线性代数introduction to vectors
适合初学者的高等数学国外教材
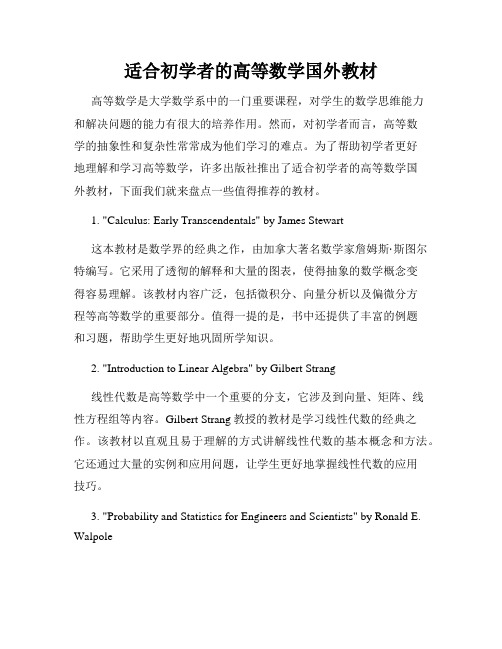
适合初学者的高等数学国外教材高等数学是大学数学系中的一门重要课程,对学生的数学思维能力和解决问题的能力有很大的培养作用。
然而,对初学者而言,高等数学的抽象性和复杂性常常成为他们学习的难点。
为了帮助初学者更好地理解和学习高等数学,许多出版社推出了适合初学者的高等数学国外教材,下面我们就来盘点一些值得推荐的教材。
1. "Calculus: Early Transcendentals" by James Stewart这本教材是数学界的经典之作,由加拿大著名数学家詹姆斯·斯图尔特编写。
它采用了透彻的解释和大量的图表,使得抽象的数学概念变得容易理解。
该教材内容广泛,包括微积分、向量分析以及偏微分方程等高等数学的重要部分。
值得一提的是,书中还提供了丰富的例题和习题,帮助学生更好地巩固所学知识。
2. "Introduction to Linear Algebra" by Gilbert Strang线性代数是高等数学中一个重要的分支,它涉及到向量、矩阵、线性方程组等内容。
Gilbert Strang教授的教材是学习线性代数的经典之作。
该教材以直观且易于理解的方式讲解线性代数的基本概念和方法。
它还通过大量的实例和应用问题,让学生更好地掌握线性代数的应用技巧。
3. "Probability and Statistics for Engineers and Scientists" by Ronald E. Walpole概率论与数理统计是高等数学中的一门重要课程,涉及到随机事件、概率分布、统计推断等内容。
这本教材是一本面向工程师和科学家的概率论与数理统计教材。
它以实际问题为导向,通过例题和应用案例讲解概率和统计的基本原理和方法。
该教材旨在帮助学生理解概率与统计的应用领域,并培养他们的问题分析和解决能力。
4. "Differential Equations and Linear Algebra" by C. Henry Edwardsand David E. Penney微分方程和线性代数是高等数学中两个重要的分支,也是许多其他学科如物理学、工程学和计算机科学的重要基础。
线性代数课程大纲
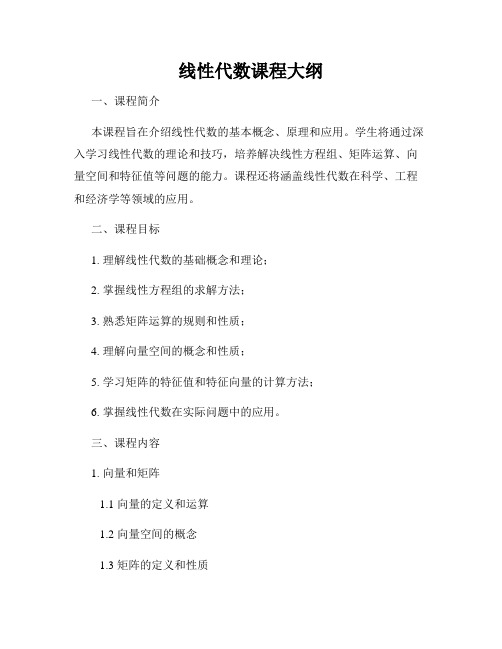
线性代数课程大纲一、课程简介本课程旨在介绍线性代数的基本概念、原理和应用。
学生将通过深入学习线性代数的理论和技巧,培养解决线性方程组、矩阵运算、向量空间和特征值等问题的能力。
课程还将涵盖线性代数在科学、工程和经济学等领域的应用。
二、课程目标1. 理解线性代数的基础概念和理论;2. 掌握线性方程组的求解方法;3. 熟悉矩阵运算的规则和性质;4. 理解向量空间的概念和性质;5. 学习矩阵的特征值和特征向量的计算方法;6. 掌握线性代数在实际问题中的应用。
三、课程内容1. 向量和矩阵1.1 向量的定义和运算1.2 向量空间的概念1.3 矩阵的定义和性质1.4 矩阵运算的规则2. 线性方程组2.1 线性方程组的基本概念2.2 线性方程组的解集和解的判定 2.3 高斯消元法和矩阵消元法2.4 线性方程组的应用3. 矩阵的特征值和特征向量3.1 特征值和特征向量的定义3.2 特征值和特征向量的计算方法 3.3 对角化和相似矩阵3.4 特征值和特征向量的应用4. 向量空间和线性变换4.1 向量空间的性质和子空间4.2 线性相关性和线性无关性4.3 线性变换的定义和性质4.4 线性变换的矩阵表示5. 内积空间5.1 内积的定义和性质5.2 正交性和正交基5.3 格拉姆-施密特正交化方法5.4 最小二乘解和投影6. 应用案例分析6.1 线性代数在图像处理中的应用6.2 线性代数在数据分析中的应用6.3 线性代数在物理学中的应用6.4 线性代数在经济学中的应用四、教学方法1. 理论课讲授:通过教师的讲解和演示,引导学生掌握线性代数的基本概念和理论。
2. 实践练习:课堂上提供典型例题和习题,帮助学生巩固所学知识并培养解决实际问题的能力。
3. 课题研究:指导学生选择一些与线性代数相关的课题进行深入研究,锻炼科研能力和创新精神。
五、考核方式1. 平时表现:包括课堂参与、作业完成情况和实验报告等。
2. 期中考试:对课程前半部分内容进行综合测试。
IntroductiontoLinearAlgebra第四版教学设计

Introduction to Linear Algebra 第四版教学设计课程背景本次教学设计的课程为Introduction to Linear Algebra,是一门展示线性代数基本概念和技巧的课程。
本课程旨在启发学生对数学的思考方式,并为日后数学学习做好铺垫。
本课程的学生对象为大学一年级的本科学生。
这些学生需要具备高中数学的知识和技能,但不需要特别的专业背景。
在此基础上,本课程将讲解线性代数的基础知识与技巧。
教学目标通过本课程的学习,学生应该达到以下教学目标:1.掌握线性代数的基本概念和技巧,包括向量、矩阵、线性方程组和线性变换等。
2.熟悉线性代数的应用领域,包括物理、工程和计算机科学等。
3.学会利用线性代数去解决实际问题,并培养其解决数学问题的思考能力。
4.培养学生的团队合作、交流和创新能力,提高其学习水平和自我发展能力。
授课内容本课程教学内容可按以下方式分布:1.向量和矩阵–向量•空间和向量的基本定义•向量的线性运算和组合–矩阵•矩阵的定义和基本运算•矩阵的乘法及其性质2.线性方程组–一元线性方程组的解法–多元线性方程组的解法3.线性变换–线性变换的定义和基本性质–线性变换的矩阵表示–主要变换的几何意义4.特征值和特征向量–特征值和特征向量的定义和性质–矩阵对角化的概念和方法5.应用举例和案例分析教学方法本课程采用多种教学方法,旨在最大限度地提高学生的学习效果:1.授课:通过课堂讲解,为学生提供线性代数的基础知识和技巧。
2.组内合作:通过团队合作的方式,引导学生在应用题目中学习解决实际问题的技能。
3.课外阅读和作业:通过布置相关读物和作业,拓展学生成长的视野和提高其创新能力。
教学评价为了评价学生对本课程的掌握程度和学习效果,本课程会针对以下方面进行评估:1.课堂表现:评价学生上课互动、发言和思考的积极性,评分期末考试成绩的20%。
2.课程作业:阅读、计算、应用和理论解题等形式多样的课程作业,评分期末考试成绩的20%。
introduction to linear algebra 每章开头方框-概述说明以及解释
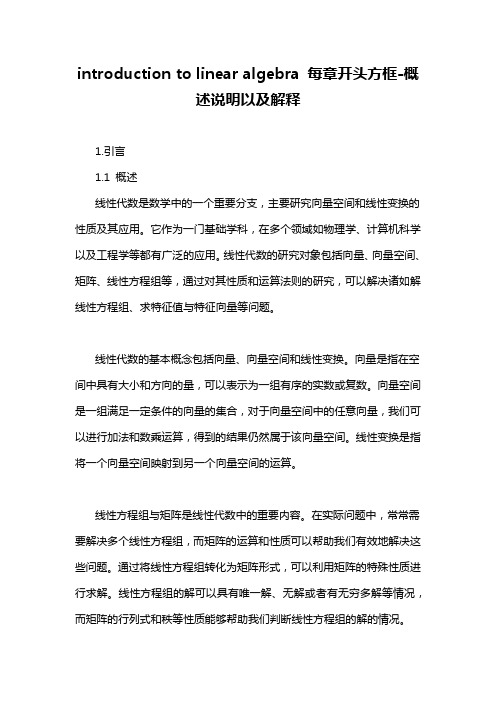
introduction to linear algebra 每章开头方框-概述说明以及解释1.引言1.1 概述线性代数是数学中的一个重要分支,主要研究向量空间和线性变换的性质及其应用。
它作为一门基础学科,在多个领域如物理学、计算机科学以及工程学等都有广泛的应用。
线性代数的研究对象包括向量、向量空间、矩阵、线性方程组等,通过对其性质和运算法则的研究,可以解决诸如解线性方程组、求特征值与特征向量等问题。
线性代数的基本概念包括向量、向量空间和线性变换。
向量是指在空间中具有大小和方向的量,可以表示为一组有序的实数或复数。
向量空间是一组满足一定条件的向量的集合,对于向量空间中的任意向量,我们可以进行加法和数乘运算,得到的结果仍然属于该向量空间。
线性变换是指将一个向量空间映射到另一个向量空间的运算。
线性方程组与矩阵是线性代数中的重要内容。
在实际问题中,常常需要解决多个线性方程组,而矩阵的运算和性质可以帮助我们有效地解决这些问题。
通过将线性方程组转化为矩阵形式,可以利用矩阵的特殊性质进行求解。
线性方程组的解可以具有唯一解、无解或者有无穷多解等情况,而矩阵的行列式和秩等性质能够帮助我们判断线性方程组的解的情况。
向量空间与线性变换是线性代数的核心内容。
向量空间的性质研究可以帮助我们理解向量的运算和性质,以及解释向量空间的几何意义。
线性变换是一种将一个向量空间映射到另一个向量空间的运算,通过线性变换可以将复杂的向量运算问题转化为简单的矩阵运算问题。
在线性变换中,我们需要关注其核、像以及变换的特征等性质,这些性质可以帮助我们理解线性变换的本质和作用。
综上所述,本章节将逐步介绍线性代数的基本概念、线性方程组与矩阵、向量空间与线性变换的相关内容。
通过深入学习和理解这些内容,我们能够掌握线性代数的基本原理和应用,为进一步研究更高级的线性代数问题打下坚实的基础。
1.2文章结构在文章结构部分,我们将介绍本文的组织结构和各章节的内容概述。
线性代数基础学习书单
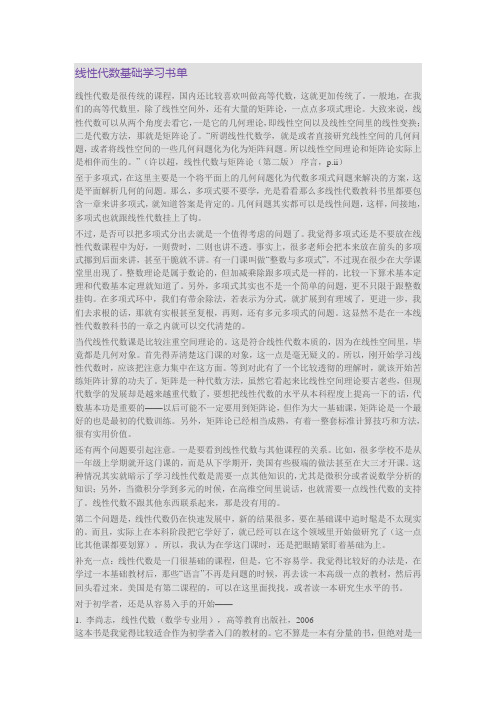
线性代数基础学习书单线性代数是很传统的课程,国内还比较喜欢叫做高等代数,这就更加传统了。
一般地,在我们的高等代数里,除了线性空间外,还有大量的矩阵论,一点点多项式理论。
大致来说,线性代数可以从两个角度去看它,一是它的几何理论,即线性空间以及线性空间里的线性变换;二是代数方法,那就是矩阵论了。
“所谓线性代数学,就是或者直接研究线性空间的几何问题,或者将线性空间的一些几何问题化为化为矩阵问题。
所以线性空间理论和矩阵论实际上是相伴而生的。
”(许以超,线性代数与矩阵论(第二版)·序言,p.ii)至于多项式,在这里主要是一个将平面上的几何问题化为代数多项式问题来解决的方案,这是平面解析几何的问题。
那么,多项式要不要学,光是看看那么多线性代数教科书里都要包含一章来讲多项式,就知道答案是肯定的。
几何问题其实都可以是线性问题,这样,间接地,多项式也就跟线性代数挂上了钩。
不过,是否可以把多项式分出去就是一个值得考虑的问题了。
我觉得多项式还是不要放在线性代数课程中为好,一则费时,二则也讲不透。
事实上,很多老师会把本来放在前头的多项式挪到后面来讲,甚至干脆就不讲。
有一门课叫做“整数与多项式”,不过现在很少在大学课堂里出现了。
整数理论是属于数论的,但加减乘除跟多项式是一样的,比较一下算术基本定理和代数基本定理就知道了。
另外,多项式其实也不是一个简单的问题,更不只限于跟整数挂钩。
在多项式环中,我们有带余除法,若表示为分式,就扩展到有理域了,更进一步,我们去求根的话,那就有实根甚至复根,再则,还有多元多项式的问题。
这显然不是在一本线性代数教科书的一章之内就可以交代清楚的。
当代线性代数课是比较注重空间理论的。
这是符合线性代数本质的,因为在线性空间里,毕竟都是几何对象。
首先得弄清楚这门课的对象,这一点是毫无疑义的。
所以,刚开始学习线性代数时,应该把注意力集中在这方面。
等到对此有了一个比较透彻的理解时,就该开始苦练矩阵计算的功夫了。
线性代数(linearalgebra)
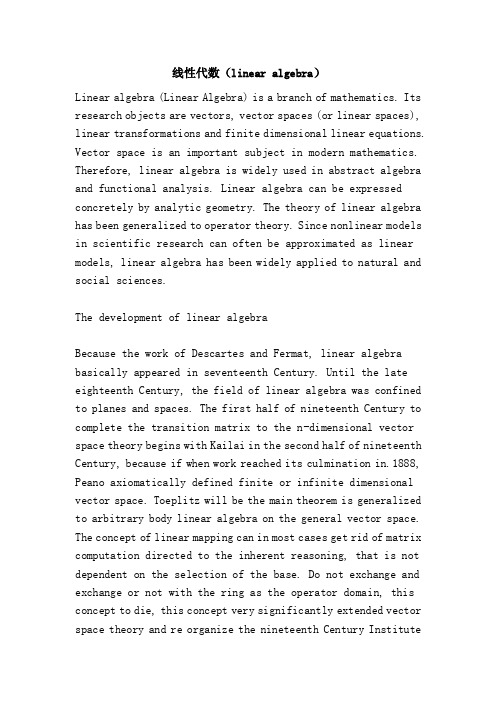
线性代数(linear algebra)Linear algebra (Linear Algebra) is a branch of mathematics. Its research objects are vectors, vector spaces (or linear spaces), linear transformations and finite dimensional linear equations. Vector space is an important subject in modern mathematics. Therefore, linear algebra is widely used in abstract algebra and functional analysis. Linear algebra can be expressed concretely by analytic geometry. The theory of linear algebra has been generalized to operator theory. Since nonlinear models in scientific research can often be approximated as linear models, linear algebra has been widely applied to natural and social sciences.The development of linear algebraBecause the work of Descartes and Fermat, linear algebra basically appeared in seventeenth Century. Until the late eighteenth Century, the field of linear algebra was confined to planes and spaces. The first half of nineteenth Century to complete the transition matrix to the n-dimensional vector space theory begins with Kailai in the second half of nineteenth Century, because if when work reached its culmination in.1888, Peano axiomatically defined finite or infinite dimensional vector space. Toeplitz will be the main theorem is generalized to arbitrary body linear algebra on the general vector space. The concept of linear mapping can in most cases get rid of matrix computation directed to the inherent reasoning, that is not dependent on the selection of the base. Do not exchange and exchange or not with the ring as the operator domain, this concept to die, this concept very significantly extended vector space theory and re organize the nineteenth Century Instituteof the.The word "algebra" appeared relatively late in China, in the Qing Dynasty when the incoming China, it was translated into "Alj Bala", until 1859, the Qing Dynasty famous mathematician, translator Li Shanlan translated it as "algebra", still in use.The status of linear algebraLinear algebra is a subject that discusses matrix theory and finite dimensional vector spaces combined with matrices and their linear transformation theory.The main theory is mature in nineteenth Century, and the first cornerstone (the solution of two or three Yuan linear equations) appeared as early as two thousand years ago (see in our ancient mathematical masterpiece "nine chapters arithmetic").The linear algebra has many important applications in mathematics, mechanics, physics and technology, so it has important place in various branches of algebra;In the computer today, computer graphics, computer aided design, cryptography, virtual reality and so on are all part of the theory and algorithm of linear algebra;.Between geometric and algebraic methods embodied in the concept of the subject of the connection from the axiomatic method on the abstract concept and rigorous logic reasoning, cleverly summed up, to strengthen people's training in mathematics, science and intelligent gain is very useful;And with the development of science, we should not only study the relationship between the individual variables, but also further study the relationship between multiple variables, all kinds of practical problems in most cases can be linearized, and because of the development of the computer, the linearized problem can be calculated, linear algebra is a powerful tool to solve these problems.Basic introduction to linear algebraLinear algebra originated from the study of two-dimensional and three-dimensional Cartesian coordinate systems. Here, a vector is a line segment with a direction that is represented by both length and direction. Thus vectors can be used to represent physical quantities, such as force, or to add and multiply scalar quantities. This is the first example of a real vector space.Modern linear algebra has been extended to study arbitrary or infinite dimensional spaces. A vector space of dimension n is called n-dimensional space. In two-dimensional andthree-dimensional space, most useful conclusions can be extended to these high-dimensional spaces. Although many people do not easily imagine vectors in n-dimensional space, such vectors (i.e., n tuples) are very useful for representing data. Since n is a tuple, and the vector is an ordered list of n elements, most people can effectively generalize and manipulate data in this framework. For example, in economics, 8 dimensional vectors can be used to represent the gross national product (GNP) of 8 countries. When all the nationalorder (such as scheduled, China, the United States, Britain, France, Germany, Spain, India, Australia), you can use the vector (V1, V2, V3, V4, V5, V6, V7, V8) showed that these countries a year each GNP. Here, each country's GNP are in their respective positions.As a purely abstract concept used in proving theorems, vector spaces (linear spaces) are part of abstract algebra and have been well integrated into this field. Some notable examples are: irreversible linear maps or groups of matrices, rings of linear mappings in vector spaces. Linear algebra also plays an important role in mathematical analysis,Especially in vector analysis, higher order derivatives are described, and tensor product and commutative mapping are studied.A vector space is defined on a domain, such as a real or complex domain. Linear operators map the elements of a linear space into another linear space (or in the same linear space), and maintain the consistency of addition and scalar multiplication in the vector space. The set of all such transformations is itself a vector space. If a basis of linear space is determined, all linear transformations can be expressed as a table, called matrix. Further studies of matrix properties and matrix algorithms (including determinants and eigenvectors) are also considered part of linear algebra.We can simply say that the linear problems in Mathematics - those that exhibit linear problems - are most likely to be solved. For example, differential calculus studies the problemof linear approximation of functions. In practice, the difference between a nonlinear problem and a nonlinear one is very important.The linear algebra method refers to the problem of using a linear viewpoint to describe it and to describe it in the language of linear algebra and to solve it (when necessary) by using matrix operations. This is one of the most important applications in mathematics and engineering.Some useful theoremsEvery linear space has a base.The nonzero matrix n for a row of N rows A, if there is a matrix B that makes AB = BA = I (I is the unit matrix), then A is nonsingular matrix.A matrix is nonsingular if and only if its determinant is not zero.A matrix is nonsingular if and only if the linear transformation it represents is a automorphism.A matrix is semi positive if and only if each of its eigenvalues is greater than or equal to zero.A matrix is positive if and only if each of its eigenvalues is greater than zero.Generalizations and related topicsLinear algebra is a successful theory, and its method has been applied to other branches of mathematics.The theory of modulus is to study the substitution of scalar domains in linear algebra by ring substitution.Multilinear algebra transforms the "multivariable" problem of mapping into the problem of each variable, resulting in the concept of tensor.In the spectral theory of operators, by using mathematical analysis, infinite dimensional matrices can be controlled.All of these areas have very large technical difficulties.Basic contents of linear algebra in Chinese UniversitiesFirst, the nature and tasks of the courseThe course of linear algebra is an important basic theory course required by students of science and Engineering in universities and colleges. It is widely used in every field of science and technology. Especially today, with the development and popularization of computer, linear algebra has become the basic theory knowledge and important mathematical tool for engineering students. Linear algebra is to train thehigh-quality specialized personnel needed for the socialist modernization construction of our country. Through the study of this course, we should make students get:1 determinant2, matrix3. The correlation of vectors and the rank of matrices4 、 linear equations5, similar matrix and two typeAnd other basic concepts, basic theories and basic operational skills, and lay the necessary mathematical foundation for further courses and further knowledge of mathematics.While imparting knowledge through various teaching links gradually cultivate students with abstract thinking ability, logical reasoning ability, spatial imagination ability and self-learning ability, but also pay special attention to cultivate students with good operation ability and comprehensive use of the knowledge to the ability to analyze and solve problems.Two, the content of the course teaching, basic requirements and class allocation(1) teaching content1 determinant(1) definition of order n determinant(2) the nature of determinant(3) the calculation of the determinant is carried out in rows (columns)(4) the Clem rule for solving linear equations2, matrix(1) the concept of matrix, unit matrix, diagonal matrix, symmetric matrix(2) linear operations, multiplication operations, transpose operations and laws of matrices(3) inverse matrix concept and its properties, and inverse matrix with adjoint matrix(4) the operation of partitioned matrices3 vector(1) the concept of n-dimensional vectors(2) the linear correlation, linear independence definition and related theorems of vector groups, and the judgement of linear correlation(3) the maximal independent group of vectors and the rank of vectors(4) the concept of rank of matrix(5) elementary transformation of matrix, rank and inverse matrix of matrix by elementary transformation(6) n-dimensional vector spaces and subspaces, bases, dimensions, coordinates of vectors4 、 linear equations(1) the necessary and sufficient conditions for the existence of nonzero solutions of homogeneous linear equations and the necessary and sufficient conditions for the existence of solutions of nonhomogeneous linear equations(2) the fundamental solution, the general solution and the solution structure of the system of linear equations(3) the condition and judgement of the solution of nonhomogeneous linear equations and the solution of the system of equations(4) finding the general solution of linear equations by elementary row transformation5, similar matrix and two type(1) eigenvalues and eigenvectors of matrices and their solutions(2) similarity matrix and its properties(3) the necessary and sufficient conditions and methods of diagonalization of matrices(4) similar diagonal matrices of real symmetric matrices(5) two type and its matrix representation(6) the method of linearly independent vector group orthogonal normalization(7) the concept and property of orthogonal transformation and orthogonal matrix(8) orthogonal transformation is used as the standard shape of the two type(9) the canonical form of quadratic form and two form of two type are formulated by formula(10) the inertia theorem, the rank of the two type, the positive definite of the two type and their discrimination(two) basic requirements1, understand the definition of order n determinant, will use the definition of simple determinant calculation2, master the basic calculation methods and properties of determinant3, master Clem's law4. Understand the definition of a matrix5, master the matrix operation method and inverse matrix method6. Understanding the concept of vector dependency defines the relevance of the vector by definition7, grasp the method of finding the rank of the matrix, and understand the relation between the rank of the matrix and the correlation of the vector group8, understand the concept of vector space, will seek vector coordinates9. Master the matrix rank and inverse matrix with elementary transformation, and solve the system of linear equations10, master the method of solving linear equations, and know the simple application of linear equations11. Master the method of matrix eigenvalue and eigenvector12. Grasp the concept of similar matrices and the concept of diagonalization of matrices13, master the orthogonal transformation of two times for standard type method14, understand the inertia theorem of the two type, and use thematching method to find the sum of squares of the two type15. Grasp the concept and application of the positive definiteness of the two typeMATLABIt is a programming language and can be used as a teaching software for engineering linear algebra. It has been introduced into many university textbooks at home and abroad.。
introduction to linear algebra 5th edition 中译 -回复
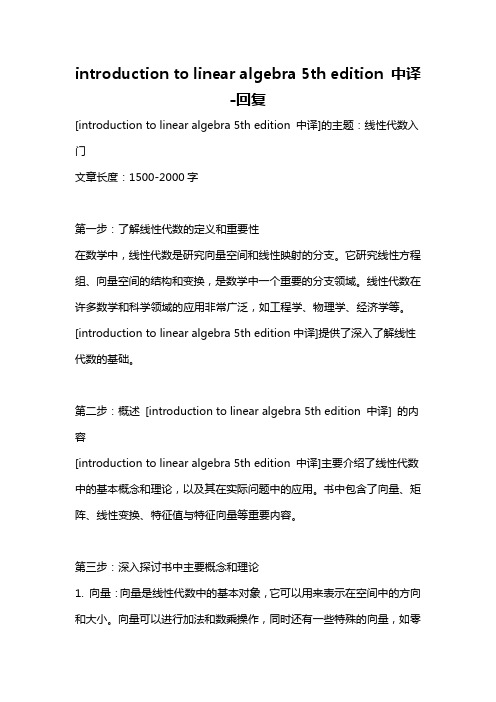
introduction to linear algebra 5th edition 中译-回复[introduction to linear algebra 5th edition 中译]的主题:线性代数入门文章长度:1500-2000字第一步:了解线性代数的定义和重要性在数学中,线性代数是研究向量空间和线性映射的分支。
它研究线性方程组、向量空间的结构和变换,是数学中一个重要的分支领域。
线性代数在许多数学和科学领域的应用非常广泛,如工程学、物理学、经济学等。
[introduction to linear algebra 5th edition中译]提供了深入了解线性代数的基础。
第二步:概述[introduction to linear algebra 5th edition 中译] 的内容[introduction to linear algebra 5th edition 中译]主要介绍了线性代数中的基本概念和理论,以及其在实际问题中的应用。
书中包含了向量、矩阵、线性变换、特征值与特征向量等重要内容。
第三步:深入探讨书中主要概念和理论1. 向量:向量是线性代数中的基本对象,它可以用来表示在空间中的方向和大小。
向量可以进行加法和数乘操作,同时还有一些特殊的向量,如零向量和单位向量。
2. 矩阵:矩阵是线性代数中的另一个重要概念,它是由一个或多个向量组成的矩形数组。
矩阵可以用来表示线性变换、解线性方程组等问题。
矩阵的乘法和转置操作是线性代数中常用的操作。
3. 线性变换:线性变换是将一个向量空间映射为另一个向量空间的变换。
线性变换具有保持加法和数乘运算的特性,可以用矩阵来表示。
线性变换在图像处理、信号处理等领域有广泛应用。
4. 特征值与特征向量:特征值与特征向量是描述线性变换的重要概念。
特征值代表了线性变换对应的变换方向,而特征向量代表了这个方向上的变换量。
特征值与特征向量在物理学和计算机图形学中有广泛应用。
introduction to linear algebra 5th edition 中译 -回复

introduction to linear algebra 5th edition 中译-回复题目: 《[线性代数引论第五版中译]》:理解线性代数的步骤和关键概念引言:线性代数作为数学的一个重要分支,研究了向量空间和线性映射的性质与结构,是解决各种数学和工程问题的基础。
而在学习线性代数的过程中,作为一本经典教材,《线性代数引论第五版中译》提供了详尽和深入的内容,对于初学者来说,理解其中的步骤和关键概念是非常重要的。
本文将一步一步回答关于该教材的一些常见问题,帮助读者更好地掌握线性代数。
第一步:了解线性代数的基本定义和概念(Introduction)在学习《线性代数引论第五版中译》之前,首先需要了解线性代数的基本定义和概念。
线性代数主要研究向量、矩阵和线性变换等概念。
向量是线性代数中的基本元素,它可以表示方向和大小。
矩阵是由数构成的矩形阵列,用于表示线性映射和线性方程组等。
线性变换是指一个向量空间到另一个向量空间的映射,保持向量间的线性关系。
第二步:掌握向量空间的概念和性质(Chapter 1)向量空间是线性代数中最重要的概念之一,它是由一组满足一定条件的向量所构成的集合。
在学习第一章时,需要掌握向量空间的定义、线性组合、线性相关性和线性无关性等重要概念。
了解这些基本概念,可以帮助我们理解向量空间的性质和操作。
第三步:理解线性变换和矩阵的关系(Chapter 2)线性变换和矩阵是线性代数中核心的概念。
一个线性变换可以由一个矩阵来表示,而一个矩阵也可以表示一个线性变换。
在第二章,我们需要理解线性变换和矩阵之间的关系,掌握线性变换的定义、线性变换的运算和性质,以及矩阵的乘法和逆矩阵等重要概念。
第四步:解析线性方程组和矩阵的特征值和特征向量(Chapter 3)线性方程组是线性代数中的一个重要应用。
在第三章,我们需要学习如何解析线性方程组,理解矩阵的秩、行列式和逆矩阵的相关概念。
此外,还需要掌握矩阵的特征值和特征向量的概念,这对于分析和处理线性变换的性质和行为非常重要。
线性代数常用单词词组中英对照

IndexEEconomics 经济学435,439 Eigencourse 457,458Eigenvalue 特征值283,287,374,499 Eigenvalue changes 特征值变换439Eigenvalues of 的特征值284,294,300 Eigenvalues of 的特征值297Eigenvalues of 的特征值362Eigenvector basis 基底的特征向量399Eigenvectors 特征向量283,287,374Eigshow 290,368Elimination 消元法45-66,83,86,135 Ellipse 椭圆290,346,366,382 Energy 能量343,409Engineering 工程409,419Error 误差211,218,219,225,481,483 Error equation 误差方程477Euler angles 欧拉角474Euler’s formula 欧拉公式311,426,430,497Even 偶数113,246,258,452 Exponential 指数的314,319,327FHilbert space 希尔伯特空间447,449Hooke’s Law 虎克定律410,412 Householder reflections 镜像变换237,469,472 Hyperplane 超平面30,42IIll-conditioned matrix 病态矩阵371,473,474 Imaginary 虚数289Independent 独立的26,27,134,168,200,300 Initial value 初值313Inner product 内积11,56,108,448,502,506 Input and output basis 基底输入输出399Integral 积分24,385,386Interior point method 内点法445Intersection of spaces 交空间129,183Inverse matrix 逆矩阵24,81,270Inverse of的逆82Invertible 可逆的86,173,200,248 Iteration 迭代481,482,484,489,492 JJacobi 雅可比481,483,485,489 Jordan form 约当型356,357,358,361,482 JPEG 364,373KKalman filter 卡尔曼滤波器93,214Kernel 核377,380Kirchhoff’s Laws 基尔霍夫定律143,189,420,424-427 Krylov 克雷洛夫491,492Lnorm 和范数225,480Lagrange multiplier 拉格朗日乘子445Lanczos method 兰索斯方法490,492LAPACK 线性代数软件包98,237,486Leapfrog method 跳步法317,329Least squares 最小平方218,219,236,405,408,453 Left nullspace左零空间184,186,192,425Left-inverse 左逆的81,86,154,405Length 长度12,232,447,448,501 Line 线34,40,221,474Line of springs 线弹簧411Linear combination 线性组合1,3Linear equation 线性方程23Linear programming 线性规划440Linear transformation 线性变换44,375-398Linearity 线性关系44,245,246Linearly independent 线性独立26,134,168,169,200 LINPACK 线性系统软件包465Loop 环路307,425,426Lower triangular 下三角9598,100,474Lucas numbers 卢卡斯数306MMaple 38,100Mathematica 38,100MATLAB 17,37,237,243,290,337,513 Matrix(see full page 570) 矩阵22,384,387Matrix exponential 矩阵指数314,319,327Matrix multiplication 矩阵乘法58,59,67,389Matrix notation 矩阵记号37Matrix space 矩阵空间121,122,175,181,311 Matrix 矩阵-1,2,-1 matrix -1,2,-1矩阵106,167,261,265,349,374,410,480Adjacency, 邻接矩阵74,80,311,369All-ones 全1矩阵251,262,307,348 Augmented 增广矩阵60,84,155Band 带状矩阵99,468,469。
线性代数英文单词
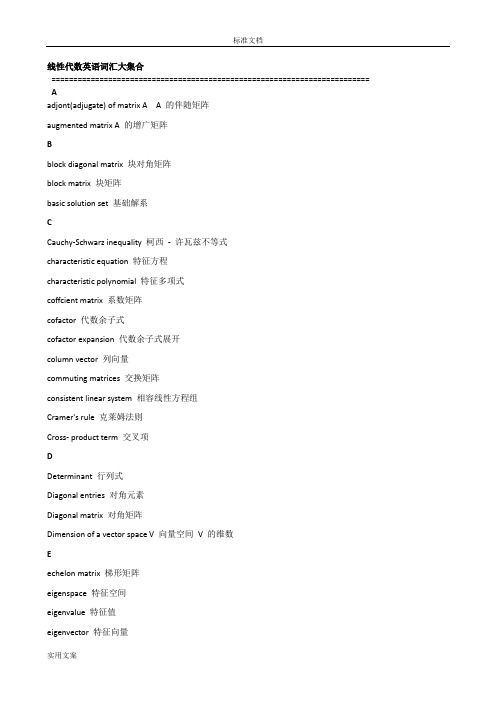
线性代数英语词汇大集合========================================================================= Aadjont(adjugate) of matrix A A 的伴随矩阵augmented matrix A 的增广矩阵Bblock diagonal matrix 块对角矩阵block matrix 块矩阵basic solution set 基础解系CCauchy-Schwarz inequality 柯西- 许瓦兹不等式characteristic equation 特征方程characteristic polynomial 特征多项式coffcient matrix 系数矩阵cofactor 代数余子式cofactor expansion 代数余子式展开column vector 列向量commuting matrices 交换矩阵consistent linear system 相容线性方程组Cramer's rule 克莱姆法则Cross- product term 交叉项DDeterminant 行列式Diagonal entries 对角元素Diagonal matrix 对角矩阵Dimension of a vector space V 向量空间V 的维数Eechelon matrix 梯形矩阵eigenspace 特征空间eigenvalue 特征值eigenvector 特征向量eigenvector basis 特征向量的基elementary matrix 初等矩阵elementary row operations 行初等变换Ffull rank 满秩fundermental set of solution 基础解系Ggrneral solution 通解Gram-Schmidt process 施密特正交化过程Hhomogeneous linear equations 齐次线性方程组Iidentity matrix 单位矩阵inconsistent linear system 不相容线性方程组indefinite matrix 不定矩阵indefinit quatratic form 不定二次型infinite-dimensional space 无限维空间inner product 内积inverse of matrix A 逆矩阵JKLlinear combination 线性组合linearly dependent 线性相关linearly independent 线性无关linear transformation 线性变换lower triangular matrix 下三角形矩阵Mmain diagonal of matrix A 矩阵的主对角matrix 矩阵Nnegative definite quaratic form 负定二次型negative semidefinite quadratic form 半负定二次型nonhomogeneous equations 非齐次线性方程组nonsigular matrix 非奇异矩阵nontrivial solution 非平凡解norm of vector V 向量V 的范数normalizing vector V 规范化向量Oorthogonal basis 正交基orthogonal complemen t 正交补orthogonal decomposition 正交分解orthogonally diagonalizable matrix 矩阵的正交对角化orthogonal matrix 正交矩阵orthogonal set 正交向量组orthonormal basis 规范正交基orthonomal set 规范正交向量组Ppartitioned matrix 分块矩阵positive definite matrix 正定矩阵positive definite quatratic form 正定二次型positive semidefinite matrix 半正定矩阵positive semidefinite quadratic form 半正定二次型Qquatratic form 二次型Rrank of matrix A 矩阵A 的秩r(A )reduced echelon matrix 最简梯形阵row vector 行向量Sset spanned by { } 由向量{ } 所生成similar matrices 相似矩阵similarity transformation 相似变换singular matrix 奇异矩阵solution set 解集合standard basis 标准基standard matrix 标准矩阵Isubmatrix 子矩阵subspace 子空间symmetric matrix 对称矩阵Ttrace of matrix A 矩阵A 的迹tr ( A )transpose of A 矩阵A 的转秩triangle inequlity 三角不等式trivial solution 平凡解Uunit vector 单位向量upper triangular matrix 上三角形矩阵Vvandermonde matrix 范得蒙矩阵vector 向量vector space 向量空间WZzero subspace 零子空间zero vector 零空间==============================================================================向量:vector 向量的长度(模):零向量: zero vector负向量: 向量的加法:addition 三角形法则:平行四边形法则:多边形法则减法向量的标量乘积:scalar multiplication 向量的线性运算线性组合:linear combination 线性表示,线性相关(linearly dependent),线性无关(linearly independent),原点(origin)位置向量(position vector)线性流形(linear manifold)线性子空间(linear subspace)基(basis)仿射坐标(affine coordinates),仿射标架(affine frame),仿射坐标系(affine coordinate system)坐标轴(coordinate axis)坐标平面卦限(octant)右手系左手系定比分点线性方程组(system of linear equations齐次线性方程组(system of homogeneous linear equations)行列式(determinant)维向量向量的分量(component)向量的相等和向量零向量负向量标量乘积维向量空间(vector space)自然基行向量(row vector)列向量(column vector)单位向量(unit vector)直角坐标系(rectangular coordinate system),直角坐标(rectangular coordinates),射影(projection)向量在某方向上的分量,正交分解,向量的夹角,内积(inner product)标量积(scalar product),数量积,方向的方向角,方向的方向余弦;二重外积外积(exterior product),向量积(cross product),混合积(mixed product,scalar triple product)==================================================================================(映射(mapping)),(象(image)),(一个原象(preimage)),(定义域(domain)),(值域(range)),(变换(transformation)),(单射(injection)),(象集),(满射(surjection)),(一一映射,双射(bijection)),(原象),(映射的复合,映射的乘积),(恒同映射,恒同变换(identity mapping)),(逆映射(inverse mapping));(置换(permutation)),(阶对称群(symmetric group)),(对换(transposition)),(逆序对),(逆序数),(置换的符号(sign)),(偶置换(even permutation)),(奇置换(odd permutation));行列式(determinant),矩阵(matrix),矩阵的元(entry),(方阵(square matrix)),(零矩阵(zero matrix)),(对角元),(上三角形矩阵(upper triangular matrix)),(下三角形矩阵(lower triangular matrix)),(对角矩阵(diagonal matrix)),(单位矩阵(identity matrix)),转置矩阵(transpose matrix),初等行变换(elementary row transformation),初等列变换(elementary column transformation);(反称矩阵(skew-symmetric matrix));子矩阵(submatrix),子式(minor),余子式(cofactor),代数余子式(algebraic cofactor),(范德蒙德行列式(Vandermonde determinant));(未知量),(系数矩阵),(方程的系数(coefficient)),(常数项(constant)),(线性方程组的解(solution)),(增广矩阵(augmented matrix)),(零解);子式的余子式,子式的代数余子式===================================================================================线性方程组与线性子空间(阶梯形方程组),(方程组的初等变换),行阶梯矩阵(row echelon matrix),主元,简化行阶梯矩阵(reduced row echelon matrix),(高斯消元法(Gauss elimination)),(解向量),(同解),(自反性(reflexivity)),(对称性(symmetry)),(传递性(transitivity)),(等价关系(equivalence));(齐次线性方程组的秩(rank));(主变量),(自由位置量),(一般解),向量组线性相关,向量组线性无关,线性组合,线性表示,线性组合的系数,(向量组的延伸组);线性子空间,由向量组张成的线性子空间;基,坐标,(自然基),向量组的秩;(解空间),线性子空间的维数(dimension),齐次线性方程组的基础解系(fundamental system of solutions);(平面束(pencil of planes))(导出组),线性流形,(方向子空间),(线性流形的维数),(方程组的特解);(方程组的零点),(方程组的图象),(平面的一般方程),(平面的三点式方程),(平面的截距式方程),(平面的参数方程),(参数),(方向向量);(直线的方向向量),(直线的参数方程),(直线的标准方程),(直线的方向系数),(直线的两点式方程),(直线的一般方程);=====================================================================================矩阵的秩与矩阵的运算线性表示,线性等价,极大线性无关组;(行空间,列空间),行秩(row rank),列秩(column rank),秩,满秩矩阵,行满秩矩阵,列满秩矩阵;线性映射(linear mapping),线性变换(linear transformation),线性函数(linear function);(零映射),(负映射),(矩阵的和),(负矩阵),(线性映射的标量乘积),(矩阵的标量乘积),(矩阵的乘积),(零因子),(标量矩阵(scalar matrix)),(矩阵的多项式);(退化的(degenerate)方阵),(非退化的(non-degenerate)方阵),(退化的线性变换),(非退化的线性变换),(逆矩阵(inverse matrix)),(可逆的(invertible),(伴随矩阵(adjoint matrix));(分块矩阵(block matrix)),(分块对角矩阵(block diagonal matrix));初等矩阵(elementary matrix),等价(equivalent);(象空间),(核空间(kernel)),(线性映射的秩),(零化度(nullity))==================================================================================== transpose of matrix 倒置矩阵; 转置矩阵【数学词汇】transposed matrix 转置矩阵【机械专业词汇】matrix transpose 矩阵转置【主科技词汇】transposed inverse matrix 转置逆矩阵【数学词汇】transpose of a matrix 矩阵的转置【主科技词汇】permutation matrix 置换矩阵; 排列矩阵【主科技词汇】singular matrix 奇异矩阵; 退化矩阵; 降秩矩阵【主科技词汇】unitary matrix 单式矩阵; 酉矩阵; 幺正矩阵【主科技词汇】Hermitian matrix 厄密矩阵; 埃尔米特矩阵; 艾米矩阵【主科技词汇】inverse matrix 逆矩阵; 反矩阵; 反行列式; 矩阵反演; 矩阵求逆【主科技词汇】matrix notation 矩阵符号; 矩阵符号表示; 矩阵记号; 矩阵运算【主科技词汇】state transition matrix 状态转变矩阵; 状态转移矩阵【航海航天词汇】torque master 转矩传感器; 转矩检测装置【主科技词汇】spin matrix 自旋矩阵; 旋转矩阵【主科技词汇】moment matrix 动差矩阵; 矩量矩阵【航海航天词汇】Jacobian matrix 雅可比矩阵; 导数矩阵【主科技词汇】relay matrix 继电器矩阵; 插接矩阵【主科技词汇】matrix notation 矩阵表示法; 矩阵符号【航海航天词汇】permutation matrix 置换矩阵【航海航天词汇】transition matrix 转移矩阵【数学词汇】transition matrix 转移矩阵【机械专业词汇】transitionmatrix 转移矩阵【航海航天词汇】transition matrix 转移矩阵【计算机网络词汇】transfer matrix 转移矩阵【物理词汇】rotation matrix 旋转矩阵【石油词汇】transition matrix 转换矩阵【主科技词汇】circulant matrix 循环矩阵; 轮换矩阵【主科技词汇】payoff matrix 报偿矩阵; 支付矩阵【主科技词汇】switching matrix 开关矩阵; 切换矩阵【主科技词汇】method of transition matrices 转换矩阵法【航海航天词汇】stalling torque 堵转力矩, 颠覆力矩, 停转转矩, 逆转转矩【航海航天词汇】thin-film switching matrix 薄膜转换矩阵【航海航天词汇】rotated factor matrix 旋转因子矩阵【航海航天词汇】transfer function matrix 转移函数矩阵【航海航天词汇】transition probability matrix 转移概率矩阵【主科技词汇】energy transfer matrix 能量转移矩阵【主科技词汇】fuzzy transition matrix 模糊转移矩阵【主科技词汇】canonical transition matrix 规范转移矩阵【主科技词汇】matrix form 矩阵式; 矩阵组织【主科技词汇】stochastic state transition matrix 随机状态转移矩阵【主科技词汇】fuzzy state transition matrix 模糊状态转移矩阵【主科技词汇】matrix compiler 矩阵编码器; 矩阵编译程序【主科技词汇】test matrix 试验矩阵; 测试矩阵; 检验矩阵【主科技词汇】matrix circuit 矩阵变换电路; 矩阵线路【主科技词汇】reducible matrix 可简化的矩阵; 可约矩阵【主科技词汇】matrix norm 矩阵的模; 矩阵模; 矩阵模量【主科技词汇】rectangular matrix 矩形矩阵; 长方形矩阵【主科技词汇】running torque 额定转速时的转矩; 旋转力矩【航海航天词汇】transposed matrix 转置阵【数学词汇】covariance matrix 协变矩阵; 协方差矩阵【主科技词汇】unreduced matrix 未约矩阵; 不可约矩阵【主科技词汇】receiver matrix 接收机矩阵; 接收矩阵变换电路【主科技词汇】torque 传动转矩; 转矩; 阻力矩【航海航天词汇】pull-in torque 启动转矩; 输入转矩, 同步转矩, 整步转矩【航海航天词汇】parity matrix 奇偶校验矩阵; 一致校验矩阵【主科技词汇】bus admittance matrix 母线导纳矩阵; 节点导纳矩阵【主科技词汇】matrix printer 矩阵式打印机; 矩阵形印刷机; 点阵打印机【主科技词汇】dynamic matrix 动力矩阵; 动态矩阵【航海航天词汇】connection matrix 连接矩阵; 连通矩阵【主科技词汇】characteristic matrix 特征矩阵; 本征矩阵【主科技词汇】regular matrix 正则矩阵; 规则矩阵【主科技词汇】flexibility matrix 挠度矩阵; 柔度矩阵【主科技词汇】citation matrix 引文矩阵; 引用矩阵【主科技词汇】relational matrix 关系矩阵; 联系矩阵【主科技词汇】eigenmatrix 本征矩阵; 特征矩阵【主科技词汇】system matrix 系统矩阵; 体系矩阵【主科技词汇】system matrix 系数矩阵; 系统矩阵【航海航天词汇】recovery diode matrix 恢复二极管矩阵; 再生式二极管矩阵【主科技词汇】inverse of a square matrix 方阵的逆矩阵【主科技词汇】torquematic transmission 转矩传动装置【石油词汇】torque balancing device 转矩平衡装置【航海航天词汇】torque measuring device 转矩测量装置【主科技词汇】torque measuring apparatus 转矩测量装置【航海航天词汇】torque-tube type suspension 转矩管式悬置【主科技词汇】steering torque indicator 转向力矩测定仪; 转向转矩指示器【主科技词汇】magnetic dipole moment matrix 磁偶极矩矩阵【主科技词汇】matrix addressing 矩阵寻址; 矩阵寻址时频矩阵编址; 时频矩阵编址【航海航天词汇】stiffness matrix 劲度矩阵; 刚度矩阵; 劲度矩阵【航海航天词汇】first-moment matrix 一阶矩矩阵【主科技词汇】matrix circuit 矩阵变换电路; 矩阵电路【计算机网络词汇】reluctance torque 反应转矩; 磁阻转矩【主科技词汇】pull-in torque 启动转矩; 牵入转矩【主科技词汇】induction torque 感应转矩; 异步转矩【主科技词汇】nominal torque 额定转矩; 公称转矩【航海航天词汇】phototronics 矩阵光电电子学; 矩阵光电管【主科技词汇】column matrix 列矩阵; 直列矩阵【主科技词汇】inverse of a matrix 矩阵的逆; 逆矩阵【主科技词汇】lattice matrix 点阵矩阵【数学词汇】lattice matrix 点阵矩阵【物理词汇】canonical matrix 典型矩阵; 正则矩阵; 典型阵; 正则阵【航海航天词汇】moment matrix 矩量矩阵【主科技词汇】moment matrix 矩量矩阵【数学词汇】dynamic torque 动转矩; 加速转矩【主科技词汇】indecomposable matrix 不可分解矩阵; 不能分解矩阵【主科技词汇】printed matrix wiring 印刷矩阵布线; 印制矩阵布线【主科技词汇】decoder matrix circuit 解码矩阵电路; 译码矩阵电路【航海航天词汇】scalar matrix 标量矩阵; 标量阵; 纯量矩阵【主科技词汇】array 矩阵式组织; 数组; 阵列【计算机网络词汇】commutative matrix 可换矩阵; 可交换矩阵【主科技词汇】标准文档实用文案。
线性代数英文版第七版课程设计
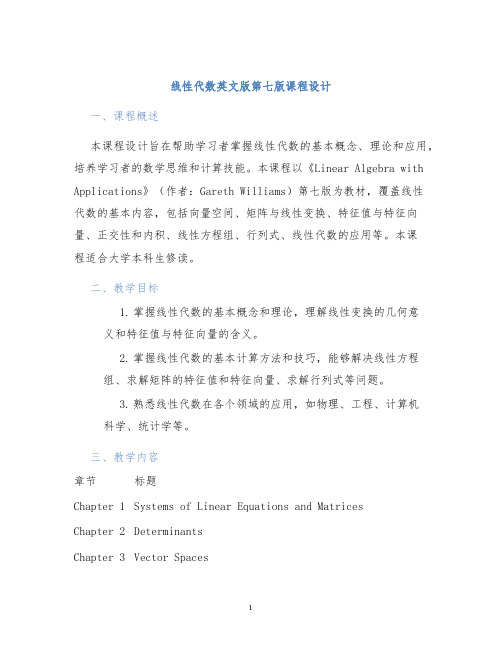
线性代数英文版第七版课程设计一、课程概述本课程设计旨在帮助学习者掌握线性代数的基本概念、理论和应用,培养学习者的数学思维和计算技能。
本课程以《Linear Algebra with Applications》(作者:Gareth Williams)第七版为教材,覆盖线性代数的基本内容,包括向量空间、矩阵与线性变换、特征值与特征向量、正交性和内积、线性方程组、行列式、线性代数的应用等。
本课程适合大学本科生修读。
二、教学目标1.掌握线性代数的基本概念和理论,理解线性变换的几何意义和特征值与特征向量的含义。
2.掌握线性代数的基本计算方法和技巧,能够解决线性方程组、求解矩阵的特征值和特征向量、求解行列式等问题。
3.熟悉线性代数在各个领域的应用,如物理、工程、计算机科学、统计学等。
三、教学内容章节标题Chapter 1 Systems of Linear Equations and MatricesChapter 2 DeterminantsChapter 3 Vector Spaces章节标题Chapter 4 Eigenvectors and EigenvaluesChapter 5 Orthogonality and Inner ProductChapter 6 Linear TransformationsChapter 7 Applications四、教学方法本课程采用讲授与练习相结合的教学方法,通过教师讲解、课堂讨论、案例分析等方式进行知识传授和交流,通过作业、实验等方式进行知识的巩固和应用。
在教学过程中,将强调数学思维的培养和计算技能的训练,鼓励学生积极思考和探究,提高学生的自主学习能力。
五、教学评估本课程的评估方式将采用考试、作业、实验等方式结合的方式进行。
具体如下:1.期末考试(占总评成绩70%),主要测试学生对于线性代数理论知识和计算技能的掌握程度。
2.作业(占总评成绩20%),包括每周指定的练习题和阶段性的大作业,主要测试学生的独立思考和解决问题的能力。
线性代数导论(一)向量介绍

线性代数导论(⼀)向量介绍参考资料:《Introduction to linear algebra》4th edition by Gilbert Strang思考:(⼀)相似矩阵是同⼀个线性变换的不同描述矩阵(⼆)对象的变换等价于坐标系的变换(运动是相对的),如对于变换Ma=b,可以看作Ma=Ib,坐标系M和I类似于环境声明,a和b为相应坐标系下的坐标向量;求解上述变换可得Ia=IM−1b,当坐标系声明均为I时,可得a=M−1b1. 线性组合:向量加法和标量乘法的组合其中,c,d为标量,v,w为向量2. 向量点积/内积:对应元素相乘后相加(1)点积为0,则表⽰两个向量相互垂直(cosθ=0,夹⾓为90度)(2)向量的长度:向量与⾃⾝点积的平⽅根(3)单位向量的长度为1,可以通过⾮零向量与⾃⾝长度相除得到(4)两个单位向量的点积即为夹⾓的余弦值(夹⾓⼩于90度为正,夹⾓⼤于90度为负)例如,当u为单位旋转向量(cosθ,sinθ),i为单位向量(1,0)时,u⋅i=cosθ;同时旋转α,即β=θ+α,点积为cosαcosβ+sinαsinβ=cos(β−α)=cosθ(5)当向量不是单位向量时,相应的余弦定理如下:由于|cosθ|≤1,可以得到施⽡茨不等式(内积的绝对值不超过长度的乘积)和三⾓不等式(两边之和⼤于第三边)如下:注:三⾓不等式的证明如下∥v+w∥2=∥v∥2+2vw+∥w∥2≤∥v∥2+2∥v∥∥w∥+∥w∥2=(∥v∥+∥w∥)2当v=(a,b),w=(b,a)时,施⽡茨不等式为:2ab≤a2+b2;令x=a2,y=b2,可以得到⼏何均值√xy⼩于算数均值12(x+y)的结论:3. 矩阵和向量的乘积可以看作是矩阵各列的线性组合4. 向量线性⽆关和线性相关线性相关的n∗n⽅阵不可逆Processing math: 100%。
向量相等的定义
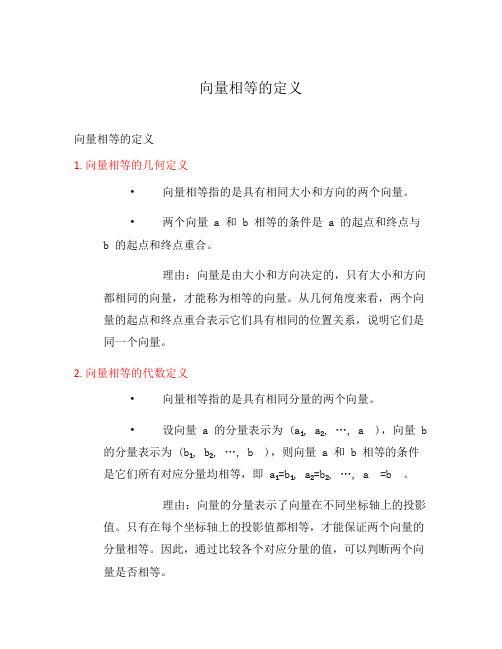
向量相等的定义向量相等的定义1. 向量相等的几何定义•向量相等指的是具有相同大小和方向的两个向量。
•两个向量 a 和 b 相等的条件是 a 的起点和终点与b 的起点和终点重合。
理由:向量是由大小和方向决定的,只有大小和方向都相同的向量,才能称为相等的向量。
从几何角度来看,两个向量的起点和终点重合表示它们具有相同的位置关系,说明它们是同一个向量。
2. 向量相等的代数定义•向量相等指的是具有相同分量的两个向量。
•设向量 a 的分量表示为 (a₁, a₂, …, aₙ),向量 b 的分量表示为 (b₁, b₂, …, bₙ),则向量 a 和 b 相等的条件是它们所有对应分量均相等,即 a₁=b₁, a₂=b₂, …, aₙ=bₙ。
理由:向量的分量表示了向量在不同坐标轴上的投影值。
只有在每个坐标轴上的投影值都相等,才能保证两个向量的分量相等。
因此,通过比较各个对应分量的值,可以判断两个向量是否相等。
书籍简介《线性代数及其应用》(Linear Algebra and Its Applications)该书由 Gilbert Strang(吉尔伯特·斯特郎)教授编写,是一本经典的线性代数教材。
本书系统地介绍了线性代数的基本理论和方法,并通过大量的例题和应用案例深入讲解线性代数的各个知识点和概念。
书中内容丰富、易于理解,适合作为线性代数的入门教材或参考书使用。
该书的第一章《Introduction to Vectors》详细讨论了向量及其基本性质,包括向量的相等定义、向量的加法和减法等运算。
通过学习该章节,读者可以对向量相等的定义有一个全面的了解,并能够理解向量的基本运算规则。
总之,《线性代数及其应用》这本书是一个很好的学习线性代数和向量概念的参考书,其中的第一章对向量相等的定义进行了详细讲解,对于理解向量相等的概念和判断两个向量是否相等具有很大帮助。
3. 向量相等的坐标定义•向量相等指的是具有相同坐标表示的两个向量。
线性代数教学资料—chapter3共75页文档
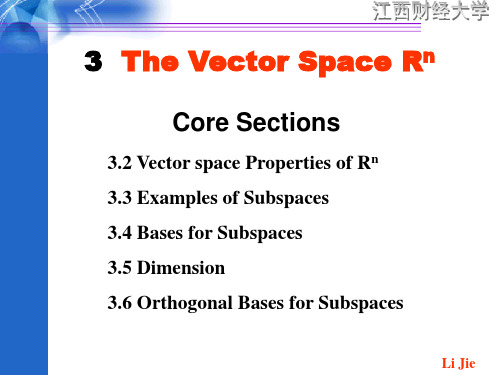
3.1 Introduction
In mathematics and the physical sciences, the term vector is applied to a wide variety of objects. Perhaps the most familiar application of the term is to quantities, such as force and velocity, that have both magnitude and direction. Such vectors can be represented in two space or in three space as directed line segments or arrows. As we will see in chapter 5,the term vector may also be used to describe objects such as matrices , polynomials, and continuous realvalued functions.
Li Jie
In this section we demonstrate that Rn, the set of n-dimensional vectors, provides a natural bridge between the intuitive and natural concept of a geometric vector and that of an abstract vector in a general vector space.
We now introduce two subspaces that have particular relevance to the linear system of equations Ax=b, where A is an (m×n) matrix. The first of these subspaces is called the null space of A (or the kernel of A) and consists of all solutions of Ax=θ. Definition 1:Let A be an (m × n) matrix. The null space of A [denoted N(A)] is the set of vectors in Rn defined by
IntroductionToLinearAlgebra第三版Wellesley-CambridgeP
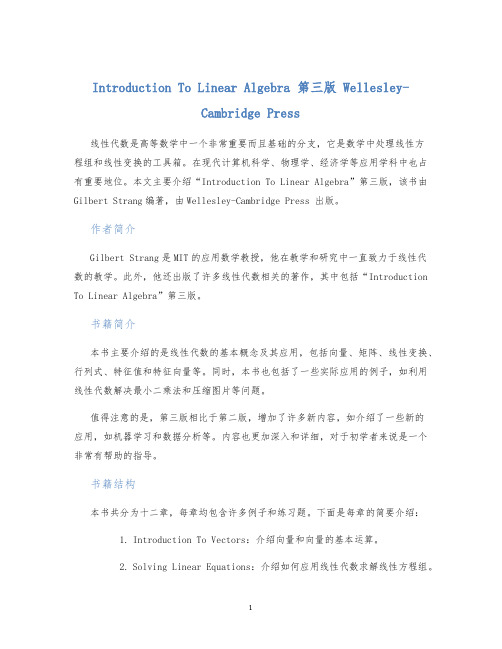
Introduction To Linear Algebra 第三版 Wellesley-Cambridge Press线性代数是高等数学中一个非常重要而且基础的分支,它是数学中处理线性方程组和线性变换的工具箱。
在现代计算机科学、物理学、经济学等应用学科中也占有重要地位。
本文主要介绍“Introduction To Linear Algebra”第三版,该书由Gilbert Strang编著,由Wellesley-Cambridge Press 出版。
作者简介Gilbert Strang是MIT的应用数学教授,他在教学和研究中一直致力于线性代数的教学。
此外,他还出版了许多线性代数相关的著作,其中包括“Introduction To Linear Algebra”第三版。
书籍简介本书主要介绍的是线性代数的基本概念及其应用,包括向量、矩阵、线性变换、行列式、特征值和特征向量等。
同时,本书也包括了一些实际应用的例子,如利用线性代数解决最小二乘法和压缩图片等问题。
值得注意的是,第三版相比于第二版,增加了许多新内容,如介绍了一些新的应用,如机器学习和数据分析等。
内容也更加深入和详细,对于初学者来说是一个非常有帮助的指导。
书籍结构本书共分为十二章,每章均包含许多例子和练习题。
下面是每章的简要介绍:1.Introduction To Vectors:介绍向量和向量的基本运算。
2.Solving Linear Equations:介绍如何应用线性代数求解线性方程组。
3.Vector Spaces And Subspaces:介绍向量空间和子空间的概念及其性质。
4.Orthogonality:介绍正交向量、正交矩阵、Gram-Schmidt正交化过程以及投影的概念。
5.Determinants:介绍行列式及其性质,以及如何计算行列式。
6.Eigenvalues And Eigenvectors:介绍特征值和特征向量以及它们的应用。
高等数学 国外经典教材
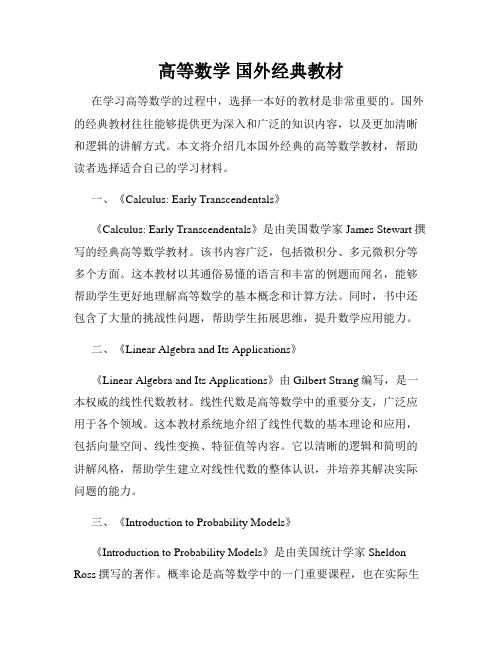
高等数学国外经典教材在学习高等数学的过程中,选择一本好的教材是非常重要的。
国外的经典教材往往能够提供更为深入和广泛的知识内容,以及更加清晰和逻辑的讲解方式。
本文将介绍几本国外经典的高等数学教材,帮助读者选择适合自己的学习材料。
一、《Calculus: Early Transcendentals》《Calculus: Early Transcendentals》是由美国数学家James Stewart撰写的经典高等数学教材。
该书内容广泛,包括微积分、多元微积分等多个方面。
这本教材以其通俗易懂的语言和丰富的例题而闻名,能够帮助学生更好地理解高等数学的基本概念和计算方法。
同时,书中还包含了大量的挑战性问题,帮助学生拓展思维,提升数学应用能力。
二、《Linear Algebra and Its Applications》《Linear Algebra and Its Applications》由Gilbert Strang编写,是一本权威的线性代数教材。
线性代数是高等数学中的重要分支,广泛应用于各个领域。
这本教材系统地介绍了线性代数的基本理论和应用,包括向量空间、线性变换、特征值等内容。
它以清晰的逻辑和简明的讲解风格,帮助学生建立对线性代数的整体认识,并培养其解决实际问题的能力。
三、《Introduction to Probability Models》《Introduction to Probability Models》是由美国统计学家Sheldon Ross撰写的著作。
概率论是高等数学中的一门重要课程,也在实际生活中有广泛应用。
这本教材全面介绍了概率论的基本概念、方法和应用,如概率分布、随机变量、极限定理等。
与其他教材相比,该书在例题选择和解题技巧上更具有创新性,能够帮助学生更好地理解和掌握概率论的知识。
四、《Differential Equations and Their Applications》《Differential Equations and Their Applications》是经典的常微分方程教材,作者是美国数学家Martin Braun。
IntroductiontoLinearAlgebra第二版课程设计
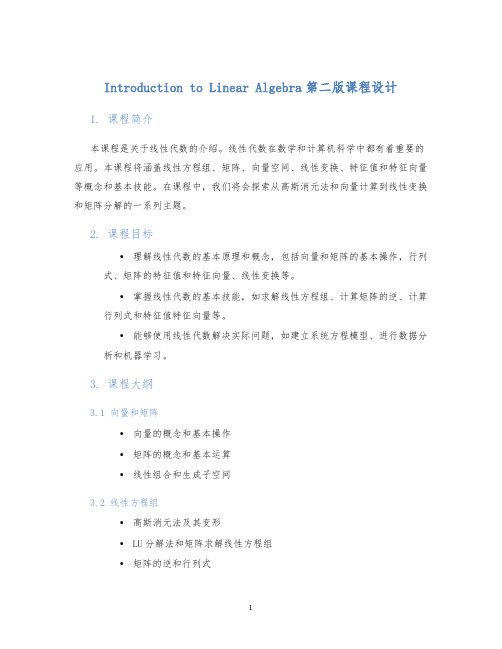
Introduction to Linear Algebra第二版课程设计1. 课程简介本课程是关于线性代数的介绍。
线性代数在数学和计算机科学中都有着重要的应用。
本课程将涵盖线性方程组、矩阵、向量空间、线性变换、特征值和特征向量等概念和基本技能。
在课程中,我们将会探索从高斯消元法和向量计算到线性变换和矩阵分解的一系列主题。
2. 课程目标•理解线性代数的基本原理和概念,包括向量和矩阵的基本操作,行列式、矩阵的特征值和特征向量、线性变换等。
•掌握线性代数的基本技能,如求解线性方程组、计算矩阵的逆、计算行列式和特征值特征向量等。
•能够使用线性代数解决实际问题,如建立系统方程模型、进行数据分析和机器学习。
3. 课程大纲3.1 向量和矩阵•向量的概念和基本操作•矩阵的概念和基本运算•线性组合和生成子空间3.2 线性方程组•高斯消元法及其变形•LU分解法和矩阵求解线性方程组•矩阵的逆和行列式3.3 基本矩阵操作和应用•矩阵乘法和转置•矩阵的秩和行空间、列空间、零空间•最小二乘法和矩阵的伪逆3.4 特征值和特征向量•线性变换和特征值特征向量的概念•对角化和相似矩阵•应用:对于连续变量模型的解析解和感性理解4. 教学方法本课程采用讲授、课堂互动和实验等多种教学方法。
•讲授:讲解课程重点和难点,引导学生理解和掌握知识点。
•课堂互动:通过提问和讨论等方式加强师生互动,促进学生主动参与和思考。
•实验:设置实验环节,让学生通过实践巩固知识点,培养解决问题的能力。
5. 评估方式本课程采用多种评估方式,包括作业、考试和小组项目等。
•作业:布置课堂和课后作业,帮助学生深入理解和掌握知识点。
•考试:每学期末进行闭卷考试,测试学生对于知识点的掌握和应用能力。
•小组项目:小组合作完成一个基于线性代数的实际问题或者算法实现,评估学生对于知识的实际运用能力。
6. 参考书目•Gilbert Strang. Introduction to Linear Algebra. [第二版]。
introduction to linear algebra 5th edition 中译
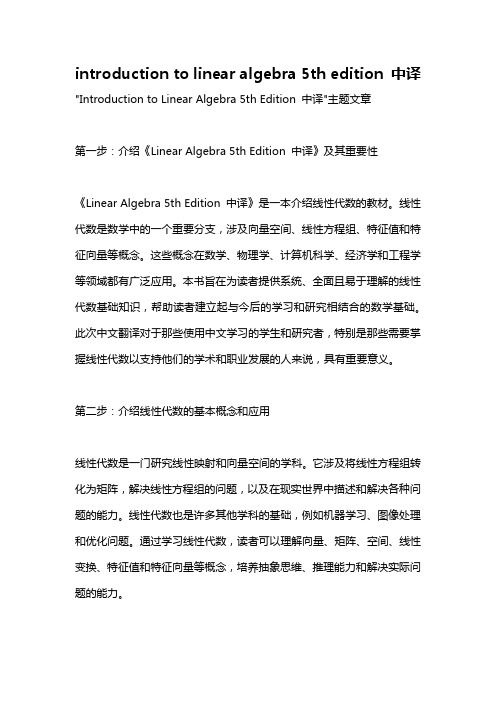
introduction to linear algebra 5th edition 中译"Introduction to Linear Algebra 5th Edition 中译"主题文章第一步:介绍《Linear Algebra 5th Edition 中译》及其重要性《Linear Algebra 5th Edition 中译》是一本介绍线性代数的教材。
线性代数是数学中的一个重要分支,涉及向量空间、线性方程组、特征值和特征向量等概念。
这些概念在数学、物理学、计算机科学、经济学和工程学等领域都有广泛应用。
本书旨在为读者提供系统、全面且易于理解的线性代数基础知识,帮助读者建立起与今后的学习和研究相结合的数学基础。
此次中文翻译对于那些使用中文学习的学生和研究者,特别是那些需要掌握线性代数以支持他们的学术和职业发展的人来说,具有重要意义。
第二步:介绍线性代数的基本概念和应用线性代数是一门研究线性映射和向量空间的学科。
它涉及将线性方程组转化为矩阵,解决线性方程组的问题,以及在现实世界中描述和解决各种问题的能力。
线性代数也是许多其他学科的基础,例如机器学习、图像处理和优化问题。
通过学习线性代数,读者可以理解向量、矩阵、空间、线性变换、特征值和特征向量等概念,培养抽象思维、推理能力和解决实际问题的能力。
第三步:介绍《Linear Algebra 5th Edition》的特点和优势《Linear Algebra 5th Edition 中译》具有以下特点和优势:1.系统全面:本书从基本概念开始介绍线性代数,逐步展开。
读者可以完整地掌握线性代数的核心概念和理论。
2.易于理解:作者使用简洁明了的语言和示例,帮助读者深入理解抽象的线性代数概念。
此外,作者还提供了大量的习题和解答,以巩固和测试理解。
3.应用广泛:本书覆盖了线性代数的许多应用领域,如物理学、计算机科学和经济学。
读者可以从实际问题出发,将所学的线性代数知识应用到实际中。
线性代数的应用论文
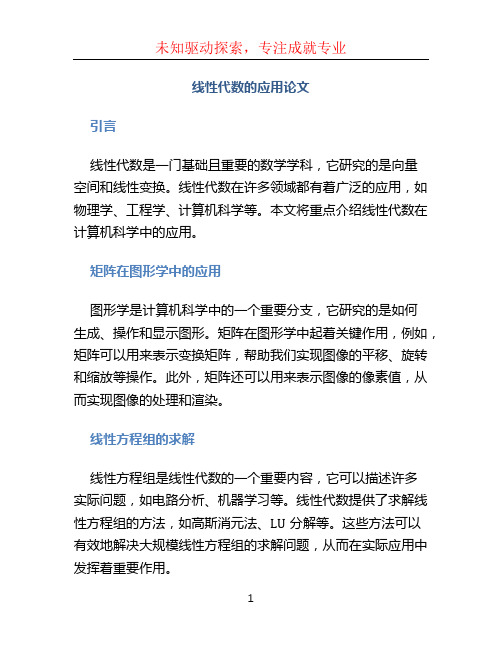
线性代数的应用论文引言线性代数是一门基础且重要的数学学科,它研究的是向量空间和线性变换。
线性代数在许多领域都有着广泛的应用,如物理学、工程学、计算机科学等。
本文将重点介绍线性代数在计算机科学中的应用。
矩阵在图形学中的应用图形学是计算机科学中的一个重要分支,它研究的是如何生成、操作和显示图形。
矩阵在图形学中起着关键作用,例如,矩阵可以用来表示变换矩阵,帮助我们实现图像的平移、旋转和缩放等操作。
此外,矩阵还可以用来表示图像的像素值,从而实现图像的处理和渲染。
线性方程组的求解线性方程组是线性代数的一个重要内容,它可以描述许多实际问题,如电路分析、机器学习等。
线性代数提供了求解线性方程组的方法,如高斯消元法、LU分解等。
这些方法可以有效地解决大规模线性方程组的求解问题,从而在实际应用中发挥着重要作用。
特征值与特征向量的应用特征值与特征向量是线性代数中的重要概念,它们可以帮助我们理解矩阵的性质和变换过程。
在图像处理中,特征值与特征向量可以用来实现图像的降噪和特征提取。
此外,在机器学习中,特征值与特征向量可以用来进行数据降维和特征选择,从而提高模型的性能和效果。
线性代数在机器学习中的应用机器学习是人工智能的一个重要领域,它研究的是如何使用数据和算法来构建模型并进行预测和决策。
线性代数在机器学习中起着关键作用,例如,线性回归模型和逻辑回归模型都是基于线性代数的理论和方法构建的。
此外,矩阵分解和特征值分解等线性代数的技术也被广泛应用于机器学习的算法中。
结论线性代数作为一门基础学科,其在计算机科学领域的应用非常重要。
本文简要介绍了线性代数在图形学、线性方程组求解、特征值与特征向量以及机器学习中的应用。
随着计算机科学的发展,线性代数的应用领域也将不断扩大,带来更多的创新和发展机会。
希望本文对读者了解线性代数在计算机科学中的应用有所帮助,并激发更多的兴趣和思考。
感谢阅读!参考文献•Strang, G. (2009). Introduction to Linear Algebra.Wellesley-Cambridge Press.•Lay, D.C., Lay, S.R., & McDonald, J.J. (2016). Linear Algebra and Its Applications. Pearson.。
线性代数英语短句带翻译

Translation: 两个垂直的向量的点积为零。
The cross product of two vectors is a vector that is perpendicular to both vectors and has a magnitude equal to the product of their magnitudes and the sine of the angle between them.
Translation: 一个向量是具有大小和方向的量。在数学中,向量通常用在字母上方的箭头表示,如 → 。
Example Sentence: The displacement of an object can be represented by a vector.
Translation: 物体的位移可以用一个向量来表示。
Translation: 一个矩阵是以行和列排列的数字或符号的矩形阵列。矩阵通常用大写字母来表示,如 。
Example Sentence: Matrix multiplication is not commutative.
Translation: 矩阵乘法不满足交换律。
5. Eigenvalues and Eigenvectors
2. Vector Addition and Subtraction
To add or subtract two vectors, we can combine their corresponding components.
Translation: 要对两个向量进行加法或减法运算,我们可以对它们的对应分量进行组合。
- 1、下载文档前请自行甄别文档内容的完整性,平台不提供额外的编辑、内容补充、找答案等附加服务。
- 2、"仅部分预览"的文档,不可在线预览部分如存在完整性等问题,可反馈申请退款(可完整预览的文档不适用该条件!)。
- 3、如文档侵犯您的权益,请联系客服反馈,我们会尽快为您处理(人工客服工作时间:9:00-18:30)。
An introduction to vectorsDefinition of a vectorA vector is an object that has both a magnitude and a direction. Geometrically, we can picture a vector as a directed line segment, whose length is the magnitude of the vector and with an arrow indicating the direction. The direction of the vector is from its tail to its head.Two vectors are the same if they have the same magnitude and direction. This means that if we take a vector and translate it to a new position (without rotating it), then the vector we obtain at the end of this process is the same vector we had in the beginning.Two examples of vectors are those that represent force and velocity. Both force and velocity are in a particular direction. The magnitude of the vector would indicate the strength of the force or the speed associated with the velocity.We denote vectors using boldface as in aor b. Especially when writing by hand where one cannot easily write in boldface, people will sometimes denote vectors using arrows as in a or b, or they use other markings. We won't need to use arrows here. We denote the magnitude of the vector a by ∥a∥. When we want to refer to a number and stress that it is not a vector, we can call the number a scalar. We willdenote scalars with italics, as in a or b.You can explore the concept of the magnitude and direction of a vector using the below applet. Note that moving the vector around doesn't change the vector, as the position of the vector doesn't affect the magnitude or the direction. But if you stretch or turn the vector by moving just its head or its tail, the magnitude or direction will change. (This applet also shows the coordinates of the vector, which you can read about in another page.)The magnitude and direction of a vector. The blue arrow represents a vector a. The two defining properties of a vector, magnitude and direction, are illustrated by a red bar and a green arrow, respectively. The length of the red bar is the magnitude ∥a∥ of the vector a. The green arrow always has length one, but its direction is the direction of the vector a.The one exception is when a is the zero vector (the only vector with zero magnitude), for which the direction is not defined. You can change either end of a by dragging it with your mouse. You can also move a by dragging the middle of the vector; however, changing the position of the ain this way does not change the vector, as its magnitude and direction remain unchanged. More information about applet.There is one important exception to vectors having a direction. The zero vector, denoted by a boldface 0, is the vector of zero length. Since it has no length, it is not pointing in any particular direction. There is only one vector of zero length, so we can speak of the zero vector. Operations on vectorsWe can define a number of operations on vectors geometrically without reference to any coordinate system. Here we define addition, subtraction, and multiplication by a scalar. On separate pages, we discuss two different ways to multiply two vectors together: the dot product and the cross product.Addition of vectorsGiven two vectors aand b, we form their sum a+b, as follows. We translate the vector b until its tail coincides with the head of a. (Recall such translation does not change a vector.) Then, the directed line segment from the tail of a to the head of b is the vector a+b.The vector addition is the way forces and velocities combine. For example, if a car is travelling due north at 20 miles per hour and a child in the back seat behind the driver throws an object at 20 miles per hour toward his sibling who is sitting due east of him, then the velocity of the object (relative to the ground!) will be in a north-easterly direction. The velocity vectors form a right triangle, where the total velocity is the hypotenuse. Therefore, the total speed of the object (i.e., the magnitude of the velocity vector) is202+202−−−−−−−−√=202√miles per hour relative to the ground.Addition of vectors satisfies two important properties.1.The commutative law, which states the order of addition doesn't matter:a+b=b+a.This law is also called the parallelogram law, as illustrated in the below image. Two of the edges of the parallelogram define a+b, and the other pair of edges define b+a∙ . But, both sums are equal to the same diagonal of the parallelogram.∙ The associative law, which states that the sum of three vectors does not depend on which pair of vectors is added first:(a+b)+c=a+(b+c).2.You can explore the properties of vector addition with the following applet. (This applet also shows the coordinates of the vectors, which you can read about in another page.)The sum of two vectors. The sum a+bof the vector a (blue arrow) and the vector b (red arrow) is shown by the green arrow. As vectors are independent of their starting position, both blue arrows represent the same vector a and both red arrows represent the same vector b. The sum a+b can be formed by placing the tail of the vector b at the head of the vector a. Equivalently, it can be formed by placing the tail of the vector a at the head of the vector b. Both constructions together form a parallelogram, with the sum a+b being a diagonal. (For this reason, the commutative lawa+b=b+a is sometimes called the parallelogram law.) You can change a and bby dragging the yellow points.Vector subtractionBefore we define subtraction, we define the vector −a, which is the opposite of a. The vector −a is the vector with the same magnitude as abut that is pointed in the opposite direction.We define subtraction as addition with the opposite of a vector:b−a=b+(−a).This is equivalent to turning vector a around in the applying the above rules for addition. Can you see how the vector x in the below figure is equal to b−a? Notice how this is the same as stating that a+x=b, just like with subtraction of scalar numbers.Scalar multiplicationGiven a vector aand a real number (scalar) λ, we can form the vector λa as follows. If λ is positive, then λa is the vector whose direction is the same as the direction of a and whose length is λ times the length of a. In this case, multiplication by λ simply stretches (if λ>1) or compresses (if0<λ<1) the vector a.If, on the other hand, λis negative, then we have to take the opposite of a before stretching or compressing it. In other words, the vector λa points in the opposite direction of a, and the length of λa is |λ| times the length of a. No matter the sign of λ, we observe that the magnitude of λa is |λ| times the magnitude of a: ∥λa∥=|λ|∥a∥.Scalar multiplications satisfies many of the same properties as the usual multiplication.1.s(a+b)=s a+s b∙ (distributive law, form 1)∙(s+t)a=s a+t a∙ (distributive law, form 2)∙1a=a∙∙(−1)a=−a∙∙0a=05.In the last formula, the zero on the left is the number 0, while the zero on the right is the vector 0, which is the unique vector whose length is zero.If a=λbfor some scalar λ, then we say that the vectors a and b are parallel. If λ is negative, some people say that a and bare anti-parallel, but we will not use that language.We were able to describe vectors, vector addition, vector subtraction, and scalar multiplication without reference to any coordinate system. The advantage of such purely geometric reasoning is that our results hold generally, independent of any coordinate system in which the vectors live. However, sometimes it is useful to express vectors in terms of coordinates, as discussed in a page about vectors in the standard Cartesian coordinate systems in the plane and in three-dimensional space.。