人脸识别源代码.doc
人脸识别代码
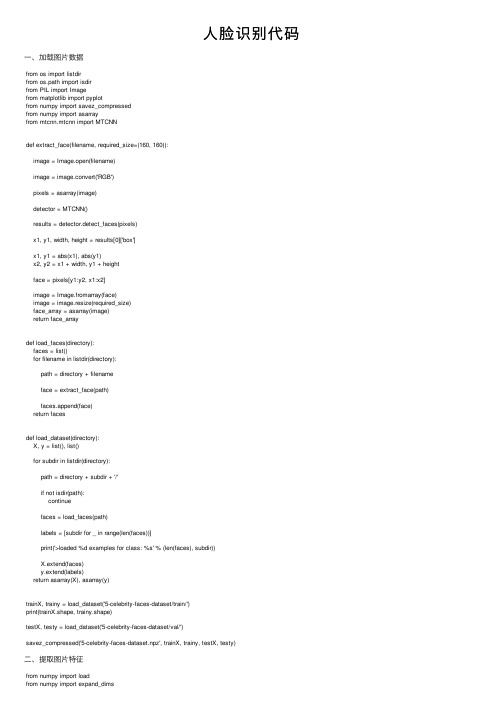
⼈脸识别代码⼀、加载图⽚数据from os import listdirfrom os.path import isdirfrom PIL import Imagefrom matplotlib import pyplotfrom numpy import savez_compressedfrom numpy import asarrayfrom mtcnn.mtcnn import MTCNNdef extract_face(filename, required_size=(160, 160)):image = Image.open(filename)image = image.convert('RGB')pixels = asarray(image)detector = MTCNN()results = detector.detect_faces(pixels)x1, y1, width, height = results[0]['box']x1, y1 = abs(x1), abs(y1)x2, y2 = x1 + width, y1 + heightface = pixels[y1:y2, x1:x2]image = Image.fromarray(face)image = image.resize(required_size)face_array = asarray(image)return face_arraydef load_faces(directory):faces = list()for filename in listdir(directory):path = directory + filenameface = extract_face(path)faces.append(face)return facesdef load_dataset(directory):X, y = list(), list()for subdir in listdir(directory):path = directory + subdir + '/'if not isdir(path):continuefaces = load_faces(path)labels = [subdir for _ in range(len(faces))]print('>loaded %d examples for class: %s' % (len(faces), subdir))X.extend(faces)y.extend(labels)return asarray(X), asarray(y)trainX, trainy = load_dataset('5-celebrity-faces-dataset/train/')print(trainX.shape, trainy.shape)testX, testy = load_dataset('5-celebrity-faces-dataset/val/')savez_compressed('5-celebrity-faces-dataset.npz', trainX, trainy, testX, testy)⼆、提取图⽚特征from numpy import loadfrom numpy import expand_dimsfrom numpy import savez_compressedfrom keras.models import load_modeldef get_embedding(model, face_pixels):face_pixels = face_pixels.astype('float32')mean, std = face_pixels.mean(), face_pixels.std()face_pixels = (face_pixels - mean) / stdsamples = expand_dims(face_pixels, axis=0)yhat = model.predict(samples)return yhat[0]data = load('5-celebrity-faces-dataset.npz')trainX, trainy, testX, testy = data['arr_0'], data['arr_1'], data['arr_2'], data['arr_3']print('Loaded: ', trainX.shape, trainy.shape, testX.shape, testy.shape)model = load_model('facenet_keras.h5')print('Loaded Model')newTrainX = list()for face_pixels in trainX:embedding = get_embedding(model, face_pixels)newTrainX.append(embedding)newTrainX = asarray(newTrainX)print(newTrainX.shape)newTestX = list()for face_pixels in testX:embedding = get_embedding(model, face_pixels)newTestX.append(embedding)newTestX = asarray(newTestX)print(newTestX.shape)# save arrays to one file in compressed formatsavez_compressed('5-celebrity-faces-embeddings.npz', newTrainX, trainy, newTestX, testy)三、识别from numpy import loadfrom sklearn.metrics import accuracy_scorefrom sklearn.preprocessing import LabelEncoderfrom sklearn.preprocessing import Normalizerfrom sklearn.svm import SVCdata = load('5-celebrity-faces-embeddings.npz')trainX, trainy, testX, testy = data['arr_0'], data['arr_1'], data['arr_2'], data['arr_3']print('Dataset: train=%d, test=%d' % (trainX.shape[0], testX.shape[0]))# normalize input vectorsin_encoder = Normalizer(norm='l2')trainX = in_encoder.transform(trainX)testX = in_encoder.transform(testX)# label encode targetsout_encoder = LabelEncoder()out_encoder.fit(trainy)trainy = out_encoder.transform(trainy)testy = out_encoder.transform(testy)# fit modelmodel = SVC(kernel='linear', probability=True)model.fit(trainX, trainy)# predictyhat_train = model.predict(trainX)yhat_test = model.predict(testX)# scorescore_train = accuracy_score(trainy, yhat_train)score_test = accuracy_score(testy, yhat_test)# summarizeprint('Accuracy: train=%.3f, test=%.3f' % (score_train*100, score_test*100))from random import choicefrom numpy import loadfrom numpy import expand_dimsfrom sklearn.preprocessing import LabelEncoderfrom sklearn.preprocessing import Normalizerfrom matplotlib import pyplotdata = load('5-celebrity-faces-dataset.npz')testX_faces = data['arr_2']data = load('5-celebrity-faces-embeddings.npz')trainX, trainy, testX, testy = data['arr_0'], data['arr_1'], data['arr_2'], data['arr_3'] in_encoder = Normalizer(norm='l2')trainX = in_encoder.transform(trainX)testX = in_encoder.transform(testX)out_encoder = LabelEncoder()out_encoder.fit(trainy)trainy = out_encoder.transform(trainy)testy = out_encoder.transform(testy)model = SVC(kernel='linear', probability=True)model.fit(trainX, trainy)selection = choice([i for i in range(testX.shape[0])])random_face_pixels = testX_faces[selection]random_face_emb = testX[selection]random_face_class = testy[selection]random_face_name = out_encoder.inverse_transform([random_face_class]) samples = expand_dims(random_face_emb, axis=0)yhat_class = model.predict(samples)yhat_prob = model.predict_proba(samples)class_index = yhat_class[0]class_probability = yhat_prob[0,class_index] * 100predict_names = out_encoder.inverse_transform(yhat_class)print('Predicted: %s (%.3f)' % (predict_names[0], class_probability))print('Expected: %s' % random_face_name[0])pyplot.imshow(random_face_pixels)title = '%s (%.3f)' % (predict_names[0], class_probability)pyplot.title(title)pyplot.show()。
教你如何用Python实现人脸识别(含源代码)
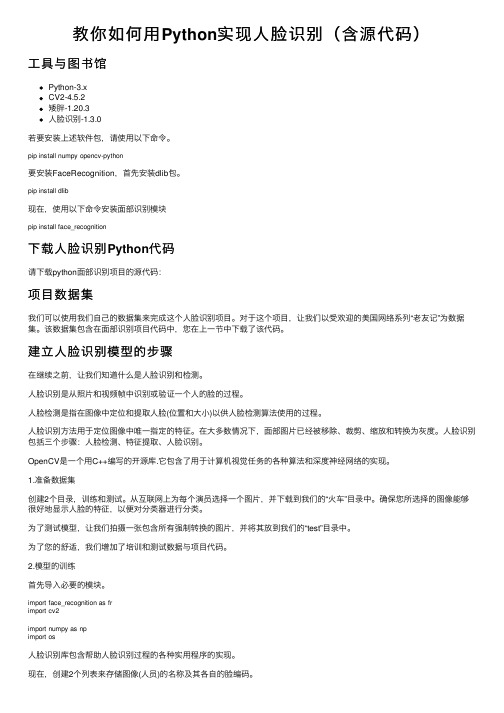
教你如何⽤Python实现⼈脸识别(含源代码)⼯具与图书馆Python-3.xCV2-4.5.2矮胖-1.20.3⼈脸识别-1.3.0若要安装上述软件包,请使⽤以下命令。
pip install numpy opencv-python要安装FaceRecognition,⾸先安装dlib包。
pip install dlib现在,使⽤以下命令安装⾯部识别模块pip install face_recognition下载⼈脸识别Python代码请下载python⾯部识别项⽬的源代码:项⽬数据集我们可以使⽤我们⾃⼰的数据集来完成这个⼈脸识别项⽬。
对于这个项⽬,让我们以受欢迎的美国⽹络系列“⽼友记”为数据集。
该数据集包含在⾯部识别项⽬代码中,您在上⼀节中下载了该代码。
建⽴⼈脸识别模型的步骤在继续之前,让我们知道什么是⼈脸识别和检测。
⼈脸识别是从照⽚和视频帧中识别或验证⼀个⼈的脸的过程。
⼈脸检测是指在图像中定位和提取⼈脸(位置和⼤⼩)以供⼈脸检测算法使⽤的过程。
⼈脸识别⽅法⽤于定位图像中唯⼀指定的特征。
在⼤多数情况下,⾯部图⽚已经被移除、裁剪、缩放和转换为灰度。
⼈脸识别包括三个步骤:⼈脸检测、特征提取、⼈脸识别。
OpenCV是⼀个⽤C++编写的开源库.它包含了⽤于计算机视觉任务的各种算法和深度神经⽹络的实现。
1.准备数据集创建2个⽬录,训练和测试。
从互联⽹上为每个演员选择⼀个图⽚,并下载到我们的“⽕车”⽬录中。
确保您所选择的图像能够很好地显⽰⼈脸的特征,以便对分类器进⾏分类。
为了测试模型,让我们拍摄⼀张包含所有强制转换的图⽚,并将其放到我们的“test”⽬录中。
为了您的舒适,我们增加了培训和测试数据与项⽬代码。
2.模型的训练⾸先导⼊必要的模块。
import face_recognition as frimport cv2import numpy as npimport os⼈脸识别库包含帮助⼈脸识别过程的各种实⽤程序的实现。
根据matlab的人脸识别源代码

function varargout = FR_Processed_histogram(varargin)%这种算法是基于直方图处理的方法%The histogram of image is calculated and then bin formation is done on the%basis of mean of successive graylevels frequencies. The training is done on odd images of 40 subjects (200 images out of 400 images)%The results of the implemented algorithm is 99.75 (recognition fails on image number 4 of subject 17)gui_Singleton = 1;gui_State = struct('gui_Name', mfilename, ...'gui_Singleton', gui_Singleton, ...'gui_OpeningFcn',@FR_Processed_histogram_OpeningFcn, ...'gui_OutputFcn',@FR_Processed_histogram_OutputFcn, ...'gui_LayoutFcn', [] , ...'gui_Callback', []);if nargin && ischar(varargin{1})gui_State.gui_Callback = str2func(varargin{1});endif nargout[varargout{1:nargout}] = gui_mainfcn(gui_State, varargin{:});elsegui_mainfcn(gui_State, varargin{:});end% End initialization code - DO NOT EDIT%--------------------------------------------------------------------------% --- Executes just before FR_Processed_histogram is made visible.function FR_Processed_histogram_OpeningFcn(hObject, eventdata, handles, varargin)% This function has no output args, see OutputFcn.% hObject handle to figure% eventdata reserved - to be defined in a future version of MATLAB% handles structure with handles and user data (see GUIDATA) % varargin command line arguments to FR_Processed_histogram (see VARARGIN)% Choose default command line output for FR_Processed_histogramhandles.output = hObject;% Update handles structureguidata(hObject, handles);% UIWAIT makes FR_Processed_histogram wait for user response (see UIRESUME)% uiwait(handles.figure1);global total_sub train_img sub_img max_hist_level bin_num form_bin_num;total_sub = 40;train_img = 200;sub_img = 10;max_hist_level = 256;bin_num = 9;form_bin_num = 29;%--------------------------------------------------------------------------% --- Outputs from this function are returned to the command line.function varargout = FR_Processed_histogram_OutputFcn(hObject, eventdata, handles)% varargout cell array for returning output args (see VARARGOUT);% hObject handle to figure% eventdata reserved - to be defined in a future version of MATLAB% handles structure with handles and user data (see GUIDATA)% Get default command line output from handles structurevarargout{1} = handles.output;%--------------------------------------------------------------------------% --- Executes on button press in train_button.function train_button_Callback(hObject, eventdata, handles)% hObject handle to train_button (see GCBO)% eventdata reserved - to be defined in a future version of MATLAB% handles structure with handles and user data (see GUIDATA)global train_processed_bin;global total_sub train_img sub_img max_hist_level bin_num form_bin_num;train_processed_bin(form_bin_num,train_img) = 0;K = 1;train_hist_img = zeros(max_hist_level, train_img);for Z=1:1:total_subfor X=1:2:sub_img %%%train on odd number of images of each subjectI = imread( strcat('ORL\S',int2str(Z),'\',int2str(X),'.bmp') );[rows cols] = size(I);for i=1:1:rowsfor j=1:1:colsif( I(i,j) == 0 )train_hist_img(max_hist_level, K) = train_hist_img(max_hist_level, K) + 1;elsetrain_hist_img(I(i,j), K) = train_hist_img(I(i,j), K) + 1;endendendK = K + 1;endend[r c] = size(train_hist_img);sum = 0;for i=1:1:cK = 1;for j=1:1:rif( (mod(j,bin_num)) == 0 )sum = sum + train_hist_img(j,i);train_processed_bin(K,i) = sum/bin_num;K = K + 1;sum = 0;elsesum = sum + train_hist_img(j,i);endendtrain_processed_bin(K,i) = sum/bin_num;enddisplay ('Training Done')save 'train'train_processed_bin;%--------------------------------------------------------------------------% --- Executes on button press in Testing_button.function Testing_button_Callback(hObject, eventdata, handles)% hObject handle to Testing_button (see GCBO)% eventdata reserved - to be defined in a future version of MATLAB% handles structure with handles and user data (see GUIDATA) global train_img max_hist_level bin_num form_bin_num;global train_processed_bin;global filename pathname Iload 'train'test_hist_img(max_hist_level) = 0;test_processed_bin(form_bin_num) = 0;[rows cols] = size(I);for i=1:1:rowsfor j=1:1:colsif( I(i,j) == 0 )test_hist_img(max_hist_level) =test_hist_img(max_hist_level) + 1;elsetest_hist_img(I(i,j)) = test_hist_img(I(i,j)) + 1;endendend[r c] = size(test_hist_img);sum = 0;K = 1;for j=1:1:cif( (mod(j,bin_num)) == 0 )sum = sum + test_hist_img(j);test_processed_bin(K) = sum/bin_num;K = K + 1;sum = 0;elsesum = sum + test_hist_img(j);endendtest_processed_bin(K) = sum/bin_num;sum = 0;K = 1;for y=1:1:train_imgfor z=1:1:form_bin_numsum = sum + abs( test_processed_bin(z) - train_processed_bin(z,y) );endimg_bin_hist_sum(K,1) = sum;sum = 0;K = K + 1;end[temp M] = min(img_bin_hist_sum);M = ceil(M/5);getString_start=strfind(pathname,'S');getString_start=getString_start(end)+1;getString_end=strfind(pathname,'\');getString_end=getString_end(end)-1;subjectindex=str2num(pathname(getString_start:getString_end));if (subjectindex == M)axes (handles.axes3)%image no: 5 is shown for visualization purposeimshow(imread(STRCAT('ORL\S',num2str(M),'\5.bmp')))msgbox ( 'Correctly Recognized');elsedisplay ([ 'Error==> Testing Image of Subject >>' num2str(subjectindex) ' matches with the image of subject >> ' num2str(M)])axes (handles.axes3)%image no: 5 is shown for visualization purposeimshow(imread(STRCAT('ORL\S',num2str(M),'\5.bmp')))msgbox ( 'Incorrectly Recognized');enddisplay('Testing Done')%--------------------------------------------------------------------------function box_Callback(hObject, eventdata, handles)% hObject handle to box (see GCBO)% eventdata reserved - to be defined in a future version ofMATLAB% handles structure with handles and user data (see GUIDATA)% Hints: get(hObject,'String') returns contents of box as text% str2double(get(hObject,'String')) returns contents of box as a double%--------------------------------------------------------------------------% --- Executes during object creation, after setting all properties.function box_CreateFcn(hObject, eventdata, handles)% hObject handle to box (see GCBO)% eventdata reserved - to be defined in a future version of MATLAB% handles empty - handles not created until after all CreateFcns called% Hint: edit controls usually have a white background on Windows.% See ISPC and COMPUTER.if ispc && isequal(get(hObject,'BackgroundColor'), get(0,'defaultUicontrolBackgroundColor'))set(hObject,'BackgroundColor','white');end%--------------------------------------------------------------------------% --- Executes on button press in Input_Image_button.function Input_Image_button_Callback(hObject, eventdata, handles) % hObject handle to Input_Image_button (see GCBO)% eventdata reserved - to be defined in a future version of MATLAB% handles structure with handles and user data (see GUIDATA) global filename pathname I[filename, pathname] = uigetfile('*.bmp', 'Test Image');axes(handles.axes1)imgpath=STRCAT(pathname,filename);I = imread(imgpath);imshow(I)%--------------------------------------------------------------------------% --- Executes during object creation, after setting all properties.function axes3_CreateFcn(hObject, eventdata, handles)% hObject handle to axes3 (see GCBO)% eventdata reserved - to be defined in a future version of MATLAB% handles empty - handles not created until after all CreateFcnscalled% Hint: place code in OpeningFcn to populate axes3 %Programmed by Usman Qayyum。
retinaface进行人脸检测的python代码
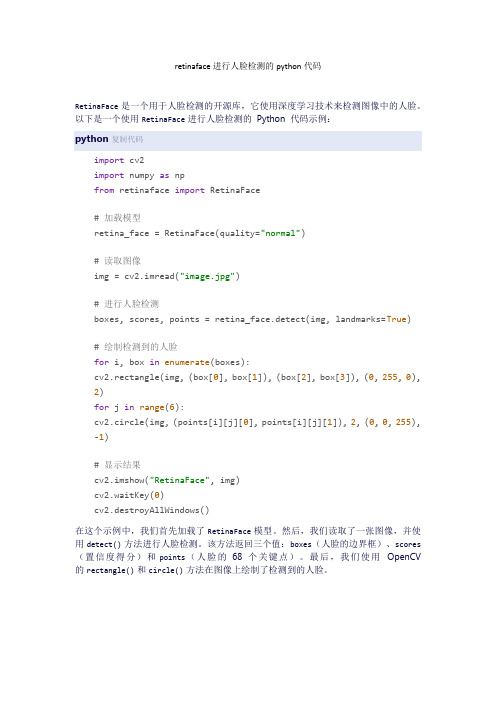
retinaface进行人脸检测的python代码RetinaFace是一个用于人脸检测的开源库,它使用深度学习技术来检测图像中的人脸。
以下是一个使用RetinaFace进行人脸检测的Python 代码示例:python复制代码import cv2import numpy as npfrom retinaface import RetinaFace# 加载模型retina_face = RetinaFace(quality="normal")# 读取图像img = cv2.imread("image.jpg")# 进行人脸检测boxes, scores, points = retina_face.detect(img, landmarks=True)# 绘制检测到的人脸for i, box in enumerate(boxes):cv2.rectangle(img, (box[0], box[1]), (box[2], box[3]), (0, 255, 0),2)for j in range(6):cv2.circle(img, (points[i][j][0], points[i][j][1]), 2, (0, 0, 255), -1)# 显示结果cv2.imshow("RetinaFace", img)cv2.waitKey(0)cv2.destroyAllWindows()在这个示例中,我们首先加载了RetinaFace模型。
然后,我们读取了一张图像,并使用detect()方法进行人脸检测。
该方法返回三个值:boxes(人脸的边界框)、scores (置信度得分)和points(人脸的68个关键点)。
最后,我们使用OpenCV 的rectangle()和circle()方法在图像上绘制了检测到的人脸。
人脸识别代码
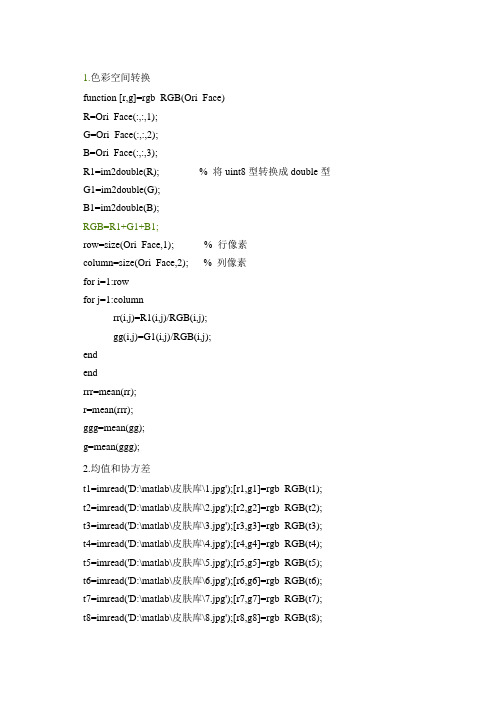
1.色彩空间转换function [r,g]=rgb_RGB(Ori_Face)R=Ori_Face(:,:,1);G=Ori_Face(:,:,2);B=Ori_Face(:,:,3);R1=im2double(R); % 将uint8型转换成double型G1=im2double(G);B1=im2double(B);RGB=R1+G1+B1;row=size(Ori_Face,1); % 行像素column=size(Ori_Face,2); % 列像素for i=1:rowfor j=1:columnrr(i,j)=R1(i,j)/RGB(i,j);gg(i,j)=G1(i,j)/RGB(i,j);endendrrr=mean(rr);r=mean(rrr);ggg=mean(gg);g=mean(ggg);2.均值和协方差t1=imread('D:\matlab\皮肤库\1.jpg');[r1,g1]=rgb_RGB(t1); t2=imread('D:\matlab\皮肤库\2.jpg');[r2,g2]=rgb_RGB(t2); t3=imread('D:\matlab\皮肤库\3.jpg');[r3,g3]=rgb_RGB(t3); t4=imread('D:\matlab\皮肤库\4.jpg');[r4,g4]=rgb_RGB(t4); t5=imread('D:\matlab\皮肤库\5.jpg');[r5,g5]=rgb_RGB(t5); t6=imread('D:\matlab\皮肤库\6.jpg');[r6,g6]=rgb_RGB(t6); t7=imread('D:\matlab\皮肤库\7.jpg');[r7,g7]=rgb_RGB(t7); t8=imread('D:\matlab\皮肤库\8.jpg');[r8,g8]=rgb_RGB(t8);t9=imread('D:\matlab\皮肤库\9.jpg');[r9,g9]=rgb_RGB(t9);t10=imread('D:\matlab\皮肤库\10.jpg');[r10,g10]=rgb_RGB(t10);t11=imread('D:\matlab\皮肤库\11.jpg');[r11,g11]=rgb_RGB(t11);t12=imread('D:\matlab\皮肤库\12.jpg');[r12,g12]=rgb_RGB(t12);t13=imread('D:\matlab\皮肤库\13.jpg');[r13,g13]=rgb_RGB(t13);t14=imread('D:\matlab\皮肤库\14.jpg');[r14,g14]=rgb_RGB(t14);t15=imread('D:\matlab\皮肤库\15.jpg');[r15,g15]=rgb_RGB(t15);t16=imread('D:\matlab\皮肤库\16.jpg');[r16,g16]=rgb_RGB(t16);t17=imread('D:\matlab\皮肤库\17.jpg');[r17,g17]=rgb_RGB(t17);t18=imread('D:\matlab\皮肤库\18.jpg');[r18,g18]=rgb_RGB(t18);t19=imread('D:\matlab\皮肤库\19.jpg');[r19,g19]=rgb_RGB(t19);t20=imread('D:\matlab\皮肤库\20.jpg');[r20,g20]=rgb_RGB(t20);t21=imread('D:\matlab\皮肤库\21.jpg');[r21,g21]=rgb_RGB(t21);t22=imread('D:\matlab\皮肤库\22.jpg');[r22,g22]=rgb_RGB(t22);t23=imread('D:\matlab\皮肤库\23.jpg');[r23,g23]=rgb_RGB(t23);t24=imread('D:\matlab\皮肤库\24.jpg');[r24,g24]=rgb_RGB(t24);t25=imread('D:\matlab\皮肤库\25.jpg');[r25,g25]=rgb_RGB(t25);t26=imread('D:\matlab\皮肤库\26.jpg');[r26,g26]=rgb_RGB(t26);t27=imread('D:\matlab\皮肤库\27.jpg');[r27,g27]=rgb_RGB(t27);r=cat(1,r1,r2,r3,r4,r5,r6,r7,r8,r9,r10,r11,r12,r13,r14,r15,r16,r17,r18,r19,r20,r21,r22, r23,r24,r25,r26,r27);g=cat(1,g1,g2,g3,g4,g5,g6,g7,g8,g9,g10,g11,g12,g13,g14,g15,g16,g17,g18,g19,g20 ,g21,g22,g23,g24,g25,g26,g27);m=mean([r,g])n=cov([r,g])3.求质心function [xmean, ymean] = center(bw)bw=bwfill(bw,'holes');area = bwarea(bw);[m n] =size(bw);bw=double(bw);xmean =0; ymean = 0;for i=1:m,for j=1:n,xmean = xmean + j*bw(i,j);ymean = ymean + i*bw(i,j);end;end;if(area==0)xmean=0;ymean=0;elsexmean = xmean/area;ymean = ymean/area;xmean = round(xmean);ymean = round(ymean);end4.求偏转角度function [theta] = orient(bw,xmean,ymean) [m n] =size(bw);bw=double(bw);a = 0;b = 0;c = 0;for i=1:m,for j=1:n,a = a + (j - xmean)^2 * bw(i,j);b = b + (j - xmean) * (i - ymean) * bw(i,j);c = c + (i - ymean)^2 * bw(i,j);end;end;b = 2 * b;theta = atan(b/(a-c))/2;theta = theta*(180/pi); % 从幅度转换到角度5.找区域边界function [left, right, up, down] = bianjie(A)[m n] = size(A);left = -1;right = -1;up = -1;down = -1;for j=1:n,for i=1:m,if (A(i,j) ~= 0)left = j;break;end;end;if (left ~= -1) break;end;end;for j=n:-1:1,for i=1:m,if (A(i,j) ~= 0)right = j;break;end;end;if (right ~= -1) break;end;end;for i=1:m,for j=1:n,if (A(i,j) ~= 0)up = i;break;end;end;if (up ~= -1)break;end;end;for i=m:-1:1,for j=1:n,if (A(i,j) ~= 0)down = i;break;end;end;if (down ~= -1)break;end;end;6.求起始坐标function newcoord = checklimit(coord,maxval) newcoord = coord;if (newcoord<1)newcoord=1;end;if (newcoord>maxval)newcoord=maxval;end;7.模板匹配function [ccorr, mfit, RectCoord] = mobanpipei(mult, frontalmodel,ly,wx,cx, cy, angle)frontalmodel=rgb2gray(frontalmodel);model_rot = imresize(frontalmodel,[ly wx],'bilinear'); % 调整模板大小model_rot = imrotate(model_rot,angle,'bilinear'); % 旋转模板[l,r,u,d] = bianjie(model_rot); % 求边界坐标bwmodel_rot=imcrop(model_rot,[l u (r-l) (d-u)]); % 选择模板人脸区域[modx,mody] =center(bwmodel_rot); % 求质心[morig, norig] = size(bwmodel_rot);% 产生一个覆盖了人脸模板的灰度图像mfit = zeros(size(mult));mfitbw = zeros(size(mult));[limy, limx] = size(mfit);% 计算原图像中人脸模板的坐标startx = cx-modx;starty = cy-mody;endx = startx + norig-1;endy = starty + morig-1;startx = checklimit(startx,limx);starty = checklimit(starty,limy);endx = checklimit(endx,limx);endy = checklimit(endy,limy);for i=starty:endy,for j=startx:endx,mfit(i,j) = model_rot(i-starty+1,j-startx+1);end;end;ccorr = corr2(mfit,mult) % 计算相关度[l,r,u,d] = bianjie(bwmodel_rot);sx = startx+l;sy = starty+u;RectCoord = [sx sy (r-1) (d-u)]; % 产生矩形坐标8.主程序clear;[fname,pname]=uigetfile({'*.jpg';'*.bmp';'*.tif';'*.gif'},'Please choose a color picture...');% 返回打开的图片名与图片路径名[u,v]=size(fname);y=fname(v); % 图片格式代表值switch ycase 0errordlg('You Should Load Image File First...','Warning...');case{'g';'G';'p';'P';'f';'F'}; % 图片格式若是JPG/jpg、BMP/bmp、TIF/tif 或者GIF/gif,才打开I=cat(2,pname,fname);Ori_Face=imread(I);subplot(2,3,1),imshow(Ori_Face);otherwiseerrordlg('You Should Load Image File First...','Warning...');endR=Ori_Face(:,:,1);G=Ori_Face(:,:,2);B=Ori_Face(:,:,3);R1=im2double(R); % 将uint8型转换成double型处理G1=im2double(G);B1=im2double(B);RGB=R1+G1+B1;m=[ 0.4144,0.3174]; % 均值n=[0.0031,-0.0004;-0.0004,0.0003]; % 方差row=size(Ori_Face,1); % 行像素数column=size(Ori_Face,2); % 列像素数for i=1:rowfor j=1:columnif RGB(i,j)==0rr(i,j)=0;gg(i,j)=0;elserr(i,j)=R1(i,j)/RGB(i,j); % rgb归一化gg(i,j)=G1(i,j)/RGB(i,j);x=[rr(i,j),gg(i,j)];p(i,j)=exp((-0.5)*(x-m)*inv(n)*(x-m)'); % 皮肤概率服从高斯分布endendendsubplot(2,3,2);imshow(p); % 显示皮肤灰度图像low_pass=1/9*ones(3);image_low=filter2(low_pass, p); % 低通滤波去噪声subplot(2,3,3);imshow(image_low);% 自适应阀值程序previousSkin2 = zeros(i,j);changelist = [];for threshold = 0.55:-0.1:0.05two_value = zeros(i,j);two_value(find(image_low>threshold)) = 1;change = sum(sum(two_value - previousSkin2));changelist = [changelist change];previousSkin2 = two_value;end[C, I] = min(changelist);optimalThreshold = (7-I)*0.1two_value = zeros(i,j);two_value(find(image_low>optimalThreshold)) = 1; % 二值化subplot(2,3,4);imshow(two_value); % 显示二值图像frontalmodel=imread('E:\我的照片\人脸模板.jpg'); % 读入人脸模板照片FaceCoord=[];imsourcegray=rgb2gray(Ori_Face); % 将原照片转换为灰度图像[L,N]=bwlabel(two_value,8); % 标注二值图像中连接的部分,L为数据矩阵,N为颗粒的个数for i=1:N,[x,y]=find(bwlabel(two_value)==i); % 寻找矩阵中标号为i的行和列的下标bwsegment = bwselect(two_value,y,x,8); % 选择出第i个颗粒numholes = 1-bweuler(bwsegment,4); % 计算此区域的空洞数if (numholes >= 1) % 若此区域至少包含一个洞,则将其选出进行下一步运算RectCoord = -1;[m n] = size(bwsegment);[cx,cy]=center(bwsegment); % 求此区域的质心bwnohole=bwfill(bwsegment,'holes'); % 将洞封住(将灰度值赋为1)justface = uint8(double(bwnohole) .* double(imsourcegray));% 只在原照片的灰度图像中保留该候选区域angle = orient(bwsegment,cx,cy); % 求此区域的偏转角度bw = imrotate(bwsegment, angle, 'bilinear');bw = bwfill(bw,'holes');[l,r,u,d] =bianjie(bw);wx = (r - l +1); % 宽度ly = (d - u + 1); % 高度wratio = ly/wx % 高宽比if ((0.8<=wratio)&(wratio<=2))% 如果目标区域的高度/宽度比例大于0.8且小于2.0,则将其选出进行下一步运算S=ly*wx; % 计算包含此区域矩形的面积A=bwarea(bwsegment); % 计算此区域面积if (A/S>0.35)[ccorr,mfit, RectCoord] = mobanpipei(justface,frontalmodel,ly,wx, cx,cy, angle);endif (ccorr>=0.6)mfitbw=(mfit>=1);invbw = xor(mfitbw,ones(size(mfitbw)));source_with_hole = uint8(double(invbw) .* double(imsourcegray));final_image = uint8(double(source_with_hole) + double(mfit));subplot(2,3,5);imshow(final_image); % 显示覆盖了模板脸的灰度图像imsourcegray = final_image;subplot(2,3,6);imshow(Ori_Face); % 显示检测效果图end;if (RectCoord ~= -1)FaceCoord = [FaceCoord; RectCoord];endendendend% 在认为是人脸的区域画矩形[numfaces x] = size(FaceCoord);for i=1:numfaces,hd = rectangle('Position',FaceCoord(i,:));set(hd, 'edgecolor', 'y');end。
人脸识别C++源代码
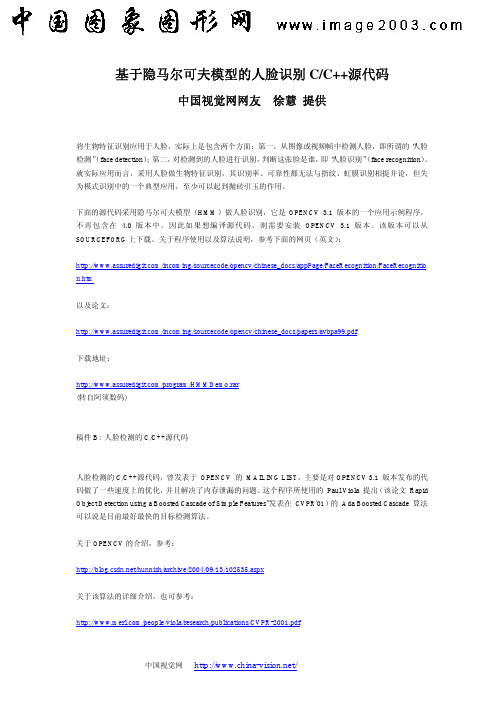
temp = cvCreateImage( cvSize(frame->width/2,frame->height/2), 8, 3 );
if( hid_cascade ) { CvSeq* faces = cvHaarDetectObjects( temp, hid_cascade, storage, 1.2, 2, CV_HAAR_DO_CANNY_PRUNING ); for( i = 0; i < (faces ? faces->total : 0); i++ ) { CvRect* r = (CvRect*)cvGetSeqElem( faces, i, 0 ); pt1.x = r->x*scale; pt2.x = (r->x+r->width)*scale; #ifdef WIN32
cvNamedWindow( WINNAME, 1 ); storage = cvCreateMemStorage(0);
if( argc == 1 || (argc == 2 && strlen(argv[1]) == 1 && isdigit(argv[1][0]))) capture = cvCaptureFromCAM( argc == 2 ? argv[1][0] - '0' : 0 ); else if( argc == 2 ) capture = cvCaptureFromAVI( argv[1] );
pca人脸识别识别率源代码
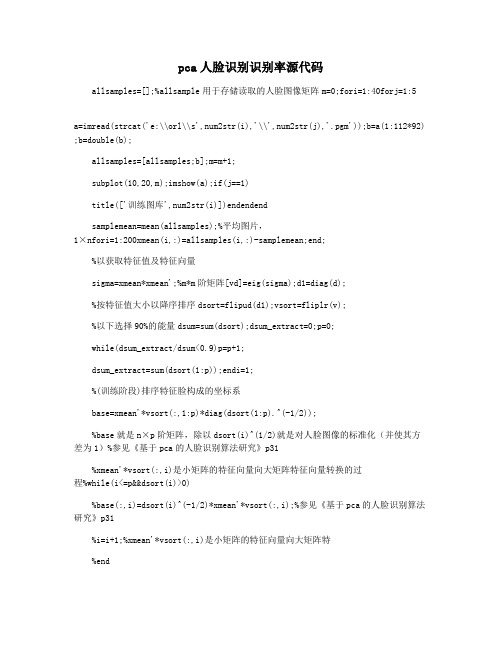
pca人脸识别识别率源代码allsamples=[];%allsample用于存储读取的人脸图像矩阵m=0;fori=1:40forj=1:5a=imread(strcat('e:\\orl\\s',num2str(i),'\\',num2str(j),'.pgm'));b=a(1:112*92) ;b=double(b);allsamples=[allsamples;b];m=m+1;subplot(10,20,m);imshow(a);if(j==1)title(['训练图库',num2str(i)])endendendsamplemean=mean(allsamples);%平均图片,1×nfori=1:200xmean(i,:)=allsamples(i,:)-samplemean;end;%以获取特征值及特征向量sigma=xmean*xmean';%m*m阶矩阵[vd]=eig(sigma);d1=diag(d);%按特征值大小以降序排序dsort=flipud(d1);vsort=fliplr(v);%以下选择90%的能量dsum=sum(dsort);dsum_extract=0;p=0;while(dsum_extract/dsum<0.9)p=p+1;dsum_extract=sum(dsort(1:p));endi=1;%(训练阶段)排序特征脸构成的坐标系base=xmean'*vsort(:,1:p)*diag(dsort(1:p).^(-1/2));%base就是n×p阶矩阵,除以dsort(i)^(1/2)就是对人脸图像的标准化(并使其方差为1)%参见《基于pca的人脸识别算法研究》p31%xmean'*vsort(:,i)是小矩阵的特征向量向大矩阵特征向量转换的过程%while(i<=p&&dsort(i)>0)%base(:,i)=dsort(i)^(-1/2)*xmean'*vsort(:,i);%参见《基于pca的人脸识别算法研究》p31%i=i+1;%xmean'*vsort(:,i)是小矩阵的特征向量向大矩阵特%end%以下两行addbygongxun将训练样本对坐标系上进行投影,得到一个m*p阶矩阵allcoorallcoor=allsamples*base;%allcoor里面是每张训练人脸图片在m*p子空间中的一个点,accu=0;%下面的人脸识别过程中就是利用这些组合系数来进行识别%测试过程fori=1:40forj=6:10%初始化40x5副测试图像a=imread(strcat('e:\\orl\\s',num2str(i),'\\',num2str(j),'.pgm'));b=a(1:10304); b=double(b);tcoor=b*base;%排序座标,就是1×p阶矩阵fork=1:200mdist(k)=norm(tcoor-allcoor(k,:));end;%三阶接邻[dist,index2]=sort(mdist);class1=floor((index2(1)-1)/5)+1;class2=floor((index2(2)-1)/5)+1;class3=floor((index2(3)-1)/5)+1;ifclass1~=class2&&class2~=class3class=class1;elseifclass1==class2class=class1;elseifclass2==class3class=class2;end;ifclass==iaccu=accu+1;end;end;end;accuracy=accu/200%输入识别率。
人脸识别的运行代码
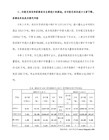
if p>mx && (Bd(k, 3)/Bd(k, 4))<1.8
% 如果满足面积块大,而且宽/高<1.8
mx = p;
j = k;
end
end
subplot(2, 2, 4);imshow(I); hold on;
j=histeq(i);imshow(j);
figure,subplot(1,2,1),imhist(i);
subplot(1,2,2),imhist(j)
i=imread('e:\2.tif');
j=edge(i,'canny',[0.04,0.25],1.5);
imshow(j)
end
y1 = y1+c; % 列跳跃
y2 = y2+c; % 列跳跃
end
x1 = x1+r; % 行跳跃
x2 = x2+r; % 行跳跃
end
[L, num] = bwlabel(BW, 8); % 区域标记
%进行5*5均值滤波
K3=filter2(fspecial('average',7),I)/255;
%进行7*7均值滤波
figure,imshow(K1)
figure,imshow(K2)
figure,imshow(K3)
clc; clear all; close all;
% 载入图像
Img = imread('e:\2.jpg');
if ndims(Img) == 3
I=rgb2gray(Img);
基于matlab的人脸识别源代码
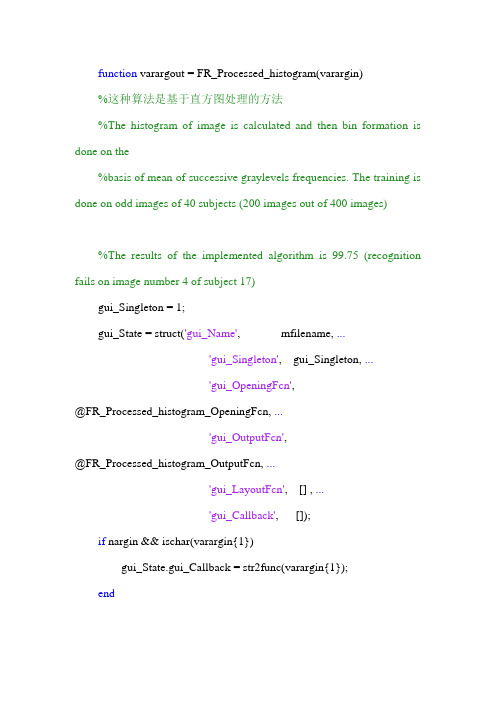
function varargout = FR_Processed_histogram(varargin)%这种算法是基于直方图处理的方法%The histogram of image is calculated and then bin formation is done on the%basis of mean of successive graylevels frequencies. The training is done on odd images of 40 subjects (200 images out of 400 images)%The results of the implemented algorithm is 99.75 (recognition fails on image number 4 of subject 17)gui_Singleton = 1;gui_State = struct('gui_Name', mfilename, ...'gui_Singleton', gui_Singleton, ...'gui_OpeningFcn',@FR_Processed_histogram_OpeningFcn, ...'gui_OutputFcn',@FR_Processed_histogram_OutputFcn, ...'gui_LayoutFcn', [] , ...'gui_Callback', []);if nargin && ischar(varargin{1})gui_State.gui_Callback = str2func(varargin{1});endif nargout[varargout{1:nargout}] = gui_mainfcn(gui_State, varargin{:});elsegui_mainfcn(gui_State, varargin{:});end% End initialization code - DO NOT EDIT%--------------------------------------------------------------------------% --- Executes just before FR_Processed_histogram is made visible.function FR_Processed_histogram_OpeningFcn(hObject, eventdata, handles, varargin)% This function has no output args, see OutputFcn.% hObject handle to figure% eventdata reserved - to be defined in a future version of MATLAB% handles structure with handles and user data (see GUIDATA) % varargin command line arguments to FR_Processed_histogram (see VARARGIN)% Choose default command line output for FR_Processed_histogramhandles.output = hObject;% Update handles structureguidata(hObject, handles);% UIWAIT makes FR_Processed_histogram wait for user response (see UIRESUME)% uiwait(handles.figure1);global total_sub train_img sub_img max_hist_level bin_num form_bin_num;total_sub = 40;train_img = 200;sub_img = 10;max_hist_level = 256;bin_num = 9;form_bin_num = 29;%--------------------------------------------------------------------------% --- Outputs from this function are returned to the command line.function varargout = FR_Processed_histogram_OutputFcn(hObject, eventdata, handles)% varargout cell array for returning output args (see VARARGOUT);% hObject handle to figure% eventdata reserved - to be defined in a future version of MATLAB% handles structure with handles and user data (see GUIDATA)% Get default command line output from handles structurevarargout{1} = handles.output;%--------------------------------------------------------------------------% --- Executes on button press in train_button.function train_button_Callback(hObject, eventdata, handles)% hObject handle to train_button (see GCBO)% eventdata reserved - to be defined in a future version of MATLAB% handles structure with handles and user data (see GUIDATA)global train_processed_bin;global total_sub train_img sub_img max_hist_level bin_num form_bin_num;train_processed_bin(form_bin_num,train_img) = 0;K = 1;train_hist_img = zeros(max_hist_level, train_img);for Z=1:1:total_subfor X=1:2:sub_img %%%train on odd number of images of each subjectI = imread( strcat('ORL\S',int2str(Z),'\',int2str(X),'.bmp') );[rows cols] = size(I);for i=1:1:rowsfor j=1:1:colsif( I(i,j) == 0 )train_hist_img(max_hist_level, K) = train_hist_img(max_hist_level, K) + 1;elsetrain_hist_img(I(i,j), K) = train_hist_img(I(i,j), K) + 1;endendendK = K + 1;endend[r c] = size(train_hist_img);sum = 0;for i=1:1:cK = 1;for j=1:1:rif( (mod(j,bin_num)) == 0 )sum = sum + train_hist_img(j,i);train_processed_bin(K,i) = sum/bin_num;K = K + 1;sum = 0;elsesum = sum + train_hist_img(j,i);endendtrain_processed_bin(K,i) = sum/bin_num;enddisplay ('Training Done')save 'train'train_processed_bin;%--------------------------------------------------------------------------% --- Executes on button press in Testing_button.function Testing_button_Callback(hObject, eventdata, handles)% hObject handle to Testing_button (see GCBO)% eventdata reserved - to be defined in a future version of MATLAB% handles structure with handles and user data (see GUIDATA) global train_img max_hist_level bin_num form_bin_num;global train_processed_bin;global filename pathname Iload 'train'test_hist_img(max_hist_level) = 0;test_processed_bin(form_bin_num) = 0;[rows cols] = size(I);for i=1:1:rowsfor j=1:1:colsif( I(i,j) == 0 )test_hist_img(max_hist_level) =test_hist_img(max_hist_level) + 1;elsetest_hist_img(I(i,j)) = test_hist_img(I(i,j)) + 1;endendend[r c] = size(test_hist_img);sum = 0;K = 1;for j=1:1:cif( (mod(j,bin_num)) == 0 )sum = sum + test_hist_img(j);test_processed_bin(K) = sum/bin_num;K = K + 1;sum = 0;elsesum = sum + test_hist_img(j);endendtest_processed_bin(K) = sum/bin_num;sum = 0;K = 1;for y=1:1:train_imgfor z=1:1:form_bin_numsum = sum + abs( test_processed_bin(z) - train_processed_bin(z,y) );endimg_bin_hist_sum(K,1) = sum;sum = 0;K = K + 1;end[temp M] = min(img_bin_hist_sum);M = ceil(M/5);getString_start=strfind(pathname,'S');getString_start=getString_start(end)+1;getString_end=strfind(pathname,'\');getString_end=getString_end(end)-1;subjectindex=str2num(pathname(getString_start:getString_end));if (subjectindex == M)axes (handles.axes3)%image no: 5 is shown for visualization purposeimshow(imread(STRCAT('ORL\S',num2str(M),'\5.bmp')))msgbox ( 'Correctly Recognized');elsedisplay ([ 'Error==> Testing Image of Subject >>' num2str(subjectindex) ' matches with the image of subject >> ' num2str(M)])axes (handles.axes3)%image no: 5 is shown for visualization purposeimshow(imread(STRCAT('ORL\S',num2str(M),'\5.bmp')))msgbox ( 'Incorrectly Recognized');enddisplay('Testing Done')%--------------------------------------------------------------------------function box_Callback(hObject, eventdata, handles)% hObject handle to box (see GCBO)% eventdata reserved - to be defined in a future version ofMATLAB% handles structure with handles and user data (see GUIDATA)% Hints: get(hObject,'String') returns contents of box as text% str2double(get(hObject,'String')) returns contents of box as a double%--------------------------------------------------------------------------% --- Executes during object creation, after setting all properties.function box_CreateFcn(hObject, eventdata, handles)% hObject handle to box (see GCBO)% eventdata reserved - to be defined in a future version of MATLAB% handles empty - handles not created until after all CreateFcns called% Hint: edit controls usually have a white background on Windows.% See ISPC and COMPUTER.if ispc && isequal(get(hObject,'BackgroundColor'), get(0,'defaultUicontrolBackgroundColor'))set(hObject,'BackgroundColor','white');end%--------------------------------------------------------------------------% --- Executes on button press in Input_Image_button.function Input_Image_button_Callback(hObject, eventdata, handles) % hObject handle to Input_Image_button (see GCBO)% eventdata reserved - to be defined in a future version of MATLAB% handles structure with handles and user data (see GUIDATA) global filename pathname I[filename, pathname] = uigetfile('*.bmp', 'Test Image');axes(handles.axes1)imgpath=STRCAT(pathname,filename);I = imread(imgpath);imshow(I)%--------------------------------------------------------------------------% --- Executes during object creation, after setting all properties.function axes3_CreateFcn(hObject, eventdata, handles)% hObject handle to axes3 (see GCBO)% eventdata reserved - to be defined in a future version of MATLAB% handles empty - handles not created until after all CreateFcnscalled% Hint: place code in OpeningFcn to populate axes3 %Programmed by Usman Qayyum。
人脸识别可能用到的代码

人脸识别可能用到的代码:1.色彩空间转换function [r,g]=rgb_RGB(Ori_Face)R=Ori_Face(:,:,1);G=Ori_Face(:,:,2);B=Ori_Face(:,:,3);R1=im2double(R); % 将uint8型转换成double型G1=im2double(G);B1=im2double(B);RGB=R1+G1+B1;row=size(Ori_Face,1); % 行像素column=size(Ori_Face,2); % 列像素for i=1:rowfor j=1:columnrr(i,j)=R1(i,j)/RGB(i,j);gg(i,j)=G1(i,j)/RGB(i,j);endendrrr=mean(rr);r=mean(rrr);ggg=mean(gg);g=mean(ggg);2.均值和协方差t1=imread('D:\matlab\皮肤库\1.jpg');[r1,g1]=rgb_RGB(t1); t2=imread('D:\matlab\皮肤库\2.jpg');[r2,g2]=rgb_RGB(t2); t3=imread('D:\matlab\皮肤库\3.jpg');[r3,g3]=rgb_RGB(t3); t4=imread('D:\matlab\皮肤库\4.jpg');[r4,g4]=rgb_RGB(t4); t5=imread('D:\matlab\皮肤库\5.jpg');[r5,g5]=rgb_RGB(t5); t6=imread('D:\matlab\皮肤库\6.jpg');[r6,g6]=rgb_RGB(t6);t7=imread('D:\matlab\皮肤库\7.jpg');[r7,g7]=rgb_RGB(t7);t8=imread('D:\matlab\皮肤库\8.jpg');[r8,g8]=rgb_RGB(t8);t9=imread('D:\matlab\皮肤库\9.jpg');[r9,g9]=rgb_RGB(t9);t10=imread('D:\matlab\皮肤库\10.jpg');[r10,g10]=rgb_RGB(t10);t11=imread('D:\matlab\皮肤库\11.jpg');[r11,g11]=rgb_RGB(t11);t12=imread('D:\matlab\皮肤库\12.jpg');[r12,g12]=rgb_RGB(t12);t13=imread('D:\matlab\皮肤库\13.jpg');[r13,g13]=rgb_RGB(t13);t14=imread('D:\matlab\皮肤库\14.jpg');[r14,g14]=rgb_RGB(t14);t15=imread('D:\matlab\皮肤库\15.jpg');[r15,g15]=rgb_RGB(t15);t16=imread('D:\matlab\皮肤库\16.jpg');[r16,g16]=rgb_RGB(t16);t17=imread('D:\matlab\皮肤库\17.jpg');[r17,g17]=rgb_RGB(t17);t18=imread('D:\matlab\皮肤库\18.jpg');[r18,g18]=rgb_RGB(t18);t19=imread('D:\matlab\皮肤库\19.jpg');[r19,g19]=rgb_RGB(t19);t20=imread('D:\matlab\皮肤库\20.jpg');[r20,g20]=rgb_RGB(t20);t21=imread('D:\matlab\皮肤库\21.jpg');[r21,g21]=rgb_RGB(t21);t22=imread('D:\matlab\皮肤库\22.jpg');[r22,g22]=rgb_RGB(t22);t23=imread('D:\matlab\皮肤库\23.jpg');[r23,g23]=rgb_RGB(t23);t24=imread('D:\matlab\皮肤库\24.jpg');[r24,g24]=rgb_RGB(t24);t25=imread('D:\matlab\皮肤库\25.jpg');[r25,g25]=rgb_RGB(t25);t26=imread('D:\matlab\皮肤库\26.jpg');[r26,g26]=rgb_RGB(t26);t27=imread('D:\matlab\皮肤库\27.jpg');[r27,g27]=rgb_RGB(t27);r=cat(1,r1,r2,r3,r4,r5,r6,r7,r8,r9,r10,r11,r12,r13,r14,r15,r16,r17,r18,r19,r20,r21,r22,r23,r24,r25,r 26,r27);g=cat(1,g1,g2,g3,g4,g5,g6,g7,g8,g9,g10,g11,g12,g13,g14,g15,g16,g17,g18,g19,g20,g21,g22,g2 3,g24,g25,g26,g27);m=mean([r,g])n=cov([r,g])3.求质心function [xmean, ymean] = center(bw)bw=bwfill(bw,'holes');area = bwarea(bw);[m n] =size(bw);bw=double(bw);xmean =0; ymean = 0;for i=1:m,for j=1:n,xmean = xmean + j*bw(i,j);ymean = ymean + i*bw(i,j);end;end;if(area==0)xmean=0;ymean=0;elsexmean = xmean/area;ymean = ymean/area;xmean = round(xmean);ymean = round(ymean);end4. 求偏转角度function [theta] = orient(bw,xmean,ymean) [m n] =size(bw);bw=double(bw);a = 0;b = 0;c = 0;for i=1:m,for j=1:n,a = a + (j - xmean)^2 * bw(i,j);b = b + (j - xmean) * (i - ymean) * bw(i,j);c = c + (i - ymean)^2 * bw(i,j);end;b = 2 * b;theta = atan(b/(a-c))/2;theta = theta*(180/pi); % 从幅度转换到角度5. 找区域边界function [left, right, up, down] = bianjie(A) [m n] = size(A);left = -1;right = -1;up = -1;down = -1;for j=1:n,for i=1:m,if (A(i,j) ~= 0)left = j;break;end;end;if (left ~= -1) break;end;end;for j=n:-1:1,for i=1:m,if (A(i,j) ~= 0)right = j;break;end;end;if (right ~= -1) break;end;for i=1:m,for j=1:n,if (A(i,j) ~= 0)up = i;break;end;end;if (up ~= -1)break;end;end;for i=m:-1:1,for j=1:n,if (A(i,j) ~= 0)down = i;break;end;end;if (down ~= -1)break;end;end;6. 求起始坐标function newcoord = checklimit(coord,maxval) newcoord = coord;if (newcoord<1)newcoord=1;end;if (newcoord>maxval)newcoord=maxval;7.模板匹配function [ccorr, mfit, RectCoord] = mobanpipei(mult, frontalmodel,ly,wx,cx, cy, angle) frontalmodel=rgb2gray(frontalmodel);model_rot = imresize(frontalmodel,[ly wx],'bilinear'); % 调整模板大小model_rot = imrotate(model_rot,angle,'bilinear'); % 旋转模板[l,r,u,d] = bianjie(model_rot); % 求边界坐标bwmodel_rot=imcrop(model_rot,[l u (r-l) (d-u)]); % 选择模板人脸区域[modx,mody] =center(bwmodel_rot); % 求质心[morig, norig] = size(bwmodel_rot);% 产生一个覆盖了人脸模板的灰度图像mfit = zeros(size(mult));mfitbw = zeros(size(mult));[limy, limx] = size(mfit);% 计算原图像中人脸模板的坐标startx = cx-modx;starty = cy-mody;endx = startx + norig-1;endy = starty + morig-1;startx = checklimit(startx,limx);starty = checklimit(starty,limy);endx = checklimit(endx,limx);endy = checklimit(endy,limy);for i=starty:endy,for j=startx:endx,mfit(i,j) = model_rot(i-starty+1,j-startx+1);end;end;ccorr = corr2(mfit,mult) % 计算相关度[l,r,u,d] = bianjie(bwmodel_rot);sx = startx+l;sy = starty+u;RectCoord = [sx sy (r-1) (d-u)]; % 产生矩形坐标8.主程序clear;[fname,pname]=uigetfile({'*.jpg';'*.bmp';'*.tif';'*.gif'},'Please choose a color picture...');% 返回打开的图片名与图片路径名[u,v]=size(fname);y=fname(v); % 图片格式代表值switch ycase 0errordlg('You Should Load Image File First...','Warning...');case{'g';'G';'p';'P';'f';'F'}; % 图片格式若是JPG/jpg、BMP/bmp、TIF/tif或者GIF/gif,才打开I=cat(2,pname,fname);Ori_Face=imread(I);subplot(2,3,1),imshow(Ori_Face);otherwiseerrordlg('You Should Load Image File First...','Warning...');endR=Ori_Face(:,:,1);G=Ori_Face(:,:,2);B=Ori_Face(:,:,3);R1=im2double(R); % 将uint8型转换成double型处理G1=im2double(G);B1=im2double(B);RGB=R1+G1+B1;m=[ 0.4144,0.3174]; % 均值n=[0.0031,-0.0004;-0.0004,0.0003]; % 方差row=size(Ori_Face,1); % 行像素数column=size(Ori_Face,2); % 列像素数for i=1:rowfor j=1:columnif RGB(i,j)==0rr(i,j)=0;gg(i,j)=0;elserr(i,j)=R1(i,j)/RGB(i,j); % rgb归一化gg(i,j)=G1(i,j)/RGB(i,j);x=[rr(i,j),gg(i,j)];p(i,j)=exp((-0.5)*(x-m)*inv(n)*(x-m)'); % 皮肤概率服从高斯分布 endendendsubplot(2,3,2);imshow(p); % 显示皮肤灰度图像low_pass=1/9*ones(3);image_low=filter2(low_pass, p); % 低通滤波去噪声subplot(2,3,3);imshow(image_low);% 自适应阀值程序previousSkin2 = zeros(i,j);changelist = [];for threshold = 0.55:-0.1:0.05two_value = zeros(i,j);two_value(find(image_low>threshold)) = 1;change = sum(sum(two_value - previousSkin2));changelist = [changelist change];previousSkin2 = two_value;end[C, I] = min(changelist);optimalThreshold = (7-I)*0.1two_value = zeros(i,j);two_value(find(image_low>optimalThreshold)) = 1; % 二值化subplot(2,3,4);imshow(two_value); % 显示二值图像frontalmodel=imread('E:\我的照片\人脸模板.jpg'); % 读入人脸模板照片FaceCoord=[];imsourcegray=rgb2gray(Ori_Face); % 将原照片转换为灰度图像[L,N]=bwlabel(two_value,8); % 标注二值图像中连接的部分,L为数据矩阵,N为颗粒的个数for i=1:N,[x,y]=find(bwlabel(two_value)==i); % 寻找矩阵中标号为i的行和列的下标bwsegment = bwselect(two_value,y,x,8); % 选择出第i个颗粒numholes = 1-bweuler(bwsegment,4); % 计算此区域的空洞数if (numholes >= 1) % 若此区域至少包含一个洞,则将其选出进行下一步运算RectCoord = -1;[m n] = size(bwsegment);[cx,cy]=center(bwsegment); % 求此区域的质心bwnohole=bwfill(bwsegment,'holes'); % 将洞封住(将灰度值赋为1)justface = uint8(double(bwnohole) .* double(imsourcegray));% 只在原照片的灰度图像中保留该候选区域angle = orient(bwsegment,cx,cy); % 求此区域的偏转角度bw = imrotate(bwsegment, angle, 'bilinear');bw = bwfill(bw,'holes');[l,r,u,d] =bianjie(bw);wx = (r - l +1); % 宽度ly = (d - u + 1); % 高度wratio = ly/wx % 高宽比if ((0.8<=wratio)&(wratio<=2))% 如果目标区域的高度/宽度比例大于0.8且小于2.0,则将其选出进行下一步运算 S=ly*wx; % 计算包含此区域矩形的面积A=bwarea(bwsegment); % 计算此区域面积if (A/S>0.35)[ccorr,mfit, RectCoord] = mobanpipei(justface,frontalmodel,ly,wx, cx,cy, angle);endif (ccorr>=0.6)mfitbw=(mfit>=1);invbw = xor(mfitbw,ones(size(mfitbw)));source_with_hole = uint8(double(invbw) .* double(imsourcegray));final_image = uint8(double(source_with_hole) + double(mfit));subplot(2,3,5);imshow(final_image); % 显示覆盖了模板脸的灰度图像imsourcegray = final_image;subplot(2,3,6);imshow(Ori_Face); % 显示检测效果图end;if (RectCoord ~= -1)FaceCoord = [FaceCoord; RectCoord];endendendend% 在认为是人脸的区域画矩形[numfaces x] = size(FaceCoord);for i=1:numfaces,hd = rectangle('Position',FaceCoord(i,:));set(hd, 'edgecolor', 'y');end部分检测原理一、基于肤色模型的人脸检测对于一个成功的人脸识别系统,人脸检测是极其重要和关键的一步,它直接影响人脸特征的提取,识别等后续工作。
人脸识别技术应用源码

人脸识别技术应用源码人脸识别技术是一种先进的生物特征识别技术,已经广泛应用于安全监控、人脸支付、门禁系统等领域。
以下是一份人脸识别技术应用的源码示例,供参考:import cv2def detect_face(image_path):加载人脸识别模型face_cascade =cv2.CascadeClassifier('haarcascade_frontalface_default.xml')读取图像image = cv2.imread(image_path)将图像转换为灰度图gray = cv2.cvtColor(image, cv2.COLOR_BGR2GRAY)检测人脸faces = face____(gray, scaleFactor=1.1, minNeighbors=5)标记检测到的人脸for (x, y, w, h) in faces:cv2.rectangle(image, (x, y), (x+w, y+h), (0, 255, 0), 2)显示标记后的图像cv2.imshow("Faces", image)___(0)cv2.destroyAllWindows()测试代码image_path = "test.jpg"detect_face(image_path)以上源码使用OpenCV库实现了一个简单的人脸识别功能。
通过加载人脸识别模型,读取图像并将其转换为灰度图,然后使用级联分类器检测人脸,并在图像上标记检测到的人脸。
最后,将标记后的图像显示出来。
请注意,以上示例仅为人脸识别技术应用的简单示例。
在实际应用中,可能需要更复杂的算法和模型来实现更准确和稳定的人脸识别。
希望以上信息对您有所帮助!如有更多问题,请随时提问。
人脸识别源码分享

人脸识别源码分享
一、人脸识别技术综述
人脸识别(Face Recognition)技术是指通过分析摄像机或图像中捕捉到的人脸图像,利用一定的方法和技术,来识别出其中一个特定的人脸和身份,确认未知身份的一种计算机视觉技术。
它所采用的基本技术包括图像分析、图像检索、模式识别等,它使用了一系列的算法来检测和识别人类脸部的特征,进而实现人脸的辨别和认证。
二、人脸识别源码实现
1、基础环境及框架
本程序基于Python 3.6版本,使用OpenCV 3.4.1 运行识别图像,使用Haar特征级联检测人脸。
编程环境为:
Windows10,Pycharm 2024.2.3
2、实现步骤
(1)载入数据集,获取每个人脸的名字及其对应的照片,将其储存为字典格式,以便后续识别。
(2)定义识别程序:调用OpenCV检测函数,用于检测想要识别的图像中的人脸位置,传入Haar特征级联检测器,传出下一步需要的X、Y轴坐标。
(3)提取特征:提取(x,y)轴坐标,定义提取脸部特征的函数,将每个人脸的照片存入列表中,然后提取特征并放入列表中。
(4)比较特征:设置一个距离阈值threshold,将提取的特征列表中的每个特征用Euclidean Distance算法比较,传入距离阈值,返回一个布尔值,如果距离大于threshold,标记为false。
人脸识别MATLAB代码doc文档_IT168文库
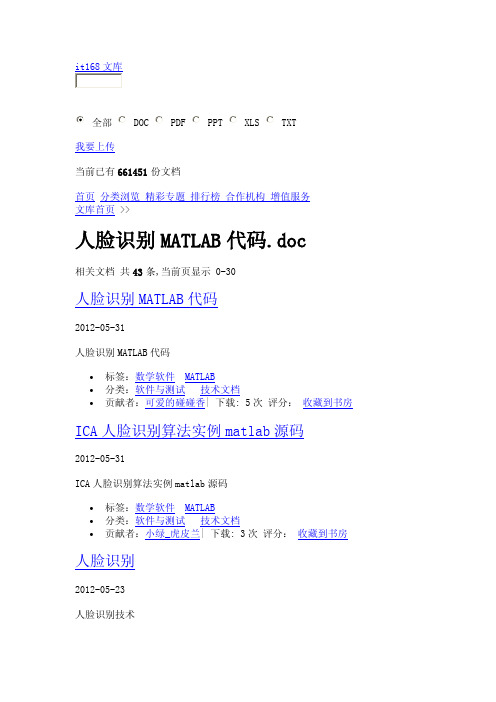
全部DOC PDF PPT XLS TXT我要上传当前已有661451份文档首页分类浏览精彩专题排行榜合作机构增值服务文库首页 >>人脸识别MATLAB代码.doc相关文档共43条,当前页显示 0-30人脸识别MATLAB代码2012-05-31人脸识别MATLAB代码∙标签:数学软件MATLAB∙分类:软件与测试技术文档∙贡献者:可爱的碰碰香| 下载: 5次评分:收藏到书房ICA人脸识别算法实例matlab源码2012-05-31ICA人脸识别算法实例matlab源码∙标签:数学软件MATLAB∙分类:软件与测试技术文档∙贡献者:小绿_虎皮兰| 下载: 3次评分:收藏到书房人脸识别2012-05-23人脸识别技术∙标签:其他信息化网络通信∙分类:网络通信课程资源∙贡献者:晓玩子LXQ| 下载: 2次评分:收藏到书房人脸识别技术初探2012-03-30随着图形图象识别算法的革命性改进和计算机处理速度的飞速提高,"人脸识别"技术脱颖而出。
他以其独特的方便、经济、准确而受到世人的瞩目。
∙标签:视频分析安防监控∙分类:安防监控∙贡献者:yueve| 下载: 1次评分:收藏到书房VC人脸识别技术论文2012-02-15人脸识别技术可以应用于基于网络的身份认证,我们实现了基于WEBCAM的人脸识别与跟踪系统。
本文以WEBCAM采集的视频流为数据源,截取视频流中的单帧图像,通过转换彩色空间、人脸肤色建模、后处理操作和人脸定位算法实现了人脸检测,并以此为基础实现了在视频流中对于人脸的跟踪。
∙标签:毕业论文VC毕业设计∙分类:开发-C/C++调研报告∙贡献者:哈哈abc123| 下载: 4次评分:收藏到书房人脸识别技术基本定义2012-03-30人脸识别技术((Face Recognition)是一种依据人的面部特征(如统计或几何特征等),自动进行身份鉴别蝗一种技术,它综合运用了数字图像/视频处理、模式识别等多种技术。
人脸识别程序源代码
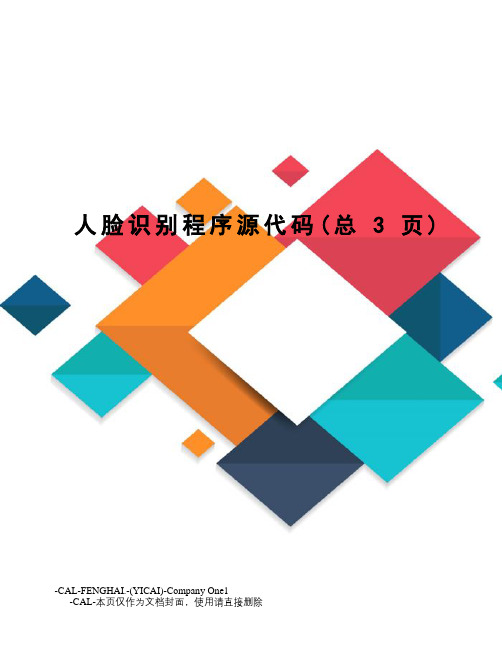
人脸识别程序源代码(总3页) -CAL-FENGHAI.-(YICAI)-Company One1-CAL-本页仅作为文档封面,使用请直接删除1.利用OpenCV进行人脸检测人脸检测程序主要完成3部分功能,即加载分类器、加载待检测图象以及检测并标示。
本程序使用OpenCV中提供的“haarcascade_frontalface_alt.xml”文件存储的目标检测分类,用cv Load函数载入后,进行强制类型转换。
OpenCV中提供的用于检测图像中目标的函数是cvHaarDet ectObjects,该函数使用指针对某目标物体(如人脸)训练的级联分类器在图象中找到包含目标物体的矩形区域,并将这些区域作为一序列的矩形框返回。
分类器在使用后需要被显式释放,所用的函数为cvReleaseHaarClassifierCascade。
这些函数原型请参看有关OpenCV手册。
2.程序实现1)新建一个Visual C++ MFC项目,取名为“FaceDetection”,选择应用程序类型为“单文档”。
将菜单中多余的项去掉,并添加一项“人脸检测”,其ID为“ID_FaceDetected”,并生成该菜单项的消息映射函数。
2)在“FaceDetectionView.h”头文件中添加以下灰底色部分程序代码:3)在“FaceDetectionView.cpp”文件中添加以下灰底色部分程序代码:需要注意的是,本程序运行时应将分类器文件置于程序目录下,如果运行的是生成的EXE文件,则应将分类器文件与该EXE文件放在同一个目录下。
三、程序运行结果运行该程序,选择人脸检测菜单项,弹出文件打开对话框,选择要检测的图像文件,程序就会将检测到的人脸用圆圈标示出来,如图3所示。
本程序能顺利检测出大部分人脸,但由于光照、遮挡和倾斜等原因,部分人脸不能正确检测,另外,也有一些非人脸部分由于具有人脸的某些特征,也被当成了人脸,这些都是本程序需要改进的部分。
人脸识别源码分享

计划实现了一个基于PCA的人脸识别方法,我称之为“特征点方法”,所有的功能简单而且实用。
下面,我使用一个简单的MATLAB脚本说明它的用法。
一般情况,你应该按照以下这个顺序执行这个方法:1. 选择实际测试和参照组人脸图像数据库的路径;2. 选择实际测试人脸图像的路径;3. 运行“CreateDatabase”函数来创建所有参照组人脸图像的二维矩阵;4. 运行“eigenfacecore"函数产生基础人脸图像空间;5. 运行“识别”功能得到参照组人脸图像数据库中等价图像的名称。
为了您的方便,我准备了实际测试和参照组人脸图像数据库,其中部分来自“Face94”埃塞克斯人脸数据库。
你只需要复制上述功能,指定实际测试和参照组人脸图像数据库的路径(比如Matlab工作路径)。
然后按照对话框提示输入图片编号,实例将运行实现。
希望您能喜欢它!引用:[1] P. N. Belhumeur, J. Hespanha, and D. J. Kriegman. Eigenfaces vs. Fisherfaces: Recognitionusing class specific linear projection. In ECCV (1), pages 45--58, 1996.[2] Available at:以下为源代码文件:----------------------------------------------------------------------------------------------------------------------CreateDatabase.mfunction T = CreateDatabase(TrainDatabasePath)% Align a set of face images (the training set T1, T2, ... , TM )%% Description: This function reshapes all 2D images of the training database % into 1D column vectors. Then, it puts these 1D column vectors in a row to % construct 2D matrix 'T'.%% Argument: TrainDatabasePath - Path of the training database%% Returns: T - A 2D matrix, containing all 1D image vectors.% Suppose all P images in the training database% have the same size of MxN. So the length of 1D% column vectors is MN and 'T' will be a MNxP 2D matrix.%% See also: STRCMP, STRCAT, RESHAPE% Original version by Amir Hossein Omidvarnia, October 2007% Email:%%%%%%%%%%%%%%%%%%%%%%%%TrainFiles = dir(TrainDatabasePath);Train_Number = 0;for i = 1:size(Train)ifnot(strcmp(TrainFiles(i).name,'.')|strcmp(TrainFiles(i).name,'..')|strcmp(TrainFil es(i).name,'Thumbs.db'))Train_Number = Train_Number + 1; % Number of all images in the training databaseendend%%%%%%%%%%%%%%%%%%%%%%%% Construction of 2D matrixfrom 1D image vectorsT = [];for i = 1 : Train_Number% I have chosen the name of each image in databases as a corresponding % number. However, it is not mandatory!str = int2str(i);str = strcat('\',str,'.jpg');str = strcat(TrainDatabasePath,str);img = imread(str);img = rgb2gray(img);[irow icol] = size(img);temp = reshape(img',irow*icol,1); % Reshaping 2D images into 1D image vectorsT = [T temp]; % 'T' grows after each turnend---------------------------------------------------------------------------------------------------------------------------EigenfaceCore.mfunction [m, A, Eigenfaces] = EigenfaceCore(T)% Use Principle Component Analysis (PCA) to determine the most% discriminating features between images of faces.%% Description: This function gets a 2D matrix, containing all training image vectors% and returns 3 outputs which are extracted from training database.%% Argument: T - A 2D matrix, containing all 1D image vectors.% Suppose all P images in the training database% have the same size of MxN. So the length of 1D% column vectors is M*N and 'T' will be a MNxP 2D matrix.%% Returns: m - (M*Nx1) Mean of the training database% Eigenfaces - (M*Nx(P-1)) Eigen vectors of thecovariance matrix of the training database% A - (M*NxP) Matrix of centered image vectors%% See also: EIG% Original version by Amir Hossein Omidvarnia, October 2007% Email:%%%%%%%%%%%%%%%%%%%%%%%% Calculating the mean image m = mean(T,2); % Computing the average face image m = (1/P)*sum(Tj's) (j = 1 : P)Train_Number = size(T,2);%%%%%%%%%%%%%%%%%%%%%%%% Calculating the deviation of each image from mean imageA = [];for i = 1 : Train_Numbertemp = double(T(:,i)) - m; % Computing the difference image for each image in the training set Ai = Ti - mA = [A temp]; % Merging all centered imagesend%%%%%%%%%%%%%%%%%%%%%%%% Snapshot method of Eigenface methos% We know from linear algebra theory that for a PxQ matrix, the maximum% number of non-zero eigenvalues that the matrix can have is min(P-1,Q-1). % Since the number of training images (P) is usually less than the number% of pixels (M*N), the most non-zero eigenvalues that can be found are equal % to P-1. So we can calculate eigenvalues of A'*A (a PxP matrix) instead of % A*A' (a M*NxM*N matrix). It is clear that the dimensions of A*A' is much% larger that A'*A. So the dimensionality will decrease.L = A'*A; % L is the surrogate of covariance matrix C=A*A'.[V D] = eig(L); % Diagonal elements of D are the eigenvalues for both L=A'*A and C=A*A'.%%%%%%%%%%%%%%%%%%%%%%%% Sorting and eliminating eigenvalues% All eigenvalues of matrix L are sorted and those who are less than a% specified threshold, are eliminated. So the number of non-zero% eigenvectors may be less than (P-1).L_eig_vec = [];for i = 1 : size(V,2)if( D(i,i)>1 )L_eig_vec = [L_eig_vec V(:,i)];endend%%%%%%%%%%%%%%%%%%%%%%%% Calculating the eigenvectors of covariance matrix 'C'% Eigenvectors of covariance matrix C (or so-called "Eigenfaces")% can be recovered from L's eiegnvectors.Eigenfaces = A * L_eig_vec; % A: centered image vectors----------------------------------------------------------------------------------------------------------------------Recognition.mfunction OutputName = Recognition(TestImage, m, A, Eigenfaces)% Recognizing step....%% Description: This function compares two faces by projecting the images into facespace and% measuring the Euclidean distance between them.%% Argument: TestImage - Path of the input test image%% m - (M*Nx1) Mean of the training% database, which is output of'EigenfaceCore' function.%% Eigenfaces - (M*Nx(P-1)) Eigen vectors of the% covariance matrix of the training% database, which is output of'EigenfaceCore' function.%% A - (M*NxP) Matrix of centered image% vectors, which is output of'EigenfaceCore' function.%% Returns: OutputName - Name of the recognized image in the training database.%% See also: RESHAPE, STRCAT% Original version by Amir Hossein Omidvarnia, October 2007% Email:%%%%%%%%%%%%%%%%%%%%%%%% Projecting centered image vectors into facespace% All centered images are projected into facespace by multiplying in% Eigenface basis's. Projected vector of each face will be its corresponding % feature vector.ProjectedImages = [];Train_Number = size(Eigenfaces,2);for i = 1 : Train_Numbertemp = Eigenfaces'*A(:,i); % Projection of centered images into facespace ProjectedImages = [ProjectedImages temp];end%%%%%%%%%%%%%%%%%%%%%%%% Extracting the PCA features from test imageInputImage = imread(TestImage);temp = InputImage(:,:,1);[irow icol] = size(temp);InImage = reshape(temp',irow*icol,1);Difference = double(InImage)-m; % Centered test image ProjectedTestImage = Eigenfaces'*Difference; % Test image feature vector%%%%%%%%%%%%%%%%%%%%%%%% Calculating Euclidean distances% Euclidean distances between the projected test image and the projection % of all centered training images are calculated. Test image is% supposed to have minimum distance with its corresponding image in the % training database.Euc_dist = [];for i = 1 : Train_Numberq = ProjectedImages(:,i);temp = ( norm( ProjectedTestImage - q ) )^2;Euc_dist = [Euc_dist temp];end[Euc_dist_min , Recognized_index] = min(Euc_dist);OutputName = strcat(int2str(Recognized_index),'.jpg');-----------------------------------------------------------------------------------------------------------example.m% A sample script, which shows the usage of functions, included in% PCA-based face recognition system (Eigenface method)%% See also: CREATEDATABASE, EIGENFACECORE, RECOGNITION% Original version by Amir Hossein Omidvarnia, October 2007% Email:clear allclcclose all% You can customize and fix initial directory pathsTrainDatabasePath = uigetdir('D:\MATLAB701\work','D:\MATLAB701\work\TrainDatabase' );TestDatabasePath = uigetdir('D:\MATLAB701\work','D:\MATLAB701\work\TestDatabase');prompt = {'1:'};dlg_title = 'Input of PCA-Based Face Recognition System';num_lines= 1;def = {'1'};TestImage = inputdlg(prompt,dlg_title,num_lines,def); TestImage = strcat(TestDatabasePath,'\',char(TestImage),'.jpg'); im = imread(TestImage);T = CreateDatabase(TrainDatabasePath);[m, A, Eigenfaces] = EigenfaceCore(T);OutputName = Recognition(TestImage, m, A, Eigenfaces);SelectedImage = strcat(TrainDatabasePath,'\',OutputName); SelectedImage = imread(SelectedImage);imshow(im)title('Test Image');figure,imshow(SelectedImage);title('Equivalent Image');str = strcat('Matched image is : ',OutputName);disp(str)源代码来自Matlab源码:。
- 1、下载文档前请自行甄别文档内容的完整性,平台不提供额外的编辑、内容补充、找答案等附加服务。
- 2、"仅部分预览"的文档,不可在线预览部分如存在完整性等问题,可反馈申请退款(可完整预览的文档不适用该条件!)。
- 3、如文档侵犯您的权益,请联系客服反馈,我们会尽快为您处理(人工客服工作时间:9:00-18:30)。
人脸识别源代码※人脸检测(文章+程序)---技术文档及代码非常全『人脸检测(文章+程序).rar(1.27 MB)』※完整的Matlab下人脸检测及识别系统源代码『Face-Recognition-Detection.rar (393.19 KB)』注:这个人脸检测和识别系统开发于Matlab 7.0.1下,非常值得学习。
※Matlab实现的基于颜色分隔的人脸人眼检测与定位及识别算法源代码『Face-Eye-Detection.part1.rar (1.91 MB)Face-Eye-Detection.part2.rar (152.54 KB)』注:这是一个matlab程序,用来检测并定位人脸及人眼。
采用的算法是肤色的颜色分隔。
附件中的文件包括 eyematch.m, eyematch2.m, face.m, findeye.m,skin.m, k001.JPG等等。
※完整的包括及动作识别的C++人脸检测源代码『FaceDetection.rar (875.84 KB)』本文的目的是提供一个我开发的SSE优化的,C++库,用于人脸检测,你可以马上把它用于你的视频监控系统中。
涉及的技术有:小波分析,尺度缩减模型(PCA,LDA,ICA),人工神经网络(ANN),支持向量机(SVM),SSE编程,图像处理,直方图均衡,图像滤波,C++编程,还有一下其它的人脸检测的背景知识。
※基于Gabor特征提取和人工智能的人脸检测系统源代码『fdp5final.rar(185.56 KB) 』使用步骤:1. 拷贝所有文件到MATLAB工作目录下(确认已经安装了图像处理工具箱和人工智能工具箱)2. 找到"main.m"文件3. 命令行中运行它4. 点击"Train Network",等待程序训练好样本5. 点击"Test on Photos",选择一个.jpg图片,识别。
6. 等待程序检测出人脸区域createffnn.m, drawrec.m, gabor.m, im2vec.m, imscan.m, loadimages.m, main.m, template1.png, template2.png, trainnet.m※Eigenface人脸识别Matlab『face_recognition.rar (42.01 KB)』注:这个Matlab程序使用eigenface系统实现人脸识别,它使用AT&T的数据库,运行代码实例前,请阅读其中的comment,下载该数据库。
※人脸检测(文章说明+Matlab程序)『facedetcer.rar (1.59 MB)』注:国外的人脸检测检测文章和matlab程序,给需要的人。
※Linux下开源的C语言实时人脸识别系统源代码(malic)『malic.rar (1.26 MB) 』注:Malic是一个完整的Linux下的人脸识别系统源代码,它是SourceForge上的一个开源项目,使用Malib实现实时处理,CSU Face Identification Evaluation System进行人脸识别。
算法包括:主成份分析(principle components analysis (PCA)),a.k.a eigenfaces 算法,混合主成份分析,线性判别分析(PCA+LDA),图像差分分类器(IIDC),弹性图像匹配算法(EBGM)等等。
※Matlab下使用局部SMQT特征和SnoW分类器的人脸检测系统『SMQT-SnoW-Face-Detection.part1.rar (1.91 MB)SMQT-SnoW-Face-Detection.part2.rar (231.79 KB)』注:这个人脸检测系统开发于Matlab下,基于局部Successive Mean QuantizationTransform (SMQT)特征和split up Sparse Network of Winnows (SNoW)分类器。
理论来源的论文是:Face Detection using local SMQT features and split up SNoW classifier。
※Matlab主成份分析(PCA)人脸识别源代码『pca.zip (32.77 KB)』注:这是一个Matlab编写的基于PCA的人脸识别分类算法,对FERET数据库进行了分类。
包含的文件有:createDistMat.m,pca.m,feret.m,dup1,dup2,fb,fc,feretGallery,listAll,trainList使用方法:Run the function pca to create a variable pcaProj.Input variable pcaProj to the function createDistMat, thus creating a distance matrix that you then use as an input to the function feret. See headers ofall three functions for more details. The sequence should look something like this:>> load trainList.mat>> pca ('C:/FERET_Normalised/', trainList, 200);>> pcaDistMatCos = createDistMat(pcaProj, 'COS');>> pcaResultsCOS = feret(pcaDistMatCos, 50);>> pcaResultsCOS.perc(1) % gives rank 1 result>> plot(pcaResultsL1.cms) % plots the CMS curve※C#光照归一化算法(人脸识别预处理)『illumination-normalization.zip (245.21 KB)』这个c#编写的程序,用来对人脸图像进行预处理,从而提升人脸识别算法的性能。
这里提出了3种用于人脸识别的图像预处理的光照归一化算法,即:Multiscale retinex和anisotropic 和isotropic平滑方法。
主要算法原理来源于:"A Comparison of Photometric Normalisation Algorithms for Face Verification",James Short, Josef Kittler and Kieron Messer(2004) 和"Lighting Normalization Algorithms for Face Verification",Guillaume Heusch ,Fabien Cardinaux, Sebastien Marcel(2005)※基于主成分分析(PCA)的人脸识别系统『PCA-Face-Recognition.rar (179.13 KB) 』注:本程序实现了基于PCA的人脸识别方法,所有的函数都非常容易使用,且注释非常多,并且,附带了一个示例脚本和两个小的训练和测试数据库来展示其使用。
※用于静态图像人脸检测与识别的Karhunen-Loeve降解算法『KL.zip (1.52 KB)』注:这个Matlab程序通过Karhunen-Loeve变换的算法,实现了人脸检测与识别。
※人脸检测中的区域检测『人脸检测中的皮肤检测程序.rar (85.66 KB)』※MATLAB实现视频摄像中的运动检测(人体等)免费源代码『Motion-Detection-video.rar (658.88 KB)』注:这个matlab实现的程序中视频识别运动中的物体(通过连续的图像帧)并在一个窗口中展示那个运动的物体。
当你执行这个代码的时候一定别忘了检查输入的视频是否被MATLAB所支持。
所以,作者在代码文件中附加了一个实例视频文件。
※Matlab实现的基于FLD的人脸识别系统源代码『FLD-Face-Recognition.rar(180.03 KB)』注:这个程序包实现了一个基于FLD的人脸识别系统,称为'Fisherface',所有函数非常容易使用,并且有完整的注释。
而且,包括一个示例脚本,和两个小的测试和训练数据库。
※一个人工智能神经网络BrainNet源代码及完整的示例教程(并实现一个简单的手写文字检测与识别系统)『Neural-Network-Handwriting-Detection.rar (503.25 KB)注:文章一步步教你如何编写一个人工智能的神经网络程序,告诉你什么是神经元、神经网络和他们的应用程序,并介绍BrainNet-开源的人工神经网络库。
最后使用这个库,开发一个简单的手写文字识别的程序。
※OpenCV库的Matlab函数封装调用『OpenCV-wraper.rar (826.12 KB)』注:cvlib_mex封装了OpenCV库大约30个函数,OpenCV是当前流行的实时计算机视觉库,拥有很多的图像处理的算法。
※彩色图像人物局域检测(IEEE2007文章+程序)『彩色图像人物皮肤局域检测(IEEE2007文章+程序).rar (1.06 MB)』※人眼定位与人脸检测程序『人眼定位与人脸检测.rar (631.13 KB)』※用Matlab做的人脸识别系统里面包含了几十张人脸数据图像『用Matlab做的人脸识别系统.zip (297.77 KB)』※含有各种供人脸检测与识别的人脸库点击下载※OPENGL人脸识别(VC++源代码)『OPENGL人脸识别(VC++源代码).zip (105.37 KB) 』注:使用开源技术OpenCV实现人脸识别,识别率高而精准※最简单的Matlab人脸识别代码『最简单的Matlab人脸识别代码.rar (626.34 KB) 』注:这个是在Mathworks公司网站上找到了,测试了一下,感觉效果还不错。
只能识别single face, multiple face的话,还需要修改代码。
运行:facedetection.m如果是其他图片的话,放在当前文件下,修改一下文件名即可。
※基于人脸识别的双边2DLDA代码『基于人脸识别的双边2DLDA代码.part1.rar (1.91 MB)基于人脸识别的双边2DLDA代码.part2.rar (795.48 KB)』注:这是一个基于人脸识别的双边2DLDA代码,我看到一般写的2DLDA都是单边的,所以上传一个双边的,大家参考一下。