计算机视觉+人体姿态识别+双目视觉
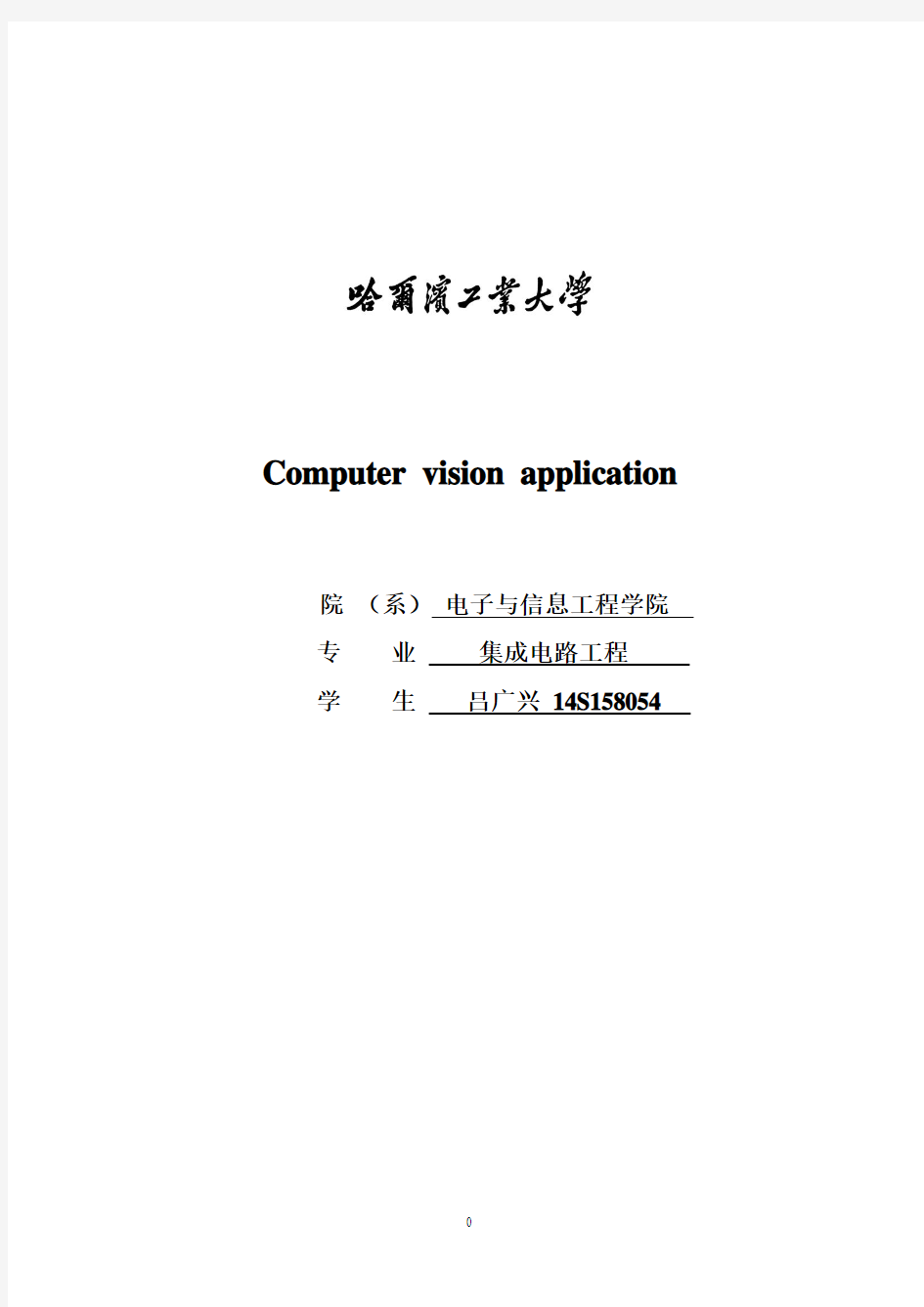

Computer vision application
院(系)电子与信息工程学院
专业集成电路工程
学生吕广兴14S158054
Computer vision application
The directory
Report: Computer vision application (2)
1.The object of the project (2)
2.The method and the principle applied to the project (2)
2.1 Platform (2)
2.2 The principle of transform the RGB image to the gray image (2)
2.3 The principle of image enhancement (2)
2.4 The principle of thresholding (3)
2.5 The principle of classifier (3)
3.The content and the result of the project (4)
3.1 The main steps in the project (4)
3.2 About human body posture recognition (4)
About three kinds of methods are most common: (4)
3.3.Stereo vision (11)
4.Reference (18)
Report: Computer vision application
1.The object of the project
The object of the project is Gesture recognition and location in the interior of people.
2.The method and the principle applied to the project
2.1 Platform
The platform is based on Visual Studio 2012 and OpenCV 2.4.10.
2.2 The principle of transform the RGB image to the gray image
There are three major methods to transform the RGB image to the gray image.
The first one is called the maximum value that is set the value of R, G, and B to the maximum of these three.
Gray=R=G=B=max(R, G, B)
The second one is called mean value which is set the value of R, G, and B to the mean value of these three.
Gray=R=G=B=(R+G+B)/3
The third one is called weighted average that is giving different weights to the R, G and B according to the importance or other indicators, and then adding the three parts together. In fact, human’s eye is very high se nsitive to green, then red, last blue.
Gray=0.30R+0.59G+0.11B
2.3 The principle of image enhancement
Image enhancement is the process of making images more useful. There are two broad categories of image enhancement techniques. The first one is spatial domain technique, and it is a direct manipulation of image pixels that includes point processing and neighborhood operations. The second one is frequency domain technique, and it is a manipulation of Fourier transform or wavelet transform of an image.
The principle of the median filter is to replace the value of a pixel by the median of the gray levels in the neighborhood of that pixel(the original value of the pixel is included in the computation of the median). It forces the points with distinct gray levels to be more like their neighbors.
In addition, we also apply the morphological image processing after smoothing. Morphological image processing (or morphology) describes a range of image processing techniques that deal with the shape (or morphology) of features in an image. The basic ideal of Morphology is to use a special structuring element to measure or extract the corresponding shape or characteristics in the input images for further image analysis and object recognition. The mathematical foundation of morphology is the set theory. There are two basic morphological operations: erosion and dilation.
2.4 The principle of thresholding
Thresholding is particularly useful for segmentation in which we want to isolate an object of interest from a background. At the same time, thresholding segmentation is usually the first step in any segmentation approach. The blow formula is the basic principle of image segmentation. When the gray level is no bigger than the threshold, we will set the pixel value zero(black). In contrast, when the gray level is bigger than the threshold, we will set the pixel value 255(white).
threshold
r threshold
r s ><==,255,0{ When it comes to the threshold, we get the value through the image histograms 2.5 The principle of classifier
The classifier is a algorithm or device that separates objects into different classes. Usually, a classifier consists of three parts. First one is the sensor, for instance, imaging device, fingerprint reader, etc...Second one is feature extractor, for example, edge detector or property descriptor. Third one is classifier which uses the extracted features for decision making or Euclidian distance, or other methods.
Features should can be regarded as the descriptors we introduced before. And the feature should be representative and useful for classification.
When it comes to the feature space, the set of all possible patterns form the feature vector. Each feature vector is a point in the so-called feature space. Similar objects yield similar measurement results. Nearby points in feature space correspond to similar objects. Distance in feature space is related to similarity. Points that belong to the same class form a cloud in feature space.
Divide the data set into a training set and a test set. The performance of a classifier should be assessed by the classification error on an independent test set. This set should not contain objects that are included in the training set. Determine a decision boundary by minimizing the classification error of the training set. Determine the classifier performance by computing the classification error of the test set.
3.The content and the result of the project
3.1 The main steps in the project
Before we segment the vessel and classify the vessel, we can find these images afforded are color. So first we should load these pictures into gray images. Because we use the method of SVM (Support Vector Machines), we should divide these images into a training set and a test set. Next is image enhancement, and the next is thresholding, and then object extraction. Next is feature extraction and vessel classification. We first use the training set to train these images with representative features and then test the test set to recognize the human’s gesture.The depth information of human is obtained by the binocular stereo vision.And then obtain the position of the people and prepare for the three-dimensional reconstruction.
3.2 About human body posture recognition
About three kinds of methods are most common:
1.Method based on template matching.
2.Method based on Classification.
3.Prediction based approach.
The method based on template matching maybe the most accurate of the all three. However this method will consume a lot of time. So it is not real time.The method based on Classification meets the accuracy requirements in the process of dealing with small data and Implementation ,so, in a single scene, for the time being, this method is used for the time being.About third methods:If the data processed by the computer in a complex scene, the data will be expanded in a geometric scale.Dealing with this problem is the most difficult problem in artificial intelligence.However, in recent years, the neural network based on deep learning's voice recognition and image processing has shown the advantages.
3.2.1 Foreground extraction
Moving target detection is the basis of the whole target detection and tracking system.
Based on the video, but also for further processing (such as encoding, target tracking, target classification, target behavior understanding foundation). The purpose of moving target detection is to extract the moving object (such as human, vehicle, etc.) from the video image.
Frame difference method, background subtraction method and optical flow
method. Based on the three kinds of commonly used methods. There are many kinds of improvement methods, one is the inter frame difference method and the background difference method combining method and good results have been achieved, but there are still retain less incomplete object contour detection and target point https://www.360docs.net/doc/7716997778.html,ing background subtraction method is better than the direct access method, access to background method and statistical average method, it is a method that through carrying on the statistics to the continuous image sequence averaged to obtain image background.
In addition to get better background, R.T.Colin proposed to established a single Gauss background model.Grimson et al. Proposed an adaptive hybrid Gauss background model to obtain a more accurate background description for target detection.At the same time, in order to increase the robustness and reduce the impact of environmental changes, to update the background is important.For example based on the recursive updating of the statistical averaging method, a simple adaptive filter is used to update the background model.
In this paper, according to the algorithm proposed in KaewTraKulPong et al.[1]Zivkovic et al.[2][3], we use the update variance to the 3 parameters in the root model, and finally, the algorithm is realized by using OpenCV basic function. The main processes are as follows:
1.Firstly, the mean, variance and weight of each Gauss are set to 0.
2.The T model used in the video is used to train the GMM model. For each pixel, the establishment of its model number of the largest GMM_MAX_COMPONT Gauss GMM model. When the first pixel is set up, the initial mean, variance, and weight are set to 1.
3.The first frame of the training process, when back to the pixel value, compared with the previous Gaussian mean, if the pixel value and mean value model in three times the variance of the difference, the task is the Gaussian. At this point, the following equation is used to update.
4.when training frames in T, different GMM pixel number adaptive selection. First of all, with the weight divided by the variance of the various Gauss from big to small sort, and then select the most in front of B Gauss, so that
Where
c is generally set to 0.3.
f
So that we can eliminate the noise points in the training process.
5. during the testing phase, the new pixel value is compared with every mean values of B a Gaussian, if the difference between 2 times the variance of the words, that is the background, or that the foreground. And as long as there is a Gauss component to meet the condition is considered a prospect. Foreground assignment is 255, and the background value is 0. So as to form a two value chart.
6. Due to foreground binary map contains a lot of noise, so the use of morphological opening operation noise is reduced to 0, followed by the closed reconstruction operation due to the edge portion of the opening operation loss of information. Eliminate the small noise points.
The above is the algorithm to achieve the general process, but when we are in the specific programming, there are still many details of the need to pay attention, such as the choice of some parameter values. Here, after testing some of the commonly used parameter values are declared as follows:
Among the 3 parameters of the value of the update variance, the learning rate is 0.005. That is to say T equals 200.
Define the maximum number of mixed Gauss number for each pixel 7.
Take video of the first 200 frames for training.
Take Cf 0.3. That is to meet the weight of the number is greater than 0.7 of the number of adaptive Gauss B.
During the training process, a new set of Gauss is needed, and the weight value is equal to the value of the learning rate, which is 0.005.
During the training process, the need to build a new Gauss, take the mean value of the input pixel value for the input of the Gauss. The variance was 15.
During the training process, the need to build a new Gauss, take the variance of the Gauss 15.
The following picture is a dynamic background in the training process.
Figure 3.1 the result of foreground extraction
3.2.2 Feature extraction
After an image has been segmented into regions. Representation and description should be considered.Representation and description used to make the data useful to a computer. Representing region in 2 ways
1.In terms of its external characteristics (its boundary)focus on shape characteristics
2.In terms of its internal characteristics (its region) focus on regional properties,
e.g., color, texture.Sometimes, we may need to use both ways.
Choosing a representation scheme, however is only the part of the task of making data useful to computer.The next task is to describe the region based on the chosen representation.For example:
Representation boundary :Description of the length of the boundary, orientation of the straight line joining its extreme points, and the number of concavities in the boundary.
To find the feature of the target , we need to extract the contour of the target, and to extract object from the background based on the area of every contour. And having the largest area is the destination physical contour. Here we use the blow function:
(1) Find contours
findContours(image,
contours,//轮廓的数组
CV_RETR_EXTERNAL,//获取外轮
CV_CHAIN_APPROX_NONE);//获取每个轮廓的每个像素
(2) Draw contours
drawContours(result,contours,
-1,//绘制所有轮廓
cv::Scalar(0),//颜色信息为黑色
2);//轮廓线的绘制宽度为2
The following image is the result of contours extraction and object extraction.
Figure 3.2 The result of extraction of contour and object At last ,we choose the characteristics of Length of boundary and the hight of Feret box to train and predict.We also test other characteristics but not as good as these two.
3.2.3. Recognition and classification
3.2.3.1 Classifier
We use the SVM (Support vector machine) classifier to recognize the ships. Support vector machines are supervised learning models with associated learning algorithms that analyze data and recognize patterns, used for classification and regression analysis. Given a set of training examples, each marked as belonging to one of two categories, an SVM training algorithm builds a model that assigns new examples into one category or the other, making it a non-probabilistic binary linear classifier. An SVM model is a representation of the examples as points in space,
mapped so that the examples of the separate categories are divided by a clear gap that is as wide as possible. There are four main steps to structure SVM:
1. A given training set T={(x1,y1), (x2,y2),…, (x n, y n)}
2.Solving two quadratic programming problem:
We get the solution:
3.Calculate the parameters of W, and selects a positive component to compute b
4.To construct a decision boundary:, thus the have
the decision function:
All the above work can be done with the OpenCv functions, but it need us to make the train file and test file for it. Training file used for learning, and with the features, we can make classification of these four vessels(testing file). In short SVM steps can be summaries as: training (learning), testing and predicting.
When choosing the images, we main keep this in mind: In order to train SVM effectively, we could not choose vessel image for training freely, instead we need to select the vessel shape with more obvious characteristics, and can be representative for vessel image type. If the vessel shape too special or similar would interfere the SVM learning. Because too diverse the sample is, it will increases the difference between feature vectors, reduces the classification of objects. As a result, it increase the burden of SVM learning.
Some main SVM codes used:
// The parameters of support vector machine settings
CvSVMParams params;
params.svm_type = CvSVM::C_SVC; //SVM type: C using support vector machine
params.kernel_type = CvSVM::LINEAR;// The type of kernel function: linear
params.term_crit = cvTermCriteria(CV_TERMCRIT_ITER, 100, 1e-6); //Termination criterion function: when the number of iterations reaches the maximum
// SVM training
CvSVM SVM; // Establish an instance of the SVM model
SVM.train(trainingDataMat, labelsMat, Mat(), Mat(), params); //The training model, parameters are: input data, response, ··,··, features
Figure 3.4 the result of pattern recognition
3.2.3.2 Recognition results
Test results
Test samples Correct
precision
identification number
550 550 100%
3.2.
4. Conclusion
From the block above, we know that the method we use can distinguish the several ships, but still exist errors. Because the number of given picture not very large .When test the category of one picture ,it is inevitable to make error. And some categories of pictures also have some same points, which the feature is similar, hence it’s hard to distinguish them. What’ more the classifier SVM also exist some errors, it could not classify exactly. It also can be the features we chose are not enough, so some more work should be done.
3.3.Stereo vision
3.3.1 Stereopsis
Fusing the pictures recorded by our two eyes and exploiting the difference (or disparity) between them allows us to gain a strong sense of depth. This chapter is concerned with the design and implementation of algorithms that mimic our ability
to perform this task, known as stereopsis. Reliable computer programs for stereoscopic perception are of course invaluable in visual robot navigation (Figure 7.1),
cartography, aerial reconnaissance, and close-range photogrammetry. They are also
of great interest in tasks such as image segmentation for object recognition or the construction of three-dimensional scene models for computer graphics applications.
figure 3.4: Left: The Stanford cart sports a single camera moving in discrete increments
along a straight line and providing multiple snapshots of outdoor scenes. Center: The
INRIA mobile robot uses three cameras to map its environment. Right: The NYU mobile robot uses two stereo cameras, each capable of delivering an image pair. As shown by these examples, although two eyes are sufficient for stereo fusion, mobile robots are sometimes equipped with three (or more) cameras. The bulk of this chapter is concerned with binocular perception but stereo algorithms using multiple cameras are discussed [4]. Photos courtesy of Hans Moravec, Olivier Faugeras, and Yann LeCun.
Stereo vision involves two processes: The fusion of features observed by two(or more) eyes and the reconstruction of their three-dimensional preimage. The latter is relatively simple: The preimage of matching points can (in principle) be found at the intersection of the rays passing through these points and the associated pupil centers (or pinholes; see Figure 3.5, left). Thus, when a single image feature is observed at any given time, stereo vision is easy. However, each picture typically consists of millions of pixels, with tens of thousands of image features such as edge elements, and some method must be devised to establish the correct correspondences and avoid erroneous depth measurements (Figure 3.5, right).
Figure 7.2: The binocular fusion problem: In the simple case of the diagram shown on the left, there is no ambiguity, and stereo reconstruction is a simple matter. In the more usual case shown on the right, any of the four points in the left picture may, a priori, match any of the four points in the right one. Only four of these correspondences are correct; the other ones yield the incorrect reconstructions shown as small gray discs.
However, camera calibration can eliminate the distortion.To obtain more accurate depth information[5].
Figure 3.4 the result of calibration of cameras. And the distortion is eliminated. The camera parameters are as follows:
extrinsics:1.0
R: !!opencv-matrix
rows: 3
cols: 3
dt: d
data: [ 9.9990360000625755e-001, 9.7790647772508701e-003,
-9.8570069802389540e-003, -9.8969610939301841e-003,
9.9987921323260354e-001, -1.1983701700849161e-002,
9.7386269890257296e-003, 1.2080100886666228e-002,
9.9987960790634012e-001 ]
T: !!opencv-matrix
rows: 3
cols: 1
dt: d
data: [ 3.4075702905319170e+000, 1.1739005828568252e-003, -7.9252820494919135e-002 ]
R1: !!opencv-matrix
rows: 3
cols: 3
dt: d
data: [ 9.9940336523117301e-001, 9.8399020411941429e-003, -3.3107248336674097e-002, -1.0040790862200610e-002,
9.9993214239488748e-001, -5.9070402429666716e-003,
3.3046877060745931e-002, 6.2359388539483330e-003,
9.9943434851076729e-001 ]
R2: !!opencv-matrix
rows: 3
cols: 3
dt: d
data: [ 9.9972958617043228e-001, 3.4440467660098648e-004, -2.3251578890794586e-002, -2.0319599978103648e-004,
9.9998152534851392e-001, 6.0751685610454320e-003,
2.3253241642441649e-002, -6.0688011236303754e-003,
9.9971118649640012e-001 ]
P1: !!opencv-matrix
rows: 3
cols: 4
dt: d
data: [ 8.9095402067418593e+002, 0., 3.2619792175292969e+002, 0., 0.,
8.9095402067418593e+002, 2.1098579597473145e+002, 0., 0., 0., 1.,
0. ]
P2: !!opencv-matrix
rows: 3
cols: 4
dt: d
data: [ 8.9095402067418593e+002, 0., 3.2619792175292969e+002,
3.0368096464054670e+003, 0., 8.9095402067418593e+002,
2.1098579597473145e+002, 0., 0., 0., 1., 0. ]
Q: !!opencv-matrix
rows: 4
cols: 4
dt: d
data: [ 1., 0., 0., -3.2619792175292969e+002, 0., 1., 0.,
-2.1098579597473145e+002, 0., 0., 0., 8.9095402067418593e+002, 0.,
0., -2.9338487571282823e-001, 0. ]
intrinsics:1.0
M1: !!opencv-matrix
rows: 3
cols: 3
dt: d
data: [ 1.1136300108848973e+003, 0., 3.0020338800373816e+002, 0.,
1.1136300108848973e+003,
2.1821348683113223e+002, 0., 0., 1. ]
D1: !!opencv-matrix
rows: 1
cols: 8
dt: d
data: [ 1.0442196198936304e-001, -2.3958410365610397e-001, 0., 0., 0.,
0., 0., 2.7243194967195151e+001 ]
M2: !!opencv-matrix
rows: 3
cols: 3
dt: d
data: [ 1.1136300108848973e+003, 0., 3.0559143946713289e+002, 0.,
1.1136300108848973e+003,
2.1736307957090108e+002, 0., 0., 1. ] D2: !!opencv-matrix
rows: 1
cols: 8
dt: d
data: [ -1.8888461100187631e-001, 5.8249894498215049e+000, 0., 0., 0.,
0., 0., 3.5710666837966521e+001 ]
Then we can get more accurate depth information:
After correction of the binocular camera,the error within 10 meters is within 4 cm.
4.Reference
1.KaewTraKulPong, P. and R. Bowden (2001). An improved adaptive
background mixture model for real-time tracking with shadow detection.
2.Zivkovic, Z. and F. van der Heijden (2004). “Recursive unsupervised learning
of finite mixture models.” Pattern Analysis and Machine Intelligence, IEEE Transactions on 26(5): 651-656.
3.Zivkovic, Z. and F. van der Heijden (2006). “Efficient adaptive de nsity
estimation per image pixel for the task of background subtraction.” Pattern recognition letters 27(7): 773-780.
https://www.360docs.net/doc/7716997778.html,PUTER VISION A MODERN APPROACH second edition avid A. Forsyth University of Illinois at Urbana-Champaign Jean Ponce Ecole Normale Supérieure:3-22
5. Learning OpenCV: Computer Vision with the OpenCV Library by Gary Bradski and Adrian Kaehler Published by O'Reilly Media, October 3, 2008
人工智能与模式识别
人工智能与模式识别 摘要:信息技术的飞速发展使得人工智能的应用范围变得越来越广,而模式识别作为其中的一个重要方面,一直是人工智能研究的重要方向。在介绍人工智能和模式识别的相关知识的同时,对人工智能在模式识别中的应用进行了一定的论述。模式识别是人类的一项基本智能,着20世纪40年代计算机的出现以及50年代人工智能的兴起,模式识别技术有了长足的发展。模式识别与统计学、心理学、语言学、计算机科学、生物学、控制论等都有关系。它与人工智能、图像处理的研究有交叉关系。模式识别的发展潜力巨大。 关键词:模式识别;数字识别;人脸识别中图分类号; Abstract:The rapid development of information technology makes the application of artificial intelligence become more and more widely. Pattern recognition, as one of the important aspects, has always been an important direction of artificial intelligence research. In the introduction of artificial intelligence and pattern recognition related knowledge at the same time, artificial intelligence in pattern recognition applications were discussed.Pattern recognition is a basic human intelligence, the emergence of the 20th century, 40 years of computer and the rise of artificial intelligence in the 1950s, pattern recognition technology has made great progress. Pattern recognition and statistics, psychology, linguistics, computer science, biology, cybernetics and so have a relationship. It has a cross-correlation with artificial intelligence and image processing. The potential of pattern recognition is huge. Key words:pattern recognition; digital recognition; face recognition; 1引言 随着计算机应用范围不断的拓宽,我们对于计算机具有更加有效的感知“能
基于MATLAB的人体姿态的检测课程设计
基于视频的人体姿态检测 一、设计目的和要求 1.根据已知要求分析视频监控中行人站立和躺卧姿态检测的处理流程,确定视频监中行人的检测设计的方法,画出流程图,编写实现程序,并进行调试,录制实验视频,验证检测方法的有效性,完成系统软件设计。 2.基本教学要求:每人一台计算机,计算安装matlab、visio等软件。 二、设计原理 图像分割中运动的运用(运动目标检测) 首先利用统计的方法得到背景模型,并实时地对背景模型进行更新以适应光线变化和场景本身的变化,用形态学方法和检测连通域面积进行后处理,消除噪声和背景扰动带来的影响,在HSV色度空间下检测阴影,得到准确的运动目标。 噪声的影响,会使检测结果中出现一些本身背景的区域像素点被检测成运动区域,也可能是运动目标内的部分区域被漏检。另外,背景的扰动,如树枝、树叶的轻微摇动,会使这部分也被误判断为运动目标,为了消除这些影响,首先对上一步的检测结果用形态学的方法进行处理,在找出经过形态学处理的后的连通域,计算每个连通域中的面积,对于面积小于一定值的区域,将其抛弃,不看做是前景运动目标。 2.2bwlabel函数 用法:L = bwlabel(BW,n) [L,num] = bwlabel(BW,n),这里num返回的就是BW中连通区域的个数。 返回一个和BW大小相同的L矩阵,包含了标记了BW中每个连通区域的类别标签,这些标签的值为1、2、num(连通区域的个数)。n的值为4或8,表示是按4连通寻找区域,还是8连通寻找,默认为8。 四连通或八连通是图像处理里的基本感念:8连通,是说一个像素,如果和其他像素在上、下、左、右、左上角、左下角、右上角或右下角连接着,则认为他们是联通的;4连通是指,如果像素的位置在其他像素相邻的上、下、左或右,则认为他们是连接着的,连通的,在左上角、左下角、右上角或右下角连接,则不认为他们连通。
时间序列分析中模式识别方法的应用-模式识别论文
时间序列分析中模式识别方法的应用 摘要:时间序列通常是按时间顺序排列的一系列被观测数据,其观测值按固定的时间间隔采样。时间序列分析(Time Series Analysis)是一种动态数据处理的统计方法,就是充分利用现有的方法对时间序列进行处理,挖掘出对解决和研究问题有用的信息量。经典时间序列分析在建模、预测等方面已经有了相当多的成果,但是由于实际应用中时间序列具有不规则、混沌等非线性特征,使得预测系统未来的全部行为几乎不可能,对系统行为的准确预测效果也难以令人满意,很难对系统建立理想的随机模型。神经网络、遗传算法和小波变换等模式识别技术使得人们能够对非平稳时间序列进行有效的分析处理,可以对一些非线性系统的行为作出预测,这在一定程度上弥补了随机时序分析技术的不足。【1】 本文主要是对时间序列分析几种常见方法的描述和分析,并重点介绍神经网络、遗传算法和小波变换等模式识别方法在时间序列分析中的典型应用。 关键字:时间序列分析模式识别应用 1 概述 1.1 本文主要研究目的和意义 时间序列分析是概率论与数理统计学科的一个分支,它是以概率统计学作为理论基础来分析随机数据序列(或称动态数据序列),并对其建立数学模型,即对模型定阶、进行参数估计,以及进一步应用于预测、自适应控制、最佳滤波等诸多方面。由于一元时间序列分析与预测在现代信号处理、经济、农业等领域占有重要的地位,因此,有关的新算法、新理论和新的研究方法层出不穷。目前,结合各种人工智能方法的时序分析模型的研究也在不断的深入。 时间序列分析已是一个发展得相当成熟的学科,已有一整套分析理论和分析工具。传统的时间序列分析技术着重研究具有随机性的动态数据,从中获取所蕴含的关于生成时间序列的系统演化规律。研究方法着重于全局模型的构造,主要应用于对系统行为的预测与控制。 时间序列分析主要用于以下几个方面:
二维人体姿态估计研究综述
研究与开发 现代计算机2019.08上 文章编号:1007-1423(2019)22-0033-05 DOI :10.3969/j.issn.1007-1423.2019.22.007 二维人体姿态估计研究综述 李崤河,刘进锋 (宁夏大学信息工程学院,银川750021) 摘要: 人体姿态估计一直是计算机视觉中一个备受关注的研究热点,在智能安防、人机交互、动作识别等领域有着重要的研究价值。近年来,随着深度学习技术的快速发展,人体姿态估计效果不断提升,已经开始广泛应用于计算机视觉的相关领域。梳理二维人体姿态估计算法的发展与现状,总结传统算法与基于深度学习的姿态估计算法的发展与改进,并做出对比;讨论二维人体姿态估计所面临的困难与挑战,并对未来的发展方向做出展望。关键词: 深度学习;人体姿态估计;关键点检测基金项目: 宁夏高等学校科学研究项目(No.NGY2015044) 0引言 人体姿态估计长久以来一直是计算机视觉领域的 一个热点问题。其主要内容,是让计算机从图像或视频中定位出人物的关键点(也称为关节点,如肘、手腕等)。人体姿态估计作为理解图像或视频中人物动作的基础,一直受到众多学者的关注。随着计算机技术的迅猛发展,人体姿态估计已经在动作识别、人机交互、智能安防、增强现实等领域获得了广泛应用。 人体姿态估计按维度可分为二维和三维两种:二维人体姿态估计通常使用线段或者矩形来描述人体各关节在图像上的投影位置,线段的长度和角度表示了人体的二维姿态;三维人体姿态估计通常使用树模型来描述估计的姿态,各关节点的位置使用三维坐标确定。在实际应用中,目前获取的大多数图像仍是二维图像,同时三维姿态估计可以使用二维预测进行推理[1],所以二维姿态估计有着重要的研究价值。 自人体姿态估计的概念提出以来,国内外的学者对此做出了不懈的努力。传统的姿态估计算法主要是基于图结构(Pictorial Structures )模型[2] 。该模型将人或物体表示为多个部件的集合,这些部件之间含有空间约束,通过人工指定的特征检测组件实现关节点检 测。传统方法过于依赖手工设计的模板,难以应付复杂的姿态变换并且推广到多人姿态估计。 随着深度学习技术在计算机视觉领域大放异彩,部分学者开始研究如何利用深度学习来解决人体姿态估计问题。Toshev 等人利用深度卷积神经网络对人体姿态进行全局推断,提出了完全基于神经网络的模型DeepPose [3]。DeepPose 是第一个将深度学习方法应用于人体姿态估计的主要模型。该模型实现了SOTA 性能并击败了当时的传统模型。之后,越来越多基于深 度学习的人体姿态估计方法相继提出。 本文总结了近几年来二维人体姿态估计的发展历程,从早期的基于模板匹配算法到目前的基于深度学习的姿态估计算法,分析比较相关算法的优缺点及性能,并结合现有问题对未来发展进行了展望。1传统算法 早期的人体关键点检测算法基本都是在几何先验 的基础上基于模板匹配的思路进行的,其中Fischler [2]于1973年提出的图结构是其中一个较为经典的算法思路。它将物体表示为多个部件的集合,部件之间具有一定的空间约束。2005年,Felzenszwalb 和Huttenlo?
图像模式识别的方法介绍
2.1图像模式识别的方法 图像模式识别的方法很多,从图像模式识别提取的特征对象来看,图像识别方法可分为以下几种:基于形状特征的识别技术、基于色彩特征的识别技术以及基于纹理特征的识别技术。其中,基于形状特征的识别方法,其关键是找到图像中对象形状及对此进行描述,形成可视特征矢量,以完成不同图像的分类,常用来表示形状的变量有形状的周长、面积、圆形度、离心率等。基于色彩特征的识别技术主要针对彩色图像,通过色彩直方图具有的简单且随图像的大小、旋转变换不敏感等特点进行分类识别。基于纹理特征的识别方法是通过对图像中非常具有结构规律的特征加以分析或者则是对图像中的色彩强度的分布信息进行统计来完成。 从模式特征选择及判别决策方法的不同可将图像模式识别方法大致归纳为两类:统计模式(决策理论)识别方法和句法(结构)模式识别方法。此外,近些年随着对模式识别技术研究的进一步深入,模糊模式识别方法和神经网络模式识别方法也开始得到广泛的应用。在此将这四种方法进行一下说明。 2.1.1句法模式识别 对于较复杂的模式,如采用统计模式识别的方法,所面临的一个困难就是特征提取的问题,它所要求的特征量十分巨大,要把某一个复杂模式准确分类很困难,从而很自然地就想到这样的一种设计,即努力地把一个复杂模式分化为若干
较简单子模式的组合,而子模式又分为若干基元,通过对基元的识别,进而识别子模式,最终识别该复杂模式。正如英文句子由一些短语,短语又由单词,单词又由字母构成一样。用一组模式基元和它们的组成来描述模式的结构的语言,称为模式描述语言。支配基元组成模式的规则称为文法。当每个基元被识别后,利用句法分析就可以作出整个的模式识别。即以这个句子是否符合某特定文法,以判别它是否属于某一类别。这就是句法模式识别的基本思想。 句法模式识别系统主要由预处理、基元提取、句法分析和文法推断等几部分组成。由预处理分割的模式,经基元提取形成描述模式的基元串(即字符串)。句法分析根据文法推理所推断的文法,判决有序字符串所描述的模式类别,得到判决结果。问题在于句法分析所依据的文法。不同的模式类对应着不同的文法,描述不同的目标。为了得到于模式类相适应的文法,类似于统计模式识别的训练过程,必须事先采集足够多的训练模式样本,经基元提取,把相应的文法推断出来。实际应用还有一定的困难。 2.1.2统计模式识别 统计模式识别是目前最成熟也是应用最广泛的方法,它主要利用贝叶斯决策规则解决最优分类器问题。统计决策理论的基本思想就是在不同的模式类中建立一个决策边界,利用决策函数把一个给定的模式归入相应的模式类中。统计模式识别的基本模型如图2,该模型主要包括两种操作模型:训练和分类,其中训练主要利用己有样本完成对决策边界的划分,并采取了一定的学习机制以保证基于样本的划分是最优的;而分类主要对输入的模式利用其特征和训练得来的决策函数而把模式划分到相应模式类中。 统计模式识别方法以数学上的决策理论为基础建立统计模式识别模型。其基本模型是:对被研究图像进行大量统计分析,找出规律性的认识,并选取出反映图像本质的特征进行分类识别。统计模式识别系统可分为两种运行模式:训练和分类。训练模式中,预处理模块负责将感兴趣的特征从背景中分割出来、去除噪声以及进行其它操作;特征选取模块主要负责找到合适的特征来表示输入模式;分类器负责训练分割特征空间。在分类模式中,被训练好的分类器将输入模式根据测量的特征分配到某个指定的类。统计模式识别组成如图2所示。
模式识别方法简述
XXX大学 课程设计报告书 课题名称模式识别 姓名 学号 院、系、部 专业 指导教师 xxxx年 xx 月 xx日
模式识别方法简述 摘要:模式识别(Pattern Recognition)是指对表征事物或现象的各种形式的( 数值的、文字的和逻辑关系的) 信息进行处理和分析, 以对事物或现象进行描述、辨认、分类和解释的过程, 是信息科学和人工智能的重要组成部分。模式识别研究主要集中在两方面, 一是研究生物体( 包括人) 是如何感知对象的,属于认识科学的范畴, 二是在给定的任务下, 如何用计算机实现模式识别的理论和方法。前者是生理学家、心理学家、生物学家和神经生理学家的研究内容, 后者通过数学家、信息学专家和计算机科学工作者近几十年来的努力, 已经取得了系统的研究成果。 关键词:模式识别; 模式识别方法; 统计模式识别; 模板匹配; 神经网络模式识别 模式识别(Pattern Recognition)是人类的一项基本智能,在日常生活中,人们经常在进行“模式识别”。随着2 0 世纪4 0 年代计算机的出现以及5 0 年代人工智能的兴起,人们当然也希望能用计算机来代替或扩展人类的部分脑力劳动。(计算机)模式识别在2 0 世纪6 0 年代初迅速发展并成为一门新学科。 模式识别研究主要集中在两方面, 一是研究生物体( 包括人) 是如何感知对象的,属于认识科学的范畴, 二是在给定的任务下, 如何用计算机实现模式识别的理论和方法。前者是生理学家、心理学家、生物学家和神经生理学家的研究内容, 后者通过数学家、信息学专家和计算机科学工作者近几十年来的努力, 已经取得了系统的研究成果。模式识别与统计学、心理学、语言学、计算机科学、生物学、控制论等都有关系。它与人工智能、图像处理的研究有交叉关系。例如自适应或自组织的模式识别系统包含了人工智能的学习机制;人工智能研究的景物理解、自然语言理解也包含模式识别问题。又如模式识别中的预处理和特征抽取环节应用图像处理的技术;图像处理中的图像分析也应用模式识别的技术。 模式识别是一种借助计算机对信息进行处理、判别的分类过程。判决分类在
人体姿态捕捉方法综述
人体姿态捕捉方法综述 XXX (大连理工大学软件学院,辽宁大连116600) 摘要:人体姿态捕捉技术在人机交互和虚拟现实等领域的重要性日益突出,为了满足人们对于高精确度、高效率的动作捕捉技术的需求,科学家从各个方面进行了创新性的尝试。文章介绍了动作捕捉技术发展历史,并给出了其概念和基本组成;并阐述了目前国内外发展现状;其次详细地对主流方案进行优缺点分析;然后结合现实,给出了常见应用领域;最后对动作捕捉技术面临难题进行总结并介绍了发展趋势。 关键词:动作捕捉;虚拟技术;人机交互;算法 Overview of Human gesture capture XXX (Dalian university of technology College of Software,Liaoning Dalian 116600)Abstract:The human body gesture capture technology in human-computer interaction and virtual reality and other areas of importance is day by day prominent, in order to meet people for high accuracy, high efficiency of motion capture technology needs, scientists from all aspects of innovative attempt. This paper introduces the motion capture technology development history, and gives the concept and basic composition; And expounds the current situation of the development at home and abroad; Secondly detail schemes to mainstream advantages and disadvantages analysis; And then combining with reality, gives the common application fields; Finally, the motion capture technology difficulties was summarized and introduced the development trend. Key words: Motion capture ;Virtual technology;Human-computer interaction;Algorithm
动作识别与行为理解综述
_________________________ 基金项目: 国家自然科学基金资助项目(60673189) 收稿日期: 2008-11-28 改回日期:2008-12-03 第一作者简介: 1940.现为普适计算教育部重点实验室,清华大学计算机系人机交互与媒体集成研究所教授,博士生导师。 目前他的主要研究领域为计算机视觉,人机交互,普适计算计算技术。IEEE 高级会员,CCF 会员。 动作识别与行为理解综述 徐光祐 曹媛媛 普适计算教育部重点实验室 清华大学计算机科学与技术系 北京,100084) 摘 要 随着“以人为中心计算”的兴起和生活中不断涌现的新应用,动作识别和行为理解逐渐成为计算机视觉领域的研究热点。本文主要从视觉处理的角度分析了动作识别和行为理解的研究现状,从行为的定义和表示、运动特征的提取和动作表示以及行为理解的推理方法三个方面对目前的工作做了分析和比较。并且指出了目前这些工作面临的难题和今后的研究方向。 关键词 以人为中心 动作识别 行为理解 中图法分类号:TP391 文献标识码:A Action Recognition and Activity Understanding: A Review XU Guangyou, CAO Yuanyuan (Key Lab of Pervasive Computing, Ministry of Education, Department of Computer Science and Technology, Tsinghua University, Beijing 100084, China) Abstract As the “Human-centered computing ” is getting more and more popular and novel applications are coming up, action recognition and activity understanding are attracting researcher s’ attention in the field of computer vision. In this paper, we give a review of the state in art of work on action and activity analysis, but focus on three parts: Definition of activity, low-level motion features extraction and action representation, and reasoning method for activity understanding. Furthermore, open problems for future research and potential directions are discussed. Keywords human-centered computing, action recognition, activity understanding 引言 计算正渗透和影响到人们生活的各个方面,根据传感器数据来识别和理解人的动作和行为就成为未来”以人为中心的计算”(Human-centered computing)中的关键[1]。其中基于视觉的动作识别和行为理解尤为重要。因为在人之间的人际(interpersonal )交互过程中,视觉是最重要的信息。视觉可以帮助人们迅速获得一些关键特征和事实,如对方的表情、手势、体态和关注点等,这些视觉线索综合起来反映了对方的态度,潜在意图和情绪等信息。未来人机交互和监控中,机器要感知人的 意图很大程度上就需要依靠视觉系统。此外,视觉传感器体积小、被动性和非接触式的特点,使得视觉传感器和视觉信息系统具备了无所不在的前提。近年来,在对计算机视觉提出的层出不穷的新要求中,行为理解是一个具有挑战性的新课题,在诸如智能家居,老年人看护,智能会议室等应用中都起着至关重要的作用。它要解决的问题是根据来自传感器(摄像机)的原始图像(包括图像序列)数据,通过视觉信息的处理和分析,识别人体的动作,并在上下镜(context)信息的指导下,理解人体动作的目的、所传递的语义信息。行为理解作为近几年开始兴起的研究,正在逐渐获得越来越多的关注。 人体检测、定位以及人体的重要部分(头部,
基于骨骼数据的人体行为识别分析
基于骨骼数据的人体行为识别 摘要 人体动作姿态识别是计算机视觉研究领域中最具挑战的研究方向,是当前的研究热点。对人体动作姿态进行自动识别将带来一种全新的交互方式,通过身体语言即人体的姿态和动作来传达用户的意思,如在机场、工厂等喧闹的环境下,采用手势、动作姿态识别等人机交互技术能够提供比语音识别更加准确的信息输入。总之,在智能监控、虚拟现实、感知用户接口以及基于内容的视频检索等领域,人体动作姿态的识别均具有广泛的应用前景。该文首先简单介绍了人体动作姿态序列的分割,然后对人体动作姿态识别的方法进行了分类介绍,并对一些典型的算法的研究进展情况及其优缺点进行了重点介绍。 关键词:人体动作姿态识别; 人工智能; 隐马尔可夫模型; 动态贝叶斯网络; 模板匹配前言 人体姿态识别是计算机视觉的一个重要研究方向,它最终目的是输出人的整体或者局部肢体的结构参数,如人体轮廓、头部的位置与朝向、人体关节点的位置或者部位类别。姿态识别的研究方法应该说,几乎涵盖了计算机视觉领域所有理论与技术,像模式识别、机器学习、人工智能、图像图形、统计学等。到目前为止,已经有众多识别方法被提出,并且也取得了许多重要的阶段性的研究成果,但是以往的方法都是基于普通光学图像,比如常见的RGB 图像,这类图像容易受光照、阴影等外界变化的影响,尤其在环境黑暗的情况下无法来识别人体姿态,并且由于人体关节自由度大,及人的体型、着装较大差异性,常导致姿态识别系统识别率低。尽管有研究者利用多个摄像机获取采集的图像来获取人体深度信息以克服以上问题[1],但是该类方法恢复的深度信息不是唯一的,而且计算量非常大,尤其是这种方法要求事先用人工对传感设备进行标定,而在选取场景中的标定物时,往往又会遇到实际环境操作困难的问题。 随着光电技术的快速发展,深度传感设备的成本逐渐降低,人们获取深图像的途径及方法也越来越多。该方向的研究也逐渐成为计算机视觉领域的研究趋势。具体原因包括:一方面,深度传感设备不仅操作简单,并且极大简化了普通摄像机的标定过程;另一方面,得到的深度图像由于直接包含了人体的深度信息,能够有效的克服普通光学图像遇到的上述问题。到目前为止,较有影响力的基于该类图像的人体姿态识别算法,应该是 Shotton 等人利用一种深度传感器 Kinect 来实时捕捉人体运动的算法,该算法虽然能够满足人们对识别系统实时性的要求,但其对硬件要求特别高,并且不适合低分辨率图像中的人体关节点提取,容易导致人体骨架扭曲。下文将具体陈述人体运动分析的主要用途和前人在不同时期对这些难题的处理办法。 主题 基于计算机视觉的人体运动分析不仅在智能监控、人机交互、虚拟现实和基于内容的视频检索等方面有着广泛的应用前景,更是成为了未来研究的前瞻性方向之一。Gavrila 总结了它的一些主要应用领域[2,3,4],下面据此对其典型应用做出进一步的介绍。 智能监控(Smart Surveillance) 所谓“智能监控”是指监控系统能够监视一定场景中人的活动,并对其行为行分析和识别,跟踪其中的可疑行为(例如在一些重要地点经常徘徊或者人流密集的场合下突发的人群拥挤等状况)从而采取相应的报警措施。智能监控系统应用最多的场合来自于那些对安全
模式识别及其在图像处理中的应用
武汉理工大学 模式识别及其在图像处理中的应用 学院(系):自动化学院 课程名称:模式识别原理 专业班级:控制科学与工程1603班 任课教师:张素文 学生姓名:王红刚 2017年1月3日
模式识别及其在图像处理中的应用 摘要:随着计算机和人工智能技术的发展,模式识别在图像处理中的应用日益广泛。综述了模式识别在图像处理中特征提取、主要的识别方法(统计决策法、句法识别、模糊识别、神经网络)及其存在的问题, 并且对近年来模式识别的新进展———支持向量机与仿生模式识别做了分析和总结, 最后讨论了模式识别亟待解决的问题并对其发展进行了展望。 关键词:模式识别;图像处理;特征提取;识别方法 Pattern Recognition and Its Application in Image Processing Abstract:With the development of computer and artificial intelli-gence , pattern recognition is w idely used in the image processing in-creasingly .T he feature extraction and the main methods of pattern recognition in the image processing , w hich include statistical deci-sion, structural method , fuzzy method , artificial neural netw ork aresummarized.T he support vector and bionic pattern recognition w hich are the new developments of the pattern recognition are also analyzed .At last, the problems to be solved and development trends are discussed. Key words:pattern recognition ;image processing ;feature extrac-tion;recognition methods
计算机视觉+人体姿态识别+双目视觉
Computer vision application 院(系)电子与信息工程学院 专业集成电路工程 学生吕广兴14S158054
Computer vision application The directory Report: Computer vision application (2) 1.The object of the project (2) 2.The method and the principle applied to the project (2) 2.1 Platform (2) 2.2 The principle of transform the RGB image to the gray image (2) 2.3 The principle of image enhancement (2) 2.4 The principle of thresholding (3) 2.5 The principle of classifier (3) 3.The content and the result of the project (4) 3.1 The main steps in the project (4) 3.2 About human body posture recognition (4) About three kinds of methods are most common: (4) 3.3.Stereo vision (11) 4.Reference (18)
Report: Computer vision application 1.The object of the project The object of the project is Gesture recognition and location in the interior of people. 2.The method and the principle applied to the project 2.1 Platform The platform is based on Visual Studio 2012 and OpenCV 2.4.10. 2.2 The principle of transform the RGB image to the gray image There are three major methods to transform the RGB image to the gray image. The first one is called the maximum value that is set the value of R, G, and B to the maximum of these three. Gray=R=G=B=max(R, G, B) The second one is called mean value which is set the value of R, G, and B to the mean value of these three. Gray=R=G=B=(R+G+B)/3 The third one is called weighted average that is giving different weights to the R, G and B according to the importance or other indicators, and then adding the three parts together. In fact, human’s eye is very high se nsitive to green, then red, last blue. Gray=0.30R+0.59G+0.11B 2.3 The principle of image enhancement Image enhancement is the process of making images more useful. There are two broad categories of image enhancement techniques. The first one is spatial domain technique, and it is a direct manipulation of image pixels that includes point processing and neighborhood operations. The second one is frequency domain technique, and it is a manipulation of Fourier transform or wavelet transform of an image. The principle of the median filter is to replace the value of a pixel by the median of the gray levels in the neighborhood of that pixel(the original value of the pixel is included in the computation of the median). It forces the points with distinct gray levels to be more like their neighbors.
基于视频的人体姿态检测方法及其应用综述
2019.09 1 研究现状与技术发展趋势 1.1单人骨架检测方法 人体骨架关键点对于描述人体姿态和预测人体行为 十分重要,它是很多计算机视觉技术的基础步骤,例如行为预测,智能监控等方面。近年来,随着人体骨架关键点检测效果的不断提升,开始比较广泛地应用于计算机视觉的相关工作中,其中,单人骨架检测是这些工作的入门基础和最简单的实践任务。1.2姿态卷积网络 姿态卷积网络(Convolutional Pose Machines,简称 CPM [1])由一系列卷积网络组成,这些卷积网络重复产 生每个部分位置的2D 置信图(2D belief maps,表征对整体骨架的一种预测),在CPM 的每个阶段,把图像特征和上一阶段所产生的置信图作为输入。为后续的阶段提供每个部分位置的空间不确定性的非参数编码,它不是通过几何处理[2][3]或者专门的后续处理步骤[4]来显性地处解析这种置信图,而是通过隐式直接从数据中学习图像和附近区域特征的方式。 同时使用多阶段监督,来避免网络过深而导致的梯度丢失导致无法进行优化 [5][6] ,除了第一阶段之外(因为 第一阶段网络的作用是预测初步的结果),对于每个阶段的预测输出都要进行监督训练从而通过反向传播来对上一个阶段的与猜测结果进行一定程度的优化和修正。 两层之间损失函数如下(见式1,式2),其中f 为 每层的损失函数,F 为总损失函数[1] : (1) (2) CPM 的流程图如图1所示。 1.3多情景关注机制 在关注一张图片的时候,通过关注图片的不同尺度 可以更准确地对人体姿态进行分析,一些局部信息,对于比如脸部、肘部的特征判断很有必要,而最终的整体姿态需要对人体进行整体理解,不同的尺度下分析可能体现更多信息,比如人体的动作,相邻节点的关系等,这是多情景关注机制的理论基础。 作者简介:连仁明(1979-),男,本科,工程师,研究方向:计算机技术。收稿日期:2019-06-14 基于视频的人体姿态检测方法及其应用综述 连仁明,刘颖,于萍,刘畅 (91001部队,北京100841) 摘 要:视觉是人类认知周围事物的重要感知。随着人类对于视频处理需求的不断增长和计算机性能 与技术的不断发展,我们希望计算机能够在特定场合具有部分与人类视觉类似的视觉功能,并且对于这种视觉功能有一定的初步处理和判断功能。基于视频的人体姿态检测是理解和识别人与人的交互运动,人与环境交互关系等。通过相关的计算机技术,实现对于视频中人的检测,动作分析以及涉及多人交互情境下的行为分析和关系分析等。尽管这种分析对于人的视觉以及大脑似乎是一件不算困难的任务,但是随着时代的发展,视频的规模不断扩大,仅仅依靠人眼进行识别是远远不能够满足现代社会需要,通过计算机技术进行识别已经成为一种必然需求。但对于计算机而言,因为涉及到背景环境的复杂性,人类体态的多样性,运动习惯的差异性,在视频中能够较为精确检测人的位置,分析人的行为还是一件比较有挑战性的任务。关键词:人体检测;计算机识别技术;情景 图1CPM 方法卷积网络流程以及效果示例 [1] 127
固定场景下的人体姿态识别
2018.11收稿日期:2018-08-15 当前人工智能技术的热点,固定情景下对于人体姿态识别具有十分重要的研究意义,对于我国实现现代化建设具有一定的推动作用,因此加强技术分析研判就显得十分重要。早在上世纪70年代,我国已经开始了对人体行为分析方面的研究,这些研究对于我国人工智能的发展有了较强的推动作用,在特定情景下或者说在比较标准的场景中分析较为简单的姿态和动作已经成为了可能,但这些工作的开展大多停留在理论的层次,并没有付诸实践,要想将这些分析技术真正应用到实际场景中仍然需要大量的实验进行探索。 1人体姿态识别 人体姿态识别主要在于研究描述人体姿态以及预测人体行为,其识别过程是指,在指定图像或视屏中,根据人体中关节点位置的变化,识别人体动作的过程。人体姿态识别的算法主要分为两类,一是基于深度图的算法,另一类直接基于RGB图像的算法。深度图是指由相机拍摄的图片,其每个像素值代表的是物体到相机XY平面的距离。这种算法的应用容易因采集设备的要求而受限,但基于RGB图像的算法直接通过对红、绿、蓝3个颜色通道的变化以及它们相互之间的叠加来得到的颜色进行识别,不会受到其他因素的干扰限制,因此更具有发展前景,同时也取得了不少成果。目前,即使是在较为复杂的、某种固定的场景中,基于RGB图像的人体姿态估计算法相较于基于深度图的人体姿态估计算法也能达到很好的识别效果。无论是深度图技术还是RGB图像技术,都是通过计算机强大的运算能力进行人体姿态的动作预算,通过这样的方式能够一定程度地实现人工图像的监测,并且能够为人工智能的普及奠定良好的基础。随着我国社会水平的不断提升,人们对于社会生活的质量要求也在不断增加,因此在实际的生活过程中视频监控已经成为人们不可或缺的一种安全措施,基于视频分析的技术要求也越来越高。例如在智能家装,医疗领域及运动分析等行业中都得到了较为广泛的应用,固态场景下的人体姿态识别在各领域起到的作用显而易见。特别是近年来,我国安保工作的加强,对于大城市人口密集流动以及犯罪分子的甄别等都有较强需求。 2人体姿态识别的实现 人体姿态是被主要分为基于计算机视角的识别和基于运动捕获技术的识别。基于计算机视觉的识别主要通过各种特征信息来对人体姿态动作进行识别,比如视频图像序列、人体轮廓、多视角等。基于计算机视觉的识别可以比较容易获取人体运动的轨迹、轮廓等信息,但没有办法具体实现表达人体的运动细节,以及容易存在因遮挡而识别错误等问题。基于运动捕获技术的人体姿态识别,则是通过定位人体的关节点、储存关节点运动数据信息来识别人体运动轨道。相较于计算机视角的人体姿态识别,基于运动捕获技术的人体姿态识别可以更好地反应人体姿态信息,也可以更好地处理和记录运动细节,不会因为物体颜色或被遮挡而影响运动轨道的识别。技术的革新对人体姿态的分析捕捉有较强的辅助作用,并且能够更好地展现动作的细节,对于专业人士进行动作分析的痕迹管理有较高的参考价值。通过良好的运动前景预算能够,在各种计算方法中做出合理的预测,并且在各种环境中的适应能力也能够得到一定程度的加强。由于未来的监控实现的方向是在全领域的视频监控,因此对于用户的特定化要求也应该及时进行技术革新,用户对于技术的需求就是技术革新的发展方向, 固定场景下的人体姿态识别 赵一秾 (辽宁科技大学,辽宁鞍山114000) 摘要:近年来,随着信息技术的发展和智能科技的普及,全球科技变革正在进一步推进,云计算、物联网、大数据和人工智能等技术也在飞速发展,其中,人体姿态识别技术已开始在计算机视觉相关领域中广泛应用。就固定场景下的人体姿态识别做出研究分析。 关键词:人体姿态识别;云计算;人工智能 150
模式识别理论的研究与应用
模式识别理论的研究与应用 摘要:通过对模式识别系统的简要评述,对近年来几种基本的模式识别方法进行了总结,并对模式识别在字符识别方面的应用原理作了介绍。字符识别技术属于模式识别的范畴,本文首先介绍模式识别的基本理论和基本方法,然后阐述了模式识别技术在光学识别技术上的应用,并将其应用到角铁字符识别系统上。实践证明,采用模式识别!能减轻人工操作的复杂性和失误。 关键字:字符识别;模式识别;凹凸字符;OCR(光学字符识别);特征抽取Research and Application of Pattern Recognition Theory Abstract:In this paper components of pattern recognition system were introduced. Several basic patternrecognition methods which were frequently utilized are summed up. Finally Chinese character recognition whichis a application of pattern recognition were introduced.Character recognition technology belongs to the category of pattern recognition, this paper first introduce the basic theory and basic methods of pattern recognition, and then expounds the application of pattern recognition technology in optical recognition technology! And apply it to the Angle iron character recognition system. Practice has proved that using pattern recognition! To reduce the complexity of manual operation and failure. KeyWord:Character Recognition;Pattern Recognition;Protuberant Characters;Optical Character Recognition;Feature Extraction