of 1 percent of measured range and a resolution of plus or minus 3 in. TAC uses a constant-current source to charge a capacitor to obtain a linear voltage ramp whose instantaneous value is a measure of elapsed time. The circuit is designed so5that the voltage across the range measurement capacitor begins ramping down from the positive power supply when the laser fires. The ramp is stopped when either a reflected pulse is received or the end of the measurement period is reached. The TAC output is then converted to digital by a fast 1 O-bit analog-to-digital converter.The VDAC sofiware processes the range data and outputs vehicle classification, vehicle speed, and so forth, via a serial interface to a remote computer. The major software functions are identified in the block diagram shown in Figure 7. The algorithms that must be implemented for each function were developed and tested, to some extent, in previous projects. The vehicle detector and speed calculator are used in SEO’s Autosense I unit. The real-time range loop, calibration, and gain adjustment routines have been used in several other projects. The vehicle profiler and vehicle classifier are related to algorithms that have been designed and tested under military research programs. The VDAC rule-based classification algorithm will classify the 11 different types of vehicles shown in Figure 8.Speed, Etc. to PCSelf Tests,Calibration,Gain Adjustment,Threshold Adjust,Dew SenseFIGURE 7 Block diagram for major VDAC software functions.P CARMOTORCYCLE PICKUP TRUCK DELIVERY TRUCK BUSTRACTOR WITHOUT TRAILERTRACTOR WITH 1 TRAILERTRACTOR WITH 2 TRAILERSTRACTOR WITH 3 TRAILERSFIGURE 8 Eleven vehicle types classified by VDAC.Range data are used by the vehicle detection algorithmto determine when a vehicle is present. The vehicledetection algorithm first calculates the range to the roadand then sets a threshold above the road that is used todetermine the presence of a vehicle. A certain number ofconsecutive range samples above the detection thresholdare required to accurately detect the presence of a vehicleand reduce false alarms.RESULTSSE0 tested VDAC at a site in front of the SE0 facilitieson Florida SR 441. VDAC was mounted to a mast armextending over the curb lane of this major arterial asshown in Figure 9. Testing was carried out 24 hr/day foran extended period of time. This permitted testing undervaried traffic conditions, including peak-hour, off-peak,and stop and go, and under varied environmentalconditions such as rain, fog, and high temperature.During testing, the VDAC algorithm was modified asrequired to optimize vehicle detection and classificationcapabilities. The program code was uploadable to VDACvia the serial interface, making possible the real-timeoptimization of VDAC performance.FIGURE 9 VDAC mounted on mast arm.FIGURE 10 Computer display of real-time VDAC classification data.The vehicle profiles were collected and organized in adata base. By using the data base, specific vehicle typeswere extracted and used for vehicle-classificationalgorithm development. After the classification algorithmwas developed, a search of the data base provided datafrom similar vehicles for classification algorithm testing.The data base contains fields that include vehicle class,height, length, speed, and so forth, corresponding to eachvehicle detected. A video image was captured and storedfor each vehicle for easy verification of the vehicle-classification algorithm. A computer display of VDACclassification data, including vehicle profile and videoimage, is shown in Figure 10. Approximately 1,200vehicles per hour can be verified using the data basedisplay software. Currently 50,000 vehicles are logged inthe data base.vehicles. The top matrix shows the vehicle count and the bottom matrix the percentage of classification. The numbers along the diagonal of the bottom matrix show the percentage of classification for each vehicle class. Off-diagonal numbers show the possibility of confusion between specific vehicle classes. For example, 5.04percent of pickups were confused with passenger cars.The overall percentage of classification for all vehicle classes is shown in the lower right comer of Figure 11.CONCLUSION Tests performed have included detection accuracy,classification accuracy, and speed accuracy. Detection of100 percent was visually confirmed in a test of 10,000vehicles. The detection accuracy tests were performed infair weather with some light rain and therefore theaccuracy might degrade some during extended tests orduring severe weather conditions. Classification accuracyof 95.5 percent for 10 vehicle classes was achieved usingthe 50,000~vehicle data base. The confusion matrix(Figure 11) shows the classification results for the 50,000Schwartz Electra-Optics has developed a diode-laser-based vehicle detector-classifier that can accurately detect and classify vehicles moving at freeway speeds. Several major ITS companies have expressed a desire to purchase VDACs when they become available for electronic toll and traffic management (ETTM) applications. Such applications require sensors that are very accurate and highly reliable and have a long lifetime. SEO is confident that VDAC will prove useful for traffic surveillance and signal control as well as ETTM applications.VDAC may find its first implementation as part of the Toronto Highway 407 project for Hughes Traffic Management Systems, where 300 to 400 VDACs will be used as part of a completely automated overhead tollcollection system. In this ETTM application VDAC isrequired to provide accurate vehicle detection,classification, separation, lane position, and cameratrigger information to the roadside toll collection system.Production of VDACs for this project was to begin in thelast quarter of 1995.GLOSSARYSeparation-the ability to detect closely spaced vehicles Camera trigger-a signal generated by the VDAC when it detects the end of a vehicle. This signal can beused to trigger a video camera to capture an image ofthe vehicle’s license plate.as individual vehicles.Transverse height profile-the height profile measured across the vehicle from side to side.Classification-the capability of differentiating among types of vehicles.REFERENCESDetection-the ability to sense that a vehicle, whether moving or stopped, has appeared in the detector’s field of view.Detection response time-the time it takes to sense that a vehicle is in the detector’s field of view.1. Strategic Plan for intelligent Vehicle-Highway Systems,Report ITS-AMER-92-3. ITS America, Washington,D.C., 1992.2. Kell, J.H., I.J. Fullerton, and M.K. Mills. TrafficDetector Handbook, 2nd ed. Report FHWA-IP-90-002,U.S. Department of Transportation, July 1990.Electronic toll collection-the completely automated process of billing on toll roads using electronic communication with the ser-a device that amplifies light and produces an intense monochromatic beam.Scan resolution-the angle between successive range measurements.iscTrck/Bus/RV 0 0. .Tractor TrailerPickup 0.001 0.000.001 2.841FIGURE 11Vehicle classification confusion matrix.APPENDIX A:VEHICLE-SENSOR SURVEY Currently used sensor(s)TYPEREQUIREMENTS METREQUIREMENTS NOT METFUTURE REQUIREMENTSApplicationq Traffic signal controlq Traffic data collection[] Electronic toll collectionq Temporary Installations[]O t h e rCHARACTERISTICS DESIRED INVEHICLE DETECTOR/CLASSIFIER 1. Please rate the importance of these functional characteristics:LOW DETECT VEHICLE PRESENCE 1 2 3 MEASURE VEHICLE SPEED 1 2 3 MEASURE VEHICLE HEIGHT 1 2 3 MEASURE VEHICLE LENGTH 1 2 3 MEASURE VEHICLE WIDTH 1 2 3 VEHICLE CLASSIFICATION 1 2 3 SEPARATE CLOSE-SPACED VEH 1 2 3 DETECT TOW BARS 1 2 3 MULTIPLE LANE COVERAGE 1 2 3HIGH 4 5 6 7 4 5 6 7 4 5 6 7 4 5 6 7 4 5 6 7 4 5 6 7 4 5 6 7 4 5 6 7 4 5 6 76. Please specify required environmental conditions:AMBIENT TEMPERATURE RANGESHOCKVIBRATION7. Please circle the appropriate value for these general characteristics:PRICE 1 2 3 4 5 6 7 8 9 10 K$ SIZE 200 400 600 800 1000. 3m WEIGHT 2 4 6 8 10 12 14 16 18 20 lb POWER 1 2 3 4 5 6 7 8 9 10 W120 VAC 24VDAC 12VDAC OTHERAdditional Comments:。
THE PROGRAM EVALUATION STANDARDS JCESS2011版
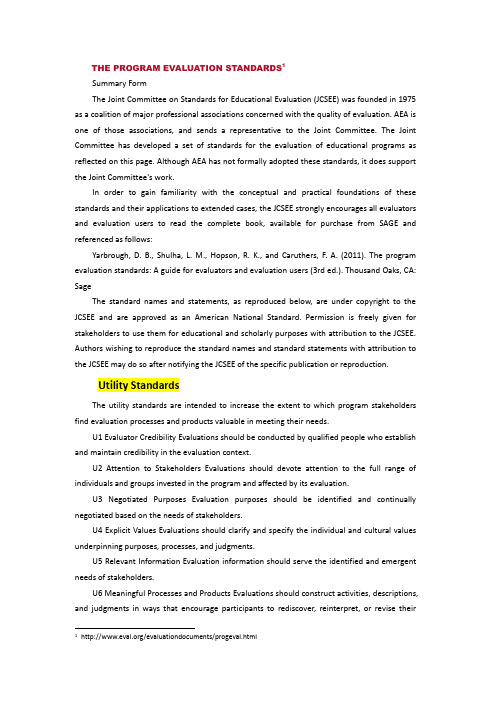
THE PROGRAM EVALUATION STANDARDS1Summary FormThe Joint Committee on Standards for Educational Evaluation (JCSEE) was founded in 1975 as a coalition of major professional associations concerned with the quality of evaluation. AEA is one of those associations, and sends a representative to the Joint Committee. The Joint Committee has developed a set of standards for the evaluation of educational programs as reflected on this page. Although AEA has not formally adopted these standards, it does support the Joint Committee's work.In order to gain familiarity with the conceptual and practical foundations of these standards and their applications to extended cases, the JCSEE strongly encourages all evaluators and evaluation users to read the complete book, available for purchase from SAGE and referenced as follows:Yarbrough, D. B., Shulha, L. M., Hopson, R. K., and Caruthers, F. A. (2011). The program evaluation standards: A guide for evaluators and evaluation users (3rd ed.). Thousand Oaks, CA: SageThe standard names and statements, as reproduced below, are under copyright to the JCSEE and are approved as an American National Standard. Permission is freely given for stakeholders to use them for educational and scholarly purposes with attribution to the JCSEE. Authors wishing to reproduce the standard names and standard statements with attribution to the JCSEE may do so after notifying the JCSEE of the specific publication or reproduction.Utility StandardsThe utility standards are intended to increase the extent to which program stakeholders find evaluation processes and products valuable in meeting their needs.U1 Evaluator Credibility Evaluations should be conducted by qualified people who establish and maintain credibility in the evaluation context.U2 Attention to Stakeholders Evaluations should devote attention to the full range of individuals and groups invested in the program and affected by its evaluation.U3 Negotiated Purposes Evaluation purposes should be identified and continually negotiated based on the needs of stakeholders.U4 Explicit Values Evaluations should clarify and specify the individual and cultural values underpinning purposes, processes, and judgments.U5 Relevant Information Evaluation information should serve the identified and emergent needs of stakeholders.U6 Meaningful Processes and Products Evaluations should construct activities, descriptions, and judgments in ways that encourage participants to rediscover, reinterpret, or revise their1/evaluationdocuments/progeval.htmlunderstandings and behaviors.U7 Timely and Appropriate Communicating and Reporting Evaluations should attend to the continuing information needs of their multiple audiences.U8 Concern for Consequences and Influence Evaluations should promote responsible and adaptive use while guarding against unintended negative consequences and misuse.Feasibility StandardsThe feasibility standards are intended to increase evaluation effectiveness and efficiency.F1 Project Management Evaluations should use effective project management strategies.F2 Practical Procedures Evaluation procedures should be practical and responsive to the way the program operates.F3 Contextual Viability Evaluations should recognize, monitor, and balance the cultural and political interests and needs of individuals and groups.F4 Resource Use Evaluations should use resources effectively and efficiently.Propriety StandardsThe propriety standards support what is proper, fair, legal, right and just in evaluations.P1 Responsive and Inclusive Orientation Evaluations should be responsive to stakeholders and their communities.P2 Formal Agreements Evaluation agreements should be negotiated to make obligations explicit and take into account the needs, expectations, and cultural contexts of clients and other stakeholders.P3 Human Rights and Respect Evaluations should be designed and conducted to protect human and legal rights and maintain the dignity of participants and other stakeholders.P4 Clarity and Fairness Evaluations should be understandable and fair in addressing stakeholder needs and purposes.P5 Transparency and Disclosure Evaluations should provide complete descriptions of findings, limitations, and conclusions to all stakeholders, unless doing so would violate legal and propriety obligations.P6 Conflicts of Interests Evaluations should openly and honestly identify and address real or perceived conflicts of interests that may compromise the evaluation.P7 Fiscal Responsibility Evaluations should account for all expended resources and comply with sound fiscal procedures and processes.Accuracy StandardsThe accuracy standards are intended to increase the dependability and truthfulness of evaluation representations, propositions, and findings, especially those that support interpretations and judgments about quality.A1 Justified Conclusions and Decisions Evaluation conclusions and decisions should be explicitly justified in the cultures and contexts where they have consequences.A2 Valid Information Evaluation information should serve the intended purposes and support valid interpretations.A3 Reliable Information Evaluation procedures should yield sufficiently dependable and consistent information for the intended uses.A4 Explicit Program and Context Descriptions Evaluations should document programs and their contexts with appropriate detail and scope for the evaluation purposes.A5 Information Management Evaluations should employ systematic information collection, review, verification, and storage methods.A6 Sound Designs and Analyses Evaluations should employ technically adequate designs and analyses that are appropriate for the evaluation purposes.A7 Explicit Evaluation Reasoning Evaluation reasoning leading from information and analyses to findings, interpretations, conclusions, and judgments should be clearly and completely documented.A8 Communication and Reporting Evaluation communications should have adequate scope and guard against misconceptions, biases, distortions, and errors.Evaluation Accountability StandardsThe evaluation accountability standards encourage adequate documentation of evaluations and a metaevaluative perspective focused on improvement and accountability for evaluation processes and products.E1 Evaluation Documentation Evaluations should fully document their negotiated purposes and implemented designs, procedures, data, and outcomes.E2 Internal Metaevaluation Evaluators should use these and other applicable standards to examine the accountability of the evaluation design, procedures employed, information collected, and outcomes.E3 External Metaevaluation Program evaluation sponsors, clients, evaluators, and other stakeholders should encourage the conduct of external metaevaluations using these and other applicable standards.方案的评价标准总结表(JCSEE)教育评价标准联合委员会成立于1975年,作为与质量评价有关的主要专业协会的联盟。
Omniture 教程

示例6 (如何搜索我负责的自营商品 “列表页”流量?)
示例6 (如何搜索我负责的自营商品 “列表页”流量?)
示例7 (焦点图引流的促销页,对订单的贡献)
1. 内部跟踪代码(eVar),不可看退出率等流量指标。 2. 页面查看代表,点开该页面后所有查看的页面PV汇总。 3. “订单”分配给最近一个触发的 内部跟踪代码。
Step3: 报表中 筛选欲查看页面
示例3 (从某渠道来的用户,主要的站内路径是什么?)
示例4 (“页面”级别太细,是否可替换其他级别?)
Step1: 用 “Debug” 查看页面代码
示例5 (如何搜索我负责的自营商品流量?)
示例5 (如何搜索我负责的自营商品流量?)
此报表的数据代表: 自营商品“详情页”的流量 若 “购物车”中包含该品类商品,也会包含。
每周独特访客 (周UV)
每日独特访客 (日UV)
每月独特访客 (月UV)
每小时独特访客 (小时UV)
访问 Visits
访问 访问量度始终与某个时段关联,因此当同一访客返回您的站点时,您可以知道是否将其认为是 新的访问。会话在用户首次到达您的网站时开始,并在遇到以下三种情况之一时结束: • 非活动状态持续 30 分钟:几乎所有会话都以这种形式结束。如果前后图像请求之间经过了 30 分钟以上,则开始一次新的访问。 • 活动状态持续 12 个小时:如果用户触发图像请求达 12 个小时且从未超过 30 分钟的间隔, 则自动开始一次新的访问。 • 2500 次点击:如果用户在不启动新会话的情况下生成大量的点击次数,那么在 2500 次图 像请求后会认为是新的访问。 • 100 秒内 100 次点击:如果某次访问在 100 秒内有超过 100 次点击,那么该次访问将自动 结束。此行为通常是机器人行为,我们会强制执行该限制,以避免这些频繁处理的访问造成 延迟增加以及生成报表的时间增加。
66种用户研究方法
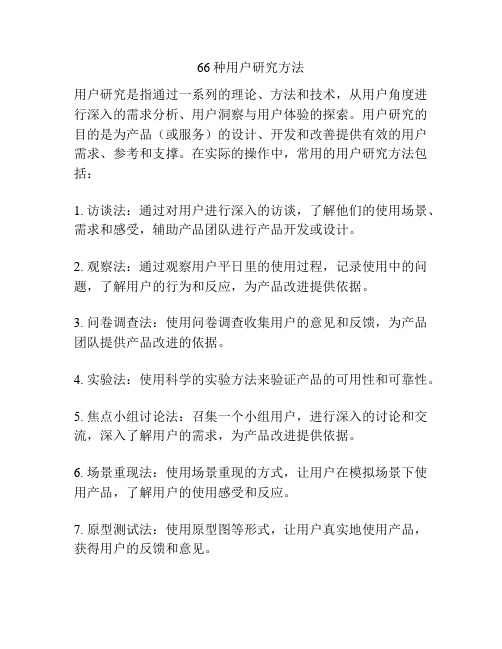
66种用户研究方法用户研究是指通过一系列的理论、方法和技术,从用户角度进行深入的需求分析、用户洞察与用户体验的探索。
用户研究的目的是为产品(或服务)的设计、开发和改善提供有效的用户需求、参考和支撑。
在实际的操作中,常用的用户研究方法包括:1. 访谈法:通过对用户进行深入的访谈,了解他们的使用场景、需求和感受,辅助产品团队进行产品开发或设计。
2. 观察法:通过观察用户平日里的使用过程,记录使用中的问题,了解用户的行为和反应,为产品改进提供依据。
3. 问卷调查法:使用问卷调查收集用户的意见和反馈,为产品团队提供产品改进的依据。
4. 实验法:使用科学的实验方法来验证产品的可用性和可靠性。
5. 焦点小组讨论法:召集一个小组用户,进行深入的讨论和交流,深入了解用户的需求,为产品改进提供依据。
6. 场景重现法:使用场景重现的方式,让用户在模拟场景下使用产品,了解用户的使用感受和反应。
7. 原型测试法:使用原型图等形式,让用户真实地使用产品,获得用户的反馈和意见。
8. 常规化思考法:通过对用户进行用户体验设计师学韵递进的常规化思考法训练,培养团队的设计能力和思考深度。
9. 网络分析法:通过分析用户在社交媒体、论坛、博客等网站上的言论行为,了解用户的需求和反馈。
10. 追踪法:通过自然形式和评价形式的追踪,观察用户的使用习惯,说明并反馈使用化的人工体验。
11. 可用性测试法:使用心理学、人机交互原理等专业技术对产品进行可用性测试,在整个产品设计和迭代过程中不断提高产品的用户体验。
12. 登入登出法:记录和呈现用户的行为,从而了解用户使用的情况。
13. 上传下载法:检查用户使用产品、文件下载、软件安装等行为,研究用户需求、偏好和使用体验。
14. 基于观察法:在用户使用产品过程中,记录用户持续时间、浏览时间、操作频次等相关数据,理解用户使用模式和行为。
15. 操作回想法:在用户绘制日志时,在用户使用过程中提供后续的关注和处理,从而了解用户使用体验。
Toward the Next Generation of Recommender Systems A Survey of the State-of-the-Art and Possible Exte

Toward the Next Generation of Recommender Systems:A Survey of the State-of-the-Art andPossible ExtensionsGediminas Adomavicius,Member,IEEE,and Alexander Tuzhilin,Member,IEEE Abstract—This paper presents an overview of the field of recommender systems and describes the current generation ofrecommendation methods that are usually classified into the following three main categories:content-based,collaborative,and hybrid recommendation approaches.This paper also describes various limitations of current recommendation methods and discussespossible extensions that can improve recommendation capabilities and make recommender systems applicable to an even broader range of applications.These extensions include,among others,an improvement of understanding of users and items,incorporation of the contextual information into the recommendation process,support for multcriteria ratings,and a provision of more flexible and less intrusive types of recommendations.Index Terms—Recommender systems,collaborative filtering,rating estimation methods,extensions to recommender systems.æ1I NTRODUCTIONR ECOMMENDER systems have become an important research area since the appearance of the first papers on collaborative filtering in the mid-1990s[45],[86],[97]. There has been much work done both in the industry and academia on developing new approaches to recommender systems over the last decade.The interest in this area still remains high because it constitutes a problem-rich research area and because of the abundance of practical applications that help users to deal with information overload and provide personalized recommendations, content,and services to them.Examples of such applica-tions include recommending books,CDs,and other products at [61],movies by MovieLens [67],and news at VERSIFI Technologies(formerly )[14].Moreover,some of the vendors have incorporated recommendation capabilities into their commerce servers[78].However,despite all of these advances,the current generation of recommender systems still requires further improvements to make recommendation methods more effective and applicable to an even broader range of real-life applications,including recommending vacations,certain types of financial services to investors,and products to purchase in a store made by a“smart”shopping cart[106]. These improvements include better methods for represent-ing user behavior and the information about the items to be recommended,more advanced recommendation modeling methods,incorporation of various contextual information into the recommendation process,utilization of multcriteria ratings,development of less intrusive and more flexible recommendation methods that also rely on the measures that more effectively determine performance of recommen-der systems.In this paper,we describe various ways to extend the capabilities of recommender systems.However,before doing this,we first present a comprehensive survey of the state-of-the-art in recommender systems in Section2.Then, we identify various limitations of the current generation of recommendation methods and discuss some initial ap-proaches to extending their capabilities in Section3.2T HE S URVEY OF R ECOMMENDER S YSTEMS Although the roots of recommender systems can be traced back to the extensive work in cognitive science[87], approximation theory[81],information retrieval[89], forecasting theories[6],and also have links to management science[71]and to consumer choice modeling in marketing [60],recommender systems emerged as an independent research area in the mid-1990s when researchers started focusing on recommendation problems that explicitly rely on the ratings structure.In its most common formulation, the recommendation problem is reduced to the problem of estimating ratings for the items that have not been seen by a user.Intuitively,this estimation is usually based on the ratings given by this user to other items and on some other information that will be formally described below.Once we can estimate ratings for the yet unrated items,we can recommend to the user the item(s)with the highest estimated rating(s).More formally,the recommendation problem can be formulated as follows:Let C be the set of all users and let S be the set of all possible items that can be recommended, such as books,movies,or restaurants.The space S of.G.Adomavicius is with the Carlson School of Management,University ofMinnesota,32119th Avenue South,Minneapolis,MN55455.E-mail:gedas@.. A.Tuzhilin is with the Stern School of Business,New York University,44West4th Street,New York,NY10012.E-mail:atuzhili@.Manuscript received8Mar.2004;revised14Oct.2004;accepted10Dec.2004;published online20Apr.2005.For information on obtaining reprints of this article,please send e-mail to:tkde@,and reference IEEECS Log Number TKDE-0071-0304.1041-4347/05/$20.00ß2005IEEE Published by the IEEE Computer Societypossible items can be very large,ranging in hundreds of thousands or even millions of items in some applications,such as recommending books or CDs.Similarly,the user space can also be very large—millions in some cases.Let u be a utility function that measures the usefulness of item s to user c ,i.e.,u :C ÂS !R ,where R is a totally ordered set (e.g.,nonnegative integers or real numbers within a certain range).Then,for each user c 2C ,we want to choose such item s 02S that maximizes the user’s utility.More formally:8c 2C;s 0c ¼arg max s 2Su ðc;s Þ:ð1ÞIn recommender systems,the utility of an item is usually represented by a rating ,which indicates how a particular user liked a particular item,e.g.,John Doe gave the movie “Harry Potter”the rating of 7(out of 10).However,as indicated earlier,in general,utility can be an arbitrary function,including a profit function.Depending on the application,utility u can either be specified by the user,as is often done for the user-defined ratings,or is computed by the application,as can be the case for a profit-based utility function.Each element of the user space C can be defined with a profile that includes various user characteristics,such as age,gender,income,marital status,etc.In the simplest case,the profile can contain only a single (unique)element,such as User ID.Similarly,each element of the item space S is defined with a set of characteristics.For example,in a movie recommendation application,where S is a collection of movies,each movie can be represented not only by its ID,but also by its title,genre,director,year of release,leading actors,etc.The central problem of recommender systems lies in that utility u is usually not defined on the whole C ÂS space,but only on some subset of it.This means u needs to be extrapolated to the whole space C ÂS .In recommender systems,utility is typically represented by ratings and is initially defined only on the items previously rated by the users.For example,in a movie recommendation application (such as the one at ),users initially rate some subset of movies that they have already seen.An example of a user-item rating matrix for a movie recommendation application is presented in Table 1,where ratings are specified on the scale of 1to 5.The “ ”symbol for some of the ratings in Table 1means that the users have not rated the corresponding movies.Therefore,the recommendation engine should be able to estimate (predict)the ratings of the nonrated movie/user combinations and issue appropriate recommendations based on these predictions.Extrapolations from known to unknown ratings are usually done by 1)specifying heuristics that define the utility function and empirically validating its performanceand 2)estimating the utility function that optimizes certain performance criterion,such as the mean square error.Once the unknown ratings are estimated,actual recommendations of an item to a user are made by selecting the highest rating among all the estimated ratings for that user,according to (1).Alternatively,we can recommend the N best items to a user or a set of users to an item.The new ratings of the not-yet-rated items can be estimated in many different ways using methods from machine learning,approximation theory,and various heuristics.Recommender systems are usually classified according to their approach to rating estimation and,in the next section,we will present such a classification that was proposed in the literature and will provide a survey of different types of recommender systems.The commonly accepted formulation of the recommendation problem was first stated in [45],[86],[97]and this problem has been studied extensively since then.Moreover,recommender systems are usually classified into the following categories,based on how recommendations are made [8]:.Content-based recommendations :The user will be recommended items similar to the ones the user preferred in the past;.Collaborative recommendations :The user will berecommended items that people with similar tastes and preferences liked in the past;.Hybrid approaches :These methods combine colla-borative and content-based methods.In addition to recommender systems that predict the absolute values of ratings that individual users would give to the yet unseen items (as discussed above),there has been work done on preference-based filtering ,i.e.,predicting the relative preferences of users [22],[35],[51],[52].For example,in a movie recommendation application,prefer-ence-based filtering techniques would focus on predicting the correct relative order of the movies,rather than their individual ratings.However,this paper focuses primarily on rating-based recommenders since it constitutes the most popular approach to recommender systems.2.1Content-Based MethodsIn content-based recommendation methods,the utility u ðc;s Þof item s for user c is estimated based on the utilities u ðc;s i Þassigned by user c to items s i 2S that are “similar”to item s .For example,in a movie recommendation application,in order to recommend movies to user c ,the content-based recommender system tries to understand the commonalities among the movies user c has rated highly in the past (specific actors,directors,genres,subject matter,TABLE 1A Fragment of a Rating Matrix for a Movie Recommender Systemetc.).Then,only the movies that have a high degree of similarity to whatever the user’s preferences are would be recommended.The content-based approach to recommendation has its roots in information retrieval[7],[89]and information filtering[10]research.Because of the significant and early advancements made by the information retrieval and filtering communities and because of the importance of several text-based applications,many current content-based systems focus on recommending items containing textual information,such as documents,Web sites(URLs),and Usenet news messages.The improvement over the tradi-tional information retrieval approaches comes from the use of user profiles that contain information about users’tastes, preferences,and needs.The profiling information can be elicited from users explicitly,e.g.,through questionnaires, or implicitly—learned from their transactional behavior over time.More formally,let ContentðsÞbe an item profile,i.e.,a set of attributes characterizing item s.It is usually computed by extracting a set of features from item s(its content)and is used to determine the appropriateness of the item for recommendation purposes.Since,as mentioned earlier, content-based systems are designed mostly to recommend text-based items,the content in these systems is usually described with keywords.For example,a content-based component of the Fab system[8],which recommends Web pages to users,represents Web page content with the 100most important words.Similarly,the Syskill&Webert system[77]represents documents with the128most informative words.The“importance”(or“informative-ness”)of word k j in document d j is determined with some weighting measure w ij that can be defined in several different ways.One of the best-known measures for specifying keyword weights in Information Retrieval is the term frequency/inverse document frequency(TF-IDF)measure[89]that is defined as follows:Assume that N is the total number of documents that can be recommended to users and that keyword k j appears in n i of them.Moreover,assume that f i;j is the number of times keyword k i appears in document d j.Then, T F i;j,the term frequency(or normalized frequency)of keyword k i in document d j,is defined asT F i;j¼f i;jmax z f z;j;ð2Þwhere the maximum is computed over the frequencies f z;j of all keywords k z that appear in the document d j. However,keywords that appear in many documents are not useful in distinguishing between a relevant document and a nonrelevant one.Therefore,the measure of inverse document frequencyðIDF iÞis often used in combination with simple term frequencyðT F i;jÞ.The inverse document frequency for keyword k i is usually defined asIDF i¼log Nn i:ð3ÞThen,the TF-IDF weight for keyword k i in document d j is defined asw i;j¼T F i;jÂIDF ið4Þand the content of document d j is defined asContentðd jÞ¼ðw1j;...w kjÞ:As stated earlier,content-based systems recommend items similar to those that a user liked in the past[56],[69], [77].In particular,various candidate items are compared with items previously rated by the user and the best-matching item(s)are recommended.More formally,let ContentBasedP rofileðcÞbe the profile of user c containing tastes and preferences of this user.These profiles are obtained by analyzing the content of the items previously seen and rated by the user and are usually constructed using keyword analysis techniques from information retrieval.For example,ContentBasedP rofileðcÞcan be defined as a vector of weightsðw c1;...;w ckÞ,where each weight w ci denotes the importance of keyword k i to user c and can be computed from individually rated content vectors using a variety of techniques.For example,some averaging approach,such as Rocchio algorithm[85],can be used to compute ContentBasedP rofileðcÞas an“average”vector from an individual content vectors[8],[56].On the other hand,[77]uses a Bayesian classifier in order to estimate the probability that a document is liked.The Winnow algorithm[62]has also been shown to work well for this purpose,especially in the situations where there are many possible features[76].In content-based systems,the utility function uðc;sÞis usually defined as:uðc;sÞ¼scoreðContentBasedP rofileðcÞ;ContentðsÞÞ:ð5ÞUsing the above-mentioned information retrieval-based paradigm of recommending Web pages,Web site URLs, or Usenet news messages,both ContentBasedP rofileðcÞof user c and ContentðsÞof document s can be represented as TF-IDF vectors~w c and~w s of keyword weights.Moreover, utility function uðc;sÞis usually represented in the information retrieval literature by some scoring heuristic defined in terms of vectors~w c and~w s,such as the cosine similarity measure[7],[89]:uðc;sÞ¼cosð~w c;~w sÞ¼~w cÁ~w sjj~w c jj2Âjj~w s jj2¼P Ki¼1w i;c w i;sffiffiffiffiffiffiffiffiffiffiffiffiffiffiffiffiffiffiffiP Ki¼1w2i;cqffiffiffiffiffiffiffiffiffiffiffiffiffiffiffiffiffiffiffiP Ki¼1w2i;sq;ð6Þwhere K is the total number of keywords in the system.For example,if user c reads many online articles on the topic of bioinformatics,then content-based recommenda-tion techniques will be able to recommend other bioinfor-matics articles to user c.This is the case because these articles will have more bioinformatics-related terms(e.g.,“genome,”“sequencing,”“proteomics”)than articles on other topics and,therefore,ContentBasedP rofileðcÞ,as defined by vector~w c,will represent such terms k i with high weights w ic.Consequently,a recommender system using the cosine or a related similarity measure will assign higher utility uðc;sÞto those articles s that have high-weighted bioinformatics terms in~w s and lower utility to the ones where bioinformatics terms are weighted less.Besides the traditional heuristics that are based mostly on information retrieval methods,other techniques for content-based recommendation have also been used,such as Bayesian classifiers[70],[77]and various machine learning techniques,including clustering,decision trees, and artificial neural networks[77].These techniques differ from information retrieval-based approaches in that they calculate utility predictions based not on a heuristic formula,such as a cosine similarity measure,but rather are based on a model learned from the underlying data using statistical learning and machine learning techni-ques.For example,based on a set of Web pages that were rated as“relevant”or“irrelevant”by the user,[77]uses the naive Bayesian classifier[31]to classify unrated Web pages.More specifically,the naive Bayesian classifier is used to estimate the following probability that page p j belongs to a certain class C i(e.g.,relevant or irrelevant) given the set of keywords k1;j;...;k n;j on that page:PðC i j k1;j&...&k n;jÞ:ð7ÞMoreover,[77]uses the assumption that keywords are independent and,therefore,the above probability is proportional toPðC iÞYxPðk x;j j C iÞ:ð8ÞWhile the keyword independence assumption does not necessarily apply in many applications,experimental results demonstrate that naı¨ve Bayesian classifiers still produce high classification accuracy[77].Furthermore,both Pðk x;j j C iÞand PðC iÞcan be estimated from the underlying training data.Therefore,for each page p j,the probability PðC i j k1;j&...&k n;jÞis computed for each class C i and page p j is assigned to class C i having the highest probability[77].While not explicitly dealing with providing recommen-dations,the text retrieval community has contributed several techniques that are being used in content-based recommen-der systems.One example of such a technique would be the research on adaptive filtering[101],[112],which focuses on becoming more accurate at identifying relevant documents incrementally by observing the documents one-by-one in a continuous document stream.Another example would be the work on threshold setting[84],[111],which focuses on determining the extent to which documents should match a given query in order to be relevant to the user.Other text retrieval methods are described in[50]and can also be found in the proceedings of the Text Retrieval Conference (TREC)().As was observed in[8],[97],content-based recommender systems have several limitations that are described in the rest of this section.2.1.1Limited Content AnalysisContent-based techniques are limited by the features that are explicitly associated with the objects that these systems recommend.Therefore,in order to have a sufficient set of features,the content must either be in a form that can be parsed automatically by a computer(e.g.,text)or the features should be assigned to items manually.While information retrieval techniques work well in extracting features from text documents,some other domains have an inherent problem with automatic feature extraction.For example,automatic feature extraction methods are much harder to apply to multimedia data,e.g.,graphical images, audio streams,and video streams.Moreover,it is often not practical to assign attributes by hand due to limitations of resources[97].Another problem with limited content analysis is that,if two different items are represented by the same set of features,they are indistinguishable.Therefore,since text-based documents are usually represented by their most important keywords,content-based systems cannot distin-guish between a well-written article and a badly written one,if they happen to use the same terms[97].2.1.2OverspecializationWhen the system can only recommend items that score highly against a user’s profile,the user is limited to being recommended items that are similar to those already rated. For example,a person with no experience with Greek cuisine would never receive a recommendation for even the greatest Greek restaurant in town.This problem,which has also been studied in other domains,is often addressed by introducing some randomness.For example,the use of genetic algorithms has been proposed as a possible solution in the context of information filtering[98].In addition,the problem with overspecialization is not only that the content-based systems cannot recommend items that are different from anything the user has seen before.In certain cases,items should not be recommended if they are too similar to something the user has already seen,such as a different news article describing the same event.Therefore, some content-based recommender systems,such as Daily-Learner[13],filter out items not only if they are too different from the user’s preferences,but also if they are too similar to something the user has seen before.Furthermore,Zhang et al.[112]provide a set of five redundancy measures to evaluate whether a document that is deemed to be relevant contains some novel information as well.In summary,the diversity of recommendations is often a desirable feature in recommender systems.Ideally,the user should be pre-sented with a range of options and not with a homogeneous set of alternatives.For example,it is not necessarily a good idea to recommend all movies by Woody Allen to a user who liked one of them.2.1.3New User ProblemThe user has to rate a sufficient number of items before a content-based recommender system can really understand the user’s preferences and present the user with reliable recommendations.Therefore,a new user,having very few ratings,would not be able to get accurate recommendations.2.2Collaborative MethodsUnlike content-based recommendation methods,collabora-tive recommender systems(or collaborative filtering systems) try to predict the utility of items for a particular user based on the items previously rated by other users.More formally, the utility uðc;sÞof item s for user c is estimated based on the utilities uðc j;sÞassigned to item s by those users c j2C who are“similar”to user c.For example,in a movierecommendation application,in order to recommend movies to user c ,the collaborative recommender system tries to find the “peers”of user c ,i.e.,other users that have similar tastes in movies (rate the same movies similarly).Then,only the movies that are most liked by the “peers”of user c would be recommended.There have been many collaborative systems developed in the academia and the industry.It can be argued that the Grundy system [87]was the first recommender system,which proposed using stereotypes as a mechanism for building models of users based on a limited amount of information on each individual ing stereotypes,the Grundy system would build individual user models and use them to recommend relevant books to each ter on,the Tapestry system relied on each user to identify like-minded users manually [38].GroupLens [53],[86],Video Recommender [45],and Ringo [97]were the first systems to use collaborative filtering algorithms to automate prediction.Other examples of collaborative recommender systems include the book recommendation system from ,the PHOAKS system that helps people find relevant information on the WWW [103],and the Jester system that recommends jokes [39].According to [15],algorithms for collaborative recom-mendations can be grouped into two general classes:memory-based (or heuristic-based )and model-based .Memory-based algorithms [15],[27],[72],[86],[97]essentially are heuristics that make rating predictions based on the entire collection of previously rated items by the users.That is,the value of the unknown rating r c;s for user c and item s is usually computed as an aggregate of the ratings of some other (usually,the N most similar)users for the same item s :r c;s ¼aggr c 02^Cr c 0;s ;ð9Þwhere ^Cdenotes the set of N users that are the most similar to user c and who have rated item s (N can range anywhere from 1to the number of all users).Some examples of the aggregation function are:ða Þr c;s ¼1N Xc 02^C r c 0;s ;ðb Þr c;s¼k X c 02^Csim ðc;c 0ÞÂr c 0;s ;ðc Þr c;s ¼"rc þk Xc 02^Csim ðc;c 0ÞÂðr c 0;s À"rc 0Þ;ð10Þwhere multiplier k serves as a normalizing factor and is usually selected as k ¼1 P c 02^Cj sim ðc;c 0Þj ,and where the average rating of user c ,"rc ,in (10c)is defined as 1"r c ¼À1 j S c j ÁX s 2S cr c;s;where S c ¼f s 2S j r c;s ¼ g :ð11ÞIn the simplest case,the aggregation can be a simple average,as defined by (10a).However,the most common aggregation approach is to use the weighted sum,shown in (10b).The similarity measure between users c and c 0,sim ðc;c 0Þ,is essentially a distance measure and is used as aweight,i.e.,the more similar users c and c 0are,the more weight rating r c 0;s will carry in the prediction of r c;s .Note that sim ðx;y Þis a heuristic artifact that is introduced in order to be able to differentiate between levels of user similarity (i.e.,to be able to find a set of “closest peers”or “nearest neighbors”for each user)and,at the same time,simplify the rating estimation procedure.As shown in (10b),different recommendation applications can use their own user similarity measure as long as the calculations are normalized using the normalizing factor k ,as shown above.The two most commonly used similarity measures will be described below.One problem with using the weighted sum,as in (10b),is that it does not take into account the fact that different users may use the rating scale differently.The adjusted weighted sum,shown in (10c),has been widely used to address this limitation.In this approach,instead of using the absolute values of ratings,the weighted sum uses their deviations from the average rating of the correspond-ing user.Another way to overcome the differing uses of the rating scale is to deploy preference-based filtering [22],[35],[51],[52],which focuses on predicting the relative prefer-ences of users instead of absolute rating values,as was pointed out earlier in Section 2.Various approaches have been used to compute the similarity sim ðc;c 0Þbetween users in collaborative recom-mender systems.In most of these approaches,the similarity between two users is based on their ratings of items that both users have rated.The two most popular approaches are correlation and cosine-based .To present them,let S xy be the set of all items corated by both users x and y ,i.e.,S xy ¼f s 2S j r x;s ¼ &r y;s ¼ g .In collaborative recom-mender systems,S xy is used mainly as an intermediate result for calculating the “nearest neighbors”of user x and is often computed in a straightforward manner,i.e.,by computing the intersection of sets S x and S y .However,some methods,such as the graph-theoretic approach to collaborative filtering [4],can determine the nearest neighbors of x without computing S xy for all users y .In the correlation-based approach,the Pearson correlation coefficient is used to measure the similarity [86],[97]:sim ðx;y Þ¼Ps 2S xyðr x;s À"rx Þðr y;s À"r y ÞffiffiffiffiffiffiffiffiffiffiffiffiffiffiffiffiffiffiffiffiffiffiffiffiffiffiffiffiffiffiffiffiffiffiffiffiffiffiffiffiffiffiffiffiffiffiffiffiffiffiffiffiffiffiffiffiffiffiffiffiffiffiffiffiffiP s 2S xyðr x;s À"r x Þ2P s 2S xyðr y;s À"ry Þ2r :ð12ÞIn the cosine-based approach [15],[91],the two users x and y are treated as two vectors in m -dimensional space,where m ¼j S xy j .Then,the similarity between two vectors can be measured by computing the cosine of the angle between them:sim ðx;y Þ¼cos ð~x ;~y Þ¼~x Á~yjj ~x jj 2Âjj ~y jj 2¼Ps 2S xy r x;s r y;s ffiffiffiffiffiffiffiffiffiffiffiffiffiffiffiffiffiP s 2S xyr 2x;sr ffiffiffiffiffiffiffiffiffiffiffiffiffiffiffiffiP s 2S xyr2y;sr ;ð13Þwhere ~x Á~y denotes the dot-product between the vectors ~xand ~y .Still another approach to measuring similarity between users uses the mean squared difference measure1.We use the r c;s ¼ notation to indicate that item s has not been rated by user c .。
CNRS-TGI中国城市居民调查(2011)

15
洞察中国市场的专业品牌
我们服务的
客户…
企业主客户…
部分…
媒介主客户…
部分…
广告公司客户…
部分…
19
洞察中国市场的专业品牌
Who is is What CTR? CNRS?
20
洞察中国市场的专业品牌
CNRS是目前国内规模最大的 城市居民连续性研究项目
中国城市居民调查(China National Resident Survey, CNRS) 是CTR在中国大陆进行的关于居民媒体接触习惯、产品及 品牌消费习惯和生活形态的同源连续性大型市场调查。 CNRS于1999年根据国际规范建立,是目前国内规模最大 的城市居民媒介消费和产品消费的连续性研究项目。历经 10年发展,CNRS已经在广告和平面媒体行业确立了“行 业货币”的地位,成为广告主、媒体和广告公司洞察市场 需求、提升营销效果的利器。
22
2011最新进展
CNRS是CTR连续性产品的重要组成部分
电视收视率测评
广播收听率测评
23
也是TGI全球研究网络的核心成员
2010年CTR正式与Kantar媒体研究集团达成战略合作,成 为其TGI全球研究的中国独家合作伙伴。
24
TGI – 具有40年历史的全球同源研究领导者
TGI = Target Group Index(目标群体指数) 由英国市场研究局(BMRB) 于1969年在英国创立,隶属于世界 知名的Kantar媒体研究集团。
8. 惠州 9. 珠海 10. 海口
郑州 武汉 襄樊 长沙 合肥 南昌
一线城市 二线城市 三线城市 四线城市
32
在市场同类研究中, 样本量最大、覆盖最广、数据最具代表性
产品工程师如何进行用户反馈的收集与分析

产品工程师如何进行用户反馈的收集与分析随着互联网和技术的迅猛发展,用户体验成为产品成功的关键因素之一。
对于产品工程师来说,及时而准确地收集和分析用户反馈意见至关重要。
本文将介绍产品工程师在进行用户反馈收集与分析工作中的几种有效方法。
一、用户反馈收集的方式1. 用户调查问卷用户调查问卷是收集用户反馈的常用方法之一。
可以通过在线调查工具,如Google Forms、SurveyMonkey等,创建一份针对产品使用者的调查问卷。
问卷内容应包括用户对产品的满意度、功能建议、问题反馈等。
通过定期发送问卷给用户,产品工程师可以获得大量用户的反馈意见。
2. 用户访谈用户访谈是一种直接和用户进行沟通的方式,可以深入了解用户对产品的使用情况和意见。
产品工程师可以通过电话、视频会议或面对面的方式与用户进行访谈。
这种方法可以更加具体地了解用户的需求,及时解决用户遇到的问题,并获得宝贵的改进建议。
3. 用户行为分析通过用户行为分析工具,如Google Analytics、Hotjar等,产品工程师可以追踪用户在产品中的行为,包括点击、鼠标移动、停留时间等。
通过分析用户的行为数据,产品工程师可以了解用户使用产品的方式和习惯,找出产品中存在的问题和用户反馈意见。
4. 在线社交媒体在社交媒体平台上,用户经常会发表对产品的评价和意见。
产品工程师可以通过监控社交媒体上的讨论,了解用户对产品的反馈和评价。
例如,通过设置关键词的搜索提醒,工程师可以实时收到用户在社交媒体上对产品的讨论和反馈。
二、用户反馈分析的方法1. 整理分类用户反馈将收集到的用户反馈进行整理和分类,按照不同的问题类型、功能建议、Bug反馈等进行分类归类。
确保每一条用户反馈都能被记录和分析,避免重复和遗漏。
2. 优先级排序根据用户反馈的重要性和紧急程度,对反馈进行优先级排序。
将与核心功能相关或用户体验较差的问题置于首位,以优先解决对用户最为关键的问题,提升产品的用户满意度。
Approximate Flavor Symmetries in the Lepton Sector
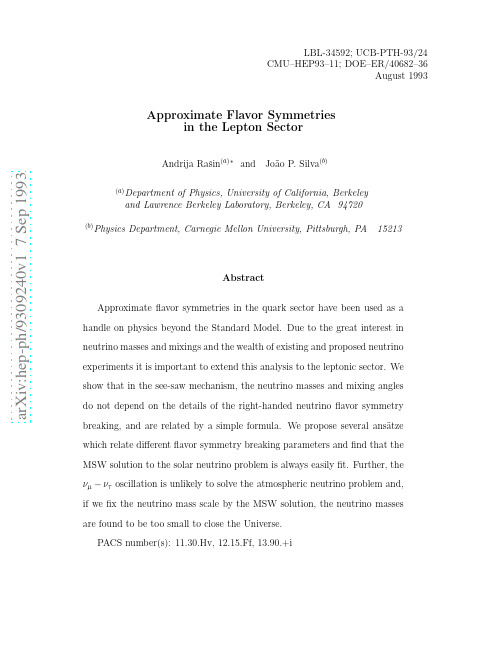
a r X i v :h e p -p h /9309240v 1 7 S e p 1993LBL-34592;UCB-PTH-93/24CMU–HEP93–11;DOE–ER/40682–36August 1993Approximate Flavor Symmetries in the Lepton SectorAndrija Raˇs in (a )∗and Jo˜a o P.Silva (b )(a )Department of Physics,University of California,Berkeley and Lawrence Berkeley Laboratory,Berkeley,CA 94720(b )Physics Department,Carnegie Mellon University,Pittsburgh,PA 15213Abstract Approximate flavor symmetries in the quark sector have been used as a handle on physics beyond the Standard Model.Due to the great interest in neutrino masses and mixings and the wealth of existing and proposed neutrino experiments it is important to extend this analysis to the leptonic sector.We show that in the see-saw mechanism,the neutrino masses and mixing angles do not depend on the details of the right-handed neutrino flavor symmetrybreaking,and are related by a simple formula.We propose several ans¨a tzewhich relate different flavor symmetry breaking parameters and find that theMSW solution to the solar neutrino problem is always easily fit.Further,theνµ−ντoscillation is unlikely to solve the atmospheric neutrino problem and,if we fix the neutrino mass scale by the MSW solution,the neutrino massesare found to be too small to close the Universe.PACS number(s):11.30.Hv,12.15.Ff,13.90.+iDisclaimerThis document was prepared as an account of work sponsored by the United States Government.Neither the United States Government nor any agency thereof,nor The Regents of the University of California, nor any of their employees,makes any warranty,express or implied,or assumes any legal liability or responsibility for the accuracy,completeness,or usefulness of any information,apparatus,product,or process disclosed,or represents that its use would not infringe privately owned rights.Reference herein to any specific commercial products process,or service by its trade name,trademark,manufacturer,or otherwise,does not necessarily constitute or imply its endorsement,recommendation,or favoring by the United States Government or any agency thereof,or The Regents of the University of California.The views and opinions of authors expressed herein do not necessarily state or reflect those of the United States Government or any agency thereof of The Regents of the University of California and shall not be used for advertising or product endorsement purposes.Lawrence Berkeley Laboratory is an equal opportunity employer.1IntroductionThe smallness of the Yukawa couplings has been related to the approximateflavor sym-metries([1],[2]).The Yukawa couplings can be understood as being naturally small [3],since by putting them equal to zero new global symmetries appear in the theory. These are theflavor symmetries under which each of the fermions transforms separately but scalars do not.In the low energy theory these symmetries are broken by different amounts as is evident from the nonzero masses of fermions.The lack of knowledge of the exact mechanism by which the symmetries were broken was parametrized by a set of small numbers(denoted byǫ).Each Yukawa coupling is then given approximately as the product of small symmetry breaking parametersǫfor allflavor symmetries broken by that coupling.So for example the coupling of a scalar doublet H to the ith generation of left-handed doublet quarks Q i and the jth generation of right-handed up quark U j is given byλU ij≈ǫQi ǫUj.(1)Whereas this gives the right order of magnitude for the couplings,the exact relation of couplings to theǫs may be offby a factor of2or3.This is because the underlying theory (possibly a GUT)by which theflavor symmetries are broken is unknown.Therefore all estimates of the possible newflavor changing interactions should be taken to have at least the same uncertainty.Theflavor symmetry breaking parametersǫcan be estimated in several ways.They can be postulated by ans¨a tze[2]which are consistent with the known values of fermion masses and mixings.Alternatively,one may use the experimental results to constrain theǫs.This was done in the quark sector[4]using the known values of quark masses and Kobayashi-Maskawa mixing matrix elements.The important result so obtained was thatflavor changing scalar interactions are possible even for the masses of the new scalars as low as a few hundred GeV to1TeV, and numerous estimates for differentflavor changing interactions were given[2,4].Hall and Weinberg[4]also noticed that allowing for complex Yukawa couplings in this scheme, the observed smallness of CP violation constrains the phases to be small(i.e of the order of10−3).In the lepton sector,the masses are small indicating that the approximateflavor symmetries are preserved to a high degree of acuracy.Therefore leptonflavor changing interactions would be extremely hard to test[2].Further,since the neutrino masses and mixing angles have not been measured yet,one cannot estimate the correspondingǫs without additional assumptions.Nevertheless wefind it important to address the ques-tion of approximateflavor symmetries in the lepton sector,because many experiments which aim to measure or set better limits on neutrino masses and mixings are under way or being planned for the near future.Indeed,in this paper wefind that statements can be made about the lepton sector,regardless of additional assumptions about theǫs.For example,while the MSW solution to the solar neutrino problem can be easilyfit,the predicted neutrino masses are unlikely to close the Universe.This paper is organised as follows.In section2we express the lepton sector Yukawa couplings in terms offlavor symmetry breaking parameters.Here we study two cases of neutrino masses.In the see-saw mechanism[5]case we show that the neutrino masses and mixings are independent of the right-handed neutrinoflavor symmetry breaking mechanism.We also include for completness the case of Dirac neutrino masses only (as in the case of charged fermions).In section3we provide several ans¨a tze for the leptonflavor symmetry breaking parameters and list their predictions in terms of ratios of neutrino masses,and mixings.We study their relevance to the solar neutrino problem, atmospheric neutrino problem,closure of the Universe,etc.General features are notedwhich are independent of the particular ansatz used.2Approximate Flavor Symmetries in the Lepton Sec-torBy adding the right-handed neutrinos N i,i=1,2,3to the particle content of the standard model we can allow for Dirac type masses.Under the action of approximateflavor symmetries,whenever an N i enters a Yukawa interaction,the corresponding coupling must contain the symmetry breaking parameterǫNi.A natural way to justify the smallness of neutrino masses is to use the see-saw mech-anism in which the smallness of the left-handed neutrino masses is explained by the new scale of heavy right-handed neutrinos.The mass matrices will have the following structurem NDij ≈ǫLiǫNjv SM,(2)m NMij ≈ǫNiǫNjv Big,(3)m Eij ≈ǫLiǫEjv SM,(4)where m ND and mE are the neutrino and charged lepton Dirac mass matrices,m NMis theright-handed neutrino Majorana mass matrix,v SM=174GeV and v Big is the new large mass scale.The generation indices i and j run from1to3.In the following we assume ahierarchy in theǫs(i.e.ǫL1<<ǫL2<<ǫL3,etc.)as suggested by the hierarchy of quarkand charged lepton masses.Then the diagonalization of the neutrino mass matrix willgive a heavy sector with masses m NHi ≈ǫ2N iv Big and a very light sector with mass matrixm NLij ≈(m NDm−1NMm T ND)ij≈ǫLiǫLjv2SMlight left-handed neutrino.The masses and mixing angles are independent of the right-handed symmetry breaking parametersǫNi:m N i≈ǫ2Liv2SMǫLj(i<j).(6)Therefore,besides the unknown scale v Big,only two sets ofǫs are needed:ǫLi andǫEi.In fact,the neutrino masses and mixings depend only onǫLiand they are approximately related throughV ij≈m N j.(7)Equation(7)reduces the number of parameters needed to describe neutrino masses and mixings by three;for example,given two mixing angles and one neutrino mass,we can predict the third mixing angle and the other two neutrino masses.These results are extremely general.They follow simply from the factorization of the Dirac masses, regardless of the specific form of m−1NM,which only contributes to set the scale.For completeness,we note that in the case of Dirac masses only,the neutrinos and the charged leptons mass matrices becomem NDij ≈ǫLiǫNjv SM,(8)m Eij ≈ǫLiǫEjv SM.(9)Their diagonalization yieldsm N i≈ǫLi ǫNiv SM(no sum on i),m E i≈ǫLi ǫEiv SM(no sum on i),(10)whereas the lepton mixing matrix is approximately diagonal with offdiagonal elements of the order ofV ij≈ǫLimay contribute coherently factors of an order of magnitude or so.In addition,the numerical results depend on the specific ansatz.What we seek are the general features of those results rather than the detailed numerical results themselves.I.As ourfirst ansatz we assume thatǫLi =ǫEi[2].This ansatz can be justified asfollows[7].Assume that in the lepton sector the only combination of symmetry which is broken is the axialflavor symmetry.This means that,instead of breaking separately left(L)and right(R)flavor symmetries,only the combination L+R is broken.Therefore we need only one set ofǫs,which are then determined fromǫLi≈ v SM.Theνe−νµmixing found in this way is consistent with the small mixing angle region[8]of the MSW explanation for the Solar Neutrino Problem(SNP).The mixing angles for this and the other ans¨a tze can be found in Table1.We checked that for these mixing angles the third flavor does in fact decouple(see[9]).If this is indeed the solution for that problem,the mass of the muon neutrino must be around3×10−3eV.This then sets the scale for the new physics and the other neutrino masses atv Big≈ǫ2L2v2SMǫ2L2mνµ≈10−5eV,mντ≈ǫ2L3ǫUii=1,2,3;as found by Hall and Weinberg[4].Inspired by an SU(5)type of unification we are led to look at an ansatz in which,ǫLi ∝ǫDi,ǫEi∝ǫQi≈ǫUi.(14)In this ansatz we predict(using the numerical values ofǫQ,ǫU andǫD from[4])the ratios of charged lepton masses to be within factors of three of the measured values. We consider this an interesting result.Further,the mixing angles are consistent with the threeflavour mixing explanations of the SNP[9]for squared masses ofνµandντof order10−4eV2.Therefore,theνµ−ντoscilation explanation of the ANP is unlikely.In addition this mass scale cannot be tested in the laboratory nor provide an explanation for Dark Matter.III.Finally,one might look for inspiration in the breaking of SO(10)into SU(4)⊗SU(2)L⊗SU(2)R.We know that at the renormalization scale of1GeV we have been working at,the SU(2)R symmetry must be badly broken since m t>>m b and m e>>mνe .Further,assuming the ansatz,ǫLi∝ǫQi,ǫEi∝ǫDi,andǫNi∝ǫUiwould lead tom e/mµ≈4.8×10−2,which is wrong by an order of magnitude.Assuming that the SU(4)might still provide some useful information for the SU(2)L singlets we look at the ansatz,ǫEi ∝ǫDi,ǫNi∝ǫUi.(15)In this ansatz we again predict aνe−νµmixing angle consistent with the small angle MSW solution to the SNP.Again,assuming that this is indeed the solution for that problemfixes the see-saw neutrino masses atmνµ≈10−3eV,mνe ≈(ǫL1ǫL2)2mνµ≈0.5eV.(16)We againfind it unlikely that the values obtained can close the Universe or solve the ANP.In conclusion,we extended the concept of approximateflavor symmetries to the lepton sector.In particular we considered the see-saw mechanism as a source of the neutrino masses and showed that the predictions do not depend on the neutrinoflavor symmetry breaking parameters.This yelds a simple relation(cf.eq.(7))between neutrino masses and mixing angles which reduces the number of parameters needed to describe the lepton sector.The lack of information on the neutrino masses and mixing angles led us to propose several ans¨a tze.These exibit the following common features.They are consistent with the MSW solution of the SNP.The ANP is unlikely to be explained throughνµ−ντoscilations and the scale of neutrino masses is too small to close the Universe.AcknowledgementsWe would like to thank Aram Antaramian,Lawrence Hall,Stuart Raby and Lincoln Wolfenstein for useful discussions.We warmly thank the organizers of the TASI93 Summer School in Boulder,Colorado where part of this work has been done.The work of J.P.S.was supported in part by DOE grant#DE-FG02-91ER40682and by the Portuguese JNICT under CIˆENCIA grant#BD/374/90-RM.∗On leave of absence from the Ruder-Boˇs kovi´c Institute,Zagreb,Croatia. References[1]C.D.Froggat and H.B.Nielsen,Nucl.Phys.B147277(1979);Nucl.Phys.B164114(1980).[2]A.Antaramian,L.J.Hall and A.Raˇs in,Phys.Rev.Lett.691871(1992).[3]G.’t Hooft,in Recent Developments in Gauge Theories,Cargese Summer InstituteLectures,1979,edited by G.’t Hooft et al.(Plenum,New York,1980).[4]L.Hall and S.Weinberg,Phys.Rev.D48,979(1993).[5]M.Gell-Mann,P.Ramond and R.Slansky,in Supergravity,edited by P.van Nieuwen-huizen and D.Freedman(North-Holland,Amsterdam,1979);T.Yanagida,in Pro-ceedings of the Workshop on Unified Theories and Baryon Number in the Universe, edited by A.Sawada and A.Sugamoto(KEK,Tsukuba,1979);R.Mohapatra andG.Senjanovi´c,Phys.Rev.Lett.44,912(1980);Phys.Rev.D23,165(1981).[6]S.P.Mikheyev and A.Yu.Smirnov,Nuovo Cimento9C,17(1986);L.Wolfenstein,Phys.Rev.D17,2369(1978).[7]We thank A.Antaramian for explaining this point to us.[8]S.A.Bludman,N.Hata,D.C.Kennedy and ngacker,Phys.Rev.D47,2220(1993).[9]D.Harley,T.K.Kuo and J.Pantaleone,Phys.Rev.D47,4059(1993);of special interest arefigures2and4of D.Harley,T.K.Kuo and J.Pantaleone,‘The Solar Neutrino Problem:Implications of threeflavor mixing’,Indiana University preprint IUHET-246,February1993.[10]IMB collaboration,R.Becker-Szendy et al.,Phys.Rev.Lett.69,1010(1992).[11]E.W.Beier,talk given at the TASI Summer School,Boulder(1993).Table CaptionsTable1:Neutrino mixing angle predictions in the three ans¨a tze introduced.As noted in the text,these results are meant as estimates rather than precise calculations.ansatz sin2(2θeτ)I10−30.20.8 III8×10−6。
项目干系人满意度评估报告
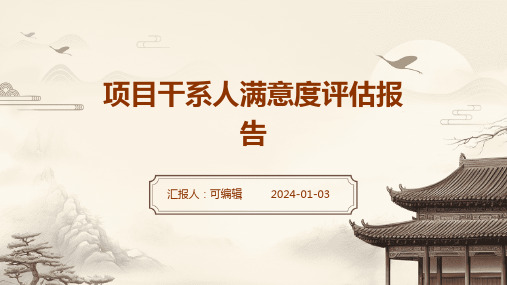
02
在项目管理中,干系人包括项目发起人、项目团队、相关方、
客户等。
干系人的利益关系可能因项目而异,也可能随着项目的进展而
03
发生变化。
干系人分类
内部干系人
与项目团队同一家公司的个人 或组织,如项目发起人、部门
经理等。
外部干系人
与项目团队不同一家公司的个 人或组织,如客户、供应商、 政府机构等。
高级干系人
详细描述
根据评估结果和反馈,我们提出以下改进建议。首先, 应加强对项目成本的控制,优化资源配置,降低不必要 的花费。其次,应提高项目团队内部和与干系人之间的 沟通效率,确保信息传递的准确性和及时性。最后,应 对项目管理流程进行优化,完善规章制度,提高项目执 行效率。通过以上改进措施的实施,有望进一步提升项 目干系人的满意度。
项目干系人满意度评估报 告
汇报人:可编辑
2024-01-03
CATALOGUE
目 录
• 项目干系人概述 • 项目干系人满意度评估方法 • 项目干系人满意度评估指标 • 项目干系人满意度评估结果 • 项目干系人满意度评估案例分析
01
CATALOGUE
项目干系人概述
干系人定义
01
干系人是指与项目有直接或间接利益关系的个人、组织或团体 。
通过干系人识别,有助于更 好地协调利益关系,提高项 目成功率。
02
CATALOGUE
项目干系人满意度评估方法
调查问卷法
总结词
通过设计问卷,收集项目干系人对项目各方面的满意度评价。
详细描述
调查问卷法是一种常用的满意度评估方法,通过设计涵盖项目各方面的问题,向项目干系人发放问卷,收集他们 对项目的满意度评价。该方法具有操作简便、覆盖面广的优点,但需要注意问卷设计的合理性和客观性。
国外学术用户信息查寻行为模型研究综述

国外学术用户信息查寻行为模型研究综述何晓阳【摘要】Papers on information searching behavior models of foreign academic users in the past 10 years were analyzed in aspects of general academic users, establishment of specific subject user model, and modification of present models. The stress was laid on uncertain model, information encountering model, medical scholar information searching behavior model, social scientific scholar information searching behavior mode, historian information searching behavior model, and musician information searching behavior model, and the general characteristics and developmental trend of foreign information searching behavior models were summarized.%采用系统性综述分析方法,从普通学术用户、特定学科用户的模型构建以及现有模型修正3个方面,对国外近十年来学术用户信息查寻行为模型的相关研究文献进行多维分析,重点介绍与评析了不确定性模型、信息偶遇模型以及医学学者、社会科学学者、历史学者及音乐学者4个特定学术群体的信息查寻行为模型,并总结了国外信息查寻行为模型的研究总体特点与发展趋势.【期刊名称】《中华医学图书情报杂志》【年(卷),期】2017(026)003【总页数】6页(P20-25)【关键词】信息行为模型;学术用户;综述【作者】何晓阳【作者单位】第三军医大学图书馆,重庆400038【正文语种】中文【中图分类】G252;G254.9;R-05820世纪90年代前后是信息查寻行为模型研究的一个高峰期。
IT用户满意度调查宏桥科技连中大奖

IT用户满意度调查宏桥科技连中大奖
无
【期刊名称】《大经贸》
【年(卷),期】2003(000)011
【摘要】@@ 近日,在"2003年中国IT用户满意度调查"中,珠海宏桥高科技有限公司的行业解决方案脱颖而出,一举荣获"服装行业解央方案应用满意度第一"和"外贸行业解决方案应用满意度第一"两项大奖.
【总页数】1页(P65)
【作者】无
【作者单位】无
【正文语种】中文
【中图分类】F49
【相关文献】
1.基于模糊综合评判法的图书馆用户满意度调查研究*--以河南科技大学为例 [J], 许景龙;温芳芳;李玲玲;程云;赵珍;宋转芳
2.27家省市科技文献资源网站用户共享满意度调查分析 [J], 白晨
3.神州数码锐行服务:荣获两项大奖,用户满意度再创新高 [J],
4.“全程服务”全面夺魁——方正电脑全面荣获“2001年中国IT产品用户服务满意度调查”服务大奖 [J], 李明富
5.IBM勇夺十项“2008中国IT用户满意度”大奖 [J], 付铮
因版权原因,仅展示原文概要,查看原文内容请购买。
- 1、下载文档前请自行甄别文档内容的完整性,平台不提供额外的编辑、内容补充、找答案等附加服务。
- 2、"仅部分预览"的文档,不可在线预览部分如存在完整性等问题,可反馈申请退款(可完整预览的文档不适用该条件!)。
- 3、如文档侵犯您的权益,请联系客服反馈,我们会尽快为您处理(人工客服工作时间:9:00-18:30)。
User Survey 2011Ogre3D presentsconducted August 2011published November 2011by the Ogre3D team1. IntroductionThis survey was conducted to collect some information about the nature of the Ogre user base and the utilization of the engine. It therefore offers a means to get an impression how Ogre3D is used and which platforms and tools it is most commonly used with. Additionally, this survey serves as a guide for the Ogre3D Team to identify weak points and hence help it to decide on future developments.The survey was conducted throughout the month of August 2011 and was advertised on the Ogre3D homepage () as well as in the Ogre3D forums (/forums) to get as many community members to participate as possible, in order to get a reliable and representative result. In the end we received 1020 responses of which 875 result sets contained answers to all 20 questions and respectively 145 which covered only a proportion of the questions.In order for the results to be comparable to the previous Ogre3D User Survey conducted in 2008, this year’s survey also included those questions from 2008 (questions 1 to 5). The results of the previous survey are also incorporated in this report to simplify comparison, which is easily possible due to a similar number of responses in both surveys (1034 in 2008).We, the Ogre3D Team, would also like to use this report as an opportunity to say “Thank you!”to all the participants of this survey as well as the whole Ogre3D Community in general for their active involvement in the forums and the wiki. After all it is the combination of the skilled and dedicated Ogre3D developers / contributors and the highly motivated and supportive forum members that sum up to the great experience that using and working with the Ogre3D engine is!So “Thank You!”to everyone involved!Your Ogre3D Team2. ResultsQ1: In which sector are you using Ogre?Note: Multiple answers per person were possible.Results 2011:1615127349406529100200300400500600In which sector are you using Ogre?Government Academic / Scientific Commercial (closed source)Commercial (FOSS)Hobby / Student (commercial later)Hobby / Student (non-commercial)Results 2008:5732465235230650100150200250300350400In which sector are you using Ogre?Government Academic / Scientific Commercial (closed source)Commercial (FOSS)Hobby / Student (commercial later)Hobby / Student (non-commercial)Q2: For which application types do you use Ogre? Note: Multiple answers per person were possible.Results 2011:Results 2008:8541412881613948302519316923For which application types do you use Ogre?GamesTraining / EducationSimulationPublic WorksResearchArchitecturalIndustrialembeddedToolsVirtual WorldOther465721553162412110362 21For which application types do you use Ogre?GamesTraining / EducationSimulationPublic WorksResearchArchitecturalIndustrialembeddedToolsVirtual WorldOtherSide-by-side comparison 2011 vs. 2008:85446514172288155 1631 13962 484130 2125193 10316962 232110020030040050060070080090020112008For which application types do you use Ogre?GamesTraining / Education Simulation Public Works Research Architectural Industrial embeddedTools Virtual World OtherOptional input of respondents who flagged “Other”:•Advertising•Augmented Reality (2x) •Civil Engineering •Computer vision •Entertainment•Hardware diagnostics •Media Player (3x) •Mobile•Photo presentation •Prototypes•Real time broadcast graphics •Robotics •Video (2x)•Video post-production •Virtual Reality simulator •Visualization (2x)•Weather presentationQ3: Years of experience with OgreNote: This question was slightly expanded compared to 2008, so a direct comparison is not possible.Results 2011:32726917712050100150200250300350Years of experience with Ogre1 year 1-2 years3-4 years > 4 yearsResults 2008:43630528950100150200250300350400450500Years of experience with Ogre1 year 1-2 years > 2 yearsQ4: Size of team using Ogre Results 2011:5623294217 5100200300400500600Size of team using Ogre12 - 56 - 1011 - 30> 31 Results 2008:490409823617100200300400500600Size of team using Ogre12 - 56 - 1011 - 30> 31Q5: Organization sizeResults 2011:Results 2008:436274652527231950100150200250300350400450500Organizaton size12 - 1011 - 3031 - 5051 - 100101 - 1000> 1001448376992726262750100150200250300350400450500Organizaton size12 - 1011 - 3031 - 5051 - 100101 - 1000> 1001Q6: Which OS are you developing on?Results 2011:29064437515917100200300400500600700Which OS are you developing on?Microsoft Windows: XP or below Microsoft Windows: Vista or above LinuxApple Mac OS XOtherOptional input of respondents who flagged “Other” [excerpt]*:•Android (4x) •FreeBSD (3x) •iOS (4x)•Windows 7 (3x) •Windows CE 6 •…* Only a proportion of the data is listed here, since some participants seem to have misinterpreted the question and answered for which OS they develop rathern than which OS they use to run their IDE. This might also apply to the listed responses.Note: Multiple answers per person were possible.Q7: Which IDE are you using?Results 2011:Optional input of respondents who flagged “Other” [excerpt]*:•Bluesfish (2x) •Codelite (8x) •DevC++ (2x) •Editor (4x) •Emacs (14x) •Geany (7x) •Gedit (5x) •Kate (3x)•MonoDevelop (3x) •Notepad++ (4x) •SciTE (2x) •Vim (25x) •…66413012414244428898100200300400500600700Which IDE are you using?Microsoft Visual Studio Apple Xcode Eclipse Code::Blocks KDevelop NetBeansQtCreator Other* Only a proportion of the data, namely entries that appeared at least two times.Note: Multiple answers per person were possible.Q8: For which platforms are you developing with Ogre?Results 2011:52575523198 11825322302815100200300400500600700800For which platforms are you developing with Ogre?Microsoft Windows: XP or below Microsoft Windows: Vista or aboveApple Mac OS XAndroid powered mobile device Apple iOS Sony Playstation 3Microsoft XBox 360Other kind of Computer Other kind of Mobile deviceOther kind of Games consoleNote: Multiple answers per person were possible.Q9: For which OS are you developing with Ogre?Results 2011:Note: Multiple answers per person were possible.5237564362351059211100200300400500600700800For which OS are you developing with Ogre?Microsoft Windows: XP or belowMicrosoft Windows: Vista or above LinuxApple Mac OS X Apple iOS Android OtherOptional input of respondents who flagged “Other” [excerpt]:•Any OS which can run Java•Attempting Xbox and BlackBerry •FreeBSD (3x) •Meego •…Q10: What configuration of builds for your applications are youusing?Results 2011:Note: Multiple answers per person were possible.3977040350100150200250300350400450What configuration of builds for your applicationsare you using?32-bit only 64-bit only 32 and 64-bitQ11: Preferred shader languageResults 2011:27213118513150100150200250300Preferred shader languageCG HLSLGLSLNone / I do not use shadersQ12: Preferred Render System (if you have a choice)Results 2011:258121371104350100150200250300350400Preferred Render System (if you have a choice)Direct3D 9Direct3D 11OpenGL OpenGL ES 1.x OpenGL ES 2Q13: Main programming language in conjunction with OgreResults 2011:14775597251011100200300400500600700800900Main programming language in conjunction withOgreC C++C#Java PythonRuby objective-C OtherOptional input of respondents who flagged “Other”:•BASIC•Common Lisp •erlang •FreePascal •Javascript •Lua (2x) •PHP•PUREBASIC •Q14: Primary modeling toolResults 2011:43360237116131262921850100150200250300350400450500Primary modeling toolBlender Maya 3D MaxWings3D LightWave TrueSpace Softimage/XSI Google Sketchup Cinema 4D Cheetah 3DDon’t do any modeling myself OtherOptional input of respondents who flagged “Other”:•3DCrafter/3DCanvas •AC3D (2x) •DAZ Studio •DeleD •Houdini•Luxology modo (2x) •Milkshape3D (3x)•My own molecular dynamics C++ code •Not just one•Only few manual meshes •Own tools•Procedural geometry •Silo •ZBrushQ15: Which GUI library do you use the most with Ogre?Results 2011:274144274642391739450100150200250300Which GUI library do you use the most with Ogre?CEGUI MyGUI HikariNavi QuickGUI buttonGUI BetaGUI GorillaNote at all OtherOptional input of respondents who flagged “Other” [excerpt]:•Awesonium (2x) •Berkelium (2x) •Canvas•Custom made / in-house (23x) •Flash •Gtk •MFC •JoyUI•libRocket (7x) •Lugre•Miyagi (7x)•Ogre SDKTrays (7x) •Nifty GUI•Ogre Overlays (2x) •QT (19x) •WPF (2x)•wxWidgets (4x) •…Q16: Which Ogre physics wrapper do you use the most? Results 2011:55623013245193 1934450100150200250Which Ogre physics wrapper do you use the most?OgreNewtNxOgreOgreODEOgreBulletbtOgreUse physics libraries directlyNo physics libraries usedOtherOptional input of respondents who flagged “Other” [excerpt]:•Box2D•BulletSharp•Custom wrapper (18x)•Havok (2x)•Lugre•OgrePhysX•OpCode•PhysX (5x)•PhysX Candy Wrapper (4x)•Rigs of Rods Physics (2x)•…Q17: Which Ogre SceneManagers (SM) do you use? Results 2011:76541 66 36 20100200300400500600700800900Which Ogre SceneManagers (SM) do you use?Which Ogre SceneManagers (SM) do you use?Generic / Octree SMOld Terrain SMPortal Connected Zone SMBSP SMOtherOptional input of respondents who flagged “Other”:•Custom (8x)•ETM•GameKit Occlusion Culling•Ogre::Terrain Component (4x)•PLSM•PLSM2Note: Multiple answers per person were possible.Q18: Most severe drawback(s) of Ogre Q19: Most important/anticipated future change for OgreNote: These were free text questions. As a consequence the result cannot be properly displayed via a chart, but instead the below written summary tries to outline the most common answers.Within the 805 responses we received for these two questions, the following points are the most frequently listed ones that would help improve the overall experience and/or are highly anticipated (in no particular order):•Official console support and official Android port•DirectX 11 and OpenGL3+ support•Updated and more extensive documentation•Enhanced mobile platform support•Good and free 3D Max exporter / better exporters in general•Official tool chain (scene editor, material and shader editor, …)•Modular design with the math part or the resource handling being own sub-libraries•More books, tutorials and other resources to help mastering the steep learning curve•More frequent releases and faster processing of bugs and patches•Better shadow support•Official C-API•Improved performance•Generally improve rapid-prototyping by offering more tools and pre-created content such as complex shader•Official x64 builds•Multithreading / multi core support•Instancing•Inverse Kinematics•Very large scene support / scene manager redesignPS: Rest assured that even though your specific remarks and suggestions for the further, future improvement of Ogre3D might not be in the list above , they will be taken into account. The Ogre3D Team is currently discussing internally how to best address and proceed on the feedback received by this survey.Additionally, there are also multiple ways for you to get active yourself and help develop the engine further, e.g. by participating in the discussions in the “Developer talk” forum section, creating patches to fix issues or add new functionality or help improving the documentation in the wiki.In case of any questions, just send us an eMail and we will get back to you as soon as possible. Details can be found on the last page of this report.Developer talk forum sectionOgre3D wikiBug trackerPatch trackerQ20: Feedback on the Ogre User Survey 2011 Note: These was a free text question. As a consequence the result cannot be properly displayed via a chart, but instead the below written summary tries to outline the most common answers.The 153 responses we received for this question, mainly focused around the following points: •Overall huge appreciation that the community gets a voice• A lot of congratulation and gratitude for the engine and the Ogre3D project in general•Desire to have more questions to get even further insight into the community and the use of Ogre3D and its ecosystem•Plenty of good ideas for new questions on suggestions on how to improve the current ones•Request to conduct that kind of survey on a regular basisThank you for your participation and interest!visit us: mail: webmaster@forum: /forumswiki: /tikiwikiIRC room: irc:///ogre3d© Ogre3D Team2011。