an adaptively spatial color gamut mapping algorithm
英文翻译
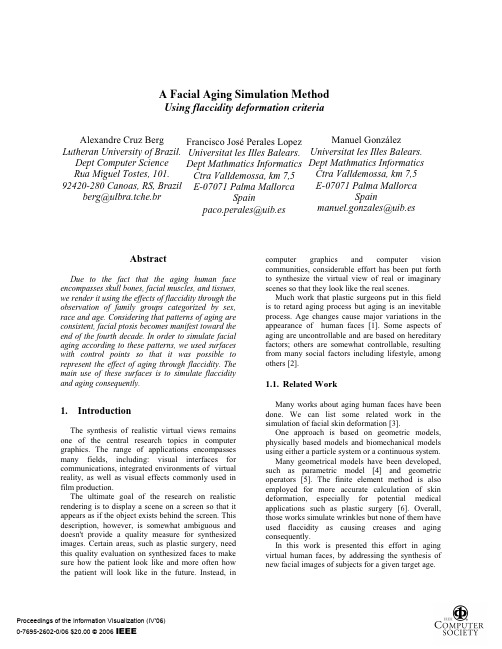
A Facial Aging Simulation Method Using flaccidity deformation criteriaAlexandre Cruz Berg Lutheran University of Brazil.Dept Computer ScienceRua Miguel Tostes, 101. 92420-280 Canoas, RS, Brazil berg@ulbra.tche.br Francisco José Perales LopezUniversitat les Illes Balears.Dept Mathmatics InformaticsCtra Valldemossa, km 7,5E-07071 Palma MallorcaSpainpaco.perales@uib.esManuel GonzálezUniversitat les Illes Balears.Dept Mathmatics InformaticsCtra Valldemossa, km 7,5E-07071 Palma MallorcaSpainmanuel.gonzales@uib.esAbstractDue to the fact that the aging human face encompasses skull bones, facial muscles, and tissues, we render it using the effects of flaccidity through the observation of family groups categorized by sex, race and age. Considering that patterns of aging are consistent, facial ptosis becomes manifest toward the end of the fourth decade. In order to simulate facial aging according to these patterns, we used surfaces with control points so that it was possible to represent the effect of aging through flaccidity. The main use of these surfaces is to simulate flaccidity and aging consequently.1.IntroductionThe synthesis of realistic virtual views remains one of the central research topics in computer graphics. The range of applications encompasses many fields, including: visual interfaces for communications, integrated environments of virtual reality, as well as visual effects commonly used in film production.The ultimate goal of the research on realistic rendering is to display a scene on a screen so that it appears as if the object exists behind the screen. This description, however, is somewhat ambiguous and doesn't provide a quality measure for synthesized images. Certain areas, such as plastic surgery, need this quality evaluation on synthesized faces to make sure how the patient look like and more often how the patient will look like in the future. Instead, in computer graphics and computer vision communities, considerable effort has been put forthto synthesize the virtual view of real or imaginary scenes so that they look like the real scenes.Much work that plastic surgeons put in this fieldis to retard aging process but aging is an inevitable process. Age changes cause major variations in the appearance of human faces [1]. Some aspects of aging are uncontrollable and are based on hereditary factors; others are somewhat controllable, resulting from many social factors including lifestyle, among others [2].1.1.Related WorkMany works about aging human faces have been done. We can list some related work in the simulation of facial skin deformation [3].One approach is based on geometric models, physically based models and biomechanical models using either a particle system or a continuous system.Many geometrical models have been developed, such as parametric model [4] and geometric operators [5]. The finite element method is also employed for more accurate calculation of skin deformation, especially for potential medical applications such as plastic surgery [6]. Overall, those works simulate wrinkles but none of them have used flaccidity as causing creases and aging consequently.In this work is presented this effort in aging virtual human faces, by addressing the synthesis of new facial images of subjects for a given target age.We present a scheme that uses aging function to perform this synthesis thru flaccidity. This scheme enforces perceptually realistic images by preserving the identity of the subject. The main difference between our model and the previous ones is that we simulate increase of fat and muscular mass diminish causing flaccidity as one responsible element for the sprouting of lines and aging human face.In the next section will plan to present the methodology. Also in section 3, we introduce the measurements procedure, defining structural alterations of the face. In section 4, we present a visual facial model. We describe age simulation thrua deformation approach in section 5. In the last section we conclude the main results and future work.2.MethodologyA methodology to model the aging of human face allows us to recover the face aging process. This methodology consists of: 1) defining the variations of certain face regions, where the aging process is perceptible; 2) measuring the variations of those regions for a period of time in a group of people and finally 3) making up a model through the measurements based on personal features.That could be used as a standard to a whole group in order to design aging curves to the facial regions defined.¦njjjpVM2.1Mathematical Background and AnalysisHuman society values beauty and youth. It is well known that the aging process is influenced by several parameters such: feeding, weight, stress level, race, religious factors, genetics, etc. Finding a standard set of characteristics that could possibly emulate and represent the aging process is a difficult proposition.This standard set was obtained through a mathematical analysis of some face measurements in a specific group of people, whose photographs in different ages were available [7]. To each person in the group, there were, at least, four digitized photographs. The oldest of them was taken as a standard to the most recent one. Hence, some face alterations were attained through the passing of time for the same person.The diversity of the generated data has led to the designing of a mathematical model, which enabled the acquiring of a behavior pattern to all persons of the same group, as the form of a curve defined over the domain [0,1] in general, in order to define over any interval [0,Į] for an individual face. The unknown points Įi are found using the blossoming principle [8] to form the control polygon of that face.The first step consisted in the selection of the group to be studied. Proposing the assessment of the face aging characteristics it will be necessary to have a photographic follow-up along time for a group of people, in which their face alterations were measurable.The database used in this work consisted of files of patients who were submitted to plastic surgery at Medical Center Praia do Guaíba, located in Porto Alegre, Brazil.3.MeasurementsAccording to anatomic principles [9] the vectors of aging can be described aswhich alter the position and appearance of key anatomic structures of the face as can be shown in figure 1 which compares a Caucasian mother age 66 (left side) with her Caucasian daughters, ages 37 (right above) and 33 (right below) respectively.Figure 1 - Observation of family groupsTherefore, basic anatomic and surgical principles must be applied when planning rejuvenative facial surgery and treating specific problems concomitantwith the aging process.4.Visual Facial ModelThe fact that human face has an especially irregular format and interior components (bones, muscles and fabrics) to possess a complex structure and deformations of different face characteristics of person to person, becomes the modeling of the face a difficult task. The modeling carried through in the present work was based on the model, where the mesh of polygons corresponds to an elastic mesh, simulating the dermis of the face. The deformations in this mesh, necessary to simulate the aging curves, are obtained through the displacement of the vertexes, considering x(t) as a planar curve, which is located within the (u,v ) unit square. So, we can cover the square with a regular grid of points b i,j =[i/m,j/n]T ; i=0,...,m; j=0,...,n. leading to every point (u,v ) asfrom the linear precision property of Bernstein polynomials. Using comparisons with parents we can distort the grid of b i,j into a grid b'i,j , the point (u,v )will be mapped to a point (u',v') asIn order to construct our 3D mesh we introduce the patch byAs the displacements of the vertexes conform to the certain measures gotten through curves of aging and no type of movement in the face is carried through, the parameters of this modeling had been based on the conformation parameter.4.1Textures mappingIn most cases the result gotten in the modeling of the face becomes a little artificial. Using textures mapping can solve this problem. This technique allows an extraordinary increase in the realism of the shaped images and consists of applying on the shaped object, existing textures of the real images of the object.In this case, to do the mapping of an extracted texture of a real image, it is necessary that the textureaccurately correspond to the model 3D of that is made use [9].The detected feature points are used for automatic texture mapping. The main idea of texture mapping is that we get an image by combining two orthogonal pictures in a proper way and then give correct texture coordinates of every point on a head.To give a proper coordinate on a combined image for every point on a head, we first project an individualized 3D head onto three planes, the front (x, y), the left (y, z) and the right (y, z) planes. With the information of feature lines, which are used for image merging, we decide on which plane a 3D-head point on is projected.The projected points on one of three planes arethen transferred to one of feature points spaces suchas the front and the side in 2D. Then they are transferred to the image space and finally to the combined image space.The result of the texture mapping (figure 2) is excellent when it is desired to simulate some alteration of the face that does not involve a type of expression, as neutral. The picture pose must be the same that the 3D scanned data.¦¦¦ mi nj lk n j m i lk k j i w B v B u B b w v u 000,,)()()(')',','(¦¦ m i nj n jmij i v B u B b v u 00,)()(),(¦¦ m i nj n j m i j i v B u B b v u 00,)()(')','(¦¦¦ mi nj lk n j m i lk k j i w B v B u B b w v u 000,,)()()(')',','(Figure 2 - Image shaped with texturemapping5.Age SimulationThis method involves the deformation of a face starting with control segments that define the edges of the faces, as¦¦¦ mi nj lk n j m i lk k j i w B v B u B b w v u 000,,)()()(')',','(Those segments are defined in the original face and their positions are changed to a target face. From those new positions the new position of each vertex in the face is determined.The definition of edges in the face is a fundamental step, since in that phase the applied aging curves are selected. Hence, the face is divided in influencing regions according to their principal edges and characteristics.Considering the face morphology and the modeling of the face aging developed [10], the face was divided in six basic regions (figure 3).The frontal region (1) is limited by the eyelids and the forehead control lines. The distance between these limits enlarges with forward aging.The orbitary region (2) is one of the most important aging parameters because a great number of wrinkles appears and the palpebral pouch increases [11]. In nasal region (3) is observed an enlargement of its contour.The orolabial region (4) is defined by 2 horizontal control segments bounding the upper and lower lips and other 2 segments that define the nasogenian fold. Figure 3 - Regions considering the agingparametersThe lips become thinner and the nasogenian fold deeper and larger. The mental region (5) have 8 control segments that define the low limit of the face and descend with aging. In ear curve (6) is observed an enlargement of its size. The choice of feature lines was based in the characteristic age points in figure 6.The target face is obtained from the aging curves applied to the source face, i.e., with the new control segment position, each vertex of the new image has its position defined by the corresponding vertex in the target face. This final face corresponds to the face in the new age, which was obtained through the application of the numerical modeling of the frontal face aging.The definition of the straight-line segment will control the aging process, leading to a series of tests until the visual result was adequate to the results obtained from the aging curves. The extremes of the segments are interpolated according to the previously defined curves, obtained by piecewise bilinear interpolation [12].Horizontal and vertical orienting auxiliary lines were defined to characterize the extreme points of the control segments (figure 4). Some points, that delimit the control segments, are marked from the intersection of the auxiliary lines with the contour of the face, eyebrow, superior part of the head and the eyes. Others are directly defined without the use of auxiliary lines, such as: eyelid hollow, eyebrow edges, subnasion, mouth, nasolabial wrinkle andnose sides.Figure 4 - Points of the control segmentsOnce the control segments characterize the target image, the following step of the aging process can be undertaken, corresponding to the transformations of the original points to the new positions in the target image. The transformations applied to the segments are given by the aging curves, presented in section 4.In the present work the target segments are calculated by polynomial interpolations, based on parametric curves [12].5.1Deformation approachThe common goal of deformation models is to regulate deformations of a geometric model by providing smoothness constraints. In our age simulation approach, a mesh-independent deformation model is proposed. First, connected piece-wise 3D parametric volumes are generated automatically from a given face mesh according to facial feature points.These volumes cover most regions of a face that can be deformed. Then, by moving the control pointsof each volume, face mesh is deformed. By using non-parallel volumes [13], irregular 3D manifolds are formed. As a result, smaller number of deformvolumes are necessary and the number of freedom incontrol points are reduced. Moreover, based on facialfeature points, this model is mesh independent,which means that it can be easily adopted to deformany face model.After this mesh is constructed, for each vertex on the mesh, it needs to be determined which particularparametric volume it belongs to and what valueparameters are. Then, moving control points ofparametric volumes in 3D will cause smooth facialdeformations, generating facial aging throughflaccidity, automatically through the use of the agingparameters. This deformation is written in matricesas , where V is the nodal displacements offace mesh, B is the mapping matrix composed ofBernstein polynomials, and E is the displacementvector of parametric volume control nodes.BE V Given a quadrilateral mesh of points m i,j ,, we define acontinuous aged surface via a parametricinterpolation of the discretely sampled similaritiespoints. The aged position is defined via abicubic polynomial interpolation of the form with d m,n chosen to satisfy the known normal and continuity conditions at the sample points x i,j .>@>M N j i ,...,1,...,1),(u @@>@>1,,1,),,( j j v i i u v u x ¦3,,),(n m n m n m v u d v u x An interactive tool is programmed to manipulate control points E to achieve aged expressions making possible to simulate aging through age ranges. Basic aged expression units are orbicularis oculi, cheek, eyebrow, eyelid, region of chin, and neck [14]. In general, for each segment, there is an associated transformation, whose behavior can be observed by curves. The only segments that do not suffer any transformation are the contour of the eyes and the superior side of the head.5.2Deformation approachThe developed program also performs shape transformations according to the created aging curves, not including any quantification over the alterations made in texture and skin and hair color. Firstly, in the input model the subjects are required to perform different ages, as previouslymentioned, the first frame needs to be approximately frontal view and with no expression.Secondly, in the facial model initialization, from the first frame, facial features points are extracted manually. The 3D fitting algorithm [15] is then applied to warp the generic model for the person whose face is used. The warping process and from facial feature points and their norms, parametric volumes are automatically generated.Finally, aging field works to relieve the drifting problem in template matching algorithm, templates from the previous frame and templates from the initial frame are applied in order to combine the aging sequence. Our experiments show that this approach is very effective. Despite interest has been put in presenting a friendly user interface, we have to keep in mind that the software system is research oriented. In this kind of applications an important point is the flexibility to add and remove test facilities. 6.Results The presented results in the following figuresrefer to the emulations made on the frontalphotographs, principal focus of this paper, with theobjective to apply the developed program to otherpersons outside the analyzed group. The comparisonswith other photographs of the tested persons dependon their quality and on the position in which theywere taken. An assessment was made of the new positions, of the control segments. It consisted in: after aging a face, from the first age to the second one, through the use of polynomial interpolation of the control segments in the models in the young age, the new positions are then compared with the ones in the model of a relative of older age (figure 5). The processed faces were qualitatively compared with theperson’s photograph at the same age. Figure 5 - Synthetic young age model,region-marked model and aged modelAlso the eyelid hollow, very subtle falling of the eyebrow, thinning of the lips with the enlarging of the nasion and the superior part of the lip, enlargingof the front and changing in the nasolabial wrinkle.7.ConclusionsModelling biological phenomena is a great deal of work, especially when the biggest part of the information about the subject involves only qualitative data. Thus, this research developed had has a challenge in the designing of a model to represent the face aging from qualitative data.Due to its multi-disciplinary character, the developed methodology to model and emulate the face aging involved the study of several other related fields, such as medicine, computing, statistics and mathematics.The possibilities opened by the presented method and some further research on this field can lead to new proposals of enhancing the current techniques of plastic face surgery. It is possible to suggest the ideal age to perform face lifting. Once the most affected aging regions are known and how this process occurs over time. Also missing persons can be recognized based on old photographs using this technique. AcknowledgementsThe project TIN2004-07926 of Spanish Government have subsidized this work.8. References[1] Burt, D. M. et al., Perc. age in adult Caucasianmale faces, in Proc. R. Soc., 259, pp 137-143,1995.[2] Berg, A C. Aging of Orbicularis Muscle inVirtual Human Faces. IEEE 7th InternationalConference on Information Visualization, London, UK, 2003a.[3] Beier , T., S. Neely, Feature-based imagemetamorphosis, In Computer Graphics (Proc.SIGGRAPH), pp. 35-42, 1992.[4] Parke, F. I. P arametrized Models for FacialAnimation, IEEE Computer & Graphics Applications, Nov. 1982.[5] Waters, K.; A Muscle Model for Animating ThreeDimensional Facial Expression. Proc SIGGRAPH'87,Computer Graphics, Vol. 21, Nº4, United States, 1987. [6] Koch, R.M. et alia.. Simulation Facial SurgeryUsing Finite Element Models, Proceedings of SIGGRAPH'96, Computer Graphics, 1996.[7] Kurihara, Tsuneya; Kiyoshi Arai, ATransformation Method for Modeling and Animation of the Human Face from Photographs, Computer Animatio n, Springer-Verlag Tokyo, pp.45-58, 1991.[8] Kent, J., W. Carlson , R. Parent, ShapeTransformation for Polygon Objects, In Computer Graphics (Proc. SIGGRAPH), pp. 47-54, 1992. [9] Sorensen, P., Morphing Magic, in ComputerGraphics World, January 1992.[10]Pitanguy, I., Quintaes, G. de A., Cavalcanti, M.A., Leite, L. A. de S., Anatomia doEnvelhecimento da Face, in Revista Brasileira deCirurgia, Vol 67, 1977.[11]Pitanguy, I., F. R. Leta, D. Pamplona, H. I.Weber, Defining and measuring ageing parameters, in Applied Mathematics and Computation , 1996.[12]Fisher, J.; Lowther, J.; Ching-Kuang S. Curveand Surface Interpolation and Approximation: Knowledge Unit and Software Tool. ITiCSE’04,Leeds, UK June 28–30, 2004.[13]Lerios, A. et al., Feature-Based VolumeMetamorphosis, in SIGGRAPH 95 - Proceedings,pp 449-456, ACM Press, N.Y, 1995.[14]Berg, A C. Facial Aging in a VirtualEnvironment. Memória de Investigación, UIB, Spain, 2003b.[15]Hall, V., Morphing in 2-D and 3-D, in Dr.Dobb's Journal, July 1993.。
主观评价图像色彩管理的关键术语
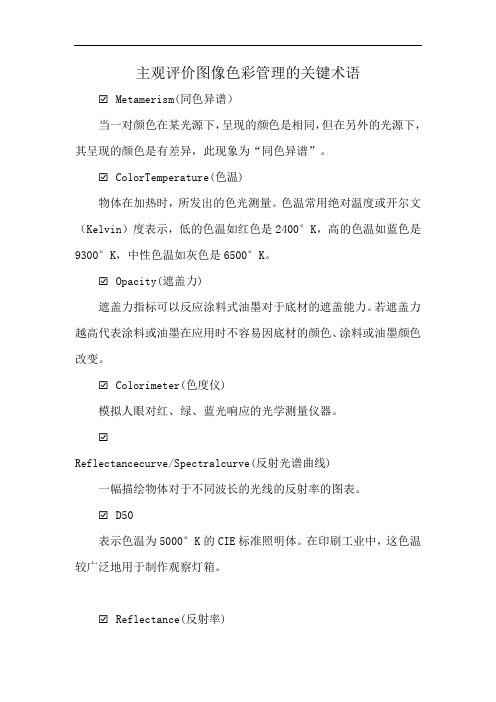
主观评价图像色彩管理的关键术语☑ Metamerism(同色异谱)当一对颜色在某光源下,呈现的颜色是相同,但在另外的光源下,其呈现的颜色是有差异,此现象为“同色异谱”。
☑ ColorTemperature(色温)物体在加热时,所发出的色光测量。
色温常用绝对温度或开尔文(Kelvin)度表示,低的色温如红色是2400°K,高的色温如蓝色是9300°K,中性色温如灰色是6500°K。
☑ Opacity(遮盖力)遮盖力指标可以反应涂料式油墨对于底材的遮盖能力。
若遮盖力越高代表涂料或油墨在应用时不容易因底材的颜色、涂料或油墨颜色改变。
☑ Colorimeter(色度仪)模拟人眼对红、绿、蓝光响应的光学测量仪器。
☑Reflectancecurve/Spectralcurve(反射光谱曲线)一幅描绘物体对于不同波长的光线的反射率的图表。
☑ D50表示色温为5000°K的CIE标准照明体。
在印刷工业中,这色温较广泛地用于制作观察灯箱。
☑ Reflectance(反射率)描写光从物体表面反射的百分率,用分光光度仪可测量出沿可见光谱的不同间隔内物体的反射率,若所可见光谱为横坐标,所反射率为纵坐标就可绘制物体色的光谱曲线。
☑ D65表示色温为6504°K的CIE标准照明体,是一般常用的测试照明体。
☑ Spectrophotometer(分光光度仪)测量光波经过物体反射或透射特性的测量仪器,并将测量结果表示为光谱数据。
☑ Electromagnetic Spectrum(电磁光谱)以不同尺寸在空气中传播的电磁波辐射带,用波长表示,不同波长具有不同性质,很多波段是人眼不能看到的。
只有波长在380—720nm之间的电磁辐射是人眼能看到的可见光波。
在可见光波以外的是不可见,如T射线、X射线、微波和无限电波等。
☑ Specular Excluded(SCE,SPEX,Ex)(排除镜面反射)利用积分球分光光度仪测量物件时,物件的镜面反射不会被测量。
TIMMS 室内地图建模系统说明书

DATASHEETTIMMS is a manually operated push-cartdesigned to accurately model interior spaceswithout accessing GPS. It consists of 3core elements: LiDAR and camera systemsengineered to work indoors in mobile mode,computers and electronics for completing dataacquisition, and data processing workflow forproducing final 2D /3D maps and models. Themodels are “geo-located”, meaning the real worldposition of each area is known.With TIMMS a walk-through of an interior spacedelivers full 360 degree coverage. The spatialdata is captured and georeferenced in real-time.Thousands of square feet are mapped in minutes,entire buildings in a single day.TIMMS is ideal for applications such assituational awareness, emergency response,and creating accurate floor plans. All typesof infrastructure can be mapped, even thoseextending over several city blocks:• Plant and factory facilities• High-rise office, residential, and governmentbuildings• Airports, train stations and othertransportation facilities• Music halls, theatres, auditoriums and otherpublic event spaces• Covered pedestrian concourses (above andbelow ground) with platforms, corridors,stair locations and ramps• Underground mines and tunnelsYOUR BENEFITS• High efficiency, accuracy and speed• Lower data acquisition cost for as-builts• Reduced infringement on operationsT rimble Indoor Mobile Mapping Solution (TIMMS)►No need for GNSS►Little or no LiDAR shadowing►Long-range LiDAR►Self-contained►Simple workflow►Fully customizable►Use survey control for precisegeoreferencingTHE OPTIMAL FUSION OF TECHNOLOGIES FOR CAPTURING SPATIAL DATA OFINDOOR AND GNSS-DENIED SPACESDATASHEETTRIMBLE APPLANIX 85 Leek CrescentRichmond Hill, Ontario L4B 3B3, Canada+1-289-695-6000 Phone +1-905-709-6027 Fax © 2017, T rimble Navigation Limited. All rights reserved. T rimble logo are trademarks of T rimble, registered in the United States and in other countries. All other trademarks are the property of their respective owners. (07/1)PERFORMANCE Onboard powerU p to 4 hours without charge or swap Hot swappable for unlimited operational time Data storage 1TB SSD OperationsNominal data collection speed at 1 meter per second Maximum distance between position fix 100 meters Typical field metricsLiDAR point clouds - 1 cm relative to position accuracy*P roductivity – in excess of 250,000 square feet per day PHYSICAL DIMENSIONSHeight with mast low..........................................................................173 cm Height with mast high........................................................................221 cm Distance to wheel with mast low (front to back)..............................80 cm Distance to wheel with mast high (front to back).............................88 cm Distance between wheels (outside surface of wheels).....................51 cm Weight...................................................................................109 lb or 49.5 kg*rms derived by comparison of TIMMS with static laser scan, results may vary according tobuilding configuration and trajectory chosen.* System performance may vary with scanner type and firmware version. Published valuesbased on X-130.TIMMS COMPONENTS Mobile Unit & MastTIMMS aquisition systemI nertial Measurement Unit (IMU)P OS Computer System (PCS)L iDAR Control Systems (LCS)One LiDARS upported scanners include:T rimble TX-5FARO Focus X-130, X-330, S-70-A, S-150-A, S-350-A One spherical camera (6 camera configuration)Field of View (FOV) >80% of full sphere 2 MegaPixel (MP) per camera Six (6) 3.3 mm focal length 1 meter/second (Up to 4 FPS)One operator and logging computer 16 batteries (8 + 8 spare)2 battery chargers SOFTWARE COMPONENTRealtime monitoring and control GUI Post-processing suiteSYSTEM DELIVERABLEGeoreferenced trajectory in SBET formatGeoreferenced point cloud in ASPRS LAS format Georeferenced spherical imagery in JPEG format Georeferenced raster 2D floorplanUSER SUPPLIED EQUIPMENT PC for post processing Windows 7 64-Bit OSMinimum of 300 GB of disk32 gigabytes of RAM required (64 recommended)USER SUPPLIED SOFTWAREBasic LiDAR processing tools: recommended functionality LAS import compatible Visualization ClippingRaster to Vector tools (manual and/or automated)Trimble Indoor Mobile Mapping Solution (TIMMS)。
高光谱英文缩写

高光谱英文缩写Hyperspectral imaging, often referred to as HSI, is a powerful and versatile technology that has revolutionized the way we perceive and analyze the world around us. This advanced imaging technique goes beyond the capabilities of traditional digital cameras by capturing a vast array of spectral information from the electromagnetic spectrum, providing a wealth of data that can be used in a wide range of applications.At its core, hyperspectral imaging involves the acquisition of high-dimensional data cubes, where each pixel in the image contains a detailed spectral signature. This signature represents the unique reflectance or emission characteristics of the target material, allowing for the identification and classification of a wide variety of substances and materials. Unlike conventional RGB (red, green, blue) imaging, which captures only three color channels, hyperspectral sensors can record hundreds or even thousands of narrow spectral bands, creating a rich and detailed spectral profile.The power of hyperspectral imaging lies in its ability to revealinformation that is invisible to the human eye or traditional imaging techniques. By capturing the subtle nuances of the electromagnetic spectrum, HSI can detect and analyze a diverse range of materials, from minerals and vegetation to man-made objects and even chemical compounds. This capability has made it an indispensable tool in a variety of fields, including remote sensing, environmental monitoring, agriculture, and even medical diagnostics.In the realm of remote sensing, hyperspectral imaging has revolutionized the way we study and manage our natural resources. By analyzing the spectral signatures of different materials, researchers can map and monitor the distribution of minerals, identify areas of vegetation stress, and detect the presence of pollutants or contaminants in the environment. This information is invaluable for a wide range of applications, from mineral exploration and forestry management to environmental impact assessments and disaster response.In the agricultural sector, hyperspectral imaging has become a crucial tool for precision farming and crop monitoring. By analyzing the spectral signatures of plants, farmers can detect early signs of disease, nutrient deficiencies, or water stress, allowing them to take targeted action to improve crop yields and reduce the environmental impact of their operations. Additionally, HSI can be used to map soil composition, monitor crop growth, and even detect the presence ofpests or weeds, enabling more efficient and sustainable farming practices.The medical field has also benefited greatly from the advances in hyperspectral imaging technology. In the area of diagnostics, HSI has shown promise in the early detection of various diseases, such as skin cancer, breast cancer, and cardiovascular conditions. By analyzing the unique spectral signatures of diseased tissues, healthcare professionals can identify subtle changes that may not be visible to the naked eye, enabling earlier intervention and improved patient outcomes.Beyond these applications, hyperspectral imaging has found its way into numerous other industries, including art conservation, forensics, and even aerospace engineering. In the field of art conservation, HSI can be used to identify pigments, detect forgeries, and monitor the condition of valuable artworks, while in forensics, it has been employed to analyze trace evidence and identify illicit substances.As the technology continues to evolve, the potential applications of hyperspectral imaging are virtually limitless. With advancements in sensor technology, data processing, and analytical algorithms, the future of HSI looks increasingly bright, promising new discoveries and innovations that will shape our understanding of the world around us.However, the widespread adoption of hyperspectral imaging technology is not without its challenges. The sheer volume of data generated by HSI systems, coupled with the complexity of the spectral analysis, can pose significant computational and storage challenges. Additionally, the cost of the specialized equipment and the expertise required to interpret the data can be barriers to entry for some organizations and individuals.Despite these challenges, the benefits of hyperspectral imaging are clear, and the technology continues to gain traction across a wide range of industries and disciplines. As researchers and engineers work to overcome the technical hurdles, the future of HSI looks increasingly promising, with the potential to unlock new insights and discoveries that will shape our understanding of the world around us.In conclusion, hyperspectral imaging is a transformative technology that has the power to revolutionize the way we perceive and interact with our environment. By capturing the rich spectral information that lies beyond the visible spectrum, HSI has opened up new frontiers of scientific exploration and practical applications, from remote sensing and precision agriculture to medical diagnostics and forensic analysis. As the technology continues to evolve and become more accessible, the potential of hyperspectral imaging to drive innovation and improve our understanding of the world around us is truly limitless.。
光学纯对映体 英文
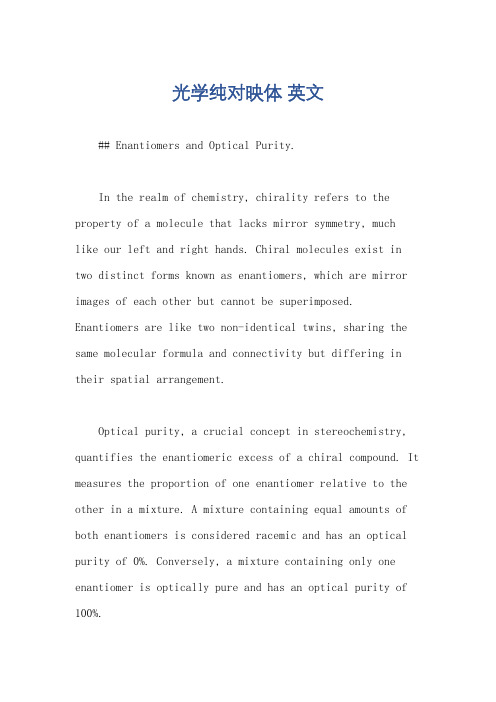
光学纯对映体英文## Enantiomers and Optical Purity.In the realm of chemistry, chirality refers to the property of a molecule that lacks mirror symmetry, muchlike our left and right hands. Chiral molecules exist in two distinct forms known as enantiomers, which are mirror images of each other but cannot be superimposed. Enantiomers are like two non-identical twins, sharing the same molecular formula and connectivity but differing in their spatial arrangement.Optical purity, a crucial concept in stereochemistry, quantifies the enantiomeric excess of a chiral compound. It measures the proportion of one enantiomer relative to the other in a mixture. A mixture containing equal amounts of both enantiomers is considered racemic and has an optical purity of 0%. Conversely, a mixture containing only one enantiomer is optically pure and has an optical purity of 100%.### Separation of Enantiomers.The separation of enantiomers is a challenging yet essential task in many fields, including pharmaceuticals, agrochemicals, and fragrances. Various techniques can be employed to achieve this, including:Chiral chromatography: This technique utilizes achiral stationary phase that interacts differently with different enantiomers, allowing for their separation.Chiral resolution: This involves converting a racemic mixture into a pair of diastereomers, which can then be separated by conventional methods.Enzymatic resolution: Enzymes, being chiral themselves, can selectively catalyze reactions with one enantiomer over the other, leading to the formation of optically pure products.### Optical Purity Measurement.Optical purity can be determined using various methods, such as:Polarimetry: This technique measures the rotation of plane-polarized light as it passes through a chiral sample. The magnitude and direction of rotation depend on the enantiomeric composition of the sample.NMR spectroscopy: Chiral solvents or chiral shift reagents can be used in NMR spectroscopy to differentiate between enantiomers based on their different chemical shifts.Chromatographic methods: Chiral chromatography or capillary electrophoresis can be used to separate enantiomers and determine their relative abundance.### Significance of Optical Purity.Optical purity is of paramount importance in several areas:Pharmacology: Many drugs are chiral, and their enantiomers can have different pharmacological properties, including efficacy, toxicity, and metabolism. Enantiopure drugs offer advantages in terms of safety and effectiveness.Agrochemicals: Herbicides and pesticides can be chiral, and their enantiomers may differ in their selectivity and environmental impact. Optical purity ensures the targeted control of pests and weeds.Fragrances and flavors: The fragrance and flavor of chiral compounds can depend on their enantiomeric composition. Optical purity control allows for the creation of specific scents and tastes.### Applications of Chiral Compounds.Chiral compounds find widespread applications invarious industries:Pharmaceuticals: Enantiopure drugs include ibuprofen,naproxen, and thalidomide.Agrochemicals: Herbicides such as glyphosate and pesticides like cypermethrin are chiral.Fragrances and flavors: Enantiopure compounds like menthol, camphor, and limonene contribute to thedistinctive scents and tastes of products.Materials science: Chiral polymers, liquid crystals, and self-assembling systems have unique properties and applications in optics, electronics, and nanotechnology.### Conclusion.The concept of enantiomers and optical purity is crucial for understanding the stereochemistry of chiral compounds. The ability to separate and determine the optical purity of enantiomers is essential in numerous fields, including pharmaceuticals, agrochemicals, and fragrances. The significance of optical purity lies in itsimplications for the safety, efficacy, and properties of chiral compounds in various applications.。
Discriminatively Trained Sparse Code Gradients for Contour Detection
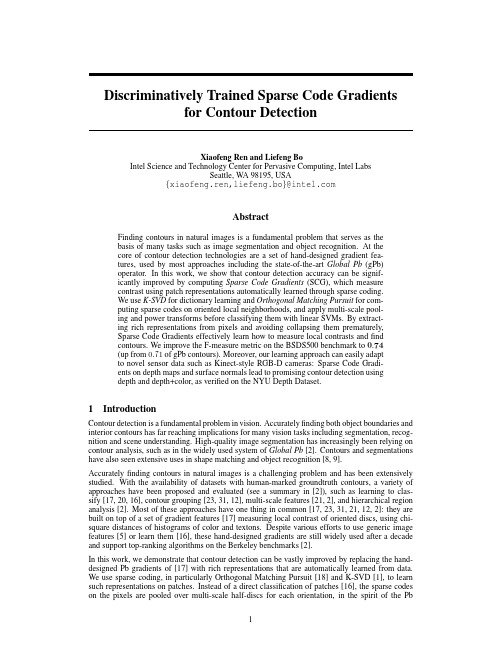
Discriminatively Trained Sparse Code Gradientsfor Contour DetectionXiaofeng Ren and Liefeng BoIntel Science and Technology Center for Pervasive Computing,Intel LabsSeattle,W A98195,USA{xiaofeng.ren,liefeng.bo}@AbstractFinding contours in natural images is a fundamental problem that serves as thebasis of many tasks such as image segmentation and object recognition.At thecore of contour detection technologies are a set of hand-designed gradient fea-tures,used by most approaches including the state-of-the-art Global Pb(gPb)operator.In this work,we show that contour detection accuracy can be signif-icantly improved by computing Sparse Code Gradients(SCG),which measurecontrast using patch representations automatically learned through sparse coding.We use K-SVD for dictionary learning and Orthogonal Matching Pursuit for com-puting sparse codes on oriented local neighborhoods,and apply multi-scale pool-ing and power transforms before classifying them with linear SVMs.By extract-ing rich representations from pixels and avoiding collapsing them prematurely,Sparse Code Gradients effectively learn how to measure local contrasts andfindcontours.We improve the F-measure metric on the BSDS500benchmark to0.74(up from0.71of gPb contours).Moreover,our learning approach can easily adaptto novel sensor data such as Kinect-style RGB-D cameras:Sparse Code Gradi-ents on depth maps and surface normals lead to promising contour detection usingdepth and depth+color,as verified on the NYU Depth Dataset.1IntroductionContour detection is a fundamental problem in vision.Accuratelyfinding both object boundaries and interior contours has far reaching implications for many vision tasks including segmentation,recog-nition and scene understanding.High-quality image segmentation has increasingly been relying on contour analysis,such as in the widely used system of Global Pb[2].Contours and segmentations have also seen extensive uses in shape matching and object recognition[8,9].Accuratelyfinding contours in natural images is a challenging problem and has been extensively studied.With the availability of datasets with human-marked groundtruth contours,a variety of approaches have been proposed and evaluated(see a summary in[2]),such as learning to clas-sify[17,20,16],contour grouping[23,31,12],multi-scale features[21,2],and hierarchical region analysis[2].Most of these approaches have one thing in common[17,23,31,21,12,2]:they are built on top of a set of gradient features[17]measuring local contrast of oriented discs,using chi-square distances of histograms of color and textons.Despite various efforts to use generic image features[5]or learn them[16],these hand-designed gradients are still widely used after a decade and support top-ranking algorithms on the Berkeley benchmarks[2].In this work,we demonstrate that contour detection can be vastly improved by replacing the hand-designed Pb gradients of[17]with rich representations that are automatically learned from data. We use sparse coding,in particularly Orthogonal Matching Pursuit[18]and K-SVD[1],to learn such representations on patches.Instead of a direct classification of patches[16],the sparse codes on the pixels are pooled over multi-scale half-discs for each orientation,in the spirit of the Pbimage patch: gray, abdepth patch (optional):depth, surface normal…local sparse coding multi-scale pooling oriented gradients power transformslinear SVM+ - …per-pixelsparse codes SVMSVMSVM … SVM RGB-(D) contoursFigure 1:We combine sparse coding and oriented gradients for contour analysis on color as well as depth images.Sparse coding automatically learns a rich representation of patches from data.With multi-scale pooling,oriented gradients efficiently capture local contrast and lead to much more accurate contour detection than those using hand-designed features including Global Pb (gPb)[2].gradients,before being classified with a linear SVM.The SVM outputs are then smoothed and non-max suppressed over orientations,as commonly done,to produce the final contours (see Fig.1).Our sparse code gradients (SCG)are much more effective in capturing local contour contrast than existing features.By only changing local features and keeping the smoothing and globalization parts fixed,we improve the F-measure on the BSDS500benchmark to 0.74(up from 0.71of gPb),a sub-stantial step toward human-level accuracy (see the precision-recall curves in Fig.4).Large improve-ments in accuracy are also observed on other datasets including MSRC2and PASCAL2008.More-over,our approach is built on unsupervised feature learning and can directly apply to novel sensor data such as RGB-D images from Kinect-style depth ing the NYU Depth dataset [27],we verify that our SCG approach combines the strengths of color and depth contour detection and outperforms an adaptation of gPb to RGB-D by a large margin.2Related WorkContour detection has a long history in computer vision as a fundamental building block.Modern approaches to contour detection are evaluated on datasets of natural images against human-marked groundtruth.The Pb work of Martin et.al.[17]combined a set of gradient features,using bright-ness,color and textons,to outperform the Canny edge detector on the Berkeley Benchmark (BSDS).Multi-scale versions of Pb were developed and found beneficial [21,2].Building on top of the Pb gradients,many approaches studied the globalization aspects,i.e.moving beyond local classifica-tion and enforcing consistency and continuity of contours.Ren et.al.developed CRF models on superpixels to learn junction types [23].Zhu ed circular embedding to enforce orderings of edgels [31].The gPb work of Arbelaez puted gradients on eigenvectors of the affinity graph and combined them with local cues [2].In addition to Pb gradients,Dollar et.al.[5]learned boosted trees on generic features such as gradients and Haar wavelets,Kokkinos used SIFT features on edgels [12],and Prasad et.al.[20]used raw pixels in class-specific settings.One closely related work was the discriminative sparse models of Mairal et al [16],which used K-SVD to represent multi-scale patches and had moderate success on the BSDS.A major difference of our work is the use of oriented gradients:comparing to directly classifying a patch,measuring contrast between oriented half-discs is a much easier problem and can be effectively learned.Sparse coding represents a signal by reconstructing it using a small set of basis functions.It has seen wide uses in vision,for example for faces [28]and recognition [29].Similar to deep network approaches [11,14],recent works tried to avoid feature engineering and employed sparse coding of image patches to learn features from “scratch”,for texture analysis [15]and object recognition [30,3].In particular,Orthogonal Matching Pursuit [18]is a greedy algorithm that incrementally finds sparse codes,and K-SVD is also efficient and popular for dictionary learning.Closely related to our work but on the different problem of recognition,Bo ed matching pursuit and K-SVD to learn features in a coding hierarchy [3]and are extending their approach to RGB-D data [4].Thanks to the mass production of Kinect,active RGB-D cameras became affordable and were quickly adopted in vision research and applications.The Kinect pose estimation of Shotton et. ed random forests to learn from a huge amount of data[25].Henry ed RGB-D cam-eras to scan large environments into3D models[10].RGB-D data were also studied in the context of object recognition[13]and scene labeling[27,22].In-depth studies of contour and segmentation problems for depth data are much in need given the fast growing interests in RGB-D perception.3Contour Detection using Sparse Code GradientsWe start by examining the processing pipeline of Global Pb(gPb)[2],a highly influential and widely used system for contour detection.The gPb contour detection has two stages:local contrast estimation at multiple scales,and globalization of the local cues using spectral grouping.The core of the approach lies within its use of local cues in oriented gradients.Originally developed in [17],this set of features use relatively simple pixel representations(histograms of brightness,color and textons)and similarity functions(chi-square distance,manually chosen),comparing to recent advances in using rich representations for high-level recognition(e.g.[11,29,30,3]).We set out to show that both the pixel representation and the aggregation of pixel information in local neighborhoods can be much improved and,to a large extent,learned from and adapted to input data. For pixel representation,in Section3.1we show how to use Orthogonal Matching Pursuit[18]and K-SVD[1],efficient sparse coding and dictionary learning algorithms that readily apply to low-level vision,to extract sparse codes at every pixel.This sparse coding approach can be viewed similar in spirit to the use offilterbanks but avoids manual choices and thus directly applies to the RGB-D data from Kinect.We show learned dictionaries for a number of channels that exhibit different characteristics:grayscale/luminance,chromaticity(ab),depth,and surface normal.In Section3.2we show how the pixel-level sparse codes can be integrated through multi-scale pool-ing into a rich representation of oriented local neighborhoods.By computing oriented gradients on this high dimensional representation and using a double power transform to code the features for linear classification,we show a linear SVM can be efficiently and effectively trained for each orientation to classify contour vs non-contour,yielding local contrast estimates that are much more accurate than the hand-designed features in gPb.3.1Local Sparse Representation of RGB-(D)PatchesK-SVD and Orthogonal Matching Pursuit.K-SVD[1]is a popular dictionary learning algorithm that generalizes K-Means and learns dictionaries of codewords from unsupervised data.Given a set of image patches Y=[y1,···,y n],K-SVD jointlyfinds a dictionary D=[d1,···,d m]and an associated sparse code matrix X=[x1,···,x n]by minimizing the reconstruction errorminY−DX 2F s.t.∀i, x i 0≤K;∀j, d j 2=1(1) D,Xwhere · F denotes the Frobenius norm,x i are the columns of X,the zero-norm · 0counts the non-zero entries in the sparse code x i,and K is a predefined sparsity level(number of non-zero en-tries).This optimization can be solved in an alternating manner.Given the dictionary D,optimizing the sparse code matrix X can be decoupled to sub-problems,each solved with Orthogonal Matching Pursuit(OMP)[18],a greedy algorithm forfinding sparse codes.Given the codes X,the dictionary D and its associated sparse coefficients are updated sequentially by singular value decomposition. For our purpose of representing local patches,the dictionary D has a small size(we use75for5x5 patches)and does not require a lot of sample patches,and it can be learned in a matter of minutes. Once the dictionary D is learned,we again use the Orthogonal Matching Pursuit(OMP)algorithm to compute sparse codes at every pixel.This can be efficiently done with convolution and a batch version of the OMP algorithm[24].For a typical BSDS image of resolution321x481,the sparse code extraction is efficient and takes1∼2seconds.Sparse Representation of RGB-D Data.One advantage of unsupervised dictionary learning is that it readily applies to novel sensor data,such as the color and depth frames from a Kinect-style RGB-D camera.We learn K-SVD dictionaries up to four channels of color and depth:grayscale for luminance,chromaticity ab for color in the Lab space,depth(distance to camera)and surface normal(3-dim).The learned dictionaries are visualized in Fig.2.These dictionaries are interesting(a)Grayscale (b)Chromaticity (ab)(c)Depth (d)Surface normal Figure 2:K-SVD dictionaries learned for four different channels:grayscale and chromaticity (in ab )for an RGB image (a,b),and depth and surface normal for a depth image (c,d).We use a fixed dictionary size of 75on 5x 5patches.The ab channel is visualized using a constant luminance of 50.The 3-dimensional surface normal (xyz)is visualized in RGB (i.e.blue for frontal-parallel surfaces).to look at and qualitatively distinctive:for example,the surface normal codewords tend to be more smooth due to flat surfaces,the depth codewords are also more smooth but with speckles,and the chromaticity codewords respect the opponent color pairs.The channels are coded separately.3.2Coding Multi-Scale Neighborhoods for Measuring ContrastMulti-Scale Pooling over Oriented Half-Discs.Over decades of research on contour detection and related topics,a number of fundamental observations have been made,repeatedly:(1)contrast is the key to differentiate contour vs non-contour;(2)orientation is important for respecting contour continuity;and (3)multi-scale is useful.We do not wish to throw out these principles.Instead,we seek to adopt these principles for our case of high dimensional representations with sparse codes.Each pixel is presented with sparse codes extracted from a small patch (5-by-5)around it.To aggre-gate pixel information,we use oriented half-discs as used in gPb (see an illustration in Fig.1).Each orientation is processed separately.For each orientation,at each pixel p and scale s ,we define two half-discs (rectangles)N a and N b of size s -by-(2s +1),on both sides of p ,rotated to that orienta-tion.For each half-disc N ,we use average pooling on non-zero entries (i.e.a hybrid of average and max pooling)to generate its representationF (N )= i ∈N |x i 1| i ∈N I |x i 1|>0,···, i ∈N |x im | i ∈NI |x im |>0 (2)where x ij is the j -th entry of the sparse code x i ,and I is the indicator function whether x ij is non-zero.We rotate the image (after sparse coding)and use integral images for fast computations (on both |x ij |and |x ij |>0,whose costs are independent of the size of N .For two oriented half-dics N a and N b at a scale s ,we compute a difference (gradient)vector DD (N a s ,N b s )= F (N a s )−F (N b s ) (3)where |·|is an element-wise absolute value operation.We divide D (N a s ,N b s )by their norms F (N a s ) + F (N b s ) + ,where is a positive number.Since the magnitude of sparse codes variesover a wide range due to local variations in illumination as well as occlusion,this step makes the appearance features robust to such variations and increases their discriminative power,as commonly done in both contour detection and object recognition.This value is not hard to set,and we find a value of =0.5is better than,for instance, =0.At this stage,one could train a classifier on D for each scale to convert it to a scalar value of contrast,which would resemble the chi-square distance function in gPb.Instead,we find that it is much better to avoid doing so separately at each scale,but combining multi-scale features in a joint representation,so as to allow interactions both between codewords and between scales.That is,our final representation of the contrast at a pixel p is the concatenation of sparse codes pooled at all thescales s ∈{1,···,S }(we use S =4):D p = D (N a 1,N b 1),···,D (N a S ,N b S );F (N a 1∪N b 1),···,F (N a S ∪N b S ) (4)In addition to difference D ,we also include a union term F (N a s ∪N b s ),which captures the appear-ance of the whole disc (union of the two half discs)and is normalized by F (N a s ) + F (N b s ) + .Double Power Transform and Linear Classifiers.The concatenated feature D p (non-negative)provides multi-scale contrast information for classifying whether p is a contour location for a partic-ular orientation.As D p is high dimensional (1200and above in our experiments)and we need to do it at every pixel and every orientation,we prefer using linear SVMs for both efficient testing as well as training.Directly learning a linear function on D p ,however,does not work very well.Instead,we apply a double power transformation to make the features more suitable for linear SVMs D p = D α1p ,D α2p (5)where 0<α1<α2<1.Empirically,we find that the double power transform works much better than either no transform or a single power transform α,as sometimes done in other classification contexts.Perronnin et.al.[19]provided an intuition why a power transform helps classification,which “re-normalizes”the distribution of the features into a more Gaussian form.One plausible intuition for a double power transform is that the optimal exponent αmay be different across feature dimensions.By putting two power transforms of D p together,we allow the classifier to pick its linear combination,different for each dimension,during the stage of supervised training.From Local Contrast to Global Contours.We intentionally only change the local contrast es-timation in gPb and keep the other steps fixed.These steps include:(1)the Savitzky-Goley filter to smooth responses and find peak locations;(2)non-max suppression over orientations;and (3)optionally,we apply the globalization step in gPb that computes a spectral gradient from the local gradients and then linearly combines the spectral gradient with the local ones.A sigmoid transform step is needed to convert the SVM outputs on D p before computing spectral gradients.4ExperimentsWe use the evaluation framework of,and extensively compare to,the publicly available Global Pb (gPb)system [2],widely used as the state of the art for contour detection 1.All the results reported on gPb are from running the gPb contour detection and evaluation codes (with default parameters),and accuracies are verified against the published results in [2].The gPb evaluation includes a number of criteria,including precision-recall (P/R)curves from contour matching (Fig.4),F-measures computed from P/R (Table 1,2,3)with a fixed contour threshold (ODS)or per-image thresholds (OIS),as well as average precisions (AP)from the P/R curves.Benchmark Datasets.The main dataset we use is the BSDS500benchmark [2],an extension of the original BSDS300benchmark and commonly used for contour evaluation.It includes 500natural images of roughly resolution 321x 481,including 200for training,100for validation,and 200for testing.We conduct both color and grayscale experiments (where we convert the BSDS500images to grayscale and retain the groundtruth).In addition,we also use the MSRC2and PASCAL2008segmentation datasets [26,6],as done in the gPb work [2].The MSRC2dataset has 591images of resolution 200x 300;we randomly choose half for training and half for testing.The PASCAL2008dataset includes 1023images in its training and validation sets,roughly of resolution 350x 500.We randomly choose half for training and half for testing.For RGB-D contour detection,we use the NYU Depth dataset (v2)[27],which includes 1449pairs of color and depth frames of resolution 480x 640,with groundtruth semantic regions.We choose 60%images for training and 40%for testing,as in its scene labeling setup.The Kinect images are of lower quality than BSDS,and we resize the frames to 240x 320in our experiments.Training Sparse Code Gradients.Given sparse codes from K-SVD and Orthogonal Matching Pur-suit,we train the Sparse Code Gradients classifiers,one linear SVM per orientation,from sampled locations.For positive data,we sample groundtruth contour locations and estimate the orientations at these locations using groundtruth.For negative data,locations and orientations are random.We subtract the mean from the patches in each data channel.For BSDS500,we typically have 1.5to 21In this work we focus on contour detection and do not address how to derive segmentations from contours.pooling disc size (pixel)a v e r a g e p r e c i s i o na v e r a g e p r e c i s i o nsparsity level a v e r a g e p r e c i s i o n (a)(b)(c)Figure 3:Analysis of our sparse code gradients,using average precision of classification on sampled boundaries.(a)The effect of single-scale vs multi-scale pooling (accumulated from the smallest).(b)Accuracy increasing with dictionary size,for four orientation channels.(c)The effect of the sparsity level K,which exhibits different behavior for grayscale and chromaticity.BSDS500ODS OIS AP l o c a l gPb (gray).67.69.68SCG (gray).69.71.71gPb (color).70.72.71SCG (color).72.74.75g l o b a l gPb (gray).69.71.67SCG (gray).71.73.74gPb (color).71.74.72SCG (color).74.76.77Table 1:F-measure evaluation on the BSDS500benchmark [2],comparing to gPb on grayscaleand color images,both for local contour detec-tion as well as for global detection (-bined with the spectral gradient analysis in [2]).Recall P r e c i s i o n Figure 4:Precision-recall curves of SCG vs gPb on BSDS500,for grayscale and color images.We make a substantial step beyondthe current state of the art toward reachinghuman-level accuracy (green dot).million data points.We use 4spatial scales,at half-disc sizes 2,4,7,25.For a dictionary size of 75and 4scales,the feature length for one data channel is 1200.For full RGB-D data,the dimension is 4800.For BSDS500,we train only using the 200training images.We modify liblinear [7]to take dense matrices (features are dense after pooling)and single-precision floats.Looking under the Hood.We empirically analyze a number of settings in our Sparse Code Gradi-ents.In particular,we want to understand how the choices in the local sparse coding affect contour classification.Fig.3shows the effects of multi-scale pooling,dictionary size,and sparsity level (K).The numbers reported are intermediate results,namely the mean of average precision of four oriented gradient classifier (0,45,90,135degrees)on sampled locations (grayscale unless otherwise noted,on validation).As a reference,the average precision of gPb on this task is 0.878.For multi-scale pooling,the single best scale for the half-disc filter is about 4x 8,consistent with the settings in gPb.For accumulated scales (using all the scales from the smallest up to the current level),the accuracy continues to increase and does not seem to be saturated,suggesting the use of larger scales.The dictionary size has a minor impact,and there is a small (yet observable)benefit to use dictionaries larger than 75,particularly for diagonal orientations (45-and 135-deg).The sparsity level K is a more intriguing issue.In Fig.3(c),we see that for grayscale only,K =1(normalized nearest neighbor)does quite well;on the other hand,color needs a larger K ,possibly because ab is a nonlinear space.When combining grayscale and color,it seems that we want K to be at least 3.It also varies with orientation:horizontal and vertical edges require a smaller K than diagonal edges.(If using K =1,our final F-measure on BSDS500is 0.730.)We also empirically evaluate the double power transform vs single power transform vs no transform.With no transform,the average precision is 0.865.With a single power transform,the best choice of the exponent is around 0.4,with average precision 0.884.A double power transform (with exponentsMSRC2ODS OIS APgPb.37.39.22SCG.43.43.33PASCAL2008ODS OIS APgPb.34.38.20SCG.37.41.27Table2:F-measure evaluation comparing our SCG approach to gPb on two addi-tional image datasets with contour groundtruth: MSRC2[26]and PASCAL2008[6].RGB-D(NYU v2)ODS OIS AP gPb(color).51.52.37 SCG(color).55.57.46gPb(depth).44.46.28SCG(depth).53.54.45gPb(RGB-D).53.54.40SCG(RGB-D).62.63.54Table3:F-measure evaluation on RGB-D con-tour detection using the NYU dataset(v2)[27].We compare to gPb on using color image only,depth only,as well as color+depth.Figure5:Examples from the BSDS500dataset[2].(Top)Image;(Middle)gPb output;(Bottom) SCG output(this work).Our SCG operator learns to preservefine details(e.g.windmills,faces,fish fins)while at the same time achieving higher precision on large-scale contours(e.g.back of zebras). (Contours are shown in double width for the sake of visualization.)0.25and0.75,which can be computed through sqrt)improves the average precision to0.900,which translates to a large improvement in contour detection accuracy.Image Benchmarking Results.In Table1and Fig.4we show the precision-recall of our Sparse Code Gradients vs gPb on the BSDS500benchmark.We conduct four sets of experiments,using color or grayscale images,with or without the globalization component(for which we use exactly the same setup as in gPb).Using Sparse Code Gradients leads to a significant improvement in accuracy in all four cases.The local version of our SCG operator,i.e.only using local contrast,is already better(F=0.72)than gPb with globalization(F=0.71).The full version,local SCG plus spectral gradient(computed from local SCG),reaches an F-measure of0.739,a large step forward from gPb,as seen in the precision-recall curves in Fig.4.On BSDS300,our F-measure is0.715. We observe that SCG seems to pick upfine-scale details much better than gPb,hence the much higher recall rate,while maintaining higher precision over the entire range.This can be seen in the examples shown in Fig.5.While our scale range is similar to that of gPb,the multi-scale pooling scheme allows theflexibility of learning the balance of scales separately for each code word,which may help detecting the details.The supplemental material contains more comparison examples.In Table2we show the benchmarking results for two additional datasets,MSRC2and PAS-CAL2008.Again we observe large improvements in accuracy,in spite of the somewhat different natures of the scenes in these datasets.The improvement on MSRC2is much larger,partly because the images are smaller,hence the contours are smaller in scale and may be over-smoothed in gPb. As for computational cost,using integral images,local SCG takes∼100seconds to compute on a single-thread Intel Core i5-2500CPU on a BSDS image.It is slower than but comparable to the highly optimized multi-thread C++implementation of gPb(∼60seconds).Figure6:Examples of RGB-D contour detection on the NYU dataset(v2)[27].Thefive panels are:input image,input depth,image-only contours,depth-only contours,and color+depth contours. Color is good picking up details such as photos on the wall,and depth is useful where color is uniform(e.g.corner of a room,row1)or illumination is poor(e.g.chair,row2).RGB-D Contour Detection.We use the second version of the NYU Depth Dataset[27],which has higher quality groundtruth than thefirst version.A medianfiltering is applied to remove double contours(boundaries from two adjacent regions)within3pixels.For RGB-D baseline,we use a simple adaptation of gPb:the depth values are in meters and used directly as a grayscale image in gPb gradient computation.We use a linear combination to put(soft)color and depth gradients together in gPb before non-max suppression,with the weight set from validation.Table3lists the precision-recall evaluations of SCG vs gPb for RGB-D contour detection.All the SCG settings(such as scales and dictionary sizes)are kept the same as for BSDS.SCG again outperforms gPb in all the cases.In particular,we are much better for depth-only contours,for which gPb is not designed.Our approach learns the low-level representations of depth data fully automatically and does not require any manual tweaking.We also achieve a much larger boost by combining color and depth,demonstrating that color and depth channels contain complementary information and are both critical for RGB-D contour detection.Qualitatively,it is easy to see that RGB-D combines the strengths of color and depth and is a promising direction for contour and segmentation tasks and indoor scene analysis in general[22].Fig.6shows a few examples of RGB-D contours from our SCG operator.There are plenty of such cases where color alone or depth alone would fail to extract contours for meaningful parts of the scenes,and color+depth would succeed. 5DiscussionsIn this work we successfully showed how to learn and code local representations to extract contours in natural images.Our approach combined the proven concept of oriented gradients with powerful representations that are automatically learned through sparse coding.Sparse Code Gradients(SCG) performed significantly better than hand-designed features that were in use for a decade,and pushed contour detection much closer to human-level accuracy as illustrated on the BSDS500benchmark. Comparing to hand-designed features(e.g.Global Pb[2]),we maintain the high dimensional rep-resentation from pooling oriented neighborhoods and do not collapse them prematurely(such as computing chi-square distance at each scale).This passes a richer set of information into learn-ing contour classification,where a double power transform effectively codes the features for linear paring to previous learning approaches(e.g.discriminative dictionaries in[16]),our uses of multi-scale pooling and oriented gradients lead to much higher classification accuracies. Our work opens up future possibilities for learning contour detection and segmentation.As we il-lustrated,there is a lot of information locally that is waiting to be extracted,and a learning approach such as sparse coding provides a principled way to do so,where rich representations can be automat-ically constructed and adapted.This is particularly important for novel sensor data such as RGB-D, for which we have less understanding but increasingly more need.。
HDR图像色调映射的自适应色彩调节算法

0引言高动态范围(High Dynamic Range,HDR)图像是一种可以记录实际场景亮度范围变化较大的图像,拥有更丰富的亮度层次,尤其是亮区域和暗区域的细节展现,远比普通图像更逼近现实的色彩效果。
但HDR图像通过普通显示设备再现时存在动态范围不匹配问题,因此动态范围的压缩算法成为了研究的热点。
近年来,已经涌现出很多HDR图像色调映射(tone mapping)算法[1-4],例如KUANG J[3]等在图像色貌模型的基础上提出了iCAM06算法;REINHARD E[4]等提出基于摄影法的动态范围压缩算法。
这些色调映射算法提供了将真实世界的亮度范围映射到输出媒介亮度范围的复杂方法,但它们通常会导致图像颜色外观的变化。
最常见的色调操作是亮度压缩,会导致较暗的色调变得更亮并且扭曲对比关系[5]。
这是由于调映射算法起初都是针对图像在亮度域进行压缩处理,但在处理彩色的高动态范围图像时,仅仅考虑亮度分量,忽略了在对亮度压缩的同时图像的色彩分量也被压缩了,颜色发生了变化。
本文提出图像经色调映射压缩处理后,在色域增加色彩调节算法,以解决因压缩后存在的褪色、偏色等色彩失真问题,从而提高图像的色彩表现。
1色彩调节算法描述整个算法分为两个部分:亮度域处理和色域处理。
亮度域处理是对采集得到的高动态图像在亮度域进行动态范围的压缩映射和限制对比度的自适应直方图均衡化处理,将图像的高动态范围映射到低动态范围内。
色域处理包含两方面,一方面是色彩恢复处理,结合下文中所给曲线的特点,根据图像处理前后亮度比值自适应地调节色彩饱和度参数,对压缩后的图像色彩恢复;另一方面对恢复后的图像做色彩增强处理,解决图像拍HDR图像色调映射的自适应色彩调节算法陈文艺1,张龙2,杨辉1(1.西安邮电大学物联网与两化融合研究院,陕西西安710021;2.西安邮电大学通信与信息工程学院,陕西西安710021)摘要:为了克服传统色调映射算法处理高动态图像过程中因忽略彩色分量而导致图像色彩失真的现象,给出一种自适应的色彩调节算法。
unparalleled color performance

unparalleled color performance Unparalleled Color Performance: Exploring the Art and Science of ColorColor is an essential aspect of our visual world. It impacts how we perceive our surroundings, engages our emotions, and communicates meaning. In a world where digital media continues to transform our content consumption patterns, color performance has never been more critical. With the rise ofhigh-definition displays, virtual and augmented reality, and advanced imaging and printing technologies, the ability to deliver accurate, consistent and captivating color performance is paramount.The term "unparalleled color performance" refers to the ability of a device or system to deliver superior color accuracy, precision, and consistency at all times. Achieving unparalleled color performance across various devices and platforms requires a multi-layered approach that combines the art and science of color. It involves careful calibration, color management, processing,and rendering to ensure that colors are reproduced precisely as intended.Understanding the Science of Color PerformanceThe science of color performance is complex and multidisciplinary. It involves the physical properties of light and color vision, human perception, digital imaging and processing, and color reproduction technologies. Accurate color reproduction requires precise measurement, control, and correction of color deviations at every stageof the imaging chain.One of the key challenges of color reproduction is that our visual system is highly adaptable and can compensate for significant color deviations. Therefore, color measurements need to be standardized, calibrated, and verified against industry standards and reference materials to ensure consistent and accurate results. Some of the tools and techniques used for color measurement and calibration include colorimeters, spectrophotometers, color targets, and software color management systems.The Art of Color PerformanceThe art of color performance is equally crucial in delivering unparalleled color accuracy and consistency. It involves understanding the role of color in visual storytelling, design, and branding, and how to leverage color to enhance emotional engagement and convey meaning.Color is essential in creating a brand identity and differentiating products from competitors. It can signify luxury, quality, innovation, or humor, depending on the context and target audience. Effective use of color requires a keen understanding of color theory, cultural associations, and audience preferences. Designers and marketers need to tailor color choices and combinations to reflect the message and values they want to convey.The Role of Unparalleled Color Performance in IndustriesUnparalleled color performance is essential in several industries, including printing, imaging, photography, cinematography, and gaming. Inprinting, accurate color reproduction is essential for producing high-quality images, logos, packaging designs, and marketing materials. Printers need to ensure that the colors match the intended hue, saturation, and brightness levels.In imaging and photography, accurate color reproduction is essential for capturing and preserving the natural colors of the subject. Cameras and displays need to have precise color calibration, white balance, and gamma adjustments to achieve accurate results. Accurate color reproduction is also essential in cinematography, where color grading and timing are critical in creating the desired mood and atmosphere.In gaming, unparalleled color performance is essential in delivering an immersive and engaging experience. The colors need to be vibrant, consistent across different platforms, and accurately reflect the intended mood and atmosphere of the game. Color performance is particularly crucial in virtual and augmented reality applications, where the color accuracy andconsistency can significantly impact the user experience.ConclusionIn conclusion, achieving unparalleled color performance is a multi-faceted endeavor that requires a combination of art and science. Accurate color reproduction is essential for conveying meaning, enhancing emotional engagement, creating a brand identity, and delivering an immersive experience. Achieving unparalleled color performance requires precision, attention to detail, and a thorough understanding of color theory, human perception, and color reproduction technologies. By mastering the art and science of color performance, companies and individuals can unlock new avenues of creativity, enhance their brand value, and improve the user experience.。
Adaptive logarithmic mapping for displaying high contrast scenes
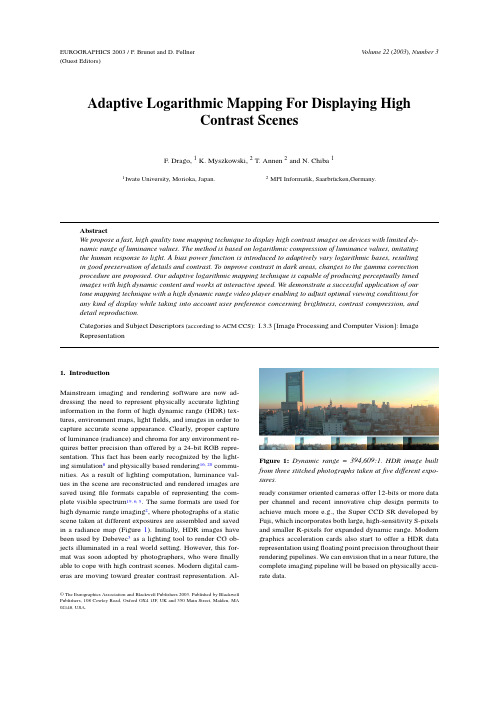
EUROGRAPHICS2003/P.Brunet and D.Fellner(Guest Editors)Volume22(2003),Number3Adaptive Logarithmic Mapping For Displaying HighContrast ScenesF.Drago,1K.Myszkowski,2T.Annen2and N.Chiba11Iwate University,Morioka,Japan.2MPI Informatik,Saarbrücken,Germany.AbstractWe propose a fast,high quality tone mapping technique to display high contrast images on devices with limited dy-namic range of luminance values.The method is based on logarithmic compression of luminance values,imitatingthe human response to light.A bias power function is introduced to adaptively vary logarithmic bases,resultingin good preservation of details and contrast.To improve contrast in dark areas,changes to the gamma correctionprocedure are proposed.Our adaptive logarithmic mapping technique is capable of producing perceptually tunedimages with high dynamic content and works at interactive speed.We demonstrate a successful application of ourtone mapping technique with a high dynamic range video player enabling to adjust optimal viewing conditions forany kind of display while taking into account user preference concerning brightness,contrast compression,anddetail reproduction.Categories and Subject Descriptors(according to ACM CCS):I.3.3[Image Processing and Computer Vision]:ImageRepresentation1.IntroductionMainstream imaging and rendering software are now ad-dressing the need to represent physically accurate lighting information in the form of high dynamic range(HDR)tex-tures,environment maps,lightfields,and images in order to capture accurate scene appearance.Clearly,proper capture of luminance(radiance)and chroma for any environment re-quires better precision than offered by a24-bit RGB repre-sentation.This fact has been early recognized by the light-ing simulation8and physically based rendering16,20commu-nities.As a result of lighting computation,luminance val-ues in the scene are reconstructed and rendered images are saved usingfile formats capable of representing the com-plete visible spectrum19,6,5.The same formats are used for high dynamic range imaging2,where photographs of a static scene taken at different exposures are assembled and saved in a radiance map(Figure1).Initially,HDR images have been used by Debevec3as a lighting tool to render CG ob-jects illuminated in a real world setting.However,this for-mat was soon adopted by photographers,who werefinally able to cope with high contrast scenes.Modern digital cam-eras are moving toward greater contrast representation.Al-Figure1:Dynamic range=394,609:1.HDR image built from three stitched photographs taken atfive different expo-sures.ready consumer oriented cameras offer12-bits or more data per channel and recent innovative chip design permits to achieve much more e.g.,the Super CCD SR developed by Fuji,which incorporates both large,high-sensitivity S-pixels and smaller R-pixels for expanded dynamic range.Modern graphics acceleration cards also start to offer a HDR data representation usingfloating point precision throughout their rendering pipelines.We can envision that in a near future,the complete imaging pipeline will be based on physically accu-rate data.©The Eurographics Association and Blackwell Publishers2003.Published by Blackwell Publishers,108Cowley Road,Oxford OX41JF,UK and350Main Street,Malden,MA 02148,USA.Unfortunately,displaying methods have not progressed in a similar pace.Except for a few specialized devices,CRT andflat panel displays are still limited to a very small dy-namic range,often less than100:1,while the dynamic range of scenes represented by HDR images can span overfive or more orders of magnitude.Tone mapping is introduced in the graphic pipeline as the last step before image display to address the problem of incompatible luminance ranges.The question answered by most of the tone mapping algorithms developed for com-puter graphics applications is:“Within the physical limita-tions of displaying hardware,how to present images percep-tually similar to the original scenes to human viewers?”Es-sentially,tone mapping should provide drastic contrast re-duction from scene values to displayable ranges while pre-serving the image details essential to appreciate the scene content.The tone mapping problem wasfirst addressed by Tum-blin and Rushmeier16and Ward20.They developed global mapping functions backed by results in psychophysics on brightness and contrast ter,Ward7proposed the Histogram Adjustment technique which allocates dy-namic range space in proportion to the percentage of pix-els with similar brightness,again taking contrast perception into account.Some researchers focused simply on computa-tion efficiency,mostly ignoring characteristics of the human visual system(HVS)14.Each of these methods can be classi-fied as spatially uniform because a single mapping function is derived and used for all pixels in a given image.The tone mapping proposed in this paper belongs to this category. Another group,the spatially varying methods,often attempt to model spatial adaptation by using locally changing map-ping functions,which depend on a given pixel neighbor-hood.While spatially varying methods might produce the most compelling images,they are significantly more expen-sive than spatially uniform techniques and their use in inter-active applications has not been shown so far.An interested reader can refer to a recent extensive survey on this topic4. Our motivation for this work is to address the need for a fast algorithm suitable for interactive applications which au-tomatically produces realistically looking images for a wide variation of scenes exhibiting high dynamic range of lumi-nance.For the sake of efficiency we use a spatially uniform tone mapping function which is based on a simple model of brightness perception.We provide the user with the possibil-ity of on-the-fly image appearance tuning in terms of bright-ness and contrast during an interactive application.The re-sulting images are detailed,and faithful representations of the original high contrast scenes reproduced within the ca-pabilities of the displaying medium.Material accompany-ing this paper can be found on the web at:http://www-cg.cis.iwate-u.ac.jp/frederic/logmap. The paper is organized as follows:Section2briefly describes the research results our technique is based upon.In Section 3we present the tone mapping function,its parameters and usage.Section4proposes a solution to the loss of detail in dark areas caused by gamma correction.In Section5we dis-cuss some essential optimizations leading to the implemen-tation of a HDR movie player which enables the presentation of high dynamic range content in realtime.Finally,we con-clude this paper and propose possible directions for future research.2.BackgroundThe term brightness B describes the response of the HVS to stimulus luminance L.This response has the form of compressive non-linearity which can be approximated by a logarithmic function(Weber-Fechner law)B=k1ln(L/L0), where L0denotes the luminance of the background and k1 is a constant factor.The relation has been derived in psy-chophysical threshold experiments through examining just noticeable differences∆L for various L0.Slightly different relations between B and L have been obtained depending on such factors as stimulus size,L0,and temporal presen-tation.For example,supra-threshold experiments resulted in an observation that equal ratios of luminance lead to equal ratios of brightness and the HVS response should be rather modeled by a power function(Stevens law)B=k2L n,where n falls in the range of0.3to1.0.In practice,both descrip-tions are relatively close so that it is difficult to discrimi-nate between them experimentally18.Therefore,we assumed the logarithmic relation in our tone mapping solution follow-ing Stockham15who recommended such a relation for image processing purposes:L d=log(L w+1)max(1) where for each pixel,the displayed luminance L d is derived from the ratio of world luminance L w and maximum lumi-nance in the scene L max.This mapping ensures that what-ever the dynamic range of the scene is,the maximum value is remapped to one(white)and other luminance values are smoothly incremented.While this formula leads to pleasant images,we found that the luminance compression is exces-sive and the feeling of high contrast content is lost.3.Adaptive Logarithmic MappingThe design of our tone mapping technique was guided by a few rules.It must provide consistent results despite the vast diversity of natural scenes and the possible radiance value inaccuracy found in HDR photographs.Additionally, it should be adaptable and extensible to address the current capabilities of displaying methods and their future evolution. Tone mapping must capture the physical appearance of the scene,while avoiding the introduction of artifacts such as contrast reversal or black halos.The overall brightness of the output image must be faithful to the context.It must be “user-friendly”i.e.,automatic in most cases,with a few in-tuitive parameters which provide possibility for adjustments.©The Eurographics Association and Blackwell Publishers2003.It must be fast for interactive and realtime applications while avoiding any trade-off between speed versus quality.3.1.Scaling Scene Luminance to Image BrightnessThe overall brightness of the output image is decided mainly by the lighting characteristics of the scene.It is then neces-sary tofind an initial scalefactor from the scene luminance to output image brightness.We can make here an analogy with the photography where the exposure settings determine the appearance of the taken picture.Modern cameras offer dif-ferent options for automatic exposure setting,such as center-weighted,center-spot,or matrix-metering.In the same fash-ion,we rely on two methods suitable for different use.For static images or when a user does not directly interact with the scene,we compute the logarithmic average of the scene based on luminance values for all pixels,similar to Tumblin and Rushmeier16(who called this scalefactor world adapta-tion luminance)or Reinhard et al.12.We also use a center-weighted scalefactor for interactive tone mapping or walk-through sequences when a user’s center of attention might shift from one location to another in the scene13.Our im-plementation of a center-weighted scalefactor calculates the logarithmic average of the region centered at a pixel(the cen-ter of viewingfixation)and convolved by a two-dimensional Gaussian distribution kernel.The area of the sampled region and Gaussian kernel default to15%of the scene area but can be adjusted interactively.This method might be used in conjunction with an eye tracking system.We also offer an exposure scale factor allowing users to adjust the brightness of the output image to their displaying conditions.3.2.Contrast AdjustmentThe principal characteristic of our tone mapping function is an adaptive adjustment of logarithmic base depending on each pixel’s radiance.We interpolate luminance values found in the scene from log2(L w)to log10(L w).This essen-tially provides for good contrast and detail preservation in dark and medium areas while permitting maximum com-pression of high luminance values.In principle,a narrower or wider interval of logarithmic bases could be used,but we could notfind any practical reason for it.The values of log x(L w)for x<2increase sharply making exposure adjust-ments difficult.On the other hand for x>10luminance com-pression is only marginally augmented and the overall image loses too much contrast.We also observed some color shift caused by high logarithmic bases.Figure2shows the difference between images which are tone mapped with log2()and log10()functions after apply-ing an initial world adaptation scalefactor.The following ba-sic property of logarithm permits an arbitrary choice of log-arithmic base:log base(x)=log(x)log(base)(2)2468100 100 200 300 400 5000.20.40.60.810 0.2 0.4 0.6 0.8 1log2(x+1)log(x+1)log10(x+1)Figure2:The Stanford MEMORIAL church mapped withfixed base two logarithm(left)and with decimal logarithm(right).The contrast and brightness difference is evident butnone of these images provides a satisfying rendition.Ourtone mapping function offers the possibility to combine thecharacteristics of both images in a single result.Plots of thelogarithm function show the difference among common log-arithmic bases.log2()increases sharply providing high con-trast while log10()drastically compresses higher values.For smooth interpolation among logarithmic bases,we relyupon Perlin and Hoffert“bias”power function9.Bias wasfirst presented as a density modulation function,to changedensity of the soft boundary between the inside and outsideof a procedural hypertexture.It became a standard tool oftexture synthesis and is also used for many different tasksthroughout computer graphics.The bias function is a powerfunction defined over the unit interval,an intuitive parameterb remaps an input value to a higher or lower value.bias b(t)=t log(b)log(0.5)(3)Figure3shows the bias curve for different b values.It is in-teresting to notice that bias0.73produces approximately thesame mapping as the gamma correction function with a pa-rameterγ=2.2.3.3.AlgorithmThe input data is converted from its original format to afloating point representation of linear RGB values.Since the©The Eurographics Association and Blackwell Publishers2003.Figure 4:The Stanford MEMORIAL Church processed with different bias parameters:b =0.65,b =0.75,b =0.85,and b =0.95(from left to right).The scene dynamic range is 343,111:1.Radiance map courtesy of Paul Debevec.0.2 0.4 0.6 0.8 1 00.20.40.60.81b = 1.00b = 0.95b = 0.85b = 0.75b = 0.50b = 0.25b = 0.10Figure 3:The bias power function for different value of the parameter b (refer to Equation (3)).In our application,use-ful value for b falls into the range 0.5–1.0.scene illuminant characteristic is not accurately known in most cases,we assume a D 65white point,to further convert tristimulus values between Rec.709RGB and CIE XYZ.The XYZ luminance component Y of each pixel (L w for world luminance)and the maximum luminance of the scene L wmax are divided by the world adaptation luminance L wa and eventually multiplied by an exposure factor set by the user.The tone mapping function presented in Equation (4)is used to compute a displaying value L d for each pixel.This function is derived by inserting Equation (3)into the de-nominator of Equation (2).Equation (4)requires luminance values L w and L wmax (scaled by L wa and the optional expo-sure factor)which characterize the scene as well as L dmax which is the maximum luminance capability of the display-ing medium.The parameter of the bias function is denotedby b (refer to Equation (3)).L d =L dmax ·0.01log 10(L wmax +1)·log (L w +1)log 2+L w L wmaxlog (b )log (0.5)·8(4)L dmax is used as a scalefactor to adapt the output to its in-tended display.In the denominator decimal logarithm is used since the maximum luminance value in the scene is always re-sampled to decimal logarithm by the bias function.We use a value for L dmax =100cd /m 2,a common reference value for CRT displays.The bias parameter b is essential to adjust compression of high values and visibility of details in dark areas.The result of different values for parameter b are visible in Figures 4and 6.The graph in Figure 5shows the curves of the mapping function for a scene with maximum luminance of 230cd /m 2.00.20.40.60.811.21.4050100150200D i s p l a y L u m i n a n c eWorld Luminancelogmap(bias(1.))logmap(bias(.9))logmap(bias(.8))logmap(bias(.7))logmap(bias(.6))logmap(bias(.5))Figure 5:Example plots of the tone mapping function (re-fer to Equation (4))for L wmax =230cd /m 2.The maximum displayable value (white)is 1.For bias parameters smaller than b =0.7clamping to L dmax will occur.©The Eurographics Association and Blackwell Publishers 2003.Values for b between0.7and0.9are most useful to gen-erate perceptually good images,but ideally a uniquefixed parameter working for most situations is needed.In an infor-mal evaluation,we askedfive persons to interactively choose from six different scenes,among four images tone mapped with different bias parameters(Figure4was a part of the sur-vey)the ones they felt looked the most realistic and the most pleasing.In terms of preference a bias parameter around0.85 was consistently proposed.Results for realism were scat-tered for different images but consistent for each subject. Averaging preferences and realism from this simple evalu-ation,we propose a default bias parameter b=0.85.This indeed produces consistent,well balanced images with any kind of scenes.A side effect of changing the biasparame-Figure6:Closeup of a light source of the ATRIUM scene at Aizu University(refer to Figure8for the full view of this scene in daylight conditions).Thisfigure illustrates how high luminance values are clamped to the maximum displayable value.The images were computed using the following bias parameter values:b=0.5,b=0.7,and b=0.9(from left to right).The scene dynamic range is11,751,307:1.ter is some brightnessfluctuation of the output image.The image brightness is approximately doubled for b=0.85and tripled for b=0.7in respect to images for b=1.0.This affects the realism of images even though the increase of contrast due to the bias value reduction naturally leads to brighter images.We introduce here a scalefactor to world adaptation luminance,aiming at keeping a constant bright-ness impression.Again the adaptation is based on a default bias parameter equal to0.85:L wa=L wa/(1+b−0.85)5Figures4and6benefited from this scalefactor,the global brightness impression is almost constant,even though the contrast among images is very different.4.Gamma CorrectionGamma correction must be applied to the tone mapped data to compensate for the non-linearity of displaying devices. It is common to use a gamma coefficientγ=2.2for non-corrected displays,the gamma correction function is L d= L1/γw.In our pipeline,this correction is applied to linear RGB tris-timulus values after tone mapping and conversion from CIE XYZ.We would like to address a potential problem of the gamma function.At the origin’s vicinity,the gamma func-tion exhibits a very steep slope.Even though we usefloat-ing point precision1,after correction and quantization to24 bit,originally dark pixels will all be transformed to medium values.This results in significant contrast and detail loss in shadowed areas.Ward’s Histogram Adjustment method7en-sures that all displayable values are represented in thefinal image.However,other tone mapping methods potentially suffer from this phenomenon and the contrast of their result-ing images might be improved by considering this problem. Gamma functions with better perceptual accuracy have been 00.10.20.30.40.50.60.70.80.910 0.2 0.4 0.6 0.8 1gamma = 2ITU linear0.10.20.30.40 0.02 0.04 0.06 0.08 0.1Figure7:Comparison of the gamma power function usually used in computer graphics with the ITU-R BT.709transfer function.The essential difference is the less drastic mapping of dark pixels in the ITU-R BT.709function.proposed,for example the sRGB color space includes a spe-cific transformation.The international standard recommen-dation is the ITU-R BT.709transfer function11;it describes the transformation done by a video camera to produce the best possible images on a calibrated display.This function is close toγ=2,but assumes aγ=1.125correction of the dis-play to compensate for the viewing environment.The prin-cipal differences between the gamma power function and the ITU-R BT.709transfer function(refer to Figure7)are smaller output values for dark pixels in the latter case.This results in better contrast and details in dark areas and po-tential attenuation of the noise often found in dark parts of photographs.In its original form,the ITU-R BT.709gamma correction is:E =4.5L L≤0.0181.099L0.45−0.099L>0.018where L is the linear value of each RGB tristimulus and E the nonlinear pixel value to be displayed.The ITU-R BT.709transfer function hasfixed parameters. This lacks convenience for computer graphics applications, where different transfer values might be needed depending on the lighting conditions surrounding the display,custom user settings,and operating system.We adapted the function to use familiarγvalues and we use a simplefit of the lin-ear segment at the origin.Our transfer function based on the©The Eurographics Association and Blackwell Publishers2003.ITU-R BT.709standard is:E =slope ·LL ≤start 1.099L 0.9γ−0.099L >startWhere slope is the elevation ratio of the line passing by the origin and tangent to the curve,and start is the abscissa at the point of tangency.All the images in this paper have been corrected with this custom gamma function using γ=2.2.Direct comparison of images shows some contrast enhance-ment in dark to medium areas,while keeping a similar over-all brightness.5.Implementation and ResultsIn our original scheme,each pixel is processed with Equa-tion (4),and computation time increases linearly with the number of pixels.This is too slow for realtime applications so we have been looking for a faster solution.We rejected the idea of using a lookup table because it would need too many entries to satisfy the wide dynamic range,and a dif-ferent table is needed if the user changes a parameter in the tone mapping procedure.Evaluation of the bias function for each pixel is particularly expensive.We found that if the luminance difference among pixels is below a certain threshold,the bias function can be evaluated just once for their average luminance value.In practice,we split the input image into 3×3pixel tiles and perform the bias computation for each group of nine pixels.This adaptation gives a substantial speedup even for very de-tailed scenes (e.g.,MEMORIAL )and performs even better for simple scenes such as the computer generated ROOM .Further acceleration was obtained by a Pade approximation of log (x +1),for low radiance values.Table 1shows the computation time of our algorithm for five scene (refer to Figures 4and 8)of different sizes,the speedup factor re-sulting from our optimizations,and the percentage of error introduced by those optimization.In all the images tested,we could not visually detect a difference between the results of the original algorithm and the faster version.SceneSize Base Fast Speedup Diff (pixels)(sec.)(sec.)#times (%)MEMORIAL 512×7680.1430.036 3.970.75NAVE 720×4800.1260.031 4.060.21ROOM 3000×1950 2.1110.428 4.930.43ATRIUM 1016×7600.2810.061 4.610.22PANORAMA2000×9010.6520.153 4.260.14Table 1:Tone mapping routine execution time before and af-ter optimization,speed increase,and the RMS image differ-ence.Algorithm running on a Pentium IV 2.2GHz,compiled with Intel C compiler version 5.The tone mapping routine is taken into account here.Image IO,color space transforma-tion,and the initial calculation of L wa remain constant and are not part of the table.5.1.A High Dynamic Range Movie PlayerIn some multimedia applications,it can be more convenient to distribute video streams in an HDR format.This way,end-users are able to tune display parameters to accommo-date for their hardware characteristics as well as external lighting conditions.Also,the animation might be adjusted according to personal preference,achieving a desired bal-ance between reproduced contrast,details,and brightness.At present,these settings must be decided by the distributor and are fixed for streamed video.To address those issues we implemented our tone map-ping method in a HDR movie player.Instead of saving al-ready tone mapped 24bit images,we created a HDR movie file format in which the physical radiance value of each pixel is available.This offers the same advantages and capabilities as for static HDR scenes.The viewer has access to a percep-tually tuned rendition of the movie through tone mapping while conserving the original HDR data.Beside exposure adjustment,setting the maximum display luminance L max makes optimal viewing possible with any kind of display,and interactive adjustment of the bias parameter results in different luminance and contrast compression.We use Ward’s RGBE format 19to build the movie file.In-stead of using three floating point values (96-bits),each pixel is represented by four integers (32-bits).The obvious advan-tage is a 2/3file size reduction,allowing to save long ani-mations and reading frames from disk in a manageable time.The downside is the exponentiation needed to convert data for tone mapping and display.Also,a major speed bottleneck is the necessary conversion from RGB tristimulus to lumi-nance values and back.For each frame,we precompute and save constants needed for tone mapping,such as world adap-tation L wa and maximum luminance L wmax .These of course would have to be calculated while playing for a VRML ap-plication or an animation rendered in realtime.We simulate the time-dependent adaptation of the human visual system by a weighted averaging of the world adaptation of the last four frames with the current.We implemented two versions of the tone mapping function.A straightforward C routine,and a GPU implementation us-ing the OpenGL fragment program extension.Modern GPUs support SIMD (Single Instruction Multiple Data)instruc-tion sets capable of performing very fast parallelized floating point calculations.The ATI Fire GL X1which we used has eight pixel pipeline and a 256bit memory interface,which means that eight pixels are processed in parallel making the GPU very powerful even with a much slower clock rate than modern CPUs.Table 2summarizes the frame rates obtained on our test PC.6.ConclusionsWe presented a perception-motivated tone mapping algo-rithm for interactive display of high contrast scenes.In our algorithm the scene luminance values are compressed using logarithmic functions,which are computed using different©The Eurographics Association and Blackwell Publishers 2003.Resolution Software Hardwarefps TM fps TM640×48011.834.323.50.01320×24039.07.474.30.01Table2:Statistics for software and hardware implementa-tions of our tone mapping operator.Values denote the aver-age number of frames per second and the overhead in mil-liseconds introduced by tone mapping.bases depending on scene content.The log2function is used in darkest areas to ensure good contrast and visibility,while the log10function is used for the highest luminance values to reinforce the contrast compression.In-between,luminance is remapped using logarithmic values based on the shape of a chosen bias function.This scheme enables fast non-linear tone mapping without objectionable image artifacts. Although our technique is fully automatic,the user can in-teractively choose varying image details versus contrast by effectively changing the shape of the bias curve using an in-tuitive parameter.Because of the computation performance our tone mapping can be used for playing HDR video se-quences while providing the user unprecedented control over the video brightness,contrast compression,and detail repro-duction.As future work we intend to perform perceptual exper-iments to determine an automatic bias value as a function of the scene content and its dynamic range of luminance. Our approach might be further extended by using different functions to interpolate between logarithmic bases.Also,the HVS models of temporal adaptation could be incorporated to our tone mapping algorithm to make the displaying of video sequences more realistic.AcknowledgementsWe would like to thank Paul Bourke for permitting us to use his OpenGL based stereo animation viewing soft-ware as a framework for our HDR movie player.Also,we would like to thank Paul Debevec,Greg Ward,Raanan Fat-tal,Jack Tumblin,and Gregory Downing for making their HDR images and animations available.We thank Grzegorz Krawczyk for help in measuring timings for the GPU ver-sion of our HDR video player.This work was supported partly by Telecommunications Advancement Organization of Japan within the framework of“A Support System for Region-specific R&D Activities”and by the European Com-munity within the scope of the RealReflect project IST-2001-34744“Realtime visualization of complex reflectance be-havior in virtual prototyping”.References1.J.Blinn.Dirty Pixels.IEEE Computer Graphics&Applications,9(4):100–105,1989.52.P.E.Debevec and J.Malik.Recovering High DynamicRange Radiance Maps from Photographs.Proceedings of ACM SIGGRAPH97,ACM,369–378,1997.13.P.E.Debevec.Rendering Synthetic Objects Into RealScenes:Bridging Traditional and Image-Based Graph-ics With Global Illumination and High Dynamic Range Photography.Proceedings of ACM SIGGRAPH98, ACM,189–198,1998.14.K.Devlin,A.Chalmers,A.Wilkie,and W.Purgathofer.Tone Reproduction and Physically Based Spectral Ren-dering.Eurographics2002:State of the Art Reports, Eurographics,101–123,2002.25.Industrial Light&Magic,OpenEXR,High DynamicRange Image File Format.Lucas Digital Ltd,20031 rson.LogLuv Encoding for Full-Gamut,High-Dynamic Range Images.Journal of Graphics Tools.A K Peters Ltd.,3(1):815–830,1998.1rson,H.E.Rushmeier,and C.Piatko.A Visi-bility Matching Tone Reproduction Operator for High Dynamic Range Scenes.IEEE Transactions on Visual-ization and Computer Graphics,3(4):291–306,1997.2,5ler,P.Y.Ngai,and ler.The Applicationof Computer Graphics in Lighting Design.Journal of the Illuminating Engineering Society,14(1):6–26,1984 19.K.Perlin and puterGraphics(Proceedings of ACM SIGGRAPH89),ACM, 23,253–262,1989.310. C.A.Poynton.A Technical Introduction to DigitalVideo.John Wiley&Sons,199611.Recommendation ITU-R BT.709-4,Parameter Valuesfor the HDTV Standards for Production and Interna-tional Programme Exchange,ITU,2000512. E.Reinhard,M.Stark,P.Shirley,and J.Ferwerda.Pho-tographic Tone Reproduction for Digital Images,ACM Transactions on Graphics,21(3):267–276,2002.3 13. A.Scheel,M.Stamminger,and H-P.Seidel.Tone Re-production for Interactive puter Graphics Forum,19(3):301–312,2000.314. C.Schlick.Quantization Techniques for the Visualiza-tion of High Dynamic Range Pictures.Photorealistic Rendering Techniques,Springer-Verlag,7–20,1994.2 15.T.G.Stockham.Image Processing in the Context of aVisual Model,Proceedings of the IEEE,60:828–8422 16.J.Tumblin and H.E.Rushmeier.Tone Reproductionfor Realistic Images.IEEE Computer Graphics and Applications,13(6):42–48,1993.1,2,3©The Eurographics Association and Blackwell Publishers2003.。
Color Transfer between Images
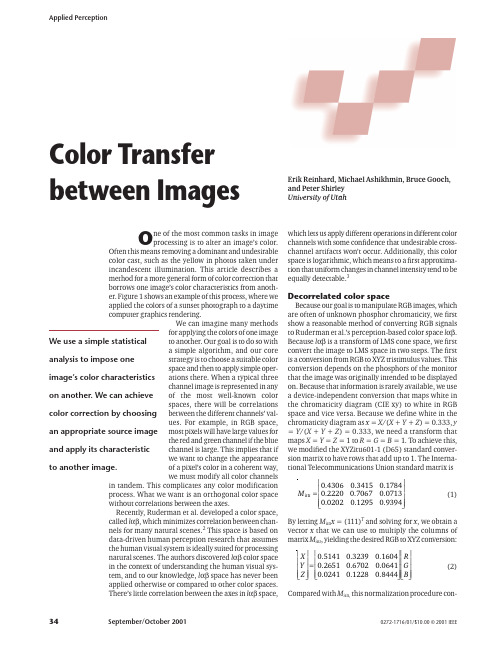
(3)
Combining these two matrices gives the following transformation between RGB and LMS cone space: L 0.3811 0.5783 0.0402 R M = 0.1967 0.7244 0.0782 G S 0.0241 0.1288 0.8444 B
(1)
By letting Mitux = (111)T and solving for x, we obtain a vector x that we can use to multiply the columns of matrix Mitu, yielding the desired RGB to XYZ conversion: X 0.5141 0.3239 0.1604 R Y = 0.2651 0.6702 0.0641 G Z 0.0241 0.1228 0.8444 B
(5)
Using an ensemble of spectral images that represents a good cross-section of naturally occurring images, Ruderman et al. proceed to decorrelate these axes. Their motivation was to better understand the human visual system, which they assumed would attempt to process input signals similarly. We can compute maximal decorrelation between the three axes using principal components analysis (PCA), which effectively rotates them. The three resulting orthogonal principal axes have simple forms and are close to having integer coefficients. Moving to those nearby integer coefficients, Ruderman et al. suggest the following transform: l α = β 1 3 0 0 1 6 0 0 0 1 1 1 L 0 1 1 −2 M 1 −1 0 S 1 2
光谱层英文版
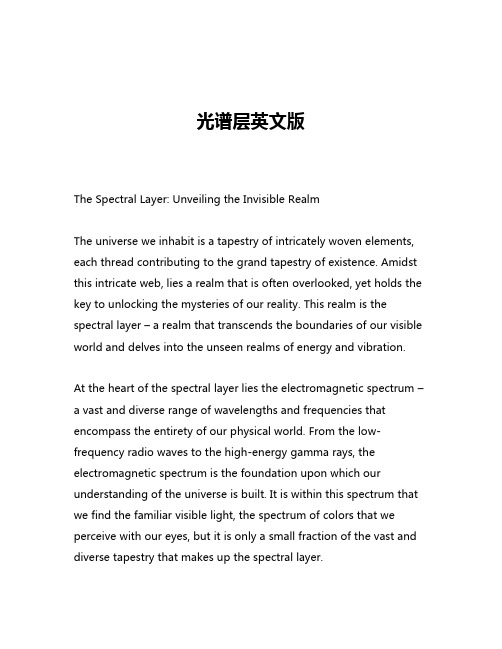
光谱层英文版The Spectral Layer: Unveiling the Invisible RealmThe universe we inhabit is a tapestry of intricately woven elements, each thread contributing to the grand tapestry of existence. Amidst this intricate web, lies a realm that is often overlooked, yet holds the key to unlocking the mysteries of our reality. This realm is the spectral layer – a realm that transcends the boundaries of our visible world and delves into the unseen realms of energy and vibration.At the heart of the spectral layer lies the electromagnetic spectrum –a vast and diverse range of wavelengths and frequencies that encompass the entirety of our physical world. From the low-frequency radio waves to the high-energy gamma rays, the electromagnetic spectrum is the foundation upon which our understanding of the universe is built. It is within this spectrum that we find the familiar visible light, the spectrum of colors that we perceive with our eyes, but it is only a small fraction of the vast and diverse tapestry that makes up the spectral layer.Beyond the visible spectrum, there lies a realm of unseen energies that are integral to the very fabric of our existence. Infrared radiation, for instance, is a form of electromagnetic radiation that is invisible to the human eye but plays a crucial role in the transfer of heat and the functioning of various biological processes. Similarly, ultraviolet radiation, though invisible to us, is essential for the production of vitamin D and the regulation of circadian rhythms.But the spectral layer extends far beyond the confines of the electromagnetic spectrum. It is a realm that encompasses the vibrations and frequencies of all matter and energy, from the subatomic particles that make up the building blocks of our universe to the vast cosmic structures that span the vastness of space. These vibrations and frequencies, though often imperceptible to our senses, are the foundation upon which the entire universe is built.At the quantum level, the spectral layer reveals the true nature of reality. Subatomic particles, such as electrons and quarks, are not merely static entities but rather dynamic oscillations of energy, each with its own unique frequency and vibration. These vibrations, in turn, give rise to the fundamental forces that govern the behavior of matter and energy, from the strong nuclear force that holds the nucleus of an atom together to the mysterious dark energy that drives the expansion of the universe.But the spectral layer is not merely a realm of the infinitely small. It also encompasses the vast and expansive structures of the cosmos, from the intricate patterns of galaxies to the pulsing rhythms of celestial bodies. The stars that dot the night sky, for instance, are not merely points of light but rather vast nuclear furnaces, each emitting a unique spectrum of electromagnetic radiation that can be detected and analyzed by scientists.Through the study of the spectral layer, we have gained unprecedented insights into the nature of our universe. By analyzing the spectra of distant galaxies, for example, we can determine their chemical composition, their age, and even their rate of expansion –information that is crucial for our understanding of the origins and evolution of the cosmos.But the spectral layer is not just a realm of scientific inquiry – it is also a realm of profound spiritual and metaphysical exploration. Many ancient and indigenous cultures have long recognized the importance of the unseen realms of energy and vibration, and have developed sophisticated systems of understanding and interacting with these realms.In the traditions of Hinduism and Buddhism, for instance, the concept of the chakras – the seven energy centers that are believed to govern various aspects of our physical, emotional, and spiritualwell-being – is a manifestation of the spectral layer. These energy centers are believed to be connected to specific frequencies and vibrations, and the practice of chakra meditation and balancing is seen as a way to align oneself with the natural rhythms of the universe.Similarly, in the traditions of shamanism and indigenous healing practices, the concept of the "spirit world" or the "unseen realm" is closely tied to the spectral layer. Shamans and healers are often said to be able to perceive and interact with the unseen energies that permeate our world, using techniques such as drumming, chanting, and plant medicine to access these realms and bring about healing and transformation.In the modern era, the spectral layer has become the subject of intense scientific and technological exploration. From the development of advanced imaging technologies that can reveal the unseen structures of the human body to the creation of sophisticated communication systems that harness the power of the electromagnetic spectrum, the spectral layer has become an essential component of our understanding and manipulation of the physical world.Yet, despite the immense progress we have made in our understanding of the spectral layer, there is still much that remainsunknown and mysterious. The nature of dark matter and dark energy, for instance, remains one of the greatest unsolved puzzles in modern physics, and the true nature of consciousness and the relationship between the physical and the metaphysical realms continues to be a subject of intense debate and exploration.As we continue to delve deeper into the spectral layer, we may uncover even more profound insights into the nature of our reality. Perhaps we will discover new forms of energy and vibration that have yet to be detected, or perhaps we will find that the boundaries between the seen and the unseen are far more permeable than we ever imagined. Whatever the future may hold, one thing is certain: the spectral layer will continue to be a source of fascination, inspiration, and mystery for generations to come.。
伊士达高Tg、低CTE、多功能填充环氧树脂和酚醛固化层压板和预浸料IT-180ABS IT-180A
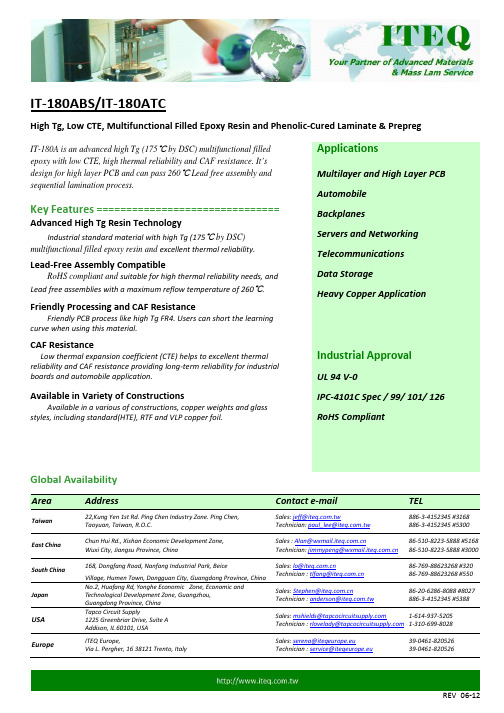
IT-180ABS/IT-180ATCHigh Tg, Low CTE, Multifunctional Filled Epoxy Resin and Phenolic-Cured Laminate & PrepregIT-180A is an advanced high Tg (175℃ by DSC) multifunctional filled epoxy with low CTE, high thermal reliability and CAF resistance. It’s design for high layer PCB and can pass 260℃ Lead free assembly and sequential lamination process.Key Features =============================== Advanced High Tg Resin TechnologyIndustrial standard material with high Tg (175℃ by DSC) multifunctional filled epoxy resin and excellent thermal reliability.Lead-Free Assembly CompatibleRoHS compliant and suitable for high thermal reliability needs, and Lead free assemblies with a maximum reflow temperature of 260℃. Friendly Processing and CAF ResistanceFriendly PCB process like high Tg FR4. Users can short the learning curve when using this material.CAF ResistanceLow thermal expansion coefficient (CTE) helps to excellent thermal reliability and CAF resistance providing long-term reliability for industrial boards and automobile application.Available in Variety of ConstructionsAvailable in a various of constructions, copper weights and glass styles, including standard(HTE), RTF and VLP copper foil. ApplicationsMultilayer and High Layer PCB AutomobileBackplanesServers and Networking TelecommunicationsData StorageHeavy Copper ApplicationIndustrial ApprovalUL 94 V-0IPC-4101C Spec / 99/ 101/ 126 RoHS CompliantGlobal AvailabilityArea Address Contact e-mail TELTaiwan 22,Kung Yen 1st Rd. Ping Chen Industry Zone. Ping Chen,Taoyuan, Taiwan, R.O.C.Sales: *************.twTechnician: *****************.tw886-3-4152345 #3168886-3-4152345 #5300East China Chun Hui Rd., Xishan Economic Development Zone,Wuxi City, Jiangsu Province, ChinaSales : ****************Technician: *********************86-510-8223-5888 #516886-510-8223-5888 #3000South China168, Dongfang Road, Nanfang Industrial Park, BeiceVillage, Humen Town, Dongguan City, Guangdong Province, China Sales: ***********.cnTechnician : ***************.cn86-769-88623268 #32086-769-88623268 #550Japan No.2, Huafang Rd, Yonghe Economic Zone, Economic andTechnological Development Zone, Guangzhou,Guangdong Province, ChinaSales: ****************.cnTechnician : *****************.tw86-20-6286-8088 #8027886-3-4152345 #5388USA Tapco Circuit Supply1225 Greenbriar Drive, Suite AAddison, IL 60101, USASales: *******************************Technician : ********************************1-614-937-52051-310-699-8028Europe ITEQ Europe,Via L. Pergher, 16 38121 Trento, Italy Sales: ********************Technician : *********************39-0461-82052639-0461-820526REV 06-12ITEQ Laminate/ Prepreg : IT-180ATC / IT-180ABS IPC-4101C Spec / 99 / 101 / 126LAMINATE( IT-180ATC)Thickness<0.50 mm[0.0197 in] Thickness≧0.50 mm[0.0197 in] Units T est MethodPropertyTypical Value Spec Typical Value SpecMetric(English)IPC-TM-650(or as noted)Peel Strength, minimumA. Low profile copper foil and very low profile copperfoil - all copper weights > 17µm [0.669 mil]B. Standard profile copper foil1.After Thermal Stress2.At 125°C [257 F]3.After Process Solutions 0.88 (5.0)1.23 (7.0)1.05 (6.0)1.05 (6.0)0.70 (4.00)0.80 (4.57)0.70 (4.00)0.55 (3.14)0.88 (5.0)1.40 (8.0)1.23 (7.0)1.23 (7.0)0.70 (4.00)1.05 (6.00)0.70 (4.00)0.80 (4.57)N/mm(lb/inch)2.4.82.4.8.22.4.8.3Volume Resistivity, minimumA. C-96/35/90B. After moisture resistanceC. At elevated temperature E-24/125 3.0x1010--5.0x1010106--103--3.0x10101.0x1010--104103MΩ-cm 2.5.17.1Surface Resistivity, minimumA. C-96/35/90B. After moisture resistanceC. At elevated temperature E-24/125 3.0x1010--4.0x1010104--103--3.0x10104.0x1010--104103MΩ 2.5.17.1Moisture Absorption, maximum -- -- 0.12 0.8 % 2.6.2.1 Dielectric Breakdown, minimum -- -- 60 40 kV 2.5.6 Permittivity (Dk, 50% resin content)(Laminate & Laminated Prepreg)A. 1MHzB. 1GHzC. 2GHzD. 5GHzE. 10GHz 4.44.44.24.14.05.44.44.44.34.14.15.4 --2.5.5.92.5.5.13Loss Tangent (Df, 50% resin content) (Laminate & Laminated Prepreg)A. 1MHzB. 1GHzC. 2GHzD. 5GHzE. 10GHz 0.0150.0150.0150.0160.0170.0350.0140.0150.0150.0160.0160.035 --2.5.5.92.5.5.13Flexural Strength, minimumA. Length directionB. Cross direction ----------------500-530(72,500-76,850)410-440(59,450-63,800)415(60,190)345(50,140)N/mm2(lb/in2)2.4.4Arc Resistance, minimum 125 60 125 60 s 2.5.1 Thermal Stress 10 s at 288°C [550.4F],minimumA. UnetchedB. Etched PassPassPass VisualPass VisualPassPassPass VisualPass VisualRating 2.4.13.1Electric Strength, minimum(Laminate & Laminated Prepreg)45 30 -- -- kV/mm 2.5.6.2 Flammability,(Laminate & Laminated Prepreg)V-0 V-0 V-0 V-0 Rating UL94 Glass Transition Temperature(DSC) 175 170 minimum 175 170 minimum ˚C 2.4.25Decomposition Temperature-- -- 345 340 minimum ˚C2.4.24.6 (5% wt loss)X/Y Axis CTE (40℃ to 125℃) -- -- 10-13 -- PPM/˚C 2.4.24 Z-Axis CTEA. Alpha 1B. Alpha 2C. 50 to 260 Degrees C ------------452102.760 maximum300 maximum3.0 maximumPPM/˚CPPM/˚C%2.4.24Thermal ResistanceA. T260B. T288 -------->60>3030 minimum15 minimumMinutesMinutes2.4.24.1CAF Resistance -- -- Pass AABUS Pass/Fail 2.6.25The above data and fabrication guide provide designers and PCB shop for their reference. We believe that these information are accurate, however, the data may vary depend on the test methods and specification used. The actual sales of the product should be according to specification in the agreement between ITEQ and its customer. ITEQ reserves the right to revise its data at any time without notice and maintain the best information available to users.REV 06-12。
冷原子光谱法 英语

冷原子光谱法英语Okay, here's a piece of writing on cold atom spectroscopy in an informal, conversational, and varied English style:Hey, you know what's fascinating? Cold atom spectroscopy! It's this crazy technique where you chill atoms down to near absolute zero and study their light emissions. It's like you're looking at the universe in a whole new way.Just imagine, you've got these tiny particles, frozen in place almost, and they're still putting out this beautiful light. It's kind of like looking at a fireworks display in a snow globe. The colors and patterns are incredible.The thing about cold atoms is that they're so slow-moving, it's easier to measure their properties. You can get really precise data on things like energy levels andtransitions. It's like having a super-high-resolution microscope for the quantum world.So, why do we bother with all this? Well, it turns out that cold atom spectroscopy has tons of applications. From building better sensors to understanding the fundamental laws of nature, it's a powerful tool. It's like having a key that unlocks secrets of the universe.And the coolest part? It's just so darn cool! I mean, chilling atoms to near absolute zero? That's crazy science fiction stuff, right?。
Optimal Transform in Perceptually Uniform Color Space and Its Application in Image Coding
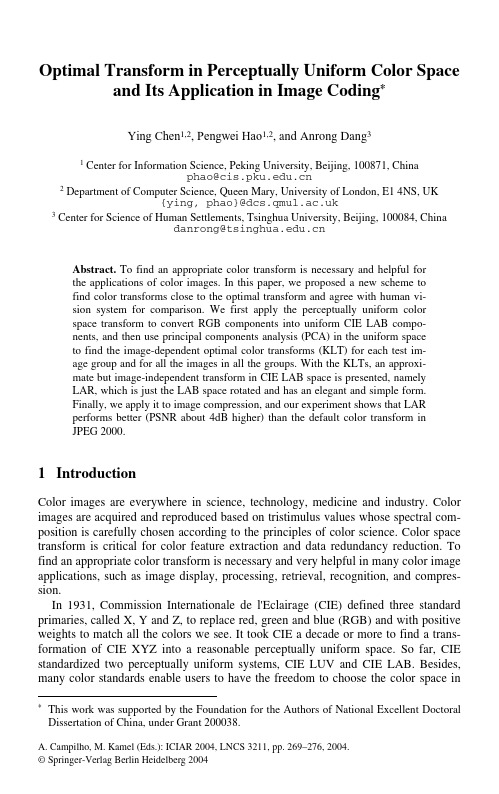
This work was supported by the Foundation for the Authors of National Excellent Doctoral Dissertation of China, under Grant 200038.
A. Campilho, M. Kamel (Eds.): ICIAR 2004, LNCS 3211, pp. 269–276, 2004. © Springer-Verlag Berlin Heidelberg 2004
Optimal Transform in Perceptually Uniform Color Space and Its Application in Image Coding*
Ying Chen1,2, Pengwei Hao1,2, and Anrong Dang3
Center for Information Science, Peking University, Beijing, 100871, China phao@ 2 Department of Computer Science, Queen Mary, University of London, E1 4NS, UK {ying, phao}@ 3 Center for Science of Human Settlements, Tsinghua University, Beijing, 100084, China danrong@
270
Y. Chen, P. Hao, and A. Dang
which to represent their data. RGB, CMYK, YIQ, HSV, CIE 1931 XYZ, CIE LUV, CIE LAB, YES, CCIR 601-2 YCbCr, and SMPTE-C RGB are proposed for diverse requirements [1]. However, in many applications, we need some appropriate color transforms and we also wish the transformed or the inverse-transformed components are inter-comparable and the comparison done by computers agrees with that we do by our human visual system. Therefore, we need compare the results in a perceptually uniform color space after applying inverse of our specific color space transforms. Our idea is to find some optimal color transforms in the uniform space. In this paper, a new scheme to find an optimal color transform is proposed. We transform color images into three components in the uniform space CIE LAB, and then use principal components analysis (PCA) to find image-dependent optimal color transforms, Karhunen-Lòeve Transform (K-L transform, or KLT). Finally, we take the optimal transform obtained from all the analyzed images as an image-independent color transform and apply it to image compression of some other test images with JPEG 2000.
ADAPTIVE SPATIAL GAMUT MAPPING VIA DYNAMIC THRESHO

专利名称:ADAPTIVE SPATIAL GAMUT MAPPING VIADYNAMIC THRESHOLDING发明人:Vishal MONGA,Raja BALA,MichaelBRANCIFORTE申请号:US12429429申请日:20090424公开号:US20100272355A1公开日:20101028专利内容由知识产权出版社提供专利附图:摘要:What is disclosed is a novel system and method for performing spatial gamut mapping on a received input color image having a plurality of pixels. A standard gamut-mapping algorithm is applied to the input color image to produce a gamut-mapped color image. A difference is computed between a selected channel of the input color image and the gamut-mapped image to produce a difference image. A local measure of complexity is derived for a given pixel in the difference image. One or more parameter values of a spatial bilateral filter are obtained from a lookup table based on the computed local measure of complexity. The spatial bilateral filter is applied, using the obtained parameter values, to the current pixel of the difference image to produce a modified pixel in a modified difference image. Thereafter, a modified gamut-mapped color image is obtained from the modified difference image and the gamut-mapped color image.申请人:Vishal MONGA,Raja BALA,Michael BRANCIFORTE地址:Webster NY US,Webster NY US,Rochester NY US国籍:US,US,US更多信息请下载全文后查看。
传感与检测技术单词

acidic酸的,酸性的actuator执行器agglomerate凝聚的agitation激励align调准,校正alignment排列整齐alkane烷烃alnico磁钢ambient周围的ammonia氨amplitude振幅analytical分析的anesthesia知觉缺失angular角的,有角的antenna天线antiparallel反平行的aperture孔,缝隙arbitrary任意的arsenide砷化物asymmetrical不对称的attenuator衰减器autotransformer自偶变压器bias偏差bidirectional双向的binary二进位的biomedical生物医学的bipolar双极的bombardment撞击, 轰击borosilicate硼硅酸盐bulky容量大的, 体积大的burden负担,荷载cadmium-based镉基的calibrate校准cancellation取消cardboard纸板cathode阴极chip芯片clarity清楚clastic可拆开的cockpit驾驶员座舱coefficient系数collision碰撞,冲突colorimetric比色的combination结合commercially商业上comparator比较器compatible兼容的compensate补偿component成分concentric同心的conductometry导热计configuration配置conformity一致consequence结果,推论constitute组成consumption消耗contaminant污染物contamination-prone易于污染的convenience便利conveniently便利地conventional常规的conveyor运输带coordinates坐标core核Coriolis科里奥利(法国数学家)criteria标准criterion标准,规范cryogenic低温学的decimal十进的deduce推论deformation变形demodulator解调器densitometer比重计density密度derivative导数diamagnetic反磁性的differential微分的diode二极管dioxide二氧化物dipole偶极子dissimilar相异的dissipation消耗dissociate分离distortion变形documentation文件dynamic动态的dynamometer测力计,功率计elapse(时间)过去, 消逝electrolyte电解液electromagnetic电磁的electron电子electrostatic静电的elementary基本的emitter发射器enamel搪瓷, 珐琅encounter遇到enzyme酶equate使相等equilibrium平衡equivalent相等的estimate估计evaluator估计器evaporate使蒸发,消失excitation激励exhaustive排气的,彻底的exponential指数的extracted萃取的extrapolation外推法, 推断fermentation发酵ferromagnetic铁磁的fiber-optic光纤filament细丝filtering过滤finite有限的flammable易燃的flowmeter流量计flux流量,通量frame框架,帧fringe边缘frit玻璃料fundamental基本的gadolinium钆gallium镓gaseous气体的gauge测量仪器generator发电机geological地质的geometrical几何学的geometry几何学geophysics地球物理学granularity粒度gravity重力guarantee保证gyro陀螺仪hairpin发夹halogen卤素horizontal水平的humidifier湿度调节器humidity湿度hycomax铝镍钴系永久磁铁hydrated含水的hydraulic水力的hydrocarbon炭氢化合物hydrostatic流体静力学的hygroscopic吸湿的hypothermia低温identical同一的,同样的identifier标识符identity一致illumination照明,照度illustrate举例说明,图解imbalance不平衡immobilization固定impedance阻抗implement实现implementation执行,落实inaccuracy不精确incubator恒温箱indeterminate不确定的indicator指示器inductosyn感应式传感器inertia惯性infrared红外线的initial初始的instability不稳定(性) instantaneous瞬间的instrumentation测试设备insulate使绝缘insulation绝缘integrate使成整体interference冲突,干涉interposition插入interpret解释ionize电离irrelevant不相关的isolation绝缘joystick操纵杆karma卡玛合金laboratory实验室layout规划,版面lead-based铅基的lithium锂logarithm对数logarithmic对数的longitudinal纵向的macroscopic宏观的magnet磁铁magnetization磁化magnetoresistive磁阻的magnetoresistor磁阻magnitude数量,量级manual手册margin边限,范围matrix矩阵mechanical机械的megahertz兆赫mercury水银metallic金属的microcoils微型线圈miniaturization小型化minority少数missile导弹mobility迁移率modification修改modifier调节器modulate调节modulus模数molecule分子monolayer单层monoxide一氧化物multilayered多层navigation航海nebulizer喷雾器negligible可以忽略的neoprene氯丁橡胶nichrome镍铬铁合金nitride氮化物nitrogen氮nonfilled未填充的noninvasive非入侵的nonmagnetic无磁性的nonmetallic非金属的nonuniformity不均匀null无效的,零numeric数字的offset偏移量ohmic欧姆的optimal优化的,最佳的optimize使最优化optocoupler光偶orbital轨道的organic有机的orientation方向,方位oscillation振动oxidize氧化palpation摸panel面板parallel平行的paramagnetic顺磁性的parameter参数partial部分的,局部的particle粒子passband通频带passivate[冶]使钝化pendulum钟摆performance性能perfume香水periodically周期性的permanent永久的permeability渗透性perpendicular正交的perturb干扰,扰乱phenomena现象photoconductor光电导体photodiode光电二极管photoetched光刻的,光蚀的photolithography光刻法piezoresistive压阻的plasma等离子体platinize镀铂Platinum-tungsten铂钨合金polarization极化,偏振polarize使极化polyurethane聚亚安酯porcelain瓷器potential可能的,电压potentiometer电位器practical实际的precision精度predict预言presentation介绍,陈述procedure程序proportional成比例的protection保护pseudonoise伪噪音quadrant象限quantitative定量的quiescent静止的quotient商radar雷达radiation辐射ramp斜坡reactance电抗rectangular矩形的repeatable可重复的reproducibility再现性reproducible可再生的residual剩余的resonant共振的respiratory呼吸的retractile收缩的robust坚固的, 耐用的rod杆,棒rotation旋转sacrificial牺牲的salinity盐分saturation饱和scatter分散,散开scheme安排,方案section节servomechanism自动控制装置simultaneously同时地slider滑块sliding滑行的slip滑动slope斜坡specification规格specify指定spectra光谱spectroscopy光谱学spectrum光谱spiral螺旋stabilization稳定性stagnation停滞statistical统计学的storage存储straight直的subliminal下意识的submarine水下的,海底的subscript下标subtracting减法sufficient充分的sulfur硫磺superconducting超导的synchronous同步的synonymous同义的tangent切线,正切tangential切线的teflon聚四氟乙烯temporal暂时的terminology术语theoretical理论的thermopile温差电堆thermoplastic热塑性的threshold阈值time-invariant时不变的titration滴定tolerance公差transducer变换器transmission发射,传输trapezoid梯形tridimensional三维空间的tungsten钨turbulence湍流ultrathin超薄的undamped欠阻尼的upstream逆流的urine尿vacuum真空validity有效性,合法性vapor水蒸汽variance变化variation变化vector矢量versus相对,相比vibration振动vibratory振动的viscosity粘度volatile易变的volume体积,量vortex涡流wherein在其中。
Navigator 600 Silica Silica 分析器说明书

Navigator 600 SilicaSilica analyzerCost-effective automated monitoring of silica for a widerange of applicationsLowest cost-of-ownership—up to 90 % lower reagent consumption than competitors' analyzers—labour-saving 5 minute annual maintenance and up to 3 months unattended operation—field upgradeable from 2 to 4; 2 to 6 or 4 to 6 streamsEasy to use—familiar Windows™ menu system—built-in context-sensitive helpFull communications—web- and ftp-enabled for easy data file access, remote viewing and configuration—optional Profibus® DP V1.0Fast, accurate and reliable—automatic cleaning, calibration and zero deliver high accuracy measurements—extensive electronics, measurement and maintenance diagnostics ensure high availability—true auto-zero compensates for sample color, turbidity and background silica in reagents—temperature-controlled reaction and measurement section for optimum responseNavigator 600 Silica Silica analyzer2DS/NAV6S–EN Rev. JIntroductionMany years of experience and innovation in the design and successful application of continuous chemical analyzers has been combined with the latest electronics and production technologies to produce the Navigator 600 Series of analyzers from ABB.Developed as fully continuous analyzers offering wide dynamic ranging, the Navigator 600 Series incorporates greater simplicity and functionality than ever before. Based on colorimetric techniques, they feature a liquid handling section carefully designed to reduce routine maintenance. Utilizing powerful electronics, advanced features such as automatic calibration,continuous sample analysis and programmable multi-stream switching ensure accurate and simple measurement of silica.Process data, as well as the content of alarm and audit logs,can be saved to a removable SD card in binary and comma-delimited formats for record keeping and analysis using ABB’s DataManager data analysis software package.A very low cost of ownership has been achieved by reducing the reagent consumption and simplifying the maintenance requirements.The size of the instrument has been reduced to a compact,ergonomically-designed, wall-mounted case thus providing a very small footprint.ApplicationsTypical applications for the Navigator 600 Silica are:⏹Demineralization Plants for Power and Process Industries.–Monitoring the outlet of the anion and mixed beds for silica breakthrough, providing indication of bed exhaustion and final water quality.⏹Boiler Systems.–Monitoring boiler drum water, providing information on the contamination levels in the boiler.–Monitoring silica carryover in saturated steam thus protecting turbine blades from potentially excessive scale build up.–Monitoring the exhaustion of ion exchangers in a Condensate Polishing Plant.OperationGeneralThe Navigator 600 Silica is an on-line analyzer, designed to provide continuous monitoring of silica concentration utilizing a standard colorimetric analysis principle.Liquid HandlingThe chemistry employed for silica measurement is the industry standard Molybdenum Blue reaction. Sample and reagents are drawn into the instrument by two multichannel peristaltic pumps. These are designed and constructed to ensure only simple yearly maintenance is required. The reagents are added to the sample in a temperature-controlled reaction block and the fully reacted sample is then passed through an in-line measuring cuvette.The optical measuring system enables accurate detection of silica concentrations from 0 to 5000 ppb.The instrument includes a manual sampling facility that enables the analysis of grab samples.Solution ReplacementLiquid Handling Section ContinuousReagents3 months Calibration Standard 3 months Cleaning Solution3 monthsNavigator 600 Silica Silica analyzerDS/NAV6S–EN Rev. J 3ElectronicsThe main electronic transmitter consists of a display and key pad accessible from the front of the unit. Indication of all parameters is provided by a large backlit LCD display that is easy to read in all light conditions. Under normal operating conditions, measured values are displayed; programming data is displayed during set-up and also on demand. Units and range of measurement, alarm values and standard solution values are examples of the many programmable functions.Keeping simplicity of operation at the forefront of design, six fingertip-operated tactile membrane switches control local operation of the analyzer and provide easy access to all parameters.The Navigator 600 Silica is provided with 4 dedicated relays,6user-programmable relays and 6 current outputs as standard.Profibus DP V1.0 is available as an option.Ethernet CommunicationsThe Navigator 600 Silica can provide 10BaseT Ethernet communications via a standard RJ45 connector and uses industry-standard protocols TCP/IP , FTP and HTTP . The use of standard protocols enables easy connection into existing PC networks.Data File Access via FTP (File Transfer Protocol)The Navigator 600 Silica features F TP server functionality. The FTP server in the analyzer is used to access its file system from a remote station on a network. This requires an FTP client on the host PC. Both MS-DOS® and Microsoft® Internet Explorer version 5.5 or later can be used as an FTP client.⏹Using a standard web-browser or other FTP client, datafiles contained within the analyzer's memory or memory card can be accessed remotely and transferred to a PC or network drive.⏹Four individual FTP users' names and passwords can beprogrammed into the Navigator 600 Silica. An access level can be configured for each user.⏹All FTP log-on activity is recorded in the audit log of theinstrument.⏹Using ABB’s data file transfer scheduler program, datafiles from multiple instruments can be backed-up automatically to a PC or network drive for long-term storage, ensuring the security of valuable process data and minimizing the operator intervention required.Display and KeypadChart View DisplayNavigator 600 Silica(FTP Server)Navigator 600 Silica(FTP Server)FTP ClientEthernetNavigator 600 Silica Silica analyzer4DS/NAV6S–EN Rev. JEmbedded Web ServerThe Navigator 600 Silica has an embedded web-server that provides access to web pages created within the instrument.The use of HTTP (Hyper Text Transfer Protocol) enables standard web browsers to view these pages.⏹Accessible through the web pages are the current displayof the analyzer, detailed information on stream values, reagent and solution levels, measurement status and other key information.⏹The audit and alarm logs stored in the Navigator 600Silica's internal buffer memory and memory card can be viewed on the web pages.⏹Operator messages can be entered via the web server,enabling comments to be logged to the instrument.⏹The web pages and the information they contain arerefreshed regularly, enabling them to be used as a supervision tool.⏹The analyzer's configuration can be selected from anexisting configuration in the internal memory or a new configuration file transferred to the instrument via FTP .⏹The analyzer's real-time clock can be set via the webserver. Alternatively, the clocks of multiple analyzers can be synchronized using ABB's File Transfer Scheduler software.Email NotificationVia the Navigator 600 Silica's built-in SMTP client, the analyzer is able to email notification of important events. Emails triggered from alarms or other critical events can be sent to multiple recipients. The analyzer can also be programmed to email reports of the current measurement status or other parameters at specific times during the day.ProfibusThe Navigator 600 Silica can be equipped (option) with Profibus DP V1.0 to enable full communications and control integrationwith distributed control systems.Navigator 600 Silica Silica analyzerDS/NAV6S–EN Rev. J 5MaintenanceThe analyzer has been designed to maximize on-line availability by reducing routine maintenance to a minimum.Yearly maintenance consists of simply replacing pump capstans and pump tube assemblies, an operation that can take as little as five minutes.F ully automatic calibration, zeroing and cleaning functions enable the analyzer to keep operational with minimal manual intervention. A predictive alarm alerts the user to reagent solution replacement being required. The cleaning and calibration solutions have a sensor to detect when replacement is necessary.OptionsMulti-stream FacilityA fully programmable multi-stream option is available on the Navigator 600 Silica on-line analyzer, providing up to six-stream capability including individual current output and visual indication as well as user-programmable stream sequencing.The analyzers are designed to be easily upgradeable in the field to two, four or six streams.Simple to replace pump tube assemblesSix Streams DisplayNavigator 600 Silica Silica analyzer6DS/NAV6S–EN Rev. JSpecificationSilica MeasurementRangeFully user programmable 0 to 5000 ppb SiO 2, minimum range 0 to 50 ppbMeasurement ModesSample stream options Available as single stream ormulti-stream in 2, 4 or 6 stream configurationsSingle-stream PerformanceMeasurement methodContinuous chemistry and measurement operation.Response time<15 mins. (90% step change)Typical accuracy<±2% of reading or ±0.5 ppb (whichever is the greater) over the range 0 to 500 ppb <±5% of reading over the range 500 to 5000 ppbRepeatability<±2% of reading or ±0.5 ppb (whichever is the greater) over the range 0 to 500 ppb <±3% of reading over the range 500 to 5000 ppbMulti-stream performanceMeasurement methodContinuous chemistry with a minimum 12 minutes per stream measurement update.Sample rate programmable between 12 minutes minimum to 60minutes maximum.Response timeMinimum update time 12 minutesTypical accuracy<±2% of reading or ±0.5 ppb (whichever is the greater) over the range 0 to 500 ppb*<±5% of reading over the range 500 to 5000 ppb** Dependent on sample rate – refer to table on page 2Repeatability<±2% of reading or ±0.5 ppb (whichever is the greater) over the range 0 to 500 ppb <±3% of reading over the range 500 to 5000 ppbSolution RequirementsNumber4 reagents (2.5 l bottles)1 standard solution (0.5 l bottle)1 cleaning solution (0.5 l bottle)Reagent ConsumptionContinuous operation mode 2.5 l max. per 90 daysDisplayColor, passive matrix, liquid crystal display (LCD) with built-in backlight and brightness adjustment 76800 pixel display(a small percentage of the display pixels may be either constantly active or inactive. Max. percentage of inoperative pixels <0.01%)Dedicated operator keys⏹Group Select/Left cursor ⏹View Select/Right cursor ⏹Menu key ⏹Up/Increment key ⏹Down/Decrement key ⏹Enter keyMechanical DataIngress protectionIP31** – Wet section (critical components IP66)IP66 – Transmitter Dimensions Materials of construction Sample connections ** Not evaluated for UL or CBDiagonal display area 144 mm (5.7 in.)Height 638 mm (25.1 in.) plus constant headbracket 186 mm (7.3 in.)Width 271 mm (10.7 in.)Depth 182 mm (7.2 in.)Weight15 kg (33 lbs)Electronics enclosure 20% glass loaded polypropylene Main enclosure NorylLower tray 10% glass loaded polypropylene DoorAcrylicInlet 6 mm (1/4 in.) flexible hose connection Outlet9 mm (1/4 in.) flexible hose connectionNavigator 600 Silica Silica analyzerDS/NAV6S–EN Rev. J 7Environmental DataAmbient Operating Temperature 5 to 45 ºC (41 to 113 ºF)Sample Temperature 5 to 55 ºC (41 to 131 ºF)Sample Particulate <60 microns <10 mgl –1Sample Flow Rate>5 ml/min / <500 ml/min Sample Pressure AtmosphericStorage Temperature–20 to 75 ºC (–4 to 167 ºF)Ambient Operating Humidity Up to 95% RH non-condensingElectricalSupply ranges100 to 240 V max. AC 50/60 Hz ± 10 % (90 to 264 V AC, 45/65 Hz)18 to 36 V DC 10A power supply typical (optional)Power consumption 60 W max. – AC 100 W max. – DCAnalog OutputsSingle and multi-stream analyzers 6 isolated current outputs:⏹galvanically isolated (to 500 V dc) from each other and all other circuitry⏹fully assignable and programmable over a 0 to 20 mA range (up to 22 mA if required)⏹drives maximum 750 loadWetted MaterialsPMMA (acrylic)PP (polypropylene)PTFEPP (20% glass filled)PEEK NBR (nitrile)EPDM SantoprenePTFE (15% polysulphane)NORYLBorosilicate glass Acrylic adhesiveAlarms/Relay OutputsSingle and multi-stream instruments One per unit:⏹Out of service alarm relay ⏹Calibration in progress alarm relay ⏹Calibration failed alarm relay ⏹Maintenance/Hold alarm relaySix per unit:⏹fully user-assignable and alarm relaysRating Connectivity/CommunicationsEthernet connection Bus communicationsProfibus DP V1 (optional)Data Handling, Storage and DisplaySecurityStorageRemovable Secure Digital (SD) card – maximum size 2 GB Trend analysis Local and remote Data transfer SD card or FTPApprovals, Certification and SafetySafety Approval cULus – PendingCE MarkCovers EMC & LV Directives (including latest version EN 61010)General Safety EN 61010–1Overvoltage Class 11 on inputs and outputs Pollution category 2EMCEmissions & immunityMeets requirements of IEC61326 for an Industrial EnvironmentVoltage 250 V AC 30 V DC Current5 A AC 5 A DC Loading (non-inductive)1250 VA150 WWeb server with ftp:for real time monitoring, configuration, data file access and email capabilityMulti level security:user, configuration, calibration and maintenance pagesNavigator 600 Silica Silica analyzerOverall DimensionsNavigator 600 Silica8DS/NAV6S–EN Rev. JNavigator 600 SilicaSilica analyzerReagent bottles mounted on optional brackets (two bottles per bracket)DS/NAV6S–EN Rev. J9Navigator 600 Silica Silica analyzer10DS/NAV6S–EN Rev. JOrdering InformationSupplied with analyzer:Reagent and calibration containersSilica Analyzer AW641/XXXXXXXXRange0 ... 5000 ppb 5Number of Streams1 – Measuring 1 stream2 – Measuring 2 streams 4 – Measuring3 or4 streams 6 – Measuring5 or6 streams 1246CommunicationsNoneProfibus DP . V .101EnclosureStandardStandard + reagent shelvesStandard + reagent shelves + reagent sensors 012Power Supply100 ... 240 V AC 50/60 Hz 18 ... 36 V DC 01ReservedBuild 9ManualEnglish French Italian German Spanish 12345CertificationNoneCertificate of calibration cULus – pending012Navigator 600 Silica Silica analyzerDS/NAV6S–EN Rev. J 11Benefits summary⏹Lowest cost-of-ownership–up to 90 % lower reagent consumption than competitors' analyzers–labour-saving 5 minute annual maintenance and up to 6 months unattended operation⏹Easy to use–familiar Windows™ menu system –built-in context-sensitive help⏹Full communications–web- and ftp-enabled for easy data file access, remote viewing and configuration –optional Profibus DP V1.0⏹Fast, Accurate and Reliable–temperature-controlled reaction and measurement section for optimum response–automatic cleaning, calibration and zero deliver high accuracy measurements–extensive electronics, measurement andmaintenance diagnostics ensure high availability⏹Field upgradeable–from 2 to 4; 2 to 6 or 4 to 6 streams, each user-programmable from 0 to 5000 ppb⏹Compact size–638 mm (25.1 in.) H x 271 mm (10.7 in.) W x 182 mm (7.2 in.) D⏹Email facility–automatically email up to 6 recipients when user-selected events occur⏹Grab sample facility–for manual sampling⏹Multiple outputs and relays–6 current outputs, 4 device state and 6 user-programmable relays as standard⏹Archiving facility–SD data card for easy backup and programming⏹Auto-zero facility–true auto-zero compensates for sample color, turbidity and background silica in reagents⏹Instrument logs–alarm and audit logs for complete, secure recordsContact usD S /N A V 6S -E N R e v . J 10.2011ABB LimitedProcess Automation Oldends Lane StonehouseGloucestershire GL10 3TA UK Tel:+44 1453 826 661Fax:+44 1453 829 671ABB Inc.Process Automation 125 E. County Line Road Warminster PA 18974USA Tel:+1 215 674 6000Fax:+1 215 674 NoteWe reserve the right to make technical changes or modify the contents of this document without prior notice. With regard to purchase orders, the agreed particulars shall prevail. ABB does not accept any responsibility whatsoever for potential errors or possible lack of information in this document.We reserve all rights in this document and in thesubject matter and illustrations contained therein. Any reproduction, disclosure to third parties or utilization of its contents in whole or in parts – is forbidden without prior written consent of ABB.Copyright© 2011 ABB All rights reserved3KXA841601R1001Windows™, Microsoft™, MS-DOS™ and Internet Explorer™ are registered trademarks of Microsoft Corporation in the United States and / or other countries.PROFIBUS™ is a registered trademark of PROFIBUS corporation.。
colorimetric method
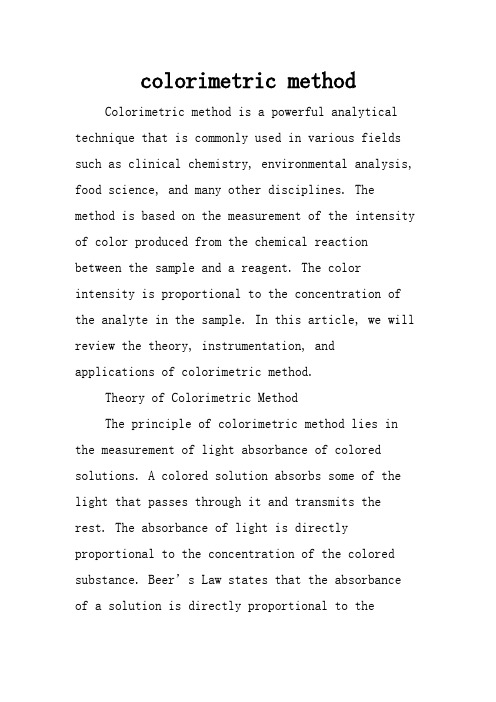
colorimetric methodColorimetric method is a powerful analytical technique that is commonly used in various fields such as clinical chemistry, environmental analysis, food science, and many other disciplines. The method is based on the measurement of the intensity of color produced from the chemical reaction between the sample and a reagent. The color intensity is proportional to the concentration of the analyte in the sample. In this article, we will review the theory, instrumentation, andapplications of colorimetric method.Theory of Colorimetric MethodThe principle of colorimetric method lies in the measurement of light absorbance of colored solutions. A colored solution absorbs some of the light that passes through it and transmits the rest. The absorbance of light is directly proportional to the concentration of the colored substance. Beer’s Law states that the absorbance of a solution is directly proportional to theconcentration of the absorbing substance and the path length of light passing through it.A = εbcWhere "A" is the absorbance, "ε" is the molar absorptivity (a constant dependent on the specific substance), "b" is the path length of the light through the sample, and "c" is the concentration of the substance.When a sample reacts with a reagent, a colored product is formed. The intensity of the color is proportional to the concentration of the analyte in the sample. The absorbance of the solution is measured at a specific wavelength with the help of a spectrophotometer. The spectrophotometer measures the ratio of the intensity of the incident light to that transmitted through the sample.Instrumentation of Colorimetric MethodThe colorimetric method requires a spectrophotometer for the measurement of absorbance. A spectrophotometer is a device that measures the intensity of light at a specific wavelength. The spectrophotometer consists of alight source, a monochromator, a sample holder, and a detector. The light source produces the light of a specific wavelength, which passes through a monochromator that isolates a particular wavelength. The sample is placed in a sample holder, and the detector measures the intensity of the transmitted light.The spectrophotometer measures absorbance by comparing the intensity of the incident light before and after passing through the sample. The absorbance is then used to calculate the concentration of the analyte in the sample. Most spectrophotometers come with pre-installed software that allows the user to store and analyze the data collected.Applications of Colorimetric MethodThe colorimetric method finds widespread applications in various fields. In clinical chemistry, the method is used to measure blood glucose, protein, and lipid levels. In environmental analysis, the method is used to measure the concentration of pollutants in air,water, and soil samples. The method is also used in the food industry to measure the concentration of vitamins, minerals, and food additives.The colorimetric method has also been used for the detection of biomolecules like DNA and proteins. Enzyme-linked immunosorbent assay (ELISA) is a colorimetric method widely used for the detection of antibodies and antigens. In ELISA, a colorimetric reaction occurs when an enzyme-labeled antigen or antibody binds to its complementary molecule. The intensity of the color produced is proportional to the concentration of the antigen or antibody in the sample.ConclusionColorimetric method is a simple yet powerful analytical technique that has a wide range of applications. The method involves the measurement of absorbance of colored solutions that are formed by the reaction of a sample with a reagent. The method requires a spectrophotometer for the measurement of the absorbance, which is used to calculate the concentration of the analyte in thesample. The colorimetric method is widely used in clinical chemistry, environmental analysis, food science, and many other fields. It has also been used for the detection of biomolecules like DNA and proteins.。
colormap
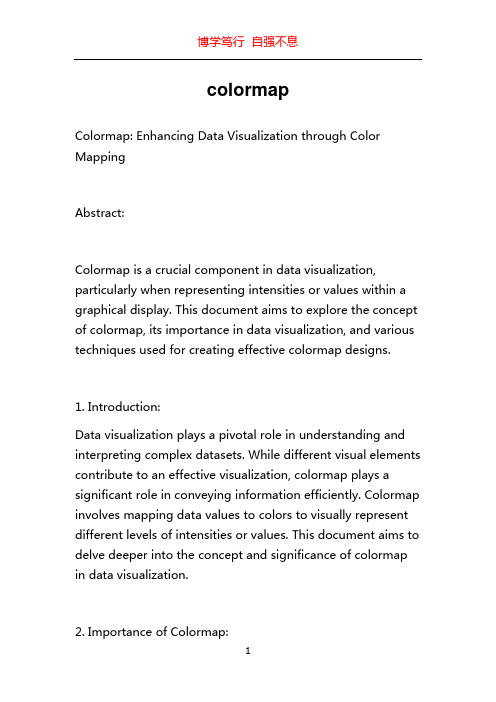
colormapColormap: Enhancing Data Visualization through Color MappingAbstract:Colormap is a crucial component in data visualization, particularly when representing intensities or values within a graphical display. This document aims to explore the concept of colormap, its importance in data visualization, and various techniques used for creating effective colormap designs.1. Introduction:Data visualization plays a pivotal role in understanding and interpreting complex datasets. While different visual elements contribute to an effective visualization, colormap plays a significant role in conveying information efficiently. Colormap involves mapping data values to colors to visually represent different levels of intensities or values. This document aims to delve deeper into the concept and significance of colormap in data visualization.2. Importance of Colormap:Colors have a profound impact on human perception, making colormap an essential element in data visualization. It enhances the readability and comprehensibility of graphical representations, making it easier for users to identify patterns, trends, and anomalies within the dataset. A well-designed colormap can effectively convey information, aid in data analysis, and support decision-making processes.3. Factors to Consider in Colormap Design:Developing an effective colormap requires careful consideration of several factors, including the data type, color selection, color map classification, and color perception. The document explores each of these factors in detail:3.1 Data Type: Different types of data require different colormap designs. For instance, sequential data requires a colormap with a gradual transition of colors, while diverging data demands a colormap that differentiates positive and negative values. Categorical data, on the other hand, needs discrete colors that can be easily distinguished.3.2 Color Selection: Appropriate selection of colors is crucial in colormap design. Colors should be visually appealing while maintaining a clear distinction between different data values. It is essential to consider colorblindness and universal colorassociations while choosing colors to ensure that the colormap is accessible to all users.3.3 Color Map Classification: Colormaps can be classified into two categories: qualitative and quantitative. Qualitative colormaps are based on categorical data and involve a set of distinct colors, while quantitative colormaps represent continuous data values through a smooth transition of colors.3.4 Color Perception: Understanding color perception is vital in design colormap effectively. Certain color combinations can lead to visual perception issues, such as color distortion or misinterpretation. Knowledge of color theory and perceptual phenomena can help avoid such issues.4. Techniques for Colormap Design:Several techniques and tools are available for creating effective colormaps. Some common techniques include:4.1 Sequential Colormaps: These colormaps consist of a smooth transition of colors often used for representing ordered data without any distinct partitions.4.2 Diverging Colormaps: These colormaps use two unique colors to represent positive and negative values, with a neutral color indicating the median or zero point.4.3 Categorical Colormaps: These colormaps are suited for representing discrete categories where distinct colors are assigned to different categories.4.4 Perceptually Uniform Colormaps: These colormaps take into account color perception phenomena and aim to maintain perceptual uniformity across the colormap.4.5 Custom Colormap Generation: Advanced techniques involve the design of custom colormaps based on specific data requirements and desired visualization outcomes.5. Guidelines for Colormap Usage:While creating a colormap is essential, utilizing it appropriately is equally important. The document provides guidelines for colormap usage, including:5.1 Ensuring clear and intuitive color mapping that aids in data interpretation.5.2 Avoiding excessive usage of colors that may lead to cluttered or confusing visual representations.5.3 Regularly testing colormap designs to assess their effectiveness and address potential issues.5.4 Considering the target audience and their specific requirements, such as accessibility or colorblindness.6. Conclusion:Colormap is a fundamental component of data visualization that significantly impacts the effectiveness of graphical representations. Designing an effective colormap involves considering various factors such as data type, color selection, and color perception. By following appropriate techniques and guidelines, data visualizers can create visually engaging and informative visualizations that help users make informed decisions and uncover valuable insights hidden within the data.References:[Include a list of the references used in creating the document]。
NEC MultiSync PA322UHD 32英寸专业显示器说明书
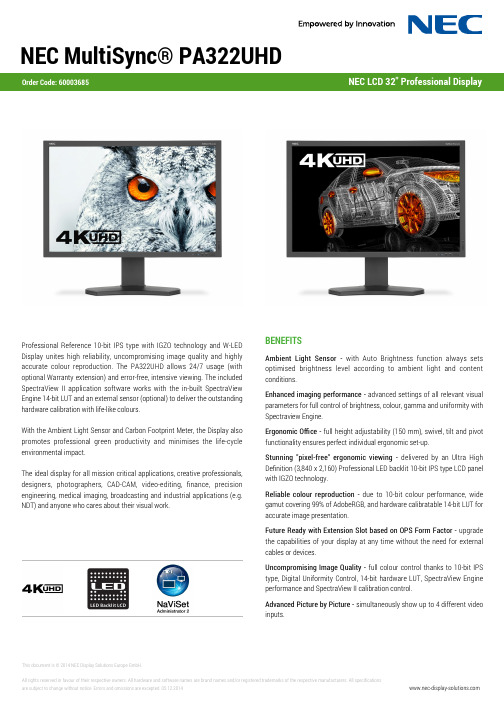
NEC MultiSync® PA322UHDProfessional Reference 10-bit IPS type with IGZO technology and W-LED Display unites high reliability, uncompromising image quality and highly accurate colour reproduction. The PA322UHD allows 24/7 usage (with optional Warranty extension) and error-free, intensive viewing. The included SpectraView II application software works with the in-built SpectraView Engine 14-bit LUT and an external sensor (optional) to deliver the outstanding hardware calibration with life-like colours.With the Ambient Light Sensor and Carbon Footprint Meter, the Display also promotes professional green productivity and minimises the life-cycle environmental impact.The ideal display for all mission critical applications, creative professionals, designers, photographers, CAD-CAM, video-editing, finance, precision engineering, medical imaging, broadcasting and industrial applications (e.g.NDT) and anyone who cares about their visual work.BENEFITSAmbient Light Sensor - with Auto Brightness function always sets optimised brightness level according to ambient light and content conditions.Enhanced imaging performance - advanced settings of all relevant visual parameters for full control of brightness, colour, gamma and uniformity with Spectraview Engine.Ergonomic Office - full height adjustability (150 mm), swivel, tilt and pivot functionality ensures perfect individual ergonomic set-up.Stunning "pixel-free" ergonomic viewing - delivered by an Ultra High Definition (3,840 x 2,160) Professional LED backlit 10-bit IPS type LCD panel with IGZO technology.Reliable colour reproduction - due to 10-bit colour performance, wide gamut covering 99% of AdobeRGB, and hardware calibratable 14-bit LUT for accurate image presentation.Future Ready with Extension Slot based on OPS Form Factor - upgrade the capabilities of your display at any time without the need for external cables or devices.Uncompromising Image Quality - full colour control thanks to 10-bit IPS type, Digital Uniformity Control, 14-bit hardware LUT, SpectraView Engine performance and SpectraView II calibration control.Advanced Picture by Picture - simultaneously show up to 4 different video inputs.This document is © 2014 NEC Display Solutions Europe GmbH.All rights reserved in favour of their respective owners. All hardware and software names are brand names and/or registered trademarks of the respective manufacturers. All specificationsare subject to change without notice. Errors and omissions are excepted. 。
- 1、下载文档前请自行甄别文档内容的完整性,平台不提供额外的编辑、内容补充、找答案等附加服务。
- 2、"仅部分预览"的文档,不可在线预览部分如存在完整性等问题,可反馈申请退款(可完整预览的文档不适用该条件!)。
- 3、如文档侵犯您的权益,请联系客服反馈,我们会尽快为您处理(人工客服工作时间:9:00-18:30)。
li g h t n
e a e
e s s
15 l
e e n
a T e s s
a
ble l t ha n
e
,
t ia l g
mut r n
o
lg
5 Pr it h m 1
P
o s e
d
t he
Pr
e
P
o s e
d
lg
,
o r
it h m
d th
g
a e s
e o r r e s e
P
o n
d
s e o r e s e o e
o r
it h m
n
,
a e e o r
t h r e e t v D i e a l s D a t ia l g a m l i t d i n g t o t h e C I E g u i d e li n e s [ 1 3 ]
o
O F
be
s
r
t he
o rl o n
t
im
a
i n d iv i d a g e 6 im
,
a
、
g
e o r r e
l a t io
r
n
e x
is t s E
-
b e t w e e r l t li e P e r e e l l t a g e s a n d t l z e 之 a lg o r ith T li e a m f e t t h a t th e p r in u a l a lg o r i t h 、 a r e r e n t 1 5 th e m dif e
.
s e o r e s
一
.
名一
Se
o r e
f SG CK
0 00
,
55
0 79
,
0 82
.
46
1 16
.
1 36
.
0 87
.
之一
Se
o r e
o
f A SCG
MA
( ) 86
0 48
1 4 1
0 73
1 44
一
0 67
0 34
0 86
T h
a
e
r e s li
lt e d
a
z
一
吕e o r e s
,
a xi a r e
m i k d y 1 3 8 9 7 0
g 1 5 0 3
3 1 0
n h w
i l
1 g m
d p
n m r f
l t g i d
i d
p m
d i
m e n i l
i p
2 [ ) p s c t
M L s d n ]
香 鑫
M P H i g t l E D N I i w m u b h t i 只 t p m h f y d n m i 1 仁 u d p h t 哪 l d p g n i t u P g n h i w t u m a g n l t 拍 i m f b 触 d t w n k i h T h t v n w 爪 g l f d s o D e d 矶 云 m h t i a b h t v n i d 1 u u r a m h t p k d g d o l 1 i t p e h 1 “ m g e f i d l p h } t w n T d e h t f m t g h l n i n m h t i b l d o p l x m n i t u n i t p y l u h n t l w H 日 币 月 i f p i d h t 士 n 1 t g n i l 介 1 n m i g t d b p h d e i f n d g n m i t h t 旦 n i n u 即 m 1 l P p t n i b m h t d C G f n 1 t n i m h ( p S U u n t S h T p d a m u m K C G g i l t d m i n t h P e f h n i t d i p m b t l u q i d p g n h f y d n t K W y m h t i g w l b w n I e f i d W i d t 1 / 4 9 1 7 6 / 9 0 2 5 0 3 7 8 9 0 2 ④ O i h C L i t p
th
)
s
.
[` 5 ]
te
a
t ll e
re s u a n
lt
a lg o r i t li s a n d m s ( Z o l li k e r K o l 邑 r in d ie a t e d t l, a t S G C K p e r f o me d o rs e
,
,
t
m
r
o s
d ily
a n
.
3
e
.
T h i s 15 b
,
th
a
e
t t he
e
e o
lo
r 、
in in
ia
g
e
2
d
,
e s
Pe
o
i a ll y
e r s
.
in
g
e
6
t a n v
o
g
a
d dr
a
wb
ks
f HP
w i l
M IN D E
ie
mm
e n
d d b y C IE b
PP in g
a
e
a s e a
d
.
7
8
C
I
O
S I C 6PI
S
J
H R N E
N / T OE IS 7 V
,
o
g
p t T T E /E S
e
e n l
b
e r
10
2 00 9
F 19
t h
e
2 ii
li
名一 S C O T e S
叮 HP MIN D E
o
,
SG CK
,
a n
d A SC G
o MA f
r
l l a
F ig
一
e o r re
P la y
e n
C
ir
a n n o n c o
in
se
te r
a
Th
c a rr
e
P y h
o u s
.
Phy
s ie a
l
im
e
t
f P
mP
a
a r e
iS O n 15
o
ie d
t
by
a n e
l
o
f 10
f th
re e
lg
o r
it h
n z、
;
e s
Pe
e
i a l ly
l, i a
P Pin g
s
a
,
lg
a
o
-
a n
e
-
ith l n
a
15
e s
,
s ll l n a n
m
a r a
iz
,
e (l, a e
to ge t h e
w it h t h e
P r i xi e ip le
a , zd
d
a
-
S G CK
e a u s e a n
g
e
Z
,
i ll u
s
t
d in F ig
m i g l t f y p h T
d m t L E I C i y p h
y e v t g d H C i p l
l i a P b p f t
t i
m g p
1 p l t m i
r a h g d i t l
l a m h t
n a b d m p h t
r a p m I h y t i g l d
geth
t t hr
e r a
w it h t h
o
e o r r e
la t io
n
o f 伍e ie
a n
h
te s
t im
g
e
t
o
r
-
m
t
o
e n
d d by C IE
e s
s e v e n
a lg o r i t h i n s d th e t ty p l d ig it a l im a g e s a r e
m
亡 七 S
b L y e ( l
i X f 仃 亡
Z u o a P O n r d M
张 ( g n a h 劲 l j 七
n ) 斗 显 m 亡
s r e v
( u X n U g j n c 8 0
) 松 海 徐 I 于 , y t j
1 3 力 Z g
f C h 不 2 0
m E i R
C h l f m D d
d
e o n
i d f
e n e e
i工z t
e r v a
.
ls f o
r
a
11 ll i工 s