Market-Based Multirobot Coordination- A Survey and Analysis(CMU-RI-TR-05-12)
智能投顾:金融与科技的时代融合

智能投顾:金融与科技的时代融合作者:潘芳来源:《中国金融电脑》 2017年第6期现代社会中,人们的工作和生活空间随着信息传播的速度与范围都有了超越以往的飞速进展,我们一边获取更丰富的信息、了解更多的知识、掌握更多的技能,一边追求更多的财富积累,实现财富保值、升值的金融工具创新逐渐成为大众渴望了解、掌握及运用的发展方向。
近期在欧美市场迅速兴起的数字化资产配置服务——智能投顾(RoboAdvisor)也进入了中国民众的投资关注领域。
智能投顾是以马科维茨(Markowitz)的现代投资组合理论(PortfolioTheory)为基础,通过网络和移动终端,借助于互联网技术和机器算法,分析投资市场的有效边界,并针对不同风险偏好的投资者,综合推导预期收益最大化的投资组合建议。
马科维茨从对回报率和风险的定量出发,系统地研究了投资组合的特性,从数学定量上解释了投资者的如何规避风险,及优化投资组合的方法。
经他研究发现,分散投资是金融市场的“免费午餐”。
另一经济学家丹尼尔·卡内曼(DanielKahneman)也指出:“大多数投资者并非是理性投资者,而是行为投资者;他们的行为不总是理性的,也并不总是回避风险的”,投资者在决策过程中存在非理性的行为偏差。
“智能投顾”的目标就是在得知每个用户自己的风险的时候,能够帮助用户决定最优配置点对应的资产配置组合以及如何达成最优组合。
通过分散的投资组合在降低风险的同时不会降低预期收益率,投资者能够在同样的风险水平上获得更高的收益率,或者在同样收益率水平上承受更低的风险。
分散化、个性化以及长期化也是智能投顾的特点及优势。
分散化的大类资产配置可以有效避免单一资产无法长期保持良好收益的局限性,毕竟“尺有所长,寸有所短”;同时,通过有效筛选的大类资产组合有着稳定收益、控制风险的轮动效应——“失之东隅,收之桑榆”也是大家喜闻乐见的投资成果。
个性化的资产配置为不同的社会群体及投资主体提供了差异化、多元化的投资组合方案。
人工智能生态相关的专业名词解释
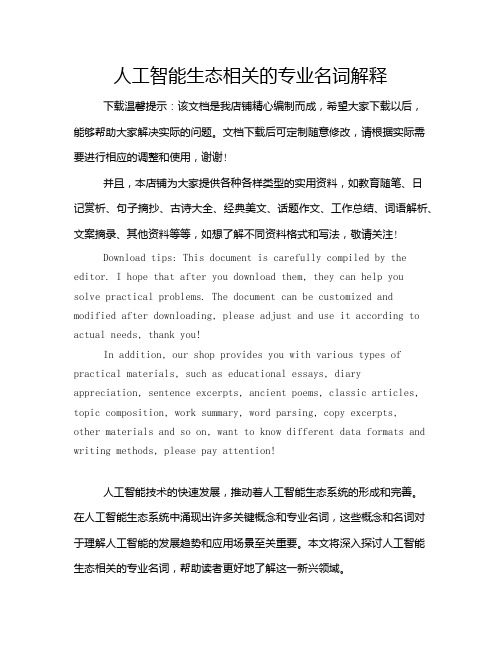
人工智能生态相关的专业名词解释下载温馨提示:该文档是我店铺精心编制而成,希望大家下载以后,能够帮助大家解决实际的问题。
文档下载后可定制随意修改,请根据实际需要进行相应的调整和使用,谢谢!并且,本店铺为大家提供各种各样类型的实用资料,如教育随笔、日记赏析、句子摘抄、古诗大全、经典美文、话题作文、工作总结、词语解析、文案摘录、其他资料等等,如想了解不同资料格式和写法,敬请关注!Download tips: This document is carefully compiled by the editor. I hope that after you download them, they can help yousolve practical problems. The document can be customized and modified after downloading, please adjust and use it according to actual needs, thank you!In addition, our shop provides you with various types of practical materials, such as educational essays, diary appreciation, sentence excerpts, ancient poems, classic articles, topic composition, work summary, word parsing, copy excerpts,other materials and so on, want to know different data formats and writing methods, please pay attention!人工智能技术的快速发展,推动着人工智能生态系统的形成和完善。
从创意到创业智慧树知到答案2024年湖南师范大学

从创意到创业湖南师范大学智慧树知到答案2024年绪论单元测试1.由IBM公司设计的()计算机与国际象棋世界冠军战胜了当时称霸世界棋坛的卡斯帕罗夫。
A:深绿 B:浅蓝 C:深蓝 D:浅绿答案:C2.在“大众创业、万众创新”的时代大潮中,越来越多的人凭借自己的创意走上了创业之旅,取得了事业的成功,下列属于通过创意走上创业之旅的有()A:罗振宇从自媒体专栏“罗辑思维”发展到“得到”app B:程维用滴滴出行重新塑造了网约车的商业模式 C:王兴把美团从一个社交类团购网站变成了生态化网络平台。
D:马东借助“奇葩说”成就了米未传媒答案:ABCD3.方军在《创意,未来的生活方式》一书中提到,创意就是创造性地分析问题和系统地解决问题,善于运用创意就会拥有未来的无限可能性。
()A:错 B:对答案:B4.()中提到,苟日新日日新又日新。
人生因创造而美好,普通人因创新而伟大,创业艰辛,正因此创业者被人们尊重;开拓艰难,正因此奠基人被世人铭记。
A:《孟子》 B:《中庸》 C:《大学》 D:《论语》答案:C5.创新创业学习不能仅限于在线课程,还可以通过()的方式开展。
A:通过影视剧学习 B:向资深专家们学习 C:大量阅读学术论文和专业书籍学习 D:向行业精英们学习答案:ABCD6.诺基亚时代手机多种型号,苹果时代手机只做iPhone一个独生子说明在乌卡时代我们应该以变化来应对变化。
()A:对 B:错答案:A第一章测试1.企业其实是企业家个性的外化,()决定了企业的发展方向。
()A:创业者的资本 B:创业者的目标 C:创业者对自身的了解 D:创业者的心态答案:C2.创业的三把钥匙包括()A:“你到哪里去” B:“你是谁” C:“你能做什么” D:“你从哪里来”答案:ABD3.经济上的成功是创业的原因,但绝不是创业的结果。
()A:错 B:对答案:A4.饿了么曾推出过以下哪些项目()A:未来餐厅 B:风车系统 C:火箭系统 D:蜂鸟系统答案:AD5.大学生创业热情高、社会经验少,很难一次性取得成功或者取得大得成功。
【国家自然科学基金】_双寡头市场_基金支持热词逐年推荐_【万方软件创新助手】_20140801
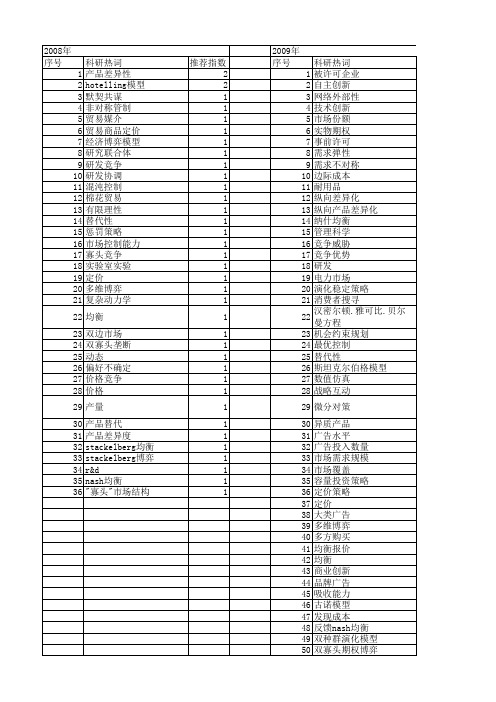
2011年 序号 1 2 3 4 5 6 7 8 9 10 11 12 13 14 15 16 17 18 19 20 21 22 23 24 25 26 27 28 29 30 31 32 33 34 35 36 37 38 39 40 41
1 1 1 1 1 1 1 1 1 1 1 1 1 1 1 1 1
2008年 序号 1 2 3 4 5 6 7 8 9 10 11 12 13 14 15 16 17 18 19 20 21 22 23 24 25 26 27 28 29 30 31 32 33 34 35 36
科研热词 产品差异性 hotelling模型 默契共谋 非对称管制 贸易媒介 贸易商品定价 经济博弈模型 研究联合体 研发竞争 研发协调 混沌控制 棉花贸易 有限理性 替代性 惩罚策略 市场控制能力 寡头竞争 实验室实验 定价 多维博弈 复杂动力学 均衡 双边市场 双寡头垄断 动态 偏好不确定 价格竞争 价格 产量 产品替代 产品差异度 stackelberg均衡 stackelberg博弈 r&d nash均衡 "寡头"市场结构
科研热词 被许可企业 自主创新 网络外部性 技术创新 市场份额 实物期权 事前许可 需求弹性 需求不对称 边际成本 耐用品 纵向差异化 纵向产品差异化 纳什均衡 管理科学 竞争威胁 竞争优势 研发 电力市场 演化稳定策略 消费者搜寻 汉密尔顿.雅可比.贝尔曼方程 机会约束规划 最优控制 替代性 斯坦克尔伯格模型 数值仿真 战略互动 微分对策 异质产品 广告水平 广告投入数量 市场需求规模 市场覆盖 容量投资策略 定价策略 定价 大类广告 多维博弈 多方购买 均衡报价 均衡 商业创新 品牌广告 吸收能力 古诺模型 发现成本 反馈nash均衡 双种群演化模型 双寡头期权博弈 双寡头市场 双寡头博弈
人工智能机器人市场调研报告
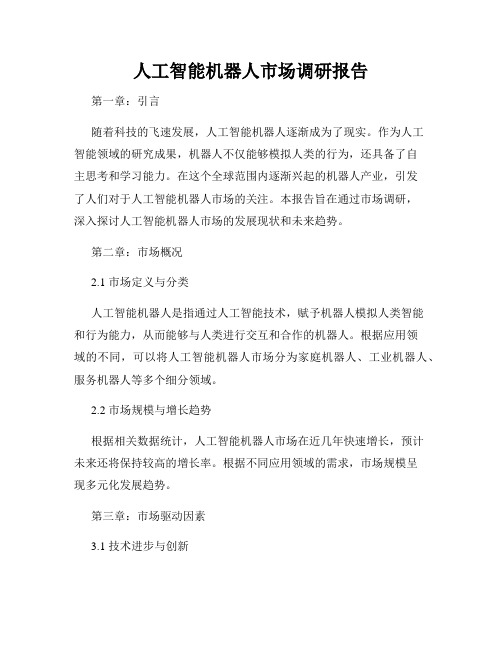
人工智能机器人市场调研报告第一章:引言随着科技的飞速发展,人工智能机器人逐渐成为了现实。
作为人工智能领域的研究成果,机器人不仅能够模拟人类的行为,还具备了自主思考和学习能力。
在这个全球范围内逐渐兴起的机器人产业,引发了人们对于人工智能机器人市场的关注。
本报告旨在通过市场调研,深入探讨人工智能机器人市场的发展现状和未来趋势。
第二章:市场概况2.1 市场定义与分类人工智能机器人是指通过人工智能技术,赋予机器人模拟人类智能和行为能力,从而能够与人类进行交互和合作的机器人。
根据应用领域的不同,可以将人工智能机器人市场分为家庭机器人、工业机器人、服务机器人等多个细分领域。
2.2 市场规模与增长趋势根据相关数据统计,人工智能机器人市场在近几年快速增长,预计未来还将保持较高的增长率。
根据不同应用领域的需求,市场规模呈现多元化发展趋势。
第三章:市场驱动因素3.1 技术进步与创新随着人工智能技术的不断进步和创新,机器人的智能化水平得到了大幅提升。
人工智能机器人具备更强大的学习、分析和决策能力,能够适应不同的环境和任务需求,因此受到了广泛的应用。
3.2 人工智能机器人在工业领域的应用在工业领域,人工智能机器人可以用于生产线的自动化控制、物流仓储的智能管理等工作。
机器人的投入不仅提高了生产效率,降低了成本,还有效避免了工人在重复性劳动中的安全问题。
3.3 人工智能机器人在服务领域的应用在服务领域,人工智能机器人可以应用于酒店、医院、银行等各类机构。
机器人可以代替人类从事客户服务、病人陪护、行李搬运等工作,大大提升了服务质量和效率。
第四章:市场挑战与机遇4.1 隐私和安全问题随着人工智能机器人的快速普及,隐私和安全问题也日益突出。
人们对于机器人获取个人信息和可能被黑客攻击的担忧使得市场面临一定的挑战。
4.2 人机协作的需求虽然人工智能机器人在某些工作领域表现出色,但在某些复杂的任务中,仍然需要人机混合协作才能达到更好的效果。
Coordination strategies for multi-robot exploration and mapping
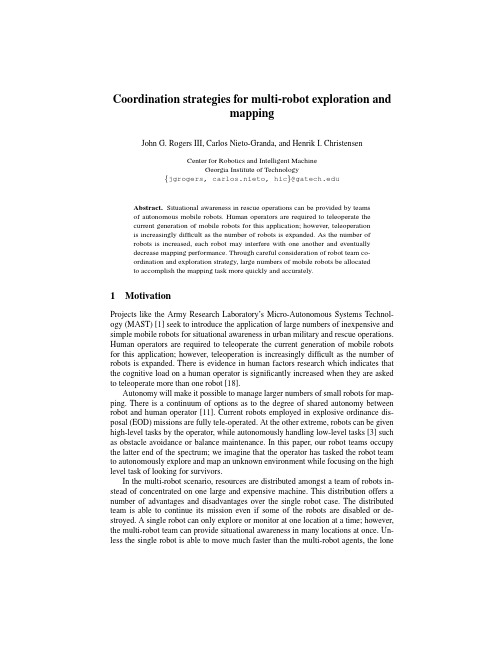
Coordination strategies for multi-robot exploration andmappingJohn G.Rogers III,Carlos Nieto-Granda,and Henrik I.ChristensenCenter for Robotics and Intelligent MachineGeorgia Institute of Technology{jgrogers,carlos.nieto,hic}@Abstract.Situational awareness in rescue operations can be provided by teamsof autonomous mobile robots.Human operators are required to teleoperate thecurrent generation of mobile robots for this application;however,teleoperationis increasingly difficult as the number of robots is expanded.As the number ofrobots is increased,each robot may interfere with one another and eventuallydecrease mapping performance.Through careful consideration of robot team co-ordination and exploration strategy,large numbers of mobile robots be allocatedto accomplish the mapping task more quickly and accurately.1MotivationProjects like the Army Research Laboratory’s Micro-Autonomous Systems Technol-ogy(MAST)[1]seek to introduce the application of large numbers of inexpensive and simple mobile robots for situational awareness in urban military and rescue operations. Human operators are required to teleoperate the current generation of mobile robots for this application;however,teleoperation is increasingly difficult as the number of robots is expanded.There is evidence in human factors research which indicates that the cognitive load on a human operator is significantly increased when they are asked to teleoperate more than one robot[18].Autonomy will make it possible to manage larger numbers of small robots for map-ping.There is a continuum of options as to the degree of shared autonomy between robot and human operator[11].Current robots employed in explosive ordinance dis-posal(EOD)missions are fully tele-operated.At the other extreme,robots can be given high-level tasks by the operator,while autonomously handling low-level tasks[3]such as obstacle avoidance or balance maintenance.In this paper,our robot teams occupy the latter end of the spectrum;we imagine that the operator has tasked the robot team to autonomously explore and map an unknown environment while focusing on the high level task of looking for survivors.In the multi-robot scenario,resources are distributed amongst a team of robots in-stead of concentrated on one large and expensive machine.This distribution offers a number of advantages and disadvantages over the single robot case.The distributed team is able to continue its mission even if some of the robots are disabled or de-stroyed.A single robot can only explore or monitor at one location at a time;however, the multi-robot team can provide situational awareness in many locations at once.Un-less the single robot is able to move much faster than the multi-robot agents,the lone2robot will be slower in performing the exploration and mapping task.These advantages are taken for a multi-robot team at the cost of increased complexity in communication and coordination.As the number of robots is increased,each robot may interfere with one another and eventually decrease the performance of the mapping task.Careful consideration of exploration strategy and coordination of large numbers of mobile robots can efficiently allocate resources to perform the mapping task more quickly and more accurately.Mobile robot simultaneous localization and mapping(SLAM)has been thoroughly addressed in the literature,see[2]and[6]for a detailed review of the history and state-of-the-art in SLAM research.The specific techniques used in this paper are based upon the Square Root SAM algorithm[4][5]which uses the well-known algorithms of linear algebra least-squares system solving to compute the map and robot trajectory based on a set of measurements.Multi-robot mapping and exploration was addressed in[9]and[17].These papers build a map using up to3robots with a decision-theoretic planner which trades off robot rendezvous operations with frontier exploration.These robots rendezvous to determine their relative pose transforms to provide constraints to recover thefinal map.In contrast, our approach does not require this rendezvous step because landmarks are globally data associated between each robot on a central map coordinator.The exploration strategy used is similar to our strategy called Reserve;however,we will not use a rendezvous step and do not require a decision-theoretic planner.2Technical ApproachWe use the Robot Operating System(ROS)from[12].ROS provides interprocess com-munication as well as coordination of sensor data with pose information.Our robot algorithms are implemented as a distributed set of programs which run in the ROS sys-tem.In addition,we make use of several implementations of common mobile robot software components which are provided in the ROS distribution such as motion plan-ning,obstacle avoidance,platform control,and IMU and odometryfiltering.2.1Mapping SystemOur mapping system is based upon the GTsam library developed at Georgia Tech.This library extends the Square Root SAM technique in[5]with sparse linear algebra in a nonlinear optimization engine.We have extended the GTsam library with a frame-work based upon the M-space formulation of Folkesson and Christensen[8]called OmniMapper.OmniMapper is a map library based upon a system of plugins which handle multiple landmark types simultaneously.We have used the OmniMapper in the past to build maps using multiple types of landmarks such as walls,doors,and ob-jects[14][13][16].This implementation builds maps of planar regions corresponding to walls and tables from[15].Each robot in the team builds a map locally with the OmniMapper and sends map data to the map coordinator.Each robot can incorporate new landmark measurements whenever it has moved far enough from the last pose where measurements were made.3Fig.1.OmniMapper.In the current implementation this is set to10cm.When a robotfinishes optimizing its local map with new landmark measurements,all relevant information needed by the map coordinator is packaged and transmitted.The information which is needed by the map coordinator to incorporate a new piece of information from a team member consists of many components.First,the sensor measurement data is needed.In the current implementation,this consists of the ex-tracted plane information consisting of a plane equation along with a convex hull of points along the perimeter of the plane.This represents a significant compression over an alternative scheme where all point-cloud data could be transmitted and processed at the master node.Secondly,the team member’s integrated odometry is transmitted.This allows the master node to compute the odometric relative pose since the prior landmark measurement data was incorporated;this is used to insert a relative pose factor and also give initial conditions for data association.Finally,the team member’s local map pose is transmitted.This is used by the master node to compute a map pose correction.This correction is sent back to the team member so that it knows it’s relative pose in the global map frame.This knowledge is needed so that the team member can interpret exploration goals correctly.The map coordinator maintains trajectories for each of the robots in the team.Mea-surements from each robot are merged into one global view of the landmarks.This is realized through a simple modification to the standard OmniMapper through duplica-tion of data structures tracking indexing data and pose information used for interaction with GTsam into arrays.This implementation potentially allows for an unlimited num-ber of team members to build a map together.4Most modern SLAM approaches use a pose graph[10]which is generated via laser scan matching in2D or point-cloud ICP in3D.This approach is effective for single robot mapping;however,it has some drawbacks for larger multirobot mapping.Scan matching and ICP algorithms are computationally intensive and matching across many robots would rapidly become intractable.Also,point cloud representations are large and their transport over a wireless link could be prohibitive if the link is limited in capacity due to mesh network routing or environmental interference.To address these limitations,our robots extract relevant,parsimonious features from the environment and transmit them to the master node.Each turtlebot in these experiments maps planar wall structures using a Microsoft Kinect sensor.Planar segments corresponding to walls are extracted from point clouds via a RANSAC[7]based algorithm[15].Points are uniformly sampled from the point cloud and any sufficiently large set of points coplanar with these three points are se-lected as a plane and are removed from the point cloud.This process is repeated until up to four planes are extracted or afixed number of iterations is reached.To improve the speed of plane extraction,the Kinect point cloud is computed at QQVGA(f rac18) resolution,which achieves˜1Hz frame rate.The Kinect sensor on each robot has a narrowfield-of-view which is not ideal for detecting exploration frontiers.To alleviate this problem,we incorporated a strategy by which each robot will rotate periodically to get a360degree view of its surroundings. This data is synchronized with robot odometry to synthesize a360degree laser scan. This synthesized laser scan is sent to the local mapper and forwarded to the global map-per.At the global mapper,it is linked to a trajectory pose element and used to populate an occupancy grid.This occupancy grid is re-computed after every map optimization so that a loop closure will result in a correct occupancy grid map.The frontier based explo-ration strategies detailed below use this occupancy grid tofind the boundary between clear and unknown grid cells.2.2Exploration StrategyEach robot team leader uses a frontier based exploration strategy similar to the one used in[17].An exploration frontier is defined on a costmap cellular decomposition where each cell has one of three labels:Clear,Obstacle,and Unknown.The costmap is initialized as Unknown.Costmap cells are set to Obstacle corresponding to locations where the Kinect sensor detects an obstacle in the environment.The cells on a line between the obstacle cell and the robot’s current location are set to Clear.Exploration frontiers are defined as Clear cells which are adjacent to at least one neighbor where the label is Unknown.The high level robot exploration goal allocation is centrally planned on the same workstation where the global map is constructed.There are many choices which can be made by the exploration planner when choosing which robot or group of robots should move towards an exploration goal.We have chosen to employ a greedy strategy by which the nearest robot or team is allocated to a goal instead of a more sophisticated traveling-salesman type of algorithm.We believe that this is appropriate because the exploration goals will change as the robots move through the environment;re-planning will be required after each robot or team reaches an exploration goal.5Fig.2.Global maps using the Reserve coordination algorithm described in this paper.2.3Coordination StrategyThe coordination strategy used between robot agents as well as the number of robots arethe independent variables in the experiments performed in this paper.The coordinationstrategy refers to the proportion of robots which are dispatched to each exploration goal.On one extreme,a single robot can be sent to explore a new goal;at the other extreme allavailable robots can be sent to a new rger robot teams sent to a new explorationgoal will improve availability of new agents at the location of new exploration goals arediscovered.The larger group has spare robots which can be quickly allocated to explorenew goals,such as those discovered when the team moves past a corridor intersectionor t-junction.If the group of robots allocated to a navigation goal is too large,then therobots can interfere with each other due to local reactive control of multiple agents withrespect to dynamic obstacles and limited space in corridors.The strategies selected fortesting trade off availability (robots are close and able to explore branching structurequickly)with non-interference (robots do not get in each other’s way).The first coordination algorithm is called Reserve .In this algorithm,all unallocatedrobots remain a the starting locations until new exploration goals are uncovered.Whena branching point is detected by an active robot,the closest reserve robot will be re-cruited into active status to explore the other path.This strategy has low availabilitybecause all of the reserve robots remain far away at the entrance;however,it has min-imal interference because the exploring robots will usually be further away from otherrobots.The second coordination algorithm is Divide and Conquer .In this strategy,the en-tire robot group follows the leader until a branching point is detected.The group splits in half,with the first n 2robots following the original leader,robot n 2+1is selected as theleader of the second group,and robots n 2+2through n are now members of its squad.Once there are n squads with one robot,no further divide operations can be made andnew exploration goals will only be allocated once a robot has reached a dead-end or6Fig.3.A map built by three robots using the Reserve cooperative mapping strategy. looped back into a previously explored area.This algorithm maximizes availability,but potentially causes significant interference between robots.An example3D map built by two robots as they approach a branch point can be seen infigure4(a).At this point,the robot team splits and each team member takes a separate path,as seen infigure4(b).The map shown is built concurrently with local maps built on each robot.The global map is used to establish a global frame of reference for robot collaboration message coordinates.(a)Two robots approach the intersection.(b)Two robots split and move past the in-tersectionFig.4.An illustration of the Divide and Conquer exploration strategy.As the robots approach an intersection,the team must split and recruit new partner robots from the reserved units.3ExperimentsThe setting for the multi-robot mapping task for this series of experiments consists of a team of robots being introduced into a single entrance in an unknown environment. Each robot is an inexpensive Willow Garage TurtleBot;a team of nine of these robots is shown infigure3.The TurtleBot was chosen for this application due to its low cost and7(a)A map built by seven robots in an experiment using the Reserve coopera-tive mapping strategy.(b)The same map shown from a different angle to demonstrate3Dplane features which are used for map landmarks.Fig.5.Global maps gathered by a team of seven mobile robots.8the ease of integrating large numbers of robots through ROS.The TurtleBot platform is based on the iRobot Create base.The robots make measurements of planes with a Kinect sensor,and use an onboard IMU together with odometry to estimate ego-motion.Fig.6.Our nine TurtleBots used in these experiments.We evaluated the performance of various robot coordination strategies in the multi-robot exploration and mapping task.An example scenario for the Divide and Conquer cooperative mapping strategy can be seen in the panorama image infigure3.Fig.7.An example scenario for the experiments described in this paper.Three teams of two robots are exploring the branching hallway structure in an office environment.In this illustration, the robots are using the Divide and Conquer cooperative mapping strategy.We performed a series of experiments to demonstrate the performance of our two cooperative mapping strategies.A total of6runs were performed for each cooperation strategy,team size,and starting location.For each experiment run,the TurtleBot team9 explored the environment from a wedge-shaped starting configuration,which can be seen infigure3.These experiments were performed in an office environment.In order to measure the exploration and mapping performance in each location,we chose spe-cific starting locations which are labeled Base1and Base2infigure3.These starting locations were chosen because the area around the robot teams could be blocked off so there is only one initial exploration frontier,directly in front of the lead robot.This ini-tial configuration was chosen to represent a breaching behavior which would be needed for implementation of collaborative mapping in a hostile environment.Fig.8.Our office environment where the experiments were performed.The areas labeled Base1 and Base2are the initial position of the robots.Red lines indicate artificial barricades to restrict the initial exploration of the robot teams to simulate a breach entrance into a hostile environment. 4ResultsWe performed a series of experiments for this paper which demonstrate team perfor-mance based upon coverage in a mapping task on an unknown office environment. Robot team sizes were varied from2to9robots.An map built with7robots at Turtle-Bots using the Reserve strategy is seen in Figure5(a).An image showing the samefinal global map from a side view demonstrates the3D plane features infigure2.3.Each of the collaboration strategy and robot team size experiments were performed from two starting locations.These starting locations are labeled Base1and Base2in figure3.A series of interesting locations was determined in advance by examining the buildingfloor-plan;these points of interest are also marked infigure3.Each experiment run gets a score based on how many of these points of interest are visited and mapped before a time limit is reached.This score represents the effectiveness of that algorithm and team size at providing coverage while exploring an unknown map.first experiment series from Base1in figure 3,both strategies achieve reducedexploration coverage per robot as the team size is increased,as can be seen in the graphsin figure 9.In this starting location,there is limited space to maneuver,so both strate-gies generate significant interference between robots trying to move to their goals.Inseveral instances,pairs of robots even crashed into each other due to the limited field-of-view of their sensors.We believe that the Divide and Conquer strategy results infigure 9(b)indicate that the team was slightly more effective than the Reserves strategyin figure 9(a).At the largest team size of 9robots,the Divide and Conquer strategyusually visited one additional point-of-interest more than the Reserves strategy.Addi-tional qualitative impressions are that the Divide and Conquer strategy explored thepoints-of-interest that it reached more quickly than with the Reserves strategy.For bothstrategies,the best team size appears to be 6robots in this starting location.(a)Reserves (b)Divide and ConquerFig.9.Results from the first starting areaIn the second set of experiments,the robot teams were placed in the starting arealabeled Base2in figure 3.As in the first experiment,the per-robot performance of bothstrategies decreased as the number of robots were increased.This series of experimentsdemonstrates a marked improvement of the Divide and Conquer strategy over the Re-serves strategy as can be seen in figure 10.The Divide and Conquer strategy causesmore robots to be making observations of exploration frontiers due to the fact thatgroups contain more than one robot.These additional observations of the frontier allowthe Divide and Conquer strategy to find exploration frontiers faster than the Reservesstrategy,and therefore explore more points-of-interest.The second experiment startedfrom an area where there is more room to maneuver.This allowed the Divide and Con-quer strategy to have less interference since the entire team moved together out of thestarting area into the larger area before any divide operations were performed.The Re-serves strategy still had to initially maneuver from the cramped starting location.As inthe first experiment,the Divide and Conquer strategy qualitatively explored the envi-ronment faster than the Reserves strategy.The best value for the number of robots is 6,which is the same value found in the first experiment.11(a)Reserves(b)Divide and ConquerFig.10.Results from the second starting area5DiscussionWe have presented experiments which evaluate two collaboration strategies which can be used by teams of mobile robots to map and explore an unknown environment.We have also evaluated the impact of the number of robots on coverage in the exploration and mapping task.Thefirst collaboration strategy,called Reserves keeps a pool of unallocated robots at the starting location.A new robot is activated when there are more exploration frontiers than currently active robots.This strategy was intended to minimize the amount of in-terference between robot agents since robots would be far away from each other during exploration.The results from our experiments do not indicate that this strategy results in less interference than other strategies since performance decreases more when more robots are added in some environments.The Reserves strategy is significantly slower at exploring the environment than other strategies.The second collaboration strategy,called Divide and Conquer has all available robots proceed in one large group.Once there are two exploration frontiers,at a corridor t-junction for example,the team will divide in half and each sub-team will follow one of the exploration frontiers.This process will be repeated with teams dividing in half each time they see branching structure in the environment.It was anticipated that this strategy would result in higher interference since robots would be maneuvering close together;however,the increased availability of robots near new exploration frontiers offsets this phenomenon.Divide and Conquer appears to be a more effective strategy than Reserves for ex-ploring and mapping an unknown environment.There are additional hybrid strategies which could now be considered such as the Buddy System,which modifies the Reserves strategy with teams of2robots instead of1.We believe that this strategy will mitigate much of the slowness of the Reserves strategy while still minimizing interference.12AcknowledgmentsThis work was made possible through generous support from the Army Research Lab (ARL)MAST CTA project,and The Boeing Corporation.References1.ARL:Army Research Lab Micro Autonomous Systems and Technology Collaborative Tech-nology Alliance MAST CTA./www/default.cfm?page=332(2006) 2.Bailey,T.,Durrant-Whyte,H.:Simultaneous localisation and mapping(SLAM):Part II stateof the art.Robotics and Automation Magazine(September2006)3.Chipalkatty,R.,Daepp,H.,Egerstedt,M.,Book,W.:Human-in-the-loop:Mpc for sharedcontrol of a quadruped rescue robot.In:Intelligent Robots and Systems(IROS),2011 IEEE/RSJ International Conference on.pp.4556–4561.IEEE(2011)4.Dellaert,F.:Square root SAM:Simultaneous localization and mapping via square root infor-mation smoothing.Robotics:Science and Systems(2005)5.Dellaert,F.,Kaess,M.:Square root SAM:Simultaneous localization and mapping via squareroot information smoothing.International Journal of Robotics Research(2006)6.Durrant-Whyte,H.,Bailey,T.:Simultaneous localisation and mapping(SLAM):Part I theessential algorithms.Robotics and Automation Magazine(June2006)7.Fischler,M.A.,Bolles,R.C.:Random sample consensus:A paradigm for modelfitting withapplications to image analysis and automated munications of the ACM 24(6),381–395(1981)8.Folkesson,J.,Christensen,H.:Graphical SLAM-a self-correcting map.IEEE InternationalConference on Robotics and Automation(2004)9.Fox,D.,Ko,J.,Konolige,K.,Limketkai,B.,Schulz,D.,Stewart,B.:Distributed multirobotexploration and mapping.Proceedings of the IEEE94(7),1325–1339(2006)10.Grisetti,G.,Grzonka,S.,Stachniss,C.,Pfaff,P.,Burgard,W.:Efficient estimation of accuratemaximum likelihood maps in3D.In:Intelligent Robots and Systems,2007.IROS2007.IEEE/RSJ International Conference on.pp.3472–3478.IEEE(2007)11.Heger,F.,Singh,S.:Sliding autonomy for complex coordinated multi-robot tasks:Analy-sis and experiments.In:Proceedings of Robotics:Science and Systems.Philadelphia,USA (August2006)12.Quigley,M.,Gerkey,B.,Conley,K.,Faust,J.,Foote,T.,Leibs,J.,Berger,E.,Wheeler,R.,Ng,A.:ROS:an open-source robot operating system.In:ICRA Workshop on Open Source Software(2009)13.Rogers,J.,Trevor,A.,Nieto,C.,Cunningham,A.,Paluri,M.,Michael,N.,Dellaert,F.,Chris-tensen,H.,Kumar,V.:Effects of sensory perception on mobile robot localization and map-ping.In:International Symposium on Experimental Robotics ISER(2010)14.Rogers III,J.G.,Trevor,A.J.B.,Nieto-Granda,C.,Christensen,H.I.:Simultaneous localiza-tion and mapping with learned object recognition and semantic data association.In:IEEE International Conference on Intelligent RObots and Systems(IROS)(2011)15.Rusu,R.B.,Cousins,S.:3D is here:Point Cloud Library(PCL).In:IEEE International Con-ference on Robotics and Automation(ICRA).Shanghai,China(20112011)16.Trevor,A.J.B.,Rogers III,J.G.,Nieto-Granda,C.,Christensen,H.I.:Tables,counters,andshelves:Semantic mapping of surfaces in3D.In:IROS Workshop on Semantic Mapping and Autonomous Knowledge Acquisition(2010)17.Vincent,R.,Fox,D.,Ko,J.,Konolige,K.,Limketkai,B.,Morisset,B.,Ortiz,C.,Schulz,D.,Stewart,B.:Distributed multirobot exploration,mapping,and task allocation.Annals ofMathematics and Artificial Intelligence52(2),229–255(2008)13 18.Zheng,K.,Glas,D.,Kanda,T.,Ishiguro,H.,Hagita,N.:How many social robots can oneoperator control?In:Proceedings of the6th international conference on Human-robot inter-action.pp.379–386.ACM(2011)。
AGV简介——精选推荐
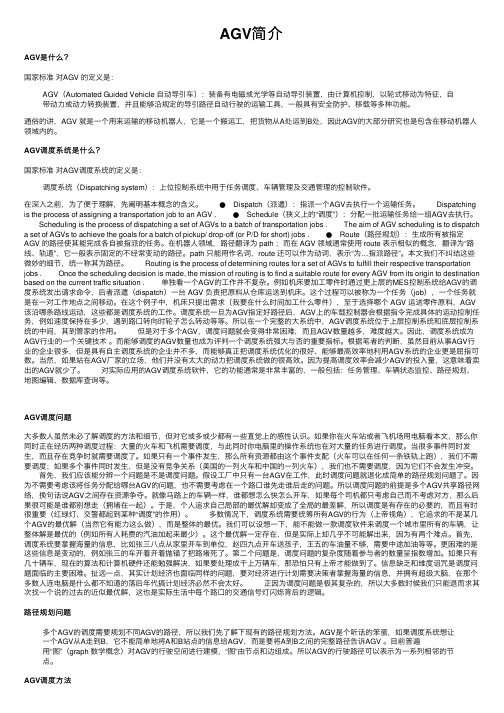
AGV简介AGV是什么?国家标准对AGV 的定义是:AGV(Automated Guided Vehicle ⾃动导引车):装备有电磁或光学等⾃动导引装置,由计算机控制,以轮式移动为特征,⾃带动⼒或动⼒转换装置,并且能够沿规定的导引路径⾃动⾏驶的运输⼯具,⼀般具有安全防护、移载等多种功能。
通俗的讲,AGV 就是⼀个⽤来运输的移动机器⼈,它是⼀个搬运⼯,把货物从A处运到B处,因此AGV的⼤部分研究也是包含在移动机器⼈领域内的。
AGV调度系统是什么?国家标准对AGV调度系统的定义是:调度系统(Dispatching system):上位控制系统中⽤于任务调度、车辆管理及交通管理的控制软件。
在深⼊之前,为了便于理解,先阐明基本概念的含义。
● Dispatch(派遣):指派⼀个AGV去执⾏⼀个运输任务。
Dispatching is the process of assigning a transportation job to an AGV . ● Schedule(狭义上的“调度”):分配⼀批运输任务给⼀组AGV去执⾏。
Scheduling is the process of dispatching a set of AGVs to a batch of transportation jobs . The aim of AGV scheduling is to dispatch a set of AGVs to achieve the goals for a batch of pickup/ drop-off (or P/D for short) jobs . ● Route(路径规划):⽣成所有被指定AGV 的路径使其能完成各⾃被指派的任务。
在机器⼈领域,路径翻译为 path ;⽽在 AGV 领域通常使⽤ route 表⽰相似的概念,翻译为“路线、轨道”,它⼀般表⽰固定的不经常变动的路径。
path 只能⽤作名词,route 还可以作为动词,表⽰“为…指派路径”。
协作机器人市场分析报告
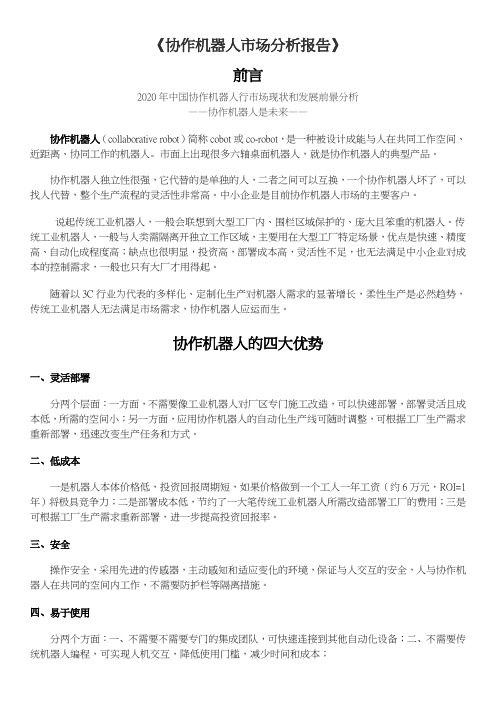
《协作机器人市场分析报告》前言2020年中国协作机器人行市场现状和发展前景分析——协作机器人是未来——协作机器人(collaborative robot)简称cobot或co-robot,是一种被设计成能与人在共同工作空间、近距离、协同工作的机器人。
市面上出现很多六轴桌面机器人,就是协作机器人的典型产品。
协作机器人独立性很强,它代替的是单独的人,二者之间可以互换,一个协作机器人坏了,可以找人代替,整个生产流程的灵活性非常高。
中小企业是目前协作机器人市场的主要客户。
说起传统工业机器人,一般会联想到大型工厂内、围栏区域保护的、庞大且笨重的机器人。
传统工业机器人,一般与人类需隔离开独立工作区域,主要用在大型工厂特定场景,优点是快速、精度高、自动化成程度高;缺点也很明显,投资高,部署成本高,灵活性不足,也无法满足中小企业对成本的控制需求,一般也只有大厂才用得起。
随着以3C行业为代表的多样化、定制化生产对机器人需求的显著增长,柔性生产是必然趋势,传统工业机器人无法满足市场需求,协作机器人应运而生。
协作机器人的四大优势一、灵活部署分两个层面:一方面,不需要像工业机器人对厂区专门施工改造,可以快速部署,部署灵活且成本低,所需的空间小;另一方面,应用协作机器人的自动化生产线可随时调整,可根据工厂生产需求重新部署,迅速改变生产任务和方式。
二、低成本一是机器人本体价格低,投资回报周期短,如果价格做到一个工人一年工资(约6万元,ROI=1年)将极具竞争力;二是部署成本低,节约了一大笔传统工业机器人所需改造部署工厂的费用;三是可根据工厂生产需求重新部署,进一步提高投资回报率。
三、安全操作安全,采用先进的传感器,主动感知和适应变化的环境,保证与人交互的安全,人与协作机器人在共同的空间内工作,不需要防护栏等隔离措施。
四、易于使用分两个方面:一、不需要不需要专门的集成团队,可快速连接到其他自动化设备;二、不需要传统机器人编程,可实现人机交互,降低使用门槛,减少时间和成本;我国协作机器人行业的发展阶段我国协作机器人行业处于深度发展阶段,2014年协作机器人在中国兴起,主要是以国外品牌UR、Rethink为主,2015年以来国产协作机器人新松、达明、遨博、大族激光等企业亦正积极布局协作机器人市场。
数字化创新下的产品市场多点竞争模型
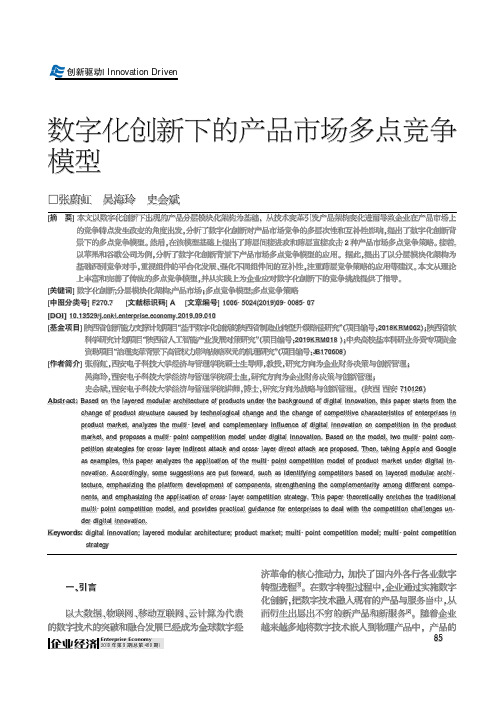
数字化创新下的产品市场多点竞争模型□张蔚虹吴海玲史会斌的竞争特点发生改变的角度出发,分析了数字化创新对产品市场竞争的多层次性和互补性影响,提出了数字化创新背景下的多点竞争模型。
然后,在该模型基础上提出了跨层间接进攻和跨层直接攻击2种产品市场多点竞争策略。
接着,以苹果和谷歌公司为例,分析了数字化创新背景下产品市场多点竞争模型的应用。
据此,提出了以分层模块化架构为基础识别竞争对手,重视组件的平台化发展、强化不同组件间的互补性,注重跨层竞争策略的应用等建议。
本文从理论上丰富和完善了传统的多点竞争模型,并从实践上为企业应对数字化创新下的竞争挑战提供了指导。
[关键词]数字化创新;分层模块化架构;产品市场;多点竞争模型;多点竞争策略[中图分类号]F270.7[文献标识码]A[文章编号]1006-5024(2019)09-0085-07[DOI]10.13529/ki.enterprise.economy.2019.09.010[基金项目]陕西省创新能力支撑计划项目“基于数字化创新的陕西省制造业转型升级路径研究”(项目编号:2018KRM062);陕西省软科学研究计划项目“陕西省人工智能产业发展对策研究”(项目编号:2019KRM018);中央高校基本科研业务费专项资金资助项目“治理变革背景下高管权力影响战略双元的机理研究”(项目编号:JB170608)[作者简介]张蔚虹,西安电子科技大学经济与管理学院硕士生导师,教授,研究方向为企业财务决策与创新管理;吴海玲,西安电子科技大学经济与管理学院硕士生,研究方向为企业财务决策与创新管理;史会斌,西安电子科技大学经济与管理学院讲师,博士,研究方向为战略与创新管理。
(陕西西安710126)Abstract:Based on the layered modular architecture of products under the background of digital innovation,this paper starts from thechange of product structure caused by technological change and the change of competitive characteristics of enterprises in product market,analyzes the multi-level and complementary influence of digital innovation on competition in the productmarket,and proposes a multi-point competition model under digital innovation.Based on the model,two multi-point com ⁃petition strategies for cross-layer indirect attack and cross-layer direct attack are proposed.Then,taking Apple and Googleas examples,this paper analyzes the application of the multi-point competition model of product market under digital in ⁃novation.Accordingly,some suggestions are put forward,such as identifying competitors based on layered modular archi ⁃tecture,emphasizing the platform development of components,strengthening the complementarity among different compo ⁃nents,and emphasizing the application of cross-layer competition strategy.This paper theoretically enriches the traditionalmulti-point competition model,and provides practical guidance for enterprises to deal with the competition challenges un ⁃der digital innovation.Keywords:digital innovation;layered modular architecture;product market;multi-point competition model;multi-point competitionstrategy一尧引言以大数据、物联网、移动互联网、云计算为代表的数字技术的突破和融合发展已经成为全球数字经济革命的核心推动力,加快了国内外各行各业数字转型进程[1]。
弱通信条件下的多水下机器人协调方法研究的开题报告
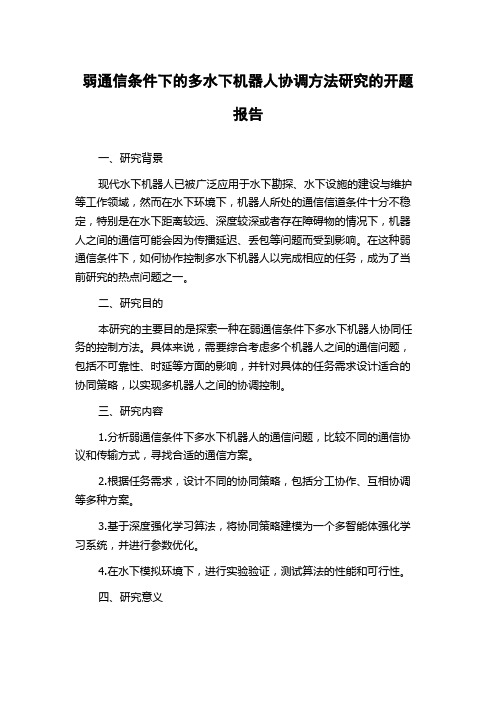
弱通信条件下的多水下机器人协调方法研究的开题报告一、研究背景现代水下机器人已被广泛应用于水下勘探、水下设施的建设与维护等工作领域,然而在水下环境下,机器人所处的通信信道条件十分不稳定,特别是在水下距离较远、深度较深或者存在障碍物的情况下,机器人之间的通信可能会因为传播延迟、丢包等问题而受到影响。
在这种弱通信条件下,如何协作控制多水下机器人以完成相应的任务,成为了当前研究的热点问题之一。
二、研究目的本研究的主要目的是探索一种在弱通信条件下多水下机器人协同任务的控制方法。
具体来说,需要综合考虑多个机器人之间的通信问题,包括不可靠性、时延等方面的影响,并针对具体的任务需求设计适合的协同策略,以实现多机器人之间的协调控制。
三、研究内容1.分析弱通信条件下多水下机器人的通信问题,比较不同的通信协议和传输方式,寻找合适的通信方案。
2.根据任务需求,设计不同的协同策略,包括分工协作、互相协调等多种方案。
3.基于深度强化学习算法,将协同策略建模为一个多智能体强化学习系统,并进行参数优化。
4.在水下模拟环境下,进行实验验证,测试算法的性能和可行性。
四、研究意义本研究所开发的多水下机器人协同控制方法,可以有效应对弱通信条件下机器人的控制问题,提高掌控机器人集群的能力和效益,缩短任务完成时间,拓展水下机器人的应用领域。
五、预期成果完成一篇研究论文,探索一种在弱通信条件下多水下机器人协同任务的控制方法。
根据具体任务需求,设计不同的协同策略,并建模为多智能体强化学习系统,进行实验验证后获得丰富的实验数据,并分析方法的性能和可行性。
最终应用所提出的方法实现水下机器人任务的实际应用。
六、研究方法本研究主要借鉴现有的多智能体强化学习方法,根据任务需求设计不同的协同策略,并将其建模为多智能体强化学习系统。
在水下模拟环境下,进行实验验证,并分析实验结果。
具体方法分为以下几步:1.调研和分析现有的多智能体强化学习方法和最新的水下机器人协同研究成果,以此为依据设计协同策略。
Multi-robot repeated area coverage
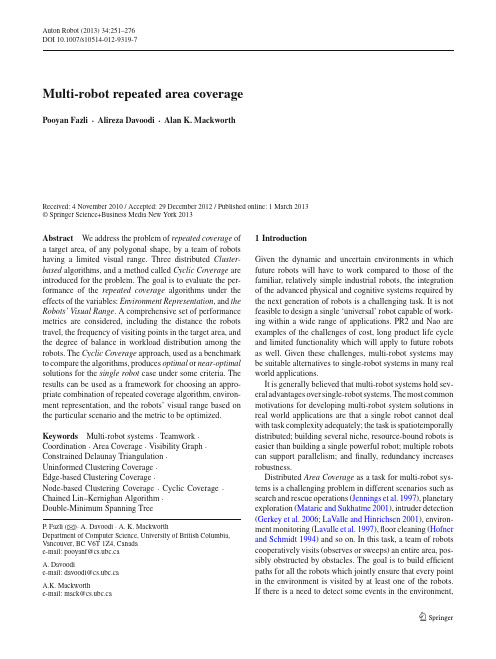
Auton Robot(2013)34:251–276DOI10.1007/s10514-012-9319-7Multi-robot repeated area coveragePooyan Fazli·Alireza Davoodi·Alan K.MackworthReceived:4November2010/Accepted:29December2012/Published online:1March2013©Springer Science+Business Media New York2013Abstract We address the problem of repeated coverage of a target area,of any polygonal shape,by a team of robots having a limited visual range.Three distributed Cluster-based algorithms,and a method called Cyclic Coverage are introduced for the problem.The goal is to evaluate the per-formance of the repeated coverage algorithms under the effects of the variables:Environment Representation,and the Robots’Visual Range.A comprehensive set of performance metrics are considered,including the distance the robots travel,the frequency of visiting points in the target area,and the degree of balance in workload distribution among the robots.The Cyclic Coverage approach,used as a benchmark to compare the algorithms,produces optimal or near-optimal solutions for the single robot case under some criteria.The results can be used as a framework for choosing an appro-priate combination of repeated coverage algorithm,environ-ment representation,and the robots’visual range based on the particular scenario and the metric to be optimized. Keywords Multi-robot systems·Teamwork·Coordination·Area Coverage·Visibility Graph·Constrained Delaunay Triangulation·Uninformed Clustering Coverage·Edge-based Clustering Coverage·Node-based Clustering Coverage·Cyclic Coverage·Chained Lin–Kernighan Algorithm·Double-Minimum Spanning TreeP.Fazli(B)·A.Davoodi·A.K.MackworthDepartment of Computer Science,University of British Columbia, Vancouver,BC V6T1Z4,Canadae-mail:pooyanf@cs.ubc.caA.Davoodie-mail:davoodi@cs.ubc.caA.K.Mackworthe-mail:mack@cs.ubc.ca 1IntroductionGiven the dynamic and uncertain environments in which future robots will have to work compared to those of the familiar,relatively simple industrial robots,the integration of the advanced physical and cognitive systems required by the next generation of robots is a challenging task.It is not feasible to design a single‘universal’robot capable of work-ing within a wide range of applications.PR2and Nao are examples of the challenges of cost,long product life cycle and limited functionality which will apply to future robots as well.Given these challenges,multi-robot systems may be suitable alternatives to single-robot systems in many real world applications.It is generally believed that multi-robot systems hold sev-eral advantages over single-robot systems.The most common motivations for developing multi-robot system solutions in real world applications are that a single robot cannot deal with task complexity adequately;the task is spatiotemporally distributed;building several niche,resource-bound robots is easier than building a single powerful robot;multiple robots can support parallelism;andfinally,redundancy increases robustness.Distributed Area Coverage as a task for multi-robot sys-tems is a challenging problem in different scenarios such as search and rescue operations(Jennings et al.1997),planetary exploration(Mataric and Sukhatme2001),intruder detection (Gerkey et al.2006;LaValle and Hinrichsen2001),environ-ment monitoring(Lavalle et al.1997),floor cleaning(Hofner and Schmidt1994)and so on.In this task,a team of robots cooperatively visits(observes or sweeps)an entire area,pos-sibly obstructed by obstacles.The goal is to build efficient paths for all the robots which jointly ensure that every point in the environment is visited by at least one of the robots. If there is a need to detect some events in the environment,area coverage guaranteesfinding all of them in the target area.There is confusion in the literature regarding the terms Exploration and Coverage.To clarify the problem defini-tion,we note that in exploration,we have an unknown envi-ronment in which a team of robots is trying to build a map of the area together(Yamauchi1998;Burgard et al.2000; Simmons et al.2000;Howard2006;Batalin and Sukhatme 2003).On the other hand,in a coverage problem,the map of the environment may be known or unknown and the team aims to cooperatively visit the whole area with their sensors or physical actuators.In other words,building a map of the environment is not the ultimate aim of the coverage mission.A similar class of problems is Boundary Coverage in which the aim is inspection of the boundaries of a target area and the obstacles inside by a team of robots rather than complete coverage of the area(Williams and Burdick2006; Amstutz et al.2008).There are two classes of coverage problems:•Single Coverage:The aim is to cover the target area until all the accessible points of interest in the environment have been visited at least once,while minimizing the time,distance traversed by the robots,and the number of visits to the points(Fazli et al.2010a,b;Hazon and Kaminka2008;Rekleitis et al.2008).•Repeated Coverage:The goal is to cover all the acces-sible points of interest in the environment repeatedly over time,while maximizing the frequency of visit-ing points in the target area,minimizing the weighted average event/intruder detection time,minimizing the sum/maximum length of the paths/tours generated for the robots,or balancing the workload distribution among the robots.Visiting the points in the area can be performed with uniform or non-uniform frequency,depending on the priorities of different parts of the area1.Several research communities including robotics/agents (Machado et al.2002),sensor networks(Gasparri et al.2008), operations research(Toth and Vigo2002b)and computa-tional geometry(Carlsson et al.1993)work on variants of the repeated coverage problem.In operations research,the Vehicle Routing Problem has some similarities to the repeated coverage scenarios(Liu and Shen1999).In this problem,a number of vehicles deliver goods located at a central depot to a set of geographically dispersed customers.The objective is to minimize the total distance travelled.In the Vehicle Routing Problem with Time Windows,the target locations have time windows within which the deliveries(or visits)must be made(Desrochers 1In this paper,we use the terms’coverage’and’repeated coverage’interchangeably.et al.1992),and in the Capacitated Vehicle Routing Prob-lem,the vehicles have limited carrying capacity for the goods that must be delivered(Toth and Vigo2002a).In computational geometry,this problem originates from the Art Gallery Problem(O’Rourke1987;Urrutia2000)and its variant for mobile guards,the(Multi)Watchman Route Problem(Chin and Ntafos1986;Packer2008;Faigl2010).In the Art Gallery Problem,the goal is tofind a minimum num-ber of static guards(control points)which can jointly cover a priori known simple polygonal workspace under differ-ent restrictions.On the other hand,in the(Multi)Watchman Route Problem the objective is to compute routes(closed curves)watchmen should take,such that any point inside the polygon is visible from at least one point along one route. Pursuit-Evasion is another closely related problem studied in both the computational geometry and the robotics commu-nities.In this task,one or more searchers move throughout a given target area in order to guarantee the detection of all the evaders,which can move arbitrarily fast(Gerkey et al.2006; Vidal et al.2002).In Pursuit-Evasion scenarios the searchers do not necessarily cover the entire target area.In the robotics community,most research in this area is carried out under the rubric of Area/Boundary/Perimeter Patrolling.In Sect.2we elaborate on the related literature regarding the Multi-Agent/Robot Patrolling scenarios.Overview and Contributions:In this study,we define four optimization metrics for comparing the efficiency of the repeated coverage algorithms.These metrics are:•Total Path Length(TPL)the robots traverse in the target area.•Total Average Visiting Period(TAVP)of the points of interest in the target area.•Total Worst Visiting Period(TWVP)of the points of inter-est in the target area.•Balance in Workload Distribution(BWD)among the robots.These metrics will be discussed in detail in Sect.3.Inter-estingly,it is impossible even to develop polynomial approx-imation algorithms,when optimizing each of the metrics of the repeated coverage problem,unless P=N P(Packer 2008).Furthermore,optimizing all these metrics simultane-ously is another challenge,because some are mutually con-flicting in the coverage mission.These considerations require us conduct an extensive experimental analysis to evaluate the performance of the algorithms.The contributions of this paper are as follows:1.We present an approach to modeling the environmentusing graph-based methods considering the limited visualrange of the robots.To this end,two environment modeling approaches are developed based on the Visibil-ity Graph and the Constrained Delaunay Triangulation.2.Three Cluster-based algorithms are introduced for thedistributed repeated coverage problem,differing as to how they cluster the graph,namely:the Uninformed Clus-tering Coverage,the Edge-based Clustering Coverage, and the Node-based Clustering Coverage algorithms. 3.An algorithm called Cyclic Coverage is introduced andused as a benchmark to compare the performance of the repeated coverage algorithms.The algorithmfinds the shortest tour on the graph similar to solving a Travelling Salesman Problem(TSP).We show that even though the Cyclic Coverage approach can produce optimal or near-optimal solutions for a single robot case under some par-ticular metrics;however,it is not always the best solution when extending the problem to multi-robot scenarios.4.The effect of different environment representations onthe performance of the repeated coverage algorithms are examined.5.The effect of varying the robots’visual range on theperformance of the repeated coverage algorithms is investigated.The results can be used as a framework for choos-ing an appropriate combination of repeated coverage algo-rithm,environment representation,and the robots’visual range based on the particular scenario and the metric to be optimized.2Background and reviewChoset(2001)divides the approaches for area coverage based on the methods they employ for decomposing the area: Exact Cellular Decomposition,and Approximate Cellular Decomposition.In the Exact Cellular Decomposition(e.g.graph-based methods),the area is divided to a set of non-overlapping regions whose union covers the whole environment.In the Approximate Cellular Decomposition(e.g.grid-based meth-ods),the environment is divided into cells which are all the samesizeandshape.However,cellsthatarepartiallyoccluded by obstacles or close to the boundary are discarded,therefore the union of the cells only approximates the target area.The methods based on the Approximate Cellular Decom-position have limitations since they do not consider the structure of the environment and as a result are unable to handle partially occluded cells or cover areas close to the boundaries in continuous spaces.In contrast,methods based on the Exact Cellular Decomposition do not suffer those restrictions.However,while traversing the graph guarantees covering the whole environment in continuous spaces,the path may include many redundant movements.Although there is a wide body of literature for sin-gle coverage scenarios(Choset2001;Agmon et al.2008a; Rekleitis et al.2008;Gabriely and Rimon2011;Zheng et al. 2005;Kurabayashi et al.1996;Batalin and Sukhatme2002), repeated coverage has not received the same attention.Two classes of the repeated coverage problem in the literature are: 1)Area Patrolling,and2)Boundary or Perimeter Patrolling (Open or Closed Polylines),and each is divided into:•Optimization-based repeated coverage,inwhichtheteam’s goal is to optimize some criteria,for example minimizing the average or worst frequency of visiting the points of interests in the target area,minimizing the total path tra-versed by the robots in the environment,or balancing the workload distribution among the robots.Optimization-based repeated coverage is the focus of this paper.•Adversarial repeated coverage,in which the team’s goal is to maximize the probability of detecting an adversary or multiple adversaries trying to penetrate the environment mainly through the patrol paths.2.1Optimization-based repeated coverage Machadoetal.(2002)studiedseveralarchitecturesforrepeated coverage in non-weighted graphs(i.e.the distance between two adjacent nodes is uniform).The goal was to minimize the time gap between two visits to the same node.The proposed architectures differ on various parameters such as agent type (reactive or goal-oriented),agent communication(central-ized,peer-to-peer,flag-based,or no-communication),coor-dination scheme(centralized vs.distributed),agent percep-tion(local vs.global),and decision-making(random selec-tion vs.goal-oriented selection).They showed that the Con-scientious Reactive Agents architecture outperforms the other multi-agentarchitectures.Anagentin Conscientious Reactive Agents chooses a node to visit from its neighbourhood with the highest time of being unvisited relative to the agent’s own visits rather than all the other agents’visits.There is no com-munication among the agents.The approach is generalized to weighted graphs in Almeida et al.(2004)Santana et al.(2004)studied adaptive agents that learn to patrol weighted graphs to minimize the time intervals between visits to the nodes,using Reinforcement Learn-ing(RL)techniques.A Markov Decision Process(MDP) formalism was used to model the patrolling problem,and the challenge was to define a state and action space for each agent individually,and to develop proper models of instantaneous rewards which could lead to satisfactory long term performance.The Q-Learning algorithm was used to train the agents,which proved to be computationally expen-sive.Aproblemwithsomeoftheexistingempiricalstudiesinthe field of area patrolling is the lack of a comprehensive popula-tion of environment maps in the experiments.In the works byAlmeida et al.(2004)and Machado et al.(2002)only six maps were used to evaluate the coverage algorithms,two of which have almost75%similarity.In two other maps called‘circu-lar’and‘corridor’,only one representation of the environment (i.e.a chain)is possible due to the structure of the environ-ments.Santana et al.(2004)also used two very similar maps to evaluate their proposed patrolling algorithm.Moreover,in none of the above patrolling tasks,did the authors provide details on how the graph is built to represent the environment. They typically presume the existence of a graph which is not a complete model of the environment,just a rough approxima-tion of it.The proposed architectures also consider the agents as points with no extent or limit on visual range,so the prob-lem dealt with is reduced to a graph exploration/coverage task rather than an area coverage scenario.This paper,on the other hand,buildsacompletemodeloftheenvironmentconsidering thelimitedvisualrangeoftherobots.Moreover,wewillinves-tigate the effect of different representations of the target area and varying the robots’visual range on the performance of the repeated coverage algorithms with extensive experiments on a set of carefully selected maps.Elmaliach et al.(2009)proposed a centralized algo-rithm which guarantees optimal uniform frequency,i.e.,all cells are visited with maximal and uniform frequency in a non-uniform,grid environment.As mentioned above,grid-based representations have limitations in handling partially occluded cells or cover areas close to the boundaries in con-tinuous spaces.Also,one of the limitations of the proposed approach is the requirement for a corridor’s size in the envi-ronment to be at least twice the size of the robot in order to be covered.Our algorithms,on the other hand,guarantee complete coverage of the area.Elmaliach et al.(2008)also addressed the problem of frequency-based patrolling of open polylines(e.g.,as in open-ended fences),where the two endpoints of the polylines are not connected.Jensen et al.(2011)extended Elmaliach et al.’s work on patrolling open polylines,with a focus on maintain-ing the patrol over the long-term.They accomplish this task by replacing the robots having power level below a thresh-old with some reserve robots.Patrolling open perimeters is challenging because robots must revisit the just visited areas when they reach an endpoint and turn back.Boardman et al. (2010)presented a distributed boundary tracking controller for multi-robot systems.Within this system,the boundary is partitioned into sub-segments,each allocated to a robot,such that the workload is balanced among the robots.They also aimed to minimize the phase difference between the robots, to limit the size of the gap created between the robots.2.2Adversarial repeated coverageSome work considered the existence of adversary agents in the workspace,where the goal is to maximize the detection rate of the intruder(s)in the work space.The main idea behind these patrolling strategies is to use non-deterministic,prob-abilistic algorithms in order to avoid static patrolling pat-terns which,in an adversarial scenario,could be exploited by intruders.Sak et al.(2008)considered the case of multi-agent patrol in adversarial environments in general graphs.The authors assumed three types of intruders in the environment:a ran-dom intruder,an intruder that waits until the patrolling agent leaves a node to penetrate the area,and an statistical intruder that collects statistics on the period between visits to a ran-dom node and predicts the timing of the next safe visit to the node.Some patrolling algorithms were experimentally evaluated by simulation.The results showed that no patrol strategy was optimal for all the possible adversaries.Ahmadi et al.(2006)addressed the problem of multi-robot repeated coverage of a grid-based target area in order to detect a set of events of interest.The frequency of the events occur-rence in the environment can possibly be non-uniform.Thus, the robots should visit the points with non-uniform frequency. The main contribution of the paper is an online area partition-ing method among the robots through a negotiation mecha-nism,which is adaptive to non-uniform frequency of event occurrence in the target area.Guo et al.(2007)studied a centralized multi-robot system for patrolling continuous environments.In this paper,the area is partitioned into sub-regions using a Voronoi Diagram. Robots are then distributed from their initial positions to their sub-regions,andfinally,each robot patrols its sub-region in order to detect a possible intrusion of an adversary agent into that sub-region.Agmon et al.(2008b)studied patrolling a cyclic bound-ary,in which the robots’goal is to maximize their rewards by detecting an adversary agent,which attempts to penetrate through a point on the boundary unknown to the robots.In their scenario,the full-knowledge adversary knows the loca-tion of the robots and the patrol strategy and needs a time interval of length t to accomplish the intrusion.They also examined the case of a zero-knowledge adversary(Agmon et al.2008c)and a partial-knowledge adversary(Agmon et al. 2009b)in perimeter patrolling.The uncertainty in the robots’perception was investigated in(Agmon et al.2009a),in which the ability to detect the intruders decreased as the distance grew.Czyzowicz et al.(2011)addressed the same problem using a team of verable speed robots.Fazli and Mackworth(2012a,b)addressed the problem of repeated coverage by a team of robots of the boundaries of a target area and the structures inside it.The robots have lim-ited visual and communication range.Events may occur on any parts of the boundaries and may have different impor-tance weights.In addition,the boundaries of the area and the structures are heterogeneous,so that events may appear with varying probabilities on different parts of the boundary,and these probabilities may change over time.The goal is to maximize the reward by detecting the maximum number of events,weighted by their importance,in minimum time. The reward a robot receives for detecting an event depends on how early the event is detected.Girard et al.(2004)studied a centralized system composed of multiple unmanned air vehicles patrolling a border area. The border is represented as a continuous two-dimensional region divided in sub-regions.Each sub-region is assigned to an air vehicle that repeatedly patrols it with a spiral trajectory to detect the possible intrusions in that sub-region.3Problem definition and preliminariesThe problem is to cover the environment repeatedly over time using a given number of robots.To this end,we make the following assumptions.Assumption1The environment boundary is a known2D simple polygon containing static polygonal obstacles. Assumption2Each robot,in the set of robots R,is repre-sented by a point in the environment.This assumption reduces the problem to covering a polyg-onal environment by a team of point robots without loss of generality.This follows from using the standard approach to robot motion planning in an environment with polygonal obstacles for the computation of the configuration space.In this case,configuration space is the set of all admissible posi-tions of the robot,i.e.the Minkowski sum(Skiena1998)of the set of obstacles and the boundary of the environment with the shape of the robotAssumption3The robots are presumed to have a360◦field of view and a predefined circular limit of visual range. Assumption4The robots are homogeneous,with the same speed,and can move in any direction.In order to evaluate the coverage mission,some metric criteria need to be determined,but before that we introduce some basic definitions:•Full Single Coverage:all the robots traverse the paths assigned to them just once.•Visiting Period(VP):the time interval between two visits to a point of interest in the target area.A point of interest can have more than one Visiting Period,due to the pos-sibility that the point may be visited more than once in different time intervals by one or more than one robot ina Full Single Coverage.For example,in Fig.1,point A(shown by the red dot)has three Visiting Periods,two are determined by the black robot/tour and one is determined by the blue robot/tour2.2Allfigures in this paper are best viewed in color.Fig.1Visiting Period and Visiting Frequency•Average Visiting Period(AVP):the average of the Visiting Periods of a point of interest.•Worst Visiting Period(WVP):the maximum period of time it takes a point of interest to be re-visited in the target area.•Visiting Frequency(VF):the number of visits to a point of interest by a single robot in a Full Single Coverage.If a point of interest is visited by more than one robot in a Full Single Coverage,the point will have more than one Visiting Frequency,each associated with a different robot.For example,in Fig.1,point A has two Visiting Frequencies,one is determined by the black robot/tour and the other is determined by the blue robot/tour.The Visiting Frequency of point A on the black tour is2and on the blue tour is1.The repeated coverage algorithms will be evaluated based on the following metrics:•Total Path Lengths(TPL):the sum of the lengths of the paths assigned to the robots in order to have a Full Single Coverage.•Total Average Visiting Period(TAVP):the average of the Average Visiting Periods of all the points of interest in the target area.•Total Worst Visiting Period(TWVP):the maximum Worst Visiting Period of all the points of interests in the target area.•Balance in Workload Distribution(BWD):the degree of balance in the workload distribution among a team of robots.A workload distribution is completely balanced if the standard deviation of the lengths of the constructed paths for the robots is zero:the paths assigned to the robots all have equal lengths.In this study,the aim is to minimize TPL,TAVP,and TWVP and to maximize BWD in the repeated coverage scenario.3.1Computing the evaluation metricsThe paths of the robots in the target area may either not overlap,that is,there is no common Point of Interest among(a )No n -o v erl a pped Pa t h s(b)O v erl a pped Pa t h sFig.2Non-overlapped versus overlapped paths for two robotsthe robots’paths (as shown in Fig.2),or may overlap,that is,there are some common Points of Interest among the robots’paths (e.g.red dots shown in Fig.2b).Considering this,the evaluation metrics are defined as below:T P L =|R | i =1Length (Path (r i )).(1)|R |is the number of robots,Path (r i )is the path built forrobot r i ,and Length (Path (r i ))is the length of the path.T AV P =node ∈PoIAV P (node ),PoI =Points of Interest ,(2)whereAV P (node )=Length (Path (r i ))V F i (node ),i ∈{1,2,...,|R |},node ∈Path (r i ),if node is not a common Point of Interest among the robots’paths,orAV P (node )=1 i V Fi (node )Length (Path (r i )),i ∈{1,2,...,|R |},node ∈Path (r i ),if node is a common Point of Interest among the robots’overlapped paths.V F i (node )is the node Visiting Frequency in Path (r i ).T W V P =max node ∈PoI (W V P (node )),(3)whereW V P (node )=max {V P i (node )},node ∈Path (r i ),if node is not a common Point of Interest among the robots’paths,orW V P (node )=min i =1,2,...,|R |{max {V P i (node )}},node ∈Path (r i ),if node is a common Point of Interest among the robots’overlapped paths.{V P i (node )}is the set of all the Visiting Periods of the node in Path (r i ).In computing the WVP of a Point of Interest which is com-mon among the robots’paths,we first calculate the maximum Visiting Period of the point in each robot’s path,and then choose the minimum of the maximum values.Recall thateach Point of Interest can belong to the path of more than one robot,and can also have more than one Visiting Period in each robot’s path.BW D (Paths )=(1−ST D ({Length (Path (r i ))|i =1,2,...,|R |})ST D ( T P L ,α1,α2,...,α|R |−1|αi =0 ))×100,(4)where ST D (.)is the population standard deviation,and Paths = Path (r 1),Path (r 2),...,Path (r |R |)is the |R |paths created for the |R |robots.For the case of one robot,we assume that BW D (Paths )=100.In BW D ’s compu-tation,ST D ({Length (Path (r i ))|i =1,2,...,|R |})is the population standard deviation of the set of paths created for the robots,and ST D (T P L ,α1,α2,...,α|R |−1|αi =0 )is the worst case scenario,in which one robot is in charge of the whole task,i.e.T P L ,and the other robots are idle with zero path length (α1,α2,...,α|R |−1).3.2Stages of the repeated coverage algorithmsThe different stages of the proposed repeated coverage algo-rithms are as follows:1.The locations of static guards (Points of Interest)required to cover visually a given 2D environment are determined,allowingforthelimitedrangeoftherobots’vision(Sect.4).2.A graph is built on the guards and the obstacles based on either the Visibility Graph or the Constrained Delaunay Triangulation (Sect.5).3.The graph is reduced to either the Reduced-Vis or the Reduced-CDT representation (Sect.6).4.Cluster-based Coverage Algorithms:The Reduced Graph is partitioned into as many clusters as the number of robots.To this end,three different clustering algorithms are introduced,namely:Uninformed Clustering ,Edge-based Clustering ,and Node-based Clustering .Finally,a tour is built for each robot on the clustered Reduced Graph .For this purpose,two tour building algorithms are proposed,namely:Double-Minimum Spanning Tree ,and the Chained Lin–Kernighan algorithms (Sect.7).5.Cyclic Coverage Algorithm:Cyclic Coverage finds the shortest tour on the whole VG or CDT graph,passing through all the static guards ,and then distributes the robots equidistantly around it (Sect.8).Since the problem is a repeated coverage scenario,we can ignore the initial cost of moving the robots from their initial locations to their assigned paths in the target area,as that time is negligible compared to the recurring patrol time.In the following sections,we will explain the different stages of the proposed algorithms for repeated coverage of a target area in detail.。
分数阶多机器人的领航-跟随型环形编队控制

第38卷第1期2021年1月控制理论与应用Control Theory&ApplicationsV ol.38No.1Jan.2021分数阶多机器人的领航–跟随型环形编队控制伍锡如†,邢梦媛(桂林电子科技大学电子工程与自动化学院,广西桂林541004)摘要:针对多机器人系统的环形编队控制复杂问题,提出一种基于分数阶多机器人的环形编队控制方法,应用领航–跟随编队方法来控制多机器人系统的环形编队和目标包围,通过设计状态估测器,实现对多机器人的状态估计.由领航者获取系统中目标状态的信息,跟随者监测到领航者的状态信息并完成包围环绕编队控制,使多机器人系统形成对动态目标的目标跟踪.根据李雅普诺夫稳定性理论和米塔格定理,得到多机器人系统环形编队控制的充分条件,实现对多机器人系统对目标物的包围控制,通过对一组多机器人队列的目标包围仿真,验证了该方法的有效性.关键词:分数阶;多机器人;编队控制;环形编队;目标跟踪引用格式:伍锡如,邢梦媛.分数阶多机器人的领航–跟随型环形编队控制.控制理论与应用,2021,38(1):103–109DOI:10.7641/CTA.2020.90969Annular formation control of the leader-follower multi-robotbased on fractional orderWU Xi-ru†,XING Meng-yuan(School of Electronic Engineering and Automation,Guilin University of Electronic Technology,Guilin Guangxi541004,China) Abstract:Aiming at the complex problem of annular formation control for fractional order multi robot system,an an-nular formation control method based on fractional order multi robot is proposed.The leader follower formation method is used to control the annular formation and target envelopment of the multi robot systems.The state estimation of multi robot is realized by designing state estimator.The leader obtains the information of the target state in the system,the followers detects the status of the leader and complete annular formation control,the multi-robot system forms the target tracking of the dynamic target.According to Lyapunov stability theory and Mittag Leffler’s theorem,the sufficient conditions of the annular formation control for the multi robot systems are obtained in order to achieve annular formation control of the leader follower multi robot.The effectiveness of the proposed method is verified by simulation by simulation of a group of multi robot experiments.Key words:fractional order;multi-robots;formation control;annular formation;target trackingCitation:WU Xiru,XING Mengyuan.Annular formation control of the leader-follower multi-robot based on fractional order.Control Theory&Applications,2021,38(1):103–1091引言近年来,随着机器人技术的崛起和发展,各式各样的机器人技术成为了各个领域不可或缺的一部分,推动着社会的发展和进步.与此同时,机器人面临的任务也更加复杂,单个机器人已经无法独立完成应尽的责任,这就使得多机器人之间相互协作、共同完成同一个给定任务成为当前社会的研究热点.多机器人系统控制的研究主要集中在一致性问题[1]、多机器人编队控制问题[2–3]、蜂拥问题[4–5]等.其中,编队控制问题作为多机器人系统的主要研究方向之一,是国内外研究学者关注的热点问题.编队控制在生活生产、餐饮服务尤其是军事作战等领域都发挥着极大的作用.例如水下航行器在水中的自主航行和编队控制、军事作战机对空中飞行器的打击以及无人机在各行业的应用等都是多机器人编队控制上的用途[6–7].目前,多机器人编队控制方法主要有3种,其中在多机器收稿日期:2019−11−25;录用日期:2020−08−10.†通信作者.E-mail:****************;Tel.:+86132****1790.本文责任编委:黄攀峰.国家自然科学基金项目(61603107,61863007),桂林电子科技大学研究生教育创新计划项目(C99YJM00BX13)资助.Supported by the National Natural Science Foundation of China(61603107,61863007)and the Innovation Project of GUET Graduate Education (C99YJM00BX13).104控制理论与应用第38卷人系统编队控制问题上应用最广泛的是领航–跟随法[8–10];除此之外,还有基于行为法和虚拟结构法[11].基于行为的多机器人编队方法在描述系统整体时不够准确高效,且不能保证系统控制的稳定性;而虚拟结构法则存在系统灵活性不足的缺陷.领航–跟随型编队控制法具有数学分析简单、易保持队形、通信压力小等优点,被广泛应用于多机器人系统编队[12].例如,2017年,Hu等人采用分布式事件触发策略,提出一种新的自触发算法,实现了线性多机器人系统的一致性[13];Zuo等人利用李雅普诺夫函数,构造具有可变结构的全局非线性一致控制律,研究多机器人系统的鲁棒有限时间一致问题[14].考虑到分数微积分的存储特性,开发分数阶一致性控制的潜在应用具有重要意义.时中等人于2016年设计了空间遥操作分数阶PID 控制系统,提高了机器人系统的跟踪性能、抗干扰性、鲁棒性和抗时延抖动性能[15].2019年,Z Yang等人探讨了分数阶多机器人系统的领航跟随一致性问题[16].而在多机器人的环形编队控制中,对具有分数阶动力学特性的多机器人系统的研究极其有限,大部分集中在整数阶的阶段.而采用分数阶对多机器人系统目标包围编队控制进行研究,综合考虑了非局部分布式的影响,更好地描述具有遗传性质的动力学模型.使得系统的模型能更准确的反映系统的性态,对多机器人编队控制的研究非常有利.目标包围控制问题是编队控制的一个分支,是多智能体编队问题的重点研究领域.随着信息技术的高速发展,很多专家学者对多机器人系统的目标包围控制问题进行了研究探讨.例如,Kim和Sugie于2017年基于一种循环追踪策略设计分布式反馈控制律,保证了多机器人系统围绕一个目标机器人运动[17].在此基础上,Lan和Yan进行了拓展,研究了智能体包围多个目标智能体的问题,并把这个问题分为两个步骤[18]. Kowdiki K H和Barai K等人则研究了单个移动机器人对任意时变曲线的跟踪包围问题[19].Asif M考虑了机器人与目标之间的避障问题,提出了两种包围追踪控制算法;并实现了移动机器人对目标机器人的包围追踪[20].鉴于以上原因,本文采用了领航–跟随型编队控制方法来控制多机器人系统的环形编队和目标包围,通过设计状态估测器,实现对多机器人的状态估计.系统中目标状态信息只能由领航者获取,确保整个多机器人系统编队按照预期的理想编队队形进行无碰撞运动,并最终到达目标位置,对目标、领航者和跟随者的位置分析如图1(a)所示,图1(b)为编队控制后的状态.通过应用李雅普诺夫稳定性理论,得到实现多机器人系统环形编队控制的充分条件.最后通过对一组多机器人队列进行目标包围仿真,验证了该方法的有效性.(a)编队控制前(b)编队控制后图1目标、领航者和追随者的位置分析Fig.1Location analysis of targets,pilots and followers2代数图论与分数阶基础假定一个含有N个智能体的系统,通讯网络拓扑图用G={v,ε}表示,定义ε=v×v为跟随者节点之间边的集合,v={v i,i=1,2,···,N}为跟随者节点的集合.若(v i,v j)∈ε,则v i与v j为相邻节点,定义N j(t)={i|(v i,v j)∈ε,v i∈v}为相邻节点j的标签的集合.那么称第j个节点是第i 个节点的邻居节点,用N j(t)={i|(v i,v j)∈ε,v i∈v}表示第i个节点的邻居节点集合.矩阵L=D−A称为与图G对应的拉普拉斯矩阵.其中:∆是对角矩阵,对角线元素i=∑jN i a ij.若a ij=a ji,i,j∈I,则称G是无向图,否则称为有向图.如果节点v i与v j之间一组有向边(v i,v k1)(v k1,v k2)(v k2,v k3)···(v kl,v j),则称从节点v i到v j存在有向路径.定义1Riemann-Liouville(RL)分数阶微分定义:RLD atf(t)=1Γ(n−a)d nd t ntt0f(τ)(t−τ)a−n+1dτ,(1)其中:t>t0,n−1<α<n,n∈Z+,Γ(·)为伽马函数.定义2Caputo(C)分数阶微分定义:CDαtf(t)=1Γ(n−α)tt0f n(τ)(t−τ)α−n+1dτ,(2)其中:t>t0,n−1<α<n,n∈Z+,Γ(·)为伽马第1期伍锡如等:分数阶多机器人的领航–跟随型环形编队控制105函数.定义3定义具有两个参数α,β的Mittag-Leffler方程为E α,β(z )=∞∑k =1z kΓ(αk +β),(3)其中:α>0,β>0.当β=1时,其单参数形式可表示为E α,1(z )=E α(z )=∞∑k =1z kΓ(αk +1).(4)引理1[21]假定存在连续可导函数x (t )∈R n ,则12C t 0D αt x T (t )x (t )=x T (t )C t 0D αt x (t ),(5)引理2[21]假定x =0是系统C t 0D αt x (t )=f (x )的平衡点,且D ⊂R n 是一个包含原点的域,R 是一个连续可微函数,x 满足以下条件:{a 1∥x ∥a V (t ) a 2∥x ∥ab ,C t 0D αt V (t ) −a 3∥x ∥ab,(6)其中:t 0,x ∈R ,α∈(0,1),a 1,a 2,a 3,a,b 为任意正常数,那么x =0就是Mittag-Leffler 稳定.3系统环形编队控制考虑包含1个领航者和N 个跟随者的分数阶非线性多机器人系统.领航者的动力学方程为C t 0D αt x 0(t )=u 0(t ),(7)式中:0<α<1,x 0(t )∈R 2是领航者的位置状态,u 0(t )∈R 2是领航者的控制输入.跟随者的动力学模型如下:C t 0D αt x i (t )=u i (t ),i ∈I,(8)式中:0<α<1,x i (t )∈R 2是跟随者的位置状态,u i (t )∈R 2是跟随者i 在t 时刻的控制输入,I ={1,2,···,N }.3.1领航者控制器的设计对于领航者,选择如下控制器:u 0(t )=−k 1(x 0(t )−˜x 0(t ))−k 2sgn(x 0(t )−˜x 0(t )),(9)C t 0D αt x 0(t )=u 0(t )=−k 1(x 0(t )−˜x 0(t ))−k 2sgn(x 0(t )−˜x 0(t )).(10)设计一个李雅普诺夫函数:V (t )=12(x 0(t )−˜x 0(t ))T (x 0(t )−˜x 0(t )).(11)根据引理1,得到该李雅普诺夫函数的α阶导数如下:C 0D αt V(t )=12C 0D αt (x 0(t )−˜x 0(t ))T (x 0(t )−˜x 0(t )) (x 0(t )−˜x 0(t ))TC 0D αt (x 0(t )−˜x0(t ))=(x 0(t )−˜x 0(t ))T [C 0D αt x 0(t )−C 0D αt ˜x0(t )]=(x 0(t )−˜x 0(t ))T [−k 1(x 0(t )−˜x 0(t ))−k 2sgn(x 0(t )−˜x 0(t ))−C 0D αt ˜x0(t )]=−k 1(x 0(t )−˜x 0(t ))T (x 0(t )−˜x 0(t ))−k 2∥x 0(t )−˜x 0(t )∥−(x 0(t )−˜x 0(t ))TC 0D αt ˜x0(t )=−2k 1V (t )−k 2∥x 0(t )−˜x 0(t )∥+∥C 0D αt ˜x0(t )∥∥x 0(t )−˜x 0(t )∥=−2k 1V (t )−(k 2−∥C 0D ∝t ˜x0(t )∥)∥x 0(t )−˜x 0(t )∥ −2k 1V (t ).(12)令a 1=a 2=12,a 3=2k 1,ab =2,a >0,b >0,得到a 1∥x 0(t )−˜x 0(t )∥a V (t ) a 2∥x 0(t )−˜x 0(t )∥ab ,(13)C t 0D αt V(t ) −a 3∥x 0(t )−˜x 0(t )∥ab .(14)根据引理2,可知lim t →∞∥x 0(t )−˜x 0(t )∥=0,即x 0(t )逐渐趋近于˜x 0(t ).为了使跟随者能够跟踪观测到领航者的状态,设计了一个状态估测器.令ˆx i ∈R 2是追随者对领航者的状态估计,给出了ˆx i 的动力学方程C 0D αt ˆx i=β(∑j ∈N ia ij g ij (t )+d i g i 0(t )),(15)其中g ij =˜x j (t )−˜x i (t )∥˜x j (t )−˜x i (t )∥,˜x j (t )−˜x i (t )=0,0,˜x j (t )−˜x i (t )=0.(16)对跟随者取以下李雅普诺夫函数:V (t )=12N ∑i =1(ˆx i (t )−x 0(t ))T (ˆx i (t )−x 0(t )).(17)计算该函数的α阶导数如下:C 0D αt V(t )=12C 0D αtN ∑i =1(ˆx i (t )−x 0(t ))T (ˆx i (t )−x 0(t )) N ∑i =1(ˆx i (t )−x 0(t ))TC 0D αt (ˆx i (t )−x 0(t ))=N ∑i =1(ˆx i (t )−x 0(t ))T [C 0D αt ˆxi (t )−C 0D αt x 0(t )]=N ∑i =1(ˆx i (t )−x 0(t ))T [β(∑j ∈N ia ijˆx j (t )−ˆx i (t )∥ˆx j (t )−ˆx i (t )∥+d iˆx 0(t )−ˆx i (t )∥ˆx 0(t )−ˆx i (t )∥)−C 0D αt x 0(t )]=N ∑i =1(ˆx i (t )−x 0(t ))T β(∑j ∈N i a ij ˆx j (t )−ˆx i (t )∥ˆx j (t )−ˆx i(t )∥+106控制理论与应用第38卷d iˆx 0(t )−ˆx i (t )∥ˆx 0(t )−ˆx i (t )∥)−N ∑i =1(ˆx i (t )−x 0(t ))TC 0D αt x 0(t )=βN ∑i =1(ˆx i (t )−x 0(t ))T ∑j ∈N i a ij ˆx j (t )−ˆx i (t )∥ˆx j (t )−ˆx i (t )∥+βN ∑i =1(ˆx i (t )−x 0(t ))Td i ˆx 0(t )−ˆx i (t )∥ˆx 0(t )−ˆx i(t )∥−N ∑i =1(ˆx i (t )−x 0(t ))TC 0D αt x 0(t ).(18)在上式中,令C 0D αt V (t )=N 1+N 2以方便后续计算,其中:N 1=βN ∑i =1(ˆx i (t )−x 0(t ))T ∑j ∈N i a ij ˆx j (t )−ˆx i (t )∥ˆx j (t )−ˆx i (t )∥+βN ∑i =1(ˆx i (t )−x 0(t ))Td i ˆx 0(t )−ˆx i (t )∥ˆx 0(t )−ˆx i (t )∥=β2[N ∑i =1N ∑j =1a ij (ˆx i (t )−x 0(t ))T ˆx j (t )−ˆx i (t )∥ˆx j (t )−ˆx i (t )∥+N ∑j =1N ∑i =1a ij (ˆx j (t )−x 0(t ))Tˆx i (t )−ˆx j (t )∥ˆx i (t )−ˆx j (t )∥]−βN ∑i =1d i∥ˆx 0(t )−ˆx i (t )∥2∥ˆx 0(t )−ˆx i (t )∥=β2N ∑i =1N ∑j =1a ij [(ˆx i (t )−x 0(t ))Tˆx j (t )−ˆx i (t )∥ˆx j (t )−ˆx i (t )∥−(ˆx j (t )−x 0(t ))T ˆx i (t )−ˆx j (t )∥ˆx i (t )−ˆx j (t )∥]−βN ∑i =1d i∥ˆx 0(t )−ˆx i (t )∥2∥ˆx 0(t )−ˆx i (t )∥=β2N ∑i =1N ∑j =1a ij [ˆx T i(t )ˆx j (t )−ˆx i (t )∥ˆx j (t )−ˆx i (t )∥−x T 0(t )ˆx j (t )−ˆx i (t )∥ˆx j (t )−ˆx i (t )∥−ˆx T j(t )ˆx i (t )−ˆx j (t )∥ˆx i (t )−ˆx j (t )∥+x T0(t )ˆx i (t )−ˆx j (t )∥ˆx i (t )−ˆx j (t )∥]−βN ∑i =1d i ∥ˆx 0(t )−ˆx i (t )∥=β2N ∑i =1N ∑j =1a ij [ˆx T i (t )ˆx j (t )−ˆx i (t )∥ˆx j (t )−ˆx i (t )∥−ˆx T j (t )ˆx i (t )−ˆx j (t )∥ˆx i (t )−ˆx j (t )∥]−βN ∑i =1d i ∥ˆx 0(t )−ˆx i (t )∥2∥ˆx 0(t )−ˆx i (t )∥=β2N ∑i =1N ∑j =1a ij (ˆx T i(t )−ˆx Tj (t ))ˆx j (t )−ˆx i (t )∥ˆx j (t )−ˆx i (t )∥−βN ∑i =1d i ∥ˆx 0(t )−ˆx i (t )∥2∥ˆx 0(t )−ˆx i (t )∥=−β(12N ∑i =1N ∑j =1a ij (ˆx T j (t )−ˆx T i (t ))׈x j (t )−ˆx i (t )∥ˆx j (t )−ˆx i (t )∥+N ∑i =1d i ∥ˆx 0(t )−ˆx i (t )∥2∥ˆx 0(t )−ˆx i (t )∥),(19)N 2=−N ∑i =1(ˆx i (t )−x 0(t ))TC 0D αt x 0(t )=N ∑i =1∥ˆx i (t )−x 0(t )∥∥C 0D αt x 0(t )∥×cos {ˆx i (t )−x 0(t ),−C 0D αt x 0(t )}.(20)由于∥C 0D αt x 0(t )∥k 1∥x 0(t )−˜x 0(t )∥+k 2∥sgn(x 0(t )−˜x 0(t ))∥ k 1∥x 0(t )−˜x 0(t )∥+k 2.(21)根据定义3,当lim t →∞∥x 0(t )−˜x 0(t )∥=0时,存在T >0(T 为实数),使得在t >T 时∥x 0(t )−˜x 0(t )∥ ε成立,那么对于t >T ,有0<∥C 0D αt x 0(t )∥ k 1ε+k 2=M 2,可得−N ∑i =1(ˆx i (t )−x 0(t ))TC 0D αt x 0(t )N ∑i =1∥ˆx i (t )−x 0(t )∥M 2M 2N max {∥ˆx i (t )−x 0(t )∥},(22)C 0D αt V(t ) −(β−M 2N )max i ∈I{∥ˆx i (t )−x 0(t )∥}−2β1λmin V (t ).(23)根据引理2,得lim t →∞∥ˆx i (t )−x 0(t )∥=0.(24)由上式可知,ˆx i (t )在对目标的追踪过程中逐渐趋近于x 0(t ).3.2跟随者控制器的设计在本文中,整个多机器人系统中领导者能够直接获得目标的位置信息,将这些信息传递给追随者,因此需要为每个追随者设计观测器来估计目标的状态.令ϕi (t )∈R 2由跟随者对目标i 的状态估计,给出ϕi (t )的动力学方程C 0D αt ϕi(t )=α(∑j ∈N ia ij f ij (t )+d i f i 0(t )),(25)其中f ij =ϕj (t )−ϕi (t )∥ϕj (t )−ϕi (t )∥,ϕj (t )−ϕi (t )=0,0,ϕj (t )−ϕi (t )=0.(26)取如下李雅普诺夫函数:V (t )=12N ∑i =1(ϕi (t )−r (t ))T (ϕi (t )−r (t )).(27)计算α阶导数如下:C 0D αt V(t )=第1期伍锡如等:分数阶多机器人的领航–跟随型环形编队控制10712N ∑i =1(ϕi (t )−r (t ))T (ϕi (t )−r (t )) N ∑i =1(ϕi (t )−r (t ))TC 0D αt (ϕi (t )−r (t ))=N ∑i =1(ϕi (t )−r (t ))T [C 0D αt ϕi (t )−C 0D αt r (t )]=N ∑i =1(φi (t )−r (t ))T [α(∑j ∈N ia ij f ij (t )+d i f i 0(t ))]−C 0D αt r (t )=N ∑i =1(ϕi (t )−r (t ))T α(∑j ∈N ia ij ϕj (t )−ϕi (t )∥ϕj (t )−ϕi (t )∥+d i ϕ(t )−ϕi (t )∥ϕ(t )−ϕi (t )∥)=βN ∑i =1(ϕi (t )−r (t ))T ∑j ∈N i a ijϕj (t )−ϕi (t )∥ϕj (t )−ϕi(t )∥+βN ∑i =1(ϕi (t )−r (t ))T d i ϕ(t )−ϕi (t )∥ϕ(t )−ϕi(t )∥−N ∑i =1(ϕi (t )−r (t ))TC 0D αt r (t ),(28)可得lim t →∞∥x i (t )−˜x i (t )∥=0.(29)由上式可知,x i (t )在对目标的追踪过程中逐渐趋近于˜x i (t ).4仿真结果与分析本节通过仿真结果来验证本文所提出的方法.图2为通信图,其中:V ={1,2,3,4}表示跟随者集合,0代表领导者.以5个机器人组成的队列为例进行验证,根据领航者对目标的跟随轨迹,分别进行了仿真.图2通信图Fig.2Communication diagrams假设系统中目标机器人的动态为C 0D αt r (t )=[cos t sin t ]T ,令初始值r 1(0)=r 2(0)=1,α=0.98,k 1=1,k 2=4,可知定理3中的条件是满足的.根据式(24)和式(29),随着时间趋于无穷,领航者及其跟随者的状态估计误差趋于0,这意味着领航者的状态可以由跟随者渐近精确地计算出来.令k 2>M 1,M 1=M +M ′>0,则lim t →∞∥x 0(t )−˜x 0(t )∥=0,x 0渐近收敛于领航者的真实状态.此时取时滞参数µ=0.05,实验结果见图3,由1个领航者及4个跟随者组成的多机器人系统在进行目标围堵时,最终形成了以目标机器人为中心的包围控制(见图3(b)).(a)领航者和跟随者的初始位置分析(b)编队形成后多机器人的位置关系图3目标、领航者和追随者的位置分析Fig.3Location analysis of target pilots and followers综合图4–5曲线,跟随者对领航者进行渐进跟踪,领航者同目标机器人的相对位置不变,表明该领航跟随型多机器人系统最终能与目标机器人保持期望的距离,并且不再变化.图4领航者及其跟随者的状态估计误差Fig.4The state estimation error of the leader and followers108控制理论与应用第38卷图5编队形成时领航者与目标的相对位置关系Fig.5The relative position relationship between leader andtarget仿真结果表明,多个机器人在对目标物进行包围编队时,领航者会逐渐形成以目标物运动轨迹为参照的运动路线,而跟随者则渐近的完成对领航者的跟踪(如图6所示),跟随者在对领航者进行跟踪时,会出现一定频率的抖振,但这些并不会影响该多机器人系统的目标包围编队控制.5总结本文提出了多机器人的领航–跟随型编队控制方法,选定了一台机器人作为领航者负责整个编队的路径规划任务,其余机器人作为跟随者.跟随机器人负责实时跟踪领航者,并尽可能与领航机器人之间保持队形所需的距离和角度,确保整个多机器人系统编队按照预期的理想编队队形进行无碰撞运动,并最终到达目标位置.通过建立李雅普诺夫函数和米塔格稳定性理论,得到了实现多机器人系统环形编队的充分条件,并通过对一组多机器人队列的目标包围仿真,验证了该方法的有效性.图6领航者与跟随者对目标的状态估计Fig.6State estimation of target by pilot and follower参考文献:[1]JIANG Yutao,LIU Zhongxin,CHEN Zengqiang.Distributed finite-time consensus algorithm for multiple nonholonomic mobile robots with disturbances.Control Theory &Applications ,2019,36(5):737–745.(姜玉涛,刘忠信,陈增强.带扰动的多非完整移动机器人分布式有限时间一致性控制.控制理论与应用,2019,36(5):737–745.)[2]ZHOU Chuan,HONG Xiaomin,HE Junda.Formation control ofmulti-agent systems with time-varying topology based on event-triggered mechanism.Control and Decision ,2017,32(6):1103–1108.(周川,洪小敏,何俊达.基于事件触发的时变拓扑多智能体系统编队控制.控制与决策,2017,32(6):1103–1108.)[3]ZHANG Ruilei,LI Sheng,CHEN Qingwei,et al.Formation controlfor multi-robot system in complex terrain.Control Theory &Appli-cations ,2014,31(4):531–537.(张瑞雷,李胜,陈庆伟,等.复杂地形环境下多机器人编队控制方法.控制理论与应用,2014,31(4):531–537.)[4]WU Jin,ZHANG Guoliang,ZENG Jing.Discrete-time modeling formultirobot formation and stability of formation control algorithm.Control Theory &Applications ,2014,31(3):293–301.(吴晋,张国良,曾静.多机器人编队离散模型及队形控制稳定性分析.控制理论与应用,2014,31(3):293–301.)[5]WANG Shuailei,ZHANG Jinchun,CAO Biao.Target tracking al-gorithm with double-type agents based on flocking control.Control Engineering of China ,2019,26(5):935–940.(王帅磊,张金春,曹彪.双类型多智能体蜂拥控制目标跟踪算法.控制工程,2019,26(5):935–940.)[6]SHAO Zhuang,ZHU Xiaoping,ZHOU Zhou,et al.Distributed for-mation keeping control of UA Vs in 3–D dynamic environment.Con-trol and Decision ,2016,31(6):1065–1072.(邵壮,祝小平,周洲,等.三维动态环境下多无人机编队分布式保持控制.控制与决策,2016,31(6):1065–1072.)[7]PANG Shikun,WANG Jian,YI Hong.Formation control of multipleautonomous underwater vehicles based on sensor measuring system.Journal of Shanghai Jiao Tong University ,2019,53(5):549–555.(庞师坤,王健,易宏.基于传感探测系统的多自治水下机器人编队协调控制.上海交通大学学报,2019,53(5):549–555.)[8]WANG H,GUO D,LIANG X.Adaptive vision-based leader-followerformation control of mobile robots.IEEE Transactions on Industrial Electronics ,2017,64(4):2893–2902.[9]LI R,ZHANG L,HAN L.Multiple vehicle formation control basedon robust adaptive control algorithm.IEEE Intelligent Transportation Systems Magazine ,2017,9(2):41–51.[10]XING C,ZHAOXIA P,GUO G W.Distributed fixed-time formationtracking of multi-robot systems with nonholonomic constraints.Neu-rocomputing ,2018,313(3):167–174.[11]LOPEZ-GONZALEA A,FERREIRA E D,HERNANDEZ-MAR-TINEZ E G.Multi-robot formation control using distance and ori-entation.Advanced Robotics ,2016,30(14):901–913.[12]DIMAROGONAS D,FRAZZOLI E,JOHNSSON K H.Distributedevent-triggered control for multi-agent systems.IEEE Transactions on Automatic Control ,2019,57(5):1291–1297.[13]HU W,LIU L,FENG G.Consensus of linear multi-agent systems bydistributed event-triggered strategy.IEEE Transactions on Cybernet-ics ,2017,46(1):148–157.第1期伍锡如等:分数阶多机器人的领航–跟随型环形编队控制109[14]ZUO Z,LIN T.Distributed robustfinite-time nonlinear consensusprotocols for multi-agent systems.International Journal of Systems Science,2016,47(6):1366–1375.[15]SHI Zhong,HUANG Xuexiang,TAN Qian.Fractional-order PIDcontrol for teleoperation of a free-flying space robot.Control The-ory&Applications,2016,33(6):800–808.(时中,黄学祥,谭谦.自由飞行空间机器人的遥操作分数阶PID控制.控制理论与应用,2016,33(6):800–808.)[16]YANG Z C,ZHENG S Q,LIU F.Adaptive output feedback con-trol for fractional-order multi-agent systems.ISA Transactions,2020, 96(1):195–209.[17]LIU Z X,CHEN Z Q,YUAN Z Z.Event-triggered average-consensusof multi-agent systems with weighted and directed topology.Journal of Systems Science and Complexity,2016,25(5):845–855.[18]AI X L,YU J Q.Flatness-basedfinite-time leader-follower formationcontrol of multiple quad rotors with external disturbances.Aerospace Science and Technology,2019,92(9):20–33.[19]KOWDIKI K H,BARAI K,BHATTACHARYA S.Leader-followerformation control using artificial potential functions:A kinematic ap-proach.IEEE International Conference on Advances in Engineering.Tamil Nadu,India:IEEE,2012:500–505.[20]ASIF M.Integral terminal sliding mode formation control of non-holonomic robots using leader follower approach.Robotica,2017, 1(7):1–15.[21]CHEN W,DAI H,SONG Y,et al.Convex Lyapunov functions forstability analysis of fractional order systems.IET Control Theory& Applications,2017,11(7):1070–1074.作者简介:伍锡如博士,教授,硕士生导师,目前研究方向为机器人控制、神经网络、深度学习等,E-mail:***************.cn;邢梦媛硕士研究生,目前研究方向为多机器人编队控制,E-mail: ****************.。
人工智能大模型优化商品定价策略 英文
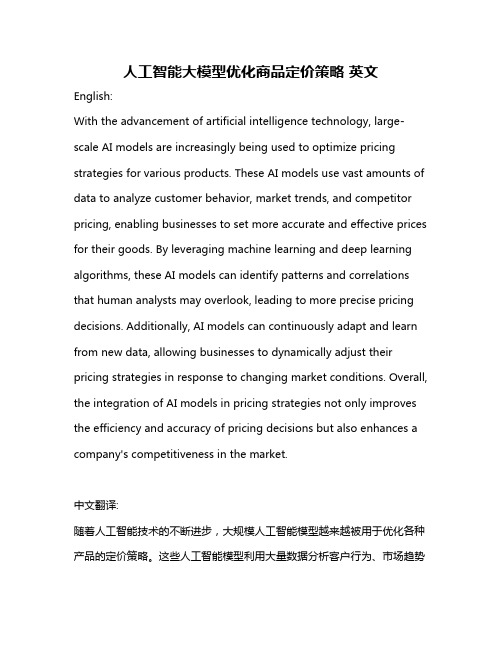
人工智能大模型优化商品定价策略英文English:With the advancement of artificial intelligence technology, large-scale AI models are increasingly being used to optimize pricing strategies for various products. These AI models use vast amounts of data to analyze customer behavior, market trends, and competitor pricing, enabling businesses to set more accurate and effective prices for their goods. By leveraging machine learning and deep learning algorithms, these AI models can identify patterns and correlations that human analysts may overlook, leading to more precise pricing decisions. Additionally, AI models can continuously adapt and learn from new data, allowing businesses to dynamically adjust their pricing strategies in response to changing market conditions. Overall, the integration of AI models in pricing strategies not only improves the efficiency and accuracy of pricing decisions but also enhances a company's competitiveness in the market.中文翻译:随着人工智能技术的不断进步,大规模人工智能模型越来越被用于优化各种产品的定价策略。
人工智能产业链出自的参考文献
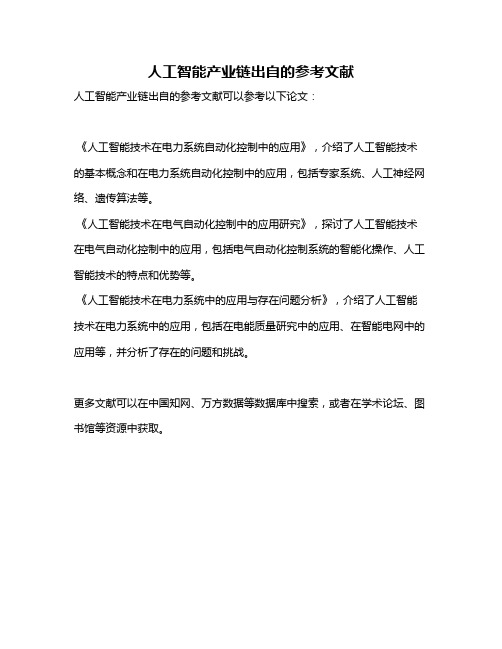
人工智能产业链出自的参考文献
人工智能产业链出自的参考文献可以参考以下论文:
《人工智能技术在电力系统自动化控制中的应用》,介绍了人工智能技术的基本概念和在电力系统自动化控制中的应用,包括专家系统、人工神经网络、遗传算法等。
《人工智能技术在电气自动化控制中的应用研究》,探讨了人工智能技术在电气自动化控制中的应用,包括电气自动化控制系统的智能化操作、人工智能技术的特点和优势等。
《人工智能技术在电力系统中的应用与存在问题分析》,介绍了人工智能技术在电力系统中的应用,包括在电能质量研究中的应用、在智能电网中的应用等,并分析了存在的问题和挑战。
更多文献可以在中国知网、万方数据等数据库中搜索,或者在学术论坛、图书馆等资源中获取。
人工智能辅助金融股票预测模型研究
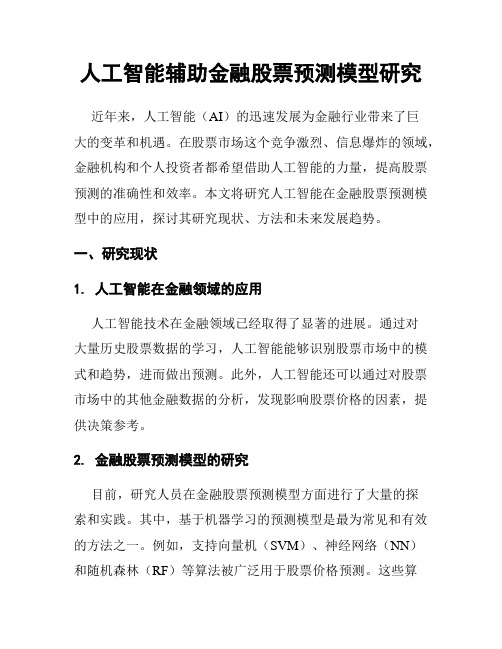
人工智能辅助金融股票预测模型研究近年来,人工智能(AI)的迅速发展为金融行业带来了巨大的变革和机遇。
在股票市场这个竞争激烈、信息爆炸的领域,金融机构和个人投资者都希望借助人工智能的力量,提高股票预测的准确性和效率。
本文将研究人工智能在金融股票预测模型中的应用,探讨其研究现状、方法和未来发展趋势。
一、研究现状1. 人工智能在金融领域的应用人工智能技术在金融领域已经取得了显著的进展。
通过对大量历史股票数据的学习,人工智能能够识别股票市场中的模式和趋势,进而做出预测。
此外,人工智能还可以通过对股票市场中的其他金融数据的分析,发现影响股票价格的因素,提供决策参考。
2. 金融股票预测模型的研究目前,研究人员在金融股票预测模型方面进行了大量的探索和实践。
其中,基于机器学习的预测模型是最为常见和有效的方法之一。
例如,支持向量机(SVM)、神经网络(NN)和随机森林(RF)等算法被广泛用于股票价格预测。
这些算法能够通过学习历史数据中的模式和规律,识别出未来股票价格的可能变化。
此外,一些研究还探索了将深度学习模型应用于股票预测中的可行性,如卷积神经网络(CNN)和长短期记忆网络(LSTM)等。
二、方法探讨1. 数据获取与预处理准确的数据是构建股票预测模型的基础。
在研究过程中,需要从可靠和全面的数据源中获取历史股票数据,包括股票价格、成交量和财务指标等。
获取数据后,还需要进行数据清洗和预处理,以确保数据的完整性和准确性。
2. 特征选择与特征工程在构建股票预测模型时,选择合适的特征对预测结果的准确性至关重要。
可以利用统计学方法、领域知识和专业分析工具来选择与股票价格相关的特征变量。
此外,特征工程也是提高模型性能的关键步骤,通过对特征进行组合、转换和降维,可以提高模型的预测能力。
3. 模型构建与优化选择合适的机器学习算法和深度学习模型对股票进行预测。
传统的机器学习算法如SVM、NN和RF能够适应不同类型的股票数据,并具有较高的预测精度。
人工智能领域模型评估和性能分析方面50个课题名称

人工智能领域模型评估和性能分析方面50个课题名称1. 基于机器学习算法的模型评估方法研究2. 人工智能模型性能分析与优化技术研究3. 对抗样本攻击与防御机制的性能评估研究4. 基于深度学习的图像识别模型评估与性能分析5. 自然语言处理模型的性能评估与优化研究6. 机器学习模型的鲁棒性评估与分析7. 分布式人工智能模型的性能评估与优化策略研究8. 稀有事件检测模型的性能评估与分析9. 基于强化学习的人工智能模型评估与性能优化10. 跨领域数据集的人工智能模型性能分析研究11. 基于迁移学习的人工智能模型性能评估与分析12. 自动驾驶系统的人工智能模型效能评估研究13. 基于深度强化学习算法的智能推荐系统的性能分析14. 人工智能模型中的数据偏见评估与修正研究15. 基于多模态数据的人工智能模型性能评估与分析16. 对抗性迁移学习模型的性能评估与优化策略研究17. 基于图神经网络的人工智能模型性能分析与改进18. 对抗生成网络模型的性能评估与鲁棒性分析19. 基于联邦学习的隐私保护人工智能模型性能评估研究20. 高效人工智能模型并行计算与性能评估技术研究21. 基于时序数据的人工智能模型性能评估与分析22. 深度强化学习模型的探索性能评估与优化研究23. 复杂环境中机器学习模型的性能评估与鲁棒性分析24. 在线学习模型的性能评估与优化策略研究25. 基于知识图谱的人工智能模型性能评估与分析26. 深度神经网络模型的性能评估与可解释性研究27. 大规模数据集上机器学习模型的性能分析与优化28. 神经网络模型中的脆弱性评估与对抗性分析29. 基于自主学习的人工智能模型性能评估与优化30. 分层学习模型的性能评估与鲁棒性分析31. 渐进学习模型的性能评估与优化策略研究32. 基于递归神经网络的自然语言处理模型性能分析33. 基于异构数据的人工智能模型性能评估与分析34. 视觉感知模型的性能评估与优化研究35. 基于生成对抗网络的图像增强模型的性能分析36. 人工智能模型中的中断恢复能力评估与优化37. 深度学习模型的认知可信度评估与分析38. 基于迁移强化学习的机器人控制模型性能评估研究39. 高维数据处理中机器学习模型性能分析与优化40. 基于自适应学习的人工智能模型性能评估与分析41. 人工智能模型中的决策可靠性评估与优化42. 非正式环境下机器学习模型性能评估与鲁棒性分析43. 基于增量学习的人工智能模型性能评估与优化策略研究44. 多任务学习模型的性能评估与分析45. 基于元学习的人工智能模型性能分析与优化研究46. 图神经网络模型的性能评估与鲁棒性分析47. 基于信息熵的人工智能模型可靠性评估与优化48. 深度生成模型的性能评估与可解释性研究49. 跨领域知识迁移模型的性能评估与分析50. 基于弱监督学习的人工智能模型性能评估与优化。
科技成果产业化目标市场顾客需求识别与评审模型研究
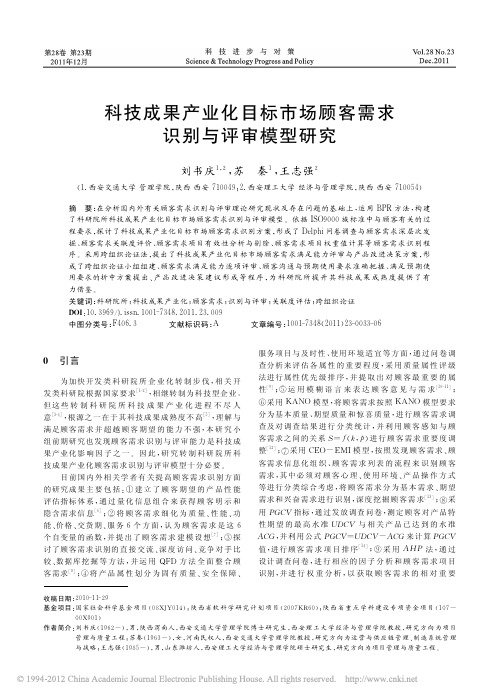
服务项目与及时性 、使 用 环 境 适 宜 等 方 面 ,通 过 问 卷 调 查分析来评 估 各 属 性 的 重 要 程 度 ,采 用 质 量 属 性 评 级 法进行属性 优 先 级 排 序 ,并 提 取 出 对 顾 客 最 重 要 的 属 性[9];⑤ 运 用 模 糊 语 言 来 表 达 顾 客 意 见 与 需 求 ; [10-11] ⑥采用 KANO 模型,将顾客需求按照 KANO 模型要 求 分为基本质量、期望 质 量 和 惊 喜 质 量 ,进 行 顾 客 需 求 调 查及对调查 结 果 进 行 分 类 统 计 ,并 利 用 顾 客 感 知 与 顾 客需求之间的关系 S=f(k,p)进 行 顾 客 需 求 重 要 度 调 整[12];⑦ 采 用 CEO-EMI模 型 ,按 照 发 现 顾 客 需 求 、顾 客需求信息 化 组 织 、顾 客 需 求 列 表 的 流 程 来 识 别 顾 客 需求,其中必 须 对 顾 客 心 理 、使 用 环 境、产 品 操 作 方 式 等进行分类综合考虑 ,将 顾 客 需 求 分 为 基 本 需 求 、期 望 需 求 和 兴 奋 需 求 进 行 识 别 ,深 度 挖 掘 顾 客 需 求[13];⑧ 采 用 PGCV 指标,通过发放调查 问 卷,测 定 顾 客 对 产 品 特 性 期 望 的 最 高 水 准 UDCV 与 相 关 产 品 已 达 到 的 水 准 ACG,并利用公式 PGCV=UDCV-ACG 来计算PGCV 值 ,进 行 顾 客 需 求 项 目 排 序[14];⑨ 采 用 AHP 法 ,通 过 设计调查问 卷,进 行 相 应 的 因 子 分 析 和 顾 客 需 求 项 目 识别,并 进 行 权 重 分 析 ,以 获 取 顾 客 需 求 的 相 对 重 要
ai模型命名术语

AI模型命名术语可以包括以下几个方面:
1. 模型类型:例如深度学习模型、神经网络模型、卷积神经网络模型等。
2. 算法名称:例如支持向量机(SVM)、决策树、随机森林、神经网络等。
3. 特征提取方法:例如主成分分析(PCA)、独立成分分析(ICA)、自编码器等。
4. 模型参数:例如训练集大小、训练轮次、学习率、正则化参数等。
5. 数据集名称:例如医疗图像数据集、面部识别数据集、情感分析数据集等。
6. 任务类型:例如分类、回归、聚类、序列生成等。
7. 评估指标:例如准确率、召回率、F1得分、AUC-ROC曲线等。
8. 优化技术:例如梯度下降算法、早停技术、正则化技术(L1正则化、L2正则化)、Dropout 技术等。
9. 版本迭代:例如第一版模型、第二版模型、优化版模型等。
在命名AI模型时,还可以考虑以下几个方面:
1. 简洁明了:模型名称应该简洁明了,易于理解,避免使用过于复杂的术语和缩写。
2. 一致性:在同一个项目或团队中,应该使用一致的命名规则,以便于沟通和交流。
3. 体现特色:模型名称应该能够体现模型的特色和优势,有助于传达模型的独特之处。
4. 尊重隐私:在命名涉及敏感信息的模型时,应该尊重相关人员的隐私权,避免泄露重要信息。
总之,AI模型命名术语应该简洁明了、易于理解、体现特色和优势,同时尊重相关人员的隐私权。
在命名时,还应该考虑一致性和版本迭代等因素。
- 1、下载文档前请自行甄别文档内容的完整性,平台不提供额外的编辑、内容补充、找答案等附加服务。
- 2、"仅部分预览"的文档,不可在线预览部分如存在完整性等问题,可反馈申请退款(可完整预览的文档不适用该条件!)。
- 3、如文档侵犯您的权益,请联系客服反馈,我们会尽快为您处理(人工客服工作时间:9:00-18:30)。
Market-Based Multirobot Coordination:A Survey and AnalysisM.Bernardine Dias,Robert Zlot,Nidhi Kalra,and Anthony StentzCMU-RI-TR-05-13April2005Robotics InstituteCarnegie Mellon UniversityPittsburgh,Pennsylvania15213c Carnegie Mellon UniversityAbstractMarket-based multirobot coordination approaches have received significant atten-tion and gained considerable popularity within the robotics research community in re-cent years.They have been successfully implemented in a variety of domains ranging from mapping and exploration to robot soccer.The research literature on market-based approaches to coordination has now reached a critical mass that warrants a survey and analysis.This paper addresses this need by providing an introduction to market-based multirobot coordination,a comprehensive review of the state of the art in thefield,and a discussion of remaining challenges.1A version of this paper will appear in the Proceedings of the IEEE–Special Issue on Multi-Robot Systems(expected publication in2006).IContents1Introduction1 2Overview22.1Definition of a Market-based Approach (2)2.2The Range of Coordination Approaches (2)3Planning43.1Related Work (4)3.1.1Planning and Task Allocation (4)3.1.2Planning and Task Decomposition (5)3.1.3Planning and Task Execution (5)3.2Future Challenges (5)4Dynamic Events and Environments54.1Related Work (6)4.1.1Robustness and Fluidity (6)4.1.2Response Speed (6)4.1.3Online Tasks (7)4.1.4Uncertainty (7)4.2Future Challenges (7)5Quality of Solution75.1Related Work (7)5.1.1Practical Approaches (7)5.1.2Theoretical Guarantees (8)5.2Future Challenges (8)6Scalability96.1Related Work (9)6.1.1Computation and Communication Considerations (9)6.1.2Opportunistic Centralization (9)6.2Future Challenges (10)7Heterogeneous Teams107.1Related Work (10)7.2Future Challenges (11)8Tight Coordination118.1Related Work (11)8.2Future Challenges (12)9Learning and Adaptation129.1Related Work (12)9.2Future Challenges (12)III10Generality1310.1Related Work (13)10.1.1Flexibility (13)10.1.2Extensibility (13)10.1.3Implementation (13)10.1.4Comparisons (14)10.2Future Challenges (14)11Conclusions and Future Directions14IV1IntroductionAs robots become an integral part of human life they are charged with increasingly difficult tasks.Many of these tasks can be better achieved by a team of robots than by a single robot.By working together,robots can complete tasks faster,increase system robustness,improve solution quality,and achieve tasks impossible for a single robot. Nevertheless,coordinating such a team requires overcoming many formidable research challenges.Given a team of robots,a limited amount of resources,and a team task,researchers must develop a method of distributing the resources among the team so the task is ac-complished well,even as teammates’interactions,the environment,and the mission change.Humans have dealt with similar problems for thousands of years with increas-ingly sophisticated market economies in which the individual pursuit of profit leads to the redistribution of resources and an efficient production of output.The principles of a market economy can be applied to multirobot coordination.In this virtual econ-omy,the robots are traders,tasks and resources are traded commodities,and virtual money acts as currency.Robots compete to win tasks and resources by participating in auctions that produce efficient distributions based on specified preferences.When the system is appropriately designed,each robot acts to maximise its individual profit and simultaneously improves the efficiency of the team.This paper is motivated by the growing popularity of maket-based multirobot coor-dination approaches and the lack of a comprehensive review of these approaches.As contributors to pioneering this research area,the authors have several years of experi-ence in designing and implementing market-based coordination approaches for multi-robot systems.In this paper the authors draw upon their collective research experience to review the current literature and recommend future research directions.A more detailed analysis of the relevant literature is available as a technical report[24].This paper makes three significant contributions to the robotics literature.First,it introduces market based approaches by discussing the motivating philosophy,defining the require-ments of such approaches,analyzing their strengths and weaknesses,and placing them appropriately in the context of the larger set of approaches to multirobot coordination. Second,this paper surveys and analyses the relevant literature.Finally,it inspires and directs future research on this topic through a discussion of remaining challenges.The scope of this paper is limited to market-based approaches for coordinating teams that include robots.Furthermore,this review principally considers approaches that actively reason about the existence of other agents when coordinating the team,in contrast to approaches in which agents coexist.Nevertheless,related work on market-based multiagent research in software agent domains and other relevant publications are included as necessary to augment discussion.The following section provides an introduction to market-based mechanisms for readers less familiar with thefield and a quick reference for researchers designing and implementing market-based coordination mechanisms.This overview is followed by a extensive review of market-based multirobot coordination approaches categorized and analysed across several relevant dimensions:planning,dynamic events and envi-ronments,solution quality,scalability,heterogeneity,tight coordination,learning and adaptation,and generality.The paper concludes with a summary of the survey and1future challenges in this research area.2OverviewThe earliest examples of market-based multi-agent coordination appeared in the lit-erature over twenty-five years ago[14,40]and have been modified and adopted for multirobot coordination in more recent years.These approaches have been success-fully implemented in a variety of domains.2.1Definition of a Market-based ApproachMost“market-based”multirobot and multiagent coordination approaches share a set of underlying elements.Market theory provides precise definitions for several of these el-ements.Borrowing from both bodies of literature,we define a market-based multirobot coordination approach based on the following requirements:•The team is given an objective that can be decomposed into subcomponents that are achievable by individuals or subteams.To solve the problem,the team has at its disposal a limited set of resources that is distributed among the team members.•A global objective function quantifies the system designer’s preferences for all possible solutions.•An individual utility function specified for each robot quantifies that robot’s pref-erences for its individual resource usage and contributions towards the team ob-jective.Evaluating this function cannot require global or perfect information about the state of the team or team objective.Subteam preferences can also be quantified through a combination of individual utilities.•A mapping is defined between the team objective function and individual and subteam utilities.This mapping addresses how the individual production and consumption of resources and individuals’advancement of the team objective affect the overall solution.•Resources and individual or subteam objectives can be redistributed using a mechanism such as an auction.This mechanism accepts as input teammates’utilities and computes an outcome that maximizes the utility of the agent control-ling the mechanism.In a well-designed mechanism,maximizing the controlling agent’s utility results in improving the team objective function value.Thus,a multirobot coordination approach that satisfies all of the above require-ments can generally be considered as a market-based approach.2.2The Range of Coordination ApproachesIn virtually all robotic application domains,generating optimal solutions in a com-putationally tractable manner is highly advantageous.Unfortunately,these objectives2conflict in multirobot systems where optimal coordination is typically N P-hard.The challenges are compounded by team requirements that include operation in dynamic environments,inconsistent information,unreliable and limited communication,inter-action with humans,and system failures.A spectrum of coordination approaches has emerged to negotiate these demands.At one end of the spectrum,fully centralized approaches employ a single agent to coordinate the entire team.In theory,this agent can produce optimal solutions by gathering all relevant information and planning for the entire team.In reality,fully cen-tralized approaches are rarely tractible for large teams,can suffer from a single point of failure,have high communication demands,and are usually sluggish to respond to changes perceived by distributed sources.Thus,centralized approaches are best suited for applications where teams are small and the environment is static or global state information is easily available.At the other end of the spectrum,in fully-distributed systems,robots rely solely on local knowledge.Such approaches are typically very fast,flexible to change,and robust to failures but can produce highly suboptimal solu-tions since good local solutions may not sum to a good global solution.Applications where large teams carry out relatively simple tasks with no strict requirements for ef-ficiency are best served by fully distributed coordination schemes.A vast majority of coordination approaches have elements that are centralized and distributed and thus reside in the middle of the spectrum.Market-based approaches fall into this hybrid cat-egory,and,if designed well,they can opportunisitically adapt to dynamic conditions to produce more centralized or more distributed solutions.Market-based approaches effectively meet the practical demands of robot teams while producing efficient solutions by capturing the respective strengths of both dis-tributed and centralized approaches.First,they can distribute much of the planning and execution over the team and therby retain the benefits of distributed approaches, including robustness,flexibility,and speed[44,45,18,13].They also have elements of centralized systems to produce better solutions.Auctions concisely gather information about the team and distribute resources in a team-aware context.Researchers have also developed methods of opportunistically centralizing markets[12,23]and providing guarantees of solution quality[28].However,market-based approaches are not without their weaknesses.In domains where fully centralized approaches approaches are feasi-ble,market-based approaches can be more complex to implement and produce poorer solutions.In domains where fully distributed approaches suffice,market-approaches can be unnecessarily complex in design and can require excessive communication and computation.In other domains,the communication demands of auctions may be in-feasible.Perhaps the biggest drawbacks of market-based approaches currently are the lack of performance guarantees and the lack of formalization in designing appropriate cost and revenue functions to capture domain requirements.The following sections discuss market-based multirobot coordination in greater de-tail along the dimensions mentioned in the introduction.Each section introduces the topic and its challenges,defines the goals and appropriate evaluation metrics,reviews the relevant literature,and identifies the remaining research challenges.33PlanningIn multirobot teams,planning can be used to coordinate robots to accomplish the team mission.Unfortunately,optimal planning for multirobot systems is typically an N P-hard problem.The challenge then is to have tractable planning that produces good so-lutions.Market-based approaches manage this by distributing planning over the entire team to produce solutions quickly.When required or when resources permit,markets can behave more centrally and plan over larger portions of the team to improve so-lution quality.We evaluate approaches by how well the local planning captures the complexity of the problem while still being tractable.3.1Related Work3.1.1Planning and Task AllocationTask allocation is the problem of feasibly assigning a set of tasks to a team of robots in a way that optimizes the global objective function.Market-based approaches often distribute planning required for task allocation through the auction process:each robot locally plans the achievement of the offered tasks,computes its costs,and encapsulates the costs in its bids.We believe TraderBots[8]is mostflexible in this respect.In addition to using local planning,TraderBots allows opportunistically centralized plan-ning:when resources permit,“leaders”plan lower-cost redistributions of a subset of tasks to a subgroup of robots and improve the team solution.Other approaches are listed in Section5.1.1.However,opportunistic centralized planning in market-based approaches is a relatively new topic that is yet to be formalized.Related problems such as the allocation of constrained subtasks and the allocation of roles have somewhat different planning requirements:Allocating Constrained Subtasks In many domains,tasks are temporally constrained with respect to each other.They may be partially ordered or may need to start orfinish within a common time frame.During assignment,robots can incorporate the cost of meeting constraints into their bids[30].More realistically,they must coordinate dur-ing execution to reschedule and accommodate team and task changes since the initial allocation[29,19].Allocating Roles In team games one usually assigns positions such as“primary of-fense”or“supporting defense”instead of tasks such as“shoot the ball”or“capture a rebound.”These positions can be classified as roles.More generally a role defines a collection of related actions or behaviors.Indeed,in many domains it is more natural to think of teammates playing roles than completing distinct tasks.In market-based ap-proaches,role allocation uses the same auction-bid-award protocol as task allocation. However,robots generate bids by evaluating afitness function that reflects how well the current state matches the requirements of the role.Once allocated,a robot locally plans the execution of actions and behaviors specified by its role.Market-based role allocation has primarily been demonstrated in the robot soccer domain(e.g.[26]).43.1.2Planning and Task DecompositionAlthough many approaches to task allocation assume that a list of primitive or simple tasks is input to the system,a mission is often more naturally described at a more abstract level.In these cases,multirobot systems must also plan the decomposition of a mission into subtasks.There are two common approaches to this planning problem.In the decompose-then-allocate method,a single agent recursively decomposes the task into simple sub-tasks which are allocated to the team[6].In the allocate-then-decompose method, complex tasks arefirst allocated to robots;then,each robot locally decomposes its awarded tasks[5].It is also possible to include instances of both techniques[19].By decoupling the decomposition and allocation,these approaches do not con-sider the complete solution space and mayfind highly suboptimal solutions.Zlot and Stentz[44]address this issue by generalizing tasks to task trees within a peer-to-peer trading market.Robots may bid at any level of task abstraction in a tree with the option of redecomposing an awarded complex task more efficiently.Experiments in complex task domains have demonstrated that using task trees improves solution quality over two-stage approaches.3.1.3Planning and Task ExecutionSignificantly less work has been done to plan coordination between teammates during task execution.Nevertheless,it becomes necessary when robots interfere with each other during execution.Azarm and Schmidt[2]address collision avoidance of inde-pendent robots using market-based techniques.It is also necessary in domains where teammates continuously constrain each other’s actions.Such coordination is more tightly-coupled and therefore difficult to distribute.In the Hoplites framework[23], constraints are captured in the utility function and planning is local when constraints are easily satisfied.When constraint satisfaction becomes difficult,teammates attempt res-olution through negotiated participation in more centrally-developed joint plans.This is related to the idea of“leaders”in TraderBots[11].3.2Future ChallengesReplanning is a major remaining challenge and relates closely to the continuing chal-lenges for teams operating in dynamic environments(Section4.2).Existing market mechanisms are not yet fully capable of replanning task distributions,redecompos-ing tasks,rescheduling commitments,and replanning coordination during execution. Another important and relevant area of research is understanding the formation of sub-teams and enabling their positive interaction using market-based methods.4Dynamic Events and EnvironmentsOperating in dynamic environments poses a variety of challenges.The principle chal-lenges relevant to coordinating a team are ensuring graceful degradation of solution5quality with failures,enabling team functionality despite imperfect and uncertain in-formation,maintaining high response speed to dynamic events,and effectively accom-modating evolving task and team composition.Relevant evaluation metrics include the diversity of failures the team can accommodate,the required quantity and certainty in perceived information,the team’s response speed to dynamic events,thefluidity of the team,and the overall solution quality produced by the team in the face of dynamic events.4.1Related Work4.1.1Robustness and FluidityMany multirobot applications require some level of robustness.Robotic systems are incredibly complex and their physical interactions with the environment make them highly prone to failures.Three principle categories of faults are communication fail-ures,partial malfunctions,and robot death,all of which are gracefully handled by market-based approaches[13].Teams can often perform more effectively if teammates can communicate[45]. However,communication failures occur in a variety of domains and range from oc-casional loss of messages to loss of all communication.If the team is informed of a common task,consequent disruptions in communication are gracefully handled by using opportunistic auctioning solely for improving solution quality[45,13].As with communication disruptions,partial malfunctions limit a robot’s capability but retain the robot’s planning facilities.Active reasoning about failed resources allows robots to reallocate tasks that they can no longer complete due to malfunctions[13],and moni-toring progress in short-duration tasks allows detection and response to faults[17].In the case of robot death,the affected robot cannot aid in the recovery process.How-ever,robots can monitor heartbeats of teammates and re-auction the tasks previously assigned to the dead robot[13].If malfunctioning robots can be repaired and return to the team,the coordination approach should accommodate both the exit and entrance of the repaired robots.Towing of disabled robots for repair[3]and re-entry of repaired robots[13]have also been demonstrated using market-based approaches.Additional fluidity can be demonstrated by changing the team composition during execution by adding a robot to the team[12].4.1.2Response SpeedA key to successful task execution is often the ability to respond quickly to dynamic conditions.If information must always be channeled to another location for replanning, conditions can change too rapidly for the planner to keep up.Market-based approaches should be able to quickly respond to dynamic events since each robot can respond to local events independently without depending on a centralized coordinator to plan a response.However,the authors are unaware of any formalized study of response speed for any multirobot coordination approach.64.1.3Online TasksIn many dynamic application domains,the demands on the robotic system can change during operation.Operators of a multirobot system may submit new tasks or alter or cancel existing tasks.Additionally,robots may generate new tasks during execution as they observe new information about their surroundings.Market-based approaches can often seamlessly incorporate online tasks by auctioning new tasks as they are in-troduced by an operator[8]or generated by the robots themselves[45,21],or as they become available due to the completion of preceding tasks[5,6,18,38].Tasks can also be canceled during execution[12].Some market-based approaches auction all tasks prior to execution and thus do not explicitly handle online tasks[4,28,31,33].4.1.4UncertaintyMost real-world multirobot applications require operation with only partial or changing information about the environment,the team,or the task.Fortunately,market-based approaches have few information requirements and can accommodate new information through frequent auctioning of tasks and resources.For instance,robots can begin tasks without prior map information[12]and dynamically reallocate tasks when new map information is gathered[42].Robots also do not need to know the size or composition of the team to coordinate[18,12]4.2Future ChallengesWhile much can be done to improve market-based approaches to effectively operate in dynamic environments,a few key challenges are paramount.These challenges are incorporating contract breaches with appropriate penalties,developing more sophisti-cated methods for cooperative handling of partial malfunctions and repairs,and evalu-ating response speed and robustness to a variety of failures.5Quality of SolutionOne of the greatest strengths of a market is its ability to utilize the local information and preferences of its participants to arrive at an efficient solution given limited resources. The fundamental optimization problem encountered in market-based multirobot sys-tems is the task allocation problem.Various global cost objectives appear in market-based systems[43],including the sum of individual robots’costs[4,8,20,28,31]and minimizing the maximum individual cost[33,29].5.1Related Work5.1.1Practical ApproachesTechniques used by market-based systems for task allocation can be broken down into two broad categories:one-task-per-robot(OTPR)approaches and sequencing ap-proaches.In OTPR approaches,each robot behaves myopically and only considers7handling one task at any given time.In sequencing approaches,robots can order(or schedule[19,29,37])a list of tasks and can therefore explicitly reason about the de-pendencies between multiple tasks and upcoming commitments.The OTPR model arises in cases where each task requires an exclusive commitment of a robot(e.g.positional roles in robot soccer[26]).At the other extreme is contin-uous task allocation,by which we mean that the tasks being assigned are short-lived partial actions that bring the team goal closer to being realized.These actions can be updated as the environment changes or new observations are made,and then assigned to the robots by an auctioneer[18,38].OTPR approaches are also sometimes used for simplicity in cases where sequencing approaches could be used[6,7,17,21];however, this simplification is expected to result in less efficient solutions.In these systems, if there are more tasks than robots the remaining tasks can be assigned once robots become available.Sequencing approaches have been used with centralized allocation,both for combi-natorial auctions[4,22,31]and single task auctions[28,33].Combinatorial auctions have the potential to exploit the synergies between tasks,and thus produce better so-lutions as compared to single task auctions.Tovey et al.[43]show that for single-task auctions,choosing the appropriate bidding rule for a given global objective is important in terms of the resulting solution quality.Dias et al.[10]demonstrate that increasing the number of tasks awarded per auction can have a negative effect on the resulting solution quality,but requires less time tofind a solution.Distributed or peer-to-peer allocation approaches allow auctions to take place between robots to improve an exist-ing allocation[8,10,20,29,34].Peer-to-peer trading can be viewed as a local search algorithm,and therefore is subject to local minima.By allowing exchanges of task sets of varying sizes[11],some local minima can be avoided[1,35].Additionally,in unknown or partially known environments where costs are constantly changing as new observations are made,peer-to-peer auctions act as a reallocation mechanism that can repair undesirable allocations.5.1.2Theoretical GuaranteesLagoudakis et al.[27]provide a set of approximation bounds on solution quality for sequential single-task auctions for various team objective functions and bidding rules. For example,bidding marginal costs results in a2-approximation when minimizing a sum-of-costs team objective,while for a makespan objective the approximation ratio scales with the number of robots on the team.Sandholm proves that by using a suffi-ciently expressive set of contract types for peer-to-peer auctions the global minimum can be reached in afinite number of steps[35].Gerkey presents some approximation results for OTPR models,including some online cases[17].5.2Future ChallengesWhile some theoretical guarantees for simple cases of auction algorithms are now known,implemented systems are generally more complex and can include online, multi-task,peer-to-peer,simultaneous,and overlapping auctions as well as task and8scheduling constraints.Additionally,solution quality depends on cost and utility esti-mates,which are sometimes difficult to accurately specify.6ScalabilityA system is scalable if it can operate effectively even as the number of inputs or the size of inputs increases arbitrarily.The scalability of a multirobot coordination approach is typically evaluated by its ability to function as the team size or the task complexity increase.It is particularly desirable for approaches that are designed to be applicable to multiple domains,complex domains,and domains without upper bounds on team size.Scalability in market-based approaches may be limited by the computation and communication needs that arise from increasing auction frequency,bid complexity,and planning demands.However,market-based approaches can scale well in applications where the team mission can be decomposed into tasks that can be independently carried out by small sub-teams.An initial comparison of three approaches,including a market-based approach,on scalability is presented by Dias[8].6.1Related Work6.1.1Computation and Communication ConsiderationsSingle task auctions require a polynomial number of bid valuations and can be cleared in polynomial time.Additionally auction calls and bids require a polynomial number of messages to be binatorial auctions,however,can in general require an exponential number of task bundles to be considered during bid valuation,and winner determination is N P-hard.Additionally,an exponential number of messages would have to be sent to submit bids for the2|T|possible task bundles.In order to make these problems tractable,the number of task bundles can be reduced either on the auctioneer side by offering only certain combinations of tasks[22],or on the bidder side by using heuristic task clustering algorithms[4,11].cases,due to the sparseness of the bids,the auction clearing step can be done optimally and quickly[36],although the resulting allocation is still likely to be suboptimal given that not all task bundles are considered.Bid valuation itself may be computationally expensive.In many cases an N P-hard problem must be solved in order to estimate task costs[4,8,20,33,34],or expensive task decompositions may be required at the bidding stage[44].When there are many tasks to consider simultaneously–either from auctions offering many tasks[4,8,20, 29,31,44]or from many robots initiating auctions at roughly the same time[8,44]–bidders can be overburdened with task valuation problems.As a result,they may not be able to meet auction deadlines or may tax their processors to the point of not being able to do any other useful work.6.1.2Opportunistic CentralizationOpportunistic-centralization[8,23]is a scalable way of incorporating the benefits of centralized planning into large market-based systems.In such approaches,centralized9。