深度学习在图像识别中的研究及应用
基于深度学习的图像识别技术研究与应用
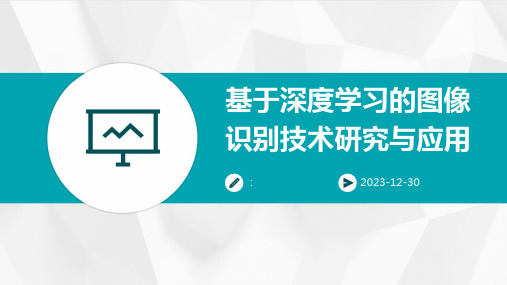
安全监控系统
人脸识别
深度学习算法能够自动识别监控视频中的人脸,实现人脸验证、追 踪和报警等功能。
行为分析
深度学习技术可以自动分析监控视频中的人体行为,如异常行为、 入侵和破坏等,提高安全监控的准确性和实时性。
场景分类
深度学习算法可以通过分析监控视频中的场景信息,自动分类和标记 场景类型,帮助安全监控系统快速响应不同场景的报警信息。
计算资源需求
总结词
深度学习模型需要强大的计算资源进行训练和推理,对硬件设备的要求较高,限制了其 在一些场景下的应用。
详细描述
深度学习模型的训练和推理需要高性能的GPU、TPU等硬件设备支持,而这些设备的成 本较高,使得一些小型企业和研究机构难以承受。此外,对于一些移动设备和嵌入式系 统等场景,由于计算资源的限制,深度学习模型的部署和应用也面临一定的挑战。因此
研究基于深度学习的图像识别技术,有助于提高图像识别的准确率和效率,推动相关行业的智 能化发展,为人们的生活带来更多便利。
图像识别技术的发展历程
传统图像识别方法
深度学习在图像识别中 的应用
深度学习模型的优化
基于特征提取和分类器的图像识别方 法,如SIFT、SURF等。这种方法需 要人工设计特征提取算法和分类器, 计算量大且效果不稳定。
基于深度学习的图像识别技
04
术应用
医学影像分析
医学影像诊断
深度学习算法能够自动识别和分析医学影像,如X光片、CT和 MRI图像,辅助医生进行疾病诊断。
病理特征提取
深度学习技术可以自动提取病理特征,如肿瘤大小、形态和位置, 为医生提供更准确和客观的病理分析依据。
药物研发
深度学习算法可以通过分析医学影像数据,预测药物对人体的作用 和效果,加速新药研发进程。
深度学习在图像识别中的应用探讨
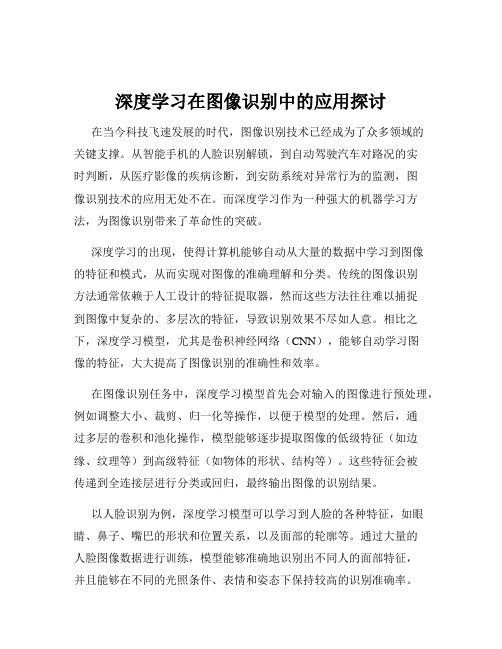
深度学习在图像识别中的应用探讨在当今科技飞速发展的时代,图像识别技术已经成为了众多领域的关键支撑。
从智能手机的人脸识别解锁,到自动驾驶汽车对路况的实时判断,从医疗影像的疾病诊断,到安防系统对异常行为的监测,图像识别技术的应用无处不在。
而深度学习作为一种强大的机器学习方法,为图像识别带来了革命性的突破。
深度学习的出现,使得计算机能够自动从大量的数据中学习到图像的特征和模式,从而实现对图像的准确理解和分类。
传统的图像识别方法通常依赖于人工设计的特征提取器,然而这些方法往往难以捕捉到图像中复杂的、多层次的特征,导致识别效果不尽如人意。
相比之下,深度学习模型,尤其是卷积神经网络(CNN),能够自动学习图像的特征,大大提高了图像识别的准确性和效率。
在图像识别任务中,深度学习模型首先会对输入的图像进行预处理,例如调整大小、裁剪、归一化等操作,以便于模型的处理。
然后,通过多层的卷积和池化操作,模型能够逐步提取图像的低级特征(如边缘、纹理等)到高级特征(如物体的形状、结构等)。
这些特征会被传递到全连接层进行分类或回归,最终输出图像的识别结果。
以人脸识别为例,深度学习模型可以学习到人脸的各种特征,如眼睛、鼻子、嘴巴的形状和位置关系,以及面部的轮廓等。
通过大量的人脸图像数据进行训练,模型能够准确地识别出不同人的面部特征,并且能够在不同的光照条件、表情和姿态下保持较高的识别准确率。
在医疗领域,深度学习在图像识别方面的应用也取得了显著的成果。
例如,在医学影像诊断中,深度学习模型可以对 X 光、CT、MRI 等图像进行分析,帮助医生快速检测出病变区域。
对于肿瘤的识别和诊断,深度学习模型能够通过分析肿瘤的形态、大小、位置等特征,提供准确的诊断结果,为医生的治疗决策提供有力的支持。
在自动驾驶领域,图像识别技术更是至关重要。
车辆需要实时识别道路上的各种物体,如行人、车辆、交通标志等,以便做出正确的驾驶决策。
深度学习模型能够快速处理车载摄像头拍摄的图像,准确识别出各种物体,并预测它们的运动轨迹,从而保障驾驶的安全。
基于深度学习的图像识别技术研究及应用案例

基于深度学习的图像识别技术研究及应用案例图像识别技术在当代社会中扮演了重要的角色,而基于深度学习的图像识别技术则是目前最先进和最有效的方法之一。
本文将探讨深度学习在图像识别领域的研究和应用案例,并着重介绍了几个成功的实例。
深度学习是一种利用多层神经网络进行学习和训练的机器学习方法。
在图像识别领域,深度学习通过构建具有多个隐层的卷积神经网络(CNN)来模拟人类视觉系统的工作原理。
这些神经网络能够从原始图像数据中自动学习特征,并用于图像分类、目标检测、物体识别等任务。
一项成功的基于深度学习的图像识别技术研究案例是人脸识别。
人脸识别是指通过计算机技术对图像或视频中的人脸进行自动识别和识别的过程。
基于深度学习的人脸识别技术已取得了巨大突破,具有更高的准确性和鲁棒性。
例如,FaceNet是一种基于CNN的人脸识别系统,它能够从大量的人脸图像中学习人脸的特征表示,并能够在不同角度、光照条件和姿势下准确地进行人脸匹配。
另一个基于深度学习的图像识别技术应用案例是自动驾驶。
近年来,自动驾驶技术成为汽车行业的研究热点。
深度学习在自动驾驶领域的应用主要集中在目标检测和场景理解方面。
通过深度学习训练的卷积神经网络可以识别和跟踪道路上的车辆、行人和交通标志,并将这些信息用于自动驾驶决策。
例如,Google的自动驾驶汽车使用了一种名为Inception的CNN模型来检测和识别道路上的不同交通参与者,以实现安全驾驶。
此外,基于深度学习的图像识别技术还可以应用于医学影像诊断。
医学影像诊断是一项重要的医疗工作,但由于影像数据复杂、数量庞大,传统的人工诊断方法存在一定的局限性。
深度学习可以从医学影像数据中自动学习疾病特征,提供更准确和可靠的诊断结果。
例如,基于CNN的深度学习模型已被应用于肺部结节识别和乳腺癌检测等任务中,取得了与经验丰富的医生相媲美的诊断准确率。
总之,基于深度学习的图像识别技术在各个领域都有广泛的应用。
无论是人脸识别、自动驾驶还是医学影像诊断,深度学习都展现出了巨大的潜力和优势。
深度学习在图像识别中的应用研究

深度学习在图像识别中的应用研究在当今科技飞速发展的时代,图像识别技术已经成为了一项至关重要的技术,广泛应用于各个领域,如医疗诊断、安防监控、自动驾驶、工业检测等等。
而深度学习作为一种强大的机器学习方法,为图像识别带来了革命性的突破。
深度学习,简单来说,就是一种通过构建多层神经网络来自动学习数据特征的方法。
在图像识别中,深度学习模型能够从大量的图像数据中学习到不同层次的特征表示,从而实现对图像的准确分类和识别。
传统的图像识别方法通常依赖于人工设计的特征提取器,如边缘检测、纹理分析等。
然而,这些方法往往存在着局限性,难以捕捉到图像中复杂的语义信息。
深度学习则克服了这一难题,它能够自动地从原始图像中学习到具有代表性的特征,大大提高了图像识别的准确性和泛化能力。
深度学习在图像识别中的应用非常广泛。
在医疗领域,它可以帮助医生快速准确地诊断疾病。
例如,通过对医学影像(如 X 光、CT 扫描、MRI 等)的分析,深度学习模型能够检测出肿瘤、骨折等异常情况。
这不仅提高了诊断的效率,还为患者提供了更早的治疗机会。
在安防监控方面,深度学习技术使得监控系统能够更加智能地识别和分析人物和场景。
它可以实时检测出可疑人员、异常行为,为保障公共安全发挥了重要作用。
比如,在机场、火车站等人员密集的场所,通过对摄像头拍摄的图像进行实时分析,能够及时发现潜在的安全威胁。
自动驾驶是另一个深度学习在图像识别中大展身手的领域。
车辆上的摄像头可以实时获取道路、车辆、行人等信息,深度学习模型能够对这些图像进行快速处理和分析,从而帮助车辆做出准确的决策,如加速、减速、转向等,提高驾驶的安全性和舒适性。
在工业检测中,深度学习也发挥着重要作用。
它可以对产品的外观进行检测,快速准确地识别出缺陷产品,提高生产效率和产品质量。
为了实现深度学习在图像识别中的应用,通常需要经过以下几个步骤:首先是数据收集,需要收集大量的相关图像数据,并对其进行标注,以告诉模型哪些是正确的分类和识别结果。
基于深度学习的图像识别技术研究与应用
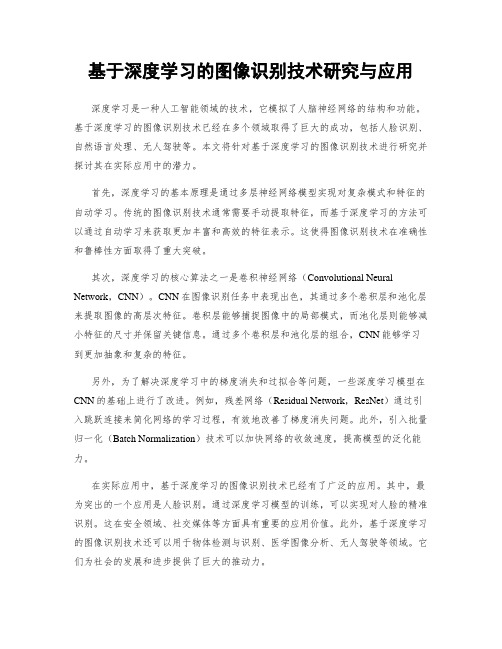
基于深度学习的图像识别技术研究与应用深度学习是一种人工智能领域的技术,它模拟了人脑神经网络的结构和功能。
基于深度学习的图像识别技术已经在多个领域取得了巨大的成功,包括人脸识别、自然语言处理、无人驾驶等。
本文将针对基于深度学习的图像识别技术进行研究并探讨其在实际应用中的潜力。
首先,深度学习的基本原理是通过多层神经网络模型实现对复杂模式和特征的自动学习。
传统的图像识别技术通常需要手动提取特征,而基于深度学习的方法可以通过自动学习来获取更加丰富和高效的特征表示。
这使得图像识别技术在准确性和鲁棒性方面取得了重大突破。
其次,深度学习的核心算法之一是卷积神经网络(Convolutional Neural Network,CNN)。
CNN在图像识别任务中表现出色,其通过多个卷积层和池化层来提取图像的高层次特征。
卷积层能够捕捉图像中的局部模式,而池化层则能够减小特征的尺寸并保留关键信息。
通过多个卷积层和池化层的组合,CNN能够学习到更加抽象和复杂的特征。
另外,为了解决深度学习中的梯度消失和过拟合等问题,一些深度学习模型在CNN的基础上进行了改进。
例如,残差网络(Residual Network,ResNet)通过引入跳跃连接来简化网络的学习过程,有效地改善了梯度消失问题。
此外,引入批量归一化(Batch Normalization)技术可以加快网络的收敛速度,提高模型的泛化能力。
在实际应用中,基于深度学习的图像识别技术已经有了广泛的应用。
其中,最为突出的一个应用是人脸识别。
通过深度学习模型的训练,可以实现对人脸的精准识别。
这在安全领域、社交媒体等方面具有重要的应用价值。
此外,基于深度学习的图像识别技术还可以用于物体检测与识别、医学图像分析、无人驾驶等领域。
它们为社会的发展和进步提供了巨大的推动力。
然而,基于深度学习的图像识别技术也面临一些挑战。
首先,深度学习模型需要大量的标记数据用于训练,而获取大规模的标记数据是非常困难的。
深度学习在图像识别领域的应用案例分析
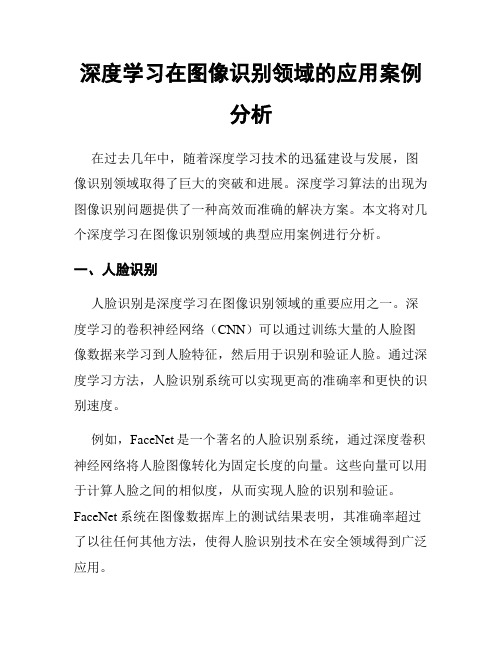
深度学习在图像识别领域的应用案例分析在过去几年中,随着深度学习技术的迅猛建设与发展,图像识别领域取得了巨大的突破和进展。
深度学习算法的出现为图像识别问题提供了一种高效而准确的解决方案。
本文将对几个深度学习在图像识别领域的典型应用案例进行分析。
一、人脸识别人脸识别是深度学习在图像识别领域的重要应用之一。
深度学习的卷积神经网络(CNN)可以通过训练大量的人脸图像数据来学习到人脸特征,然后用于识别和验证人脸。
通过深度学习方法,人脸识别系统可以实现更高的准确率和更快的识别速度。
例如,FaceNet是一个著名的人脸识别系统,通过深度卷积神经网络将人脸图像转化为固定长度的向量。
这些向量可以用于计算人脸之间的相似度,从而实现人脸的识别和验证。
FaceNet系统在图像数据库上的测试结果表明,其准确率超过了以往任何其他方法,使得人脸识别技术在安全领域得到广泛应用。
二、自动驾驶深度学习技术在自动驾驶领域的应用也取得了显著的进展。
通过利用深度神经网络对道路图像进行处理和分析,自动驾驶车辆可以实现对交通标志的识别、车道线的检测以及前方障碍物的感知。
以特斯拉公司为例,他们利用深度学习技术开发了自动驾驶系统。
该系统通过卷积神经网络对道路图像进行处理,并能够准确地检测并识别道路上的交通标志和车辆。
同时,深度学习算法还能够实时地分析和预测交通场景,帮助车辆做出相应的行驶决策,提高行车安全性和驾驶舒适度。
三、医学影像识别深度学习在医学影像识别领域也有着广泛的应用。
通过对医学影像数据进行深度学习训练,可以实现对疾病的早期诊断和预测。
例如,针对乳腺癌的检测,研究人员利用深度学习方法开发了一种自动识别的算法。
该算法可以对乳腺X光摄影图像进行分析,并准确地检测和识别出潜在的乳腺肿瘤。
相比传统的人工判断方法,深度学习算法在识别准确率和效率上都取得了显著的提升。
四、工业质检深度学习技术在工业质检领域的应用也越来越广泛。
通过使用深度学习算法,可以对传感器采集到的工业产品图像进行分析和识别,实现自动化的质量检测。
深度学习算法在图像识别中的研究及应用
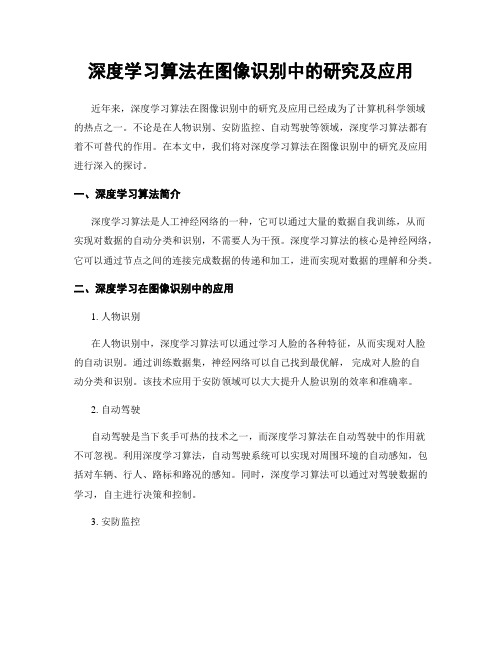
深度学习算法在图像识别中的研究及应用近年来,深度学习算法在图像识别中的研究及应用已经成为了计算机科学领域的热点之一。
不论是在人物识别、安防监控、自动驾驶等领域,深度学习算法都有着不可替代的作用。
在本文中,我们将对深度学习算法在图像识别中的研究及应用进行深入的探讨。
一、深度学习算法简介深度学习算法是人工神经网络的一种,它可以通过大量的数据自我训练,从而实现对数据的自动分类和识别,不需要人为干预。
深度学习算法的核心是神经网络,它可以通过节点之间的连接完成数据的传递和加工,进而实现对数据的理解和分类。
二、深度学习在图像识别中的应用1. 人物识别在人物识别中,深度学习算法可以通过学习人脸的各种特征,从而实现对人脸的自动识别。
通过训练数据集,神经网络可以自己找到最优解,完成对人脸的自动分类和识别。
该技术应用于安防领域可以大大提升人脸识别的效率和准确率。
2. 自动驾驶自动驾驶是当下炙手可热的技术之一,而深度学习算法在自动驾驶中的作用就不可忽视。
利用深度学习算法,自动驾驶系统可以实现对周围环境的自动感知,包括对车辆、行人、路标和路况的感知。
同时,深度学习算法可以通过对驾驶数据的学习,自主进行决策和控制。
3. 安防监控在安防监控领域,深度学习算法可以实现对视频监控画面的自动识别。
当出现重要事件时,系统可以自动发出警报并进行响应。
深度学习算法的应用可以极大地提升监控效率和准确率,减轻人工监控的负担。
三、深度学习在图像识别中的研究1. 深度卷积神经网络深度卷积神经网络是目前图像识别中最为常用的一种深度学习算法。
其核心是卷积层和池化层,可以通过对图像的特征提取和下采样完成图像的分类和识别。
2. 生成对抗网络生成对抗网络是一种将深度学习算法和博弈论相结合的新颖技术。
生成对抗网络是由两个神经网络互相博弈的过程,其中生成网络利用深度学习算法生成逼真的图像,判别网络来判断生成网络生成的图像是否真实。
3. 转移学习转移学习是指在一个任务上训练出的深度学习算法,可以通过调整部分参数,用于另一个任务上。
深度学习在图像识别中的应用与优势
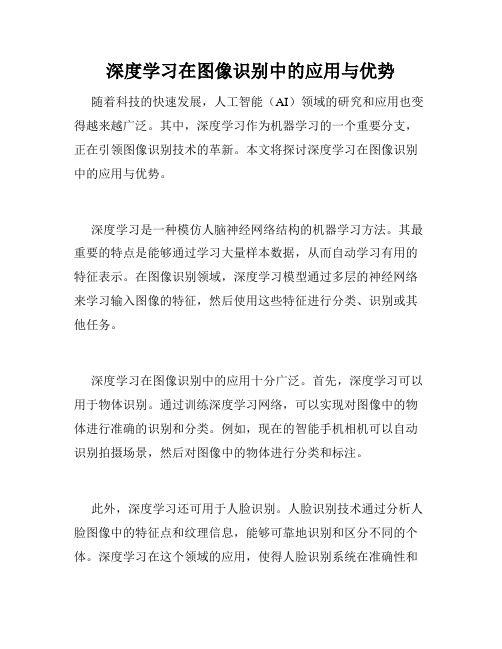
深度学习在图像识别中的应用与优势随着科技的快速发展,人工智能(AI)领域的研究和应用也变得越来越广泛。
其中,深度学习作为机器学习的一个重要分支,正在引领图像识别技术的革新。
本文将探讨深度学习在图像识别中的应用与优势。
深度学习是一种模仿人脑神经网络结构的机器学习方法。
其最重要的特点是能够通过学习大量样本数据,从而自动学习有用的特征表示。
在图像识别领域,深度学习模型通过多层的神经网络来学习输入图像的特征,然后使用这些特征进行分类、识别或其他任务。
深度学习在图像识别中的应用十分广泛。
首先,深度学习可以用于物体识别。
通过训练深度学习网络,可以实现对图像中的物体进行准确的识别和分类。
例如,现在的智能手机相机可以自动识别拍摄场景,然后对图像中的物体进行分类和标注。
此外,深度学习还可用于人脸识别。
人脸识别技术通过分析人脸图像中的特征点和纹理信息,能够可靠地识别和区分不同的个体。
深度学习在这个领域的应用,使得人脸识别系统在准确性和鲁棒性方面取得了重大突破。
现在,人脸识别已经广泛应用于手机解锁、安全监控等场景。
另外,深度学习还可以用于图像分割和语义分析等任务。
图像分割是将图像分成若干个区域,每个区域内具有相似的特征。
通过深度学习模型,可以实现对图像中不同区域的准确分割,从而实现图像内容的理解和解释。
语义分析则是根据图像中的内容,推断出图像所表达的语义信息。
深度学习模型可以自动学习图像中不同物体的特征和上下文关系,从而对图像进行语义分析和理解。
深度学习在图像识别中的应用具有许多优势。
首先,相对于传统的机器学习方法,深度学习不需要手动提取特征。
传统方法通常需要人工选择和设计一些特征,而这个过程往往非常复杂和耗时。
而深度学习通过自动学习特征表示,减轻了人工特征提取的负担,提高了图像识别的准确性和效率。
其次,深度学习可以更好地处理大规模数据。
随着互联网的发展,图像数据量呈指数级增长。
深度学习模型由于其强大的表达能力和大规模并行计算的能力,能够处理海量的图像数据,并从中学习到有用的特征表示。
基于深度学习的图像识别与分类技术研究与应用

基于深度学习的图像识别与分类技术研究与应用摘要:图像识别与分类技术是计算机视觉领域的一个重要研究方向,而深度学习作为一种强大的机器学习方法,已经在图像识别与分类领域取得了许多突破性的进展。
本文通过综述相关文献和最新研究成果,探讨了基于深度学习的图像识别与分类技术的研究和应用。
1. 引言随着互联网的发展和智能设备的普及,图像数据的规模呈现爆炸性增长。
如何从这些海量的图像数据中高效准确地识别和分类图像,成为了一个具有挑战性的问题。
传统的图像识别与分类方法往往需要手动提取特征,并且面临着溢出、数据稀疏和高维的问题。
因此,基于深度学习的图像识别与分类技术应运而生。
2. 深度学习技术概述深度学习是一种模仿人脑神经网络结构的机器学习方法,其核心思想是通过多层次的非线性变换来提取高层次的特征表示。
与传统的浅层神经网络相比,深度学习模型具有更强的表达能力和泛化能力,能够自动学习特征表示。
3. 基于深度学习的图像识别与分类技术基于深度学习的图像识别与分类技术主要包括以下几个环节:3.1 数据预处理在深度学习任务中,数据预处理是非常重要的一步。
常见的预处理方法包括图像尺寸调整、灰度化、增强对比度、标准化等。
这些预处理方法可以提高图像的质量,有利于提高模型的性能和效果。
3.2 特征提取深度学习模型通过多层的卷积层和池化层来提取图像的特征。
卷积层可以学习到图像的低层次特征,而池化层可以进一步提取图像的高层次特征。
模型中的卷积核可以自动学习图像的纹理和形状等特征。
3.3 模型训练与优化深度学习模型通常使用大量的图像数据进行训练。
在训练过程中,通过反向传播算法来更新模型的参数,使其能够更好地拟合训练数据。
为了提高模型的泛化能力,通常还会采用正则化技术和dropout技术等方法进行模型优化。
3.4 图像识别与分类在模型训练完成后,可以利用该模型对新的图像数据进行识别和分类。
通过输入一张图像,模型会输出该图像所属的类别标签,并给出一个概率值作为置信度。
基于深度学习的图像识别技术研究与应用

基于深度学习的图像识别技术研究与应用摘要:图像识别技术是计算机视觉领域的研究热点之一。
随着深度学习算法的发展,基于深度学习的图像识别技术在各个领域得到了广泛应用。
本文首先介绍了深度学习算法的基本原理和常用模型,然后详细探讨了基于深度学习的图像识别技术的研究现状和方法,并通过具体案例分析了其在实际应用中的表现和效果。
最后,对基于深度学习的图像识别技术进行了总结,并对未来的发展进行了展望。
1. 引言1.1 研究背景与意义1.2 国内外研究现状2. 深度学习算法基本原理2.1 神经网络2.2 深度学习中常用的网络模型2.2.1 卷积神经网络(CNN)2.2.2 循环神经网络(RNN)2.2.3 生成对抗网络(GAN)2.2.4 注意力机制网络(Attention)3. 基于深度学习的图像识别技术研究现状3.1 图像分类3.1.1 基于深度学习的图像分类方法3.1.2 深度学习模型的训练与优化3.2 目标检测3.2.1 基于深度学习的目标检测方法3.2.2 多尺度目标检测与跟踪3.3 图像分割3.3.1 基于深度学习的图像分割方法3.3.2 实例分割与语义分割的研究进展4. 基于深度学习的图像识别技术应用案例分析4.1 人脸识别技术的应用4.1.1 人脸识别在安全领域的应用4.1.2 人脸识别在社交媒体中的应用4.2 物体识别技术的应用4.2.1 物体识别在智能交通中的应用4.2.2 物体识别在无人驾驶领域的应用5. 基于深度学习的图像识别技术的挑战与未来发展5.1 数据集与标注问题5.2 计算资源与算法性能问题5.3 特殊场景下的识别问题5.4 可解释性与隐私保护问题6. 结论6.1 研究总结6.2 技术展望在本课题报告中,我们详细研究了基于深度学习的图像识别技术的研究现状和应用方向,对其在图像分类、目标检测和图像分割等任务中的表现进行了深入分析。
同时,我们通过具体案例分析了基于深度学习的图像识别技术在人脸识别和物体识别领域的应用。
深度学习在图像识别中的研究与应用

深度学习在图像识别中的研究与应用一、引言随着科技的不断发展,图像识别技术越来越成熟。
深度学习作为人工智能的一个重要分支,在图像识别领域发挥着重要作用。
本文将详细介绍深度学习在图像识别中的研究和应用。
二、深度学习的概述深度学习是一种机器学习的方法,它模仿人类大脑的神经网络结构,通过多层模型对数据进行特征提取和转换,最终实现预测和分类任务。
深度学习在图像识别中的优势在于它可以自动学习多层次的抽象特征,并通过大量的数据进行模型的训练,从而提高图像识别的准确性和稳定性。
三、深度学习在图像分类中的应用1.卷积神经网络(CNN)卷积神经网络是深度学习在图像分类中应用最为广泛的模型之一。
它通过卷积层和池化层对图像进行特征提取,然后通过全连接层实现分类。
卷积神经网络具有良好的局部特征提取能力,可以捕捉图像中的细节信息,广泛应用于人脸识别、物体检测和图像分割等领域。
2.循环神经网络(RNN)循环神经网络是一种适用于序列数据处理的深度学习模型,对于图像分类中的序列问题(例如识别手写数字),循环神经网络具有良好的效果。
循环神经网络通过隐藏层之间的反馈连接,能够记忆之前的状态,从而更好地利用序列数据的上下文信息。
四、深度学习在目标检测中的应用1.区域卷积神经网络(R-CNN)R-CNN是一种先进的目标检测算法,它是结合了卷积神经网络和区域建议网络(RPN)的模型。
R-CNN首先通过RPN生成候选框,然后使用卷积神经网络提取每个候选框的特征,最后通过支持向量机(SVM)进行分类和边界框回归。
R-CNN在目标检测领域取得了良好的性能。
2.单阶段目标检测神经网络(YOLO)YOLO是一种快速而有效的目标检测算法,它使用单个神经网络直接从图像中预测候选框和类别概率。
YOLO将目标检测任务转化为一个回归问题,通过卷积神经网络实现目标位置的定位和分类。
YOLO在速度和准确性上都表现出色,广泛应用于实时目标检测和无人驾驶等领域。
五、深度学习在图像分割中的应用图像分割是将图像中的像素进行分类的任务,深度学习也在这一领域取得了重大突破。
深度学习技术在像识别中的应用与优势

深度学习技术在像识别中的应用与优势深度学习技术在图像识别中的应用与优势深度学习技术是近年来在人工智能领域取得突破的重要技术之一,其在图像识别方面应用广泛且表现出众的优势。
本文将介绍深度学习技术在图像识别中的应用领域,探讨其优势,并讨论未来发展趋势。
一、深度学习技术在图像识别中的应用领域在当今社会中,图像识别已经渗透到了诸多领域,如人脸识别、物体检测、医学影像分析、自动驾驶等。
而深度学习技术作为图像识别的重要手段,为这些领域带来了革命性的突破。
1. 人脸识别深度学习技术在人脸识别领域表现出色。
基于深度学习的人脸识别算法能够高效准确地检测和识别人脸,实时判断身份信息,并广泛应用于安防监控、人员考勤、手机解锁等场景中。
2. 物体检测与识别传统的物体检测算法需要人工提取特征并设计复杂的分类器,而基于深度学习的物体检测算法可以实现端到端的训练,不需要手动设计特征。
这使得物体检测与识别更加高效准确,在无人驾驶、智能零售、智能监控等领域得到广泛应用。
3. 医学影像分析深度学习技术在医学影像分析领域有着广泛的应用,如肺部图像的肺结节检测和分类、乳腺癌图像的良恶性识别等。
这些应用大大提高了疾病的早期诊断和治疗效果,为医疗行业带来了巨大的改变。
二、深度学习技术在图像识别中的优势相比于传统的图像识别算法,深度学习技术具有以下优势:1. 自动特征提取传统算法需要手动提取图像的特征,而深度学习算法通过多层神经网络自动提取图像中的特征。
这种自动化的特征提取能力大大简化了算法的设计和实现过程,同时也提高了识别的准确性。
2. 可扩展性深度学习技术具有良好的扩展性,能够应对大规模的图像识别任务。
随着训练数据集的增大,深度学习模型可以更好地学习到数据中的特征,并提高图像识别的准确率。
这使得深度学习技术在大规模图像处理和分析中具备较高的应用潜力。
3. 鲁棒性深度学习技术对于光照、角度、噪声等环境变化具有一定的鲁棒性。
在实际应用中,深度学习模型的泛化能力较强,能够较好地应对各种复杂的图像场景。
基于深度学习的图像识别算法优化研究及应用
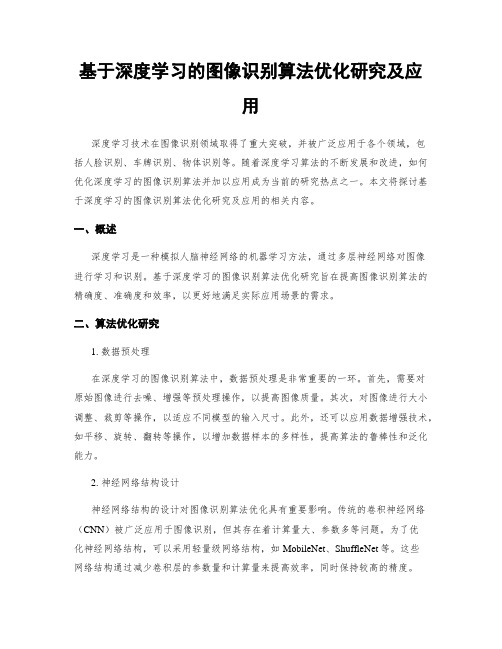
基于深度学习的图像识别算法优化研究及应用深度学习技术在图像识别领域取得了重大突破,并被广泛应用于各个领域,包括人脸识别、车牌识别、物体识别等。
随着深度学习算法的不断发展和改进,如何优化深度学习的图像识别算法并加以应用成为当前的研究热点之一。
本文将探讨基于深度学习的图像识别算法优化研究及应用的相关内容。
一、概述深度学习是一种模拟人脑神经网络的机器学习方法,通过多层神经网络对图像进行学习和识别。
基于深度学习的图像识别算法优化研究旨在提高图像识别算法的精确度、准确度和效率,以更好地满足实际应用场景的需求。
二、算法优化研究1. 数据预处理在深度学习的图像识别算法中,数据预处理是非常重要的一环。
首先,需要对原始图像进行去噪、增强等预处理操作,以提高图像质量。
其次,对图像进行大小调整、裁剪等操作,以适应不同模型的输入尺寸。
此外,还可以应用数据增强技术,如平移、旋转、翻转等操作,以增加数据样本的多样性,提高算法的鲁棒性和泛化能力。
2. 神经网络结构设计神经网络结构的设计对图像识别算法优化具有重要影响。
传统的卷积神经网络(CNN)被广泛应用于图像识别,但其存在着计算量大、参数多等问题。
为了优化神经网络结构,可以采用轻量级网络结构,如MobileNet、ShuffleNet等。
这些网络结构通过减少卷积层的参数量和计算量来提高效率,同时保持较高的精度。
3. 损失函数优化损失函数是衡量模型对输入图像进行分类的准确度的指标。
优化损失函数可以进一步提高图像识别算法的精确度和准确度。
常用的损失函数优化方法包括交叉熵损失函数、平方损失函数、极大似然估计等。
此外,可以结合正则化技术,如L1正则化、L2正则化等,以降低模型的过拟合风险。
4. 梯度优化算法梯度优化算法对深度学习的图像识别算法优化至关重要。
传统的梯度下降算法存在着局部最优解问题和收敛速度慢等缺点。
为了提高算法的效率和准确度,可以采用自适应学习率算法,如Adam、RMSProp等,以自动调整学习率,加快模型的收敛速度。
深度学习在图像识别领域的应用研究
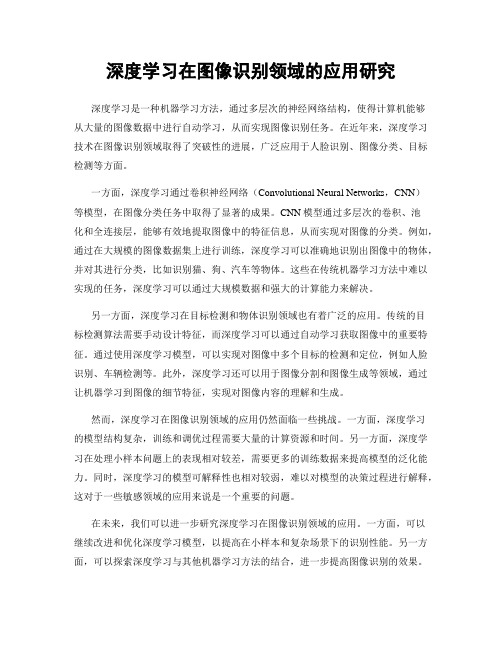
深度学习在图像识别领域的应用研究深度学习是一种机器学习方法,通过多层次的神经网络结构,使得计算机能够从大量的图像数据中进行自动学习,从而实现图像识别任务。
在近年来,深度学习技术在图像识别领域取得了突破性的进展,广泛应用于人脸识别、图像分类、目标检测等方面。
一方面,深度学习通过卷积神经网络(Convolutional Neural Networks,CNN)等模型,在图像分类任务中取得了显著的成果。
CNN模型通过多层次的卷积、池化和全连接层,能够有效地提取图像中的特征信息,从而实现对图像的分类。
例如,通过在大规模的图像数据集上进行训练,深度学习可以准确地识别出图像中的物体,并对其进行分类,比如识别猫、狗、汽车等物体。
这些在传统机器学习方法中难以实现的任务,深度学习可以通过大规模数据和强大的计算能力来解决。
另一方面,深度学习在目标检测和物体识别领域也有着广泛的应用。
传统的目标检测算法需要手动设计特征,而深度学习可以通过自动学习获取图像中的重要特征。
通过使用深度学习模型,可以实现对图像中多个目标的检测和定位,例如人脸识别、车辆检测等。
此外,深度学习还可以用于图像分割和图像生成等领域,通过让机器学习到图像的细节特征,实现对图像内容的理解和生成。
然而,深度学习在图像识别领域的应用仍然面临一些挑战。
一方面,深度学习的模型结构复杂,训练和调优过程需要大量的计算资源和时间。
另一方面,深度学习在处理小样本问题上的表现相对较差,需要更多的训练数据来提高模型的泛化能力。
同时,深度学习的模型可解释性也相对较弱,难以对模型的决策过程进行解释,这对于一些敏感领域的应用来说是一个重要的问题。
在未来,我们可以进一步研究深度学习在图像识别领域的应用。
一方面,可以继续改进和优化深度学习模型,以提高在小样本和复杂场景下的识别性能。
另一方面,可以探索深度学习与其他机器学习方法的结合,进一步提高图像识别的效果。
此外,还可以研究针对深度学习模型的可解释性问题,以增加对模型输出的理解和可信度。
深度学习在图像识别中的应用研究

深度学习在图像识别中的应用研究一、引言近年来,随着科技的不断发展和深入,机器学习领域的研究也日趋深入,深度学习作为机器学习领域的一部分,被广泛应用于图像识别、自然语言处理、语音识别等多个领域。
在图像识别方面,如何快速准确地对图像进行分类识别一直是学术和产业界关注的焦点。
本文针对深度学习在图像识别中的应用进行研究和探讨。
二、深度学习深度学习是机器学习和人工神经网络领域的一种方法,其主要原理是通过对数据进行监督学习和无监督学习,从而得到高层次的抽象特征,并利用这些特征来进行模式识别和分类任务。
深度学习模型通常包括多个层次,更深的层次会根据之前的层次在进行更高层次的特征提取和学习,最终形成具有高度抽象特征的表示。
常用的深度学习网络结构包括卷积神经网络(CNN)、循环神经网络(RNN)和深度置信网络(DBN)等。
三、图像识别图像识别是利用计算机技术对图像进行自动分类和识别的过程。
传统的图像识别算法主要是基于手动设计的特征提取算法,如SIFT、SURF等算法,但这些传统算法通常需要经过长时间的手动调参和特征选择,无法很好地适应多样的数据分布和复杂的场景环境。
近年来,随着深度学习方法的兴起,尤其是卷积神经网络的成功应用,图像识别领域发生了天翻地覆的变化,神经网络可以将手工设计的特征提取算法自动化,并对于之前难以分类的新型数据集具有很好的识别能力,成为当前图像识别领域的主流算法。
四、深度学习在图像分类中的应用(一)卷积神经网络(CNN)CNN是深度学习中最常见的图像识别算法,其主要思想是利用卷积核对输入图像进行卷积提取特征,在经过多个卷积层和池化层的处理后,利用全连接层输出分类结果。
CNN最大的优势在于它能够自动提取适合分类任务的最佳特征,使得在不同的应用场景中都可以得到可靠的分类结果。
LeNet-5是CNN最早的经典模型之一,它是由Yann LeCun等人于1998年提出的,以MNIST手写数字图片为数据集进行训练。
深度学习在图像识别中的应用研究

深度学习在图像识别中的应用研究在当今的科技领域,深度学习在图像识别方面的应用取得了令人瞩目的成就。
图像识别作为计算机视觉的重要组成部分,其在众多领域都发挥着关键作用,从医疗诊断到自动驾驶,从安防监控到工业生产,无处不在。
深度学习之所以能够在图像识别中大放异彩,主要得益于其强大的特征学习能力。
传统的图像识别方法往往依赖于人工设计的特征提取器,然而这些方法在面对复杂多变的图像数据时往往显得力不从心。
深度学习模型,特别是卷积神经网络(CNN),能够自动从大量的图像数据中学习到具有代表性和判别性的特征。
在医疗领域,深度学习的图像识别技术为疾病的诊断带来了新的可能。
例如,通过对 X 光片、CT 扫描图像和病理切片等的分析,深度学习模型可以帮助医生更准确地检测出肿瘤、骨折等病变。
以肺癌的诊断为例,深度学习模型可以对肺部 CT 图像中的微小结节进行检测和分类,其准确性甚至能够超越经验丰富的医生。
这不仅提高了诊断的效率,还为患者争取了宝贵的治疗时间。
在自动驾驶领域,图像识别是确保车辆安全行驶的核心技术之一。
车辆需要实时识别道路上的各种物体,如行人、车辆、交通标志和障碍物等。
深度学习模型能够快速处理来自车载摄像头的图像数据,并做出准确的判断和决策。
例如,当车辆检测到前方有行人时,系统会立即发出警报并采取制动措施,从而避免碰撞事故的发生。
此外,深度学习还可以用于预测车辆的行驶轨迹和周围物体的运动趋势,为自动驾驶提供更可靠的保障。
在安防监控领域,深度学习的图像识别技术可以实现对人员和物体的实时监测和追踪。
通过对监控摄像头拍摄的图像进行分析,系统可以快速识别出可疑人员和异常行为,并及时发出警报。
这对于保障公共安全和防范犯罪具有重要意义。
例如,在机场、火车站等人员密集的场所,深度学习系统可以对旅客的面部进行识别,快速筛选出潜在的危险分子。
工业生产中,图像识别也有着广泛的应用。
在质量检测环节,深度学习模型可以对产品的外观进行检测,识别出表面的瑕疵和缺陷。
深度学习技术在图像识别中的应用

深度学习技术在图像识别中的应用图像识别是人工智能领域的一个重要研究方向,而深度学习技术在图像识别中的应用广泛而深入。
深度学习是一种基于人工神经网络的机器学习方法,具有高效、准确和自动化的特点,被广泛应用于图像识别任务中。
1. 深度学习在物体识别中的应用物体识别是图像识别的一个重要方面,深度学习技术在物体识别中的应用得到了广泛关注和研究。
深度学习模型可以通过学习大量的图像样本来自动提取物体的特征,并根据这些特征进行分类识别。
通过构建深层神经网络,模型可以对物体进行高精度的识别,例如在图像分类任务中,可以识别出狗、猫、飞机等各种不同的物体。
2. 深度学习在人脸识别中的应用人脸识别是图像识别中的一个热门领域,深度学习技术在人脸识别中的应用取得了巨大的突破。
利用深度学习模型可以从图像中提取出人脸的关键特征,并将其转化为计算机可以理解的向量表示。
基于这些向量表示,深度学习模型可以进行人脸的匹配和识别,从而实现人脸检测、身份验证等应用。
例如,深度学习技术在安全领域的应用能够实现从大量监控视频中准确识别出特定的人脸。
3. 深度学习在图像分割中的应用图像分割是将图像中的像素分成不同的区域,每个区域具有相似的特征或属性的任务。
深度学习技术在图像分割中的应用可以更准确地分割图像,并且具有较高的效率。
通过构建卷积神经网络(CNN),可以将图像中的每个像素都与周围的像素进行关联,并通过多层次的网络结构进行特征提取和分类。
深度学习模型可以学习到图像的上下文信息,从而实现更准确的图像分割。
4. 深度学习在目标检测中的应用目标检测是在图像中定位和识别出特定目标的任务,深度学习技术在目标检测中取得了显著的效果。
深度学习模型可以通过学习大量标注好的图像样本,自动提取图像中的目标特征,并利用这些特征进行目标的定位和识别。
通过使用卷积神经网络和多层感知机等深度学习模型,可以在实时性能不受太大影响的情况下实现高精度的目标检测。
总结:深度学习技术在图像识别中的应用已经取得了巨大的突破,使得图像识别更加准确、高效和自动化。
深度学习算法在像识别中的应用调研报告

深度学习算法在像识别中的应用调研报告深度学习算法在图像识别中的应用调研报告在当今科技飞速发展的时代,图像识别技术已经成为了一项至关重要的技术,广泛应用于各个领域。
深度学习算法作为一种强大的工具,为图像识别带来了显著的突破和进步。
本调研报告将深入探讨深度学习算法在图像识别中的应用情况。
一、深度学习算法概述深度学习是一种基于人工神经网络的机器学习方法,它通过构建多层的神经网络模型,自动从大量的数据中学习特征和模式。
在图像识别中,深度学习算法能够提取图像中的复杂特征,如边缘、形状、纹理等,并对图像进行分类、目标检测、语义分割等任务。
常见的深度学习算法模型包括卷积神经网络(CNN)、循环神经网络(RNN)和生成对抗网络(GAN)等。
其中,卷积神经网络是图像识别中应用最为广泛的模型之一。
它通过卷积层和池化层对图像进行特征提取和降维,然后通过全连接层进行分类或预测。
二、深度学习算法在图像识别中的应用领域1、安防监控在安防领域,图像识别技术可以用于人脸识别、行为分析、车辆识别等。
深度学习算法能够快速准确地识别出监控画面中的目标,及时发出警报,保障公共安全。
例如,在机场、火车站等公共场所,人脸识别系统可以快速识别出可疑人员,提高安检效率。
2、医疗诊断医学图像识别是医疗领域中的一个重要应用方向。
深度学习算法可以对 X 光、CT、MRI 等医学图像进行分析,辅助医生进行疾病诊断。
例如,在肿瘤检测中,算法可以自动识别肿瘤的位置、大小和形状,为医生提供决策支持。
3、自动驾驶自动驾驶汽车需要对周围的环境进行实时感知和理解,图像识别技术在其中发挥着关键作用。
深度学习算法可以识别道路标志、交通信号灯、行人、车辆等物体,为车辆的自动驾驶提供可靠的信息。
4、工业检测在工业生产中,图像识别技术可以用于产品质量检测、缺陷检测等。
深度学习算法能够快速准确地检测出产品表面的瑕疵和缺陷,提高生产效率和产品质量。
5、电子商务在电子商务领域,图像识别技术可以用于商品识别、图像搜索等。
深度学习在图像识别方面的应用

深度学习在图像识别方面的应用近年来,深度学习在图像识别方面的应用日益广泛,成为一种强大的工具和技术。
深度学习通过构建和训练多层神经网络,可以辨别和分析复杂的图像信息,实现高精度的图像识别。
下面将介绍深度学习在图像识别方面的应用,并探讨其在不同领域中的重要性和潜在的发展前景。
一、深度学习在计算机视觉领域的应用计算机视觉是深度学习在图像识别方面的重要应用领域之一。
深度学习模型可以通过学习大量的标记图像,从而实现对图像特征的学习和提取。
通过卷积神经网络(CNN)等深度学习模型,可以有效地实现图像分类、目标检测、图像分割等任务。
例如,基于深度学习的图像分类算法在ImageNet图像识别竞赛中取得了显著的成绩,超过了传统方法。
此外,深度学习还被广泛应用于人脸识别、人体姿态估计、行为分析等计算机视觉任务中。
二、深度学习在医疗领域的应用深度学习在医疗领域的应用也吸引了广泛的关注。
图像识别是医学影像领域的重要任务之一,如CT、MRI扫描等。
深度学习可以通过学习大量的医学影像数据,自动识别并定位肿瘤、病灶等异常结构,辅助医生进行诊断和治疗。
此外,深度学习还可以用于药物发现和基因组数据分析等领域,帮助科学家更好地理解和治疗疾病。
三、深度学习在自动驾驶领域的应用自动驾驶技术是近年来备受关注的领域之一,而深度学习在该领域的应用也越发重要。
图像识别和分析是自动驾驶系统中必不可少的功能之一。
通过使用深度学习模型,自动驾驶车辆可以实现实时的道路标志识别、行人检测、车辆跟踪等功能,从而提高行驶安全性和驾驶体验。
此外,深度学习还可以用于车牌识别、行为预测等任务,为自动驾驶技术的进一步发展提供支持。
四、深度学习在工业检测领域的应用工业检测是深度学习在图像识别方面的重要应用之一。
在工业生产中,深度学习模型可以通过学习和识别产品表面的缺陷、异物等问题,提高产品质量和生产效率。
例如,通过使用深度学习算法,可以对电子产品、汽车零部件等进行缺陷检测,减少产品召回的风险。
- 1、下载文档前请自行甄别文档内容的完整性,平台不提供额外的编辑、内容补充、找答案等附加服务。
- 2、"仅部分预览"的文档,不可在线预览部分如存在完整性等问题,可反馈申请退款(可完整预览的文档不适用该条件!)。
- 3、如文档侵犯您的权益,请联系客服反馈,我们会尽快为您处理(人工客服工作时间:9:00-18:30)。
摘要对图像进行精确识别,具有非常重要的研究意义,图像识别技术在医药学、航天、军事、工农业等诸多方面发挥着重要的作用。
当前图像识别方法大多采用人工提取特征,不仅费时费力,而且提取困难;而深度学习是一种非监督学习,学习过程中可以不知道样本的标签值,整个过程无需人工参与也能提取到好的特征。
近年来,将深度学习用于图像识别成为了图像识别领域的研究热点,已取得了良好的效果,并且有广阔的研究空间。
本文基于深度学习在图像识别的相关理论,分析了深度学习的基本模型和方法,并在相关图像数据集上实验论证;另外鉴于深度学习多用于大样本集,本文基于小样本提出了一种改进算法,具体工作内容如下:(1)分析深度学习中卷积神经网络(Convolutional Neural Networks,CNNs)的基本原理,研究其训练过程和模型结构。
其中卷积层通过卷积运算,可以使原信号增强,并且降低噪声,提高信噪比;降采样对卷积层的图像进行子抽样,在保证了有用信息不降低的基础上,减少数据处理量。
将其在 MNIST手写字体数据集上进行实验,通过对比分析了该方法和其他经典算法在识别率和时间方面的优劣。
(2)针对于卷积神经网络训练时间过长这一缺陷,分析了深度学习中深度信念网络(Deep Belief Networks,DBNs)的基本原理、训练过程和模型结构。
DBNs的分层训练机制大大减少了训练难度,减少了训练时间。
引入Softmax作为模型的分类器,将其在MNIST手写字体数据集上进行实验,实验表明:深度信念网络在识别率上和卷积神经网络持平,但训练方法的改善使得消耗时间大大减少。
此外,该方法在自然场景CIFAR-10库上也有较好的实验效果。
(3)鉴于深度学习多适用于较大的数据集,针对小样本提出了一种改进的深度信念网络结构:深度信念网络整个过程可以分为预训练和参数微调两个阶段,改进的算法在预训练阶段对样本进行降采样;在参数微调阶段引入随机隐退(Dropout),将隐含层的结点随机清零掉一部分,保持其权重不更新。
将改进的模型在MNIST子集和ORL数据集上进行实验,实验表明:在小样本中,引入降采样和随机隐退后,深度信念网络在识别率和耗时方面都有不错的改善,过拟合现象得到有效缓解。
关键字:深度学习图像识别卷积神经网络深度信念网络小样本集AbstractPrecise recognition for image Has very important research significance, imagerecognition technology is widely used in Medicine , space military ,industry and agriculture.As now most method of image recognition Used artificial feature extraction which Not onlylaborious, but also difficult to extract. Deep Learning is a kind of unsupervised learning, Inthe learning process we need not know the values of samples, The whole process can alsoextracted good characteristics without human participation. in recent years , The deeplearning used in image recognition become the hot research topic in the field of imagerecognition , Has achieved good effect, and have a broad space for research.In this paper, we based on the study on the theory of image recognition analyzes thedeep learning the basic models and methods ,then do experiment on some image data sets .Given deep learning more for large sample set , we improved a algorithm proposed to use itinto small sample set, the work can be described as follows:( 1 ) Analysis basic principles of the convolution neural networks (CNNs) , introducethe training process and model structure of it. The convolution layer can make the originalsignal enhancement, and reduce noise as well as improve signal-to-noise ratio byconvolution operation, use the model into the handwriting data set MNIST, compared toother classical algorithms, analyze their advantages and disadvantages about time andrecognition rate.( 2 ) aim at the inadequacies of the convolution neural network , Analysis the basicprinciples , training process and model structure of deep belief networks (DBNs). Thestratified training mechanism of DBNs greatly reduces the difficulty and reduces the trainingtime of it. We use Softmax classification system as the classifier , the use this model doexperiment on MNIST datasets , compared to convolution neural network, mainly in therecognition rate and time-consuming ,which can be proved that DBNs has the sameidentifying rate of CNNs, but the elapsed time is greatly reduced, and then analyze thereasons ; addition, use the model into the CIFAR-10 databases , compared to the otheralgorithms .( 3 ) aim at the deep belief networks algorithm is only applicable to large data sets, raisea improved algorithm of deep belief network aim at small sample set . Before the pre-training , down-sampling of samples , after the training, in the parameter fine-tuning phase ,use the dropout ideas. Down-sampling and dropout can effectively prevent of overfitting ,the improved system is applied on a MNIST subset and ORL datasets , experiments showthat this system indeed prevent over-fitting , the algorithm has good improvements in therecognition rate and time-consuming both.Keywords: Deep learning Image recognition Convolutional Neural Networks DeepBelief Networks Small sample set.目录摘要 (I)Abstract (II)第 1 章绪论 (1)1.1课题来源 (1)1.2课题研究背景及意义 (1)1.3国内外研究现状 (5)1.4论文主要内容和组织结构 (6)第 2 章基于卷积神经网络的图像识别 (7)2.1传统人工神经网络结构 (7)2.2卷积神经网络结构 (9)2.2.1卷积神经网络结构 (10)2.2.2卷积神经网络的参数减少和权值共享 (11)2.2.3卷积神经网络的参数更新 (13)2.3基于卷积神经网络的手写字体识别 (16)2.3.1MNIST数据库及手写字体识别一般方法 (16)2.3.2基于卷积神经网络的手写字体识别 (16)2.3.3实验结果和对比 (18)2.4本章小结 (21)第 3 章基于深度信念网络的图像识别 (22)3.1深度网络分层训练的思想 (22)3.1.1 浅层学习和深度学习 (22)3.1.2 深度网络的分层学习思想 (24)3.2深度信念网络(DBNs)基本原理 (25)3.2.1 受限制玻尔兹曼机模型 (25)3.2.2 受限制玻尔兹曼机(RBM)学习方法 (27)3.2.3 深度信念网络(DBNs)的模型和方法 (29)3.3 基于深度信念网络的图像识别 (31)3.3.1 基于深度信念网络的手写字体识别 (31)3.3.2基于深度信念网络的自然场景识别 (35)3.4 本章小结 (36)第 4 章基于小样本的深度信念网络的应用研究 (37)4.1 随机隐退思想及其应用 (37)4.1.1 随机隐退的思想 (37)4.1.2 随机隐退应用于神经网络 (39)4.2 随机隐退和深度学习算法的结合 (42)4.3基于降采样和随机隐退的深度信念网络 (45)4.3.1图像降采样 (46)4.3.2降采样和随机隐退相结合应用于深度信念网络 (47)4.4小结 (50)第 5 章总结与展望 (51)5.1 课题总结 (51)5.2 课题展望 (52)致谢 (53)参考文献 (54)第 1 章绪论1.1课题来源本课题来源于国家自然科学基金面上项目,基金号为:61273241。