文本情感分析总结
文本标注总结
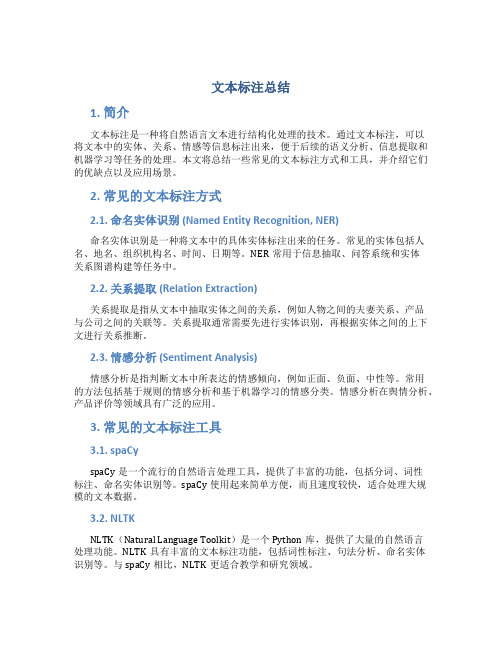
文本标注总结1. 简介文本标注是一种将自然语言文本进行结构化处理的技术。
通过文本标注,可以将文本中的实体、关系、情感等信息标注出来,便于后续的语义分析、信息提取和机器学习等任务的处理。
本文将总结一些常见的文本标注方式和工具,并介绍它们的优缺点以及应用场景。
2. 常见的文本标注方式2.1. 命名实体识别 (Named Entity Recognition, NER)命名实体识别是一种将文本中的具体实体标注出来的任务。
常见的实体包括人名、地名、组织机构名、时间、日期等。
NER常用于信息抽取、问答系统和实体关系图谱构建等任务中。
2.2. 关系提取 (Relation Extraction)关系提取是指从文本中抽取实体之间的关系,例如人物之间的夫妻关系、产品与公司之间的关联等。
关系提取通常需要先进行实体识别,再根据实体之间的上下文进行关系推断。
2.3. 情感分析 (Sentiment Analysis)情感分析是指判断文本中所表达的情感倾向,例如正面、负面、中性等。
常用的方法包括基于规则的情感分析和基于机器学习的情感分类。
情感分析在舆情分析、产品评价等领域具有广泛的应用。
3. 常见的文本标注工具3.1. spaCyspaCy是一个流行的自然语言处理工具,提供了丰富的功能,包括分词、词性标注、命名实体识别等。
spaCy使用起来简单方便,而且速度较快,适合处理大规模的文本数据。
3.2. NLTKNLTK(Natural Language Toolkit)是一个Python库,提供了大量的自然语言处理功能。
NLTK具有丰富的文本标注功能,包括词性标注、句法分析、命名实体识别等。
与spaCy相比,NLTK更适合教学和研究领域。
3.3. Standford NERStandford NER是一种基于统计模型的命名实体识别工具。
它的模型基于条件随机场(Conditional Random Fields),在英文命名实体识别任务中表现较好。
课文分析文本情感归纳
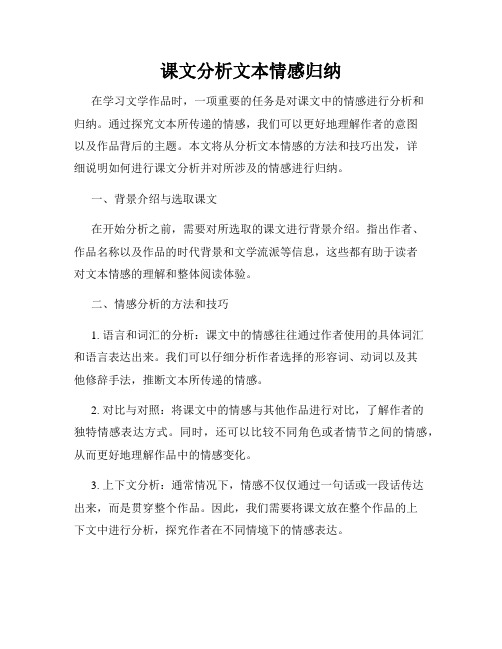
课文分析文本情感归纳在学习文学作品时,一项重要的任务是对课文中的情感进行分析和归纳。
通过探究文本所传递的情感,我们可以更好地理解作者的意图以及作品背后的主题。
本文将从分析文本情感的方法和技巧出发,详细说明如何进行课文分析并对所涉及的情感进行归纳。
一、背景介绍与选取课文在开始分析之前,需要对所选取的课文进行背景介绍。
指出作者、作品名称以及作品的时代背景和文学流派等信息,这些都有助于读者对文本情感的理解和整体阅读体验。
二、情感分析的方法和技巧1. 语言和词汇的分析:课文中的情感往往通过作者使用的具体词汇和语言表达出来。
我们可以仔细分析作者选择的形容词、动词以及其他修辞手法,推断文本所传递的情感。
2. 对比与对照:将课文中的情感与其他作品进行对比,了解作者的独特情感表达方式。
同时,还可以比较不同角色或者情节之间的情感,从而更好地理解作品中的情感变化。
3. 上下文分析:通常情况下,情感不仅仅通过一句话或一段话传达出来,而是贯穿整个作品。
因此,我们需要将课文放在整个作品的上下文中进行分析,探究作者在不同情境下的情感表达。
4. 肢体语言和非语言因素:在一些作品中,作者可能通过描写角色的肢体语言、面部表情或者其他非语言因素来传递情感。
通过对这些因素的分析,我们可以更加深入地理解文本中的情感。
三、情感归纳在对课文的情感进行分析之后,我们可以开始对所涉及的情感进行归纳。
通过对文本情感的总结和概括,我们可以更好地把握作品的主题和作者想要传递的信息。
1. 按照主题进行归纳:将课文中的情感按照主题进行分类归纳,列出各个情感所代表的主题是什么,这样可以更好地理解和传达作品的基本情感。
2. 角色情感的归纳:如果课文中有多个角色,我们可以对每个角色的情感进行归纳。
通过比较不同角色的情感表达,可以更好地理解作品中的人物关系和情感发展。
3. 情感随时间变化的归纳:有些作品中,情感可能会随着时间的推移发生变化。
我们可以按时间顺序归纳各个阶段的情感,进一步了解作品的情感发展与变化。
wlisa实验总结
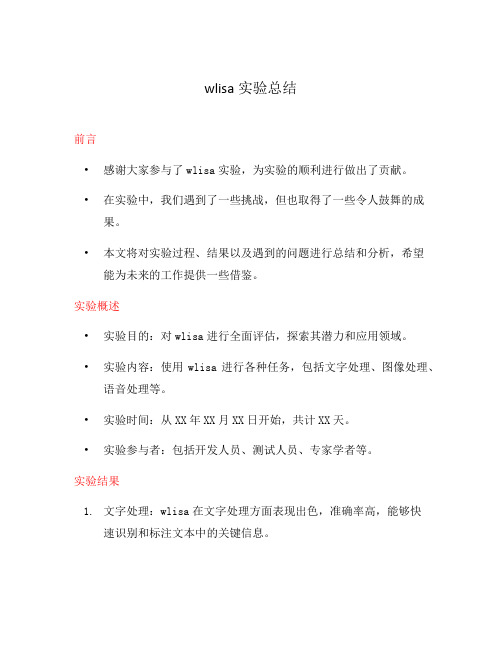
wlisa实验总结前言•感谢大家参与了wlisa实验,为实验的顺利进行做出了贡献。
•在实验中,我们遇到了一些挑战,但也取得了一些令人鼓舞的成果。
•本文将对实验过程、结果以及遇到的问题进行总结和分析,希望能为未来的工作提供一些借鉴。
实验概述•实验目的:对wlisa进行全面评估,探索其潜力和应用领域。
•实验内容:使用wlisa进行各种任务,包括文字处理、图像处理、语音处理等。
•实验时间:从XX年XX月XX日开始,共计XX天。
•实验参与者:包括开发人员、测试人员、专家学者等。
实验结果1.文字处理:wlisa在文字处理方面表现出色,准确率高,能够快速识别和标注文本中的关键信息。
–识别和提取实体:wlisa能够准确识别文本中的人物、地点、时间等实体,并能够将其标注出来。
–文本情感分析:wlisa可以对文本进行情感分析,识别出其中的情感倾向。
2.图像处理:wlisa在图像处理方面也表现出色,能够准确判断图像内容并进行相应的处理。
–图像分类和识别:wlisa能够将图像分为不同的类别,并对图像进行准确的识别。
–物体检测和定位:wlisa能够对图像中的物体进行精准的检测和定位。
3.语音处理:wlisa在语音处理方面取得了一定的成果,但仍然存在一些改进空间。
–语音识别:wlisa能够对语音进行识别,但在嘈杂环境下准确率有所下降。
–语音合成:wlisa能够将文字转化为语音,但还需要进一步优化语音的自然度和流畅度。
遇到的问题及改进方向1.性能问题:在处理大量数据时,wlisa的响应速度较慢,需要进一步优化算法和数据处理。
2.准确性问题:在某些情况下,wlisa的准确率不够高,需要进一步改进模型和训练方法。
3.多语言支持:wlisa目前只支持中文,未来需要考虑增加对其他语言的支持。
4.系统集成:wlisa目前作为独立的系统存在,未来考虑将其集成到其他应用系统中,提高其实用性和便利性。
结尾•通过这次实验,我们对wlisa的性能和潜力有了更深入的了解。
情绪分析技术总结范文

随着互联网技术的飞速发展,大数据和人工智能技术逐渐深入到我们的日常生活和工作之中。
情绪分析作为人工智能领域的一个重要分支,近年来得到了广泛关注和应用。
本文将对情绪分析技术进行总结,旨在梳理现有技术方法,分析其优缺点,并展望未来发展趋势。
一、情绪分析技术概述情绪分析,又称为情感分析,是指利用自然语言处理、机器学习等技术,对文本、语音、图像等多模态数据中的情绪信息进行提取、识别和分类的过程。
情绪分析技术广泛应用于舆情分析、智能客服、心理健康等领域。
二、情绪分析技术方法1. 基于词典的方法词典法是情绪分析技术中最简单、最直接的方法。
该方法通过构建情绪词典,将文本中的词语与情绪标签进行关联,从而实现情绪识别。
词典法的主要优点是简单易行,但存在以下缺点:(1)词典的构建和维护成本较高;(2)对复杂情感和隐喻表达识别能力有限;(3)受文本语境影响较大。
2. 基于规则的方法规则法是通过分析文本中的语法、语义、逻辑关系等特征,构建情绪识别规则,从而实现情绪分类。
规则法的主要优点是准确率高,但存在以下缺点:(1)规则构建复杂,需要大量人工干预;(2)对复杂情感和隐喻表达识别能力有限;(3)难以适应不同领域和语言环境。
3. 基于机器学习的方法机器学习方法通过训练大量标注好的数据集,让计算机自动学习情绪识别规律。
常见的机器学习方法有:(1)朴素贝叶斯:适用于文本分类任务,准确率较高;(2)支持向量机(SVM):适用于高维数据,准确率较高;(3)深度学习:通过神经网络模型,实现更高级别的特征提取和情感识别。
机器学习方法的主要优点是能够自动学习情绪识别规律,但存在以下缺点:(1)需要大量标注数据;(2)对数据质量要求较高;(3)模型复杂,训练和推理时间较长。
4. 基于情感计算的方法情感计算是研究人类情感和情感表达的技术。
通过分析文本、语音、图像等多模态数据,情感计算可以识别、理解和模拟人类情感。
情感计算的主要优点是能够处理多模态数据,但存在以下缺点:(1)技术复杂,涉及多个学科领域;(2)对数据质量要求较高;(3)难以实现跨领域和跨语言的情感识别。
人工智能工程师情感分析算法总结
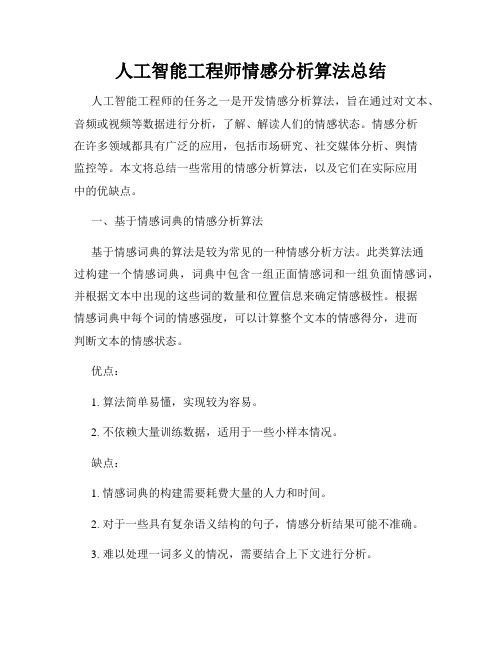
人工智能工程师情感分析算法总结人工智能工程师的任务之一是开发情感分析算法,旨在通过对文本、音频或视频等数据进行分析,了解、解读人们的情感状态。
情感分析在许多领域都具有广泛的应用,包括市场研究、社交媒体分析、舆情监控等。
本文将总结一些常用的情感分析算法,以及它们在实际应用中的优缺点。
一、基于情感词典的情感分析算法基于情感词典的算法是较为常见的一种情感分析方法。
此类算法通过构建一个情感词典,词典中包含一组正面情感词和一组负面情感词,并根据文本中出现的这些词的数量和位置信息来确定情感极性。
根据情感词典中每个词的情感强度,可以计算整个文本的情感得分,进而判断文本的情感状态。
优点:1. 算法简单易懂,实现较为容易。
2. 不依赖大量训练数据,适用于一些小样本情况。
缺点:1. 情感词典的构建需要耗费大量的人力和时间。
2. 对于一些具有复杂语义结构的句子,情感分析结果可能不准确。
3. 难以处理一词多义的情况,需要结合上下文进行分析。
二、基于机器学习的情感分析算法基于机器学习的情感分析算法通过使用已标注的训练数据,确定词语和文本特征之间的关联,进而进行情感分类。
常见的方法包括支持向量机(SVM)、朴素贝叶斯(Naive Bayes)和随机森林(Random Forest)等。
优点:1. 可以充分利用大量的标注数据进行训练,提高情感分析的准确性。
2. 能够处理一些复杂的语义结构和上下文信息。
缺点:1. 对于数据量较小的情况,容易出现过拟合问题。
2. 模型的训练和优化需要较长的时间和计算资源。
3. 需要大量的特征工程,提取合适的文本特征。
三、基于深度学习的情感分析算法基于深度学习的情感分析算法近年来得到了快速发展,特别是使用循环神经网络(RNN)和长短时记忆网络(LSTM)进行情感分析已取得不错的效果。
这些算法通过构建深度神经网络来自动学习文本中的上下文信息和语义表示。
优点:1. 能够处理更复杂的语义结构和长距离依赖关系,提高情感分析的准确性。
情感分析和文本分类从文本中提取情感信息

上下文信息
利用上下文信息,理解词 在特定语境下的含义。
局限性
难以捕捉复杂的语义关系 ,且对语料库的依赖较大 。
03
文本分类技术
基于规则的方法
词法分析
基于规则的方法首先对文本进行 词法分析,包括分词、词性标注 和命名实体识别等,以提取文本
中的关键信息。
情感词典
利用情感词典,基于规则的方法可 以计算文本中每个单词或短语的极 性和情感倾向,从而得出整个文本 的情感倾向。
情感分析和文本 分类从文本中提 取情感信息
汇报人:XXX
2023-11-22
目录
• 情感分析概述 • 情感分析技术 • 文本分类技术 • 情感分析和文本分类的应用场景 • 情感分析和文本分类的挑战与未
来发展
01
情感分析概述
情感分析的定义
01
情感分析是指通过自然语言处理 技术,对文本中的情感信息进行 分析、理解和提取的过程。
提高客户服务质量和效率
详细描述
通过对客户服务热线的通话记录进行分析,企业可以了解客户的需求、问题和意见,从而优化客户服务流程,提 高客户满意度。
新闻报道分析
总结词
快速了解新闻事件的发展趋势
详细描述
通过对新闻报道进行分析,可以快速了解新闻事件的发展趋势、公众对新闻的反应和态度,为决策者 提供参考。
05
局限性
基于规则的方法往往需要手动制定 规则和情感词典,因此时间和人力 成本较高,且规则和词典的覆盖范 围有限。
基于机器学习的方法
特征提取
基于机器学习的方法通常需要 从文本中提取特征,如词频、
n-gram、TF-IDF等。
模型训练
使用已标记的文本数据集进行 模型训练,学习文本特征与情 感类别之间的关系。
文本挖掘知识点总结初中
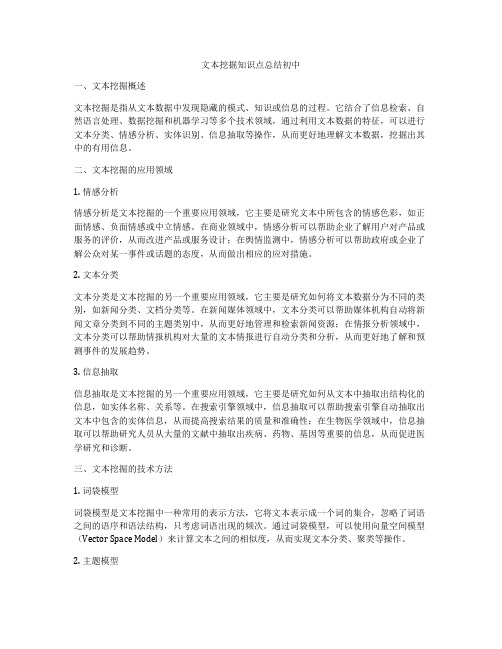
文本挖掘知识点总结初中一、文本挖掘概述文本挖掘是指从文本数据中发现隐藏的模式、知识或信息的过程。
它结合了信息检索、自然语言处理、数据挖掘和机器学习等多个技术领域,通过利用文本数据的特征,可以进行文本分类、情感分析、实体识别、信息抽取等操作,从而更好地理解文本数据,挖掘出其中的有用信息。
二、文本挖掘的应用领域1. 情感分析情感分析是文本挖掘的一个重要应用领域,它主要是研究文本中所包含的情感色彩,如正面情感、负面情感或中立情感。
在商业领域中,情感分析可以帮助企业了解用户对产品或服务的评价,从而改进产品或服务设计;在舆情监测中,情感分析可以帮助政府或企业了解公众对某一事件或话题的态度,从而做出相应的应对措施。
2. 文本分类文本分类是文本挖掘的另一个重要应用领域,它主要是研究如何将文本数据分为不同的类别,如新闻分类、文档分类等。
在新闻媒体领域中,文本分类可以帮助媒体机构自动将新闻文章分类到不同的主题类别中,从而更好地管理和检索新闻资源;在情报分析领域中,文本分类可以帮助情报机构对大量的文本情报进行自动分类和分析,从而更好地了解和预测事件的发展趋势。
3. 信息抽取信息抽取是文本挖掘的另一个重要应用领域,它主要是研究如何从文本中抽取出结构化的信息,如实体名称、关系等。
在搜索引擎领域中,信息抽取可以帮助搜索引擎自动抽取出文本中包含的实体信息,从而提高搜索结果的质量和准确性;在生物医学领域中,信息抽取可以帮助研究人员从大量的文献中抽取出疾病、药物、基因等重要的信息,从而促进医学研究和诊断。
三、文本挖掘的技术方法1. 词袋模型词袋模型是文本挖掘中一种常用的表示方法,它将文本表示成一个词的集合,忽略了词语之间的语序和语法结构,只考虑词语出现的频次。
通过词袋模型,可以使用向量空间模型(Vector Space Model)来计算文本之间的相似度,从而实现文本分类、聚类等操作。
2. 主题模型主题模型是文本挖掘中一种常用的分析方法,它通过发现文本中隐藏的主题结构,从而实现话题检测、话题建模等操作。
基于文本挖掘的评论情感分析方法研究
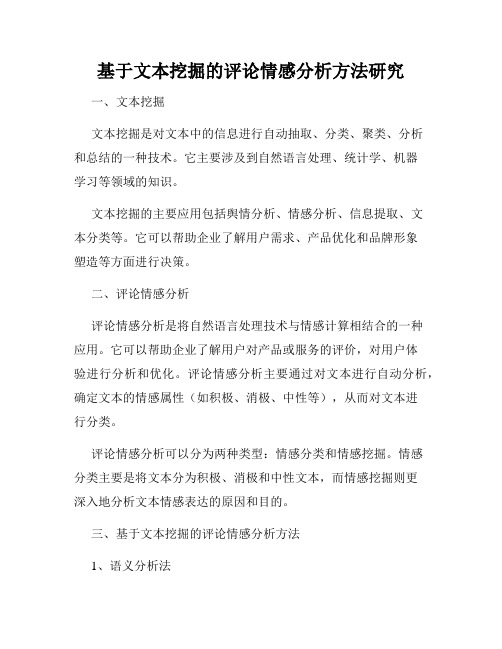
基于文本挖掘的评论情感分析方法研究一、文本挖掘文本挖掘是对文本中的信息进行自动抽取、分类、聚类、分析和总结的一种技术。
它主要涉及到自然语言处理、统计学、机器学习等领域的知识。
文本挖掘的主要应用包括舆情分析、情感分析、信息提取、文本分类等。
它可以帮助企业了解用户需求、产品优化和品牌形象塑造等方面进行决策。
二、评论情感分析评论情感分析是将自然语言处理技术与情感计算相结合的一种应用。
它可以帮助企业了解用户对产品或服务的评价,对用户体验进行分析和优化。
评论情感分析主要通过对文本进行自动分析,确定文本的情感属性(如积极、消极、中性等),从而对文本进行分类。
评论情感分析可以分为两种类型:情感分类和情感挖掘。
情感分类主要是将文本分为积极、消极和中性文本,而情感挖掘则更深入地分析文本情感表达的原因和目的。
三、基于文本挖掘的评论情感分析方法1、语义分析法语义分析法主要利用自然语言处理和文本挖掘技术,将文本处理成结构化的数据。
通过分析词汇、句法结构、语义关系等因素来确定情感类别,从而进行情感分类和情感挖掘。
2、情感词典法情感词典法主要基于一系列预设的情感词汇表,将文本中的情感单词与词汇表进行匹配,从而确定情感类别。
其中积极词汇和消极词汇的权值不一样,可以通过权值进行情感倾向的计算。
3、机器学习法机器学习法主要通过对大量训练数据进行学习和建模,从而确定文本的情感类别。
它包括决策树、支持向量机、朴素贝叶斯等多种算法。
机器学习法可以通过人工标注的训练数据和自动化的特征提取来进行训练,得到高准确率的情感分类模型。
四、评论情感分析的应用评论情感分析可以应用于电商、社交网络、网络游戏、在线客服等多个领域。
例如,电商企业可以通过评论情感分析技术了解用户对商品的评价和需求,及时调整产品策略,提高销售额度和用户体验。
在社交网络领域中,企业可以通过评论情感分析技术了解用户对话题或事件的看法和态度,为信息传播和用户引导提供参考。
五、结论评论情感分析技术应用广泛,可以帮助企业深入了解用户需求和行为,从而优化产品和服务,提高企业竞争力。
调查报告情感分析方法
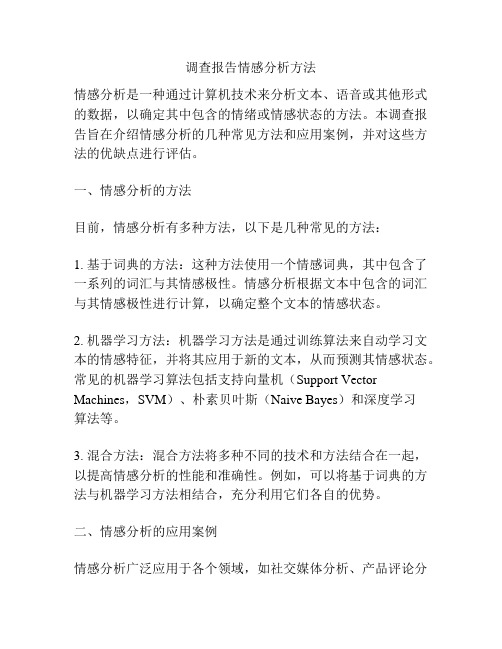
调查报告情感分析方法情感分析是一种通过计算机技术来分析文本、语音或其他形式的数据,以确定其中包含的情绪或情感状态的方法。
本调查报告旨在介绍情感分析的几种常见方法和应用案例,并对这些方法的优缺点进行评估。
一、情感分析的方法目前,情感分析有多种方法,以下是几种常见的方法:1. 基于词典的方法:这种方法使用一个情感词典,其中包含了一系列的词汇与其情感极性。
情感分析根据文本中包含的词汇与其情感极性进行计算,以确定整个文本的情感状态。
2. 机器学习方法:机器学习方法是通过训练算法来自动学习文本的情感特征,并将其应用于新的文本,从而预测其情感状态。
常见的机器学习算法包括支持向量机(Support Vector Machines,SVM)、朴素贝叶斯(Naive Bayes)和深度学习算法等。
3. 混合方法:混合方法将多种不同的技术和方法结合在一起,以提高情感分析的性能和准确性。
例如,可以将基于词典的方法与机器学习方法相结合,充分利用它们各自的优势。
二、情感分析的应用案例情感分析广泛应用于各个领域,如社交媒体分析、产品评论分析、舆情监测等。
以下是几个常见的应用案例:1. 社交媒体分析:情感分析可以帮助企业或机构分析社交媒体平台上的用户评论、帖子和推文,以了解公众对其产品、服务或品牌的情感倾向。
2. 产品评论分析:情感分析可以帮助企业分析产品评论中的情感态度,以了解用户对其产品的满意度或不满意度,并根据分析结果来改进产品。
3. 舆情监测:情感分析可以对舆情进行监测,了解公众对某一事件、话题或个体的情感态度,帮助企业或政府及时了解社会舆论动向。
三、评估情感分析方法的优缺点不同的情感分析方法具有各自的优缺点,以下是对几种常见方法的评估:1. 基于词典的方法:优点是简单且易于实现,缺点是对于新词汇的情感判断可能不准确,并且无法考虑词汇在上下文中的语义。
2. 机器学习方法:优点是能够自动学习文本的情感特征,并具有较高的准确性。
自然语言处理中常见的文本情感识别模型(六)
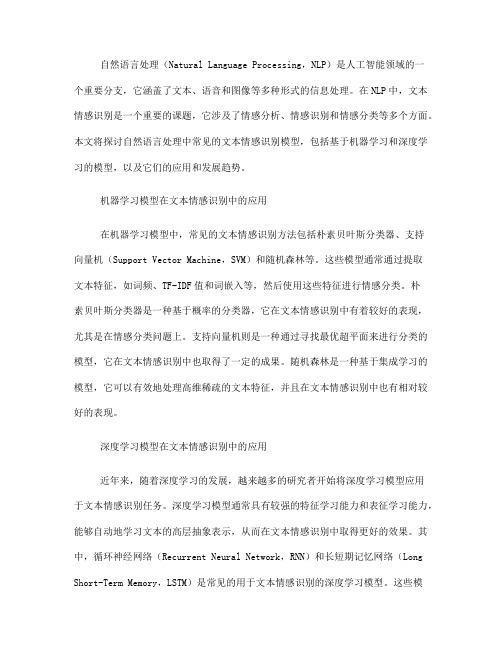
自然语言处理(Natural Language Processing,NLP)是人工智能领域的一个重要分支,它涵盖了文本、语音和图像等多种形式的信息处理。
在NLP中,文本情感识别是一个重要的课题,它涉及了情感分析、情感识别和情感分类等多个方面。
本文将探讨自然语言处理中常见的文本情感识别模型,包括基于机器学习和深度学习的模型,以及它们的应用和发展趋势。
机器学习模型在文本情感识别中的应用在机器学习模型中,常见的文本情感识别方法包括朴素贝叶斯分类器、支持向量机(Support Vector Machine,SVM)和随机森林等。
这些模型通常通过提取文本特征,如词频、TF-IDF值和词嵌入等,然后使用这些特征进行情感分类。
朴素贝叶斯分类器是一种基于概率的分类器,它在文本情感识别中有着较好的表现,尤其是在情感分类问题上。
支持向量机则是一种通过寻找最优超平面来进行分类的模型,它在文本情感识别中也取得了一定的成果。
随机森林是一种基于集成学习的模型,它可以有效地处理高维稀疏的文本特征,并且在文本情感识别中也有相对较好的表现。
深度学习模型在文本情感识别中的应用近年来,随着深度学习的发展,越来越多的研究者开始将深度学习模型应用于文本情感识别任务。
深度学习模型通常具有较强的特征学习能力和表征学习能力,能够自动地学习文本的高层抽象表示,从而在文本情感识别中取得更好的效果。
其中,循环神经网络(Recurrent Neural Network,RNN)和长短期记忆网络(Long Short-Term Memory,LSTM)是常见的用于文本情感识别的深度学习模型。
这些模型能够有效地捕捉文本中的时序信息和上下文信息,从而提高情感识别的准确性和鲁棒性。
深度学习模型在文本情感识别中的应用还包括卷积神经网络(Convolutional Neural Network,CNN)和注意力机制(Attention Mechanism)等。
舆情文本分析总结范文

随着互联网技术的飞速发展,网络舆情已经成为社会舆论的重要组成部分。
对舆情文本进行深入分析,有助于我们更好地了解社会热点、把握舆论动态,为政府、企业和社会组织提供决策参考。
以下是一篇关于舆情文本分析的总结范文,供参考。
一、舆情文本分析概述舆情文本分析是指通过对网络上的文本信息进行收集、整理、分析和解读,以了解公众关注的热点、观点和情感倾向。
本文以2023年某热点事件为例,对舆情文本进行分析。
二、舆情文本分析过程1. 数据收集针对该热点事件,我们从微博、微信、新闻网站等渠道收集了大量的文本数据,包括新闻报道、网友评论、政府回应等。
2. 数据整理对收集到的文本数据进行初步筛选,剔除无关信息,保留与事件相关的有效文本。
然后,对有效文本进行分词、去停用词等预处理操作。
3. 关键词提取利用关键词提取技术,从预处理后的文本中提取出反映事件核心内容的关键词。
例如,本次事件的关键词包括“事件名称”、“涉事方”、“影响”、“应对措施”等。
4. 情感分析运用情感分析技术,对提取出的关键词进行分析,了解公众对事件的整体情感倾向。
本次事件中,网友评论主要分为三类:积极、中立和消极。
其中,消极评论占比最高,主要集中在事件处理不公、损害群众利益等方面。
5. 舆情传播路径分析通过分析事件传播路径,了解事件在社交媒体上的传播规律。
本次事件中,微博作为主要传播渠道,事件信息迅速在网友之间传播,形成舆论热点。
三、舆情分析总结1. 事件关注度较高从舆情数据来看,该热点事件引起了广泛关注,网友评论数量众多,传播速度快,影响范围广。
2. 公众情感以消极为主从情感分析结果来看,公众对事件的整体情感倾向以消极为主,主要表现在对事件处理不公、损害群众利益等方面。
3. 舆情传播迅速,影响力大事件通过微博等社交媒体迅速传播,形成舆论热点,对政府、企业和社会组织产生了一定影响。
四、建议1. 政府部门应加强舆情监测,及时了解公众关注的热点问题,提高事件处理效率。
自然语言处理中常见的文本情感识别模型(九)
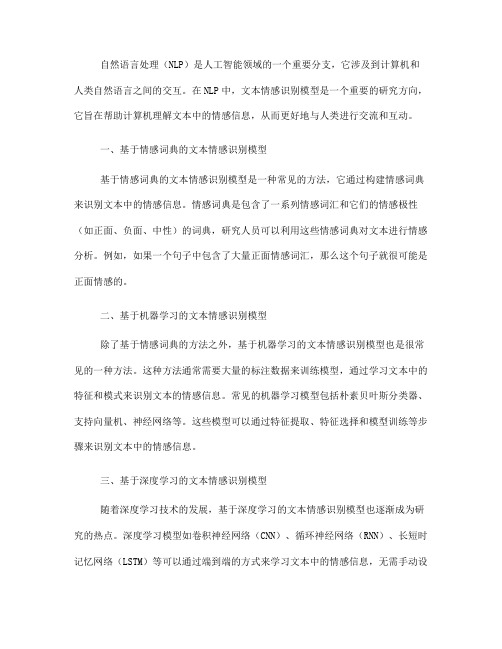
自然语言处理(NLP)是人工智能领域的一个重要分支,它涉及到计算机和人类自然语言之间的交互。
在NLP中,文本情感识别模型是一个重要的研究方向,它旨在帮助计算机理解文本中的情感信息,从而更好地与人类进行交流和互动。
一、基于情感词典的文本情感识别模型基于情感词典的文本情感识别模型是一种常见的方法,它通过构建情感词典来识别文本中的情感信息。
情感词典是包含了一系列情感词汇和它们的情感极性(如正面、负面、中性)的词典,研究人员可以利用这些情感词典对文本进行情感分析。
例如,如果一个句子中包含了大量正面情感词汇,那么这个句子就很可能是正面情感的。
二、基于机器学习的文本情感识别模型除了基于情感词典的方法之外,基于机器学习的文本情感识别模型也是很常见的一种方法。
这种方法通常需要大量的标注数据来训练模型,通过学习文本中的特征和模式来识别文本的情感信息。
常见的机器学习模型包括朴素贝叶斯分类器、支持向量机、神经网络等。
这些模型可以通过特征提取、特征选择和模型训练等步骤来识别文本中的情感信息。
三、基于深度学习的文本情感识别模型随着深度学习技术的发展,基于深度学习的文本情感识别模型也逐渐成为研究的热点。
深度学习模型如卷积神经网络(CNN)、循环神经网络(RNN)、长短时记忆网络(LSTM)等可以通过端到端的方式来学习文本中的情感信息,无需手动设计特征。
这些模型可以通过大规模语料库来学习文本的表示,并通过反向传播算法来更新模型参数,从而识别文本中的情感信息。
四、基于知识图谱的文本情感识别模型除了上述方法之外,近年来还出现了一些基于知识图谱的文本情感识别模型。
知识图谱是一种结构化的知识表示形式,可以帮助计算机更好地理解文本中的实体和关系。
基于知识图谱的情感识别模型可以利用知识图谱中的情感知识来识别文本中的情感信息,从而提高情感识别的准确性和效果。
总结文本情感识别模型是NLP领域的一个重要研究方向,它在情感分析、舆情监测、情感交互等方面具有广泛的应用前景。
如何利用自然语言处理技术进行文本情感分类的实践经验(五)

自然语言处理技术是一种人工智能技术,它可以帮助计算机理解、解释和处理人类语言。
其中,文本情感分类是自然语言处理技术的一个重要应用,它可以帮助我们分析文本中的情感倾向,了解人们对某个话题的态度和情感,对于市场调研、舆情监控等方面都具有重要意义。
在实践中,利用自然语言处理技术进行文本情感分类需要考虑到多方面因素,下面将从数据收集、特征提取、模型选择和性能评估等方面进行探讨。
数据收集是进行文本情感分类的第一步,良好的数据质量对于模型训练和性能表现至关重要。
在实践中,我们可以通过爬虫技术从互联网上收集相关文本数据,也可以从已有的数据集中进行选择和整理。
在选择数据集时,需要考虑到数据的多样性和代表性,以及数据的标注质量。
同时,还需要考虑到数据的规模和平衡性,确保数据集中各类别样本的数量基本均衡,避免由于样本不均衡导致模型训练和性能评估出现偏差。
特征提取是文本情感分类的关键环节,它直接影响了模型对文本情感的理解和分类能力。
在实践中,我们可以通过词袋模型、TF-IDF、词嵌入等技术将文本数据转化为特征向量。
其中,词嵌入技术由于其可以将单词表示为低维稠密向量,能够较好地捕捉单词之间的语义关系,因此在文本情感分类任务中得到了广泛的应用。
除此之外,还可以考虑将文本数据进行预处理,包括分词、去除停用词、词形还原等操作,以提高特征的表示能力和模型的泛化能力。
模型选择是进行文本情感分类的另一个关键环节,不同的模型对于文本情感的理解和分类能力有所差异。
在实践中,我们可以选择传统的机器学习模型如朴素贝叶斯、支持向量机等,也可以选择深度学习模型如卷积神经网络、循环神经网络等。
在选择模型时,需要考虑到数据规模、特征维度、计算资源等因素,选择适合实际应用场景的模型。
同时,还需要考虑模型的训练时间、调参难度、模型的解释性等方面的因素,综合考虑选择最适合的模型。
性能评估是进行文本情感分类的最后一步,它可以帮助我们评估模型的分类能力和泛化能力。
小说思想情感分析方法总结

小说思想情感分析方法总结小说思想情感分析方法总结小说是一种通过虚构故事来传达作者思想和情感的文学形式。
对于读者来说,理解和分析小说中的思想和情感是阅读的重要目标之一。
本文将总结一些常用的小说思想情感分析方法,帮助读者更好地理解和品味小说的内涵。
首先,了解作者的背景和生平经历是分析小说思想情感的重要依据。
作者的思想和情感往往受其生活经历、社会环境和文化背景的影响。
通过了解作者的个人背景,读者可以更好地理解小说中所传达的思想和情感,并从中找到与自己的生活经验和情感共鸣之处。
其次,细读文本并注意语言运用也是分析小说思想情感的重要途径。
小说作为一种文学形式,通过语言来传达作者的思想和情感。
读者可以仔细分析小说中的文字、词语和句法结构,通过文字的选择和安排来揭示作者的思想和情感态度。
同时,也要注意作者在描写人物形象、场景和事件时所运用的修辞手法,例如对比、隐喻、夸张等,这些手法往往能够深化情感表达,烘托出小说的思想内涵。
第三,注意角色形象和情节发展是分析小说思想情感的重要方面。
小说中的人物形象和情节往往是作者传达思想和情感的具体表现形式。
通过细致描写人物的特征和性格,读者可以窥探到作者对这些角色的看法和态度。
同时,情节的发展和转折也可以反映出作者的思想观点和情感体验。
通过对角色形象和情节的分析,读者可以更深入地理解小说的意义和主题。
最后,与其他作品和文化背景进行对比也是分析小说思想情感的有效方法。
每个小说都有其独特的思想和情感特点,但也会受到其所处的时代和文化背景的影响。
读者可以将小说与其他作品进行对比,寻找共同点和差异,从中得出对作者思想和情感的更深入理解。
同时,了解作者所处的时代和文化背景也有助于读者更好地把握小说的思想和情感内涵。
综上所述,分析小说思想情感的方法包括了解作者背景、细读文本、注意角色形象和情节发展,以及进行对比分析等。
通过运用这些方法,读者可以更深入地理解和品味小说中所蕴含的思想和情感,增强阅读的体验和感悟。
自然语言处理实训课程学习总结文本分类与情感分析技术研究
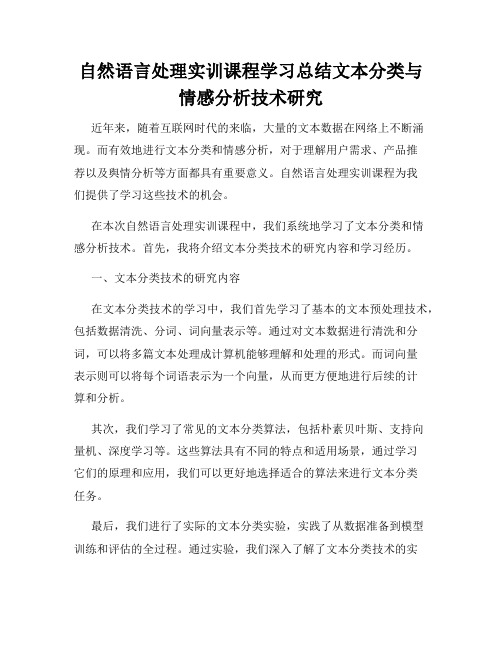
自然语言处理实训课程学习总结文本分类与情感分析技术研究近年来,随着互联网时代的来临,大量的文本数据在网络上不断涌现。
而有效地进行文本分类和情感分析,对于理解用户需求、产品推荐以及舆情分析等方面都具有重要意义。
自然语言处理实训课程为我们提供了学习这些技术的机会。
在本次自然语言处理实训课程中,我们系统地学习了文本分类和情感分析技术。
首先,我将介绍文本分类技术的研究内容和学习经历。
一、文本分类技术的研究内容在文本分类技术的学习中,我们首先学习了基本的文本预处理技术,包括数据清洗、分词、词向量表示等。
通过对文本数据进行清洗和分词,可以将多篇文本处理成计算机能够理解和处理的形式。
而词向量表示则可以将每个词语表示为一个向量,从而更方便地进行后续的计算和分析。
其次,我们学习了常见的文本分类算法,包括朴素贝叶斯、支持向量机、深度学习等。
这些算法具有不同的特点和适用场景,通过学习它们的原理和应用,我们可以更好地选择适合的算法来进行文本分类任务。
最后,我们进行了实际的文本分类实验,实践了从数据准备到模型训练和评估的全过程。
通过实验,我们深入了解了文本分类技术的实际应用和不同算法的性能对比,进一步提升了我们的实践能力和问题解决能力。
二、情感分析技术的研究内容在情感分析技术的学习中,我们首先了解了情感分析的基本概念和应用场景。
情感分析是一种通过自然语言处理技术来识别和分析文本中蕴含的情感倾向的方法,可以帮助我们了解用户的情感态度和情绪变化。
接着,我们学习了情感分析的主要方法和模型,包括基于规则的方法、基于机器学习的方法和基于深度学习的方法。
不同的方法适用于不同的情感分析场景,我们通过学习它们的原理和实际应用案例,进一步了解了情感分析技术的发展和应用前景。
最后,我们进行了情感分析的实践项目,通过实际的文本数据,构建情感分析模型,并对其进行评估和优化。
这个实践项目不仅提升了我们的实践能力,还加深了我们对情感分析技术的理解和掌握程度。
知识点归纳 自然语言处理中的情感分析与文本生成
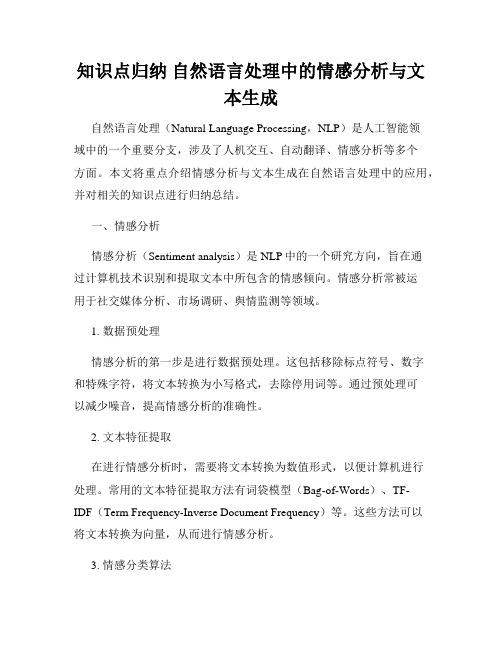
知识点归纳自然语言处理中的情感分析与文本生成自然语言处理(Natural Language Processing,NLP)是人工智能领域中的一个重要分支,涉及了人机交互、自动翻译、情感分析等多个方面。
本文将重点介绍情感分析与文本生成在自然语言处理中的应用,并对相关的知识点进行归纳总结。
一、情感分析情感分析(Sentiment analysis)是NLP中的一个研究方向,旨在通过计算机技术识别和提取文本中所包含的情感倾向。
情感分析常被运用于社交媒体分析、市场调研、舆情监测等领域。
1. 数据预处理情感分析的第一步是进行数据预处理。
这包括移除标点符号、数字和特殊字符,将文本转换为小写格式,去除停用词等。
通过预处理可以减少噪音,提高情感分析的准确性。
2. 文本特征提取在进行情感分析时,需要将文本转换为数值形式,以便计算机进行处理。
常用的文本特征提取方法有词袋模型(Bag-of-Words)、TF-IDF(Term Frequency-Inverse Document Frequency)等。
这些方法可以将文本转换为向量,从而进行情感分析。
3. 情感分类算法情感分析的核心在于情感分类算法。
常见的情感分类算法包括朴素贝叶斯分类器(Naive Bayes Classifier)、支持向量机(Support Vector Machine)、神经网络等。
这些算法可以通过训练数据进行学习,从而对新的文本进行情感分类。
4. 情感分析应用情感分析在实际应用中有着广泛的应用。
例如,在社交媒体分析中,可以通过分析用户的评论和情感倾向,了解用户对产品或事件的态度;在舆情监测中,可以通过分析大量的新闻报道和社交媒体信息,了解公众对某一事件的情感倾向。
二、文本生成文本生成是NLP中的另一个研究热点,旨在让计算机能够自动生成符合语法和语义规则的自然语言文本。
文本生成有多种应用,如自动摘要、文本生成模型等。
1. 语言模型语言模型是文本生成的基础。
使用ChatGPT进行文本分类与情感分析的技巧与方法总结
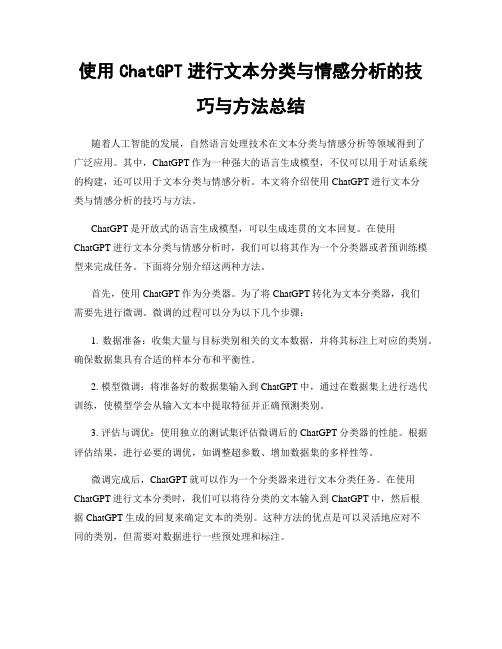
使用ChatGPT进行文本分类与情感分析的技巧与方法总结随着人工智能的发展,自然语言处理技术在文本分类与情感分析等领域得到了广泛应用。
其中,ChatGPT作为一种强大的语言生成模型,不仅可以用于对话系统的构建,还可以用于文本分类与情感分析。
本文将介绍使用ChatGPT进行文本分类与情感分析的技巧与方法。
ChatGPT是开放式的语言生成模型,可以生成连贯的文本回复。
在使用ChatGPT进行文本分类与情感分析时,我们可以将其作为一个分类器或者预训练模型来完成任务。
下面将分别介绍这两种方法。
首先,使用ChatGPT作为分类器。
为了将ChatGPT转化为文本分类器,我们需要先进行微调。
微调的过程可以分为以下几个步骤:1. 数据准备:收集大量与目标类别相关的文本数据,并将其标注上对应的类别。
确保数据集具有合适的样本分布和平衡性。
2. 模型微调:将准备好的数据集输入到ChatGPT中,通过在数据集上进行迭代训练,使模型学会从输入文本中提取特征并正确预测类别。
3. 评估与调优:使用独立的测试集评估微调后的ChatGPT分类器的性能。
根据评估结果,进行必要的调优,如调整超参数、增加数据集的多样性等。
微调完成后,ChatGPT就可以作为一个分类器来进行文本分类任务。
在使用ChatGPT进行文本分类时,我们可以将待分类的文本输入到ChatGPT中,然后根据ChatGPT生成的回复来确定文本的类别。
这种方法的优点是可以灵活地应对不同的类别,但需要对数据进行一些预处理和标注。
其次,使用ChatGPT作为预训练模型。
ChatGPT通过大规模无监督学习得到了强大的语言模型,可以用于生成连贯的文本回复。
在使用ChatGPT进行情感分析时,我们可以采取以下方法:1. 数据预处理:将待分析的文本转化成ChatGPT的输入格式。
一般来说,我们需要将文本进行分段,并在开始和结束处添加特殊标记,以指示生成回复的边界。
2. 模型生成:将预处理后的文本输入到ChatGPT中,生成相应的回复。
文本情感分析论文总结
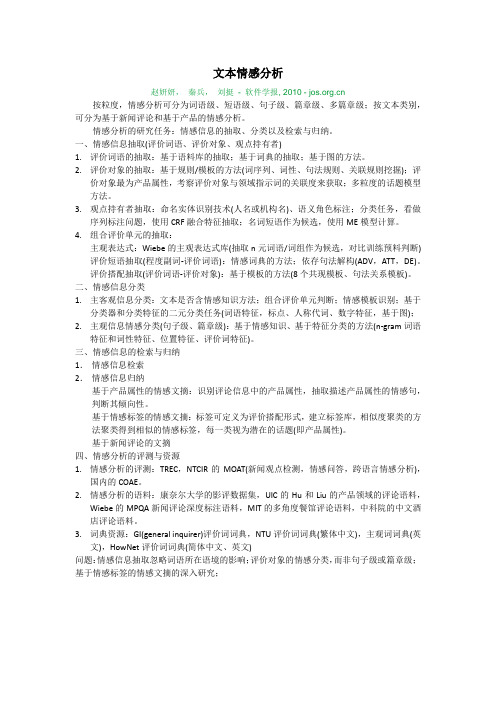
文本情感分析赵妍妍,秦兵,刘挺- 软件学报, 2010 - 按粒度,情感分析可分为词语级、短语级、句子级、篇章级、多篇章级;按文本类别,可分为基于新闻评论和基于产品的情感分析。
情感分析的研究任务:情感信息的抽取、分类以及检索与归纳。
一、情感信息抽取(评价词语、评价对象、观点持有者)1.评价词语的抽取:基于语料库的抽取;基于词典的抽取;基于图的方法。
2.评价对象的抽取:基于规则/模板的方法(词序列、词性、句法规则、关联规则挖掘);评价对象最为产品属性,考察评价对象与领域指示词的关联度来获取;多粒度的话题模型方法。
3.观点持有者抽取:命名实体识别技术(人名或机构名)、语义角色标注;分类任务,看做序列标注问题,使用CRF融合特征抽取;名词短语作为候选,使用ME模型计算。
4.组合评价单元的抽取:主观表达式:Wiebe的主观表达式库(抽取n元词语/词组作为候选,对比训练预料判断) 评价短语抽取(程度副词-评价词语):情感词典的方法;依存句法解构(ADV,ATT,DE)。
评价搭配抽取(评价词语-评价对象):基于模板的方法(8个共现模板、句法关系模板)。
二、情感信息分类1.主客观信息分类:文本是否含情感知识方法;组合评价单元判断;情感模板识别;基于分类器和分类特征的二元分类任务(词语特征,标点、人称代词、数字特征,基于图);2.主观信息情感分类(句子级、篇章级):基于情感知识、基于特征分类的方法(n-gram词语特征和词性特征、位置特征、评价词特征)。
三、情感信息的检索与归纳1.情感信息检索2.情感信息归纳基于产品属性的情感文摘:识别评论信息中的产品属性,抽取描述产品属性的情感句,判断其倾向性。
基于情感标签的情感文摘:标签可定义为评价搭配形式,建立标签库,相似度聚类的方法聚类得到相似的情感标签,每一类视为潜在的话题(即产品属性)。
基于新闻评论的文摘四、情感分析的评测与资源1.情感分析的评测:TREC,NTCIR的MOAT(新闻观点检测,情感问答,跨语言情感分析),国内的COAE。
情感标注总结
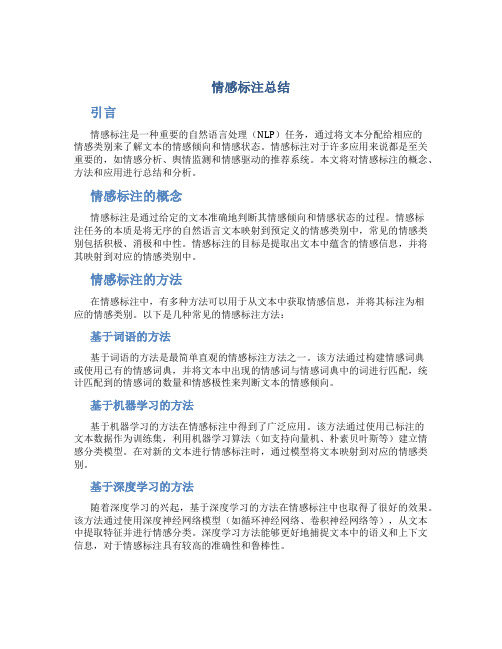
情感标注总结引言情感标注是一种重要的自然语言处理(NLP)任务,通过将文本分配给相应的情感类别来了解文本的情感倾向和情感状态。
情感标注对于许多应用来说都是至关重要的,如情感分析、舆情监测和情感驱动的推荐系统。
本文将对情感标注的概念、方法和应用进行总结和分析。
情感标注的概念情感标注是通过给定的文本准确地判断其情感倾向和情感状态的过程。
情感标注任务的本质是将无序的自然语言文本映射到预定义的情感类别中,常见的情感类别包括积极、消极和中性。
情感标注的目标是提取出文本中蕴含的情感信息,并将其映射到对应的情感类别中。
情感标注的方法在情感标注中,有多种方法可以用于从文本中获取情感信息,并将其标注为相应的情感类别。
以下是几种常见的情感标注方法:基于词语的方法基于词语的方法是最简单直观的情感标注方法之一。
该方法通过构建情感词典或使用已有的情感词典,并将文本中出现的情感词与情感词典中的词进行匹配,统计匹配到的情感词的数量和情感极性来判断文本的情感倾向。
基于机器学习的方法基于机器学习的方法在情感标注中得到了广泛应用。
该方法通过使用已标注的文本数据作为训练集,利用机器学习算法(如支持向量机、朴素贝叶斯等)建立情感分类模型。
在对新的文本进行情感标注时,通过模型将文本映射到对应的情感类别。
基于深度学习的方法随着深度学习的兴起,基于深度学习的方法在情感标注中也取得了很好的效果。
该方法通过使用深度神经网络模型(如循环神经网络、卷积神经网络等),从文本中提取特征并进行情感分类。
深度学习方法能够更好地捕捉文本中的语义和上下文信息,对于情感标注具有较高的准确性和鲁棒性。
情感标注的应用情感标注在许多领域和应用中都起到了重要的作用,以下是几个典型的情感标注应用:情感分析情感分析是情感标注的一种主要应用,通过对文本进行情感标注,可以分析出文本的情感倾向和情感状态。
情感分析广泛应用于舆情分析、产品评论分析、社交媒体情绪分析等领域,能够帮助人们了解用户对产品、事件或话题的情感态度。
本本情感分析技术的核心问题

本本情感分析技术的核心问题
文本情感分类是情感分析领域的核心问题,旨在解决评论情感极性的自动判断问题。
本次使用深度学习技术来解决文本分类问题,主要分为以下两个部分:
归纳总结传统情感分类技术,包括基于字典的方法、基于机器学习的方法、两者混合方法、基于弱标注信息的方法以及基于深度学习的方法;
针对前人情感分类方法的不足,详细介绍所提出的面向情感分类问题的弱监督深度学习框架。
此外,还介绍了评论主题提取相关的经典工作。
最后,总结了情感分类问题的难点和挑战,并对未来的研究工作进行了展望;
有实验对aclImdb电影评价数据集进行深度学习,用pytorch构建和训练基于循环神经网络LSTM的模型,并利用此模型对于给定的电影评论文本进行预测,判断改评论是正面的还是负面的。
试验后准确率高达98%。
- 1、下载文档前请自行甄别文档内容的完整性,平台不提供额外的编辑、内容补充、找答案等附加服务。
- 2、"仅部分预览"的文档,不可在线预览部分如存在完整性等问题,可反馈申请退款(可完整预览的文档不适用该条件!)。
- 3、如文档侵犯您的权益,请联系客服反馈,我们会尽快为您处理(人工客服工作时间:9:00-18:30)。
Vital Filtering(VF)
Features generation
To present the document, we extract 10 features of one document as follows:
number of category in one document; An entity’s first mention place in the document; An entity’s last mention place in the document; length of a document; the cosine similarity of the document and the mean
We use the method of query expansion from VF task directly
The office offered information of co-reference resolution in the data structure
Stream Slot Filling
Challenge & Strategy
Vital Filtering(VF)
Vital Filtering(VF)
Query Expansion
The entity has a DBpedia page we extract keywords from the corresponding DBpedia page as expansion terms
Content
Challenge & Strategy
New Situation and Challenge Strategy
Vital Filtering
System overview Query expansion Features generation Vital classification Result
category profile
Vital Filtering(VF)
Features generation
To present the document, we extract 10 features of one document as follows:
number of target name of an entity; number of redirect name of an entity; number of category of an entity; number of target name in one document; number of redirect name in one document;
value of related documents of an entity
Vital Filtering(VF)
Vital classification
We treat the task as a classify task, so we use three different ways to classify the vital documents:
Preprocessing
Bootstrapping
• Find Seed Pattern • Pattern Learning • Pattern Matching • Pattern Scoring
Stream Slot Filling
Query expansion and co-reference resolution
Support Vector Machine (SVM); we choose Radial Basis Function as kernel function
Random Forest (RF); we set the number of trees is 10
K-Nearest Neighbor (KNN); we make the k=5
Stream Slot Filling
System overview Query expansion and co-reference resolution Pattern learning and matching Result
Q&A?
Challenge & Strategy
Use the training data to learn the models parameters with the ten features as input
Vital Filtering(VF)
Result:
Table 1 The best result with useful + vital
P
R
F
SU
Run 1
0.837
0.789 0.812 0.808
Run 2Байду номын сангаасRun 3 Run 4
0.928
0.772 0.843 0.828
0.916
0.723 0.808 0.793
0.875
0.240 0.377 0.482
Table 2 The best result with vital only
P
R
F
SU
Run 1
0.185
0.907 0.307 0.000
Run 2 Run 3 Run 4
0.201 0.245 0.200
0.879 0.836 0.245
0.328 0.380 0.220
0.000 0.034 0.170
Stream Slot Filling
• Build Index • Query Expansion • Co-reference
query entity
The entity doesn’t have a
DBpedia page we extract Support
keywords from the
docs
wiki
corresponding twitter page as
expansion terms
redirect label