The Nei’s Standard Genetic Distance in Artificial Evolution
新视野大学英语第四册第七单元完型填空翻译
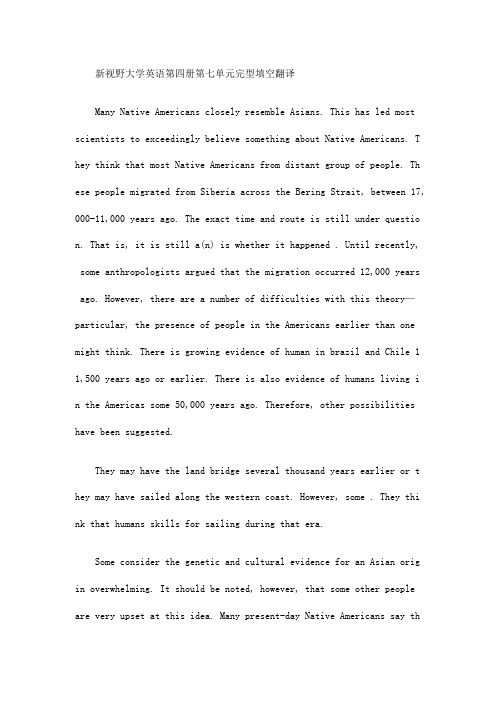
新视野大学英语第四册第七单元完型填空翻译Many Native Americans closely resemble Asians. This has led most scientists to exceedingly believe something about Native Americans. T hey think that most Native Americans from distant group of people. Th ese people migrated from Siberia across the Bering Strait, between 17, 000-11,000 years ago. The exact time and route is still under questio n. That is, it is still a(n) is whether it happened . Until recently, some anthropologists argued that the migration occurred 12,000 years ago. However, there are a number of difficulties with this theory—particular, the presence of people in the Americans earlier than one might think. There is growing evidence of human in brazil and Chile 1 1,500 years ago or earlier. There is also evidence of humans living i n the Americas some 50,000 years ago. Therefore, other possibilities have been suggested.They may have the land bridge several thousand years earlier or t hey may have sailed along the western coast. However, some . They thi nk that humans skills for sailing during that era.Some consider the genetic and cultural evidence for an Asian orig in overwhelming. It should be noted, however, that some other people are very upset at this idea. Many present-day Native Americans say those who put forward such theories have political motivation. They have their own traditional stories that offer of where they came from. T heir own stories claim that their are different from what scientists say. Those accounts, though, have mostly been by scholars. Therefore, the origin of Americans still remains a mystery to be explored.许多印第安人酷似亚洲人。
nature原文
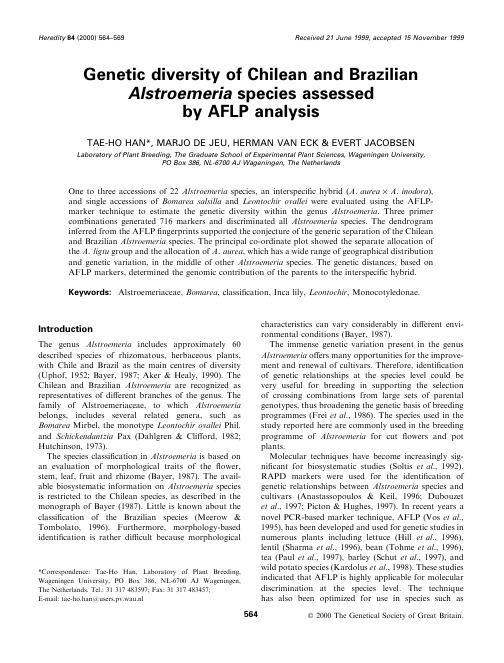
Genetic diversity of Chilean and Brazilian Alstroemeria species assessedby AFLP analysisTAE-HO HAN*,MARJO DE JEU,HERMAN VAN ECK&EVERT JACOBSEN Laboratory of Plant Breeding,The Graduate School of Experimental Plant Sciences,Wageningen University,PO Box386,NL-6700AJ Wageningen,The NetherlandsOne to three accessions of22Alstroemeria species,an interspeci®c hybrid(A.aurea´A.inodora), and single accessions of Bomarea salsilla and Leontochir ovallei were evaluated using the AFLP-marker technique to estimate the genetic diversity within the genus Alstroemeria.Three primer combinations generated716markers and discriminated all Alstroemeria species.The dendrogram inferred from the AFLP®ngerprints supported the conjecture of the generic separation of the Chilean and Brazilian Alstroemeria species.The principal co-ordinate plot showed the separate allocation of the A.ligtu group and the allocation of A.aurea,which has a wide range of geographical distribution and genetic variation,in the middle of other Alstroemeria species.The genetic distances,based on AFLP markers,determined the genomic contribution of the parents to the interspeci®c hybrid.Keywords:Alstroemeriaceae,Bomarea,classi®cation,Inca lily,Leontochir,Monocotyledonae.IntroductionThe genus Alstroemeria includes approximately60 described species of rhizomatous,herbaceous plants, with Chile and Brazil as the main centres of diversity (Uphof,1952;Bayer,1987;Aker&Healy,1990).The Chilean and Brazilian Alstroemeria are recognized as representatives of di erent branches of the genus.The family of Alstroemeriaceae,to which Alstroemeria belongs,includes several related genera,such as Bomarea Mirbel,the monotype Leontochir ovallei Phil. and Schickendantzia Pax(Dahlgren&Cli ord,1982; Hutchinson,1973).The species classi®cation in Alstroemeria is based on an evaluation of morphological traits of the¯ower, stem,leaf,fruit and rhizome(Bayer,1987).The avail-able biosystematic information on Alstroemeria species is restricted to the Chilean species,as described in the monograph of Bayer(1987).Little is known about the classi®cation of the Brazilian species(Meerow& Tombolato,1996).Furthermore,morphology-based identi®cation is rather di cult because morphological characteristics can vary considerably in di erent envi-ronmental conditions(Bayer,1987).The immense genetic variation present in the genus Alstroemeria o ers many opportunities for the improve-ment and renewal of cultivars.Therefore,identi®cation of genetic relationships at the species level could be very useful for breeding in supporting the selection of crossing combinations from large sets of parental genotypes,thus broadening the genetic basis of breeding programmes(Frei et al.,1986).The species used in the study reported here are commonly used in the breeding programme of Alstroemeria for cut¯owers and pot plants.Molecular techniques have become increasingly sig-ni®cant for biosystematic studies(Soltis et al.,1992). RAPD markers were used for the identi®cation of genetic relationships between Alstroemeria species and cultivars(Anastassopoulos&Keil,1996;Dubouzet et al.,1997;Picton&Hughes,1997).In recent years a novel PCR-based marker technique,AFLP(Vos et al., 1995),has been developed and used for genetic studies in numerous plants including lettuce(Hill et al.,1996), lentil(Sharma et al.,1996),bean(Tohme et al.,1996), tea(Paul et al.,1997),barley(Schut et al.,1997),and wild potato species(Kardolus et al.,1998).These studies indicated that AFLP is highly applicable for molecular discrimination at the species level.The technique has also been optimized for use in species such as*Correspondence:Tae-Ho Han,Laboratory of Plant Breeding,Wageningen University,PO Box386,NL-6700AJ Wageningen,The Netherlands.Tel.:31317483597;Fax:31317483457;E-mail:tae-ho.han@users.pv.wau.nlHeredity84(2000)564±569Received21June1999,accepted15November1999564Ó2000The Genetical Society of Great Britain.Alstroemeria spp.,which are characterized by a large genome size(2C-value:37±79pg)(Han et al.,1999). In this study,we produced AFLP®ngerprints of 22Alstroemeria species,one interspeci®c hybrid (A.aurea´A.inodora)and the distantly related species Bomarea salsilla and Leontochir ovallei,and we analysed their genetic relationships.The interspeci®c hybrid was included in our study in order to investigate the possibility of identifying the parental genotypes. Materials and methodsPlant materialSeeds and plants of22Alstroemeria species were obtained from botanical gardens and commercial breeders.The collection has been maintained for many years in the greenhouse of Unifarm at the Wageningen Agricultural University.When available,three acces-sions were selected for each Alstroemeria species,and both B.salsilla and L.ovallei were chosen as outgroups. One interspeci®c hybrid(A.aurea´A.inodora)was obtained from earlier research(Buitendijk et al.,1995) (Table1).All accessions were identi®ed according to their morphological traits(Uphof,1952;Bayer,1987).AFLP protocolGenomic DNA was isolated from young leaves of greenhouse-grown plants using the cetyltrimethy-lammonium bromide(CTAB)method according to Rogers&Bendich(1988).The AFLP technique followed the method of Vos et al.(1995)with modi®-cations of selective bases of pre-and®nal ampli®cationsTable1Accessions and origin of Alstroemeria species for AFLP analysisCode Plant material Accession Distribution/altitudeàChilean speciesC1 A.andina Phil.IX-2Chile26°±31°S.L.,2900±3700m(1)C2 A.angustifolia Herb.ssp.angustifolia AN1S,AN2S,AN7K Chile,33°S.L.,<1000m(1)C3 A.aurea Grah.A001,A002,A003Chile,36°±42°/47°S.L.,200±1800m(1) C4 A.diluta Bayer AD2W,AD4K,AD5K Chile,29°±31°S.L.,0±100m(1)C5 A.exserens Meyen AO2S,AO5S,AO7Z Chile,34°±36°S.L.,1500±2100m(1)C6 A.garaventae Bayer AH6Z,AH8K Chile,33°S.L.,2000m(1)C7 A.gayana Phil.XIII-2Chile29°±32°S.L.,0±200m(1)C8 A.haemantha Ruiz and Pav.J091±1.J091±4Chile,33°±35°S.L.,0±1800m(1)C9 A.hookeri Lodd.ssp.c umminghiana AQ5S,AQ6Z,AQ7Z Chile,32°±34°S.L.,0±500m(1)C10 A.hookeri Lodd.ssp.hookeri AP2S,AP3S,AP8K Chile,35°±37°S.L.,0±300m(1)C11 A.ligtu L.ssp.incarnata AJ7S,AJ12K Chile,35°S.L.,1100±1400m(1)C12 A.ligtu L.ssp.ligtu AL4S,AL6K,AL11K Chile,33°±38°S.L.,0±800m(1)C13 A.ligtu L.ssp.s imsii AM6K,AM7K,K101±1Chile,33°±35°S.L.,0±1800m(1)C14 A.magni®ca Herb.ssp.magni®ca Q001±4,Q001±5,Q007Chile,29°±32°S.L.,0±200m(1)C15 A.modesta Phil.AK2W,AK3W Chile29°±31°S.L.,200±1500m(1)C16 A.pallida Grah.AG4Z,AG7K,AG8K Chile33°±34°S.L.,1500±2800m(1)C17A.pelegrina L.AR4S,C057±1,C100±1Chile,32°±33°S.L.,0±50m(1)C18 A.pulchra Sims.ssp.pulchra AB3W,AB7S,AB8S Chile,32°±34°S.L.,0±1000m(1)C19 A.umbellata Meyen AU2Z Chile,33°±34°S.L.,2000±3000m(1) Brazilian speciesB1 A.brasiliensis Sprengel BA1K,BA2K,R001±1,Central Brazil(2)R001±2B2A.inodora Herb.P002,P004±6,P008±3Central and Southern Brazil(2)B3 A.pstittacina(D)Lehm.D031,D032,D92±02±1Northern Brazil(2)B4 A.pstittacina(Z)Lehm.93Z390±2,93Z390±4,Northern Brazil(2)96Z390±6O1Bomarea salsilla Mirbel.M121Central and Southern South America(3) O2Leontochir ovallei Phil.U001Central Chile(4)Interspeci®c hybridF1A1P2±2(A001´P002)-2Buitendijk et al.(1995)Codes from accessions of species maintained at the Laboratory of Plant Breeding,Wageningen University and Research centre.àLiterature source:(1)Bayer,1987;(2)Aker&Healy,1990;(3)Hutchinson1959;(4)Wilkin(1997).EVALUATION OF THE CHILEAN AND BRAZILIAN ALSTROEMERIA SPP.565ÓThe Genetical Society of Great Britain,Heredity,84,564±569.(Han et al.,1999).To assess interspeci®c variation, autoradiograms comprising the AFLP®ngerprints of a mixture of three accessions per species were analysed by pooling5l L of the®nal selective ampli®cation products according to Mhameed et al.(1997).The low level of variation between individual samples showed that pool-ing accessions was justi®ed.Three primer combinations (E+ACCA/M+CATG,E+ACCT/M+CATC and E+AGCC/M+CACC)were selected from a test of96primer combinations,and these produced272, 211and233bands,respectively(Table2).The choice of the primers used in the study was based upon the visual clarity of banding patterns generated and a preferably low®ngerprint complexity.The complexity of the banding pattern is a major limiting factor for scoring AFLP®ngerprints of large-size genomes.Data analysisPositions of unequivocally visible and polymorphic AFLP markers were transformed into a binary matrix, with`1'for the presence,and`0'for the absence of a band at a particular position.The genetic distance(GD) between species was based on pair-wise comparisons and calculated according to the equation:GD xy 1) [2N xy/(N x+N y)],where N x and N y are the numbers of fragments to individuals x and y,respectively,and N xy is the number of fragments shared by both(Nei&Li, 1979).Genetic distances were computed by the software package TREECON(v.1.3b)(Van De Peer&De Wachter, 1993).The dendrogram of the22Alstroemeria species, the interspeci®c hybrid,Bomarea and Leontochir was generated based on the GD matrix by using cluster analysis,the UPGMA(unweighted pair group method using arithmetic averages)method with1000bootstraps (Sneath&Sokal,1973;Felsenstein,1985)(Fig.1). Principal co-ordinate analysis was performed to access interspecies relationships based on the Nei&Li(1979) coe cient[2N xy/(N x+N y)]using the NTSYS-PC pro-gram(Rohlf,1989).Results and discussionThe average genetic distance among species excluding Bomarea,Leontochir,the interspeci®c hybrid and A.umbellata was0.65GD(a table showing the genetic distances between all the species studied is available from the authors on request).Alstroemeria umbellata was excluded because the accessions used were found to be highly related and possibly wrongly classi®ed as di erent from A.pelegrina.The average GD among accessions within a species was0.32GD(data not shown).In addition,the average GD between Brazilian species(GD:0.27)and between Chilean species(GD: 0.33)was not signi®cantly di erent.Buitendijk&Ramanna(1996)suggested that the Chilean and Brazilian species form distinct lineages.The genetic diversi®cation of Alstroemeria species as detected by the AFLP technique revealed three main clusters with99%bootstrap values:the Chilean species,the Brazilian species and the outgroup(Fig.1).This®nding would support an early divergence of these groups and is consistent with the occurrence of interspeci®c cross-ing barriers between the Chilean and Brazilian species (De Jeu&Jacobsen,1995;Lu&Bridgen,1997).The variance of the®rst three principal co-ordinates accounted for34.9%of the total variation,di erentia-ted e ectively among the species and re¯ected the main clustering of the dendrogram.From the principal co-ordinate plot,four groups were clearly demarcated:Table2Sequences of adaptors and primers usedEco RI adaptor5¢-CTCGTAGACTGCGTACC-3¢3¢-CTGACGCATGGTTAA-5¢Mse I adaptor5¢-GACGATGAGTCCTGAG-3¢3¢-TACTCAGGACTCAT-5¢Eco RI+0primer E005¢-GACTGCGTACCAATTC-3¢Eco RI+2primers E+AC5¢-GACTGCGTACCAATTCAC-3¢E+AG5¢-GACTGCGTACCAATTCAG-3¢Eco RI+4primers E+ACCA5¢-GACTGCGTACCAATTCACCA-3¢E+ACCT5¢-GACTGCGTACCAATTCACCT-3¢E+AGCC5¢-GACTGCGTACCAATTCAGCC-3¢Mse I+0primer M005¢-GATGAGTCCTGAGTAA-3¢Mse I+2primers M+CA5¢-GATGAGTCCTGAGTAACA-3¢M+CT5¢-GATGAGTCCTGAGTAACT-3¢Mse I+4primers M+CACC5¢-GATGAGTCCTGAGTAACACC-3¢M+CTAC5¢-GATGAGTCCTGAGTAACTAC-3¢M+CTAG5¢-GATGAGTCCTGAGTAACTAG-3¢566T.-H.HAN ET AL.ÓThe Genetical Society of Great Britain,Heredity,84,564±569.(i)the Brazilian group;(ii)the Chilean group;(iii)the A.ligtu group;and (iv)the outgroup (Fig.2).The Brazilian species (A.brasiliensis , A.psittacina and A.inodora )were consistently assigned to one cluster with 98%bootstrap values,whereas the Chilean species were rather weakly clustered with 62%bootstrap values containing several subgroups within the Chilean group (Figs 1and 2).The dispersion of the Chilean species on the principal co-ordinate plot re¯ected a wider geneticvariation than the Brazilian species.However,the narrow variation of the Brazilian species might be caused by the limited number of species investigated.Buitendijk &Ramanna (1996)described the similar-ities between C-banding patterns of A.inodora and A.psittacina ;in our study these species clustered strongly,reinforcing this ®nding (Fig.1).The similarity between A.psittacina and A.inodora was also revealed by allozyme analysis (Meerow &Tombolato,1996)and by a study using species-speci®c repetitive probes (De Jeu et al.,1995).These ®ndings are also supported by the fact that A.inodora and A.psittacina are easily crossed (De Jeu &Jacobsen,1995).In addition,the Chilean species A.aurea was posi-tioned between three subgroups (Fig.2).The unique position of A.aurea ,and the observation that this species has a wide geographical spread,suggest that other Chilean species may have evolved from A.aurea ecotypes.Alstroemeria aurea is indeed a widespread inhabitant in the regions with higher rainfall at the more southern latitudes between 33and 47°S in Chile (Bayer,1987;Buitendijk &Ramanna,1996).It is not found in Brazil,although A.aurea plants are found on both sides of the Andes mountains in Argentina,supporting the possibility that A.aurea ecotypes were also the ancestors of the Brazilian species (A.F.C.Tombolato,personal communication).Alstroemeria pelegrina and A.umbellata were assigned as sister species with a GD of 0.26showing a remarkable genetic similarity (data available on request).The species we coded under the name A.umbellata actually seemed to be an A.pelegrina species that did not ¯ower for many years.Alstroemeria haemantha was assigned to a group together with A.ligtu ssp.ligtu ,A.ligtussp.Fig.1Dendrogram of 22Alstroemeria species,Bomarea salsilla and Leontochir ovallei resulting from a UPGMA cluster analysis based on Nei's genetic distances obtained from 716AFLP bands.The bootstrap analysis was conducted using TREECON (v.1.3b)with 1000bootstrap subsamples of the data matrix.Percent-age values for those branches occurring in at least 60%of the bootstrap topologies areshown.Fig.2Relationships among 22Alstroemeria species,the F 1hybrid,Bomarea salsilla and Leontochir ovallei by principal co-ordinate analysis using Nei and Li coe cients.The three principal co-ordinates accounted for 34.9%of the totalvariation.PC1,PC2and PC3:®rst,second and third principal co-ordinates.See Table 1for species names.EVALUATION OF THE CHILEAN AND BRAZILIAN ALSTROEMERIA SPP.567ÓThe Genetical Society of Great Britain,Heredity ,84,564±569.incarnata and A.ligtu ssp.simsii(Figs1and2)(Aker& Healy,1990;Ishikawa et al.,1997).Bayer(1987) suggested the synonymous name of A.ligtu ssp.ligtu for A.haemantha Ruiz and Pavon.Our results support this hypothesis.Alstroemeria exserens was positioned between the Chilean group and the A.ligtu group (Fig.2).Alstroemeria andina and A.angustifolia ssp. angustifolia,and A.hookeri ssp.cumminghiana and A.hookeri ssp.hookeri were clustered together with 95%and93%bootstrap values,respectively.The interspeci®c hybrid(A1P2±2)was included in our study in order to investigate the possibility of the identi®cation of the parental genotypes.The F1hybrid A1P2±2showed a0.45-GD value with A.inodora and 0.59GD value with A.aurea showing genomic contri-bution of both parents(data available on request).It indicated the feasibility of the AFLP technique as a tool for the identi®cation of parental genotypes (Sharma et al.,1996;Marsan et al.,1998).Bomarea and Leontochir showed the mean GD value of0.83as the outgroup,thus showing large genetic distances within the Alstroemeriaceae family.In conclusion,the genetic variation and the genetic relationships among Alstroemeria species were e ciently rationalized by using AFLP markers for the character-ization of germplasm resources.In general,the topolo-gies of the dendrogram and the principal co-ordinate analysis of our study were in agreement with Bayer's views(Bayer,1987)on the classi®cation of the Als-troemeria species.Furthermore,this technique might be useful for the identi®cation of parental genotypes in interspeci®c hybrids.AcknowledgementThe authors would like to thank Anja G.J.Kuipers and Jaap B.Buntjer for critical reading of the manuscript and for helpful comments.ReferencesAKER,S.AND HEALY,W.1990.The phytogeography of the genus Alstroemeria.Herbertia,46,76±87. ANASTASSOPOULOS,E.AND KEIL,M.1996.Assessment of natural and induced genetic variation in Alstroemeria using random ampli®ed polymorphic DNA(RAPD)markers.Euphytica, 90,235±244.BAYER,E.1987.Die Gattung Alstroemeria in Chile.Mitt.Bot. Staatsamml.MuÈnchen,24,1±362.BUITENDIJK,J.H.AND RAMANNA,M.S.1996.Giemsa C-banded karyotypes of eight species of Alstroemeria L.and some of their hybrids.Ann.Bot.,78,449±457. BUITENDIJK,J.H.,PINSONNEAUX,N.A.C.,VAN DONK,M.S.AND LAMMEREN, A. A.M.1995.Embryo rescue by half-ovuleculture for the production of interspeci®c hybrids in Alstroemeria.Sci.Hortic.,64,65±75.DAHLGREN,R.M.T.AND CLIFFORD,H.T.1982.Monocotyledons.A Comparative Study.Academic Press,London.DE JEU,M.J.AND JACOBSEN, E.1995.Early postfertilization ovule culture in Alstroemeria L.and barriers to interspeci®c hybridization.Euphytica,86,15±23.DE JEU,M.J.,LASSCHUIT,J.,CHEVALIER,F.AND VISSER,R.G.F. 1995.Hybrid detection in Alstroemeria by use of species-speci®c repetitive probes.Acta Hortic.,420,62±64. DUBOUZET,J.G.,MURATA,N.AND SHINODA,K.1997.RAPD analysis of genetic relationships among Alstroemeria L. cultivars.Sci.Hortic.,68,181±189. FELSENSTEIN,J.1985.Con®dence limits on phylogenies:an approach using the bootstrap.Evolution,39,783±791. FREI,O.M.,STUBER,C.W.AND GOODMAN,e of allozymes as genetic markers for predicting performance in maize single cross hybrids.Crop Sci.,26,37±42.HAN,T.H.,VAN ECK,H.J.,DE JEU,M.J.AND JACOBSEN,E.1999. Optimization of AFLP®ngerprinting of organisms with a large genome size:a study on Alstroemeria spp.Theor.Appl. Genet.,98,465±471.HILL,M.,WITSENBOER,H.,ZABEAU,M.,VOS,P.,KESSELI,R.AND MICHELMORE,R.1996.PCR-based®ngerprinting using AFLPs as a tool for studying genetic relationships in Lactuca spp.Theor.Appl.Genet.,93,1202±1210. HUTCHINSON,J.1973.The Families of Flowering Plants. Clarendon Press,Oxford.ISHIKAWA,T.,TAKAYAMA,T.,ISHIZAKA,H.,ISHIKAWA,K.AND MII,M.1997.Production of interspeci®c hybrids between Alstroemeria ligtu L.hybrid and A.pelegrina L.var.rosea by ovule culture.Breed.Sci.,47,15±20. KARDOLUS,J.P.,VAN ECK,H.J.AND VAN DEN BERG,R.G.1998. The potential of AFLPs in biosystematics:a®rst application in Solanum taxonomy.Pl.Syst.Evol.,210,87±103.LU,C.AND BRIDGEN,M.P.1997.Chromosome doubling and fertility study of Alstroemeria aurea´A.caryophyllaea. Euphytica,94,75±81.MARSAN,P.A.,CASTIGLIONI,P.,FUSARI, F.,KUIPER,M.AND MOTTO,M.1998.Genetic diversity and its relationship to hybrid performance in maize as revealed by RFLP and AFLP markers.Theor.Appl.Genet.,96,219±227. MEEROW,A.W.AND TOMBOLATO,A.F.C.1996.The Alstroemeria of Itatiaia.Herbertia,51,14±21.MHAMEED,S.,SHARON,D.,KAUFMAN,D.,LAHAV,E.,HILLEL,J., DEGANI,C.AND LAVI,U.1997.Genetic relationships within avocado(Persea americana Mill.)cultivars and between Persea species.Theor.Appl.Genet.,94,279±286.NEI,M.AND LI,W.H.1979.Mathematical model for studying genetic variation in terms of restriction endonucleases.Proc. Natl.Acad.Sci.U.S.A.,76,5269±5273.PAUL,S.,WACHIRA, F.N.,POWELL,W.AND WAUGH,R.1997. Diversity and genetic di erentiation among populations of Indian and Kenyan tea(Camellia sinensis(L.)O.Kuntze) revealed by AFLP markers.Theor.Appl.Genet.,94,255±263. PICTON, D.D.AND HUGHES,H.G.1997.Characterization of Alstroemeria species using Random Ampli®ed Polymorphic DNA(RAPD)analysis.HortScience,32,482,Abstract:323.568T.-H.HAN ET AL.ÓThe Genetical Society of Great Britain,Heredity,84,564±569.ROGERS,S.O.AND BENDICH,A.J.1988.Extraction of DNA from plant tissues.Plant Mol.Biol.Manual,6,1±10. ROHLF, F.J.1989.NTSYS-Pc Numerical Taxonomy and Multivariate Analysis System,version1.80.Exeter Publica-tions,New York,NY.SCHUT,J.W.,QI,X.AND STAM,P.1997.Association between relationship measures based on AFLP markers,pedigree data and morphological traits in barley.Theor.Appl.Genet., 95,1161±1168.SHARMA,S.K.,KNOX,M.R.AND ELLIS,T.H.1996.AFLP analysis of the diversity and phylogeny of Lens and its comparison with RAPD analysis.Theor.Appl.Genet.,93, 751±758.SNEATH,P.H.A.AND SOKAL,R.R.1973.Numerical Taxonomy. W.H.Freeman,San Francisco,CA.SOLTIS,P.S.,SOLTIS, D.E.AND DOYLE,J.J.1992.Molecular Systematics of Plants.Chapman&Hall,New York,NY. TOHME,J.,GONZALEZ,D.O.,BEEBE,S.AND DUQUE,M.C.1996. AFLP analysis of gene pool of a wild bean core collection. Crop Sci.,36,1375±1384.UPHOF,J.C.T.1952.A review of the genus Alstroemeria.Plant Life,8,37±53.VAN DE PEER,Y.AND DE WACHTER,R.1993.TREECON:a software package for the construction and drawing of evolutionary put.Applic.Biosci.,9,177±182.VOS,P.,HOGERS,R.,BLEEKER,M.,REIJANS,M.,VAN DE LEE,T., HORNES,M.ET AL.1995.AFLP:a new technique for DNA ®ngerprinting.Nucl.Acids Res.,23,4407±4414. WILKIN,P.1997.Leontochir ovallei Alstroemeriaceae.Curtis's Bot.Magazine,14,7±12.EVALUATION OF THE CHILEAN AND BRAZILIAN ALSTROEMERIA SPP.569ÓThe Genetical Society of Great Britain,Heredity,84,564±569.。
Modeling the price dynamics of CO2 emission allowances
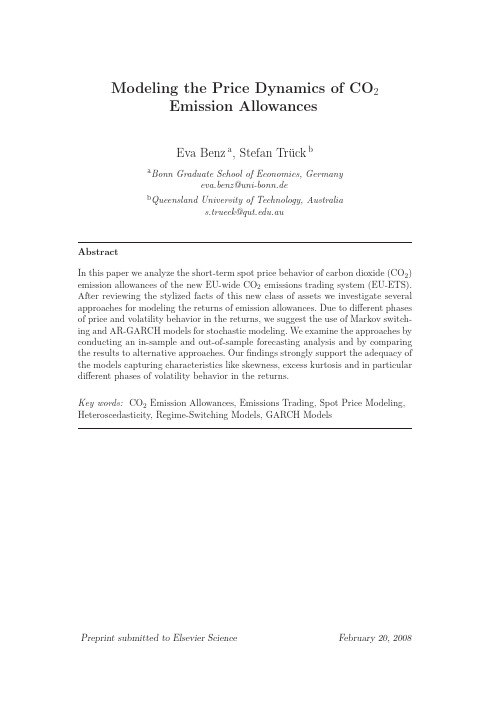
Preprint submitted to Elsevier Science
February 20, 2008
1
Introduction
In January 2005 the EU-wide CO2 emissions trading system (EU-ETS) has formally entered into operation. 1 The new system represents a shift in paradigms, since environmental policy has historically been a command-andcontrol type regulation where companies had to strictly comply with emission standards or implement particular technologies. The EU-ETS requires a cap-and-trade program whereby the right to emit a particular amount of CO2 becomes a tradable commodity. By forcing the participating companies to hold an adequate stock of allowances that corresponds to their CO2 output, the carbon market provides new business development opportunities for market intermediaries and service providers. Risk management consultants, brokers and traders buy and sell emission allowances and their derivatives. Especially for these groups, the price behavior and dynamics of this new asset class - CO2 emission allowances - is of major importance. According to the IETA (2005) and PointCarbon (2005) previous carbon trading activities have been mostly conducted by OTC activities and brokers. Since allowance trading has primarily been applied in the US, the majority of publications about price behavior of tradable emission allowances assesses the market for SO2 emissions under the Acid Rain Program of the US Environmental Protection Agency (EPA). 2 By using industrial organization models they account for changes in parameters of technology (Rezek, 1999) and electricity demand (Schennach, 2000) and their impact on the optimal equilibrium price path for SO2 permits. There is also a number of empirical investigations on ex-post market price analysis, among them Ellerman and Montero (1998), Burtraw (1996) and Carlson et al. (2000). For CO2 market price simulation studies with respect to changes in market design parameters see e.g. Burtraw et al. (2002), B¨ ohringer and Lange (2005), Kosobud et al. (2005) or Schleich et al. (2006). Kosobud et al. (2005) analyze monthly returns of SO2 allowances with respect to other financial assets and find no statistically significant correlation between spot prices in the US and returns from various financial investments. However, literature examining the CO2 allowance prices from an econometThe agreement on a common position was reached in December 2002 and passed the EU-parliament’s second reading in the summer of 2003 (European Union, 2003). The Commision of the European Communities (2001) had already published a proposal for a Directive in October 2001. 2 Trading was established in the 1990 Clean Air Act Amendments, but first trades did not occur until 1992 and emission permits did not have to be submitted to the EPA to cover emissions before 1995.
大口黑鲈北方亚种和佛罗里达亚种的同工酶分析
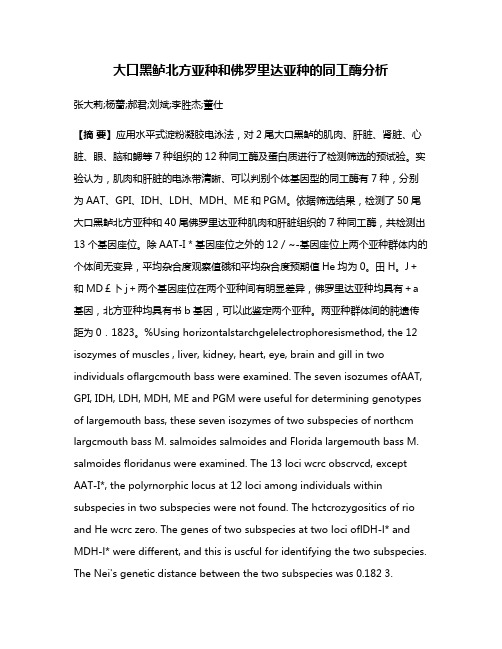
大口黑鲈北方亚种和佛罗里达亚种的同工酶分析张大莉;杨蔷;郝君;刘斌;李胜杰;董仕【摘要】应用水平式淀粉凝胶电泳法,对2尾大口黑鲈的肌肉、肝脏、肾脏、心脏、眼、脑和鳃等7种组织的12种同工酶及蛋白质进行了检测筛选的预试验。
实验认为,肌肉和肝脏的电泳带清晰、可以判别个体基因型的同工酶有7种,分别为AAT、GPI、IDH、LDH、MDH、ME和PGM。
依据筛选结果,检测了50尾大口黑鲈北方亚种和40尾佛罗里达亚种肌肉和肝脏组织的7种同工酶,共检测出13个基因座位。
除AAT-I*基因座位之外的12/~-基因座位上两个亚种群体内的个体间无变异,平均杂合度观察值硪和平均杂合度预期值He均为0。
田H。
J+和MD£卜j+两个基因座位在两个亚种间有明显差异,佛罗里达亚种均具有+a 基因,北方亚种均具有书b基因,可以此鉴定两个亚种。
两亚种群体间的肫遗传距为0.1823。
%Using horizontalstarchgelelectrophoresismethod, the 12 isozymes of muscles , liver, kidney, heart, eye, brain and gill in two individuals oflargcmouth bass were examined. The seven isozumes ofAAT, GPI, IDH, LDH, MDH, ME and PGM were useful for determining genotypes of largemouth bass, these seven isozymes of two subspecies of northcm largcmouth bass M. salmoides salmoides and Florida largemouth bass M. salmoides floridanus were examined. The 13 loci wcrc obscrvcd, except AAT-I*, the polyrnorphic locus at 12 loci among individuals within subspecies in two subspecies were not found. The hctcrozygositics of rio and He wcrc zero. The genes of two subspecies at two loci oflDH-l* and MDH-l* were different, and this is uscful for identifying the two subspecies. The Nei's genetic distance between the two subspecies was 0.182 3.【期刊名称】《天津农学院学报》【年(卷),期】2012(019)003【总页数】5页(P8-12)【关键词】大口黑鲈;同工酶;遗传多样性【作者】张大莉;杨蔷;郝君;刘斌;李胜杰;董仕【作者单位】天津师范大学生命科学学院天津市细胞遗传与分子调控重点实验室,天津300387;天津师范大学生命科学学院天津市细胞遗传与分子调控重点实验室,天津300387;天津师范大学生命科学学院天津市细胞遗传与分子调控重点实验室,天津300387;天津市天祥水产有限责任公司,天津301500;中国水产科学研究院珠江水产研究所,广州510380;天津师范大学生命科学学院天津市细胞遗传与分子调控重点实验室,天津300387【正文语种】中文【中图分类】Q344.51.1 试验鱼大口黑鲈北方亚种于2011年9月21日采自天津市天祥水产有限责任公司,共50尾,平均体长为(15.2±2.63) cm,平均体质量为(114.4±39.48)g;大口黑鲈佛罗里达亚种于2012年1月11日由中国水产科学研究院珠江水产研究所提供,共40尾,平均体长为(15.8±1.98)cm,平均体质量为(93.2±41.49)g。
高一英语七选五专题提高训练(含答案)
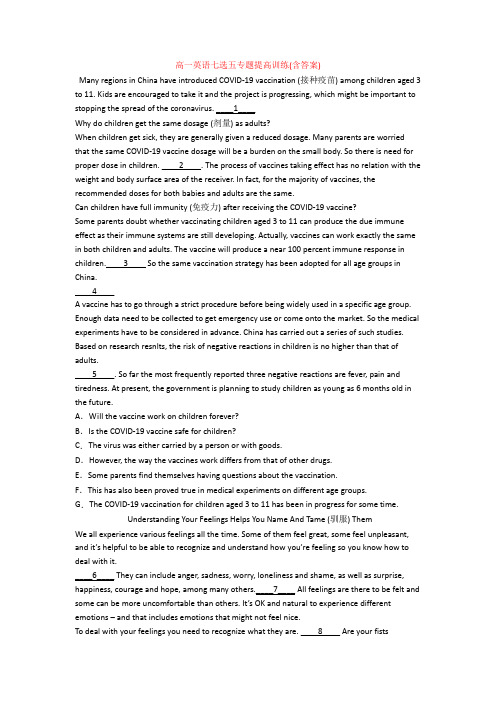
高一英语七选五专题提高训练(含答案)Many regions in China have introduced COVID-19 vaccination (接种疫苗) among children aged 3 to 11. Kids are encouraged to take it and the project is progressing, which might be important to stopping the spread of the coronavirus. ____1____Why do children get the same dosage (剂量) as adults?When children get sick, they are generally given a reduced dosage. Many parents are worried that the same COVID-19 vaccine dosage will be a burden on the small body. So there is need for proper dose in children. ____2____. The process of vaccines taking effect has no relation with the weight and body surface area of the receiver. In fact, for the majority of vaccines, the recommended doses for both babies and adults are the same.Can children have full immunity (免疫力) after receiving the COVID-19 vaccine?Some parents doubt whether vaccinating children aged 3 to 11 can produce the due immune effect as their immune systems are still developing. Actually, vaccines can work exactly the same in both children and adults. The vaccine will produce a near 100 percent immune response in children.____3____ So the same vaccination strategy has been adopted for all age groups in China.____4____A vaccine has to go through a strict procedure before being widely used in a specific age group. Enough data need to be collected to get emergency use or come onto the market. So the medical experiments have to be considered in advance. China has carried out a series of such studies. Based on research resnlts, the risk of negative reactions in children is no higher than that of adults.____5____. So far the most frequently reported three negative reactions are fever, pain and tiredness. At present, the government is planning to study children as young as 6 months old in the future.A.Will the vaccine work on children forever?B.Is the COVID-19 vaccine safe for children?C.The virus was either carried by a person or with goods.D.However, the way the vaccines work differs from that of other drugs.E.Some parents find themselves having questions about the vaccination.F.This has also been proved true in medical experiments on different age groups.G.The COVID-19 vaccination for children aged 3 to 11 has been in progress for some time.Understanding Your Feelings Helps You Name And Tame (驯服) ThemWe all experience various feelings all the time. Some of them feel great, some feel unpleasant, and it’s helpful to be able to recognize and understand how you’re feeling so you know how to deal with it.____6____ They can include anger, sadness, worry, loneliness and shame, as well as surprise, happiness, courage and hope, among many others.____7____ All feelings are there to be felt and some can be more uncomfortable than others. It’s OK and natural to experience different emotions – and that includes emotions that might not feel nice.To deal with your feelings you need to recognize what they are. ____8____ Are your fistsclenched (攥紧)? Does it seem like there’s a knot in your stomach? Next, pay attention to what you’re thinking at this time. ____9____ Or are you thinking that you really don’t want to do something? Once you have identified how you’re feeling, you can label it by saying, for example, “I’m feeling angry” or “I’m feeling lonely” .You can understand a difficult feeling and help yourselfto handle it. ____10____ If you’re upset about a difficult feeling, like “I’m feeling angry”, you might count to ten to calm down. Perhaps you notice “I’m feeling nervous”, and you might talk to someone about it. The person you talk to may be able to give you reassurance, more information, a different point of view, or even help you take action to deal with the cause of your difficult feeling.A.Experts call this “name it to tame it” .B.How can you deal with different feelings?C.Perhaps you have t he thought, “It’s not fair” .D.Feelings are how people experience emotions.E.First of all, notice what’s going on with your body.F.They are shown through various body movements, to begin with.G.Feelings are sometimes labelled as good or bad but that is n’t helpful.Volunteering offers vital help to people in need and the community.______11______.Volunteering can help you find friends, learn new skills, and even advance your career.Volunteering can connect you to others. ______12______ Unpaid volunteers are often the glue (黏合剂). They hold a community together. Volunteering helps meet new people for a newcomer. Dedicating your time as a volunteer helps you make new friends, expand your network, and boost your social skills.______13______Volunteering gives you the opportunity to practice and develop your social skill. You can also gain new skills through it. For example, you could learn nursing skills by volunteering at a nursing home. ______14______ Many volunteering opportunities provide extensive training so that volunteers can gain more professional skills.Volunteering can advance your career. If you’re considering a new career, volunteering can help you get experience in your area of interest and meet people in the field. Even if you’re not planning on changing careers, volunteering gives you the opportunity to practice important skills used in the workplace, such as teamwork, communication, problem solving, project planning, task management, and organization. ______15______A.Volunteering can reduce depression.B.Volunteering can increase your skills.C.It can be hard to find time to volunteer.D.But the benefits can be even greater for you, the volunteer.E.One of the benefits of volunteering is the impact on the community.F.Just because volunteer work is unpaid does not mean the skills you learn are basic.G.You might feel more comfortable at work once you’ve learned these skills in volunteering.Some healthy people have flat abs (腹肌) and thin bodies. ____16____ In fact, the official definition of health—at least, the one used by the World Health Organization (WHO)—saysnothing about the way you look. WHO says health is “a state of complete physical, mental and social well-being and not merely the absence of disease or infirmity.” ____17____You e at when you’re hungry and stop when you’re full. ____18____ “It sounds really silly, but it’s amazing how many of us don’t do that,” Dr. Cindy Geyer, member of the True Health Initiative and medical director at Canyon Ranch in Lenox, Massachusetts, told IN SIDER. “We forget to eat so we’re starving and then we eat a ton, or we’re eating mindlessly in front of the TV, or we’re eating in an emotional context because it’s how we re self-soothing. A healthy relationship with food is trusting your internal cues, not external ones, to decide what and how much to eat,” she said. “I encourage clients to eat until they’re satisfied, but not stuffed.”____19____ A diverse diet ensures that you’re more likely to get all the vitamins and nutrients you need, she explained. This is even more true if the diet is rich in whole foods (天然食品), which tend to be more nutrient-dense than processed stuff.You’re eating enough. Remember, calories aren’t your enemy or some evil force to be reduced at all costs. ____20____ And if you’r e not eating enough of them, you could end up feeling moody, weak, achy, and more.A.How can you tell if you fit that definition?B.You’re eating a varied diet rich in whole foods.C.They give special attention to the way they live.D.This simple behavior is a typical feature of healthy eating.E.But that doesn’t mean these qualities are necessary for good health.F.A healthy relationship with food contributes greatly to good health.G.They’re an energy source that helps you live your life and do what you l ove.Made out of thin airThe world has experienced a lot of extreme weather this year due to climate change, which carbon emissions are believed to be most responsible for. ____21____Meat made from airIt is hard to imagine that food consisting of protein could be produced from CO2, but that is exactly what Solar Foods is working on. To create the protein, the company uses renewable energy to split water cells into hydrogen and oxygen. ____22____ This is fed to microbes(微生物), which in turn create an eatable food, according to science website Futurism. This process makes alternative protein 100 times more climate- friendly than other sources of protein, the company said.____23____What about wearing a pair of shoes made of carbon emissions? On Running, a Swiss sports shoe brand, is trying to make foam(泡沫) for its shoed from captured carbon. In November, it announced it was teaming up with US-based company LanzaTech to make ethanol(乙醇) out of waste CO2, which would otherwise be burned, releasing CO2. On Running hopes to produce its first pair of shoes made wholly from carbon sometime next yeat. ____24____Turning CO2 into perfumeWhat is the smell of a perfume made from CO2? New York-based startup Air Company is selling perfume made from CO2. Perfume has an alcohol base. When mixed with a bit of water andfragrance(芳香) oil, it becomes perfume. Ethanol is widely used in perfume production because it has a neutral smell. This means you only smell the oil. ____25____ And with the addition of water and fragrance oil, you get perfume made mainly from air.A.Running on foamB.Stepping on carbonC.This kind of fragrance oil is made from CO2 by Air Company.D.Then it mixes the hydrogen with CO2 and adds other nutrients.E.They are expected to not cost much more than a regular pair of shoes.F.To solve the problem, capturing and reusing CO2 is an option for tech companies.G.What Air Company is able to do is transform CO2 into a very pure form of ethanol.Whether it’s to improve your fitness, health or environment, ta king up bicycle riding can be one of the best decisions you have ever made.Save the planet____26____. Twenty bicycles can be parked in the same space as one car. It takes around 5 percent of the materials and energy used to make a car to build a bike, and a bike produces zero pollution.____27____.Cycling just 20 miles a week reduces your risk of heart disease to less than half that of those who take no exercise. Studies from Purdue University in the US have shown that regular cycling can cut your risk of heart disease by 50 percent.Enjoy healthy family timeCycling is an activity the whole family can do together. It’s kind on your joints, and there’s nothing to stop grandparents joining in too. Moreover, your riding habit can be sowing the seeds for your kids.____28____. Put simply, if your kids see you riding regularly, they will think it’s normal and want to follow your example.Increase your brain powerDo you want to be smarter and increase your brain power?____29____. That’s because cycling helps build new brain cells in the hippocampus—the part responsible for memory which goes worse from the age of 30. ____30____. It increases blood flow and oxygen to the brain.Make you happyEven if you’re not in a good mood, just three 30-minute rides a week can be enough to give people the lift they need.A.Then just go cyclingB.Keep your heart strongC.Make creative breakthroughsD.Bicycles can save a lot of spaceE.The benefits of cycling is beyond wordsF.Cycling through the miles will lift your spiritsG.Th ey are influenced by their parents’ exercise choicesSymptoms of Dehydration (脱水)You’re bad-tempered.Researchers tested the mood and concentration of 25 women who drank healthy amounts of water one day, and then less the next two days. When slightly dehydrated, the women reported tiredness, bad temper, headaches and difficulty in focusing. In a separate test, men with mild dehydration also had trouble with mental tasks. ____31____ Scientists are still trying to figure out why.You have a bad workout.____32____ It impacts how much you can push yourself. Even a 2 to 3 percent fluid loss affects your ability to get a good workout and more than 5 percent dehydration decreases exercise capacity by about 30 percent.____33____Driving while you’re dehydrated may be just as dangerous as getting behind the wheel drunk, in terms of how many mistakes you could make on the road. British researchers had participants take 2-hour drives using a simulator (模拟器). When they drank enough water, there were 47 driving errors. ____34____You feel dizzy when you stand up too fast.Dehydration can make you feel dizzy or faint, or bring on that rush of light-headedness after you quickly get up from sitting or lying down.The exact treatment for dehydration symptoms depends on age and how severely dehydrated someone is. ____35____ Most of the time, however, people use some over-the-counter solutions for kids, and adults can drink more water.A.You drive like you’re drunk.B.Sometimes dehydration can be life-threatening.C.To get rid of dehydration you have to drink much water quickly.D.In extreme cases, people might go to the hospital for a treatment.E.Dehydration reduces blood pressure and makes the heart work harder.F.But when it came to mood changes, women changed much more than men.G.But when they were short of water, there were more than double the driving errors to 101. In recent years, science fictions are becoming increasingly popular. Science fiction writers using their magical imagination create imaginary worlds that attract a great number of readers especially teenagers. But how can they make it so believable? ____36____The way things work in your imaginary worlds will be based on actual science. So you must be familiar with the scientific laws related to your creat ion. If you’re writing about humans living on a planet with zero gravity, then you need to know the effects of zero gravity on the human body. ____37____ Only in this way can you gain the readers’ trust.Then the rules in your creation can be different from our daily life, so you have to figure out the exact rules of your imaginary worlds. ____38____ For example, if humans in your creation are able to breathe underwater in Chapter 1, your characters can’t drown(溺水)in a swimming pool or river in Chapter 3.____39____ You should decide the following issues: the history of the world, the geography, what possibilities it offers, how everything works in this new reality, as well as how all of these factors affect the way your characters think, feel, and react. You don’t have to tell your readers allthe rules in the first chapter. But you have to let readers know enough to understand what’s going on.When you are writing, remember to make it feel real. You are creating a new real world for the readers. ____40____ They are able to see, hear, feel, smell, and even taste what it’s like in the new world. Whether your novel is about a world without disease or an undiscovered planet, help your readers feel like they’ re actually there.A.And you have to follow them.B.You are inviting them to visit the new world.C.You have to get rich imagination to create science fictions.D.Make sure what you are writing is not against basic science.E.Characters in the imaginary worlds always have super power.F.Here you will find the answer if you are longing to create one.G.Your preparation work also involves planning everything in great detail.One after another, celebrities (名人) have been shocking fans with their dishonesty and disappointing activities. ____41____ She has been asked to pay an surprisingly 1.34 billion yuan in taxes.Before Viya's case came to light, calls for "regulating (规范)" the celebrity fan clubs had been raised after Chinese-American singer-songwriter Wang Leehom's wife accused (控告) him of having disappointing affairs with the other persons. ______42______ Despite Wang trying to argue against the netizens'naming and shaming, various brands have dropped him as their ambassador (大使).Celebrities always have a large crowd of crazy fans. Many of them were born in the 1990s or 2000s, raising large amounts of money to promote their idols. _____43_____Concerns over the rising influence of crazy fans on young minds have come to public attention once again. ____44____ They promise to make greater efforts to make sure youngsters don't become crazy celebrity fans.____45____ But they should behave properly. The celebrities, on their part, should also guide their fans to develop friendly fan culture. It' time to stop following the celebrities blindly. A.Wang is under fire from Asian netizens.B.These youngsters, mostly students, are easily affected.C.The latest on the list is Huang Wei, popularly known as Viya.D.The Ministry of Culture and Tourism has promised to take action.E.This is very important because of the huge number of fans in China.F.There is nothing wrong in some people cheering for a certain celebrity.G.The problem is that most fan clubs use the online platforms as war zones.What is cross country running? Cross country is an outdoor endurance (耐久性) sport that can be mentally challenging and fun. ____46____ You’re not only putting one foot in front of the other; you’re also thinking ahead to barriers and changes in the trail or course.____47____ However, before you begin training and racing, you should be aware of potential injuries and how to avoid them. How can you prevent cross country running injuries? The bestways for outdoor runners to stay healthy include the following running tips:Stretch daily. For all runners and athletes, a regular stretching routine is an important part of conditioning. ____48____Warm up and cool down. Muscles and tendons (跟腱) are less likely to overstretch or tear if they’ve been properly prepared for running and racing. High-intensity workouts require more prep and post-workout cool down than low-impact activities.Wear the right shoes. Make sure your shoes fit properly and that they are neither too tight-fitting nor too loose. ____49____Eat and drink enough. Competitive distance runners like to keep their weight low. However, consuming too few calories can harm your body, especially if you’re a girl or woman subject to eating disorders. ____50____ It’s effective to prevent injury and heat illness. Remember that especially in full sun or in humid regions of the state, you particularly tend to have heat stroke. Drink plenty of water before and after running.A.Cross country running is a sport requiring money.B.Tie double-knots to prevent tripping over undone laces.C.It’s mostly very safe in comparison to other team sp orts.D.Have a well-balanced diet and be sure to stay well-hydrated.E.You may encounter unexpected, non-natural barriers.F.It is needed to keep your bones, joints, and muscles healthy.G.This type of running and racing has both a physical and psychological role.【参考答案】***试卷处理标记,请不要删除1.无1、E牝2、D牝3、F牝4、B牝5、G【分析】这是一篇说明文。
微卫星标记遗传多样性的度量指标及影响因素
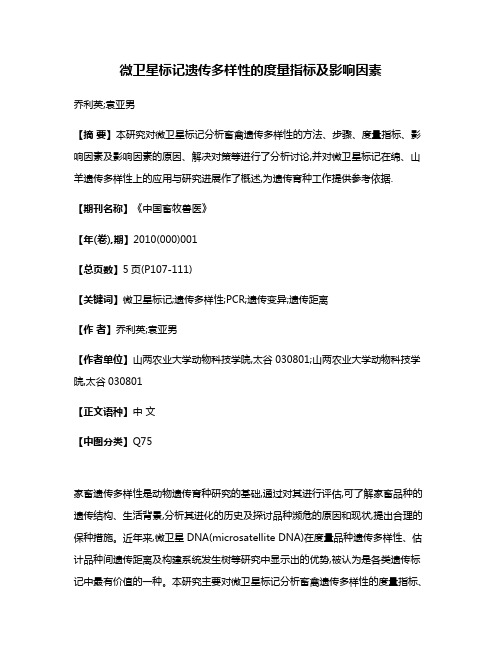
微卫星标记遗传多样性的度量指标及影响因素乔利英;袁亚男【摘要】本研究对微卫星标记分析畜禽遗传多样性的方法、步骤、度量指标、影响因素及影响因素的原因、解决对策等进行了分析讨论,并对微卫星标记在绵、山羊遗传多样性上的应用与研究进展作了概述,为遗传育种工作提供参考依据.【期刊名称】《中国畜牧兽医》【年(卷),期】2010(000)001【总页数】5页(P107-111)【关键词】微卫星标记;遗传多样性;PCR;遗传变异;遗传距离【作者】乔利英;袁亚男【作者单位】山两农业大学动物科技学院,太谷030801;山两农业大学动物科技学院,太谷030801【正文语种】中文【中图分类】Q75家畜遗传多样性是动物遗传育种研究的基础,通过对其进行评估,可了解家畜品种的遗传结构、生活背景,分析其进化的历史及探讨品种濒危的原因和现状,提出合理的保种措施。
近年来,微卫星DNA(microsatellite DNA)在度量品种遗传多样性、估计品种间遗传距离及构建系统发生树等研究中显示出的优势,被认为是各类遗传标记中最有价值的一种。
本研究主要对微卫星标记分析畜禽遗传多样性的度量指标、影响因素及微卫星遗传多样性在绵、山羊上的应用与研究进展作了概述,为绵、山羊的遗传育种工作提供参考依据。
1 微卫星标记的检测程序从动物血液或组织中提取基因组DNA,选择特异性较高的引物通过聚合酶链式反应(polymerase chain reaction,PCR)技术进行DNA扩增。
PCR技术的反应体系与条件的优化至关重要,特别是引物的特异性高低直接影响到分析结果的准确性。
然后在1%~2%的琼脂糖凝胶上检测有无产物带,再取检测阳性产物用6%~10%聚丙烯酰胺凝胶电泳分离,用数字凝胶成像分析系统进行等位基因分型。
据研究,微卫星产物在非变性聚丙烯酰胺凝胶中,表现为有较多的非特异带,而在变性胶中微卫星扩增产物条带清晰,易于鉴定(曲鲁江等,2004)。
2 遗传多样性的度量指标及影响因素2.1 等位基因频率和等位基因数2.1.1 等位基因频率(allele frequencies)微卫星呈共显性遗传,其基因型直接反映表型。
利用17个微卫星标记分析鳙鱼的遗传多样性
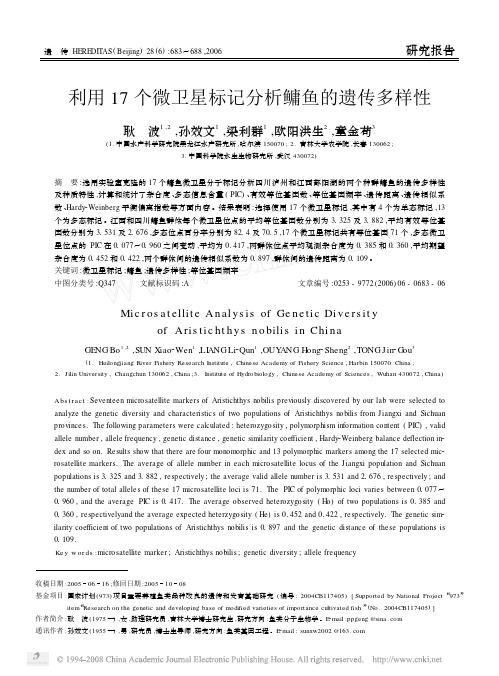
遗 传HEREDIT AS(Beijing)28(6):683~688,2006研究报告利用17个微卫星标记分析鳙鱼的遗传多样性耿 波1,2,孙效文1,梁利群1,欧阳洪生2,童金苟3(1.中国水产科学研究院黑龙江水产研究所,哈尔滨150070;2.吉林大学农学院,长春130062;3.中国科学院水生生物研究所,武汉430072)摘 要:选用实验室克隆的17个鳙鱼微卫星分子标记分析四川泸州和江西鄱阳湖的两个种群鳙鱼的遗传多样性及种质特性,计算和统计了杂合度、多态信息含量(PIC)、有效等位基因数、等位基因频率、遗传距离、遗传相似系数、Hardy2Weinberg平衡偏离指数等方面内容。
结果表明:选择使用17个微卫星标记,其中有4个为单态标记,13个为多态标记。
江西和四川鳙鱼群体每个微卫星位点的平均等位基因数分别为31325及31882,平均有效等位基因数分别为31531及21676,多态位点百分率分别为8214及7015,17个微卫星标记共有等位基因71个,多态微卫星位点的PIC在01077~01960之间变动,平均为0.417,两群体位点平均观测杂合度为01385和01360,平均期望杂合度为01452和01422,两个群体间的遗传相似系数为01897,群体间的遗传距离为01109。
关键词:微卫星标记;鳙鱼;遗传多样性;等位基因频率中图分类号:Q347 文献标识码:A 文章编号:0253-9772(2006)06-0683-06Mi c r os a t ellit e Anal ys is of Ge ne tic Di v e r s it yof Aris ti c ht h ys n o bilis i n Chi naGE NG Bo1,2,SUN X iao2Wen1,LI ANGLi2Qun1,OUY ANG H ong2Sheng2,TONG Jin2G ou3(1.Heilongjiang River Fishery Research Institute,Chinese Academy of Fishery Science,Harbin150070China;2.Jilin University,Changchun130062,China;3.Institute of Hydrobiology,Chinese Academy of Sciences,Wuhan430072,China)Abs t ra ct:Seventeen microsatellite markers of Aristichthys nobilis previously discovered by our lab were selected to analyze the genetic diversity and characteristics of two populations of Aristichthys nobilis from Jiangxi and Sichuan province s.The following parameters were calculated:heterozygosity,polymorphism in formation content(PIC),valid allele number,allele frequency,genetic distance,genetic similarity coefficient,Hardy2Weinberg balance deflection in2 dex and so on.Results show that there are four monomorphic and13polymorphic markers among the17selected mic2 rosatellite markers.The average of allele number in each microsatellite locus of the Jiangxi population and Sichuan populations is31325and31882,respectively;the average valid allele number is31531and21676,respectively;and the number of total allele s of these17microsatellite loci is71.The PIC of polymorphic loci varies between01077~01960,and the average PIC is01417.The average observed heterozygosity(H o)of two populations is0.385and 01360,re spectivelyand the average expected heterzygosity(He)is0.452and0.422,respectively.The genetic sim2 ilarity coefficient of two populations of Aristichthys nobilis is01897and the genetic distance of these populations is 01109.Ke y w or ds:microsatellite marker;Aristichthys nobilis;genetic diversity;allele frequency收稿日期:20050616;修回日期:20051008基金项目:国家计划(973)项目重要养殖鱼类品种改良的遗传和发育基础研究(编号:2004CB117405)[Supported by National Project“973”item“Research on the genetic and developing base of modified varieties of importance cultivated fish”(No.2004CB117405)]作者简介:耿 波(1975—),女,助理研究员,吉林大学博士研究生,研究方向:鱼类分子生物学。
GENETIC DIVERSITY OF FIVE FRESHWATER MUSSELS IN GENUS ANODONTA MOLLUSCA BIVALVIA REVEALED BY RAP
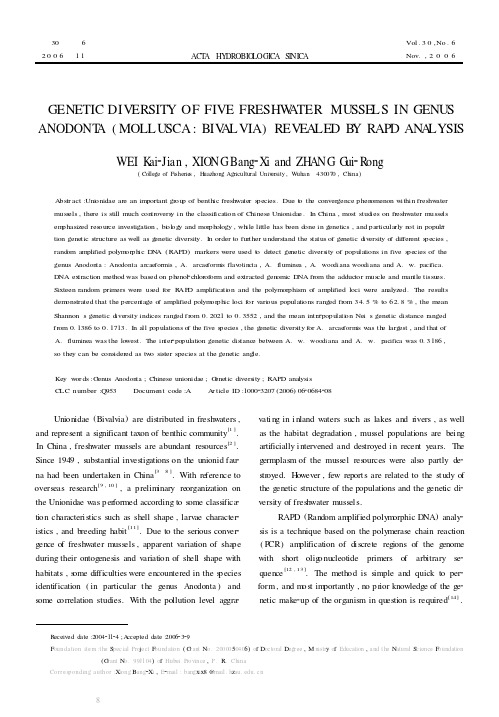
Unionidae ( Bivalvia ) are distributed in freshwaters , and represent a significant taxon of benthic community . In China , freshwater mussels are abundant resources[2 ] . Since 1949 , substantial investigations on the unionid fau2 na had been undertaken in China . With reference to [ 9 , 10 ] overseas research , a preliminary reorganization on the Unionidae was performed according to some classifica2 tion characteri stics such as shell shape , larvae character2 istics , and breeding habit [1 1 ] . Due to the serious conver2 gence of freshwater mussels , apparent variation of shape during their ontogenesis and variation of shell shape with habitats , some difficulties were encountered in the species identification ( in particular t he genus Anodonta ) and some correlation studies. With the pollution level aggra2
微卫星标记分析福建地方品种猪的遗传多样性

微卫星标记分析福建地方品种猪的遗传多样性翁 润 赖丽萍 罗锦坦 王寿昆3福建农林大学动物科学学院 福州 350002摘 要 采用8个微卫星座位对福建省5个地方猪种(槐猪、官庄花猪、莆田黑猪、闽北花猪、武夷黑猪)的遗传结构进行了分析,计算了遗传杂合度、有效等位基因数、多态信息含量、N ei 氏标准遗传距离。
结果表明:8个位点均为高度多态位点;5个猪种群体内的遗传多样性比较高,8个微卫星位点平均杂合度介于017006~017760之间;多态信息含量在016917~017609之间;槐猪与武夷黑猪的遗传距离最小015531,官庄花猪与闽北花猪的遗传距离最大018618。
关键词 猪 地方品种 微卫星 遗传多样性中图分类号:S81319 文献标识码:A 文章编号:1003-4331(2007)07-0001-03G enetic diver sity o f indigenous pig br eeds in Fuji an Pr ovi nce based on micr osatellite mar ker s studyWeng Run Lai lipin g Lu o Jintan Wang Sh oukun(College of An imal Science ,Fujian A gricultural and F orestry University ,Fu zh ou 350002,China)Abstract Eight microsatellite DN A markers w ere used to s tudy the genetic diversity of five indigen ous pig breeds (H uai pig;G uan zhuang pig;Putian pig;M inbei pig ;W uyi pig ).Heterozyg osity ,effective number o f alleles ,polym orph ism information con tent (PIC )and N ei ’s standard g enetic dis tance were counted.Th e results were sh owed as follow :8microsatellite loci w ere mediu m or high polym orph ism ;T he g enetic div ersity in five populations was higher ;and the averag e genetic heterozyg osity w as between 0.7006and 0.7760;the av erage polym orph ism in formation content w as b etw een 0.6917and 0.7609.T he genetic dis tan ce bet ween Putian pig and Minb ei pig was the neares t (0.5531),w hile the g enetic dis tance betw een G uan zhuang pig and M inbei pig w as the furthest (0.8618).K ey w or ds Pig indigen ous breed M icrosatellite G enetic divers ity 福建省目前还保存着6个地方猪品种即槐猪、莆田黑猪、闽北花猪、武夷黑猪、官庄花猪、福安花猪,其中的槐猪、莆田黑猪被列入国家级畜禽遗传资源保护品种。
Measures_of_Genetic_Distance
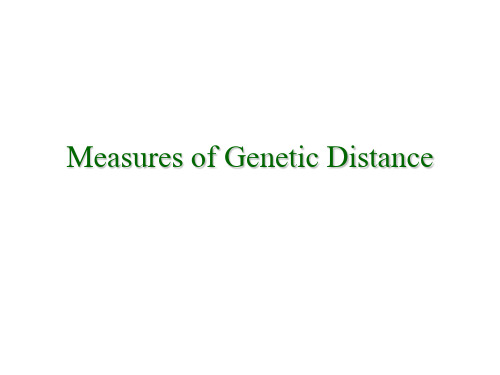
Measures of Genetic Distance 四氟板、尼龙棒、有机玻璃板Genetic Distance (D)•Quantitative measure of genetic divergencebetween two sequences, individuals, or taxa •Relative estimate of the time that has past sincetwo populations existed as a single, panmicticpopulation•Units of D depend on the kind of molecular data collected (allozymes, nucleotide sequences, etc.)3 Most Commonly used DistanceMeasures•Nei’s genetic distance (Nei, 1972)•Cavalli-Sforza chord measure (Cavalli-Sforza and Edwards, 1967)•Reynolds, Weir, and Cockerham’s genetic distance (1983)•Nei’s assumes that differences arise due to mutation and genetic drift, C-S and RWC assume genetic drift onlyNei’s Genetic Distance•D= -ln Iwhere I= Σx i y i/ (Σx i2Σy i2)0.5•For multiple loci, use the arithmetic means across all loci•Interpreted as mean number of codon substitutions per locusAssumptions for Nei’s Distance•IAM•All loci have same rate of neutral mutation •Mutation-genetic drift equilibrium •Stable effective population sizeCavalli-Sforza Chord Distance •populations are conceptualised as existing as points in a m-dimensional Euclidean space which are specified by mallele frequencies (i.e. m equals the total number of alleles in both populations). The distance is the angle betweenthese points:•xi and yi are the frequencies of the i th allele in populations x and y •Assumes genetic drift only (no mutation)•Geometric distance b/w points in multi-dimensional spaceReynold’s Distance•Assumes IAM•Developed for allozyme data on small populations and assumes genetic drift is only force operating on allelic frequencies (i.e. no mutation)•Based on the coancestry coefficient, θD = -ln(1-θ)What is Coancestry?•Degree of relationship by descent betweentwo individuals•Probability that a randomly picked allele from one individual is IBD to a randomly picked allele in another individualTesting Significance of DistanceMeasures •Bootstrap: generation of many new data sets by resampling original data with replacement•For each bootstrap data set, obtain estimates of parameters of interest and their variances •Generates confidences intervals of parameter estimatesPhylip•Computes Nei’s, C-S, and Reynold’s genetic distances using GENDIST (we will do this in lab today)•Uses Bootstrap to generate confidence intervals (but we don’t know how to view that output)•Other programs that estimate distance: TFPGA, GDA, Popgene, DISPANLots of other Distance Measures!•Euclidean distance•Shared allele distance•Roger’s distance•Goldstein distance (for microsatellites)In Lab Today:•Use Phylip to estimate genetic distance for Bear data•AMOV A using Arlequin。
Inference with difference-in-differences and other panel data
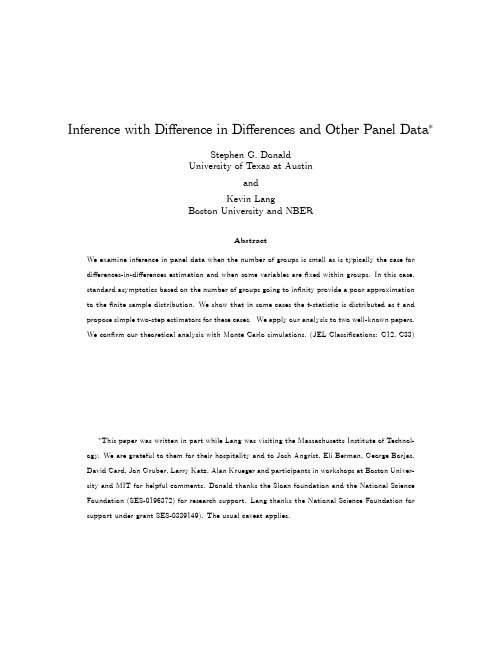
Inference with Difference in Differences and Other Panel Data∗Stephen G.DonaldUniversity of Texas at AustinandKevin LangBoston University and NBERAbstractWe examine inference in panel data when the number of groups is small as is typically the case fordifferences-in-differences estimation and when some variables arefixed within groups.In this case,standard asymptotics based on the number of groups going to infinity provide a poor approximationto thefinite sample distribution.We show that in some cases the t-statistic is distributed as t andpropose simple two-step estimators for these cases.We apply our analysis to two well-known papers.We confirm our theoretical analysis with Monte Carlo simulations.(JEL Classifications:C12,C33)∗This paper was written in part while Lang was visiting the Massachusetts Institute of Technol-ogy.We are grateful to them for their hospitality and to Josh Angrist,Eli Berman,George Borjas,David Card,Jon Gruber,Larry Katz,Alan Krueger and participants in workshops at Boston Univer-sity and MIT for helpful comments.Donald thanks the Sloan foundation and the National ScienceFoundation(SES-0196372)for research ng thanks the National Science Foundation forsupport under grant SES-0339149).The usual caveat applies.1IntroductionMany policy analyses rely on panel data in which the dependent variable differs across individuals but at least some explanatory variables,such as the policies being studied,are constant among all members of a group.For example,in the typical differences-in-differences model,we regress outcomes at the individual level(e.g.employment in afirm in state s in year t)on a policy that applies to all individuals in the group(e.g.the minimum wage in state s in year t).Moulton(1990)shows that in regression models with mixtures of individual and grouped data,the failure to account for the presence of common group errors can generate estimated standard errors that are biased downwards dramatically.1The differences-in-differences estimator is a special case of this model.Researchers use a number of standard techniques to adjust for common group effects:•random-effects feasible GLS estimation which under certain conditions is asymptotically efficient,•correcting the standard errors using the error covariance matrix based on common group errors as in Moulton,•correcting the standard errors using a robust covariance estimator according to a formula developed by Liang and Zeger(1986)and more commonly known as the Stata cluster command.This paper makes two simple,but,we believe,important points.First,when applied to variables that are constant within a group,the t-statistics generated using each of these techniques for correcting for common group errors are asymptotically normally distributed only as the number of groups goes to infinity.Second,under standard restrictions,the efficient estimator can be implemented by a simple two-step procedure,and the resulting t-statistic may have,under restrictions on the distribution of the group level error,an asymptotic t-distribution as the number of observations per group goes to infinity.In addition,under more restrictive assumptions,when the same procedure is used infinite samples,the t-statistics have a t-distribution.Consequently,standard asymptotics cannot be applied when the number of groups is small as in the case where we compare two states in two years,two cities over a small number of years,or self-employed workers and employees over a small number of years.In such cases,failing to take account of the group-error structure will not only generate underestimates of the standard errors as in Moulton, but applying the normal distribution to corrected t-statistics will dramatically overstate the significance of the statistics.Standard asymptotics should apply to comparisons across allfifty states although otherproblems may arise in common time-series/cross-section estimates based on states and using long panels (see Bertrand et al,2004).2In the next section,wefirst present an intuitive argument and then formalize the conditions under which we can derive the distribution of the t-statistic when the number of groups is small.Readers who are not interested in the details can skip the later part of this section and proceed to the third section,where we discuss the common two group/two period case and also apply our approach to two influential papers:the Gruber and Poterba(1994)paper on health insurance and self-employment and Card’s(1990)study of the Mariel boatlift.We show that analyzing the t-statistic,taking into account a possible group error component,dramatically reduces our estimate of the precision of their results.In the fourth section,we consider two other approaches to common group errors,the Moulton correc-tion and the commonly applied Stata cluster correction.In sectionfive,we present Monte Carlo evidence regarding the distribution of the t-statistic using a variety of estimators.Our results indicate that the t-statistics produced by standard estimators have distributions that differ quite substantially from both the normal and the t-distributions.However,when the theory predicts that they should,the two-step estimators we propose produce t-statistics with approximately a t-distribution with degrees of freedom equal to number of groups minus number of group-constant variables.Moreover,one of the two-step estimators we consider appears to be reasonably robust to the departures from the assumptions needed to guarantee that the t-statistic has a t-distribution.2The Error Components Model with a Small Number of Groups We begin with a standard time-series/cross-section model of the formY is=a+X sβ+Z isγ+αis+εis.(1)whereαis is an error term that is correlated within group s andεis is an individual-specific term that is independent of the other errors.With a single cross-section Y might be income,X state laws and Z characteristics of individuals.In this case it would be natural to follow Moulton and assume thatαis a state effect that does not vary among members in a group,that is thatαis=Σiαis/N s≡αs.We do not require that the error term take the Moulton structure,only that theσ2α(the variance ofαs) depend only on the number of observations from group s and that,as group size gets large,it converge in probability to somefinite value.3If the covariance matrix of the error term is known,GLS estimation of(1)is efficient.With some regularity conditions,feasible GLS is efficient if the covariance matrix can be estimated consistently.De-pending on the structure of the covariance matrix,GLS can be computationally burdensome.Moreover, if the exact structure of the dependence is unknown,GLS estimation may be infeasible.Estimatingβin two stages is often computationally simpler.In this case,we use OLS to estimatey=Zγ+WΓ+ε(2) where W is a set of dummy variables indicating group membership.Note thatΓ=Xβ+α.(3) We then can use the estimated bΓin GLS estimation ofbΓs=X sβ+αs+(bΓs−Γs)(4) where the error term has varianceσ2αI+var(bΓ).Amemiya(1978)shows that if the covariance matrices ofαandεare known,then the two-step procedure and the GLS procedure applied directly to(1)are numerically identical.If instead feasible GLS is used,then provided the covariance terms are estimated in the same fashion,numerical equivalence continues to hold.More commonly,the two approaches lend themselves to different methods for obtaining consistent estimates of the covariance terms.If so,the equivalence is asymptotic rather than numeric.Our contribution is twofold.First,we can see from(4)that if the number of groups is small,then it is not possible to rely on the consistency of estimates ofσ2αto justify feasible GLS estimation of(4). However,ifσ2αI+var(bΓ)is homoskedastic and diagonal,by the usual arguments,it is still possible to obtain an unbiased estimate of the variance of the error term in(4).Under normality assumptions onαs,the resulting t-statistic will have a t-distribution rather than a normal distribution.We explore circumstances under which the assumption of homoskedasticity is reasonable.In particular,the error term will be homoskedastic under at least two circumstances:1.if the number of observations per group is large,or2.if there are no within-group varying characteristics and the number of observations is the same forall groups.Second,when the error term in(4)is homoskedastic,by standard theorems,OLS estimation of(4) is efficient.Since OLS estimation of(4)is numerically equivalent to feasible GLS estimation of(1),we have full efficiency of estimation even when the number of groups is small.We begin our formal treatment with the case where all variables arefixed within groups.2.1Only Within-Group-Constant Explanatory VariablesWe begin by treating the case where X s is a scalar and there are no within-group varying explanatory variables(γ=0),so thatY is=a+X sβ+αis+εis.(5)This case provides much of the intuition for the more general case.4Throughout we will assume that theεis are independent of each other and ofαfor all i and s.We further assume thatαis andαjs0are independent for s=s0,but do not assume thatαis andαjs are uncorrelated.The two-step estimator in this case has a very simple interpretation.Thefirst-stage is equivalent to taking group means,b d s=P N s i=1Y is s(6) so that the second stage becomesb d s=Y s=a+X sβ+P N s i=1αis N s+P N s i=1εis N s(7)≡a+X sβ+αs+εs(8)≡a+X sβ+ηs.(9) which is just the“between-groups”estimator ofβ.A few points follow immediately from the equivalence of GLS estimation of(5)and(9).1.βcan always be estimated efficiently by appropriate weighted least squares estimation of(9)if theweights are known or by feasible weighted least squares if they can be estimated consistently.2.If eitherηis homoskedastic or var(ηs)is uncorrelated with X s,then the efficient estimator isthe unweighted between estimator.Note that homoskedasticity is a natural assumption whenever either all groups have the same number of observations(N s=N,∀s)or when the number of observations in each group is large.The latter point demonstrates that in many circumstances unweighted between-group estimation is the most efficient estimator5and that this efficient estimator can be achieved without knowledge of the exact covariance structure ofα,although as noted this does require that the variance of ofηs is constant across groups.2.1.1InferenceIt should be apparent that our ability to perform inference on bβdepends primarily on S and not on N s. If the number of groups is large,then the standard theorems establish that whenηis homoskedastic,bβols is normally distributed and the t-statistic follows the normal distribution.Whenηis heteroskedastic, the same is true either for feasible GLS or for appropriately calculated standard errors.In many cases it will be natural to treat S as large and the error term as homoskedastic.For example, studies that use difference in laws across states and have large samples for allfifty states are likely to meet this requirement approximately.However,in many applications the number of groups is small. The well-known Card and Krueger(1994)minimum-wage study is a case in point in which there is a large number of observations per group but only four groups(New Jersey before and after the law and eastern Pennsylvania before and after the law).Other studies(Gruber and Poterba,1994;Card,1990; Eissa and Liebman,1996)are based on a small number of group/year cells.When the number of groups is small,in order to have a standard solution for the distribution of the t-statistic,we require thatηbe i.i.d.normally distributed.Below,we present formal sufficient conditions for this requirement to be satisfied.If the distribution ofηis i.i.d.normal,then it follows from standard theorems that the t-statistic for bβhas a t-distribution with S−2degrees of freedom.In effect,failure to recognize that the variance of the error term is estimated using very few observations can dramatically overstate the significance of findings.Why does the distribution of b T remain t despite the large number of observations?The answer is quite intuitive.If we relied on published census data to estimate a relation based on the New England states,we would automatically assume that the resulting t-statistic had a t-distribution.Relying on the underlying individual data cannot help us if all of the information in the data is included in the mean.Somewhat more formally,rewrite(9)ase Y s=e X sβ+eηs(10)where˜denotes a deviation from the mean.The usual t-statistic for hypotheses concerningβis given byˆT=ˆβ−βˆση(P s X2s)1/2,ˆσ2η=1S−2S X s=1(e Y s−e X s bβ)2.(11) Given this fact,we can easily see that it will be reasonable to use a t(S−2)distribution for conducting inference wheneverηs is exactly or is approximately a homoskedastic normal random variable.Finite Sample Result:Here for theˆT statistic to have an exact t(S−2)distribution it is sufficient thatηs=P N s i=1αis N s+P N s i=1εis N s∼N(0,σ2η)where it is important thatσ2ηis constant across s.Although there may be a variety of conditions the most obvious case is whereαis=αs∼N(0,σ2α)for all i,εis∼N(0,σ2ε)and N s=N for all s so thatηs∼N(0,σ2η)where,σ2η=σ2α+σ2ε(12)This is the standard random effects time-series/cross-section model as well as the specification used by Moulton.This includes the possibility that there are no group specific effects.Large Sample Result:For theˆT statistic to have a distribution that is well approximated by t(S−2)it is sufficient that there be large N s and that,ηs A∼N(0,σ2η)(13) which requires some form of asymptotic theory regardingηs with N s→∞but with the number of groupsfixed.Here there are at least two interesting possibilities.(i)For each s,αis=αs∼N(0,σ2α)for all i andεis satisfy conditions for a Law of Large Numbers toimply thatp limN s→∞P N s i=1εis s=0(14) In this instancep limN s→∞ηs=αs∼N(0,σ2α)so that the condition(13)is met.This does not require that N s be the same in all groups but for the approximation to be valid we would need all groups to have large N s so that(14)is approximately true.(ii)For each s,αis=αs∼N(0,σ2α/N s)for all i,εis satisfy conditions for a Central Limit Theorem and N s/N t→1for any s=t then,ηs A∼N(0,(σ2α+σ2ε)/N s)where the variance asymptotically does not depend on s.This case is possible under a parameter sequence that keepsσ2αof the same(or smaller)order asσ2ε/N s as might be appropriate when the group effects are relatively small.Indeed this includes the possibility thatαs=0so that there are no group specific effects Also note that in this case we do not require normality for theεis but for the approximation to be a good one we require that there be similar numbers of observations per group.There may be other possibilities in the large sample case although we refrain from giving more explicit conditions.For instance provided that one can show that if(P iαis)/N s is approximately normal(with constant variance independent of s asymptotically)and thatεis satisfy conditions for a Law of Large Numbers then the condition in(13)will hold and using a t(S−2)will provide a good approximation. There are a variety of possibile assumptions one could use to obtain the approximate normality of (P iαis)/N s that relate to the dependence across observations within a group—the simplest case is given in(i)above,but it is apparent that the two-stage technique can accommodate inference even when the nature of the dependence within groups is unknown provided that there is no correlation of errors across groups.6Also,as in case(ii)for this result to hold one would require N s to be(asymptotically) constant across groups.It is worth noting that estimating the between groups estimator is a matter of convenience.When N s=N,the between-groups and OLS estimators are identical.If N s/N t→1∀s,t,then OLS converges to the between-groups estimator.Therefore,it is possible to calculate a corrected standard error for the OLS estimate and generate a t-statistic that has a t-distribution.The between-groups estimator is, however,much more convenient.2.2Variables that Vary Within-GroupWe consider now hybrid models in which some variables differ across observations within groups.For simplicity we ignore complications associated with nonconstant correlation of the group effect since they do not add to the analysis.As was seen in the discussion without within-group varying variables,all that is important is the variance of the mean group error.Thus we analyze the standard Moulton modelY is=a+X sβ+Z isγ+αs+εis.(15)We assume for simplicity that Y is and X s are scalars.The extension to the case of more than one group-varying or group variable is straightforward.We further assume thatαs∼N(0,σ2α)for all sεis∼N(0,σ2ε)for all i,sand that these residuals are mutually independent for all i and s.As before we know that GLS estimation of(15)is efficient and that if we can obtain consistent estimates ofσ2αandσ2εfeasible GLS estimation is asymptotically efficient.Finally,we know from Amemiya that if these variance terms are estimated in the same way that feasible GLS estimation is numerically identical to the following estimator—first use OLS to estimate the“within-group”estimate ofγY is=d s+Z isγ+εis.(16) Then estimateβby feasible GLS estimation of¯Ys−¯Z0sˆγ=b d s=a+βX s+u s(17) where,¯Y=P N s i=1Y is s,¯Z s=P N s i=1Z is s,sb d s is the estimate of d s in(16)and var(u)=σ2αI+Σe dwhereΣe d is the covariance matrix of thefixed-effect parameter estimates.Note that estimates ofΣe d can be obtained by selecting the covariance matrix corresponding to the fixed effects.σ2a can be estimated byfirst estimating(17)by OLS and then usingP S i=1b u2i−1S X i=1var(b d i)=bσ2awhere var(b d i)is the variance of the i thfixed effect and K is the number of explanatory variables in(17). Finally,it is worth noting that since the groups are distinct,covariance of the b d0s arises only because γis estimated rather than known.If each bγi is calculated from a separate sample,the covariances will be zero.Thus the covariance problem can be avoided by estimatingγfor the s th state asˆγ(s)=(Z0s M s Z s)−1Z0s M s Y s(18) whereM s=I Ns−ιN s(ι0N sιN s)−1ι0N sis a vector of10s of length N s.andιNsSinceˆγW involves the restriction thatγis constant across states,it is more efficient but less robust.7 Our focus,however,is not on estimation ofγbut ofβ.When the number of groups is large,it is possible to estimate(17)by feasible GLS.If the number of groups is small,then we can still get efficient estimates and determine the distribution of the t-statistic if the error term in(17)is i.i.d.normal except possibly for an error term common to all groups.The following propositions summarize conditions under which this condition holds and the resulting t-statistic has the t-distribution.Let b T1be the t-statistic using bγw and b T2be the t-statistic using bγ(s).Proposition1:If theεis are normally distributed,(i)ˆT1∼t(S−2)when N s are identical for all s and either(a)there are no Z is or(b)¯Z s is constantacross s(ii)ˆT2∼t(S−2)when N s are identical for all s and either(a)there are no Z is or(b)¯Z0s(Z0s M s Z s)−1¯Z s is constant across sWe can also show that the statistics will have asymptotic t(S−2)distributions under more general situations so that the t(S−2)distribution can be used quite generally.Proposition2:If theεis are not normally distributed and ifσ2αisfixed,thenˆT j A∼t(S−2)(for j=1,2)when N s→∞for all s.As in the case where there are no individual specific covariates one can show that the statisticsˆT j will be approximately t(S−2)when the group specific errors are small relative to the idiosyncratic errors.The conditions that give rise to this are essentially asymptotic analogs of the conditions in Proposition 1.Proposition3:Ifσ2αis small in the sense thatσ2α=O(N−1/2s)then regardless of the distribution of εis(i)ˆT1A∼t(S−2)when N s are asymptotically identical8for all s and either(a)there are no Z is or(b)p lim¯Z s is constant across s(ii)ˆT2A∼t(S−2)when N s are asymptotically identical for all s and either(a)there are no Z is or(b) p lim¯Z0s(Z0s M s Z s/N s)−1¯Z s is constant across sThese situations can be stated with reference to the residuals in the second-stage equation:¯Ys−¯Z0sˆγ=a+βX s+αs+εs+¯Z0s(γ−ˆγ).(19) For the distribution of the t-statistic to be exactly t,we require that the error term be normally distributed and i.i.d.except possibly for a common component for all observations.When all groups have the same sample size,we can readily check whether:¯Zis identical across groups,in the case where we use the within estimator to obtain bγw,or svar(bγ(s))is identical for all groups,in the case whereγis estimated separately for each group.The t(S−2)can be justified as an approximation based on large N s asymptotics under more general conditions.This occurs because the error term in(19)converges to the homoskedastic normal errorαs as N s→∞because of the consistency ofˆγand the fact thatεs p→0by the usual Law of Large Numbers. Whenσ2αis small,as in Proposition3,the approximation can be justified because¯εs and¯Z0s(γ−ˆγ) are approximately normally distributed by the Central Limit Theorem so that the error term in(19)is approximately normal.While the theory above and the Monte Carlo evidence below suggest that using one of the two-stage estimators will generally be preferable to using OLS,there are two caveats which must be recognized. First,whenσ2α=0,OLS is the efficient estimator of equation(15)and the t-statistic has the conventional distribution.If one knows thatσ2α=0,OLS is the preferred estimator.What Proposition3tells us is that if we proceed under the mistaken belief thatσ2α>0,two-stage estimation will still produce a statistic with a t-distribution.Second,the distinction between two-stage estimation and one-stage estimation is really one of con-venience.Whenever b T1has a t-distribution,the same statistic can be produced by relying on the OLS coefficients.For example,in the case where there are no group-varying covariates and each group has the same number of observations,the OLS coefficient is identical to the between-groups(two-step)estima-tor.However,it is more convenient to estimate the standard error of the estimate using between-group estimation.Similarly,it is easy to show that when¯Z s is identical across groups and all groups have the same sample size,OLS produces the same bβas in the case where we use the within estimator to obtain bγw and estimateβin two steps.Again,however,estimation of the correct standard error is much easier using the two-step estimator.3ExamplesIn this section,wefirst review the two by two case,which features prominently in the literature.The main feature of this case is that we cannot calculate the standard error of the estimate and thus must exercise considerable caution in drawing conclusions.We then review two prominent papers that provide at least some differences-in-differences estimates in which there are no covariates that vary within group. Thefirst case,Gruber and Poterba(1994),shows that accounting properly for error components can dramatically reduce the implied precision of the estimates in some specifications but that the estimate remains precise in at least one specification.In the second case we reexamine Card’s(1990)Mariel boatlift study and suggest that the data cannot exclude large effects of the migration on blacks in Miami.This is consistent with the results of Angrist and Krueger’s(1999)finding of a large impact of the“Mariel boatlift that didn’t happen.”3.1The Two by Two CaseIn the canonical differences-in-differences model,mean outcomes are calculated for groups A(the treat-ment group)and B(the control group)in each of periods0(the pre-treatment period)and1(the post-treatment period).A standard table shows each of these means,plus the difference between groups A and B in each period and the difference between the pre-and post-treatment outcomes for each group.Finally the difference between either pair of differences is the classic differences-in-differences estimate.Classic and recent examples that include tables in this form are Card and Krueger’s study of the minimum wage(1994),Eissa and Leibman’s study of the effect of the earned-income tax credit (1996),Meyer et al’s study of workers’compensation(1995),Imbens et al’s study of lottery winners andlabor supply(2001),Eberts et al’s study of merit pay for teachers(2002)and Finkelstein’s(2002)study of tax subsidies and health insurance provision.Each of these studies provides additional analysis,but in each case,the two by two analysis is an important component of the study.In a well developed two by two case,the authors make a compelling case that other than the treat-ment,there is no reason to expect the outcome variable to evolve differently for the treatment and control groups.The statistical model isy igt=αgt+bT gt+εigt(20)where y is the outcome being measured for individual i in group g in year t,T is a dummy variable for the treatment group in the post-treatment period.αis a group/year error which may be correlated over time or across groups andεis an i.i.d.error term.Without loss of generality,we can subtract thefirst period from the second period and rewrite the equation as∆y ig=∆a g+b∆T g+εig1−εig0.(21) Now∆T equals1for the treatment group and0for the control group.Therefore we can replace∆T with A,a dummy variable for group A.And we can rewrite∆a g=c+eαA,where eα=∆αA−∆αB. Lettingεig2−εig1=εig,we have∆y ig=c+(eα+b)A g+εig.(22)The weighted least squares(where weights are chosen to make the sample sizes identical)coefficient on A is the differences-in-differences estimator and is numerically identical to taking the difference between the change in the outcome for the treatment and control groups.It is an unbiased estimate of eα+b. Since E(eα)=0,it is also an unbiased estimate of b.However,it is not consistent.No matter how many observations from either the control or treatment groups we add to the sample,the coefficient will not converge in probability to b.The variance reported by econometric packages includes the sampling variance but not that part of the variance due to the common error.Thus if there are any shocks that are correlated within year/group cells,the reported t-statistic will be too high.We will tend tofind an effect of the treatment even if none exists.Unfortunately,if there are common errors,the two by two model has zero degrees of freedom.Therefore it is not possible to determine the significance of any estimate solely from within-sample information.It may be possible to use information from outside the sample to get a plausible estimate of the magnitude of common within-group errors,but even in this case,we will not know the sampling distribution of the resulting statistic.Thus analysis of the two by two case requires extreme caution.3.2Card(1990)Card examines the impact of the mass migration of Cubans to Miami during the Mariel boatlift.He compares,among other outcomes,unemployment rates for whites,blacks and Hispanics in Miami with unemployment rates of these groups in four comparison cities(Atlanta,Houston,Los Angeles and Tampa-St.Petersburg).Surprisingly,hefinds little evidence that the mass migration significantly affected the Miami labor market.For example,from1979to1981black unemployment in Miami increased by1.3 percentage points compared with2.6percentage points in the comparison communities.Angrist and Krueger(1999)replicate Card’s study for a Cuban boatlift that was anticipated but did not occur.They find that“the Mariel boatlift that didn’t happen”had a large adverse effect on unemployment in Miami. Their analysis cast doubt on the power of Card’s originalfinding.Our analysis helps to explain why Card found no effect and why it is possible tofind a large effect of a nonexistent event.To understand this,we need to examine the true confidence interval around Card’s estimates.Because Card provides seven years of data for both Miami and the comparison cities,we can, with auxiliary assumptions,calculate the variance of his estimate.Wefirst assume that the difference between the annual unemployment rates in Miami and the com-parison cities is subject to an i.i.d.shock.This allows for a common year shock which may be persistent but assumes that any shocks that are idiosyncratic to a city are not persistent.Given this assumption, we use the data reported by Card to regress the difference between the unemployment rate for blacks in Miami and the control cities on a dummy for the period after1980on all years except1980.The resulting coefficient is1.4with a standard error of4.0.Under the assumption that the error terms are homoskedastic and normal,and given that we have four degrees of freedom,the confidence interval is from-9.7to12.1,effectively including very large positive and negative impacts on blacks.In sum,while the data certainly provide no support for the view that the Mariel immigration dra-matically increased unemployment among blacks in Miami,they do not provide much evidence against this view either.In this case,the differences-in-differences approach lacks power.。
福建4个家鸭品种的分子遗传多样性
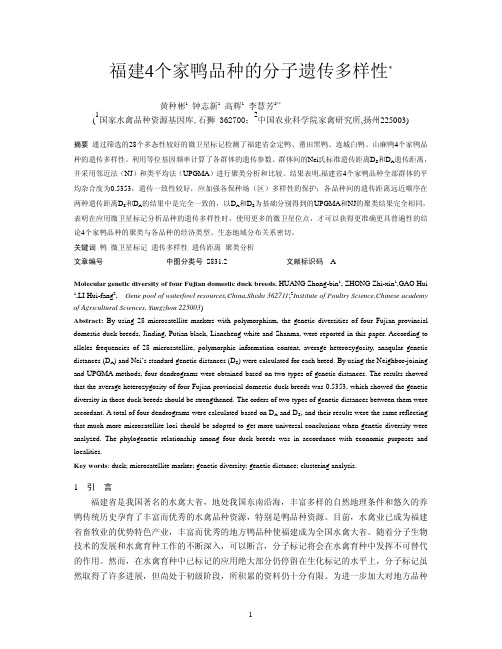
福建4个家鸭品种的分子遗传多样性*黄种彬1钟志新1高辉1李慧芳2**(1国家水禽品种资源基因库,石狮 362700;2中国农业科学院家禽研究所,扬州225003)摘要通过筛选的28个多态性较好的微卫星标记检测了福建省金定鸭、莆田黑鸭、连城白鸭、山麻鸭4个家鸭品种的遗传多样性。
利用等位基因频率计算了各群体的遗传参数、群体间的Nei氏标准遗传距离D S和D A遗传距离,并采用邻近法(NJ)和类平均法(UPGMA)进行聚类分析和比较。
结果表明,福建省4个家鸭品种全部群体的平均杂合度为0.5353,遗传一致性较好,应加强各保种场(区)多样性的保护;各品种间的遗传距离远近顺序在两种遗传距离D S和D A的结果中是完全一致的,以D A和D S为基础分别得到的UPGMA和NJ的聚类结果完全相同,表明在应用微卫星标记分析品种的遗传多样性时,使用更多的微卫星位点,才可以获得更准确更具普遍性的结论4个家鸭品种的聚类与各品种的经济类型、生态地域分布关系密切。
关键词鸭微卫星标记遗传多样性遗传距离聚类分析文章编号中图分类号S831.2文献标识码 AMolecular genetic diversity of four Fujian domestic duck breeds. HUANG Zhong-bin1, ZHONG Zhi-xin1,GAO Hui 1,LI Hui-fang2, Gene pool of waterfowl resources,China,Shishi 362711;2Institute of Poultry Science,Chinese academy of Agricultural Sciences, Yangzhou 225003)Abstract: By using 28 microsatellite markers with polymorphism, the genetic diversities of four Fujian provincial domestic duck breeds, Jinding, Putian black, Liancheng white and Shanma, were reported in this paper. According to alleles frequencies of 28 microsatellite, polymorphic information content, average heterozygosity, anaqular genetic distances (D A) and Nei’s standard genetic distances (D S) were calculated for each breed. By using the Neighbor-joining and UPGMA methods, four dendrograms were obtained based on two types of genetic distances. The results showed that the average heterozygosity of four Fujian provincial domestic duck breeds was 0.5353, which showed the genetic diversity in those duck breeds should be strengthened. The orders of two types of genetic distances between them were accordant. A total of four dendrograms were calculated based on D A and D S, and their results were the same reflecting that much more microsatellite loci should be adopted to get more universal conclusions when genetic diversity were analyzed. The phylogenetic relationship among four duck breeds was in accordance with economic purposes and localities.Key words:duck; microsatellite marker; genetic diversity; genetic distance; clustering analysis.1 引言福建省是我国著名的水禽大省,地处我国东南沿海,丰富多样的自然地理条件和悠久的养鸭传统历史孕育了丰富而优秀的水禽品种资源,特别是鸭品种资源。
遗传多样性的分类和评估方法研究
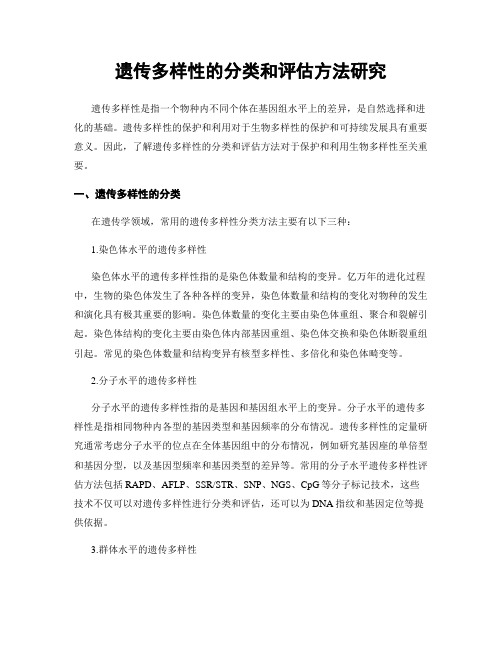
遗传多样性的分类和评估方法研究遗传多样性是指一个物种内不同个体在基因组水平上的差异,是自然选择和进化的基础。
遗传多样性的保护和利用对于生物多样性的保护和可持续发展具有重要意义。
因此,了解遗传多样性的分类和评估方法对于保护和利用生物多样性至关重要。
一、遗传多样性的分类在遗传学领域,常用的遗传多样性分类方法主要有以下三种:1.染色体水平的遗传多样性染色体水平的遗传多样性指的是染色体数量和结构的变异。
亿万年的进化过程中,生物的染色体发生了各种各样的变异,染色体数量和结构的变化对物种的发生和演化具有极其重要的影响。
染色体数量的变化主要由染色体重组、聚合和裂解引起。
染色体结构的变化主要由染色体内部基因重组、染色体交换和染色体断裂重组引起。
常见的染色体数量和结构变异有核型多样性、多倍化和染色体畸变等。
2.分子水平的遗传多样性分子水平的遗传多样性指的是基因和基因组水平上的变异。
分子水平的遗传多样性是指相同物种内各型的基因类型和基因频率的分布情况。
遗传多样性的定量研究通常考虑分子水平的位点在全体基因组中的分布情况,例如研究基因座的单倍型和基因分型,以及基因型频率和基因类型的差异等。
常用的分子水平遗传多样性评估方法包括RAPD、AFLP、SSR/STR、SNP、NGS、CpG等分子标记技术,这些技术不仅可以对遗传多样性进行分类和评估,还可以为DNA指纹和基因定位等提供依据。
3.群体水平的遗传多样性群体水平的遗传多样性是指某一物种内不同个体间的遗传多样性差异。
在遗传多样性评估中,常通过测量不同基因型间的遗传距离来反映群体水平的遗传多样性。
常用的遗传距离包括匀性指数、F统计量、Mantel-样本关联系数等,其中最常使用的距离是匀性指数(Nei's standard genetic distance)。
二、遗传多样性的评估方法遗传多样性的评估方法应该考虑不同的分类方法,和不同的评估指标及其作用。
组合使用染色体、分子及其群体水平的评估指标,可以建立遗传多样性框架图,进一步研究遗传多样性的演化和单倍型组成情况。
今日标准(16)
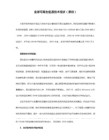
Perls and his colleagues publish their study in the online edition of the journal Science.
The Boston University researcher says this kind of analysis could play a role, not just in predicting who will live longest, but in actually helping people live longer and healthier lives.
So it's pretty clear genetics plays some role in longevity.
In this study, the research team developed a new statistical way of analyzing the genetic code of people who had reached age 100 as compared with people who had a more typical lifespan. Tom Perls, who heads the New England Centenarian Study, explains what they found.
Perls says the key to successfully predicting long life was the sophisticated statistical analysis of many different gene variations that each played some role.
mmod用户指南说明书
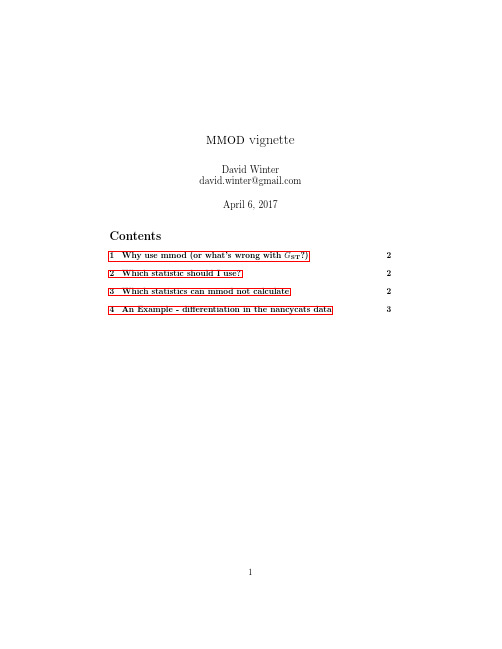
mmod vignetteDavid Winter**********************April6,2017Contents1Why use mmod(or what’s wrong with G ST?)2 2Which statistic should I use?2 3Which statistics can mmod not calculate2 4An Example-differentiation in the nancycats data311Why use mmod(or what’s wrong with G ST?) Population geneticists,molecular ecologists and evolutionary biologists often want to be able to determine the degree to which populations are divided into smaller sub-populations.One very widely used approach to this question uses “F analogues”(measures based on Wrigtht’s F ST)to compare diversity within and between predefined sub-populations.Until recently,the most widely used of these statistics has been Nei’s G ST.Unfortunately,the value of G ST is a at least partially dependent on the number of alleles at each locus and the number of populations sampled.This makes simple interpretations of G ST difficult, and comparisons between studies(or even between loci in the same population) potentially misleading.A number of new F ST analogues have been developed that compensate for these short comings,and give values that can be compared between studies.mmodis a package that allows three of these statistics,GST ,D est andϕST,to becalculated from genind objects(the standard representation of genetic datasets in the adegenet library)2Which statistic should I use?With the proliferation of F ST analogues,it can be hard to decide on the most appropriate measure to use for your study.I encourage you to read Meirmans and Hedrick(2011doi:10.1111/j.1755-0998.2010.02927.x),which includes a dis-cussion on this topic.As you’ll see in the demonstration below,the correctedstatistics often tell a similar story.Interestingly,GST can be directly related tothe rate of migration between populations while D est andϕST are about parti-tioning distances or diversity between genes.You may consider which approach is most appropriate for the specific questions you wish to ask.3Which statistics can mmod not calculateThere are at least two population genetic statistics related to the ones discussed above that mmod can’t calculate.R ST was developed for micorsattelite data, and takes the relationship between alleles(and therefore the mutation rate) into account when measuring between-allele distances.It is not clear how the maximum potential value of R ST for a given dataset can be calculated,so it isnot possible to correct this statistic in a way similar to GST andϕST.Similarly,the calculation of the maximum value of Weir and Cockerham’sθis complex(and not yet published).If you wish to calculate a corrected ver-sion of this statistic you can use RecodeData(http://www.bentleydrummer. nl/software/software/)to create a dataset in which all between-population2differences are maximised.You can then calculateθfor each dataset using Fstfrom the package pegas.If the statistic calculated form the recoded data is.θmax then the corrected statistic is simplyθθmax4An Example-differentiation in the nancycats dataWith the description out of the way,let’s see how these functions work in prac-tice.As an example,we are going to examine the nancycats data that comeswith adegenet.This dataset contains microsattelite genotypes taken from feralcats in Nancy,France.So let’s start.>library(mmod)>data(nancycats)>nancycats///GENIND OBJECT///////////237individuals;9loci;108alleles;size:145.3Kb//Basic content@tab:237x108matrix of allele counts@loc.n.all:number of alleles per locus(range:8-18)@loc.fac:locus factor for the108columns of@tab@s:list of allele names for each locus@ploidy:ploidy of each individual(range:2-2)@type:codom@call:genind(tab=truenames(nancycats)$tab,pop=truenames(nancycats)$pop)//Optional content@pop:population of each individual(group size range:9-23)@other:a list containing:xyThe nancycats data comes in adegenet’s default class for genotypic data,thegenind class.The functions in mmod work on genind objects,so you would usu-ally start by reading in your data using read.genpop or read.fstat dependingon the format it’s in.Now that we have our data on hand,our goal is to see•Whether this population is substantially differentiated into smaller sub-populations•Whether such differentiation can be explained by the geographical distancebetween sub-populations.3We can look at several statistics to ask answer thefirst question by using the diff_stats()function:>diff_stats(nancycats)$per.locusHs Ht Gst Gprime_st Dfca80.77400440.86161800.101684930.47504450.41190817fca230.74151020.79926210.072256500.29566880.23738411fca430.74167960.79351200.065320170.26757660.21319208fca450.70855540.76422480.072844220.26531630.20374594fca770.77663690.86556180.102736700.48558290.42300076fca780.63162020.67720450.067312450.19333270.13147655fca900.73695870.81415910.094822210.38075780.31183460fca960.67256000.76560830.121535070.39139240.30192942fca370.56232590.60243540.066578940.16095760.09737005$globalHs Ht Gst_est Gprime_st D_het D_mean 0.705094590.771509530.086084410.308489480.239283100.20931242 OK,so what is that telling us?Thefirst table has statistics calculated individu-ally for each locus in the dataset.Hs and Ht are estimates of the heterozygosity expected for this population with and without the sub-populations defined in the nancycats data respectively.We need to use those to calculate the mea-sures of population divergence so we might as well display them at the sametime.Gst is the standard(Nei)G ST,Gprime_st is Hedrick’s GST and D is Jost’sD est.Because all of these statistics are estimated from estimators of H S and H T,it’s possible to get negative values for each of these differentiation measures. Populations can’t be negatively differentiated,so you should think of these as estimates of a number close to zero(it’s up to you and your reviewers to decide if you report the negative numbers of just zeros).D est is the easiest statistic to interpret,as you expect tofind D=0for popu-lations with no differentiation and D=1for completely differentiated popula-tions.As you can see,different loci give quite different estimates of divergence but they range from∼0.1–0.4.mmod can calculate another statistic of differentiation calledϕ ST.This statistic is based on the Analysis of Molecular Variance(AMOVA)method,which par-titions the variance in genetic distances in a dataset to among-population and within-population components(it is possible to use this framework to partition variance using more than two levels of population structure,but that has notbeen implemented in mmod yet).BecauseϕST can take some time to calcu-late it’s not included in diff_stat by default(but you can include it using diff_stat(x,phi_st=TRUE)).You might want to see how all these different measures compare to each other across the loci we’ve looked at.You can see the corrected measures(all those4Gst0.150.250.350.450.250.350.450.070.090.110.150.250.350.45Gprime_stD0.100.200.300.400.070.090.110.250.350.450.100.200.300.40Phi_stFigure 1:Comparison of differentiation measuresother than G ST )show a similar pattern,and G ST is a bit strange (Figure 1):>nc.diff_stats <-diff_stats(nancycats,phi_st=TRUE)>with(nc.diff_stats,pairs(per.locus[,3:6],upper.panel=panel.smooth))The second part of the list returned by diff_stat contains global estimates of each of these statistics.For G ST and G ST these are based on the average of Hs and Ht across loci.For D est you get two,the harmonic mean of the D est for each locus and,because that method won’t work if you end up with negative estimates of D est ,one calculated as per G ST and G ST .The global estimate of ϕ ST is calculated from the average distance among individuals across all loci.Now that we have a point-estimate for how differentiated these populations are we will want to have some idea of how robust this result is.mmod has a few functions for performing bootstrap samples of genind objects and calculating statistics from those samples.Because some of these functions can take a long time to run,we will create a very small (10repetition)bootstrap sample of the nancycats data,then calculate D est from that sample:>bs <-chao_bootstrap(nancycats,nreps=10)>bs.D <-summarise_bootstrap(bs,D_Jost)5>bs.DEstimates for each locusLocus Mean95%CIfca80.4119(0.339-0.485)fca230.2374(0.153-0.321)fca430.2132(0.165-0.262)fca450.2037(0.148-0.259)fca770.4230(0.366-0.480)fca780.1315(0.045-0.218)fca900.3118(0.259-0.365)fca960.3019(0.228-0.376)fca370.0974(0.063-0.132)Global Estimate based on average heterozygosity0.2393(0.214-0.264)Global Estimate based on harmonic mean of statistic0.2093(0.177-0.242)As you can see,printing a summarised bootstrap sample gives us shows a basic overview of that data.In this case the confidence intervals are calculated using the“normal method”,which it to say the the intervals are the observed value statistic+/-1.96x the standard error of the boostrap sample.There is more to these objects than gets printed—use str(bs.D)to check it out.I don’t think there is much point trying to interpret confidence intervals estimated from10samples,but the point estimates seem to show a population with some substantial differentiation.Next,we want to know if geography can explain that differentiation.The nan-cycats data comes with coordinates for each population.We can use these to get Euclidean distances:>head(nancycats@other$xy,4)x yP01263.3498171.10939P02183.5028122.40790P03391.1050254.70148P04458.612141.72336>nc.pop_dists<-dist(nancycats@other$xy,method="euclidean")mmod provides functions to calculate pairwise versions of each of the differen-tiation statistics.Because we want to perform a Mantel test,we’ll use the “linearized”version of D est,which is just x/(1−x)(each of the pairwise stats has and argument to return this version).>nc.pw_D<-pairwise_D(nancycats,linearized=TRUE)6The library ade4,which is loaded with mmod,provides functions to perform Mantel tests on distance matrices.>mantel.rtest(nc.pw_D,log(nc.pop_dists),999)Monte-Carlo testCall:mantelnoneuclid(m1=m1,m2=m2,nrepet=nrepet) Observation:-0.02584796Based on999replicatesSimulated p-value:0.594Alternative hypothesis:greaterStd.Obs Expectation Variance-0.2756103159-0.00089145320.0081992989So,the geographic distance between these populations can’t explain the genetic divergences we see:the correlation is small and non-significant.If you like,we can also visualize this relationship(Figure2).>fit<-lm(as.vector(nc.pw_D)~as.vector(nc.pop_dists))>plot(as.vector(nc.pop_dists),as.vector(nc.pw_D),+ylab="pairwise D",xlab="physical distance")>abline(fit)There are a couple of other functions that are not used here,and a few of use the functions we have used have help messages that guide interpretation of their ressults-use help(package="mmod")to see the full documentation.7q q q q q q q qq q q q q q q qq qq q q qq q qqqq qq q qq qqq q qq q q qq q qqq qqq q qqqqq qq q qq qq q q q q q q q qq qq q qq qq q q q qqqqqqq q q qq q q q q q q q qq q qq q qqq q q qqqq q qqq q qq qq q q q qq qq q q q q q501001502002503003500.10.20.30.40.50.6physical distancep a i r w i s e DFigure 2:Geographic distance does not explain genetic differentiation8。
桉树4个种遗传多样性的ISSR分析
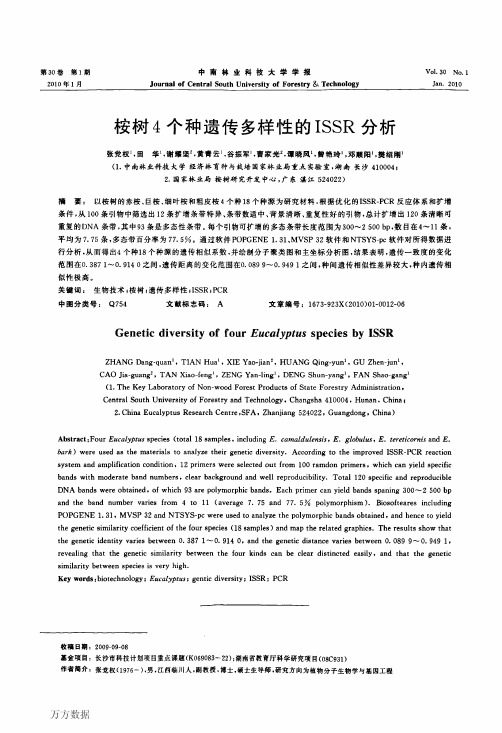
万方数据 万方数据14中南林业科技大学学报第30卷物,然后再对不同的桉树基因组DNA进行全面有效的扩增,以保证实验结果的可靠、科学和高效。
随机选取3个不同的种源,用于筛选全部100条ISSR引物。
1.5ISSR—PCR扩增及数据处理采用筛选出的引物对桉树4个种的模板DNA进行ISSR—PCR扩增。
按照相同迁移位置上有扩增条带记为“l”、无则记为“0”的方法记录每个引物的电泳谱带,且仅记录清晰、重复性好的扩增条带,并将“0”、“1”数据输入Excel表格中用于下一步分析。
应用POPGENE软件计算Nei’S遗传距离和遗传一致度,绘制遗传关系进化树。
最后统计数据,应用软件POPGENE、MVSP32软件以及NTSYS—pc软件对所得数据进行总结分析,并绘制相关图形。
2结果与分析2.1桉树基因组DNA提取及质量检测本实验对18个桉树幼苗进行了基因组DNA抽提,通过纯化后最终用TE缓冲液定容至100pL,并电泳检测(见图1)。
结果表明,所提取的DNA条带清晰,无明显拖带,提取效果较好。
通过DUO一640核酸蛋白分析仪测定分析,所提取的18个桉树幼苗叶片DNA样品的浓度为20~70ng/uL,且纯度R(oD:。
/0D:。
)值在1.7~1.8之间(见表1),表明样品中蛋白质和RNA含量较少,浓度和纯度都满足特异PCR扩增的要求。
为了统一反应体系,最后将18个种源根据测得的浓度相应地稀释成终浓度10ng//.tL。
图1桉树18个样品的基因组DNA的电泳图Fig.1TheeIectrophoresispatternofgenomicDNAfrom18speciesofEucalyptus2.2桉树ISSR—PCR扩增结果利用已经优化的桉树ISSR—PCR扩增体系,对100条合成的UBC随机引物进行筛选,获得12条适合18个桉树样品ISSR扩增反应的引物,编号分别为811、814、818、825、826、827、828、850、864、88l、895、900,该12条引物的扩增条带特异,条带数适中,背景清晰,重复性好(见图2)。
基于荧光SSR_标记的紫薇遗传多样性分析
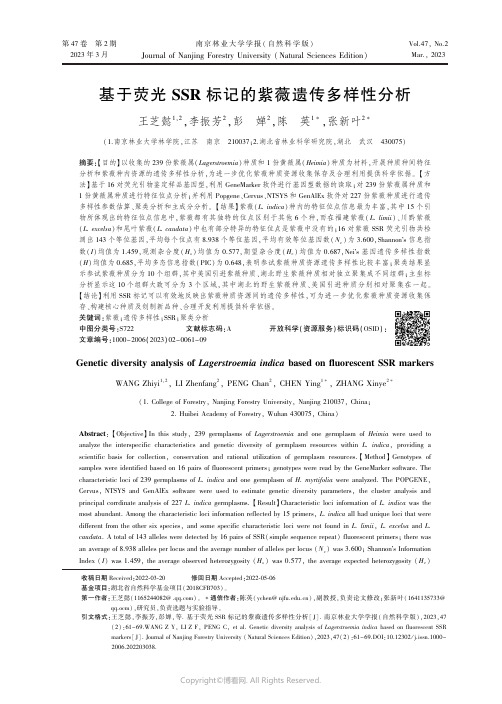
samples, a genetic cluster map was drawn and the samples were divided into 10 groups. The L. indica germplasm
analyze the interspecific characteristics and genetic diversity of germplasm resources within L. indica, providing a
scientific basis for collection, conservation and rational utilization of germplasm resources. 【 Method 】 Genotypes of
分析和紫薇种内资源的遗传多样性分析,为进一步优化紫薇种质资源收集保存及合理利用提供科学依据。 【 方
法】 基于 16 对荧光引物鉴定样品基因型,利用 GeneMarker 软件进行基因型数据的读取;对 239 份紫薇属种质和
1 份黄薇属种质进行特征位点分析;并利用 Popgene、Cervus、NTSYS 和 GenAlEx 软件对 227 份紫薇种质进行遗传
存、构建核心种质及创制新品种、合理开发利用提供科学依据。
关键词:紫薇;遗传多样性;SSR;聚类分析
中图分类号:S722 文献标志码:A
文章编号:1000-2006(2023)02-0061-09
开放科学( 资源服务) 标识码( OSID) :
Roughening of close-packed singular surfaces
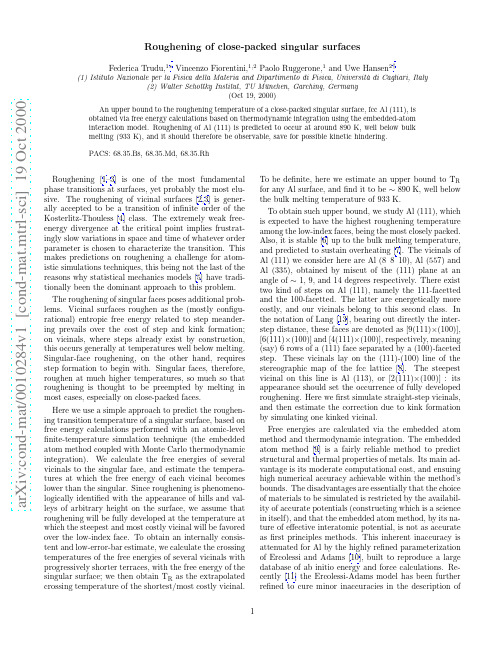
a r X i v :c o n d -m a t /0010284v 1 [c o n d -m a t .m t r l -s c i ] 19 O c t 2000Roughening of close-packed singular surfacesFederica Trudu,1∗Vincenzo Fiorentini,1,2Paolo Ruggerone,1and Uwe Hansen 2†(1)Istituto Nazionale per la Fisica della Materia and Dipartimento di Fisica,Universit`a di Cagliari,Italy(2)Walter Schottky Institut,TU M¨u nchen,Garching,Germany(Oct 19,2000)An upper bound to the roughening temperature of a close-packed singular surface,fcc Al (111),is obtained via free energy calculations based on thermodynamic integration using the embedded-atom interaction model.Roughening of Al (111)is predicted to occur at around 890K,well below bulk melting (933K),and it should therefore be observable,save for possible kinetic hindering.PACS:68.35.Bs,68.35.Md,68.35.RhRoughening [1–3]is one of the most fundamental phase transitions at surfaces,yet probably the most elu-sive.The roughening of vicinal surfaces [2,3]is gener-ally accepted to be a transition of infinite order of the Kosterlitz-Thouless [4]class.The extremely weak free-energy divergence at the critical point implies frustrat-ingly slow variations in space and time of whatever order parameter is chosen to characterize the transition.This makes predictions on roughening a challenge for atom-istic simulations techniques,this being not the last of the reasons why statistical mechanics models [5]have tradi-tionally been the dominant approach to this problem.The roughening of singular faces poses additional prob-lems.Vicinal surfaces roughen as the (mostly configu-rational)entropic free energy related to step meander-ing prevails over the cost of step and kink formation;on vicinals,where steps already exist by construction,this occurs generally at temperatures well below melting.Singular-face roughening,on the other hand,requires step formation to begin with.Singular faces,therefore,roughen at much higher temperatures,so much so that roughening is thought to be preempted by melting in most cases,especially on close-packed faces.Here we use a simple approach to predict the roughen-ing transition temperature of a singular surface,based on free energy calculations performed with an atomic-level finite-temperature simulation technique (the embedded atom method coupled with Monte Carlo thermodynamic integration).We calculate the free energies of several vicinals to the singular face,and estimate the tempera-tures at which the free energy of each vicinal becomes lower than the singular.Since roughening is phenomeno-logically identified with the appearance of hills and val-leys of arbitrary height on the surface,we assume that roughening will be fully developed at the temperature at which the steepest and most costly vicinal will be favored over the low-index face.To obtain an internally consis-tent and low-error-bar estimate,we calculate the crossing temperatures of the free energies of several vicinals with progressively shorter terraces,with the free energy of the singular surface;we then obtain T R as the extrapolated crossing temperature of the shortest/most costly vicinal.To be definite,here we estimate an upper bound to T R for any Al surface,and find it to be ∼890K,well below the bulk melting temperature of 933K.To obtain such upper bound,we study Al (111),which is expected to have the highest roughening temperature among the low-index faces,being the most closely packed.Also,it is stable [6]up to the bulk melting temperature,and predicted to sustain overheating [7].The vicinals of Al (111)we consider here are Al (8810),Al (557)and Al (335),obtained by miscut of the (111)plane at an angle of ∼1,9,and 14degrees respectively.There exist two kind of steps on Al (111),namely the 111-facetted and the 100-facetted.The latter are energetically more costly,and our vicinals belong to this second class.In the notation of Lang [13],bearing out directly the inter-step distance,these faces are denoted as [9(111)×(100)],[6(111)×(100)]and [4(111)×(100)],respectively,meaning (say)6rows of a (111)face separated by a (100)-faceted step.These vicinals lay on the (111)-(100)line of the stereographic map of the fcc lattice [8].The steepest vicinal on this line is Al (113),or [2(111)×(100)]:its appearance should set the occurrence of fully developed roughening.Here we first simulate straight-step vicinals,and then estimate the correction due to kink formation by simulating one kinked vicinal.Free energies are calculated via the embedded atom method and thermodynamic integration.The embedded atom method [9]is a fairly reliable method to predict structural and thermal properties of metals.Its main ad-vantage is its moderate computational cost,and ensuing high numerical accuracy achievable within the method’s bounds.The disadvantages are essentially that the choice of materials to be simulated is restricted by the availabil-ity of accurate potentials (constructing which is a science in itself),and that the embedded atom method,by its na-ture of effective interatomic potential,is not as accurate as first principles methods.This inherent inaccuracy is attenuated for Al by the highly refined parameterization of Ercolessi and Adams [10],built to reproduce a large database of ab initio energy and force calculations.Re-cently [11]the Ercolessi-Adams model has been further refined to cure minor inaccuracies in the description of1surface diffusion and high-energy scattering. Thermodynamic integration is adopted because the roughening transition occurs(if at all)well above the De-bye temperature(∼400K for Al bulk),and it is therefore imperative to properly include anharmonic effects in the free energy of the relevant surfaces.While useful at lower temperatures,the commonly adopted quasi-harmonic ap-proximation is not very reliable at high temperature,as shown by recent simulations[11]on Al(100).In ther-modynamic integration[12],the potential energy of the system is progressively switched on,through a parameter λ,starting from a reference system whose free energy is known:V(λ)=λW−(1−λ)U h(1) with W and U h the potentials of the actual system and of an harmonic crystal.Since[12],∂Fd T F T2(4) the free energy in the interval[T ref,T]isF(T)=T F ref T2dT .(5)The integrand is calculated again by canonical Monte Carlo.The surface free energy per unit area is1F surf(T)=transition.46810121416182022Interstep distance (A)750770790810830850870890910930C r o s s i n g t e m p e r a t u r e (K)FIG.2.Crossing points of surface free energies of vici-nals and singular surface.Upper curve:straight steps;lower curve:kinked steps.T R is defined as the temperature corre-sponding to the Al (113)interstepdistance.FIG.3.Top view of kinked Al (557)as studied in free en-ergy calculation.As all other cells,it contains two periodi-cally-repeated steps per side.The simulation of vicinal surfaces with kinks is de-manding in periodic boundary conditions;here we re-strict to a single case,kinked Al (557),chosen because of its favorable geometry.Each side of the simulation slab,depicted in Fig.3,contains one straight and one kinked step.The latter exhibits two kinks,with a rel-atively low linear density of 0.05˚A −1.The number of atoms is preserved by this procedure,as required by nu-merical considerations.As shown in Fig.4,the kinked Al (557)turns out to have a crossing point with Al (111)at T=845K,with a reduction of 4%over the straight-step value.Assuming that the other crossing points are lowered by about the same amount due to kinks,and ap-plying the same procedure as before,we find T R =887K (lower-laying curve in Fig.2).This is a strong upper bound because accounting for lower-cost (111)-faceted steps should lower this figure.In addition,account for meandering will also lower (moderately)our estimate.Roughening has not been reported for any (111)face so far.The predicted T R is rather close to,but lower than the melting temperature,so it is quite conceivable that roughening of Al (111)could be observed.Our pre-diction concerns energetics,however.Kinetic effects are not considered in any way.However,Al (111)was ob-served [6]in Medium Energy Ion Scattering experiments to remain stable up to the melting temperature.Also,molecular dynamics simulations [7]showed Al (111)to be stable for at least 2ns up to 1088K,or 150K above bulk melting.While the length and time scales accessible in simulation are not comparable with those of relevance in roughening,this is an indication that kinetics may play a role,slowing down or hindering the transforma-tion.Thus,it is possible that experiments aiming at the observation of the roughening of Al (111)predicted here,may have to observe the surface over time spans of hours,or produce “nucleation”defects by e.g.nanoindentation.830840850860870880890Temperature (K)3839404142S u r f a c e f r e e e n e r g y (m e V /A 2)FIG.4.Lowering of the free energy crossing point of Al (557)with Al (111)due to the presence of kinks.As a further check on the predictions based on the embedded atom Al potential,reinforcing the plausibility of our estimate,we calculate T R for vicinals within the Terrace-Ledge-Kink (TLK)model of Villain et al.[15],through the relationK =W mand it equals2for the original TLK model;values of2 for Cu(113)[16]and2.1for Ag(115)[3]have been sug-gested based on experiments or MonteCarlo simulations on vicinals.We evaluate these parameters from total en-ergy calculations on slabs containing at least5steps per slab side,and comprising from1700to4000atoms de-pending on orientation.The parameter W m is calculated removing one complete atomic row of step-edge atoms.If N is the total number of atoms,and L that of step-edge atoms,the total energy for row remowal isL W m=E N−L−[E N−LE b],(8) with E N−L and E N the internal energy of system after and before row removal,and E b the bulk energy per atom. W m is thus defined per atom.For a kink we only remove half a row,creating two kinks:2W0=E N−L/2−[E N−(L/2)E b]−W m.(9) with E N−L/2the internal energy of the slab after half-row removal.For Al(335)wefind W m=3meV,W0=112meV,T R= 411K;for Al(557)wefind W m=1meV,W0=108meV, T R=314K;for Al(8810)wefind W m=0.1meV, W0=106meV,and T R=209K.These values are quite comparable with results of previous investigations on stepped metal surfaces[17,18].Our numbers for Al(335) are compatible with those inferred from STM measure-ments on Ag(115)[19],which has the same step-step separation:W m=3meV,W0=114meV,and T R=427 K.[The(115)face consists of(111)-faceted steps sepa-rated by a(100)terrace four atomic rows wide,whereas the(335,has(100)steps and(111)terraces.]Concerning T R of Al(111),den Nijs et al.observed[20]roughening of Ni(115)at about200K,and estimated420K for the roughening of Ni(100),the nearest singular face on the stereographic plot.Our value of412K for Al(335)simi-larly suggests that our upper bound of890K for the asso-ciated singular(111)is quite plausible.Our predictions for both singular and vicinal faces await experimental verification.In summary we have calculated an upper bound to the roughening temperature of a singular metal surface using an atomistic simulation method.Our results for Al(111)suggest that roughening may occur apprecia-bly below melting,and be therefore observable,save for kineting hindering.VF thanks the Alexander von Humboldt-Stiftung for supporting his stays at the Walter Schottky Institut.。
dmu计算遗传相关
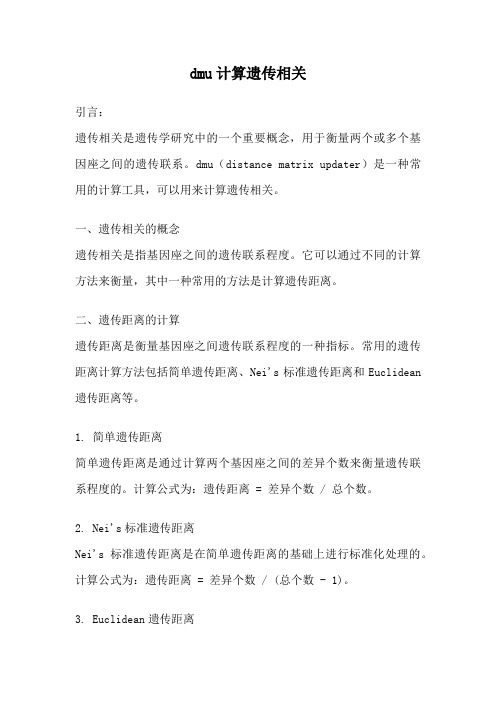
dmu计算遗传相关引言:遗传相关是遗传学研究中的一个重要概念,用于衡量两个或多个基因座之间的遗传联系。
dmu(distance matrix updater)是一种常用的计算工具,可以用来计算遗传相关。
一、遗传相关的概念遗传相关是指基因座之间的遗传联系程度。
它可以通过不同的计算方法来衡量,其中一种常用的方法是计算遗传距离。
二、遗传距离的计算遗传距离是衡量基因座之间遗传联系程度的一种指标。
常用的遗传距离计算方法包括简单遗传距离、Nei's标准遗传距离和Euclidean 遗传距离等。
1. 简单遗传距离简单遗传距离是通过计算两个基因座之间的差异个数来衡量遗传联系程度的。
计算公式为:遗传距离 = 差异个数 / 总个数。
2. Nei's标准遗传距离Nei's标准遗传距离是在简单遗传距离的基础上进行标准化处理的。
计算公式为:遗传距离 = 差异个数 / (总个数 - 1)。
3. Euclidean遗传距离Euclidean遗传距离是通过计算两个基因座之间的欧氏距离来衡量遗传联系程度的。
计算公式为:遗传距离= sqrt(∑(基因频率1-基因频率2)^2)。
三、dmu的介绍dmu是一种常用的计算工具,可以用来计算遗传相关。
它提供了多种计算遗传距离的方法,并可以根据用户的需求自定义计算参数。
1. 安装和配置dmu要使用dmu进行遗传相关的计算,首先需要在计算机上安装dmu软件,并进行相关的配置。
具体的安装和配置步骤可以参考dmu官方的安装手册。
2. 使用dmu进行遗传相关计算一旦安装和配置完成,就可以使用dmu进行遗传相关的计算了。
用户可以根据自己的需求选择不同的遗传距离计算方法,并输入相应的参数进行计算。
dmu会输出计算结果,并提供可视化的图表展示。
四、dmu的优势和应用dmu作为一种计算工具,具有以下优势和应用:1. 灵活性:dmu可以根据用户的需求进行自定义计算,可以选择不同的遗传距离计算方法,并可以输入相应的参数进行计算。
宽阔水自然保护区棘胸蛙种群的RAPD遗传多样性
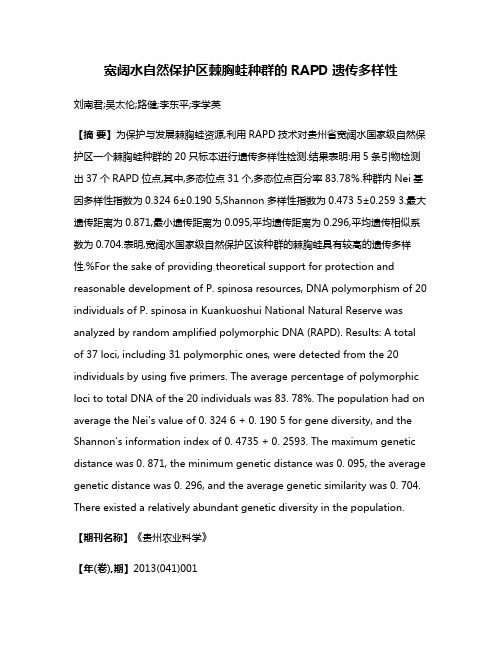
宽阔水自然保护区棘胸蛙种群的RAPD遗传多样性刘南君;吴太伦;路健;李东平;李学英【摘要】为保护与发展棘胸蛙资源,利用RAPD技术对贵州省宽阔水国家级自然保护区一个棘胸蛙种群的20只标本进行遗传多样性检测.结果表明:用5条引物检测出37个RAPD位点,其中,多态位点31个,多态位点百分率83.78%.种群内Nei基因多样性指数为0.324 6±0.190 5,Shannon多样性指数为0.473 5±0.259 3.最大遗传距离为0.871,最小遗传距离为0.095,平均遗传距离为0.296,平均遗传相似系数为0.704.表明,宽阔水国家级自然保护区该种群的棘胸蛙具有较高的遗传多样性.%For the sake of providing theoretical support for protection and reasonable development of P. spinosa resources, DNA polymorphism of 20 individuals of P. spinosa in Kuankuoshui National Natural Reserve was analyzed by random amplified polymorphic DNA (RAPD). Results: A total of 37 loci, including 31 polymorphic ones, were detected from the 20 individuals by using five primers. The average percentage of polymorphic loci to total DNA of the 20 individuals was 83. 78%. The population had on average the Nei's value of 0. 324 6 + 0. 190 5 for gene diversity, and the Shannon's information index of 0. 4735 + 0. 2593. The maximum genetic distance was 0. 871, the minimum genetic distance was 0. 095, the average genetic distance was 0. 296, and the average genetic similarity was 0. 704. There existed a relatively abundant genetic diversity in the population.【期刊名称】《贵州农业科学》【年(卷),期】2013(041)001【总页数】4页(P14-17)【关键词】宽阔水自然保护区;棘胸蛙;遗传多样性【作者】刘南君;吴太伦;路健;李东平;李学英【作者单位】遵义医学院细胞生物学与遗传学教研室,贵州遵义563099;贵州宽阔水国家级自然保护区管理局,贵州绥阳563300;遵义医学院细胞生物学与遗传学教研室,贵州遵义563099;遵义医学院形态学实验室,贵州遵义563099;遵义医学院细胞生物学与遗传学教研室,贵州遵义563099【正文语种】中文【中图分类】Q38棘胸蛙(Paa spinosa),俗称石亢、石蛙,其肉质鲜美且富含营养,具清凉滋补和药用功能,具有很高的经济价值[1]。
- 1、下载文档前请自行甄别文档内容的完整性,平台不提供额外的编辑、内容补充、找答案等附加服务。
- 2、"仅部分预览"的文档,不可在线预览部分如存在完整性等问题,可反馈申请退款(可完整预览的文档不适用该条件!)。
- 3、如文档侵犯您的权益,请联系客服反馈,我们会尽快为您处理(人工客服工作时间:9:00-18:30)。
The Nei’s Standard Genetic Distance inArtificial EvolutionYoshiaki KatadaGraduate School ofScience and TechnologyKobe UniversityKobe657-8501,JAPAN Email:katada@rci.scitec.kobe-u.ac.jpKazuhiro OhkuraFaculty ofEngineeringKobe UniversityKobe657-8501,JAPANEmail:ohkura@rci.scitec.kobe-u.ac.jpKanji UedaRACE(Research into Artifacts,Center for Engineering)The University of TokyoMeguro153-8904,JAPANEmail:ueda@race.u-tokyo.ac.jpAbstract—In recent years,not only ruggedness but also neutrality has been recognized as an important feature of a fitness landscape for genetic search.Following that the concept of neutrality in artificial evolution originates from Kimura’s neutral theory in natural evolution,it is expected that the dynamics of artificial evolution in the landscapes including neutrality would be described by using techniques in population genetics. Furthermore,new theoretical guidelines might be developed for effective genetic search.In a recent paper[25],we have discussed the use of the Nei’s standard genetic distance,which originates from population genetics,for measuring neutrality offitness landscapes.In our results,several consistencies with the population genetics have been found by applying the Nei’s standard genetic distance to a tunably neutral NK landscape.In this paper,computer simulations are systematically conducted by using a standard genetic algorithm in order to clarify the characteristics of the Nei’s standard genetic distance.The terraced NK landscape is adopted as a test function.I.I NTRODUCTIONMany EA researchers have been inspired by natural evo-lution and trying to model the process of natural evolution in order to develop powerful optimization methods[1][2][3]. Therefore,the dynamics in artificial evolution would be ex-plained by the theory of natural evolution.But until recently, few theories which are applicable to artificial evolution have been found.Since the concept of neutrality was introduced into the EA community,EA researchers have expected that the dynamics of artificial evolution would be described by using techniques in population genetics.This is because the concept of neutrality in artificial evolution originates from Kimura’s neutral theory in population genetics.Therefore,neutrality has attracted much research interest in recent years[4][5].This feature,due to highly redundant mappings from genotype to phenotype or from phenotype to fitness,is also found in natural systems.From this point of view,evolutionary theorists[6]and molecular biologists[7][8] also have investigated it.Neutrality has been found in many real-world applications of artificial evolution,such as evolution of neural network controllers in robotics[9][10][11][12],on-chip electronic cir-cuit evolution[13][14][15].Landscapes which include neutral-ity have been conceptualized as containing neutral networks [16][17][18].Harveyfirst introduced the concept of neutral networks into the EA community[16].He defined it as follows:“A neutral network of afitness landscape is defined as a set of connected points of equivalentfitness,each repre-senting a separate genotype:here connected means that there exists a path of single(neutral)mutations which can traverse the network between any two points on it without affecting fitness.”For these years,several papers[19][20][17][18]have been published for investigating the evolutionary dynamics. Population geneticists have been trying to explain evo-lutionary change quantitatively,that is,the change of gene frequency in the population.Statistical methods for estimating the number of gene differences and the divergence time between related species have been developed.These methods use electrophoretic data for investigating protein variation. The results are compared with the divergence time derived from the fossil records.However,population geneticists cannot get complete information about the genetic material through electrophoresis.In artificial evolution,EA researchers can get all the genetic information of a population.Furthermore they can define genetic operators.Therefore,the introduction of such statistical methods for estimating the number of gene substitutions would be helpful to understand the mechanism of EAs for solving difficult optimization problems.From a theoretical point of view,it would be beneficial to investigate whether the number of substitutions estimated in EAs can be understood by the theory of natural evolution. According to Kimura’s neutral theory[6]and Ohta’s nearly neutral theory[21][22],the following assertions have been made[23]:1)For each protein,the rate of evolution in terms of aminoacid substitutions is approximately constant per year persite for various lines,as long as the function and tertiarystructure of the molecule remain essentially unaltered.2)Functionally less important molecules or parts of amolecule evolve(in terms of mutant substitutions)fasterthan more important ones.3)Those mutant substitutions that disrupt less the existingstructure and function of a molecule(conservative sub-stitutions)occur more frequently in evolution than moredisruptive ones.4)Gene duplication must always precede the emergence ofa gene having a new function.5)Selective elimination of definitely deleterious mutantsand randomfixation of selectively neutral or veryslightly deleterious mutants occur far more frequently inevolution than positive Darwinian selection of definitelyadvantageous mutants.Recently,we have discussed the use of the Nei’s standard genetic distance[24],which is one of such statistical methods for estimating the number of substitutions,for measuring neutrality offitness landscapes1[25].In our experiments, several consistencies with population genetics have been found by applying the Nei’s standard genetic distance to the results of evolution on tunably neutral NK landscapes.These can be summarized as follows:Under small mutation rate per locus andfixed population size,•The number of gene substitutions increases with the increase of neutrality.•The number of gene substitutions decreases with the increase of ruggedness where the landscape includes neutrality.•The number of gene substitutions is largest when random sampling is applied with mutation.To clarify whether these results hold for different population sizes and to discuss the consistencies with population genetics, a systematic investigation should be done.This paper investigates the characteristics of the Nei’s standard genetic distance infitness landscapes including neu-trality in various conditions.The next section describes the Nei’s standard genetic distance.Section III applies the Nei’s genetic distance to one of tunably neutral landscapes called the terraced NK landscape and shows the results.Section IV discusses the error threshold on the population size and the mutation rate based on the obtained results.Conclusions are given in the last section.II.T HE N EI’S S TANDARD G ENETIC D ISTANCE Genetic distance is a term of population genetics used for estimating gene differences per locus between populations. Although there are several definitions for this,the Nei’s standard genetic distance[24]is adopted in this paper.The Nei’s standard genetic distance is defined as follows. Consider two populations,X and Y.Let x ik and y ik be the frequencies of the k-th alleles(i=1,···,N,k∈{1,2}in a binary coded GA)in X and Y,respectively.The probability of identity of two randomly chosen genes is j xi=x2i1+x2i2in the population X,while it is j yi=y2i1+y2i2in the population Y.The probability of identity of a gene from X and a gene from Y is j xyi=x i1y i1+x i2y i2.The normalized identity of genes between X and Y with respect to a locus is defined asI i=j xyi√j xij yi,(1)1Since the assertion1),2)and3)can be interpreted as the number of gene substitutions of each genotype increases with the increase of neutrality,the number of gene substitutions could be an index of neutrality.where,I i=1.0if the two populations have the same alleles in identical frequencies,and I i=0.0if they have no common alleles.The normalized identity of genes between X and Y with respect to the average in all loci is defined asI=J XY√J X√J Y,(2) where,J X=Ni=1j xi/N,J Y=Ni=1j yi/N and J XY= Ni=1j xyi/N.The genetic distance between X and Y is defined asD=−log e I,(3)under the assumption that the mutation rate per locus is sufficiently small.However,the above definition cannot be applied to the standard GA directly,because it is assumed that a new allele always appears on a locus when a mutation occurs, while“back mutations[21]”frequently occur in the standard GA,due to the binary coding scheme.Therefore,the genetic distance between the population at the initial generation and the one at the last generation is calculated as:D final=T−11D t,t+1(4)where T is the number of the last generation and D t,t+1is the genetic distance between the population in the t-th and the (t+1)-th generation.The rate of gene substitution is defined as the genetic distance per generation.III.T HE N EI’S S TANDARD G ENETI C D ISTANCE IN A T UNABLY N EUTRAL NK L ANDSC APEA.A Terraced NK LandscapeA terraced NK landscape was employed as the test function in our computer simulations.This is the tunably neutral NK landscape proposed by Newman and Engelhardt[26].A terraced NK landscape has three parameters:N,the length of the genotype;K,the number of epistatic linkages between genes;and w,the contribution of a locus to thefitness of the entire genotype.Thefitness value is calculated as follows:Thefitness contri-bution of the i-th locus,w i,is an integer generated randomly in the range0≤w i<F,i=1,···,N.To calculate thefitness, W,of a genotype,thefitness contribution of each locus is averaged,and then divided by F−1,normalizing W to the range0.0to1.0.More formally:W=1N(F−1)Ni=1w i.(5)The neutrality of the landscape can be tuned by changing the value of F.The neutrality of the landscape is maximized when F=2,and is effectively non-existent as F→∞.120500100015002000N u m b e r o f s u b s t i t u t i o n sGeneration(a)K =0012500100015002000N u m b e r o f s u b s t i t u t i o n sGeneration(b)K =2120500100015002000N u m b e r o f s u b s t i t u t i o n sGeneration(c)K =6120500100015002000N u m b e r o f s u b s t i t u t i o n sGeneration(d)K =12120500100015002000N u m b e r o f s u b s t i t u t i o n sGeneration(e)K =19Fig.1.Number of substitutions at each generation for the SGA with q =0.008and M =50for F =∞in 50runsB.Simulation ConditionsWe applied two genetic algorithms:the standard GA (SGA)and the (random-sampling ,q )-algorithm.The (random-sampling ,q )-algorithm employs standard bit mutation at the rate of q as the genetic operation and random sampling as a selection method where M offsprings are sampled from M ancestors with replacements.This model was used to investigate the effect of random sampling with mutation on the genetic distance.This is approximately equivalent with Kimura’s stochastic genetic models to study random genetic drift and the expected time of fixation of a mutant gene [6].Computer simulations were conducted by varying the land-scape parameters,the population size,M ,and the mutation rate,q .The SGA used standard bit mutation as the genetic operation.Crossover was not employed.Tournament selection was adopted for the SGA.The tournament size was set at 2for the SGA.Each run lasted 2,000generations.We conducted 50independent runs for each problem under the landscape pa-rameters,N =20,K ∈{0,2,6,12,19},F ∈{2,3,4,6,∞2}.The results were averaged over 50runs.C.Existence And Non-existence of NeutralityThe first experiments were conducted to investigate the effect of the existence of neutrality on the transition of the genetic distance and the number of substitutions.Fig.1shows the number of substitutions of the SGA for F =∞,where q was set at 0.008based on the assumption of eq.(3).They2ForF =∞,the NK fitness landscape[27]was employed instead of theterraced NK landscape as [5],which results in practically non-existence of neutrality.02468100500100015002000N u m b e r o f s u b s t i t u t i o n sGenerationFig.2.Number of substitutions at each generation for the (random-sampling ,q )-algorithm with q =0.008and M =50in 50runsleveled off in the very early generations.This means that the population converged to a certain point in the genotype space then the genetic distance between the generations (D t,t +1in eq.(4))became zero.In contrast,the number of substitutions of the (random-sampling ,q )-algorithm (Fig.2)and the SGA for F =∞(for instance,the results for F =2are shown in Fig.3.)with q =0.008increased approximately linearly over generations in all runs.This differentiates between the existence and the non-existence of neutrality in the fitness landscape.That is,the increase of the number of substitutions over generations indicates the presence of neutrality in the fitness landscape.In the remainder of this paper,the rates of substitution for the (random-sampling ,q )-algorithm and the SGA for F =∞are shown by using the method of least squares on the results of all the runs because the rate of substitution is equivalent to the gradient of the number of substitutions over generations.02468100500100015002000N u m b e r o f s u b s t i t u t i o n sGeneration(a)K =00123450500100015002000N u m b e r o f s u b s t i t u t i o n sGeneration(b)K =20123450500100015002000N u m b e r o f s u b s t i t u t i o n sGeneration(c)K =60123450500100015002000N u m b e r o f s u b s t i t u t i o n sGeneration(d)K =120123450500100015002000N u m b e r o f s u b s t i t u t i o n sGeneration(e)K =19Fig.3.Number of substitutions at each generation for the SGA with q =0.008and M =50for F =2in 50runsTABLE IT HE RATE OF SUBSTITUTION FOR THE SGA WITH q =0.008ANDM =50HH H HF K 026121920.0020420.0006660.0002200.0001310.00010630.0013070.0004210.0001730.0001020.00010440.0010630.0003180.0001300.0000990.00008760.0007380.0002350.0001280.0000900.000087TABLE IIT HE RATE OF SUBSTITUTION FOR THE SGA WITH q =0.008ANDM =100H H H HF K 026121920.0013700.0004010.0001480.0000790.00006830.0008430.0002550.0000960.0000510.00005040.0006070.0001640.0000730.0000570.00005060.0004050.0001280.0000560.0000480.000044D.Neutrality And Selective ConstraintWith respect to the assertions 1),2)and 3)in Section I,Kimura has suggested that the rate of gene substitution is largest when the selective advantage of a new mutation over the original allele is zero except that the new mutation is deleterious in a small population [6].Thus,it seems likely that the number of substitutions increases with the increase of neutrality and that for the (random-sampling ,q )-algorithm is largest,because random-sampling can be considered com-pletely neutral for selection.In addition to this,according toTABLE IIIT HE RATE OF SUBSTITUTION FOR THE SGA WITH q =0.008ANDM =200HH H HFK 026121920.0007810.0002630.0000860.0000400.00002630.0004860.0001610.0000510.0000390.00002840.0003590.0001190.0000410.0000290.00002760.0002380.0000670.0000310.0000250.000026TABLE IVT HE RATE OF SUBSTITUTION FOR THE SGA WITH q =0.008ANDM =400H H H HF K 026121920.0004340.0001460.0000430.0000320.00002430.0002610.0000870.0000320.0000250.00001340.0001930.0000620.0000190.0000140.00001960.0001270.0000500.0000170.0000170.000015Ohta’s nearly neutral theory [21][22],the stronger the selective constraint on the molecule is,the lower its rate of evolution becomes.That is,the number of substitutions is likely to decrease with the increase of selective constraint,K .Table I and Fig.4show the rate of substitution for the SGA with q =0.008and M =50.Notice first that the rate of substitution increased with the decrease of F for all K s.This means that the rate of substitution increases with the increase of neutrality as predicted.Secondly,the rate of substitution decreased with the increase of K for all F s.0.00000.00050.00100.00150.0020024681012141618R a t e o f s u b s t i t u t i o nKF=2F=3F=4F=6Fig.4.Rate of substitution for the SGA with q =0.008and M =500.00000.00050.00100.00150.0020024681012141618R a t e o f s u b s t i t u t i o nKF=2F=3F=4F=6Fig.5.Rate of substitution for the SGA with q =0.008and M =1000.00000.00050.00100.00150.0020024681012141618R a t e o f s u b s t i t u t i o nKF=2F=3F=4F=6Fig.6.Rate of substitution for the SGA with q =0.008and M =2000.00000.00050.00100.00150.0020024681012141618R a t e o f s u b s t i t u t i o nKF=2F=3F=4F=6Fig.7.Rate of substitution for the SGA with q =0.008and M =4000.00000.00050.00100.00150.0020024681012141618R a t e o f s u b s t i t u t i o nKM=50M=100M=200M=400Fig.8.Rate of substitution for the SGA with q =0.008for F =20.00000.00050.00100.00150.0020024681012141618R a t e o f s u b s t i t u t i o nKM=50M=100M=200M=400Fig.9.Rate of substitution for the SGA with q =0.008for F =30.00000.00050.00100.00150.0020024681012141618R a t e o f s u b s t i t u t i o nKM=50M=100M=200M=400Fig.10.Rate of substitution for the SGA with q =0.008for F =40.00000.00050.00100.00150.0020024681012141618R a t e o f s u b s t i t u t i o nKM=50M=100M=200M=400Fig.11.Rate of substitution for the SGA with q =0.008for F =6TABLE VT HE RATE OF SUBSTITUTION FOR THE(random-sampling,q)-ALGORITHM WITH q=0.008FOR EACH POPULATION SIZEM50100200400rate0.0045760.0031940.0019710.001120This means that not only neutrality but also ruggedness hasan influence on the rate of substitution.This tendency is consistent with Ohta’s results for NK landscapes with weak selection based on the nearly neutral theory,where the number of substitutions decreases with the increase of K[22][28]. Similar behavior to M=50is shown for each population size M={100,200,400}(Table II,III and IV,and Fig.5,6 and7).The rate of substitution for the(random-sampling,q)-algorithm with each M is shown in Table V.It is confirmed that for each M,the rate of substitution for the(random-sampling,q)-algorithm was always larger than any others for the SGA with K and F(from Table I to IV).This agrees with our expectation.E.V arying The Population SizeIn the next experiments,the analysis was extended by varying the population size.According to Ohta’s nearly neu-tral theory[21][22],population movement depends on the population size.That is,mutant dynamics becomes slower by increasing the population size.This is demonstrated from Fig.8to11and Table V.With the increase of the population size,the rate of substitution decreased for each K and F. Therefore,the larger the population size becomes,the slower the population moves.This tendency is also consistent with Ohta’s results for NK landscapes with weak selection based on the nearly neutral theory,where the number of substitutions decreases with the increase of the population size[22][28]. Table V shows the rate of substitution for the(random-sampling,q)-algorithm with each M.The rate of substitution also decreased with the increase of the population size.F.V arying The Mutation RateIn population genetics,it is assumed that the mutation rate per locus is sufficiently small as mentioned in Section II.In the last series of experiments,the transition of the Nei’s genetic distance were observed by varying the mutation rate from q= 0.005to0.010and0.1for the SGA with M=50.Fig.12shows the results with q= {0.005,0.006,0.007,0.008,0.009,0.010}.In this range, the rate of substitution increased with the increase of the mutation rate for each K and F.For each q,similar behaviors were observed to the results with q=0.008in the previous subsections.In contrast,the results with q=0.1show the different behaviors(Fig.13).Surprisingly,the rate of substitution increased with the increase of K for all F s. In addition to this,no significant differences were found between the graphs of different F s.The rate of substitution for the(random-sampling,q)-algorithm with q=0.1was0.00000.00050.00100.00150.0020024681012141618RateofsubstitutionKF=2F=3F=4F=6Fig.12.Rate of substitution for the SGA with M=50:The solid lines, from left to right,correspond to the rate of substitution for F=2with q={0.005,0.006,0.007,0.008,0.009,0.010}.Similaly,the dashed lines correspond to the rate of substitution for F∈{3,4,6}.0.0100.0110.0120.0130.0140.0150.0160.017024681012141618RateofsubstitutionKF=2F=3F=4F=6Fig.13.Rate of substitution for the SGA with q=0.1and M=500.0135733.Thus,the rate of substitution for the SGA was higher than that for the(random-sampling,q)-algorithm for K>2and all F s.This implies that artificial evolution has changed into random search,caused by the mutation rate which is larger than the error threshold[17].From the above,we confirmed that the Nei’s genetic dis-tance depends on the mutation rate,and can be used as long as the mutation rate is sufficiently small compared with the error threshold.IV.D ISCUSS IONIn the previous section,it has been shown that population movement depends on the population size.If the population size is small,the population for the SGA moves quickly.This would have the advantage offlexibility.As pointed out in[22], evolution would become moreflexible for a small population size than for a large population size,particularly when the environment is not static,that is,thefitness landscape changes occasionally.On the other hand,it has been reported that as the population size becomes too small,it becomes easier for the population to lose the current best individuals through random sampling or mutation and fall to lower neutral networks[20]. This phenomenon is due to the influence of the error threshold on the population size3.This would be more understandable 3It has been known that there are two kinds of error threshold:on the mutation rate and on the population size[20][17].by considering the population movement.Due to the small population size,the population on the neutral networks moves too quickly to keep the current neutral network.This implies that there exists the optimal population size that keeps the fastest speed as well as avoids the influence of the error threshold.The same discussion can be applied to the error threshold on the mutation rate mentioned in Section III-F[17][18].V.C ONCLUSIONSWe have investigated the characteristics of the Nei’s stan-dard genetic distance by applying it to the Terraced NK landscapes,and shown the consistencies of the results with the neutral theory and the nearly neutral theory in population genetics.Based on the presented results,we discussed the influence of the error threshold on the population size and the mutation rate.The characteristics of the number of substitutions estimated by the Nei’s genetic distance can be summarized as follows: When the mutation rate per locus is small,•Random sampling with mutation results in the largest number of substitutions.•The number of substitutions increases with the increase of neutrality.•The number of substitutions decreases with the increase of ruggedness where the landscape includes neutrality.•The number of substitutions decreases with the increase of the population size.These results can be predicted mainly by the assertion of the neutral theory and the nearly neutral theory,“functionally less important molecules or parts of a molecule evolve faster than more important ones”.Consequently,these allow us to under-stand the evolutionary dynamics of GAs from the viewpoint of population genetics using the Nei’s standard genetic distance. Therefore,this method will play a significantly important role that connects artificial evolution and natural evolution.R EFERENCE S[1]J.H.Holland,Adaptation in Natural and Artificial Systems,Universityof Michigan Press,1975.[2]T.B¨a ck and H.-P.Schwefel,”An Overview of Evolutionary Algo-rithms for Parameter Optimization,”Evolutionary Computation,1(1):1-23,1993.[3]H.M¨u hlenbein,”Predictive Models for the Breeder Genetic Algorithm,”Evolutionary Computation,1(1):25–49,1993.[4]M.Ebner,ngguth,J.Albert,M.Shackleton and R.Shipman,”OnNeutral Networks and Evolvability,”In Proceedings of the2001IEEE Congress on Evolutionary Computation:CEC2001,IEEE Press pp.1–8, 2001.[5]T.Smith,P.Husbands,yzell and M.O’Shea,”Fitness Landscapesand Evolvability,”Evolutionary Computation,10(1):1-34,2002.[6]M.Kimura,The Neutral Theory of Molecular Evolution,CambridgeUniversity,Press,New Y ork,1983.[7] C.V.Forst,C.Reidys and J.Weber,”Evolutionary Dynamics and Opti-mization:Neutral Networks as Model-Landscapes for RNA Secondary-Structure Folding-Landscapes,”In Proceedings of the Third European Conference on Artificial Life ECAL95,pp.128–147,1995.[8]M.Huynen,P.Stadler and W.Fontana,”Smoothness within ruggedness:The role of neutrality in adaptation,”In Proceedings of the National Academy of Science USA,93,pp.397–401,1996.[9]I.Harvey,”Artificial Evolution for Real Problems,”In EvolutionaryRobotics:From Intelligent Robots to Artificial Life(ER’97),T.Gomi, Ed.AAI Books,1997.[10]T.Smith,P.Husbands and M.O’Shea,”Neutral Networks and Evolv-ability with Complex Genotype-Phenotype Mapping,”In Proceedings of the European Conference on Artificial Life:ECAL2001,pp.23–36, 2001.[11]T.Smith,P.Husbands and M.O’Shea,”Neutral Networks in anEvolutionary Robotics Search Space,”In Proceedings of the2001IEEE Congress on Evolutionary Computation,pp.136–145,2001.[12]T.Smith,A.Philippides,P.Husbands and M.O’Shea,”Neutrality andRuggedness in Robot Landscapes,”In Proceedings of the2002IEEE Congress on Evolutionary Computation,pp.1348–1353,2002.[13] A.Thompson,”An Evolved Circuit,Intrinsic in Silicon,Entwinedwith Physics,”In Proceedings of thefirst International Conference on Evolvable Systems:From Biology to Hardware,pp.390–405,1996. [14]V.K.V assilev,T.C.Fogarty and ler,”Information Charac-teristics and the Structure of Landscapes,”Evolutionary Computation, 8(1):31–60,2000.[15]V.K.V assilev and ler,”The Advantages of Landscape Neutralityin Digital Circuit Evolution,”In Proceedings of the Third International Conference on Evolvable Systems:From Biology to Hardware,pp.252-263,2000.[16]I.Harvey and A.Thompson,”Through the Labyrinth Evolution Findsa Way:A Silicon Ridge,”In Proceedings of the First InternationalConference on Evolvable Systems:From Biology to Hardware,pp.406–422,1996.[17] E.Nimwegen,J.Crutchfield and M.Mitchell,”Statistical dynamics ofthe royal road genetic algorithm,”In Theoretical Computer Science, V ol.229,No.1,pp.41-102,1999.[18]L.Barnett,”Netcrawling-Optimal Evolutionary Search with NeutralNetworks,”In Proceedings of the2001IEEE Congress on Evolutionary Computation,pp.30–37,2001.[19]L.Barnett,”Tangled Webs:Evolutionary Dynamics on Fitness Land-scapes with Neutrality,”In MSc.dissertation,School of Cognitive and Computing Sciences,Sussex University,UK,1997.[20] E.Nimwegen and J.Ctrutchfield,”Optimizing epochal evolutionarysearch:Population-size dependent theory,”In SFI Working Paper9810-090,Santa Fe Institute,1998.[21]T.Ohta,”The nearly neutral theory of molecular evolution,”Annu.Rev.Ecol.Syst.,23:263-286,1992.[22]T.Ohta,”Evolution by nearly-neutral mutations,”In Genetica,102/103,pp.83-90,1998.[23]M.Kimura and T.Ohta,”On Some Principles Governing MolecularEvolution,”In Proc.Nat.Acad.Sci.,V ol.71,No.7,pp.2848–2852, 1974.[24]M.Nei,”Genetic Distance between Populations,”In The AmericanNaturalist,V ol.106,pp.283-292,1972.[25]Y.Katada,K.Ohkura and K.Ueda,”Measuring Neutrality of FitnessLandscapes Based on the Nei’s Standard Genetic Distance,”In Proceed-ings of2003Asia Pacific Symposium on Intelligent and Evolutionary Systems:Technology and Applications,pp.107-114,2003.[26]M.Newman and R.Engelhardt,”Effect of neutral selection on theevolution of molecular species,”In Proceedings of the Royal Society of London B,Morgan Kaufmann,256,pp.1333-1338,1998.[27]S.Kauffman,The origins of order,Oxford University Press,1993.[28]T.Ohta,”Role of random genetic drift in the evolution of interactivesystems,”In Journal of Molecular Evolution,V ol.44,pp.S9-S14,1997.。