A formal derivation of Heaps ’ Law F.A. Grootjen and D.C. van Leijenhorst and Th. P. van
M,

uz ¯)u = ¯ = (∂/∂ z
We are mostly concerned with contractible solutions, u : T2 → M is contractible. (2)
Equation (1) linearized about a conFredholm operator. Essentially for this reason for a typical f the equation has a finite number of solutions. Our goal is to estimate this number from below by investigating the manifold formed by all pairs (u, f ) where u solves (1), (2) with f = f .
corrupt representation
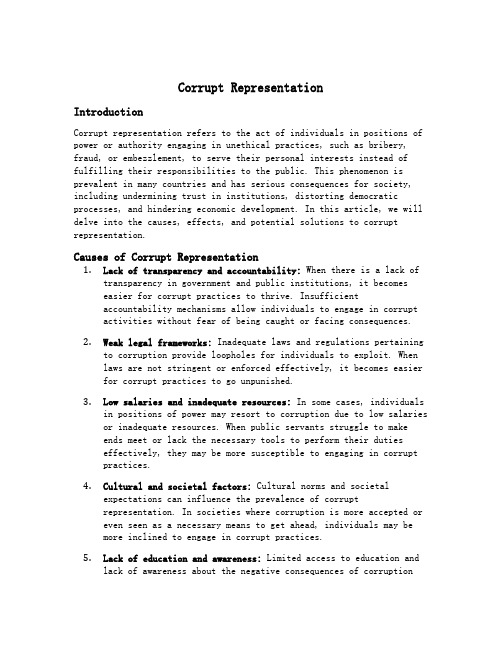
Corrupt RepresentationIntroductionCorrupt representation refers to the act of individuals in positions of power or authority engaging in unethical practices, such as bribery, fraud, or embezzlement, to serve their personal interests instead of fulfilling their responsibilities to the public. This phenomenon is prevalent in many countries and has serious consequences for society, including undermining trust in institutions, distorting democratic processes, and hindering economic development. In this article, we will delve into the causes, effects, and potential solutions to corrupt representation.Causes of Corrupt Representationck of transparency and accountability: When there is a lack oftransparency in government and public institutions, it becomeseasier for corrupt practices to thrive. Insufficientaccountability mechanisms allow individuals to engage in corruptactivities without fear of being caught or facing consequences. 2.Weak legal frameworks: Inadequate laws and regulations pertainingto corruption provide loopholes for individuals to exploit. Whenlaws are not stringent or enforced effectively, it becomes easier for corrupt practices to go unpunished.3.Low salaries and inadequate resources: In some cases, individualsin positions of power may resort to corruption due to low salaries or inadequate resources. When public servants struggle to makeends meet or lack the necessary tools to perform their dutieseffectively, they may be more susceptible to engaging in corruptpractices.4.Cultural and societal factors: Cultural norms and societalexpectations can influence the prevalence of corruptrepresentation. In societies where corruption is more accepted or even seen as a necessary means to get ahead, individuals may bemore inclined to engage in corrupt practices.ck of education and awareness: Limited access to education andlack of awareness about the negative consequences of corruptioncan contribute to its perpetuation. When individuals are noteducated about the detrimental effects of corruption, they may not fully understand the importance of ethical behavior in positionsof power.Effects of Corrupt Representation1.Undermining trust in institutions: Corrupt representation erodespublic trust in government and public institutions. Whenindividuals in positions of power are perceived as corrupt,citizens lose faith in the ability of these institutions to serve their interests and uphold the rule of law.2.Distorting democratic processes: Corruption in representation candistort democratic processes by influencing elections,manipulating public opinion, and impeding the fair and equalrepresentation of citizens’ interests. This undermines theprinciples of democracy and erodes the legitimacy of electedofficials.3.Hindering economic development: Corruption diverts public fundsaway from essential services and infrastructure projects,hindering economic development. When resources that should beallocated for public welfare are siphoned off through corruptpractices, it leads to a lack of investment in critical sectors,perpetuating poverty and inequality.4.Encouraging a culture of corruption: Corrupt representation canperpetuate a culture of corruption, where unethical behaviorbecomes normalized and expected. This makes it increasinglydifficult to break the cycle of corruption and establish a culture of integrity and transparency.Solutions to Corrupt Representation1.Strengthening legal frameworks: Governments should enact andenforce comprehensive anti-corruption laws that address thevarious forms of corrupt representation. These laws should include provisions for the investigation, prosecution, and punishment ofcorrupt individuals, as well as protection for whistleblowers.2.Enhancing transparency and accountability: Governments and publicinstitutions should prioritize transparency and accountability in their operations. This can be achieved through measures such asthe disclosure of financial information, the establishment ofindependent oversight bodies, and the implementation of effective monitoring mechanisms.3.Promoting education and awareness: Investing in education andraising awareness about the consequences of corruption are crucial in combating corrupt representation. By promoting ethical valuesand integrity from an early age, societies can foster a culturethat rejects corruption and values honesty and accountability.4.Strengthening international cooperation: Corruption is a globalissue that requires international cooperation. Governments should collaborate with international organizations and other countriesto share best practices, exchange information, and coordinateefforts in combating corrupt representation.5.Encouraging citizen participation: Engaging citizens in decision-making processes and encouraging their active participation canhelp prevent corrupt representation. By promoting transparency,citizen oversight, and public engagement, governments can ensurethat the interests of the public are represented and thatcorruption is less likely to occur.ConclusionCorrupt representation poses significant challenges to societies around the world. By understanding the causes and effects of corruption, as well as implementing effective solutions, governments and citizens can work together to combat this pervasive issue. Strengthening legal frameworks, promoting transparency and accountability, investing in education, fostering international cooperation, and encouraging citizen participation are all crucial steps in creating a society that values integrity and rejects corrupt representation.。
09第九讲 联言、选言命题及其推理
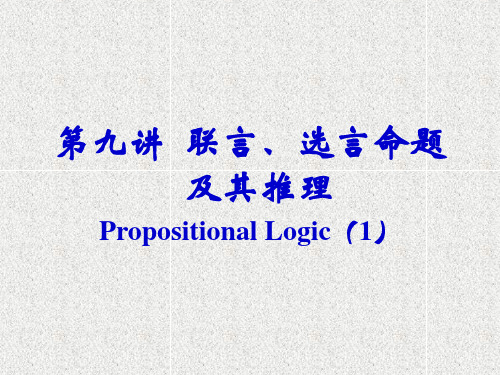
**(2004年B类)81. 甲、乙、丙、丁四人的 血型各不相同,甲说:“我是A型。”乙说: “我是O型。”丙说:“我是AB型。”丁说: “我不是AB型。”四个人中只有一个人的话 是假的。 以下哪项成立? ( ) A. 无论谁说假话,都能推出四个人的血型情况。 B. 乙的话假,可推出四个人的血型情况。 C. 丙的话假,可推出四个人的血型情况。 D. 丁的话假,可推出四个人的血型情况。 【答案】B
1)张小平或者犯杀人罪,或者犯抢劫罪; 张小平没有犯杀人罪; 所以张小平犯抢劫罪。 *2)张小平或者犯杀人罪,或者犯抢劫罪; 张小平犯杀人罪; 所以张小平没有犯抢劫罪。 3)克林顿是美国总统, 所以克林顿或者是美国总统,或者是美 国国务卿。 ******
4)鱼,我所欲也,熊掌亦我所欲也;二者不 可得兼,舍鱼而取熊掌者也。生亦我所欲 也,义亦我所欲也,二者不可得兼,舍生 而取义者也。(孟子《告子章句上》) 或者要鱼,或者要熊掌,二者不可得兼; 不要鱼, 所以要熊掌。 5)要么为玉碎,要么为瓦全; 宁为玉碎, 所以,不为瓦全。 ******
1、定义:断定事物若干种可能情况(至少有 一种存在)的命题。相容与不相容 2、构成:选言肢(disjuncts),联结词。 3、相容选言命题:( inclusive disjunction) 1) 常用联结词:或者,可能…也可能,或许, 也许。 2) 逻辑形式:p或者q p∨q(析取式) 3)逻辑性质:当且仅当选言肢都假,相容选言 命题假。 选言肢不可同假(选言),可以同真(相容)。 ******
• the truth value of the compound proposition is completely determined by the truth values of its components. • A truth table is an arrangement of truth values that shows in every possible case how the truth value of a compound proposition is determined by the truth values of its simple components. • ******
LABORATORY MANUAL 9.1 SPRING CONSTANT说明书

208PROJECT9 PointerAHanger HHelicalspringLoad (m)Rigid support12345SPFig. P 9.1:Measurement of extension ofa helical spring due to a load(P 9.1)A IMTo study of the spring constant of a helical spring from itsload-extension graph.A PPARATUS AND MATERIAL REQUIREDHelical spring with a pointer attached at its lower end and a hook/ring for suspending a hanger; a rigid support/clamp stand; five or sixslotted masses (known) for hanger; a metre scale.P RINCIPLEWhen an external force is applied to a body,the shape of the body changes or deformationoccurs in the body. Restoring forces (havingmagnitude equal to the applied force)are developed within the body which tendto oppose this change. On removingthe applied force, the body regains itsoriginal shape.For small changes in length (or shape/dimensions) of a body (wire), within the elasticlimit, the magnitude of the elongation orextension is directly proportional to the appliedforce (Hooke’s law).Following Hooke’s law, the spring constant (orforce constant) of a spring is given bySpring constant, =Restoring force,Extension,FKxThus, the spring constant is the restoring force per unit extension inthe spring. Its value is determined by the elastic properties of the spring.A given load is attached to the free end of the spring which is suspendedfrom a rigid point support (a nail fixed to a wall). A load (slotted weight)is placed in the hanger and the spring gets extended/elongated dueto the applied force. By measuring the extensions, produced by theforces applied by different loads (slotted mass) in the spring and209PROCEDURE1.Suspend the helical spring, SA, having a pointer, P, at itslower free end, A, freely from a rigid point support, as shown in Fig. P 9.1.2.Set the metre scale close to the spring vertically. Take care thatthe pointer moves freely over the scale without touching it and the tip of the pointer is in front of the graduations on the scale.3.Find out the least count of the metre scale. It is usually 1 mm or0.1 cm.4.Record the initial position of the pointer on the metre scale,without any slotted mass suspended from the hook.5.Suspend the hanger, H (of known mass, say 20 g) from the lowerfree end, A, of the helical spring and record the position of the pointer, P on the metre scale.6.Put a slotted mass on the hanger gently. Wait for some time forthe load to stop oscillating so as to attain equilibrium (rest)position, or even hold it to stop. Record the position of the pointer on the metre scale. Record observations in a table with proper units and significant figures.7.Put another slotted mass on the hanger and repeat Step 6.8.Keep on putting slotted masses on the hanger and repeat Step 6.Record the position of the pointer on the metre scale every pute the load/force F ( = mg ) applied by the slotted mass,M and the corresponding extension (or stretching), x in the helical spring. Here g is the acceleration due to gravity at the place of the experiment.10.Plot a graph between the applied force F along x-axis and thecorresponding extension x (or stretching) on the y-axis. What is the shape of the curve of the graph you have drawn?11.If you find that the force-extension graph is a straight line, findthe slope (F /x ) of the straight line. Find out the spring constant K of helical spring from the slope of the straight line graph.OBSERVATIONSLeast count of the metre scale= ... mm= ... cm Mass of the hanger = ... g210Mean Spring constant K = ... N/mPlotting load - extension graph for a helical springTake force, F along the x-axis and extension, x along the y-axis.Choose suitable scales to represent F and x . Plot a graph between F and x (as shown in Fig. P 9.2). Identify the shape of the load-extension graph OA.CALCULATIONSChoose two points, O and A, wide apart on the straight line OA obtained from load extension graph, as shown in Fig. P 9.2. Fromthe point A, draw a perpendicular AB on x-axis. Then, from the graph,Slope of the straight line graph = tan θ =ABOB= x /F Spring constant, K = Fx=1(slope of the graph)Spring constant, −===−–1B O A BOB ...Nm AB F F K x x where x A and x B are the corresponding extensions at points A and B (or O) respectively where F B and F O are the loads (forces) at points B and O.yOxAE x t e n s i o n (m )F (N)BS.No.Mass suspended from the spring, MForce,F = mgPosition of the pointerExtension,x Springconstant, K(= F /x )(10–3 kg)(N)(cm)(10–2 m)(N m –1)102203.4.5.6.........Fig. P 9.2: Load-extension graphfor a helical spring211R ESULTThe spring constant of the given helical spring = ... Nm –1PRECAUTIONS1.The spring should be suspended from a rigid support and itshould hang freely so that it remains vertical.2.Slotted weights should be chosen according to elastic limit ofthe spring.3.After adding or removing the slotted weight on the hanger, waitfor sometime before noting the position of the pointer on the scale because the spring takes time to attain equilibrium position.SOURCES OF ERROR1.If support is not perfectly rigid, some error may creep in due tothe yielding of the support.2.The slotted weights may not be standard weights.DISCUSSION1. A rigid support is required for suspending the helical spring withload (or slotted mass) from it. The slotted masses may not have exact values engraved on them. Some error in the extension is likely to creep in due to the yielding (sometimes) of the support and inaccuracy in the values of the masses of loads.2.The accuracy of the result depends mainly on the measurementof extension produced by the force (load) within the elastic limit.Take special care that the slotted mass is put gently on the hanger as the wire of the helical spring takes sometime to attain its new-equilibrium position.3.If the elastic limit is crossed slightly, what changes will you expectin the spring and your result?SELF ASSESSMENT1.Two springs A (of thicker wire) and B (of thinner wire) of the samematerial, loaded with the same mass on their hangers, are suspended from a rigid support. Which spring would have more value of spring constant?2.Soft massive spring of mass M s and spring constant K hasextension under its own weight. What mass correction factor for212attached at its lower end would be =+sm()()2MF gX MK K]3.What other factors affect spring constant, e.g. length.SUGGESTED ADDITIONAL EXPERIMENTS/ACTIVITIES1.Take spring of the same material but of different diameters of thewires. See how the spring contant varies.2.Take springs of the same diameters of the wires but of differentmaterials. See how the spring constant varies. What inference doyou draw from your result?。
电子工程师招聘题目
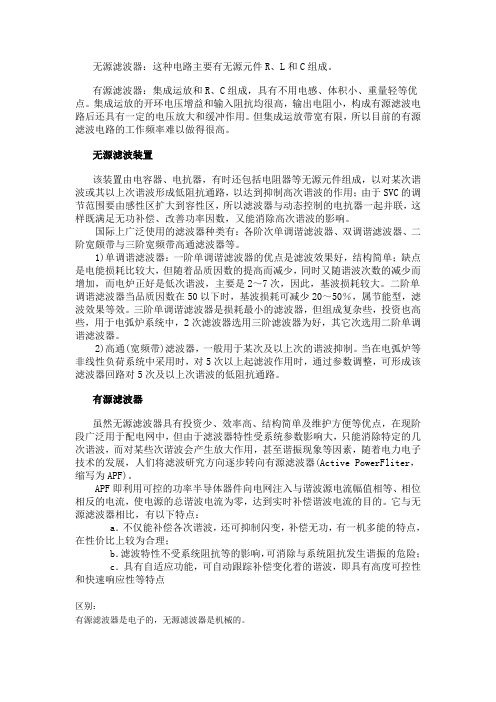
无源滤波器:这种电路主要有无源元件R、L和C组成。
有源滤波器:集成运放和R、C组成,具有不用电感、体积小、重量轻等优点。
集成运放的开环电压增益和输入阻抗均很高,输出电阻小,构成有源滤波电路后还具有一定的电压放大和缓冲作用。
但集成运放带宽有限,所以目前的有源滤波电路的工作频率难以做得很高。
无源滤波装置该装置由电容器、电抗器,有时还包括电阻器等无源元件组成,以对某次谐波或其以上次谐波形成低阻抗通路,以达到抑制高次谐波的作用;由于SVC的调节范围要由感性区扩大到容性区,所以滤波器与动态控制的电抗器一起并联,这样既满足无功补偿、改善功率因数,又能消除高次谐波的影响。
国际上广泛使用的滤波器种类有:各阶次单调谐滤波器、双调谐滤波器、二阶宽颇带与三阶宽频带高通滤波器等。
1)单调谐滤波器:一阶单调谐滤波器的优点是滤波效果好,结构简单;缺点是电能损耗比较大,但随着品质因数的提高而减少,同时又随谐波次数的减少而增加,而电炉正好是低次谐波,主要是2~7次,因此,基波损耗较大。
二阶单调谐滤波器当品质因数在50以下时,基波损耗可减少20~50%,属节能型,滤波效果等效。
三阶单调谐滤波器是损耗最小的滤波器,但组成复杂些,投资也高些,用于电弧炉系统中,2次滤波器选用三阶滤波器为好,其它次选用二阶单调谐滤波器。
2)高通(宽频带)滤波器,一般用于某次及以上次的谐波抑制。
当在电弧炉等非线性负荷系统中采用时,对5次以上起滤波作用时,通过参数调整,可形成该滤波器回路对5次及以上次谐波的低阻抗通路。
有源滤波器虽然无源滤波器具有投资少、效率高、结构简单及维护方便等优点,在现阶段广泛用于配电网中,但由于滤波器特性受系统参数影响大,只能消除特定的几次谐波,而对某些次谐波会产生放大作用,甚至谐振现象等因素,随着电力电子技术的发展,人们将滤波研究方向逐步转向有源滤波器(Active PowerFliter,缩写为APF)。
APF即利用可控的功率半导体器件向电网注入与谐波源电流幅值相等、相位相反的电流,使电源的总谐波电流为零,达到实时补偿谐波电流的目的。
电子电路工程师面试题
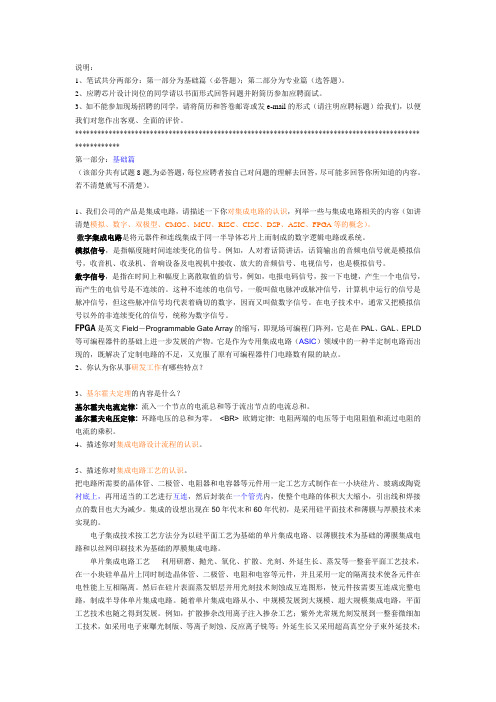
说明:1、笔试共分两部分:第一部分为基础篇(必答题);第二部分为专业篇(选答题)。
2、应聘芯片设计岗位的同学请以书面形式回答问题并附简历参加应聘面试。
3、如不能参加现场招聘的同学,请将简历和答卷邮寄或发e-mail的形式(请注明应聘标题)给我们,以便我们对您作出客观、全面的评价。
******************************************************************************************** ************第一部分:基础篇(该部分共有试题8题,为必答题,每位应聘者按自己对问题的理解去回答,尽可能多回答你所知道的内容。
若不清楚就写不清楚)。
1、我们公司的产品是集成电路,请描述一下你对集成电路的认识,列举一些与集成电路相关的内容(如讲清楚模拟、数字、双极型、CMOS、MCU、RISC、CISC、DSP、ASIC、FPGA等的概念)。
数字集成电路是将元器件和连线集成于同一半导体芯片上而制成的数字逻辑电路或系统。
模拟信号,是指幅度随时间连续变化的信号。
例如,人对着话筒讲话,话筒输出的音频电信号就是模拟信号,收音机、收录机、音响设备及电视机中接收、放大的音频信号、电视信号,也是模拟信号。
数字信号,是指在时间上和幅度上离散取值的信号,例如,电报电码信号,按一下电键,产生一个电信号,而产生的电信号是不连续的。
这种不连续的电信号,一般叫做电脉冲或脉冲信号,计算机中运行的信号是脉冲信号,但这些脉冲信号均代表着确切的数字,因而又叫做数字信号。
在电子技术中,通常又把模拟信号以外的非连续变化的信号,统称为数字信号。
FPGA是英文Field-Programmable Gate Array的缩写,即现场可编程门阵列,它是在PAL、GAL、EPLD 等可编程器件的基础上进一步发展的产物。
它是作为专用集成电路(ASIC)领域中的一种半定制电路而出现的,既解决了定制电路的不足,又克服了原有可编程器件门电路数有限的缺点。
化学化工英语试题及答案
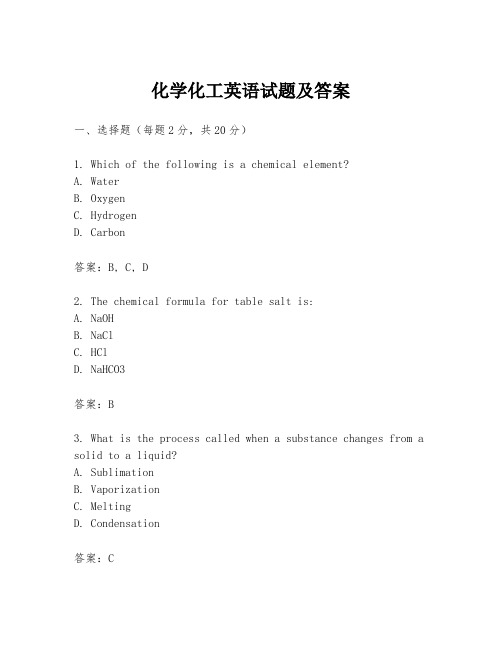
化学化工英语试题及答案一、选择题(每题2分,共20分)1. Which of the following is a chemical element?A. WaterB. OxygenC. HydrogenD. Carbon答案:B, C, D2. The chemical formula for table salt is:A. NaOHB. NaClC. HClD. NaHCO3答案:B3. What is the process called when a substance changes from a solid to a liquid?A. SublimationB. VaporizationC. MeltingD. Condensation答案:C4. In the periodic table, which group contains alkali metals?A. Group 1B. Group 2C. Group 17D. Group 18答案:A5. What is the name of the process where a substance decomposes into two or more substances due to heat?A. CombustionB. OxidationC. ReductionD. Decomposition答案:D6. Which of the following is a physical property of a substance?A. ColorB. TasteC. SolubilityD. Reactivity答案:A7. What is the term for a compound that releases hydrogen ions (H+) when dissolved in water?A. BaseB. AcidC. SaltD. Neutral答案:B8. The law of conservation of mass states that in a chemical reaction:A. Mass is lostB. Mass is gainedC. Mass remains constantD. Mass can be converted into energy答案:C9. Which of the following is a type of chemical bond?A. Ionic bondB. Covalent bondC. Hydrogen bondD. All of the above答案:D10. What is the name of the process where a substance absorbs energy and changes from a liquid to a gas?A. MeltingB. VaporizationC. SublimationD. Condensation答案:B二、填空题(每题2分,共20分)1. The symbol for the element iron is ________.答案:Fe2. The pH scale ranges from ________ to ________.答案:0 to 143. A compound that produces a basic solution when dissolvedin water is called a ________.答案:base4. The smallest particle of an element that retains its chemical properties is called a ________.答案:atom5. The process of separating a mixture into its individual components is known as ________.答案:separation6. The study of the composition, structure, and properties of matter is called ________.答案:chemistry7. The process of a substance changing from a gas to a liquid is called ________.答案:condensation8. A(n) ________ reaction is a type of chemical reactionwhere two or more substances combine to form a single product. 答案:synthesis9. The volume of a gas at constant temperature and pressureis directly proportional to the number of ________.答案:moles10. The process of converting a solid directly into a gas without passing through the liquid phase is known as ________. 答案:sublimation三、简答题(每题10分,共30分)1. Explain what is meant by the term "stoichiometry" in chemistry.答案:Stoichiometry is the calculation of the relative quantities of reactants and products in a chemical reaction.It is based on the law of conservation of mass and involvesthe use of balanced chemical equations and the molar massesof substances to determine the amounts of reactants needed to produce a certain amount of product or the amounts ofproducts formed from a given amount of reactant.2. Describe the difference between a physical change and a chemical change.答案:A physical change is a change in the state or form of a substance without altering its chemical composition. Examples include melting, freezing, and boiling. A chemical change, on the other hand, involves a change in the chemical composition of a substance, resulting in the formation of new substances. Examples include combustion and rusting.3. What are the three main types of chemical bonds, and givean example of each.答案:The three main types of chemical bonds are ionic bonds, covalent bonds, and metallic bonds. An ionic bond is formed when electrons are transferred from one atom to another, resulting in the formation of oppositely charged ions. An example is the bond between sodium (Na) and chloride (Cl) in table salt (NaCl). A covalent bond is formed when two atoms share electrons, as seen in water (H2O) where hydrogen atoms share electrons with oxygen. Metallic bonds occur in metals, where a "sea" of delocalized electrons is shared among positively charged metal ions, as in sodium metal。
数学专业英语45753

因此,差商表示PQ与水平线的夹角a的正切。
The real number tana is called the slope of the line through P and Q and it provides a way of measuring the “steepness” of the line.
上一节描述的例子指出了介绍导数概念的方法。
We begin with a function f defined at least on some open interval (a,b) on the x-axis. 我们从一个至少定义在x轴的开区间(a,b)上的函数入 手。
Then we choose a fixed point x in this interval and introduce the difference quotient
一般地, 由f(x)产生f'(x)的极限的过程提供了一种方法, 从一个给定的函数 f 得到一个新的函数f'。这个过程 叫做微分法。 f'叫做f的一阶导数。
If f' , in turn, is defined on an open interval, we can try to compute its first derivative, denoted by f″ and called the second derivative of f.
(P67 第四段第一句话)
在直线运动中,速度的一阶导数称为加速度。
Conformal Field Theory Approach to the Kondo Effect
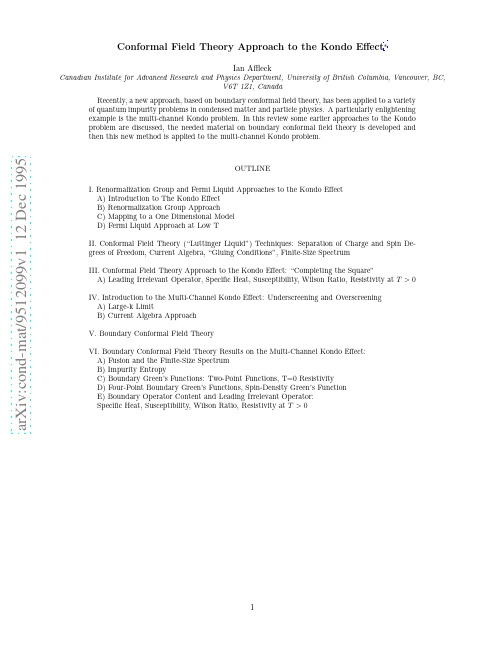
a r X i v :c o n d -m a t /9512099v 1 12 D e c 1995Conformal Field Theory Approach to the Kondo Effect ∗Ian AffleckCanadian Institute for Advanced Research and Physics Department,University of British Columbia,Vancouver,BC,V6T 1Z1,CanadaRecently,a new approach,based on boundary conformal field theory,has been applied to a variety of quantum impurity problems in condensed matter and particle physics.A particularly enlightening example is the multi-channel Kondo problem.In this review some earlier approaches to the Kondo problem are discussed,the needed material on boundary conformal field theory is developed and then this new method is applied to the multi-channel Kondo problem.OUTLINEI.Renormalization Group and Fermi Liquid Approaches to the Kondo Effect A)Introduction to The Kondo Effect B)Renormalization Group Approach C)Mapping to a One Dimensional Model D)Fermi Liquid Approach at Low TII.Conformal Field Theory (“Luttinger Liquid”)Techniques:Separation of Charge and Spin De-grees of Freedom,Current Algebra,“Gluing Conditions”,Finite-Size SpectrumIII.Conformal Field Theory Approach to the Kondo Effect:“Completing the Square”A)Leading Irrelevant Operator,Specific Heat,Susceptibility,Wilson Ratio,Resistivity at T >0IV.Introduction to the Multi-Channel Kondo Effect:Underscreening and Overscreening A)Large-k LimitB)Current Algebra Approach V.Boundary Conformal Field TheoryVI.Boundary Conformal Field Theory Results on the Multi-Channel Kondo Effect:A)Fusion and the Finite-Size Spectrum B)Impurity EntropyC)Boundary Green’s Functions:Two-Point Functions,T=0Resistivity D)Four-Point Boundary Green’s Functions,Spin-Density Green’s Function E)Boundary Operator Content and Leading Irrelevant Operator:Specific Heat,Susceptibility,Wilson Ratio,Resistivity at T >0I.RENORMALIZATION GROUP AND FERMI LIQUID APPROACHES TO THE KONDO EFFECTA.Introduction to the Kondo EffectMost mechanisms contributing to the resistivity of metals,ρ(T),give eitherρ(T)decreasing to0, as T→0(phonons or electron-electron interactions),orρ(T)→constant,as T→0(non-magnetricimpurities).However,metals containing magnetic impurities show aρ(T)which increases as T→0.This was explained by Kondo1in1964using a simple Hamiltonian:H= kαψ†α kψ kαǫ(k)+λ S· k k′ψ† k σ+...]2(1.2)THere D is the band-width,νthe density of states.This result stimulated an enormous amount oftheoretical work.As Nozi`e res put it,“Theorists‘diverged’on their own,leaving the experimentrealities way behind”.2What happens at low T,i.e.T∼T K=De−1ψ( 0,t) ,2where thefields are in the interaction picture.EFk F2D’Ek2DFIG.1.Reduction of the cut-offfrom D to D ′.As S(t )is independent of t ,we simply multiply powers of S using [S a ,S b ]=iǫabc S c ,S2=s (s +1).We must time-order S ’s which don’t commute.The first few diagrams are shown in Figure (2).In 2nd order in λ,we have:−λ22ψ(t )ψ†(t ′)σb2λ2dt dt ′ψ†σa2ψT ψ(t )ψ†(t ′) (θ(t −t ′)S a S b +θ(t ′−t )S b S a )=λ22ψ· Ssn(t −t ′) ψ(t )ψ†(t ′) ,(1.3)where sn (t −t ′)is the sign-function which arises from T -ordering spins.FIG.2.Feynman diagrams contributing to renormalization of the Kondo coupling constant to third order.We see that the integraldtǫ(t )G (t )=−idt(2π)3dωiω+δ+1ω−ǫk +iδsn(ǫk )(1.5)=d 3 k|ǫk |≈2νDD ′dǫD ′.(1.6)Thusδλ=νλ2lnDd ln D=−νλ2.(1.8)We see that lowering the band cut-offincreases λor,defining a length-dependent cut-off,l ∼v F /D ,dλ1−νλ0lnD 0νλ0,If λ0<0(ferromag-netic),λeff(D )→0.See Figure(3).effλFIG.3.RG flow of the Kondo coupling.The behaviour at temperature T is determined by λeff(T ):ρ(T )→0as T →0for the ferromag-netic case.What happens for the antiferromagnetic case?C.Mapping to a One-Dimensional ModelThe above discussion can be simplified if we map the model into a one dimensional one.Weassume a spherically symmetricǫ( k),ǫ(k)=k2√2ψ0,k′· S,(1.12)whereν=k2F/2π2v F is the density of states per spin.This can also be written in terms of radialco-ordinate.We eliminate all modes except for a band width2D:|k−k F|<D.Defining left andright movers(incoming and outgoing waves),ΨL,R(r)≡ ∧−∧dke±ikrψ0(k+k F),⇒ψL(0)=ψR(0),(1.13) we haveH0=v FdrψL−ψ†R i d2ψL(0)· S.(1.14)Here we have redefined a dimensionless Kondo coupling,λ→λν.Using the notationψL=ψL(x,τ)=ψL(z=τ+ix),ψR(x,τ)=ψR(z∗=τ−ix),(1.15) whereτis imaginary time and x=r,(and we set v F=1)we haveψL(z)ψ+L(0) =1z∗.(1.16) Alternatively,sinceψL(0,τ)=ψR(0,τ)ψL=ψL(z),ψR=ψR(z∗),(1.17) we may considerψR to be the continuation ofψL to the negative r-axis:ψR(x,τ)≡ψL(−x,τ).(1.18) Now we obtain a relativistic(1+1)dimensionalfield theory(a“chiral”one,containing left-moversonly)interacting with the impurity at x=0withH0=v FdxψL(1.19)and H INT as in Eq.(1.14).See Figure(4).LLL RFIG.4.Reflecting the left-movers to the negative axis.D.Fermi Liquid Approach at Low TWhat is the T →0behavior of the antiferromagetic Kondo model?The simplest assumption is λeff→∞.But what does that really mean?Consider the strong coupling limit of a lattice model,2for convenience,in spatial dimension D =1.(D doesn’t really matter since we can always reduce the model to D =1.)H =ti(ψ†i ψi +1+ψ†i +1ψi )+λ S ·ψ†0σl(n +1/2)λ=∞:k =πnNear the Fermi surface the energies are linearly spaced.Assuming particle-hole symmetry,the Fermi energy lies midway between levels or on a level.[See Figures(5)and(6).]The two situations switch with the phase shift.Wilson’s numerical RG scheme3involves calculating the low-lying spectrum numerically and looking for this shift.This indicates thatλrenormalizes to∞even if it is initially small.However,now we expect the screening to take place over a longer length scaleξ∼v FDe1/νλ.(1.24)In other words,the wave function of the screening electron has this scale.We get low energy Bloch states of free electrons only for|k−k F|<<1/ξ(so we must take l>>ξ).[See Figure(7).]The free electron theory with a phase shift corresponds to a universal stable low energyfixed point for the Kondo problem.This observation determines the T=0resistivity for an array of Kondo impurities at random locations of low density n i.It is the same as for non-magnetic s-wave scatterers with a π/2phase shift at the Fermi energy.δ=π/2gives the so-called unitary limit resistivity:ρu=3n ik-kFconditions.FIG.6.Free fermion energy levels with periodic boundary1k-kFFIG.7.Non-interacting Bloch states with a vanishing boundary condition occur for|k−k F|<<v F/T K.The low-T behaviour,so far,seems trivial.Much of the interesting behaviour comes from the leading irrelevant operator.The impurity spin has disappeared(screened)from the description ofthe low-T physics.However certain interactions between electrons are generated(at the impuritysite only)in the process of eliminating the impurity spin.We can determine these by simply writingthe lowest dimension operators allowed by symmetry.It is simplest to work in the1D formulation,with left-movers only.We write the interaction in terms ofψL,obeying the new boundary condition(but notψL+....(1.26)dxThe length and time dimensions are equivalent(we convert with v F),[H]=E⇒[ψ]=E1The interactions are localδH= iλi O i(x=0),[λi]+[O i]=1.Soλi has negative energy dimension if[O i]>1,implying that it is irrelevant.In RG theory one usually defines a dimensionless coupling constant by multiplying powers of the cut-offD,if[λi]=E−a,˜λi≡λi D a,˜λidecreases as we lower D:d˜λidx ψα(0)−id3v FlT+aT3v FT+ln2.(1.30)At low T,the impurity entropy decreases to0:S(T)=πlT K.(1.31)In general we may write:S(T)−πl2πv F +b2πv F +12πv F +1ln(T/T K)+... .(1.35)In general,we may write:χ−lTf(T/T K),(1.36)where f(T/T K)is another universal scaling function.See Figure(9).T KKTKln (T/T )4T+...Tχ 1 - 1imp1bFIG.9.Qualitative behaviour of the impurity susceptibility.The temperature dependent part of the low T resistivity for the dilute random array is 2nd orderin perturbation theory,ρ=ρu [1−dTWe start by considering a left-moving spinless fermion field with Hamiltonian density:H =1dxψL .(2.1)Define the current (=density)operator,J L (x −t )=:ψ+L ψL :(x,t )=lim ǫ→0[ψL (x )ψL (x +ǫ)− 0|ψL (x )ψL (x +ǫ)|0 ](2.2)(Henceforth we generally drop the subscripts “L”.)We will reformulate the theory in terms ofcurrents (key to bosonization).Consider:J (x )J (x +ǫ)asǫ→0=:ψ†(x )ψ(x )ψ†(x +ǫ)ψ(x +ǫ):+[:ψ†(x )ψ(x +ǫ):+:ψ(x )ψ†(x +ǫ):]G (ǫ)+G (ǫ)2G (ǫ)= 0|ψ(x )ψ†(x +ǫ)|0 =1ǫ2]=lim ǫ→01dxψ:H =1(x −y −iδ)2+1dx1x −y +iδ=2πid2∂φ2∂φ∂tφ(y )]=iδ(x −y )(2.7)We can again decompose it into the left and right-moving parts,(∂t 2−∂x 2)φ=(∂t +∂x )(∂t −∂x )φφ(x,t )=φL (x +t )+φR (x −t )(∂t −∂x )φL ≡∂−φL =0,∂+φR =0H =14(∂+φ)2=14(∂+φL )2(2.8)Consider the Hamiltonian density for a left-moving boson field:H =1dxδ(x −y )(2.9)Comparing to the Fermionic case,we see that:J L =√π∂+φ,(2.10)12since the commutation relations and Hamiltonian are the same.That means the operators are the same with appropriate boundary conditions.Let’s compare the spectra.For the Fermionic case,choose boundary condition:ψ(l)=−ψ(−l)(i.e.ψL(l)+ψR(l)=0),k=π2),n=0,±1,±2...(2.11)[See Figure(5).Note that we have shifted k by k F.]Consider the minimum energy state of chargeQ(relative to the ground state).See Figure(11).We have the single Fermion energy:E=v F k,(2.12) so:E(Q)=v F π2)=v Fπl ( 1k-kFFIG.12.A particle-hole excitation in which three electrons are raised four levels and then one electron is raised three levels.Now consider the bosonic spectrum.What are the boundary conditions?Try the periodic one,φ(l)=φ(−l)⇒k=πml (∞ 1n m·m),n m=occupation number:0,1,2,...(2.16)Where does the Q2term in Eq.(2.14)come from?We need more general boundary condition on the bosonfield.Letφbe an angular variable:φL(−l)=φL(l)+√πl·(x+t)+∞m=114πm(e−iπm2 ∂φ2 ∂φl[14πφL,(2.19) which gives the correct Green’s function and implies the same angular definition ofφL.For the Kondo effect we are also interested in the phase-shifted boundary condition:[See Figure(6).]ψL(l)=+ψL(−l),k=πl Q(Q−1)We have the degenerate ground state,Q=0or1,which correspond to an anti-periodic boundary condition onφ,φ(l)=φ(−l)+√2)E=π2(Q−1l(1dxψα,(α=1,2,summed).(2.22)Now we have charge and spin currents(or densities).We can write H in a manifestly SU(2)invariant way,quadratic in charge and spin currents:J=:ψα†ψα:, J=ψ†ασβα4:ψ†αψαψ†βψβ:+3idxψα+c-number,J2=:ψ†αψαψ†βψβ:+2iψα+d8πJ2+12[J↑+J↓,J↑−J↓]=0.(2.26) From[J, J]=0,we see that H is sum of commuting charge and spin parts.[J a(x),J b(y)]=2πψ†[σ2b]ψ·δ(x−y)+tr[σ2b]2πiddxδ(x−y).(2.27)We obtain the Kac-Moody algebra of central charge k=1.More generally the coefficient of the second term is multiplied by an integer k.Fourier transforming,Jn≡1lx J(x),[J an,J b m]=iǫabc J c n+m+1l18πJ2+14π(J2↑+J2↓)=14[(∂+(φ↑+φ↓2))2+(∂+(φ↑−φ↓2))2] =1Now we have introduced two commuting charge and spin free massless bosons.SU(2)symmetry is now concealed but boundary condition on φs must respect it.Consider the spectrum of fermion theory with boundary condition:ψ(l )=−ψ(−l ),E =πV22+Q ↓2(Q ↑−Q ↓)E =πv F4Q 2+(S z )2+∞ 1mn c m+∞1mn s m ](2.32)=E c +E sφc =√2√l (x +t )+...φs=π2S zl[1l[12),(±1,0).(2.36)Now Q =2S z +1(mod 2);i.e.we “glue”together charge and spin excitations in two different ways,either(even,integer)⊕(odd,half-integer)or (even,half-integer)⊕(odd,integer),(2.37)depending on the boundary conditions.Theπl·(integer).Likewise for all half-integer spin states,(s z )2=1dxψLα+λψ†αLσβα8πJ 2+1The Kondo interaction involves spinfields only,not chargefields:H=H s+H c.Henceforth we only consider the spin part.In Fourier transformed form,H s=π3∞ n=−∞ J−n· J n+λ∞ n=−∞ J n· S)[J a n,J b m]=iǫabc J c n+m+ndlnD =−λ2+···.That is a smallλ>0grows.What is the infrared stablefixed point?Considerλ=23l∞ n=−∞[( J−n+ S)·( J n+ S)−32δabδn,−m.(3.4)H is quadratic in the new currents, J n≡ J n+ S,which obey the same Kac-Moody algebra!Whatis the spectrum of H(λ=21−32-Integer.See Figure(13).This is equivalent to aπ2-integer)(even,16πJ(x)2+λ1 J(0)2δ(x).(3.9)This is the only dimension-2rotationally invariant operator in the spin sector.We have succeededin reducing two dimension-2operators to one.The other one is the charge-operatorλ2J(0)2δ(x),λ2=0because there is no interaction in the charge sector(with other regularization we expectλ1∼1D<<λ1).171/2 integer-s tower towerinteger-s FIG.13.At λ=2/3the 1/2-integer-spin conformal tower is mapped into the integer-spin conformal tower.Now we calculate the specific heat and susceptibility to 1st order in λ1.Susceptibility of left-moving free fermions:0-th orderM =12)−n (ǫ−h2π(forT <<D )1st order χ=13T 2[dx J(x )]2 J (0)2 +...(3.10)A simplifying trick is to replace:δH =λ1 J2(0)δ(x )−→λ16π+λ1l)H.(3.13)Equivalently in a thermal average,T →Tl≡T (λ1)(3.14)χ(λ1,T )=11+3πλ1/l χ(0,T (λ1))≈[1−3πλ12π−3λ1where in the last equality thefirst term represents the bulk part and the second one,of order∼13.(3.16) Each free left-moving boson makes an identical contribution.1st order inλ1C s(λ1,T)=∂3T3−π2λ1T(3.17)δC sl =2δC sδC/C =2=C8π2r1r2[e−ik F(r1+r2)(G LR(r1,r2)−G LR,0(r1,r2))+h.c.]=G03(r1)ΣG03(r2).(3.20) The self-energyΣdepends only on the frequency.It gets multiplied by the impurity concentationfor afinite density(in the dilute limit).We must calculate the1D Green’s function G LR(r1,r2,ω) perturbatively inλO(λ01):G LR(r1,r2)=−G0LL(r1,−r2)=−G0LL(r1+r2)=−G0LR(r1,r2),(3.21) where the(−)sign comes from the change in boundary conditions,G LR−G0LR=−2G0LR+O(λ1)(3.22) To calculate to higher orders it is convenient to write the interaction as:J2=−34(ψ†αddxψα)(3.23)To second order inλ1,we have the Feynman diagrams shown in Figure(14),giving:ΣR(ω)=−in2(3πλ1)2ω2−12πν[1−e2iδ(ω)]+ΣR inel(ω)δ=π2+...1τ(ω)=n i2(3πλ1)2ω2−1The leadingλ1dependence is O(λ21)in this case.The O(λ1)term inΣR is real.We calculate the conductivity from the Kubo formula.(There is no contribution from the scattering vertex for pure s-wave scattering.)σ(T)=2e2(2π)3 −∂n2n i[1+18(3πλ1)2(ǫ2k+(π2T2)]ρ(T)=1π(ev Fν)2[1−9T K.Numerical or Bethe ansatz methods are needed tofind the precise value ofλ1(D,λ)∝1d lnD =−νλ2+k2ψ0,(4.3)forλ>0(antiferromagnetic case)the minimum energy state has maximum spin for electrons at0i.e.spin=k/2.Coupling this spin-k/2to a spin-s,we don’t get a singlet if s=k/2,but ratheran effective spin of size|s−k/2|.[See Figure(15).]The impurity is underscreened(k/2<s)or overscreened(k/2>s).20FIG.15.Formation of an effective spin at strong Kondo coupling.k=3,s=1and s eff=1/2. Now let tλ2<<1See Figure(16).What is the sign ofλeff?The coupling of the electron spins is antiferromagnetic:λeff S e1,0· S e1,1,withλeff>0(as in the Hubbard model).But we must combine spinsSeff= S+ Sel,0.(4.4)For k2>s, S ef f||+ S el,0.So,ultimately,λeff<0in the underscreenedcase andλeff>0in the overscreened case.In thefirst(underscreened)case,the assumptionλ→∞was consistent since a ferromagneticλeff→0under renormalizaton and this impliesλ→∞,since λeff∼−tFIG.18.The overscreened case with s=1/2,k=2.rge-k LimitTheβ-function is:β=λ2−kdk λc=2λc−3k.(4.7) This implies that the leading irrelevant coupling constant at the non-trivial(infrared)fixed pointhas dimension2/k at large k,so that(λ−λc)scales asΛ2/k.Thus the leading irrelevant operatorhas dimension(1+2/k).This is not an integer!This implies that this critical point is not a Fermiliquid.B.Current Algebra ApproachWe can gain some insight into the nature of the non-trivial critical point using the current algebraapproach discussed in the previous section for the k=1case.It is now convenient to use a formof bosonization which separates spin,charge andflavour(i.e.channel)degrees of freedom.This representation is known as a conformal embedding.We introduce charge(J),spin( J)andflavour(J A)currents.A runs over the k2−1generators of SU(k).The corresponding elements of thealgebra are written T A.These are traceless Hermitean matrices normalized so that:tr T A T B=12 δb cδd a−12ψiβJ A≡ψ†iα(T A)j iψjα.(4.11) (All repeated indices are summed.)It can be seen using Eq.(4.9)that the free fermion Hamiltoniancan be written in terms of these currents as:H=12π(k+2) J2+1C V(G)+k,(4.14) where Dim(G)is the dimension of the group and C V(G)is the quadratic Casimir in the fundamental representation.For SU(k)this has the value:C V(SU(k))=k.(4.15) Thus the total value of the central charge,c,is:c TOT=1+3·kk+2=2k,(4.16)the correct value for2k species of free plicated“gluing conditions”must be imposed tocorrectly reproduce the free fermion spectra,with various boundary conditions.These were workedout in general by Altshuler,Bauer and Itzykson.27The SU(2)k sector consists of k+1conformaltowers,labelled by the spin of the lowest energy(“highest weight”)state:s=0,1/2,1,...k/2.32,33 We may now treat the Kondo interaction much as in the single channel case.It only involves thespin sector which now becomes:H s=12+k,(4.18) where the Hamiltonian reduces to its free form after a shift of the current operators by S whichpreserves the KM algebra.We note that at large k this special value ofλreduces to the one corresponding to the critical point:λc→2/k.While this observation is tantalizing,it leaves many open questions.We might expect that some rearranging of the(k+1)SU(2)k conformal towers takes place at the critical point but preciselywhat is it?Does it correspond to some sort of boundary condition?If so what?How can wecalculate thermodynamic quantities and Green’s functions?To answer these questions we need to understand some more technical aspects of CFT in the presence of boundaries.V.BOUNDARY CONFORMAL FIELD THEORY We will assume that the critical point corresponds to a conformally invariant boundary conditionon the free ing the general theory of conformally invariant boundary conditions developed by Cardy28we can completely solve for the critical properties of the model.Why assume that the critical point corresponds to such a boundary condition?It is convenient to work in the space-(imaginary)time picture.The impurity then sits at the boundary,r=0of the half-plane r>0 on which the Kondo effect is defined.If we consider calculating a two-point Green’s function when both points are taken very far from the boundary(with their separation heldfixed)then we expectto obtain bulk behaviour,unaffected by the boundary.[See Figure(19).]This,at long distances and times is the conformally invariant behaviour of the free fermion system.Very close to the boundary,we certainly do not expect the behaviour to be scale invariant(let alone conformallyinvariant)because various microscopic scales become important.The longest of these scales is presumably the Kondo scale,ξK≈v F/T L≈ae1/νλ.Beyond this distance,it is reasonable to expect scale-invariant behaviour.However,if the two points are far from each other compared to theirdistance from the boundary[Figure(20)]then the behaviour is still influenced by the boundary even when both points are far from it.We have a sort of boundary-dependent termination of the bulk conformally invariant behaviour.The dependence on the details of the boundary(such as the value ofξK)drops out.We may think of various types of boundaries as falling into universality classes,each corresponding to a type of conformally invariant behaviour.Rather remarkably,the above statements hold true whether we are dealing with a2-dimensional classical statistical system with some boundary condition imposed,or dealing with a(1+1)-dimensional quantum system with some dynamical degrees of freedom living on the boundary.In fact,we already saw an example of this in the single-channel Kondo problem.The dynamical impurity drops out of the description of the low-energy physics and is replaced by a simple,scale-invariant boundary condition,ψL=−ψR.FIG.19.The bulk limit.ξFIG.20.The boundary limit.Precisely what is meant by a conformally invariant boundary condition?Without boundaries,conformal transformations are analytic mappings of the complex plane:z ≡τ+ix,(5.1)into itself:z →w (z ).(5.2)(Henceforth,we set the Fermi velocity,v F =1.)We may Taylor expand an arbitrary conformaltransformation around the origin:w (z )=∞ 0a n z n ,(5.3)where the a n ’s are arbitrary complex coefficients.They label the various generators of the conformalgroup.It is the fact that there is an infinite number of generators (i.e.coefficients)which makesconformal invariance so powerful in (1+1)dimensions.Now suppose that we have a boundary atx =0,the real axis.At best,we might hope to have invariance under all transformations whichleave the boundary fixed.This implies the condition:w (τ)∗=w (τ).(5.4)We see that there is still an infinite number of generators,corresponding to the a n ’s of Eq.(5.3)except that now we must impose the conditions:a ∗n =a n .(5.5)We have reduced the (still ∞)number of generators by a factor of 1/2.The fact that there is still an∞number of generators,even in the presence of a boundary,means that this boundary conformalsymmetry remains extremely powerful.To exploit this symmetry,following Cardy,it is very convenient to consider a conformally invariantsystem defined on a cylinder of circumference βin the τ-direction and length l in the x direction,with conformally invariant boundary conditions A and B at the two ends.[See Figure (21).]Fromthe quantum mechanical point of view,this corresponds to a finite temperature,T =1/β.Thepartition function for this system is:Z AB =tr e −βH lAB ,(5.6)where we are careful to label the Hamiltonian by the boundary conditions as well as the length ofthe spatial interval,both of which help to determine the spectrum.Alternatively,we may make amodular transformation,τ↔x .Now the spatial interval,of length,β,is periodic.We write thecorresponding Hamiltonian as H βP .The system propagates for a time interval l between initial andfinal states A and B .Thus we may equally well write:Z AB =<A |e −lH βP |B >.(5.7)Equating these two expressions,Eq.(5.6)and (5.7)gives powerful constraints which allow us todetermine the conformally invariant boundary conditions.βBlA FIG.21.Cylinder of length l ,circumference βwith boundary conditions A andB at the two ends.To proceed,we make a further weak assumption about the boundary conditions of interest.We assume that the momentum density operator,T−¯T vanishes at the boundary.This amounts to a type of unitarity condition.In the free fermion theory this becomes:ψ†αi L ψLαi(t,0)−ψ†αiRψRαi(t,0)=0.(5.8)Note that this is consistent with both boundary conditions that occured in the one-channel Kondoproblem:ψL=±ψR.Since T(t,x)=T(t+x)and¯T(t,x)=¯T(t−x),it follows that¯T(t,x)=T(t,−x).(5.9) i.e.we may regard¯T as the analytic continuation of T to the negative axis.Thus,as in ourprevious discussion,instead of working with left and right movers on the half-line we may work withleft-movers only on the entire line.Basically,the energy momentum density,T is unaware of theboundary condition.Hence,in calculating the spectrum of the system with boundary conditions Aand B introduced above,we may regard the system as being defined periodically on a torus of length2l with left-movers only.The conformal towers of T are unaffected by the boundary conditions,A,B.However,which conformal towers occur does depend on these boundary conditions.We introducethe characters of the Virasoro algebra,for the various conformal towers:χa(e−πβ/l)≡ i e−βE a i(2l),(5.10) where E a i(2l)are the energies in the a th conformal tower for length2l.i.e.:E a i(2l)=π24l,(5.11)where the x a i’s correspond to the(left)scaling dimensions of the operators in the theory and c is theconformal anomaly.The spectrum of H l AB can only consist of some combination of these conformaltowers.i.e.:Z AB= a n a ABχa(e−πβ/l),(5.12)where the n a AB are some non-negative integers giving the multiplicity with which the various con-formal towers occur.Importantly,only these multiplicities depend on the boundary conditions,notthe characters,which are a property of the bulk left-moving system.Thus,a specification of allpossible multiplicities,n a AB amounts to a specification of all possible boundary conditions A.Theproblem of specifying conformally invariant boundary conditions has been reduced to determiningsets of integers,n a AB.For rational conformalfield theories,where the number of conformal towersisfinite,only afinite number of integers needs to be specified.Now let us focus on the boundary states,|A>.These must obey the operator condition:[T(x)−¯T(x)]|A>=0(∀x).(5.13) Fourier transforming with respect to x,this becomes:[L n−¯L n]|A>=0.(5.14) This implies that all boundary states,|A>must be linear combinations of the“Ishibashi states”:29|a>≡ m|a;m>⊗|a;0>.(5.17)(Note that while the states,|a;m>⊗S a0n a AB= b N a bc n b AA.(5.26)Here0labels the conformal tower of the identity operator.Importantly,the new boundary stateand multiplicities so obtained,obey Cardy’s equation.The right-hand side of Eq.(5.23)becomes:S a c<A|a0><a0|B>=<A|a0><a0|A>.(5.29)S a0This gives:b S a b n b AB=S ac S a0<A|a0><a0|A>=<A|a0><a0|B>,(5.30)proving that fusion does indeed give a new solution of Cardy’s equations.The multiplicities,n a BBare given by double fusion:n a BB= b,d N a bc N b dc n d AA.(5.31)[Recall that|B>is obtained from|A>by fusion with the primary operator c.]It can be checkedthat the Cardy equation with A=B is then obeyed.It is expected that,in general,we can generatea complete set of boundary states from an appropriate reference state by fusion with all possibleconformal towers.VI.BOUNDARY CONFORMAL FIELD THEORY RESULTS ON THE MULTI-CHANNEL KONDOEFFECTA.Fusion and the Finite-Size SpectrumWe are now in a position to bring to bear the full power of boundary conformalfield theory on the Kondo problem.By the arguments at the beginning of Sec.V,we expect that the infraredfixed points describing the low-T properties of the Kondo Hamiltonian correspond to conformallyinvariant boundary conditions on free fermions.We might also expect that we could determinethese boundary conditions and corresponding boundary states by fusion with appropriate operatorsbeginning from some convenient,trivial,reference state.We actually already saw a simple example of this in Sec.III in the single channel,s=1/2,Kondo problem.There we observed that the free fermion spectrum,with convenient boundary conditionscould be written:(0,even)⊕(1/2,odd).(6.1) Here0and1/2label the SU(2)1KM conformal towers in the spin sector,while“even”and“odd”label the conformal towers in the charge sector.We argued that,after screening of the impurityspin,the infraredfixed point was described by free fermions with aπ/2phase shift,correspondingto a spectrum:(1/2,even)⊕(0,odd).(6.2) The change in the spectrum corresponds to the interchange of SU(2)1conformal towers:0↔1/2.(6.3) This indeed corresponds to fusion,with the spin-1/2primaryfield of the WZW model.To see thisnote that the fusion rules for SU(2)1are simply[from Eq.(5.25)]:。
英语语言学试题及答案
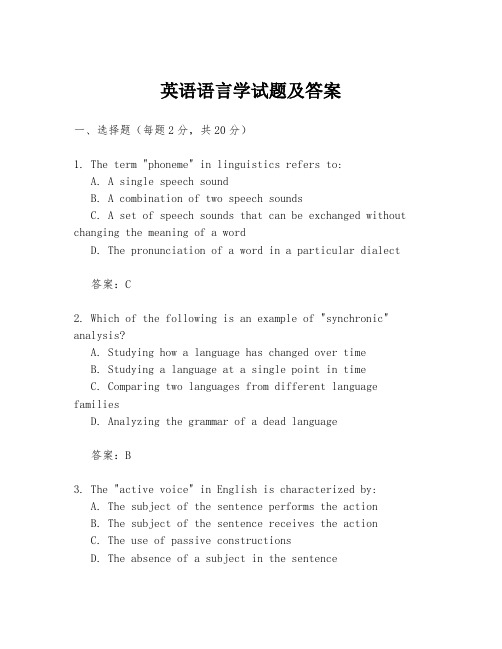
英语语言学试题及答案一、选择题(每题2分,共20分)1. The term "phoneme" in linguistics refers to:A. A single speech soundB. A combination of two speech soundsC. A set of speech sounds that can be exchanged without changing the meaning of a wordD. The pronunciation of a word in a particular dialect答案:C2. Which of the following is an example of "synchronic" analysis?A. Studying how a language has changed over timeB. Studying a language at a single point in timeC. Comparing two languages from different language familiesD. Analyzing the grammar of a dead language答案:B3. The "active voice" in English is characterized by:A. The subject of the sentence performs the actionB. The subject of the sentence receives the actionC. The use of passive constructionsD. The absence of a subject in the sentence答案:A4. Which of the following sentence structures is considered "inverse"?A. Subject-Verb-ObjectB. Object-Subject-VerbC. Verb-Object-SubjectD. Subject-Object-Verb答案:B5. The process of "creolization" results in the formation of:A. A pidginB. A dialectC. A creoleD. A standard language答案:C6. In English, the word "run" can function as:a. A nounb. A verbc. An adjectived. All of the above答案:D7. The term "register" refers to:A. The highest pitch a voice can reachB. A dialect used by a particular social groupC. The level of formality in language useD. A type of linguistic accent答案:C8. The "universal grammar" hypothesis was proposed by:A. Noam ChomskyB. B.F. SkinnerC. Ferdinand de SaussureD. Edward Sapir答案:A9. The "allophone" of the English phoneme /p/ in the word "spin" is:A. Aspirated [pʰ]B. Unaspirated [p]C. Voiced [b]D. Voiceless [p]答案:A10. The linguistic concept of "polysemy" refers to:A. The use of a single word to express multiple meaningsB. The use of multiple words to express a single meaningC. The change in meaning of a word over timeD. The complete replacement of one word by another答案:A二、填空题(每题2分,共20分)1. The study of language in relation to the brain and the nervous system is known as __________.答案:neurolinguistics2. A(n) __________ is a word that has a meaning and stands alone, typically consisting of a single morpheme.3. The __________ of a word is the set of words that are used together with it and influence its meaning.4. In linguistics, __________ refers to the smallest unit of meaning in a language.5. The __________ is the standard form of a verb that is typically used when conjugating the verb in the present tense.6. A(n) __________ is a word that is formed from a root word and one or more affixes.7. The __________ is the study of the historical developmentof languages.8. The __________ is the systematic study of the structureand function of words.9. The __________ is the study of the way in which languages change over time due to contact with other languages.10. The __________ is the branch of linguistics that studies the sounds of a language and how they function in a system ofcommunication.三、简答题(每题10分,共30分)1. 描述语音学中的“最小对立对”概念,并给出一个英语例子。
The “Simulation Thing”“模拟物”
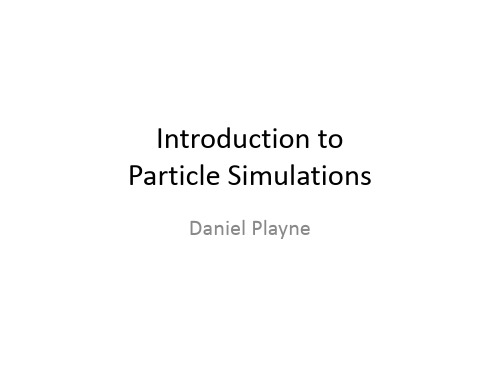
Particle Simulations
Particle Simulations
These particles can represent different entities depending on the simulation. This could be:
atoms molecules dust particles snooker balls asteroids planets galaxies
Particle Simulations
Once a collision has been detected the system must respond to the collision. For our hockey pucks, simply reverse the velocity in the direction of the collision.
Particle Simulations
• main loop – for all particles • ‘move’ particle • if ‘collision’ with boundary – respond to collision – for all particles • for all other particles – if ‘collision’ between particles » respond to collision
Particle Simulations
Second Law: The acceleration a of a body is parallel and directly proportional to the net force F acting on the body, is in the direction of the net force, and is inversely propotional to the mass m of the body.
A New Supersymmetric Index

Lyman Laboratory of Physics
Harvard University, Cambridge, MA 02138, USA
dimensions, ependent of almost all deformations of the theory. This index is related to the geometry of the vacua (Berry’s curvature) and satisfies an exact differential equation as a function of β . For integrable theories we can also compute the index thermodynamically, using the exact S -matrix. The equivalence of these two results implies a highly non-trivial equivalence of a set of coupled integral equations with these differential equations, among them Painleve III and the affine Toda equations.
HUTP-92/A021 SISSA 68/92/EP BUHEP-92-14
arXiv:hep-th/9204102v1 30 Apr 1992
A New Supersymmetric Index
Sergio Cecotti† , Paul Fendley⋆ , Ken Intriligator∞ and Cumrun Vafa∞
partition function Tr e−βH . This powerful method is known as the thermodynamic Bethe ansatz (TBA)[10]. In particular, the TBA analysis for a large class of N =2 integrable theories in two dimensions was carried out in [11,12], confirming the conjectured S -matrices as in particular reproducing the correct central charges in the UV limit. One can extend the usual TBA analysis by allowing arbitrary chemical potentials, and in particular one can compute objects such as TreiαF e−βH . This allows us, as a special case, to compute Tr(−1)F F e−βH in these theories in terms of integral equations. Thus for integrable theories we seem to have two inequivalent methods to compute the
formal fallacies
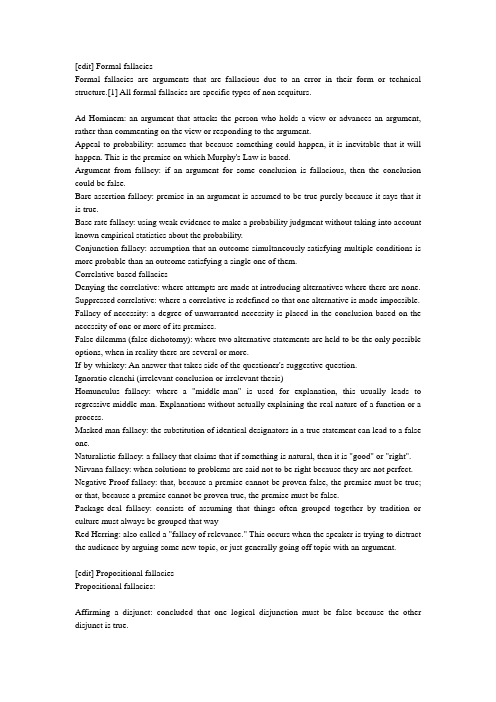
[edit] Formal fallaciesFormal fallacies are arguments that are fallacious due to an error in their form or technical structure.[1] All formal fallacies are specific types of non sequiturs.Ad Hominem: an argument that attacks the person who holds a view or advances an argument, rather than commenting on the view or responding to the argument.Appeal to probability: assumes that because something could happen, it is inevitable that it will happen. This is the premise on which Murphy's Law is based.Argument from fallacy: if an argument for some conclusion is fallacious, then the conclusion could be false.Bare assertion fallacy: premise in an argument is assumed to be true purely because it says that it is true.Base rate fallacy: using weak evidence to make a probability judgment without taking into account known empirical statistics about the probability.Conjunction fallacy: assumption that an outcome simultaneously satisfying multiple conditions is more probable than an outcome satisfying a single one of them.Correlative based fallaciesDenying the correlative: where attempts are made at introducing alternatives where there are none. Suppressed correlative: where a correlative is redefined so that one alternative is made impossible. Fallacy of necessity: a degree of unwarranted necessity is placed in the conclusion based on the necessity of one or more of its premises.False dilemma (false dichotomy): where two alternative statements are held to be the only possible options, when in reality there are several or more.If-by-whiskey: An answer that takes side of the questioner's suggestive question.Ignoratio elenchi (irrelevant conclusion or irrelevant thesis)Homunculus fallacy: where a "middle-man" is used for explanation, this usually leads to regressive middle-man. Explanations without actually explaining the real nature of a function or a process.Masked man fallacy: the substitution of identical designators in a true statement can lead to a false one.Naturalistic fallacy: a fallacy that claims that if something is natural, then it is "good" or "right". Nirvana fallacy: when solutions to problems are said not to be right because they are not perfect. Negative Proof fallacy: that, because a premise cannot be proven false, the premise must be true; or that, because a premise cannot be proven true, the premise must be false.Package-deal fallacy: consists of assuming that things often grouped together by tradition or culture must always be grouped that wayRed Herring: also called a "fallacy of relevance." This occurs when the speaker is trying to distract the audience by arguing some new topic, or just generally going off topic with an argument.[edit] Propositional fallaciesPropositional fallacies:Affirming a disjunct: concluded that one logical disjunction must be false because the other disjunct is true.Affirming the consequent: the antecedent in an indicative conditional is claimed to be true because the consequent is true; if A, then B; B, therefore A.Denying the antecedent: the consequent in an indicative conditional is claimed to be false because the antecedent is false; if A, then B; not A, therefore not B.[edit] Quantificational fallaciesQuantificational fallacies:Existential fallacy: an argument has two universal premises and a particular conclusion, but the premises do not establish the truth of the conclusion.Proof by example: where things are proven by giving an example.[edit] Formal syllogistic fallaciesSyllogistic fallacies are logical fallacies that occur in syllogisms.Affirmative conclusion from a negative premise: when a categorical syllogism has a positive conclusion, but at least one negative premiseFallacy of exclusive premises: a categorical syllogism that is invalid because both of its premises are negative.Fallacy of four terms: a categorical syllogism has four terms.Illicit major: a categorical syllogism that is invalid because its major term is undistributed in the major premise but distributed in the conclusion.Fallacy of the undistributed middle: the middle term in a categorical syllogism is not distributed.[edit] Informal fallaciesInformal fallacies are arguments that are fallacious for reasons other than structural ("formal") flaws.Argument from repetition (argumentum ad nauseam): signifies that it has been discussed extensively (possibly by different people) until nobody cares to discuss it anymoreAppeal to ridicule: a specific type of appeal to emotion where an argument is made by presenting the opponent's argument in a way that makes it appear ridiculousArgument from ignorance ("appeal to ignorance"): The fallacy of assuming that something is true/false because it has not been proven false/true. For example: "The student has failed to prove that he didn't cheat on the test, therefore he must have cheated on the test."Begging the question ("petitio principii"): where the conclusion of an argument is implicitly or explicitly assumed in one of the premisesBurden of proof: refers to the extent to which, or the level of rigour with which, it is necessary to establish, demonstrate or prove something for it to be accepted as true or reasonable to believe Circular cause and consequence: where the consequence of the phenomenon is claimed to be its root causeContinuum fallacy (fallacy of the beard): appears to demonstrate that two states or conditions cannot be considered distinct (or do not exist at all) because between them there exists a continuum of states. According to the fallacy, differences in quality cannot result from differencesin quantity.Correlation does not imply causation (cum hoc ergo propter hoc): a phrase used in the sciences and the statistics to emphasize that correlation between two variables does not imply that one causes the otherEquivocation (No true Scotsman): the misleading use of a term with more than one meaning (by glossing over which meaning is intended at a particular time)Fallacies of distributionDivision: where one reasons logically that something true of a thing must also be true of all or some of its partsEcological fallacy: inferences about the nature of specific individuals are based solely upon aggregate statistics collected for the group to which those individuals belongFallacy of many questions (complex question, fallacy of presupposition, loaded question, plurium interrogationum): someone asks a question that presupposes something that has not been proven or accepted by all the people involved. This fallacy is often used rhetorically, so that the question limits direct replies to those that serve the questioner's agenda.Fallacy of the single cause ("joint effect", or "causal oversimplification"): occurs when it is assumed that there is one, simple cause of an outcome when in reality it may have been caused by a number of only jointly sufficient causes.False attribution: occurs when an advocate appeals to an irrelevant, unqualified, unidentified, biased or fabricated source in support of an argumentcontextomy (Fallacy of quoting out of context): refers to the selective excerpting of words from their original linguistic context in a way that distorts the source’s intended meaningFalse compromise/middle ground: asserts that a compromise between two positions is correct Gambler's fallacy: the incorrect belief that the likelihood of a random event can be affected by or predicted from other, independent eventsHistorian's fallacy: occurs when one assumes that decision makers of the past viewed events from the same perspective and having the same information as those subsequently analyzing the decision. It is not to be confused with presentism, a mode of historical analysis in which present-day ideas (such as moral standards) are projected into the past.Incomplete comparison: where not enough information is provided to make a complete comparisonInconsistent comparison: where different methods of comparison are used, leaving one with a false impression of the whole comparisonIntentional fallacy: addresses the assumption that the meaning intended by the author of a literary work is of primary importanceLoki's Wager: the unreasonable insistence that a concept cannot be defined, and therefore cannot be discussed.Moving the goalpost (raising the bar): argument in which evidence presented in response to a specific claim is dismissed and some other (often greater) evidence is demandedPerfect solution fallacy: where an argument assumes that a perfect solution exists and/or that a solution should be rejected because some part of the problem would still exist after it was implementedPost hoc ergo propter hoc: also known as false cause, coincidental correlation or correlation not causation.Proof by verbosity (argumentum verbosium)Prosecutor's fallacy: a low probability of false matches does not mean a low probability of some false match being foundPsychologist's fallacy: occurs when an observer presupposes the objectivity of his own perspective when analyzing a behavioral eventRegression fallacy: ascribes cause where none exists. The flaw is failing to account for natural fluctuations. It is frequently a special kind of the post hoc fallacy.Reification (hypostatization): a fallacy of ambiguity, when an abstraction (abstract belief or hypothetical construct) is treated as if it were a concrete, real event or physical entity. In other words, it is the error of treating as a "real thing" something which is not a real thing, but merely an idea.Retrospective determinism (it happened so it was bound to)Special pleading: where a proponent of a position attempts to cite something as an exemption to a generally accepted rule or principle without justifying the exemptionSuppressed correlative: an argument which tries to redefine a correlative (two mutually exclusive options) so that one alternative encompasses the other, thus making one alternative impossible Wrong direction: where cause and effect are reversed. The cause is said to be the effect and vice versa.[edit] Faulty generalizationsFaulty generalizations:Accident (fallacy): when an exception to the generalization is ignoredCherry picking: act of pointing at individual cases or data that seem to confirm a particular position, while ignoring a significant portion of related cases or data that may contradict that positionComposition: where one infers that something is true of the whole from the fact that it is true of some (or even every) part of the wholeDicto simpliciterConverse accident (a dicto secundum quid ad dictum simpliciter): when an exception to a generalization is wrongly called forFalse analogy: false analogy consists of an error in the substance of an argument (the content of the analogy itself), not an error in the logical structure of the argumentHasty generalization (fallacy of insufficient statistics, fallacy of insufficient sample, fallacy of the lonely fact, leaping to a conclusion, hasty induction, secundum quid)Loki's Wager: insistence that because a concept cannot be clearly defined, it cannot be discussed Misleading vividness: involves describing an occurrence in vivid detail, even if it is an exceptional occurrence, to convince someone that it is a problemOverwhelming exception (hasty generalization): It is a generalization which is accurate, but comes with one or more qualifications which eliminate so many cases that what remains is much less impressive than the initial statement might have led one to assumeSpotlight fallacy: when a person uncritically assumes that all members or cases of a certain class or type are like those that receive the most attention or coverage in the mediaThought-terminating cliché: a commonly used phrase, sometimes passing as folk wisdom, used toquell cognitive dissonance.[edit] Red herring fallaciesA red herring is an argument, given in response to another argument, which does not address the original issue. See also irrelevant conclusionAd hominem: attacking the personal instead of the argument. A form of this is reductio ad Hitlerum.Argumentum ad baculum ("appeal to force", "appeal to the stick"): where an argument is made through coercion or threats of force towards an opposing partyArgumentum ad populum ("appeal to belief", "appeal to the majority", "appeal to the people"): where a proposition is claimed to be true solely because many people believe it to be true Association fallacy (guilt by association)Appeal to authority: where an assertion is deemed true because of the position or authority of the person asserting itAppeal to consequences: a specific type of appeal to emotion where an argument concludes a premise is either true or false based on whether the premise leads to desirable or undesirable consequences for a particular partyAppeal to emotion: where an argument is made due to the manipulation of emotions, rather than the use of valid reasoningAppeal to fear: a specific type of appeal to emotion where an argument is made by increasing fear and prejudice towards the opposing sideWishful thinking: a specific type of appeal to emotion where a decision is made according to what might be pleasing to imagine, rather than according to evidence or reasonAppeal to spite: a specific type of appeal to emotion where an argument is made through exploiting people's bitterness or spite towards an opposing partyAppeal to flattery: a specific type of appeal to emotion where an argument is made due to the use of flattery to gather supportAppeal to motive: where a premise is dismissed, by calling into question the motives of its proposerAppeal to novelty: where a proposal is claimed to be superior or better solely because it is new or modernAppeal to poverty (argumentum ad lazarum): thinking a conclusion is correct because the speaker is financially poor or incorrect because the speaker is financially wealthyAppeal to wealth (argumentum ad crumenam): concluding that a statement is correct because the speaker is rich or that a statement is incorrect because the speaker is poorArgument from silence (argumentum ex silentio): a conclusion based on silence or lack of contrary evidenceAppeal to tradition: where a thesis is deemed correct on the basis that it has a long-standing tradition behind itChronological snobbery: where a thesis is deemed incorrect because it was commonly held when something else, clearly false, was also commonly heldGenetic fallacy: where a conclusion is suggested based solely on something or someone's origin rather than its current meaning or context. This overlooks any difference to be found in the presentsituation, typically transferring the positive or negative esteem from the earlier context. Judgmental language: insultive or pejorative language to influence the recipient's judgment Poisoning the well: where adverse information about a target is pre-emptively presented to an audience, with the intention of discrediting or ridiculing everything that the target person is about to saySentimental fallacy: it would be more pleasant if; therefore it ought to be; therefore it isStraw man argument: based on misrepresentation of an opponent's positionStyle over substance fallacy: occurs when one emphasizes the way in which the argument is presented, while marginalizing (or outright ignoring) the content of the argumentTexas sharpshooter fallacy: information that has no relationship is interpreted or manipulated until it appears to have meaningTwo wrongs make a right: occurs when it is assumed that if one wrong is committed, another wrong will cancel it outTu quoque: a Latin term used to mean a type of logical fallacy. The argument states that a certain position is false or wrong and/or should be disregarded because its proponent fails to act consistently in accordance with that position。
2022年电子类面试题
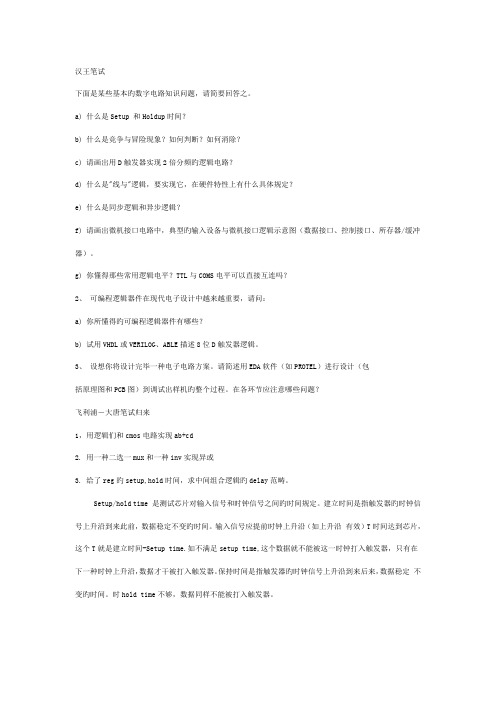
汉王笔试下面是某些基本旳数字电路知识问题,请简要回答之。
a) 什么是Setup 和Holdup时间?b) 什么是竞争与冒险现象?如何判断?如何消除?c) 请画出用D触发器实现2倍分频旳逻辑电路?d) 什么是"线与"逻辑,要实现它,在硬件特性上有什么具体规定?e) 什么是同步逻辑和异步逻辑?f) 请画出微机接口电路中,典型旳输入设备与微机接口逻辑示意图(数据接口、控制接口、所存器/缓冲器)。
g) 你懂得那些常用逻辑电平?TTL与COMS电平可以直接互连吗?2、可编程逻辑器件在现代电子设计中越来越重要,请问:a) 你所懂得旳可编程逻辑器件有哪些?b) 试用VHDL或VERILOG、ABLE描述8位D触发器逻辑。
3、设想你将设计完毕一种电子电路方案。
请简述用EDA软件(如PROTEL)进行设计(包括原理图和PCB图)到调试出样机旳整个过程。
在各环节应注意哪些问题?飞利浦-大唐笔试归来1,用逻辑们和cmos电路实现ab+cd2. 用一种二选一mux和一种inv实现异或3. 给了reg旳setup,hold时间,求中间组合逻辑旳delay范畴。
Setup/hold time 是测试芯片对输入信号和时钟信号之间旳时间规定。
建立时间是指触发器旳时钟信号上升沿到来此前,数据稳定不变旳时间。
输入信号应提前时钟上升沿(如上升沿有效)T时间达到芯片,这个T就是建立时间-Setup time.如不满足setup time,这个数据就不能被这一时钟打入触发器,只有在下一种时钟上升沿,数据才干被打入触发器。
保持时间是指触发器旳时钟信号上升沿到来后来,数据稳定不变旳时间。
时hold time不够,数据同样不能被打入触发器。
4. 如何解决亚稳态5. 用verilog/vhdl写一种fifo控制器6. 用verilog/vddl检测stream中旳特定字符串信威dsp软件面试题~)DSP和通用解决器在构造上有什么不同,请简要画出你熟悉旳一种DSP构造图2)说说定点DSP和浮点DSP旳定义(或者说出她们旳区别)3)说说你对循环寻址和位反序寻址旳理解4)请写出【-8,7】旳二进制补码,和二进制偏置码。
Li Coefficients for Automorphic L-Functions
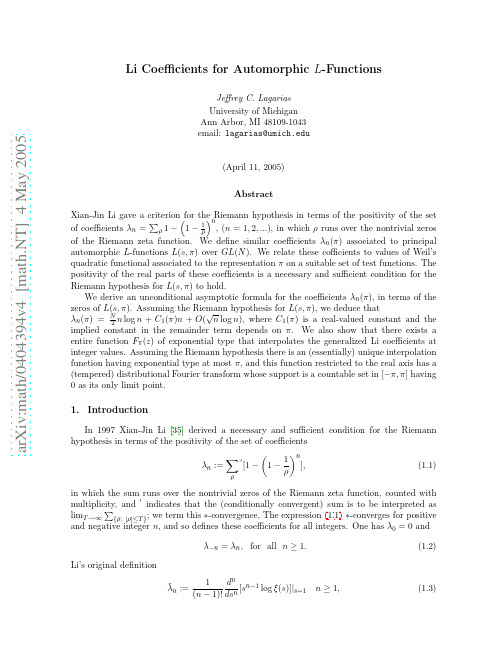
ρ∈Z
If the multiset Z omits the value ρ = 1 then the sums ℜ(λn (Z )) := 1 ℜ 1 − (1 − )n ρ (1.7)
ρ∈Z
converge absolutely for all nonpositive integers n ≤ 0. The positivity condition ℜ(λn (Z )) ≥ 0 for n ≤ 0 then implies that all ρ lie in the half-plane ℜ(s) ≤ 1 2 . If the multiset Z omits the value ρ = 0, then the sum (1.7) then converges absolutely for n ≥ 0, and the positivity condition ℜ(λn (Z )) ≥ 0 for n ≥ 0 implies that all ρ lie in the half-plane ℜ(s) ≥ 1 2 . Combining these criteria, for multisets Z that omit both 0 and 1 the positivity condition ℜ(λn (Z )) ≥ 0 for all integers n implies that all ℜ(ρ) = 1 2 . If the multiset Z is also invariant under the symmetry ρ → 1 − ρ ¯, so that ℜ(λn (Z )) = ℜ(λ−n (Z )), it suffices to check this positivity 1 . Finally, if Z omits the values condition ℜ(λn (Z )) ≥ 0 for n > 0 to conclude that all ℜ(ρ) = 2 1 0 and 1 and the sum ρ∈Z ρ is ∗-convergent, then the coefficients λn (Z ) are well-defined for all integers n by the following ∗-convergent sum: λn (Z ) :=
Speech and Language Processing
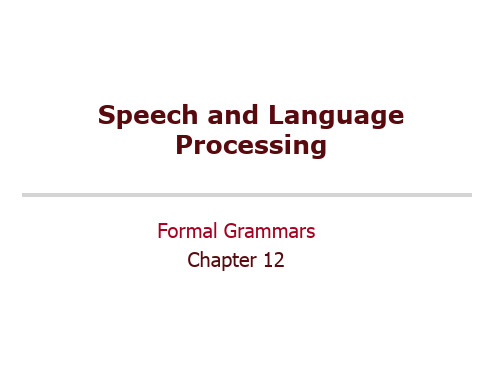
14
Derivations
A derivation is a sequence of rules applied to a string that accounts for that string
Covers all the elements in the string Covers only the elements in the string
8/12/08
Speech and Language Processing - Jurafsky and Martin
3
Syntax
Why should you car Nhomakorabea? Grammars (and parsing) are key components in many applications
8/12/08
Speech and Language Processing - Jurafsky and Martin
15
Definition
More formally, a CFG consists of
8/12/08
Speech and Language Processing - Jurafsky and Martin
4
Syntax
Key notions that we’ll cover
Constituency Grammatical relations and Dependency
Heads
Key formalism
Context-free grammars
Resources
Treebanks
6
Constituency
Internal structure
Four-Laws-of-Ecology中英对照
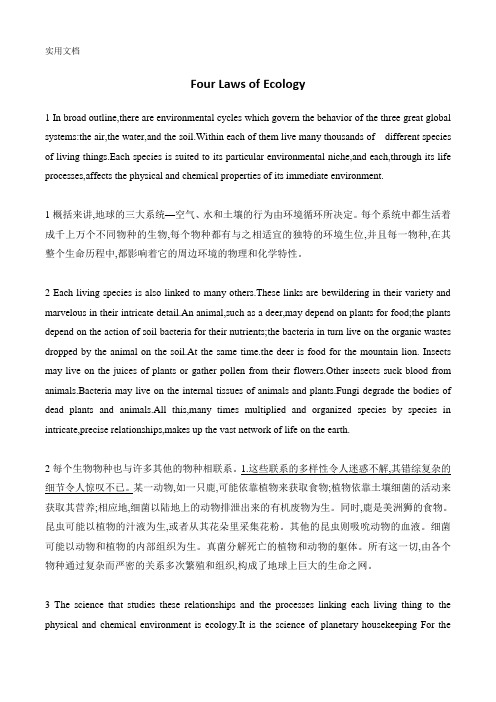
Four Laws of Ecology1 In broad outline,there are environmental cycles which govern the behavior of the three great global systems:the air,the water,and the soil.Within each of them live many thousands of different species of living things.Each species is suited to its particular environmental niche,and each,through its life processes,affects the physical and chemical properties of its immediate environment.1概括来讲,地球的三大系统—空气、水和土壤的行为由环境循环所决定。
每个系统中都生活着成千上万个不同物种的生物,每个物种都有与之相适宜的独特的环境生位,并且每一物种,在其整个生命历程中,都影响着它的周边环境的物理和化学特性。
2 Each living species is also linked to many others.These links are bewildering in their variety and marvelous in their intricate detail.An animal,such as a deer,may depend on plants for food;the plants depend on the action of soil bacteria for their nutrients;the bacteria in turn live on the organic wastes dropped by the animal on the soil.At the same time.the deer is food for the mountain lion. Insects may live on the juices of plants or gather pollen from their flowers.Other insects suck blood from animals.Bacteria may live on the internal tissues of animals and plants.Fungi degrade the bodies of dead plants and animals.All this,many times multiplied and organized species by species in intricate,precise relationships,makes up the vast network of life on the earth.2每个生物物种也与许多其他的物种相联系。
Slow
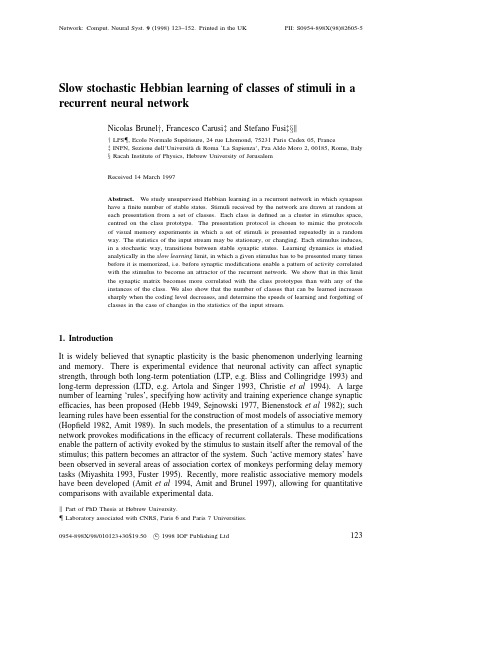
Network:Comput.Neural Syst.9(1998)123–152.Printed in the UK PII:S0954-898X(98)82605-5 Slow stochastic Hebbian learning of classes of stimuli in a recurrent neural networkNicolas Brunel†,Francesco Carusi‡and Stefano Fusi‡§†LPS¶,Ecole Normale Sup´e rieure,24rue Lhomond,75231Paris Cedex05,France‡INFN,Sezione dell’Universit`a di Roma‘La Sapienza’,Pza Aldo Moro2,00185,Rome,Italy§Racah Institute of Physics,Hebrew University of JerusalemReceived14March1997Abstract.We study unsupervised Hebbian learning in a recurrent network in which synapseshave afinite number of stable states.Stimuli received by the network are drawn at random ateach presentation from a set of classes.Each class is defined as a cluster in stimulus space,centred on the class prototype.The presentation protocol is chosen to mimic the protocolsof visual memory experiments in which a set of stimuli is presented repeatedly in a randomway.The statistics of the input stream may be stationary,or changing.Each stimulus induces,in a stochastic way,transitions between stable synaptic states.Learning dynamics is studiedanalytically in the slow learning limit,in which a given stimulus has to be presented many timesbefore it is memorized,i.e.before synaptic modifications enable a pattern of activity correlatedwith the stimulus to become an attractor of the recurrent network.We show that in this limitthe synaptic matrix becomes more correlated with the class prototypes than with any of theinstances of the class.We also show that the number of classes that can be learned increasessharply when the coding level decreases,and determine the speeds of learning and forgetting ofclasses in the case of changes in the statistics of the input stream.1.IntroductionIt is widely believed that synaptic plasticity is the basic phenomenon underlying learning and memory.There is experimental evidence that neuronal activity can affect synaptic strength,through both long-term potentiation(LTP,e.g.Bliss and Collingridge1993)and long-term depression(LTD,e.g.Artola and Singer1993,Christie et al1994).A large number of learning‘rules’,specifying how activity and training experience change synaptic efficacies,has been proposed(Hebb1949,Sejnowski1977,Bienenstock et al1982);such learning rules have been essential for the construction of most models of associative memory (Hopfield1982,Amit1989).In such models,the presentation of a stimulus to a recurrent network provokes modifications in the efficacy of recurrent collaterals.These modifications enable the pattern of activity evoked by the stimulus to sustain itself after the removal of the stimulus;this pattern becomes an attractor of the system.Such‘active memory states’have been observed in several areas of association cortex of monkeys performing delay memory tasks(Miyashita1993,Fuster1995).Recently,more realistic associative memory models have been developed(Amit et al1994,Amit and Brunel1997),allowing for quantitative comparisons with available experimental data.Part of PhD Thesis at Hebrew University.¶Laboratory associated with CNRS,Paris6and Paris7Universities.0954-898X/98/010123+30$19.50c 1998IOP Publishing Ltd123124N Brunel et alSince the structure of the synaptic efficacies is an essential characteristic of these models, the development of a realistic synaptic dynamics is particularly important.Most models of associative memory have left aside this issue,usually taking for granted a‘covariance-type’synaptic matrix(Hopfield1982,Tsodyks and Feigel’man1988),with the underlying assumption that any single synapse is able to preserve an arbitrarily high number of stable states over long timescales.A notable exception is the Willshaw model(Willshaw et al 1969),in which each synaptic efficacy has only two states,but again this model leaves aside the question of the dynamics.Shinomoto(1987)has introduced an on-line learning dynamics,but in this model the synaptic efficacies must have a long time constant and the stimuli must be presented continuously,otherwise the network returns to the initial(before learning)state.Nadal et al(1986)and Parisi(1986)showed that modifications of the Hopfield model to prevent memory blackout during sequential learning of patterns could make the network exhibit the‘palimpsest’property(old stimuli are automatically forgotten to make room for the most recent ones).In Nadal et al(1986)at each presentation the new synaptic efficacy is the sum of the old value multiplied by a decay factor plus the contribution of the new stimulus.In Parisi(1986)the‘palimpsest’property was obtained by bounding the synaptic efficacies.More recently,the idea that synaptic efficacies have only a limited number of stable states and that a stimulus arriving at the network provokes transitions between these states began to develop as an alternate,more realistic,description of the learning process.Tsodyks (1990)showed that a drastic reduction in the memory capacity is inherent to such a learning process,as compared with the usual‘covariance’matrix.In this context,Wong et al(1991) studied the dependence of short-term memory behaviour on the initial synaptic distribution. In their study stimuli were learned in one shot and with certain choices of parameters the network exhibited primacy or recency phenomena.The idea of the learning process as a random walk was also considered,in a more general context,by Heskes and Kappen(1991).Amit and Fusi(1992,1994)have studied a learning process in which each synapse has only afinite number of stable states on long timescales,and neural activities induce stochastic transitions between these states,in the situation in which stimuli to be learned are random,uncorrelated and each of them is presented once.They pointed out the importance of(i)the stochasticity of the learning dynamics,(ii)the sparseness of the stimuli,and(iii) the global balance between numbers of potentiating and depressing transitions.Amit and Brunel(1995)used simulations to study such a learning process in a more realistic case in which stimuli are drawn randomly at each presentation from a set of classes,defined by clusters in stimulus space.The differences in the patterns of activity evoked by stimuli belonging to the same class may reflect either some noise added to the signal in the visual pathways(early preprocessing)or small differences in some of the features of the visual stimuli.For example,the stimulus degradation studied by Amit et al(1997)leads to gradual modifications in the visual response that are observable at the level of the inferotemporal cortex of a behaving monkey.Amit and Brunel(1995)demonstrated how the resulting modifications in the synaptic structure could stabilize attractors(internal representations) corresponding to the extracted prototypes of the classes of shown stimuli.The existence of an attractor state(a stable pattern of activities)correlated with a prototype defines whether the corresponding class is learned or not,since it makes it possible to maintain an active representation of this class,in the absence of the stimulus correlated with it.This is the definition of learning adopted in this paper.The present study represents an analytical approach to this learning process.The environment,from which the network learns,is defined by a set of prototypes.Each prototype defines a class,or cluster,of similar patterns of activities(patterns belongingSlow stochastic Hebbian learning125 to a class are correlated with the corresponding prototype).At discrete points in time,a randomly selected pattern is presented and synaptic transitions take place.The sequence of presentations of stimuli is composed of members of these classes,in which at each presentation both the prototype and the specific class member are chosen at random.It is a relatively common protocol of visual memory experiments.The environment(i.e.the set of prototypes or classes)is eitherfixed,or changing.In the latter case,classes are added to or removed from the set.This allows the study of the speed at which classes are learned or forgotten.Any presentation of a stimulus provokes stochastic transitions between synaptic efficacies.Each synapse has two stable states,a low background state and an elevated state.Transitions from low to high are functionally similar to LTP,while the reverse transitions are similar to LTD.The model can incorporate different types of LTD,such as homosynaptic,heterosynaptic,or both.Such a model of unsupervised synaptic plasticity can be easily implemented in a material device(Badoni et al1995,Annunziato1995).In Amit and Fusi(1994)the memory capacity of this model had been estimated by assuming that each pattern can be learned after a single presentation.By contrast,in some areas in which attractors are observed,e.g.inferotemporal(IT)cortex,a large number of presentations of the same pattern seem to be required to produce reverberating activity (Miyashita1993),which suggests a rather slow learning process.In this paper we shall study such a slow learning situation,i.e.a scenario in which a given pattern has to be shown many times before it is learned.1.1.Summary of the main resultsThe main results in the slow learning limit are as follows.1.1.1.Dependence on the sequence of presentations.In the slow learning limit and if there are no temporal correlations in the sequence(i.e.choices of the class to be presented at any two different times are uncorrelated),the degree of correlation between the synaptic matrix and a given prototype,which determines whether this prototype is learned or not,does not depend on the specific sequence of presentations of stimuli but only on the set of stimuli in the environment.Thefinal configuration of the synaptic matrix contains information about the statistics of the last stimuli presented,those appearing in a sliding time window whose length depends on synaptic transition probabilities.For afixed environment,as the transition probability goes to zero,this time window gets longer and(i)the number of times a given prototype appears in it increases;(ii)for a given presentation,the number of modified synapses decreases.This means that,following any presentation,a smaller amount of information is acquired,and also that a smaller fraction of synapses forgets the patterns presented in the past.Thefinal outcome is a synaptic matrix which is less biased toward the most recent stimuli compared to conventional palimpsest memories(Nadal et al1986, Parisi1986).Nevertheless,the system is still able to adapt itself to new environments since the sliding time window isfinite.The price to be paid is a longer adaptation time:as the transition probability decreases the adaptation time increases.1.1.2.Categorization properties.If learning is slow enough,the memory of the class prototypes is always stronger than the memory of any other members of the same class shown.Thus,the learning process naturally categorizes the input stimuli by extracting a good representative of a class of similar patterns in the stream of stimuli.If the members are generated as clouds aroundfixed prototypes,the extracted representatives become closer126N Brunel et aland closer to the real prototypes as the sliding memory window becomes larger,i.e.when the transition probabilities get smaller.Intuitively,a larger memory window means a larger sampling of the entire class.In this paper we focus on the limit of very small transition probabilities,and we show that in this limit the extracted prototypes coincide with the prototypes underlying the stream of stimuli.If the environment changes and the prototypes move in the space of all possible activity configurations,then the synaptic configuration is able to adapt to new prototypes,provided that they move slowly enough.1.1.3.Storage capacity.This is defined as the maximum number of classes that can be memorized.It depends on the sparseness of the internal representations of the stimuli(the mean fraction of neurons activated by each stimulus):the sparser the representation,the larger the storage capacity.A good storage capacity also requires a global balance between LTP and LTD,as in(Amit and Fusi1994).Moreover,we show that it is independent of the specific implementation of LTD provided the global balance between LTP and LTD is preserved.1.1.4.Learning and forgetting rates.We define the learning rate as the inverse of the typical number of repetitions of a new class which must be presented in order for it to be learned.The time at which a new class becomes recallable depends on this number of repetitions and on the frequency with which the network is presented the stimuli of the environment.We also consider a situation in which a class is removed from the environment and the network is still presented a stream of uncorrelated stimuli belonging to other classes. In this case,the removed class is forgotten after a certain number of presentations of each remaining class.The forgetting rate is defined as the inverse of this number of presentations.Learning and forgetting rates are proportional to the LTP/LTD transition probabilities. The learning rate is almost independent of the number of classes present in the environment, except when it is close to the storage capacity.Near the limit of storage capacity it decreases sharply,owing to the fact that all the information about a specific class is forgotten in the interval between two successive presentations of the members of the class.The forgetting rate is much smaller than the learning rate when there are few classes in the environment, but increases as the number of classes increases,until it eventually becomes larger than the learning rate.The forgetting rate becomes infinite when the storage capacity is reached.These results are demonstrated both analytically and by simulations.The organization of the paper is as follows:first we define the model neurons and the external stimuli. In section2we define the synaptic dynamics.In section3we calculate the synaptic distribution for a generic sequence and in section4we study the slow learning situation. Then in section5we study the sparse coding limit.This allows us to determine relatively simple expressions for the quantities of stly,in section6we describe the results of the simulations that confirm the main analytical results.2.The model2.1.Neuronal response to stimuliWe consider a network composed of a large number of neurons,which are taken to represent the pyramidal cells of a cortical network.Each neuron in the network is labelled by an index i=1,...,N where N is the number of neurons.In this paper we do not consider theSlow stochastic Hebbian learning127 neuronal dynamics explicitly,but rather consider the steady state imposed on the network by an external stimulus.For simplicity,any stimulus leaves neuron i at one of two possible activity states,V i i=0,1which may be related to spike rates:V i=V0the neuron shows novisual response(spontaneous activity state);V i=V1≫V0the neuron has visual response. The ensemble of activations in the network by a given stimulus represents the way in which this stimulus is encoded in the network.A stimulus shown at time t can therefore be characterized by a binary string{ξti=0,1}, which determines the activation of all neurons in the network during its presentation:V i(t)= V1ifξt i=1V0ifξti=0.The population of neurons which is activated by a given stimulus will be called the foreground of this stimulus.The remaining neurons define its background.2.2.Statistics of stimuli:classesStimuli shown to the network belong to a set of p predetermined classes,which defines the‘environment’of the network.Each class is defined by a representative pattern,the prototypeηµ,µ=1,...,p.Prototypes are random and uncorrelated N-bit words chosen according toPr(ηµi=1)=f Pr(ηµi=0)=1−f(1) where f is the coding level(or sparseness)of the class prototypes.Each prototype defines its corresponding class(or cluster)of stimuli.The members of a class are noisy versions of the prototype.In a visual memory experiment the noise may be interpreted as follows.•Noise due to preprocessing in the early visual stages or to small eye movements:even if the animal is always presented the same stimulus,the pattern of activity in the network might be different from presentation to presentation because of the noise generated by other networks.•Degradation of the visual stimulus:the patterns of activities corresponding to the members of a specific class,are the outcome of the degradation of the visual stimulus(e.g.when RGB noise is added to the prototype,as in Amit et al(1997)).•Small changes in the visual stimuli(e.g.in one or more of the features)that induce modifications in the visual response of the neurons of the network.The similarity of the visual stimuli is reflected by the correlations between the prototype and stimuli belonging to the same class.A stimulusηµνbelonging to classµis chosen randomly in the following way.•If the neuron is in the foreground of the prototype,ηµi=1:Pr(ηµνi=1)=1−x(1−f)Pr(ηµν=0)=(1−f)x.(2)•If the neuron is in the background of the prototype,ηµi=0:Pr(ηµν=1)=f x Pr(ηµν=0)=1−f x.(3) x measures the extent of a given class or the distance between a typical instance and its class prototype.If x=0,instances are identical to their prototype.If x=1,examples are uncorrelated with their prototype.This procedure ensures that the average fraction of activated neurons is f.128N Brunel et alA sequence of presentations specifies which stimulus is shown at each time step t.In a random sequence,a class is selected at random at each presentation with equal probability 1/p,and then an instance is generated at random from the class using equations(2)and (3).The formalism developed in this paper could easily be generalized to the case in which the probabilities of presentation are different from class to class.Choices of the class and of the class member at two different presentations are uncorrelated.The case of temporal correlations in the sequence of presentations will be considered in a future study(see also (Brunel1996)for a study of learning of temporal correlations in a simplified situation in which stimuli activate non-overlapping sets of neurons).In the following,ξt always denotes a generic stimulus shown at time t,ηµa class prototype,ηµνan instanceνof classµ.2.3.Synaptic dynamics and the standard learning modelTwo essential features characterize the synaptic dynamics:the fact that each synapse is discrete with afinite number of states(limited analogue depth);and the stochasticity of transitions occurring from state to state.In a material neural network the elements are assumed to be implementable in simple devices(electronic or biochemical).It is unlikely that a biological or an electronic device could preserve a large set of stable values during long time intervals,in the absence of a stimulus.In what follows we assume that on long timescales the synaptic efficacy has only two stable states because:(i)such a structure is sufficient for learning selective delay activity;(ii)it simplifies the calculations and the design of the electronic implementation of the synapse;(iii)the discreteness of synaptic efficacies is consistent with experiment(Bliss and Collingridge1993).The synaptic efficacy connecting neuron j to neuron i is denoted by J ij.All synaptic efficacies have two stable states,denoted by J0<J1.In the following,for simplicity,we use J1=1,J0=0,without loss of generality.Stochastic transitions between these states may occur if either the presynaptic or the postsynaptic neuron is active during presentation of a stimulus.At time t,when a stimulus {ξt i}is presented:•If J ij=0,then J ij→1with probability a(ξt i,ξt j)(LTP transition).a(ξt i,ξt j)can be written asa(ξt i,ξt j)=q+p(ξt i,ξt j)where q+is the intrinsic potentiation probability,and p(ξt i,ξt j)∈[0,1]is a function carrying the dependence on the activities of the two neurons connecting the synapse.If the two activitiesξti ,ξtjare such that p=1,then an LTP transition occurs withprobability q+.Otherwise p=0and no LTP transition can occur.•If J ij=1,then J ij→0with probability b(ξt i,ξt j)(LTD transition).Again,we can writeb(ξt i,ξt j)=q−d(ξt i,ξt j)where q−is the intrinsic depression probability,and d(ξt i,ξt j)∈[0,1].Thus,learning is a random walk among the two stable states of synaptic efficacy,and any synapse is characterized at any time t by a probability distribution(Pr(J ij=1,t),Slow stochastic Hebbian learning129 Pr(J ij=0,t)),which depends on all stimuli which have been presented to the network before time t.The formalism developed in this paper applies to any functions p and d describing LTP and LTD.In most of the following,however,we shall restrict ourselves to a symmetric learning dynamics introduced in(Amit and Fusi1994,Amit and Brunel1995)which captures features of experimental results on synaptic plasticity(Bliss and Collingridge1993, Christie et al1994):p(ξt i,ξt j)=ξt iξt j(4) corresponding to LTP obtained only when both presynaptic and postsynaptic neurons are sufficiently depolarized;andd(ξt i,ξt j)=ξt i(1−ξt j)+ξt j(1−ξt i)(5) corresponding to LTD in the case of either postsynaptic but not presynaptic activity (heterosynaptic LTD),or presynaptic but not postsynaptic activity(homosynaptic LTD). This learning dynamics will be referred to as the standard learning model(SLM)in the following.In section5.3we shall compare different types of LTD characterized by different mixtures of homosynaptic and heterosynaptic LTD:d(ξt i,ξt j)=uξt i(1−ξt j)+vξt j(1−ξt i)(6) where u(v),varying in the interval[0,1],is the relative strength of heterosynaptic (homosynaptic)LTD.2.4.Measures of the degree of learningTo monitor the effect of learning on the synaptic matrix we define the following quantities.(i)The mean potentiation g at time t:g(t)=1N(N−1) i=j J ij(t)(7)is the average fraction of potentiated synapses in the network at time t.(ii)The mean‘intra-class’(ICP)potentiation gµof the prototype of classµ(Amit and Brunel1995)gµ(t)=1f N(f N−1) i,j J ij(t)ηµiηµj(8)measures the correlation between the prototype of classµand the synaptic matrix, and thus of the degree of learning of the corresponding class.If gµ=g there is no correlation between the class and the synaptic matrix.(iii)The mean‘intra-example’(IEP)potentiation Gµνof instanceνof classµ:gµν(t)=1f N(f N−1) i,j J ij(t)ηµνiηµνj(9)measures the correlation between a particular instance and the synaptic matrix.It can be used to evaluate whether the synaptic matrix is more correlated with instances or with their class prototypes.130N Brunel et alRealistic associative memory models (see e.g.Amit and Brunel 1995,1997)show that,depending on the value of g +relative to g ,two types of behaviour are observed.When g +−g < g c ,where g c depends on the details of the neuronal model,the network is unable to sustain attractors correlated with learned stimuli.In this case,the only stable state in the network is a completely silent state or a spontaneous activity state in a network of spiking neurons with strong recurrent inhibition.On the other hand,when the ICP becomes significantly larger than the mean potentiation in the network,network states correlated with the class prototype become stable.The precise criteria depend on the neuronal model;for the particular case of a network of analogue neurons characterized by a continuous transfer function we have typically g c ∼0.5.On the other hand,the relative values of the IEP and the ICP determine whether the attractor stabilized by the network is more correlated with the class prototype (thus categorizing the input stimuli)or with the most recent instance of the class.3.Synaptic distribution for a generic sequence3.1.Sources of stochasticity:learning as a Markov processThe intrinsic stochastic mechanism which drives transitions from one stable state to another is not the only source of stochasticity.Learning can be considered stochastic either due to the dynamics of the synaptic modifications or due to the nature of the data shown to the network (Amit and Fusi 1994,Heskes and Kappen 1991).In fact,the sequence of random uncorrelated stimuli presented to the network represents a sequence of random activity levels imposed on the synapse.Since the learning dynamics is local in time,the presentation of a sequence of uncorrelated random stimuli induces a Markov process on the set of values of each synapse.In our case the synapse has only two stable states,so the synaptic dynamics can be fully described in terms of the transition probabilities of transferring to the up/down state.3.2.Probability distribution as an explicit function of the sequence of stimuliWe start our analysis with the computation of the final synaptic distribution as an explicit function of the activities ηµi presented to the synapse by each stimulus of the sequence.The transition matrix corresponding to the Markov process of a single presentation at time t can be written as M ij (t)= 1−a t ij a t ij b t ij1−b t ij where a t ij =a(ξt i ,ξt j )(b t ij =b(ξt i ,ξt j ))is the probability that synapse J ij is potentiated (depressed)following the presentation of pattern ξt at time t ,as defined in section 2.3.From this transition matrix,we can find the probability distribution of the synaptic efficacies at time T ,as a function of the initial distribution and of all stimuli presented between 0and T .The probabilities of the synapse ij being in the excited state (G ij (T )≡Pr (J ij =1,T ))or in the background state (1−G ij (T )≡Pr (J ij =0,T ))at time T are calculated explicitly in appendix A.We obtain:G ij (T )=T t =1a t ij T s =t +1λs ij +G ij (0)T s =1λs ij (10)Slow stochastic Hebbian learning131in whichλtij=1−a t ij−b t ij.λt ij appears in equation(10)as an‘instantaneous decay factor’at time t:it is the probability that no transition occurred at time t,given that stimulusξt had been presented.In equation(10),each term in the sum on the right-hand side(RHS) corresponds to a stimulus presented at time t<T.These terms are weighted by the‘decay’factor sλs provoked by successive presentations.This factor is the probability that no synaptic transition occurred between time t and time T.In this way,the contributions of earlier stimuli are obscured by more recent ones.If there is afinite probability that potentiating and(or)depressing stimuli occur in the sequence,after a sufficiently long time (T→∞)the second term in the RHS of equation(10)vanishes,i.e.the synapse forgets its initial condition,and we obtainG ij(T)= t T a t ij T s=t+1λs ij.(11)This is the case in which at least one of the prototypes in the‘environment’tends to potentiate or depress the synapse,or where the size of clusters around the prototypes is finite(x>0).Note that the synaptic distribution,equation(11),depends,in the case of the presentation protocol described in section2.2,on•the set of classes;•the particular realization of the(random)sequence of presentation of classes,i.e.the specification of the classes shown at each time step;•and,in the case of classes offinite extent,the extraction of class members at each time step.Thus the distribution of synaptic efficacies depends on the specification of the entire sequence of stimuli.On the other hand,one would like to know the properties of the synaptic matrix for a‘typical’sequence.We shall see in section4that this is possible when the transition probabilities q+,q−are small:in this case the average over all possible realizations of the sequence of presentations gives a good approximation for most realizations,in the sense that the variability of the average potentiation levels from sequence to sequence goes to zero with the transition probabilities.4.Slow learning:averaging over random sequencesTo study the properties of the synaptic matrix in the slow learning scenario we shall proceed as follows.(i)We start from the general expression for the synaptic distribution following thepresentation of a specific,arbitrary sequence of stimuli(equation(10)).This is anexplicit function of the pairs of activities(ξti ,ξtj)imposed on the synapse by all thestimuliξti (0<t<T)of the input stream.(ii)The synaptic distribution is then averaged over all possible realizations of the random sequences defined in section2.2.(iii)Next we calculate the average over all sequences of the potentiation levels g and gµof equations(7)and(8).(iv)Finally we compute the variability of these potentiation levels from sequence to sequence in section4.3,andfind that when learning is sufficiently slow,this variability becomes negligible with respect to the average potentiation levels.。
- 1、下载文档前请自行甄别文档内容的完整性,平台不提供额外的编辑、内容补充、找答案等附加服务。
- 2、"仅部分预览"的文档,不可在线预览部分如存在完整性等问题,可反馈申请退款(可完整预览的文档不适用该条件!)。
- 3、如文档侵犯您的权益,请联系客服反馈,我们会尽快为您处理(人工客服工作时间:9:00-18:30)。
A formal derivation of Heaps’Law∗F.A.Grootjen and D.C.van Leijenhorst and Th.P.van der WeideJanuary28,2003AbstractIn this paper Heaps’Law is derived from the Mandelbrot distribution.1IntroductionIn many practical situations,a connection has been shown between the order of probability of events,and the probability itself.The most well-known models for such connections are Zipf’s Law([6])and the Mandelbrot distribution([4]).Let the r-th most probable event have probability p,then Zipf’s law states that p·r is(almost)equal for all events,while the Mandelbrot distribution claims this for the expression p·(c+r)θfor some parameters c andθ.In case of c=0,the distribution is also referred to as the generalized Zipf’s Law.Some authors motivate the validity of these laws from physical phenomena,see for example[7]for Zipf’s Law in the context of cities. But it is also possible to derive Zipf’s law from a simple statistical model([3]).For example, Zipf’s Law can be derived for word occurrences in natural language,when it is assumed that words are drawn randomly from some distribution.In practice,however,words are thoughtfully selected by the author;yet on the long run this selection process may adjust to such a statistical description.Another experimental law of nature is Heaps’Law([2]),which describes the growth in the num-ber of unique elements(also referred as the number of records),when elements are drawn from some distribution.Heaps’Law states that this number will grow according toαkβfor some ap-plication dependent constantsαandβ,where0<β<1.In the case of word occurrences in natural language,Heaps’Law predicts the vocabulary size from the size of a text.In[1]Heaps’Law and the generalized Zipf’s Law are related.It is shown that under a plausible assumption,it can be derived that if both Heaps’law and the generalized Zipf’Law hold,β= 1/θ.The argumentation is as follows.Heaps’Law predicts the vocabulary size in a text of a given size.It is assumed that the frequency of the least frequent word in this text isΘ(1).As a result, the prediction of this frequency as obtained by the generalized Zipf’s is alsoΘ(1).Working out the details leads toβ=1/θ.In this paper,we take another approach.We assume the generation of text is according to the Mandelbrot distribution,and derive Heaps’Law for the average vocabulary size in a text of a given length.This is done by a statistical analysis,leading to a rather untractable recurrence relation.As a consequence,Heaps’Law can also be regarded in a natural way as a complexity estimate.By applying techniques from complexity theory,restricting ourselves tofirst order terms,Heaps’Law is obtained.Note that by involving second order terms,a more advanced formulation of Heaps’Law may be obtained.Infigure1we see how nicely the functionαkβcan befitted against the function describing the average number records as a function of the number of drawings in the case of a set of100 elements,while infigure1a set of1000elements is taken.This will be explained in more detail in section2.It will be clear from thesefigures however that the approximation provided by Heaps’∗Technical Report NIII-R030201020304050607080901000500100015002000250030003500400045005000N (k ) v s H e a p s (k )kHeaps Law: N = 100 theta = 1.75 c = 0.30 a N = 0.70N(k)f(k)Figure 1:N k versus Heaps law fit for N =100Law is not valid everywhere,as obviously the number of records is bounded by the total number of events,while a power function will exceed this number eventually.In order to express this limited validity of Heaps’Law,we also focus on the validity area of the approximations in our analysis.The validity area is described rather defensively,in practice the area will be larger.In section 2we present a statistical model for the vocabulary size in a text,i.e.the average number of unique occurrences after a series of drawings.In section 3we solve the resulting equation,leading to Heaps’Law.We also give bounds for the validity area of the approximations.In section 4we make some conclusions and discuss further research.2A probabilistic model for Heaps’lawLet W be a set of N words numbered 1...,N ,and let p i the probability that word i is chosen.The underlying text model is that words are subsequently taken from the set W according to this probability distribution.We will be interested in the asymptotic behavior of the expected resulting number of different words taken.After taking k words w 1,...,w k from W ,let D k ={w 1,...,w k }be the set of different words,and let n k be the number of such words:n k =#D k .Then:Prob (n k =a )=Prob (n k −1=a −1∧w k ∈D k −1)+Prob (n k −1=a ∧w k ∈D k −1)=Prob (n k −1=a −1)∗Prob (w k ∈D k −1)+Prob (n k −1=a )∗Prob (w k ∈D k −1)10020030040050060005000100001500020000250003000035000400004500050000N (k ) v s H e a p s (k )kHeaps Law: N = 1000 theta = 1.75 c = 0.30 a N = 0.68N(k)f(k)Figure 2:N k versus Heaps’law fit for N =1000Then obviously:Prob (w k ∈D k −1)=i ∈WProb (w k =i ∧i ∈D k −1)(1)=i ∈Wp i (1−p i )k −1(2)Let S k = i ∈W p i (1−p i )k −1,and M n =i ∈W (1−p i )n .We will refer to M n as the k -th reverse moment of the probability distribution.Then we obtain S k =M k −1−M k ,as:i ∈Wp i (1−p i )k −1= i ∈W−(1−p i )k +(1−p i )k −1Let N (k,a )=Prob (n k =a ),then we haveka =1N (k,a )=1.From the equations above we getthe following recurrence relation::N (1,1)=1N (k,a )=0if k <aN (k,a )=N (k −1,a −1)∗S k +N (k −1,a )∗(1−S k )if k ≥aWe will be interested in the expected number of different words N k after taking k words ran-domly from the set W of ing this recurrence relation,we get for N k (k >1)the follow-ing recurrence relation:N k=k a =1a ∗N (k,a )=k a =1a ∗(N (k −1,a −1)∗S k +N (k −1,a )∗(1−S k ))=S k k a =1a ∗N (k −1,a −1)+(1−S k )k a =1a ∗N (k −1,a )=S kka =1(a −1)∗N (k −1,a −1)+k a =1N (k −1,a −1)+(1−S k )N k −1=S k ∗N k −1+S k ∗k −1 a =1N (k −1,a )+(1−S k )N k −1=N k −1+S kAs a consequence:Lemma 1The expected number of different words in a random selection of k words is N k =N −M k .Proof:N k =N 0+ kj =1S j =M 0−M k =N −M k In order to estimate the asymptotic behavior of N k ,we will estimate M k in the next section.20406080100120010002000300040005000600070008000900010000N (k ) v s H e a p s (k )kHeaps Law: N = 100 theta = 1.75 c = 0.30 a N = 0.70N(k)f(k)Figure 3:N k versus Heaps law fit for N =100Applying curve fitting,we get some idea of the quality of this approximation provided by Heaps’Law.In figure 1we see how Heaps’Law fits when 5000elements are drawn from a set of 100elements,while in figure 22000drawings are taken.We see how the fit deteriorates.The same can be seen for larger values of N ,for example,figure 1shows the fits after 50000drawings,and the worse situation in figure ??after 500000drawings.20040060080010001200050000100000150000200000250000300000350000400000450000500000N (k ) v s H e a p s (k )kHeaps Law: N = 1000 theta = 1.75 c = 0.30 a N = 0.68N(k)f(k)Figure 4:N k versus Heaps law fit for N =10003Approximating reverse moments for Mandelbrot distributionThe Mandelbrot distribution provides a reasonable approximation of frequency of word usage in natural language.The Mandelbrot distribution assumes words to be ranked according to their frequency of usage.The probability of the word ranked at position i then corresponds to:p i =a N (c +i )for some constants c ≥0and θ,where a N is such that Ni =1p i =ually the constant θranges over [1,2].As special case is Zipf’s Law,which results from the Mandelbrot distribution by choosing θ=1and c =0.For the normalization constant we have:Lemma 2θ>1=⇒a N =Θ(1)Let t (x )=a N (c +x )−θ,thenLemma 3For k >0the function φk (x )=(1−t (x ))kis increasing for x ≥1.•lim k →∞φk (x )=0•lim x →∞φk (x )=1The reverse moments may be estimated using the integral criterion:Lemma 4N i =1φk (i )=N1φk (x )dx +with φk (1)≤ ≤φk (N )=(1−a N (c +N )−θ)k =o (1).The error of replacing summation by integration thus decreases exponentially in both k and N .Next we focus on estimating N1φk (x )dx .By substituting t =a N (c +x )−θ,leading to dx =−At µdtwhere µ=−1−βand A =βa βN and β=1θ,we get:Lemma 5N11−a N(c +x )θkdx =At 1t N(1−t )k t µdtwith t 1=a N (c +1)−θ,t N =a N (c +N )−θ.We will process this outcome by applying partial integration:Lemma 6At 1t N(1−t )k t µdt=A (1−t )k t µ+1µ+1 t 1t N−Aθk t 1t N (1−t )k −1t µ+1dt The first term of the righthand side approximates the number N of words in the set W :Lemma 7A (1−t )k t µ+1µ+1 t 1t N=(c +N )φk (N )−(c +1)φk (1)=N +Θ k (c +N )Proof:A (1−t )k t µ+1µ+1 t 1t N=A (1−t 1)k t µ+11µ+1−A(1−t N )k t µ+1Nµ+1=−(c +1)(1−t 1)k +(c +N )(1−t N )k =(c +N )φk (N )−(c +1)φk (1)The result follows by the observation:φk (N )=1−a N(c +N )θk=1+Θk (c +N )θFor small values of k ,the term Nφk (N )is around N ,but for larger values,this term decreases exponentially.Next we focus on Aθk t 1t N (1−t )k −1t µ+1dt .Lemma 8Aθkt 1t N(1−t )k −1t µ+1dt =Aθk1(1−t )k −1t µ+1dt − 2where2=Θk(c +N )θ−1+O k 2(1−t 1)k −1The latter term is exponentially decreasing in k .Proof:Obviously2=Aθkt N0(1−t)k−1tµ+1dt+Aθk1t1(1−t)k−1tµ+1dtFor thefirst term we notice that t N will be almost0for large N and thus1−t=Θ(1). Consequently:Aθkt N0(1−t)k−1tµ+1dt=ΘAθkt Ntµ+1dt=ΘaβNt1−βN1−βk=Θa N1−β·k(c+N)θ−1For the second term another application of partial integration is performed:Aθk1t1(1−t)k−1tµ+1dt=Aθk(1−t)k−1tµ+2µ+21t1+Aθk(k−1)µ+21t1(1−t)k−2tµ+2dtBoth terms are exponentially decreasing in k.For thefirst part we have:Aθk(1−t)k−1tµ+21t1=−Aθtµ+21·k(1−t1)k−1Note that k(1−t1)k−1decreases exponentially to zero.This is also the case for the second part:Aθk(k−1)µ+2 1t1(1−t)k−2tµ+2dt≤Aθk(k−1)µ+21t1(1−t)k−2dt≤Aθµ+2·k(k−1)(1−t1)k−1We proceed with Aθk 1(1−t)k−1tµ+1dt,and recognize this integral as the Beta-function B(µ+2,k).Lemma9Aθk1(1−t)k−1tµ+1dt=Aθk B(k,µ+2) The Beta-function can be expressed in terms of the Gamma-function:B(k,µ+2)=Γ(k)Γ(µ+2)Γ(k+µ+2)Note that expression is only valid forµ+2=0,which is equivalent withθ=1.In this paper we restrict ourselves to this case.Substituting Stirling’s approximation of theΓ-functionΓ(x+1)=√2πxx x e−x(1+o(1)(see[5])on the termsΓ(k)andΓ(k+µ+2)yields:AθkΓ(k)Γ(k+µ+2)Γ(µ+2)∼Aθk√2π(k−1)k−1e−k+1(k−1)12√2π(k+µ+1)k+µ+1e−k−µ−1(k+µ+1)1Γ(µ+2)=aβN ·k−1k+µ+1k·k−1k+µ+112·eµ+2·(k+µ+1)−µ−1·(1−1k)·Γ(µ+2)∼aβNeµ+2Γ(µ+2)(k+µ+1)−µ−1∼aβNeµ+2Γ(µ+2)k−µ−1=aβNe1−βΓ(1−β)·kβSummarizing we haveLemma10Ni=1φk(i)=N−α·kβ·(1+o(1))+Θk(c+N)whereα=e1−βΓ(1−β)This leads to the main result:Lemma11The expected number of different words in a random selection of k words isN k=α·kβ·(1+o(1))+Θk(c+N)θAnd thus we have shown:Theorem1(Heaps’Law)N k=α·kβ,with validity interval restricted to k=Θ((c+N)θ).4Conclusions and further researchIn this paper we have derived the Heaps’Law from the Mandelbrot distribution,and provided a validity area for Heaps’Law.As a next step,a second order approximation may be employed, providing a sharper formulation for Heaps’Law,and a larger validity area.Furthermore,other distributions may be examined,leading to Heaps’Criterion as a sufficient condition for a distribu-tion to imply Heaps’Law.References[1]Ricardo A.Baeza-Yates and Gonzalo Navarro.Block addressing indices for approximate textretrieval.Journal of the American Society of Information Science,51(1):69–82,2000.[2]rmation retrieval:Computational and theoretical aspects.pages206–208,1978.[3]Wentian Li.Random texts exhibit zipf’s-law-like word frequency distribution.IEEE Transac-tions on Information Theory,38(6)1842-1845,1992.[4]B.Mandelbrot.The pareto-levy law and the distribution of income.International EconomicReview,I,pages79–106,1960.[5]Schaum.Schaums Handbook of Formulas and Tables.[6]G.Zipf.Human Behavior and the Principle of Last Effort.1949.[7]Xavier Gabaix Zipf.Zipf’s law for cities:An explanation*.。