基于人工神经网络的路面模量反算
基于人工神经网络的沥青路面模量评估
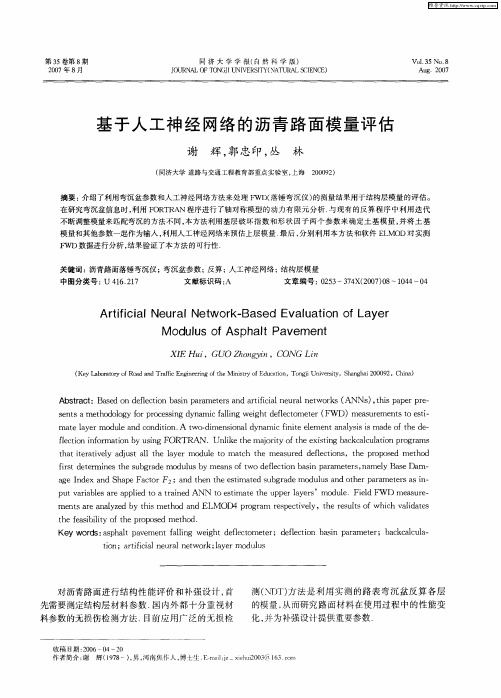
l t ni omai yui OR R f ci fr t nb s gF T AN.U l e h jr y f h xs n ak a uai rga s e o n o n ni e o i e i igb ccl lt npo r k t ma t o t e t c o m ta i rt e du t l tel e d uet th temesrd d f ci s h rl e to h tt ai l ajs l h yrmo l o mac h aue e et n ,tepo e v y a a l o msd meh d
Ar i c a u a t i i lNe r lNet f wor — s d Ev l a i n o y k Ba e a u to fLa er M o u u fAs h t Pa emen d ls o p al v t
XI H u ,GUO l n y n,C E i Z, g i o ONG n Li
维普资讯
第3 5卷第 8期
20 0 7年 8月
同 济 大 学 学 报( 然 科 学 版) 自
J U NA FT N J U V R I Y N T R L S I N E O R L O O G I NI E ST ( A U A C E C )
模量和其他参数一起作 为输入 , 利用人工神经 网络来预估 实测
F WD数据进 行分析 , 结果验证 了本 方法的可行性 . 关键词 : 沥青路面落锤 弯沉仪 ;弯沉盆参数 ; 反算 ; 人工神经网络 ; 结构层 模量
中图 分 类 号 : 4 6 2 7 U 1 .1 文 献标 识 码 : A 文章 编 号 : 2 3 7 X(0 70 ~14 0 5 —34 2 0 ) 8 04—0 4
神经网络算法在公路动态称重中的应用
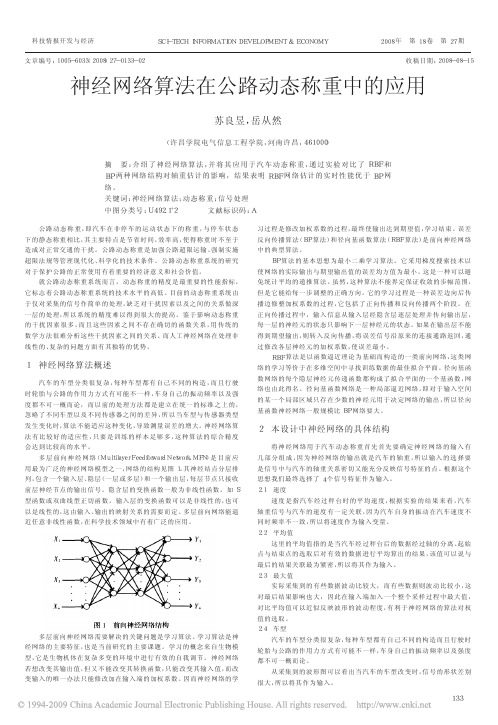
公路动态称重,即汽车在非停车的运动状态下的称重,与停车状态下的静态称重相比,其主要特点是节省时间,效率高,使得称重时不至于造成对正常交通的干扰。
公路动态称重是加强公路超限运输、强制实施超限法规等管理现代化、科学化的技术条件。
公路动态称重系统的研究对于保护公路的正常使用有着重要的经济意义和社会价值。
就公路动态称重系统而言,动态称重的精度是最重要的性能指标,它标志着公路动态称重系统的技术水平的高低。
目前的动态称重系统由于仅对采集的信号作简单的处理,缺乏对干扰因素以及之间的关系做深一层的处理,所以系统的精度难以得到很大的提高。
鉴于影响动态称重的干扰因素很多,而且这些因素之间不存在确切的函数关系,用传统的数学方法很难分析这些干扰因素之间的关系。
而人工神经网络在处理非线性的、复杂的问题方面有其独特的优势。
1神经网络算法概述汽车的车型分类很复杂,每种车型都有自己不同的构造,而且行驶时轮胎与公路的作用力方式有可能不一样,车身自己的振动频率以及强度都不可一概而论,而以前的处理方法都是建立在统一的标准之上的,忽略了不同车型以及不同传感器之间的差异,所以当车型与传感器类型发生变化时,算法不能适应这种变化,导致测量误差的增大。
神经网络算法有比较好的适应性,只要是训练的样本足够多,这种算法的综合精度会达到比较高的水平。
多层前向神经网络(MultilayerFeedforwardNetwork,MFN)是目前应用最为广泛的神经网络模型之一,网络的结构见图1。
其神经结点分层排列,包含一个输入层、隐层(一层或多层)和一个输出层,每层节点只接收前层神经节点的输出信号。
隐含层的变换函数一般为非线性函数,如S型函数或双曲线型正切函数。
输入层的变换函数可以是非线性的,也可以是线性的,这由输入、输出的映射关系的需要而定。
多层前向网络能逼近任意非线性函数,在科学技术领域中有着广泛的应用。
多层前向神经网络需要解决的关键问题是学习算法。
学习算法是神经网络的主要特征,也是当前研究的主要课题。
基于BP神经网络的沥青路面压实度检测
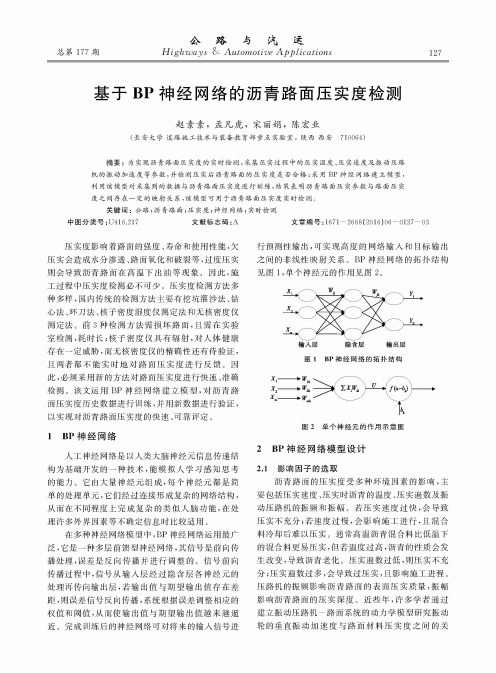
总第177期公路与汽运H ighw ays&A utom otive A pplications127基于BP神经网络的沥青路面压实度检测赵素素,孟凡虎,宋丽娟,陈宏业(长安大学道路施工技术与装备教育部重点实验室,陕西西安 710064)摘要:为实现沥青路面压实度的实时检测,采集压实过程中的压实温度、压实速度及振动压路机的振动加速度等参数,并检测压实后沥青路面的压实度是否合格;采用BP神经网络建立模型,利用该模型对采集到的数据与沥青路面压实度进行训练,结果表明沥青路面压实参数与路面压实度之间存在一定的映射关系,该模型可用于沥青路面压实度实时检测。
关键词:公路;沥青路面;压实度;神经网络;实时检测中图分类号:U416.217 文献标志码:A文章编号:1671 —2668(2016)06 —0127 —03压实度影响着路面的强度、寿命和使用性能,欠压实会造成水分渗透、路面氧化和破裂等,过度压实则会导致沥青路面在高温下出油等现象。
因此,施工过程中压实度检测必不可少。
压实度检测方法多种多样,国内传统的检测方法主要有挖坑灌沙法、钻心法、环刀法、核子密度湿度仪测定法和无核密度仪测定法。
前3种检测方法需损坏路面,且需在实验室检测,耗时长;核子密度仪具有辐射,对人体健康存在一定威胁,而无核密度仪的精确性还有待验证,且两者都不能实时地对路面压实度进行反馈。
因此,必须采用新的方法对路面压实度进行快速、准确检测。
该文运用B P神经网络建立模型,对沥青路面压实度历史数据进行训练,并用新数据进行验证,以实现对沥青路面压实度的快速、可靠评定。
1 B P神经网络人工神经网络是以人类大脑神经元信息传递结构为基础开发的一种技术,能模拟人学习感知思考的能力。
它由大量神经元组成,每个神经元都是简单的处理单元,它们经过连接形成复杂的网络结构,从而在不同程度上完成复杂的类似人脑功能,在处理许多外界因素等不确定信息时比较适用。
在多种神经网络模型中,BP神经网络运用最广泛,它是一种多层前馈型神经网络,其信号是前向传播处理,误差是反向传播并进行调整的。
基于人工神经网络的沥青路面水膜厚度预测

基于人工神经网络的沥青路面水膜厚度预测马耀鲁;耿艳芬;陈先华;卢艳坤【摘要】为了研究多因素影响下沥青路面水膜厚度的变化,结合基于水动力学理论的二维浅水方程,提出一种利用人工神经网络(ANN)预测沥青路面水膜厚度的方法.多因素包括降雨强度、路面宽度、路面横坡、路面纵坡和路面粗糙系数.二维水动力仿真模型经过实测数据验证并根据沈山高速公路工程设计方案仿真得到有限数量的训练数据用于沥青路面水膜厚度的预测,进而分析了多因素对水膜厚度在路面分布的影响.经过18组数据的验证,人工神经网络模型预测精度可达0.991.预测结果表明:水膜厚度从中央分隔带向道路边缘逐渐增加,降雨强度对水膜厚度的变化有明显影响.在路面宽度20 m,降雨强度30 mm/h的条件下,路面内侧车道内的水膜厚度低于10 mm,外侧车道的水膜厚度为20 mm.受训练样本数量的影响,预测结果存在一定的波动,但与现行规范和理论计算值相比,人工神经网络模型能够更好地描述沥青路面水膜的宏观分布特性.%In order to study the variation of the asphalt pavement water film thickness influenced by multi-factors,a new method for predicting water film thickness was developed by the combination of the artificial neural network (ANN) and two-dimensional shallow water equations based on hydrodynamic theory.Multi-factors included the rainfall intensity,pavement width,cross slope,longitudinal slope and pavement roughness coefficient.The two-dimensional hydrodynamic method was validated by a natural rainfall event.Based on the design scheme of Shen-Shan expressway engineering project,the limited training data obtained by the two-dimensional hydrodynamic simulation model was used to predict water film thickness.Furthermore,the distribution ofthe water film thickness influenced by multi-factors on the pavement was analyzed.The accuracy of the ANN model was verified by the 18 sets of data with a precision of 0.991.The simulation results indicate that the water film thickness increases from the median strip to the edge of the pavement.The water film thickness variation is obviously influenced by rainfall intensity.Under the condition that the pavement width is 20 m and the rainfall intensity is 30 mm/h,the water film thickness is below 10 mm in the fast lane and 20 mm in the lateral lane.Although there is fluctuation due to the amount of training data,compared with the calculation on the basis of the existing criterion and theory,the ANN model exhibits a better performance for depicting the macroscopic distribution of the asphalt pavement water film.【期刊名称】《东南大学学报(英文版)》【年(卷),期】2017(033)004【总页数】6页(P490-495)【关键词】路面工程;水膜厚度;人工神经网络;水动力学方法;预测分析【作者】马耀鲁;耿艳芬;陈先华;卢艳坤【作者单位】东南大学交通学院,南京210096;东南大学交通学院,南京210096;东南大学交通学院,南京210096;辽宁省交通规划设计院有限责任公司,沈阳110000【正文语种】中文【中图分类】U416.2An excess of water on the pavement leads to a lower bearing capacity of the pavement structure and reduces pavement life. The existence of water on the pavement may cause hydroplaning because of splash and spray. Little is known clearly so far if there is a well-defined quantitative relationship between the factors and water film variation. The reason is perhaps that the water film variation is difficult to obtain with a high precise based on the limit samples[1]. Most of the previous studies focused on the introduction of the theories or empirical equations, and lacked consideration of pavement width. Most of them simply studied the single hydrodynamic parameter, and the influence of multi-factors such as width, slope, and rainfall intensity is still unclear[2]. Furthermore, traditional theoretical calculation cannot provide a well depiction on pavement water film variation. Therefore, a suitable prediction method for water film on the asphalt pavement is essential.Efficient numeric modeling is a beneficial tool for water film simulation[3]. Compared with one-dimensional models, for pavement surface flow, two-dimensional shallow water equations have the advantage on solving the numerical instability and the backwater phenomenon caused by irregular bottom and curbs[4-5]. It is acceptable to mathematically simulate a variety of free surface flows. However, numerous simulation cases are required to analyze the water film variation. For this reason, the artificial neural network (ANN) is a forecasting tool that can handle the complicated issue efficiently. It does not rely on the subjective factors from multivariate regression analysis[6]. The ANN model has been extensively applied forsolving highly nonlinear function approximations in the fields of asphalt pavement, rigid pavement and composite pavement[7-9]. Fast and accurate prediction results can be achieved by the ANN model based on limited data[10-11].However, most previous studies focused on the pavement water film calculation by empirical equations, and limited work has described the prediction approach for water film distribution on the pavement. In this paper, a prediction model based on the ANN is proposed. The training data obtained by the hydrodynamic method is used to predict the water film thickness. Cases of the pavement with different widths and rainfall intensities are taken as examples to analyze the spatial variation of water film thickness. Pavement submerge risk identification is further explored. This approach is beneficial for predicting pavement water film rapidly once the amount of training data is obtained, which is important for the pavement driving safety.Artificial neural network (ANN) functions as a multilayer feed forward network depending on the error back-propagation algorithm by training data. Unlike a model which addresses the mapping interaction by a mathematical equation, the ANN model is a supervised learning procedure that minimizes the sum of error between the desired and predicted outputs by back-propagation. Mathematically, the ANN model can be treated as a universal approximation. The net structure, activation function, net parameters (weights, thresholds, learning speed and momentum coefficient) and learning error are essential for an ANN model. Thenetwork consists of three layers: input layer, hidden layer and output layer[12]. The data is put into the input layer and the hidden layer, and then the information is transmitted to the output layer on the basis of activation function[13]. The structure of the ANN model is shown in Fig.1. Fig.1 illustrates the structure of an ANN model, in which x and y refer to the input and output; i and j represent the number of neurons in each hidden layer. Mathematically, the ANN basically carries out the training process by combining the input samples as[14]where y is the output result; X1, X2,… represent the input variables; w1,w2, … represent the weighting valu e of each input variable; and e is the bias.The sigmoid function is widely used by a majority of ANN models[15], and it can be represented asIn order to eliminate the error caused by the order of magnitude, the input and output data should be normalized before the training process in the ANN model according to Ref.[16].The normalized equation iswhere Yi is the output data after normalization and training; Yminand Ymax are the minimum and maximum values of the training data after being normalized; Xmin and Xmax are the minimum and maximum values of the training data before being normalized; Xi is the input data before being normalized.To calibrate the simulation model for obtaining the training data, pavement flow depth is monitored through a remote road surface statesensor with infrared light detection based on the practical project of the Shen-Shan expressway (The pavement cross slope is 2% and longitudinal slope is 0.464%). An on-site monitoring program was launched in the year of 2013 and lasted for more than one year (see Fig.2).To analyze the effects of multi-factors on pavement water film thickness, a three-dimensional model is established with the length of 100 m in the X direction, and the width varying from 11 to 25 m (see Fig.3). The influence of pavement aggregate is not considered based on two-dimensional shallow water equations. Simulation is implemented by the following equations:wherewhere h is the water depth on the pavement surface, m; t is the time, s; u and v are the horizontal velocity components in X and Y directions, m/s; Q is the rainfall intensity, mm/h; zb is the bottom elevation, m; g is the acceleration of gravity, m/s2; n is the roughness coefficient of the pavement; S0 is the bottom slope; ζ is the experience coefficient; τb is the bottom shear stress, (kg·m)/s2; Sf is the bottom friction gradient.Fig.4 shows that the simulated value is in agreement with the measured value under a natural rainfall event, and the two curves are identical for the peak value. This means that the two-dimensional shallow water equations are accepted for simulating flow characteristics of the pavement water film. However, many more cases need to be done when the multi-factors are considered. For this reason, the ANN model is proposed for predicting the water film thickness rapidly and effectively once an amount of trainingdata is collected. This capability makes it very powerful for precisely predicting the variation of the water film on the pavement surface. Although the ANN model shows good performance in many situations,Ji[11] showed that the ANN model cannot precisely predict the water film thickness which exceeds the range of training data. Therefore, obtaining representative typical training data as much as possible can improve the prediction accuracy of the ANN model. In this paper, the number of training data is 414 which is sufficiently reliable for expressing the distribution characteristics of the water film thickness. The water film prediction model by the ANN includes one input layer of five neurons, one hidden layer of fifteen neurons and one output layer of one neuron. The input variables are the distance from the median strip, rainfall intensity, cross slope, longitudinal slope, and roughness coefficient. The output variable is the water film thickness (see Tab.1). The sigmoid function is selected as the activation function. Refs.[16-17] illustrated that the number of neurons was from 6 to 15 based on the empirical formula. After the comparison, the suitable neurons’ number in the hidd en layer is 15 with the error of 0.003 58 (see Fig.5) and the scatter plot accuracy of 0.991 (see Fig.6). Then, 18 sets of data are randomly selected to verify the application of the ANN model. The relative error of the predicted values are all below 10% (see Tab.2), which demonstrates that the predictive results have a good correlation with the simulation data.The goal of this application is to predict the water film thickness from the influence of multi-factors which are the distance from median strip, rainfallintensity, cross slope, longitudinal slope, and roughness coefficient[18]. When the selected variable changes between its minimum and its maximum value of initial data, other variables are invariable. It is necessary to add a parameter to the fixed variables’ in order to analyze the variables’ variation in an accepted range[18]. The variation of input variables are determined bywhere Pi is the input data; P is the average value of input data; and a is the accepted range.For the pavement with a width of 20 m, according to the specifications for drainage design of highway (JTGT D33—2012) [19], the calculated value of water film thickness is 11.21 mm under the conditions as shown in Tab.3. From Fig.7, it is found that the trend of variations of the prediction value on the pavement are different from the calculation value. The thickness of the water film increases clearly as the distance from the median strip increases. The maximum value exceeds 20 mm. It can be concluded that the two-dimensional characteristics on the pavement surface cannot be explained clearly by the calculation value. As schematically shown in Fig.8, it can be concluded that the area of lateral lanes (Y=14 to 17 m) has a larger water film thickness, which has a significant impact on the driving vehicles.Tab.3 Calculated value by the specifications for the drainage design of highwayRainfall is a crucial factor for water film variation. As is shown in Tab.4, compared to the minimum value of water film thickness, the maximumvalue of water film thickness of the pavement is obviously influenced by rainfall intensity due to the flow confluence on the pavement. In particular, Fig.9 shows that when the rainfall intensity is 100 mm/h, the water film thickness is greater than 50 mm in the location of 14 to 20 m away from the median strip. So, the lateral lane may suffer a worse situation in a strong rain intensity. On the other hand, the prediction value curve shows a fluctuation under the condition of various rainfall intensities. The fluctuation becomes more obvious as the rainfall intensity becomes stronger. It can be seen from Fig.9 that the pavement water film thickness has an abnormal fluctuation on the location of 8 to 10 m from the median strip because the small thickness is influenced by multi-factors more easily, and this also illustrates that the ANN model has not achieved enough accuracy for small local water depths.Having investigated the impact of water film thickness distribution by the ANN model, we subsequently studied the risk of hydroplaning depending on water film thickness, and this is beneficial to driving safety under bad weather conditions. The pavement area can be classified into a high risk area, medium risk area and low risk area. Fig.10 shows the pavement risk identification by a variation width from 11 to 25 m. Low risk means that the pavement water film thickness is 30% lower than that of the maximum value; high risk means that a pavement water film thickness is 70% higher than that of the maximum value; and others are medium risk. The high risk area exceeds half of the pavement surface when the width of the pavement increases to 25 m. It can definitely be seen that high risk areagradually expands with the increase in the width. This is because more discharge is accumulated easily by a wider pavement. As a result, a wider pavement requires efficient drainage facilities to eliminate the adverse influence caused by the water film.In this study, the distribution of the asphalt pavement water film is systematically predicted by an ANN model. The artificial neural network is an alternative approach for revealing the influence of multi-factors for pavement water film thickness. The approach is capable of predicting the water film distribution on asphalt pavement by using limited data. It is valuable for the design of expressway geometry and drainage. Based on this method, an operational forecasting circumstance can be achieved when using a trained ANN network, which means that the ANN model can depict the distribution of water film thickness on the asphalt pavement. Future work will focus on developing and increasing the capability of this methodology by comprising the geometric line type design of the pavement. Expanding the model to a superelevation transition section and transition curve will allow it to be applied more widely in pavement engineering.【相关文献】[1]Zhang L, Zhang Z. Impact of road slope on water film thickness [J]. Journal of Chongqing Jiaotong University (Natural Science), 2013, 32(3): 404-406. (in Chinese) [2]Zhou Q. Study on the theoretical calculation of the thickness of water film on road surface and its effect on the pavement skid resistance condition [D]. Nanjing: School of Civil Engineering, Nanjing Forestry University, 2013. (in Chinese)[3]Cristina C M, Sansalone J J. Kinematic wave model of urban pavement rainfall-runoff subject to traffic loadings [J]. Journal of Environmental Engineering, 2003,129(7): 629-636. DOI:10.1061/(asce)0733-9372(2003)129:7(629).[4]Wolff A. Simulation of pavement surface runoff using the depth-averaged shallow water equations [D]. Stuttgart: Institute for Transport and Streets, University of Stuttgart. 2013:15-17.[5]Wang Z L, Geng Y F. 2-D shallow water equations with porosity and their numerical scheme on unstructured grids [J]. Water Science and Engineering, 2013, 6(1): 91-105. [6]Li H, Xu J C, Li C Z, et al. The evaluation of intensive land use in the development zone based on BP artificial neural network: A case of Zhejiang province [J]. Areal Research and Development, 2014, 30(4): 122-125. (in Chinese)[7]Ceylan H, Bayrak M B, Gopalakrishnan K. Neural networks applications in pavement engineering: A recent survey [J]. International Journal of Pavement Research and Technology, 2014, 7(6): 434-444.[8]Roberts C A, Attoh-Okine N O. A comparative analysis of two artificial neural networks using pavement performance prediction [J]. Computer-Aided Civil and Infrastructure Engineering, 1998, 13(5): 339-348. DOI:10.1111/0885-9507.00112.[9]Shafabakhsh G A, Talebsafa M, Motamedi M, et al. Analytical evaluation of load movement on flexible pavement and selection of optimum neural network algorithm [J]. KSCE Journal of Civil Engineering, 2015, 19(6):1738-1746.[10]Londhe S N, Shah S, Dixit P R, et al. A coupled numerical and artificial neural network model for improving location specific wave forecast [J]. Applied Ocean Research, 2016, 59:483-491. DOI:10.1016/j.apor.2016.07.004.[11]Ji T J, Huang X M, Liu Q Q, et al. Prediction model of rain water depth on road surface [J]. Journal of Transportation Engineering, 2004, 4(3): 1-3. (in Chinese)[12]Tahershamsi A, Tabatabai M R M, Shirkhani R. An evaluation model of artificial neural network to predict stable width in gravel bed rivers [J]. International Journal of Environmental Science & Technology, 2012, 9(2):333-342. DOI:10.1007/s13762-012-0036-8.[13]Plati C, Georgiou P, Papavasiliou V. Simulating pavement structural condition using artificial neural networks [J]. Structure and Infrastructure Engineering, 2016, 12(9): 1127-1136.[14]Vimala J, Latha G, Venkatesan R. Real time wave forecasting using artificial neural network with vary input parameter [J]. India Journal of Geo-Marine Sciences, 2014, 43(1): 82-87.[15]Gajewski J, Sadowski T. Sensitivity analysis of crack propagation in pavement bituminous layered structures using a hybrid system integrating artificial neural networksand finite element method [J]. Computational Materials Science, 2014, 82(3): 114-117. DOI:10.1016/matsci.2013.09.025.[16]Jiao B, Ye M X. Determination of hidden unit number in a BP neural network [J]. Journal of Shanghai Dianji University, 2013, 16(3): 113-116. (in Chinese)[17]Shen H Y, Wang Z X, Gao C Y, et al. Determining the number of BP neural network hidden layer units [J]. Journal of Tianjin University of Technology, 2008, 24(5): 13-15. (in Chinese)[18]Gevrey M, Dimopoulos I, Lek S. Review and comparison of methods to study the contribution of variables in artificial neural network models [J]. Ecological Modelling, 2003, 160(3): 249-264. DOI:10.1016/s0304-3800(02)00257-0.[19]Liu B Y, Guan Y B, Ding X J, et al. JTGT D33—2012 Specifications for drainage designof highway [S]. Beijing: China Communications Press, 2012. (in Chinese)。
利用BP神经网络反算沥青路面结构层弹性模量的研究
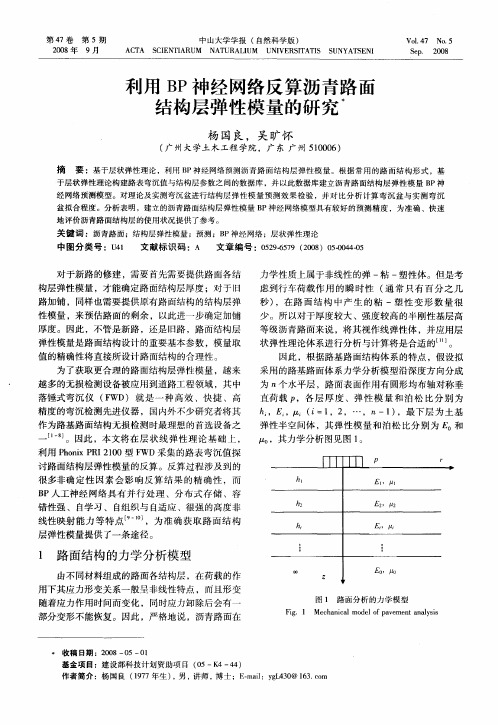
中山大学学报 ( 自然科 学版) A T S IN I R M N T R LU U IE ST TS S N A S N C A CE TA U A U A I M NV R IA I U Y T E I
Vo. No 5 147 . S p. 2 08 e 0
向2 , 2
hl
r
El
, I
线 性映 射 能力 等 特 点
,为 准 确获 取 路 面结 构
h,
El u , l
层 弹性模 量提供 了一 条途径 。
1 路 面结 构 的力 学 分 析模 型
由不 同材料 组成 的路 面各结 构层 ,在荷 载 的作
∞
1
路 加铺 ,同样也 需要 提供原 有路 面结构 的结构 层 弹
力学性 质上 属于 非线性 的 弹 一粘 一塑性 体 。但是 考 虑到行 车荷 载作 用 的 瞬 时 性 ( 常 只 有 百 分 之 几 通
秒) ,在路 面 结 构 中产 生 的 粘 一塑 性 变 形 数 量 很
性 模量 ,来预估 路 面的剩余 ,以此进 一步确定 加铺
h ,E , ( =1 ,… ,/—1 ,最 下 层 为 土基 i ,2 2 )
落锤 式弯 沉 仪 ( WD)就 是一 种 高 效 、快 捷 、高 F 精度 的弯沉 检测 先进 仪器 ,国 内外 不少研 究者 将其
作 为路基路 面结 构无 损检测 时最 理想 的首选设 备之
一
弹性半 空 间体 ,其 弹性 模 量 和 泊松 比分 别 为 和
厚 度 。因此 ,不 管是 新路 ,还是 旧路 ,路面结 构层
少 。所 以对于厚 度较 大 、强度较 高 的半刚性 基层 高 等 级 沥青路 面来 说 ,将 其视 作线 弹性体 ,并 应用层
基于道路工程的神经网络预测模型

基于道路工程的神经网络预测模型摘要:本文将提取出基础设施投资主要风险,分析了外汇风险和主要材料市场风险和工程质量以及进度风险,通过设置所能接受的投资扩大的上限值,当投资出现或即将出现偏离的时候,通过分析其对其资金的有效利用率与资产负债比率和流动资金等与项目运营现状紧密相关的财务指标的影响程度来准确判断其影响程度,对其及时采取措施或者未雨绸缪。
关键词:基础设施投资风险外汇材料风险工程质量及进度投资上限值研究背景2006年以来,中资的大型建筑公司企业开始积极投生海外投资项目,这其中涉及外交政治很多方面,对外项目要实地考察,充分考虑各种因素,比如外国汇率政策,当地拆借市场供需情况,以及贷款融资的金融政策等。
这些需要从整体的金融环境和整个宏观政策来预测与及时采取稳健的措施。
比如选择保险分摊风险,管理和利用好合同处理。
如果签约时仅仅利用概念设计,那么业主就将承受巨大的工程量的风险,如果考虑以后价格浮动和其他不可能预见的重大政治影响对价格的影响,那么就必须预先估计可能的最坏情况,考虑所有情况的最坏情况,考虑政治上的风险,国家经济政策的风向标对价格和进度的最大影响,比如价格上调或下降的最大限,进度最有可能被影响到什么程度,而预测出一个最坏的结果,由此来考虑应对方法,如果工程量存在特大偏差,那么承包方就可能受到很到损失。
凡是有业主原因造成的工程量大幅增加,那么在结算时必然会认这部分工程量,这部分,这要报价得当,那么很可能会盈利,但是现实状况是,如果出现气候异常的务工,二者大多都属于承包商应该估计到的风险,还有政策法规导致的不能开工,市场因素导致的原材料价格大幅上涨。
外汇风险的预测与控制,包括所能接受的最大限度,即阀值与其对总目标的影响权重的确定,因为在工程实践中,不能每一次都以身试法,才从中吸取教训,而且对于整体的把握也难以仅靠经验和预估取得,为此我们将对对外投资的项目外汇风险进行预测,为经营管理者提供决策的理论依据。
人工神经网络在路面模量反分析中的应用
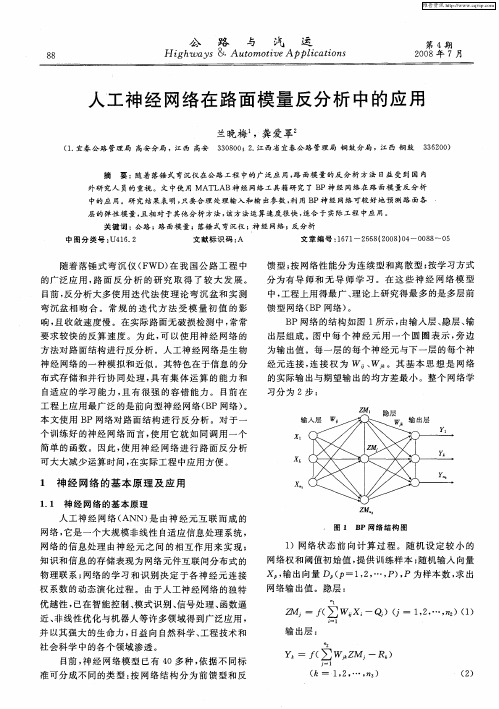
B P网络 的结构 如 图 1 示 , 所 由输 入层 、 隐层 、 输 出层 组成 。图 中每 个 神 经 元 用 一 个 圆 圈表 示 , 边 旁 为输 出值 。每 一层 的每 个 神经元 与下 一层 的每个神
神 经 网络 的一种 模拟 和近似 。其 特色 在于信 息 的分
布 式存储 和并 行 协 同处 理 , 有 集体 运 算 的能 力 和 具 自适 应 的学 习能 力 , 有 很 强 的容 错 能 力 。 目前 在 且 工 程上应 用最 广 泛 的是 前 向型神 经 网络 ( P网络 ) B 。 本 文使 用 B P网络对 路面 结构 进 行 反 分析 。对 于 一 个训 练好 的神 经 网络 而 言 , 用 它 就如 同调 用 一个 使 简单 的 函数 。 因此 , 用 神 经 网络 进行 路 面 反 分析 使 可 大大减 少运 算 时间 , 实 际工程 中应用 方便 。 在
网络 , 它是 一个 大规 模非线 性 自适应 信息 处理 系统 , 网络 的信 息处 理 由神 经元 之 间 的相 互 作用 来 实 现 ; 知识 和信 息 的存储 表现 为 网络 元件互 联 问分布 式的
物理 联 系 ; 网络 的 学 习和 识别 决 定 于各 神 经元 连 接
权 系数 的动 态演 化过 程 。 由于人 工神 经 网络 的独 特
优越性, 已在智 能控 制 、 式识别 、 号处 理 、 模 信 函数 逼 近 、 线性 优化 与机 器人 等许 多领域 得到 广泛应 用 , 非 并 以其 强 大的 生命力 , 日益 向 自然科学 、 工程 技术 和
社 会科 学 中的各 个领域 渗 透 。 目前 , 经 网 络模 型 已有 4 神 O多 种 , 依据 不 同标 准 可 分成 不 同的类 型 : 网 络结 构 分 为前 馈 型 和 反 按
用人工神经网络预测沥青路面材料的回弹模量
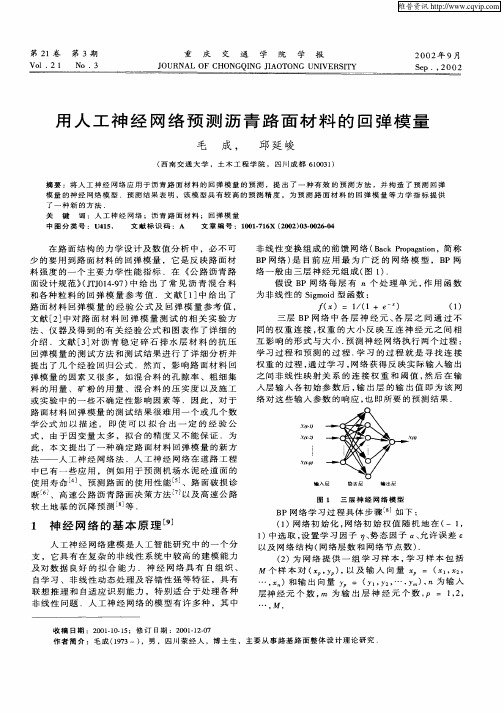
用 人 工 神 经 网 络 预 测 沥 青 路 面 材 料 的 回 弹 模 量
毛 成 , 邱 延 峻
( 南 交 通 大 学 ,土 木 工 程 学 院 ,四 川 成 都 6 0 3 ) 西 10 1 摘 要 :将 人 工 神 经 网 络 应 用 于 沥 青 路 面 材 料 的 回 弹 模 量 的 预 测 ,提 出 了 一 种 有 效 的 预 测 方 法 ,并 构 造 了 预 测 回 弹 模 量 的 神 经 网 络 模 型 .预 测 结 果 表 明 , 该 模 型 具 有 较 高 的 预 测 精 度 , 为 预 测 路 面 材 料 的 回 弹 模 量 等 力 学 指 标 提 供
Xt (O - Xt ( -
!
x - ap )
辅 ^层
隐笛 层
辅 出 坛
图 1 三 层 神 经 网 络 模 型
B P网 络 学 习 过 程 具 体 步 骤 如 下 : ( )网络 初始 化 , 络 初 始 权 值 随 机 地 在 ( 1 1 网 一 , 1 )中选 取 , 置 学 习 因子 孙 势 态 因子 a 允 许 误 差 e 设 、 以及 网 络 结 构 ( 络 层 数 和 网络 节 点 数 ) 网 . ( )为 网 络 提 供 一 组 学 成 的前 馈 网 络 ( ak Po aai ,简 称 B c rpgt n o B P网 络 ) 目前 应 用 最 为 广 泛 的 网 络 模 型 ,B 网 是 P 络 一般 由三 层 神 经 元 组 成 ( 1 . 图 ) 假设 B P网络 每 层 有 /个 处 理 单 元 , 用 函 数 7 , 作 为 非线 性 的 Sg i imod型 函数 :
了一 种新 的方 法 .
关
键
词 : 人 工 神 经 网 络 ; 沥 青 路 面 材 料 ; 回 弹 模 量 文 献 标 识 码 :A 文 章 编 号 :1 0 . 1 X ( o 2 0 .o 6 0 0 17 6 2 o ) 3 o 2 -4
沥青混合料动态模量研究(1)
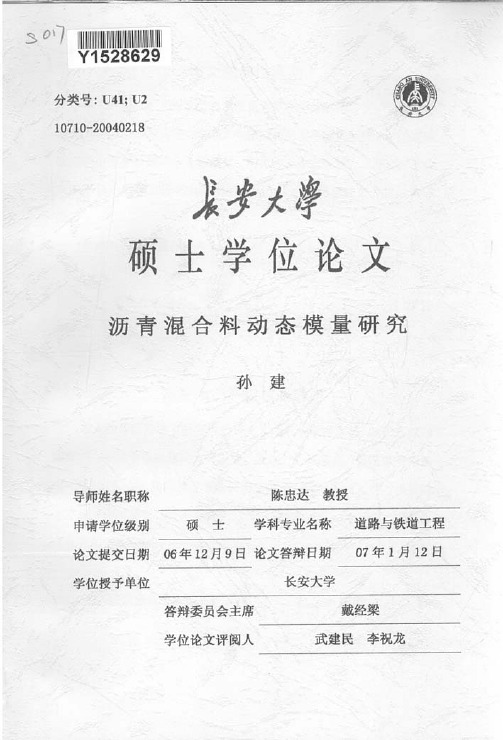
国内外现行的路面设计方法一般是基于静态弹性体系模型,材料设计参数也 是在非完全弹性状态下测定的。而实际车轮荷载为动态荷载,它对路面施以随时 间变化的垂直振动冲击作用以及水平推挤作用,所以路面在实际动态荷载作用下 表现的力学性能与静态模型相比区别较大。且静力分析中忽略了惯性、阻尼和共
玲立乡戈挚
振等动力因素的影响。即路面的实际受力状态与路面设计方法存在着模型与参数 的动态与静态、弹性与粘性的不同。所以,引入动态荷载作用下沥青路面结构的 动态参数和动力特性是十分必要的,这样不但可以对路面动态性能进行评价,而 且可以逐步实现沥青路面设计体系由静态力学体系向动态力学体系的转化。
3 D_
分类号:U41;U2
10710—20040218
⑨
该孝夫海
硕士学位论文
沥青混合料动态模量研究
孙建
导师姓名职称 申请学位级别
硕士
陈忠达教授 学科专业名称 道路与铁道工程
论文提交日期06年12月9日论文答辩日期07年1月12日
学位授予单位
长安大学
答辩委员会主席
戴经粱
学位论文评闻人
武建民李祝龙
每宏乡戈乎
路面设计规程); (3)Several technical manuals developed for use with designs for U.S.Air
Force,Army,and Navy military airfield and highway installations.(军队采用的部分 设计规程)。
load
神经网络与马尔科夫组合预测模型在高速公路沥青路面使用性能中的应用

模型中 T1 ( t) 和 Y2 ( t) 均为确定量, 这里我们根 据预测路段预测值与实际值越逼近越好的原则 , 建立 二次规划模型来确定 w1 和 w2 。 minJ' ( t) =
2
[ Y( t) ∑ t =1
n
-
w j Y j ( t) ] ∑ j =1
2
2
s. t.
wj ∑ j =1
2
= 1, w2 ≥ 0 且 w1 ,
②加权平方和平均组合预测模型 Y( t) = 建立二次规划模型来确定 w1 和 w2 。
n
w 1 Y1 ( t ) 槡
+ w w Y2 t = 1, 2, …, n 2 ( t) ,
minJ' ( t) =
[ Y( t) ∑ t =1
-
w 1 Y1 ( t ) 槡
表1
2001 35. 7 35. 7 1. 03 1. 03 0. 61 0. 61
1
1. 1
模型简介
BP 神经网络 BP 神经网络, 它是一种非线性映射人工神经网
络。BP 神经网络由 3 层组成, 即输入层、 隐含层和 输出层。研究表明, 应用 BP 网络具有较高的预测精 度。为此,本文采用 BP 网络预测路面使用性能, 其 本结构如图 1 所示。 BP 算法把网络的学习过程分为 正向传播和反向传播两种交替过程 ,如果正向传播输 出的误差平方和达到预期的精度 ,则沿误差的负梯度 力方向将修正各层神经元的权值和闭值 , 如此反复, 直至网络全局误差平方和达到预期精度。 BP 神经网 络模型可以通过 MATLAB 中的神经网络工具包实现。
年份 月平均最高温度 T / ℃ 累加值 / ℃ 预测值 / ℃ 年平均降雨量 W × 10 3 / mm 累加值 / mm 预测值 / mm AADT × 10 4 累加值 × 10 4 预测值 × 10 4
基于动态弯沉分析的路面结构模量反算方法研究

基于动态弯沉分析的路面结构模量反算方法研究成晟;倪富健【摘要】落锤式弯沉仪作为一种动态无损检测方法,在世界范围内得到了广泛应用.弯沉盆包含了大量路面结构信息,可据此对结构层模量进行评价.该文利用谱元法路面计算程序,通过逐步回归和多层感知元神经网络2种方法,对路面模量反算模型进行了研究,并提出了基于弯沉盆的动态模量反算方法.对半刚性沥青路面的实测弯沉数据进行反算后,发现由于实测和理论弯沉盆不完全相同,因此基于理论弯沉盆拟合得到的回归公式虽然相关性很好,但在应用于实测数据时却无法得到正确结果;而数值验证显示神经网络方法的反算值稳定性较好.反算数据显示沥青混合料模量受温度影响很大,水泥稳定碎石基层模量与龄期有关.通过弯沉反算得到的材料模量值可以为路面结构设计提供参考.【期刊名称】《现代交通技术》【年(卷),期】2011(008)003【总页数】4页(P4-7)【关键词】道路工程;落锤式弯沉仪;动态模量;反算;逐步回归;多层感知元神经网络【作者】成晟;倪富健【作者单位】泰州市公路管理处,江苏泰州,225300;东南大学交通学院,江苏南京,210096【正文语种】中文【中图分类】U416.01无损检测技术由于具有不损害路面的独特优势,近20年来在国内外得到了迅速发展。
落锤式弯沉仪(FWD)是目前比较流行的路面无损检测设备,它能模拟行车荷载对路面的作用,相比静态检测技术具有一定优势。
目前常用的FWD分析方法都是基于弹性层状体系的静态分析,由于没有考虑荷载的冲击特性,理论模型和实际情况差别较大[1]。
为提高计算精度,更好地模拟现场特征,有必要引入相应的动态分析手段。
工程上常用有限元进行力学计算,但在波动分析中,为了得到精确解,单元的尺寸必须和波长相当,频率越高,波长越短,所需的计算资源也就越多,由于FWD的荷载波动传导频率非常高,故用常规有限元方法进行分析会有较大困难。
Doyle引入了谱元计算方法,他将单元中的波动描述为入射波和反射波的叠加[2];在这一理论指导下,Rizzi研究了2D层状固体中波的传播[3]。
基于PCA-BP原理预测公路最终沉降量

基于PCA-BP原理预测公路最终沉降量□ 甘海龙[摘 要] 路基的最终沉降量是公路耐久性评估的重要参数,影响路基最终沉降量的主要因素有空气中软土层厚度、软土压缩模量、硬层厚度、硬层压缩模量、路堤宽高比、路基施工工期、竣工时沉降量。
基于以上7个参数,结合BP神经网络较好的预测性,以及主成分分析(PCA)能消除自变量间的多重共线性和降低输入数据维度的特点,建立了基于PCA-BP神经网络的路基最终沉降量预测模型。
以30组实测数据为例,对7个影响因素进行主成分分析,最终降为4个主成分,进而将其作为BP神经网络的输入因子,对路基的最终沉降量进行了预测。
结果表明:PCA-BP神经网络预测误差低,实现了对路基的最终沉降量的较准确预测,PCA-BP神经网络模型为路基的最终沉降量预测提供了一种科学、可靠的方法。
[关键词] 路基的最终沉降量;主成分分析法;BP神经网络;预测模型1 引言近年来,随着我国城镇化建设步伐的加快,各地城市中高等级道路在设计与施工方面取得了很大的进步。
作为道路主体的路基,对其综合稳定技术的研究也取得了新的进展。
道路路基综合稳定性及其强度是路面工程质量的重要保证。
其施工过程中如果施工方法不当或者用料不合理,对路基的稳定性均会产生不良的影响,导致路基在使用过程中极易出现路基沉陷、边坡滑塌等问题。
因此道路路基最终沉降量的研究对今后道路的养护维修以及交通的正常运行具有重要意义。
此外,研究如何科学计算路基的最终沉降量,具有良好的社会效益和极大的经济、技术价值。
2 最终沉降量的计算方法目前预测路基最终沉降量的方法主要有经验公式法、Asaoka法、遗传算法、灰色系统法和神经网络预测法[1]。
孙常青[2]等提出一种双曲线零点平移拟合法来确定最终沉降量;李凡[3]等建议在采用传统的双曲线法推算软土地基最终沉降量的同时,可运用 Asaoka推算法进行校核;朱胜利[4]采用实测沉降曲线推算地基最终沉降量的新型双曲线型表达式;李凡[5]等提出利用小波神经网络预测高速公路软土地基的最终沉降量的方法。
利用FWD数据反算模量方法研究

图表法 和公式 回归法 :通过研究各种弯沉盆 参 数 (B ,df co ai prm t s 路 面 各 D P eetn bs a e r 与 l i n a e) 层模量 或 应力 应变 关 系 ,得 出 回归公 式 或诺 模 图 ,这种 方法快速 ,简便 ,利 于推广使用 。并且 通 过表 达式 ,可 以较 为清 晰地研 究 弯沉 盘 的特 性 ,研究各个 因素堆反算模量 的影响状况 。这种 方 法 的研 究 结 果 比较 丰 硕 。 迭代法 :即通过 一组初始模量值 ,基于理论 分 析模型计 算理论 弯沉盆 ,并 与 F WD实 测弯沉
中 图 分 类 号 : 4 60 文 献 标 识 码 : 文 章 编 号 :0 9 7 1 (0 7) 1 O9 — 3 U 1 .l A 10 — 7 6 20 0 一 O 4 0
0 引 言
落锤 式弯沉仪 F WD是 国 内正在 逐步 发展 的 种较先进 的无破损弯沉检测设备 ,所 测路 面弯 沉数据可用于评估路面结构状 况 ,其 中反算路 面 各结构层模量是最广泛 、重要的用 途之一。利用 F WD实测弯 沉反算 路 面结构 层模量 ,其 有效性 主要取决 于路面结构模型 、测试数 据 、反算方法 和结果修正等 因素 。本文主要介绍 常用 的一些模 量 反 算 的方 法 ,并 就 各 种 反算 方法 的 可靠 性 进 行 初步探讨 。 国外对 F WD数据 反算 各个结 构层 的模量 开 展 了大量 的研究工作 。国 内在这 方面起步较 晚 。 国内外对 于模量反算采用 的路面结构模型主要有 线性 和非线性两种 ,模量反算 方法主要有 图表法 和回归公式法 、迭代法 、数据库搜索法 、遗传算 法 和人工神经 网络法等五大类 。
维普资讯
9 4
使用人工神经网络来确定和应用权重对滑坡进行敏感性填图
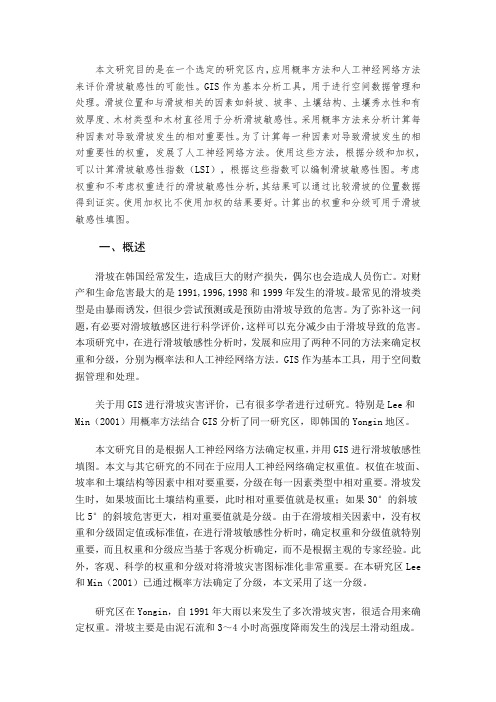
本文研究目的是在一个选定的研究区内,应用概率方法和人工神经网络方法来评价滑坡敏感性的可能性。
GIS作为基本分析工具,用于进行空间数据管理和处理。
滑坡位置和与滑坡相关的因素如斜坡、坡率、土壤结构、土壤秀水性和有效厚度、木材类型和木材直径用于分析滑坡敏感性。
采用概率方法来分析计算每种因素对导致滑坡发生的相对重要性。
为了计算每一种因素对导致滑坡发生的相对重要性的权重,发展了人工神经网络方法。
使用这些方法,根据分级和加权,可以计算滑坡敏感性指数(LSI),根据这些指数可以编制滑坡敏感性图。
考虑权重和不考虑权重进行的滑坡敏感性分析,其结果可以通过比较滑坡的位置数据得到证实。
使用加权比不使用加权的结果要好。
计算出的权重和分级可用于滑坡敏感性填图。
一、概述滑坡在韩国经常发生,造成巨大的财产损失,偶尔也会造成人员伤亡。
对财产和生命危害最大的是1991,1996,1998和1999年发生的滑坡。
最常见的滑坡类型是由暴雨诱发,但很少尝试预测或是预防由滑坡导致的危害。
为了弥补这一问题,有必要对滑坡敏感区进行科学评价,这样可以充分减少由于滑坡导致的危害。
本项研究中,在进行滑坡敏感性分析时,发展和应用了两种不同的方法来确定权重和分级,分别为概率法和人工神经网络方法。
GIS作为基本工具,用于空间数据管理和处理。
关于用GIS进行滑坡灾害评价,已有很多学者进行过研究。
特别是Lee和Min(2001)用概率方法结合GIS分析了同一研究区,即韩国的Yongin地区。
本文研究目的是根据人工神经网络方法确定权重,并用GIS进行滑坡敏感性填图。
本文与其它研究的不同在于应用人工神经网络确定权重值。
权值在坡面、坡率和土壤结构等因素中相对要重要,分级在每一因素类型中相对重要。
滑坡发生时,如果坡面比土壤结构重要,此时相对重要值就是权重;如果30°的斜坡比5°的斜坡危害更大,相对重要值就是分级。
由于在滑坡相关因素中,没有权重和分级固定值或标准值,在进行滑坡敏感性分析时,确定权重和分级值就特别重要,而且权重和分级应当基于客观分析确定,而不是根据主观的专家经验。
基于自适应信息遗传算法的路面模量反算研究
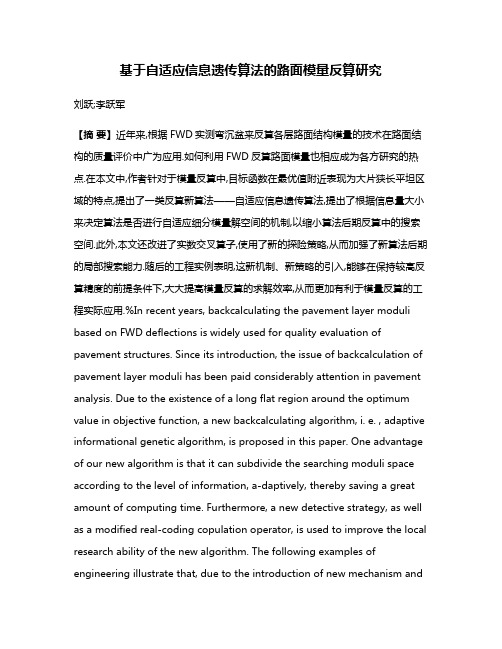
基于自适应信息遗传算法的路面模量反算研究刘跃;李跃军【摘要】近年来,根据FWD实测弯沉盆来反算各层路面结构模量的技术在路面结构的质量评价中广为应用.如何利用FWD反算路面模量也相应成为各方研究的热点.在本文中,作者针对于模量反算中,目标函数在最优值附近表现为大片狭长平坦区域的特点,提出了一类反算新算法——自适应信息遗传算法,提出了根据信息量大小来决定算法是否进行自适应细分模量解空间的机制,以缩小算法后期反算中的搜索空间.此外,本文还改进了实数交叉算子,使用了新的探险策略,从而加强了新算法后期的局部搜索能力.随后的工程实例表明,这新机制、新策略的引入,能够在保持较高反算精度的前提条件下,大大提高模量反算的求解效率,从而更加有利于模量反算的工程实际应用.%In recent years, backcalculating the pavement layer moduli based on FWD deflections is widely used for quality evaluation of pavement structures. Since its introduction, the issue of backcalculation of pavement layer moduli has been paid considerably attention in pavement analysis. Due to the existence of a long flat region around the optimum value in objective function, a new backcalculating algorithm, i. e. , adaptive informational genetic algorithm, is proposed in this paper. One advantage of our new algorithm is that it can subdivide the searching moduli space according to the level of information, a-daptively, thereby saving a great amount of computing time. Furthermore, a new detective strategy, as well as a modified real-coding copulation operator, is used to improve the local research ability of the new algorithm. The following examples of engineering illustrate that, due to the introduction of new mechanism andstrategy, the new algorithm improve the backcalculating efficiency greatly for given precision of results. This means that the new algorithm can better meet the requirements for further engineering application.【期刊名称】《公路工程》【年(卷),期】2012(037)003【总页数】9页(P136-143,172)【关键词】道路工程;路面模量反算;自适应信息遗传算法;路面结构;落锤式弯沉仪(FWD);实数交叉算子【作者】刘跃;李跃军【作者单位】湖南省凤大高速公路建设开发有限公司,湖南凤凰 416800;湖南省交通科学研究院,湖南长沙 410015【正文语种】中文【中图分类】U416.230 引言根据实测弯沉盆来反算各层路面结构模量的技术在路面结构的质量评价中广为应用。
模量反算

摘 要<通过总结国内外路面结构层模量反算方法的研究概 况E分析 了图表 法和回 归公式 法G迭代
法G数据库搜索法G遗传算法和人工神经网络法五类方法的优缺 点E提出 了模量 反算 需进一 步研究
解 决 的 初 始 值 和 局 部 收 敛 G解 的 唯 一 性 和 反 算 结 果 的 合 理 性 三 个 关 键 问 题 H
关 键 词 <模 量 反 算 I落 锤 式 弯 沉 仪 @JK :BI最 优 化 I弯 沉 盆
中 图 分 类 号 <L"$?8#$
文 献 标 识 码 <M
NOPPQRSTUVQWXWQYWOYQZ[T\P]Z^T_‘TUaQb]P]\ZYQS]RPT_OY[
cde fg=hijk
@:62*(3l6)3&,m&*0*)0-(*,,./4)5.)66(.)5Eno*)51o*n&ll’)./*3.&)1L).p6(1.3qEno*)51o*"$##D?Eno.)*B
面 结 构 性 能E根 据 JK:实 测 弯 沉 盆 反 算 路 面 结 构 层的模量也就成为路面工程的研究热点之一H
实 际 上E路 面 模 量 反 算 是 一 个 非 常 复 杂 而 困 难 的问题E不管是 采 用 线 性 或 非 线 性 还 是 考 虑 静 载 或 动载等 力学 分析 模 型 计 算 路 面 结 构 的 弯 沉E模 量 反 算最 终都可 归结 为 非 线 性 最 优 化 问 题E即 如 何 采 用 有效的最优化算法和数据处理方法寻找最优的路面
rV‘ZRQWZ<-o6(616*(/o61&) s*/t/*+/’+*3.&) l63o&01&,2*p6l6)3+*q6(l&0’+..) no.)**)0 &3o6(/&’)3(.61u6(6(6p.6u60;-o6*0p*)3*561*)00.1*0p*)3*561&,>t.)01&,s*/t/*+/’+*3.&) l63o&01E )&l&5(*2o.61 *)0 (65(611.p6 6v’*3.&)1E.36(*3.p6 l63o&01E 0*3*s*16 16*(/o.)5 36/o).v’61E56)63./*+5&(.3ol1*)0*(3.,./.*+)6’(*+)63u&(t1Eu6(60.1/’1160;w3.12&.)360&’3 3o*3 .).3.*+ p*+’6 *)0 +&/*+ /&)p6(56)/qE ’).v’6)611 &, 1&+’3.&)E *)0 (6*1&)*s+6)611 &, s*/t/*+/’+*3.&)(61’+31*(63o6Ft6q.11’61;$3*sE$#(6,1; x]S yTR_‘< s*/t/*;0’+.I,*++.)5 u6.5o3 06,+6/3&l636(@JK:BI &23.l.z*3.&)I 06,+6/3.&)s*1.) rOZ^TR R]‘OP]<{|M }’=0&)5@$CD#=BEl*+6E~o:E*) *11&/.*36 2(&,611&( &, no*)51o* n&ll’)./*3.&)1L).p6(1.3qE6)5*560.)(616*(/o&,(&*0*)0(*.+u*q6)5.)66(.)5;
- 1、下载文档前请自行甄别文档内容的完整性,平台不提供额外的编辑、内容补充、找答案等附加服务。
- 2、"仅部分预览"的文档,不可在线预览部分如存在完整性等问题,可反馈申请退款(可完整预览的文档不适用该条件!)。
- 3、如文档侵犯您的权益,请联系客服反馈,我们会尽快为您处理(人工客服工作时间:9:00-18:30)。
JI I S FW D c o d n o t e a c r ig t h mo e f -a e e B e r l ewo k n h h o y o ea t d l 3 ly r d P n u a n t r a d t e t e 据 三 层 B 神 经 网 络 模 型 和 弹 性 层 状 体 系理 论 , 合 JI W D 研 究 了层 状 体 系路 面 的 根 P 结 I sF
模 量反 算 。通过 理 论和 实测 弯沉 盆的反 算 , 比较 了精 确 网络 与噪 音 网络 的反 算 能 力 , 而提 出 了人 从 工神 经 网络 实现 模 量反 算 的 关键技 术 。 音 网络 与 国 内外常 用反 算 程序 的 比较 结 果表 明 , 经网络 噪 神 法 的反 算结 果具 有 良好 的精 度 和 可靠性 。 关键 词 : 面 ; 量反 算 ; 工神 经 网络 ; 路 模 人 弯沉 盆 ; 落锤 式 弯沉 仪
l y r d ys e .T he a e e s t m ba kc l u a i g a lt a c at n t o k an n s n t o k i o p r d c a c l tn biiy of c ur e e w r d oie e w r s c m a e t o h t e ba kc c a i n oft e r tc la e s r d d fe to sns hr ug h c alul to h o e ia nd m a u e e lc i n ba i .T he ke e hni e r y tc qu sa e pr s nt d o h ck a c l to odu iw ih a tfca n ur lne wor . he c e e e f r t e ba c l u a i n Ofm l t r iiil e a t ks T om p rs o a ii ns Of t oie n t o k t he c m m o c c l u a i n pr gr m ss w ha he ba kc l ul ton r s t he n s e w r o t o n ba k a c l to o a ho t tt c a c a i e uls f r t e m e h fne a e w o ks a e g od i c ur c nd r la lt o h t od o ur ln t r r o n a c a y a e i biiy. 3 t bs, fg, e s a 1 i 6 r f K e o ds:pa e e ; c c l u ato odu i a tfca u aln t o k; fe to a i f li yw r v m nt ba k a c l i n ofm l ; r iii lne r e w r de lc i n b s n;a lng w e g fe t e e i htde l c om t r A u h r s m e:ZH A Xu— on (1 7 一 t or e u d g 0 ), m a e,Ph. ., a a s c a e p of s or Of C h ng ha 9 l D n s o i t r e s a s Co m unia i n v r iy, ng ge n r s a c a e e ng ne r n m c ton U i e s t e a d i e e r h ofp v m nte i e i g.
Z t u do 。 A N G n ga g t4 X ng Bi g— n
( Depa t e i 1. r m ntofH ghway an a fc d Tr fi En ne rng.Cha gi e i ngs a Com m u c i s U n v r iy. h niaton i e st Cha ngs a 41 76.C h na; 2 Sc olofH i h 00 i . ho ghwa y.Chan n U ni r t ga ve siy.X ia 71 64.C h na) n 00 i
A b t a t T he ba k a c l i o v m e l e o sr c : c c l u aton f pa e nt ay r m dul or a e e s s e i e e r he w ih i f l y r d y t m s r s a c d t
中 图分 类号 : 1. 1 U4 6 0 文 献标 识 码 : A
Ba k a c a i n o v m e a e o ul b s d o c c l ul to f pa e ntl y r m d i a e n a tf c a ur ln t r i i i lne a e wor s k
V o1 N O. .2 2
Jn 0 2 u e2 0
文 章 编 号 : 6 ll 3 ( 0 2 0 — 0 2 O l7 6 7 2 0 )2 0 l 一4
基 于 人 工 神 经 网 络 的 路 面 模 量 反 算
查 旭 东 , 秉 纲。 王
( .长 沙 交通 学 院 道 路 与 交 通 工 程 系 , 南 长 沙 . 1 0 6 .长 安 大学 公 路 学 院 . 西 西 安 7 0 6 ) 1 湖 4 7 ;2 0 陕 1 0 4
维普资讯
第 2卷 第 2期
20 0 2年 6月
交 通 运 输 工 程 学 报 J u n l f af n a s o t to g n e ig o r a O fi a d Tr n p r a in En i e rn Tr c