Bootstrap Approach for Estimating Seemingly Unrelated Regressions with Varying Degrees
关于bootstrap的思想的讨论

关于bootstrap的思想的讨论1楼rtist看了/view.php?tid=48&id=86之后想留言,可是那里不让留了,就贴在这儿了。
bootstrap的思想并不是再抽样,而是plug-in principle;再抽样仅仅是实现这个思想的一种手段。
所以bootstrap也并不一定非要抽样B次,只要可以plug-in,有时候一次也不用抽就可以得到精确结果。
但是不可否认,样本的重复利用的确是一个incredibly amazing的方法,而且经常counter-intuitive。
最难理解的(对我来说)就是,信息都蕴含在样本中,再抽样究竟能提不提供样本之外的新的信息;如果不能,怎么抽得到的都是原来样本里面的信息;如果能,新信息从哪儿来?2楼keynesI think the information contained in a sample is not always fully exploited by a particular statistic. That is, a statistic typically only uses part of information in the data and the principle of data-reduction is one of such examples. To use bootstrap (maybe other resampling methods as well), we have to impose some extra assumptions, such like the sample (the data at hand, so to speak) is representative and informative of the population so that we can treat the former as the latter.Not quite clear what do you mean by the statement that the bootstrap is mainly a plug-in method. Would you make it more clear and detailed? Thanks.3楼rtistI don't think so, as bootstrap often works on complete sufficient statistics too. So it's not that the original statistic always loses information that allows bootstrap to exploit further.It's still counter-intuitive to me. My current understanding (subject to change in the future) is that we often make reasonable assumptions that give us the extra-information, so that bootstrap often works by using the information in the assumptions.For the plug-in principle, see Efron's review: /view/08834237/sp040007/04x0072t/04楼keynesWell, "sufficient" just means that the statistic contain enough information for the purpose of point estimation of the parameter of interest. It does not say that all information is used up. Neither it prevents from exploiting other information contained in the sample.5楼rtistThis is also point that I was thinking of when I was writing the last post.It sounds reasonable, but it didn't completely persuade me to believe it at the timeas I could not figure out just what is the "other" information. Are we treating the sd ofsome statistic as a new parameter to estimate? Probably.6楼statax我也一直觉得这个问题很玄乎。
No1【方法学导读】SPSS和SAS在简单中介模型中间接效应估计中的应用(中文译校)

推荐序中介分析的初衷在于揭示变量间作用的“机制”,最简单的完全中介模型就是包含一个中介变量的模型,即X→M→Y。
在中国知网平台《心理学报》发表论文的初步内容分析发现,2000年以来涉及“中介”分析的论文发表数量呈缓慢增长趋势,特别是,2016年以来每年约10篇左右的论文涉及到“中介”分析。
在众多探讨中介方法的中文文献中,尤其以温忠麟、侯杰泰、张雷老师合作发表的《中介效应检验程序及其应用》、《调节效应与中介效应的比较和应用》两篇文章最为引人注目,引用率高达8000余次,对于推动国内心理学界更多、更正式地使用中介分析做出了有目共睹的贡献。
这种趋势还是继续。
国内有限的几本发表实证研究的期刊,再也很难看到没有中介调节分析而能发表的非实验类、非综述类的文章了。
据初步估计,本校心理学本科生的论文中70%左右有用到中介分析,而心理学硕士学位论文使用中介分析的比例几乎高达90%,但用错、报告错、得出奇怪结论的情况总是难免,但研究者有时不自知、他人也不容易发现。
这当中,所引用的外文文献都比较一致地指向2004年发表于的一篇文章:《SPSS and SAS procedures for estimating indirect effects in simple mediation models》,引用率已突破6000次,这其中可能还不包括规模庞大的中文期刊论文以及中国学士以上学位论文引用的人数。
个人推测,这篇文章最受欢迎之处在于它提供了傻瓜式的宏。
然而,真正阅读英文原文的本科生和研究生人数可能并不乐观,老师们也未必能有雅兴拜读这篇原文。
本次推介的目的,反而并不是要介绍“宏”,而是尽量准确地翻译作者开发宏背后的“理”;误差也是难免的,请大家指正,是为序。
SPSS和SAS宏程序在简单中介模型的间接效应估计中的应用KRISTOPHER J. PREACHER北卡罗来纳大学ANDREW F . HAYES俄亥俄州立大学摘要:中介分析(mediation analysis)常用来间接评估假设的某个原因是否通过中介变量(mediator)对结果产生影响。
健康预期寿命的测量指标与测算方法的应用及比较
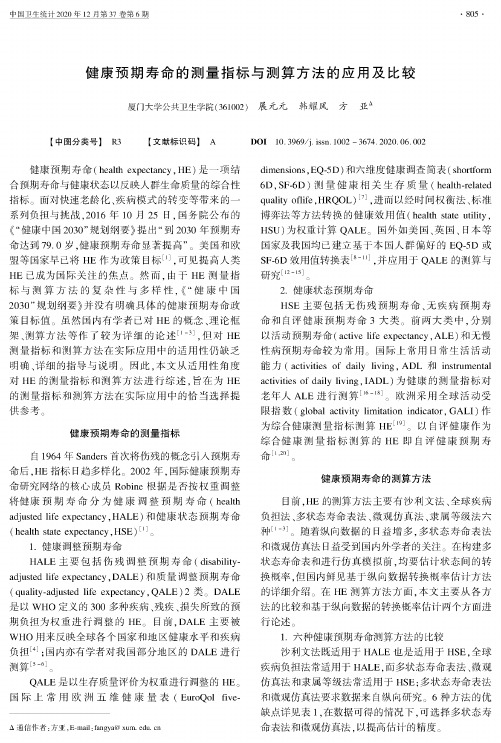
健康预期寿命的测量指标与测算方法的应用及比较厦门大学公共卫生学院(361002)展元元韩耀风方亚*【中图分类号】R3 【文献标识码】A DOI 10. 3969/j.issn. 1002 -3674. 2020.06.002健康预期寿命(health expectancy , HE )是一项结 合预期寿命与健康状态以反映人群生命质量的综合性 指标。
面对快速老龄化、疾病模式的转变等带来的一系列负担与挑战,2016年10月25日,国务院公布的《“健康中国2030”规划纲要》提出“到2030年预期寿 命达到79.0岁,健康预期寿命显著提高”。
美国和欧盟等国家早已将HE 作为政策目标[1],可见提高人类HE 已成为国际关注的焦点。
然而,由于HE 测量指 标与测算方法的复杂性与多样性,《“健康中国2030”规划纲要》并没有明确具体的健康预期寿命政策目标值。
虽然国内有学者已对HE 的概念、理论框 架、测算方法等作了较为详细的论述[1-3],但对HE测量指标和测算方法在实际应用中的适用性仍缺乏 明确、详细的指导与说明。
因此,本文从适用性角度 对HE 的测量指标和测算方法进行综述,旨在为HE 的测量指标和测算方法在实际应用中的恰当选择提供参考。
△通信作者:方亚,E-mail : fangya@ xum. edu. cn健康预期寿命的测量指标自1964年Sanders 首次将伤残的概念引入预期寿命后,HE 指标日趋多样化。
2002年,国际健康预期寿命研究网络的核心成员Robine 根据是否按权重调整将健康预期寿命分为健康调整预期寿命(health adjusted life expectancy ,HALE )和健康状态预期寿命(health state expectancy , HSE )[1] o1.健康调整预期寿命HALE 主要包括伤残调整预期寿命(disability adj u sted life expectancy ,DALE )和质量调整预期寿命(quality-adjusted life expectancy , QALE ) 2 类。
[转载]k-折交叉验证(K-fold
![[转载]k-折交叉验证(K-fold](https://img.taocdn.com/s3/m/e3435e6b178884868762caaedd3383c4bb4cb43c.png)
[转载]k-折交叉验证(K-fold cross-validation)原⽂地址:k-折交叉验证(K-fold cross-validation)作者:清风⼩荷塘k-折交叉验证(K-fold cross-validation)是指将样本集分为k份,其中k-1份作为训练数据集,⽽另外的1份作为验证数据集。
⽤验证集来验证所得分类器或者回归的错误码率。
⼀般需要循环k次,直到所有k份数据全部被选择⼀遍为⽌。
Cross validation is a model evaluation method that is better than residuals. The problem with residual evaluations is that they do not give an indication of how well the learner will do when it is asked to make new predictions for data it has not already seen. One way to overcome this problem is to not use the entire data set when training a learner. Some of the data is removed before training begins. Then when training is done, the data that was removed can be used to test the performance of the learned model on ``new'' data. This is the basic idea for a whole class of model evaluation methods called cross validation.The holdout method is the simplest kind of cross validation. The data set is separated into two sets, called the training set and the testing set. The function approximator fits a function using the training set only. Then the function approximator is asked to predict the output values for the data in the testing set (it has never seen these output values before). The errors it makes are accumulated as before to give the mean absolute test set error, which is used to evaluate the model. The advantage of this method is that it is usually preferable to the residual method and takes no longer to compute. However, its evaluation can have a high variance. The evaluation may depend heavily on which data points end up in the training set and which end up in the test set, and thus the evaluation may be significantly different depending on how the division is made.K-fold cross validation is one way to improve over the holdout method. The data set is divided into k subsets, and the holdout method is repeated k times. Each time, one of the k subsets is used as the test set and the other k-1 subsets are put together to form a training set. Then the average error across all k trials is computed. The advantage of this method is that it matters less how the data gets divided. Every data point gets to be in a test set exactly once, and gets to be in a training set k-1 times. The variance of the resulting estimate is reduced as k is increased. The disadvantage of this method is that the training algorithm has to be rerun from scratch k times, which means it takes k times as much computation to make an evaluation. A variant of this method is to randomly divide the data into a test and training set k different times. The advantage of doing this is that you can independently choose how large each test set is and how many trials you average over.Leave-one-out cross validation is K-fold cross validation taken to its logical extreme, with K equal to N, the number of da ta points in the set. That means that N separate times, the function approximator is trained on all the data except for one point and a prediction is made for that point. As before the average error is computed and used to evaluate the model. The evaluation given by leave-one-out cross validation error (LOO-XVE) is good, but at first pass it seems very expensive to compute. Fortunately, locally weighted learners can make LOO predictions just as easily as they make regular predictions. That means computing the LOO-XVE takes no more time than computing the residual error and it is a much better way to evaluate models. We will see shortly that Vizier relies heavily on LOO-XVE to choose its metacodes.Figure 26: Cross validation checks how well a model generalizes to new dataFig. 26 shows an example of cross validation performing better than residual error. The data set in the top two graphs is a simple underlying function with significant noise. Cross validation tells us that broad smoothing is best. The data set in the bottom two graphs is a complex underlying function with no noise. Cross validation tells us that very little smoothing is best for this data set.Now we return to the question of choosing a good metacode for data set a1.mbl:File -> Open -> a1.mblEdit -> Metacode -> A90:9Model -> LOOPredictEdit -> Metacode -> L90:9Model -> LOOPredictEdit -> Metacode -> L10:9Model -> LOOPredictLOOPredict goes through the entire data set and makes LOO predictions for each point. At the bottom of the page itshows the summary statistics including Mean LOO error, RMS LOO error, and information about the data point with the largest error. The mean absolute LOO-XVEs for the three metacodes given above (the same three used to generate the graphs in fig. 25), are 2.98, 1.23, and 1.80. Those values show that global linear regression is the best metacode of those three, which agrees with our intuitive feeling from looking at the plots in fig. 25. If you repeat the above operation on data set b1.mbl you'll get the values 4.83, 4.45, and 0.39, which also agrees with our observations.What are cross-validation and bootstrapping?--------------------------------------------------------------------------------Cross-validation and bootstrapping are both methods for estimatinggeneralization error based on "resampling" (Weiss and Kulikowski 1991; Efronand Tibshirani 1993; Hjorth 1994; Plutowski, Sakata, and White 1994; Shaoand Tu 1995). The resulting estimates of generalization error are often usedfor choosing among various models, such as different network architectures.Cross-validation++++++++++++++++In k-fold cross-validation, you divide the data into k subsets of(approximately) equal size. You train the net k times, each time leavingout one of the subsets from training, but using only the omitted subset tocompute whatever error criterion interests you. If k equals the samplesize, this is called "leave-one-out" cross-validation. "Leave-v-out" is amore elaborate and expensive version of cross-validation that involvesleaving out all possible subsets of v cases.Note that cross-validation is quite different from the "split-sample" or"hold-out" method that is commonly used for early stopping in NNs. In thesplit-sample method, only a single subset (the validation set) is used toestimate the generalization error, instead of k different subsets; i.e.,there is no "crossing". While various people have suggested thatcross-validation be applied to early stopping, the proper way of doing so isnot obvious.The distinction between cross-validation and split-sample validation isextremely important because cross-validation is markedly superior for smalldata sets; this fact is demonstrated dramatically by Goutte (1997) in areply to Zhu and Rohwer (1996). For an insightful discussion of thelimitations of cross-validatory choice among several learning methods, seeStone (1977).Jackknifing+++++++++++Leave-one-out cross-validation is also easily confused with jackknifing.Both involve omitting each training case in turn and retraining the networkon the remaining subset. But cross-validation is used to estimategeneralization error, while the jackknife is used to estimate the bias of astatistic. In the jackknife, you compute some statistic of interest in eachsubset of the data. The average of these subset statistics is compared withthe corresponding statistic computed from the entire sample in order toestimate the bias of the latter. You can also get a jackknife estimate ofthe standard error of a statistic. Jackknifing can be used to estimate thebias of the training error and hence to estimate the generalization error,but this process is more complicated than leave-one-out cross-validation(Efron, 1982; Ripley, 1996, p. 73).Choice of cross-validation method+++++++++++++++++++++++++++++++++Cross-validation can be used simply to estimate the generalization error of a given model, or it can be used for model selection by choosing one of several models that has the smallest estimated generalization error. For example, you might use cross-validation to choose the number of hidden units, or you could use cross-alidation to choose a subset of the inputs (subset selection). A subset that contains all relevant inputs will be called a "good" subsets, while the subset that contains all relevant inputs but no others will be called the "best" subset. Note that subsets are "good" and "best" in an asymptotic sense (as the number of training cases goes to infinity). With a small training set, it is possible that a subset that is smaller than the "best" subset may provide better generalization error.Leave-one-out cross-validation often works well for estimating generalization error for continuous error functions such as the mean squared error, but it may perform poorly for discontinuous error functions such as the number of misclassified cases. In the latter case, k-fold cross-validation is preferred. But if k gets too small, the error estimate is pessimistically biased because of the difference in training-set size between the full-sample analysis and the cross-validation analyses. (For model-selection purposes, this bias can actually help; see the discussion below of Shao, 1993.) A value of 10 for k is popular for estimating generalization error.Leave-one-out cross-validation can also run into trouble with variousmodel-selection methods. Again, one problem is lack of continuity--a smallchange in the data can cause a large change in the model selected (Breiman,1996). For choosing subsets of inputs in linear regression, Breiman andSpector (1992) found 10-fold and 5-fold cross-validation to work better thanleave-one-out. Kohavi (1995) also obtained good results for 10-foldcross-validation with empirical decision trees (C4.5). Values of k as smallas 5 or even 2 may work even better if you analyze several different randomk-way splits of the data to reduce the variability of the cross-validationestimate.Leave-one-out cross-validation also has more subtle deficiencies for modelselection. Shao (1995) showed that in linear models, leave-one-outcross-validation is asymptotically equivalent to AIC (and Mallows' C_p), butleave-v-out cross-validation is asymptotically equivalent to Schwarz's Bayesian criterion (called SBC or BIC) when v =n[1-1/(log(n)-1)], where n is the number of training cases. SBCprovides consistent subset-selection, while AIC does not. That is, SBC will choose the "best" subset with probability approaching one as the size of the training set goes to infinity. AIC has an asymptotic probability of one of choosing a "good" subset, but less than one of choosing the "best" subset (Stone, 1979). Many simulation studies have also found that AIC overfits badly in small samples, and that SBC works well (e.g., Hurvich and Tsai, 1989; Shao and Tu, 1995). Hence, these results suggest that leave-one-out cross-validation should overfit in small samples, but leave-v-outcross-validation with appropriate v should do better. However, when true models have an infinite number of parameters, SBC is not efficient, and other criteria that are asymptotically efficient but not consistent formodel selection may produce better generalization (Hurvich and Tsai, 1989). Shao (1993) obtained the surprising result that for selecting subsets of inputs in a linear regression, the probability of selecting the "best" doesnot converge to 1 (as the sample size n goes to infinity) for leave-v-out cross-validation unless the proportion v/n approaches 1. At first glance, Shao's result seems inconsistent with the analysis by Kearns (1997) ofsplit-sample validation, which shows that the best generalization is obtained with v/n strictly between 0 and 1, with little sensitivity to the precise value of v/n for large data sets. But the apparent conflict is dueto the fundamentally different properties of cross-validation andsplit-sample validation.To obtain an intuitive understanding of Shao (1993), let's review some background material on generalization error. Generalization error can be broken down into three additive parts, noise variance + estimation variance + squared estimation bias. Noise variance is the same for all subsets of inputs. Bias is nonzero for subsets that are not "good", but it's zero forall "good" subsets, since we are assuming that the function to be learned is linear. Hence the generalization error of "good" subsets will differ only in the estimation variance. The estimation variance is (2p/t)s^2 where pis the number of inputs in the subset, t is the training set size, and s^2is the noise variance. The "best" subset is better than other "good" subsetsonly because the "best" subset has (by definition) the smallest value of p. But the t in the denominator means that differences in generalization error among the "good" subsets will all go to zero as t goes to infinity.Therefore it is difficult to guess which subset is "best" based on the generalization error even when t is very large. It is well known that unbiased estimates of the generalization error, such as those based on AIC, FPE, and C_p, do not produce consistent estimates of the "best" subset (e.g., see Stone, 1979).In leave-v-out cross-validation, t=n-v. The differences of thecross-validation estimates of generalization error among the "good" subsets contain a factor 1/t, not 1/n. Therefore by making t small enough (and thereby making each regression based on t cases bad enough), we can make the differences of the cross-validation estimates large enough to detect. It turns out that to make t small enough to guess the "best" subset consistently, we have to have t/n go to 0 as n goes to infinity.The crucial distinction between cross-validation and split-sample validation is that with cross-validation, after guessing the "best" subset, we trainthe linear regression model for that subset using all n cases, but withsplit-sample validation, only t cases are ever used for training. If ourmain purpose were really to choose the "best" subset, I suspect we would still have to have t/n go to 0 even for split-sample validation. Butchoosing the "best" subset is not the same thing as getting the best generalization. If we are more interested in getting good generalizationthan in choosing the "best" subset, we do not want to make our regression estimate based on only t cases as bad as we do in cross-validation, because in split-sample validation that bad regression estimate is what we're stuck with. So there is no conflict between Shao and Kearns, but there is a conflict between the two goals of choosing the "best" subset and getting the best generalization in split-sample validation.Bootstrapping+++++++++++++Bootstrapping seems to work better than cross-validation in many cases (Efron, 1983). In the simplest form of bootstrapping, instead of repeatedly analyzing subsets of the data, you repeatedly analyze subsamples of the data. Each subsample is a random sample with replacement from the fullsample. Depending on what you want to do, anywhere from 50 to 2000 subsamples might be used. There are many more sophisticated bootstrap methods that can be used not only for estimating generalization error butalso for estimating confidence bounds for network outputs (Efron and Tibshirani 1993). For estimating generalization error in classification problems, the .632+ bootstrap (an improvement on the popular .632 bootstrap) is one of the currently favored methods that has the advantage of performing well even when there is severe overfitting. Use of bootstrapping for NNs is described in Baxt and White (1995), Tibshirani (1996), and Masters (1995). However, the results obtained so far are not very thorough, and it is known that bootstrapping does not work well for some other methodologies such as empirical decision trees (Breiman, Friedman, Olshen, and Stone, 1984; Kohavi, 1995), for which it can be excessively optimistic.For further information+++++++++++++++++++++++Cross-validation and bootstrapping become considerably more complicated for time series data; see Hjorth (1994) and Snijders (1988).More information on jackknife and bootstrap confidence intervals is available at ftp:///pub/neural/jackboot.sas (this is a plain-text file).References:Baxt, W.G. and White, H. (1995) "Bootstrapping confidence intervals forclinical input variable effects in a network trained to identify thepresence of acute myocardial infarction", Neural Computation, 7, 624-638. Breiman, L., and Spector, P. (1992), "Submodel selection and evaluationin regression: The X-random case," International Statistical Review, 60,291-319.Dijkstra, T.K., ed. (1988), On Model Uncertainty and Its StatisticalImplications, Proceedings of a workshop held in Groningen, TheNetherlands, September 25-26, 1986, Berlin: Springer-Verlag.Efron, B. (1982) The Jackknife, the Bootstrap and Other ResamplingPlans, Philadelphia: SIAM.Efron, B. (1983), "Estimating the error rate of a prediction rule:Improvement on cross-validation," J. of the American StatisticalAssociation, 78, 316-331.Efron, B. and Tibshirani, R.J. (1993), An Introduction to the Bootstrap,London: Chapman & Hall.Efron, B. and Tibshirani, R.J. (1997), "Improvements on cross-validation: The .632+ bootstrap method," J. of the American Statistical Association, 92, 548-560.Goutte, C. (1997), "Note on free lunches and cross-validation," NeuralComputation, 9, 1211-1215,ftp://eivind.imm.dtu.dk/dist/1997/goutte.nflcv.ps.gz.Hjorth, J.S.U. (1994), Computer Intensive Statistical Methods Validation, Model Selection, and Bootstrap, London: Chapman & Hall.Hurvich, C.M., and Tsai, C.-L. (1989), "Regression and time series model selection in small samples," Biometrika, 76, 297-307.Kearns, M. (1997), "A bound on the error of cross validation using theapproximation and estimation rates, with consequences for thetraining-test split," Neural Computation, 9, 1143-1161.Kohavi, R. (1995), "A study of cross-validation and bootstrap foraccuracy estimation and model selection," International Joint Conference on Artificial Intelligence (IJCAI), pp. ?,/users/ronnyk/Masters, T. (1995) Advanced Algorithms for Neural Networks: A C++Sourcebook, NY: John Wiley and Sons, ISBN 0-471-10588-0Plutowski, M., Sakata, S., and White, H. (1994), "Cross-validationestimates IMSE," in Cowan, J.D., Tesauro, G., and Alspector, J. (eds.)Advances in Neural Information Processing Systems 6, San Mateo, CA: Morgan Kaufman, pp. 391-398.Ripley, B.D. (1996) Pattern Recognition and Neural Networks, Cambridge: Cambridge University Press.Shao, J. (1993), "Linear model selection by cross-validation," J. of theAmerican Statistical Association, 88, 486-494.Shao, J. (1995), "An asymptotic theory for linear model selection,"Statistica Sinica ?.Shao, J. and Tu, D. (1995), The Jackknife and Bootstrap, New York:Springer-Verlag.Snijders, T.A.B. (1988), "On cross-validation for predictor evaluation intime series," in Dijkstra (1988), pp. 56-69.Stone, M. (1977), "Asymptotics for and against cross-validation,"Biometrika, 64, 29-35.Stone, M. (1979), "Comments on model selection criteria of Akaike and Schwarz," J. of the Royal Statistical Society, Series B, 41, 276-278.Tibshirani, R. (1996), "A comparison of some error estimates for neural network models," Neural Computation, 8, 152-163.Weiss, S.M. and Kulikowski, C.A. (1991), Computer Systems That Learn, Morgan Kaufmann.Zhu, H., and Rohwer, R. (1996), "No free lunch for cross-validation,"Neural Computation, 8, 1421-1426.。
bootstrap置信区间公式
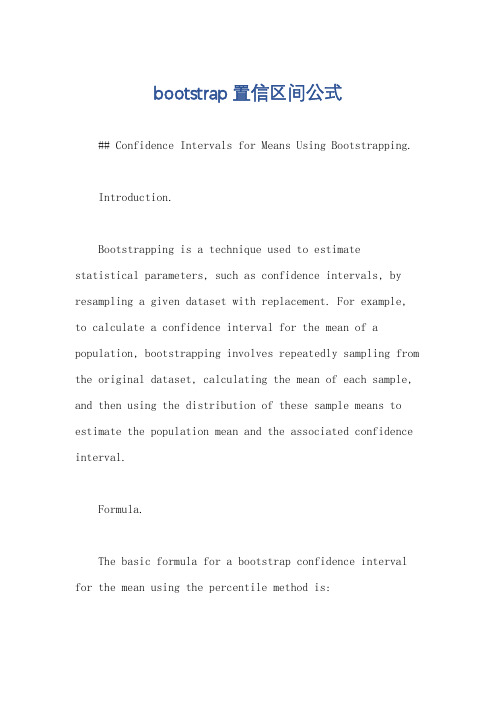
bootstrap置信区间公式## Confidence Intervals for Means Using Bootstrapping.Introduction.Bootstrapping is a technique used to estimatestatistical parameters, such as confidence intervals, by resampling a given dataset with replacement. For example, to calculate a confidence interval for the mean of a population, bootstrapping involves repeatedly sampling from the original dataset, calculating the mean of each sample, and then using the distribution of these sample means to estimate the population mean and the associated confidence interval.Formula.The basic formula for a bootstrap confidence interval for the mean using the percentile method is:CI = (L, U)。
where:L is the lower bound of the confidence interval, which is the _p_th percentile of the sample means, where _p_ is the desired level of confidence.U is the upper bound of the confidence interval, which is the _q_th percentile of the sample means, where _q_ is 1 _p_.For example, if we want a 95% confidence interval, then _p_ = 0.025 and _q_ = 0.975.Steps.The steps for calculating a bootstrap confidence interval for the mean are as follows:1. Resample: Draw B bootstrap samples of size n from the original dataset with replacement.2. Calculate: Compute the mean for each bootstrap sample.3. Percentile: Determine the lower and upper bounds of the confidence interval by finding the _p_th and _q_th percentiles of the sample means.4. Confidence Interval: The interval (L, U) is the bootstrap confidence interval for the mean.Advantages.Bootstrapping has several advantages over traditional methods for estimating confidence intervals, such as:It is non-parametric, so it does not require assumptions about the distribution of the data.It can be used with small sample sizes.It is computationally efficient and easy to implement.Limitations.However, bootstrapping also has some limitations:It can be biased for certain types of data or if the sample size is too small.It can be computationally intensive for large datasets.It may not be accurate if the data is notrepresentative of the population.## 置信区间公式。
基于Bootstrap方法数据包络分析的回归分析_全林(1)
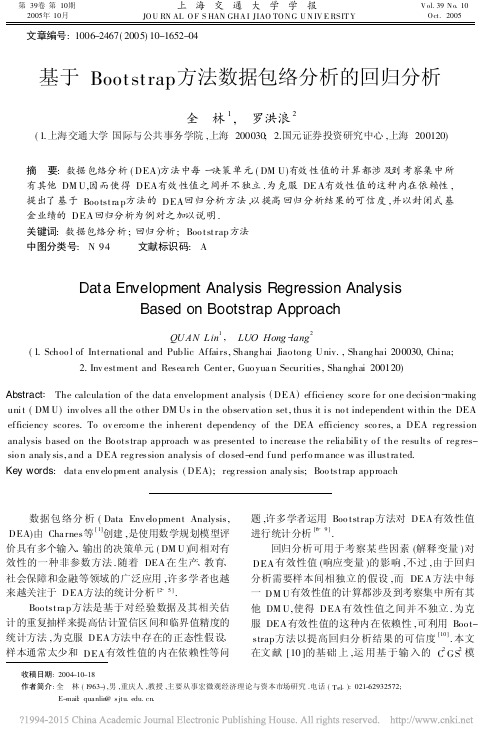
θ j = U 0+ U 1 F1j + U 2 F2j + … + U q Fqj Fs 的回归系数 ; j = 1, 2, … , n .
( 2)
式中 : U 0 为回归截距 ; U s ( s = 1, 2, … , q )为解释变量 ( 2) 利用配对 Boot strap 方法 , 有放回地从原样 本 ( z 1 , z 2 ,… , z n )中随机抽取 c (常数 )个 n 维样本 , 产 生所谓的 Bo ot st rap 样本 Sk : Sk = ( z k 1 , zk 2 , … , z kn ) k = 1, 2, … , c zk j = ( ukj , v kj ) ( 3) 对每一 Boo tst rap 样本 Sk , 运行 C GS 模 型 , 重新计算 所有 n 个 DM U 的 有效性 值 θ k1 , θ k2 , … ,θ kn . ( 4) 在每一 Boo tst rap 样本 Sk 内 , 拟合模型 :
2 ห้องสมุดไป่ตู้实 例
以 2000 年前上市的 20 只封闭式基金 2000 年 ( 1) 业绩为考察对象 (即 n = 20) , 所采用的输入指标为 管 理 费 用 ( M FP U)、 交 易成 本 ( T CPU ) 和标 准 差 ( SD ) , 输出指标则 为基金收益率 . 其中 , M F PU (不 包括基金经理业绩报酬 )、 T CPU (用年佣金总计代 替 , 即显性交易成本 )均折算为每单位基 金的数值 (单位为元 ) , SD指的是评价期内基金周收益率的标 准差 , 基金周收益率计算公式为 Rit = ln N AVit + Dit N AVi , t - 1 ( 5)
bootstrap法

bootstrap法Bootstrap法是一种常用的统计学方法,它可以用来评估统计学中的参数估计和假设检验的准确性。
Bootstrap法最初由布拉德利·埃夫隆和皮特·哈尔在1979年提出,并在之后的几十年里得到了广泛的应用。
本文将介绍Bootstrap法的基本原理、应用场景以及实现方法。
一、Bootstrap法的原理Bootstrap法的基本思想是通过从样本中重复抽取数据来估计统计量的分布。
具体而言,Bootstrap法包括以下步骤:1. 从原始数据样本中随机抽取一个固定数量的样本(通常与原始样本大小相同),并将其作为一个新的样本。
2. 重复步骤1多次,通常是1000次或更多次。
3. 对每个新样本计算统计量(如均值、方差、中位数等)。
4. 将所有计算出的统计量按升序排列。
5. 根据需要计算出置信区间和标准误等统计量。
Bootstrap法的核心在于重复抽样。
通过从原始数据样本中重复随机抽样,我们可以获得更准确的统计量估计和假设检验结果。
在某些情况下,原始数据可能不符合正态分布或其他假设检验的前提条件。
Bootstrap法可以通过生成新的样本来解决这些问题。
二、Bootstrap法的应用场景Bootstrap法可以用于各种统计学应用中,包括参数估计、假设检验、回归分析、时间序列分析等。
以下是Bootstrap法的一些常见应用场景:1. 参数估计:Bootstrap法可以用来估计统计量的标准误和置信区间,如均值、中位数、方差、相关系数等。
2. 假设检验:Bootstrap法可以用来检验假设检验的显著性,如两个总体均值是否相等、回归系数是否显著等。
3. 回归分析:Bootstrap法可以用来估计回归系数的标准误和置信区间,以及模型的预测误差等。
4. 时间序列分析:Bootstrap法可以用来估计时间序列模型的参数和预测误差,以及分析时间序列的置信区间和假设检验结果等。
三、Bootstrap法的实现方法Bootstrap法的实现方法相对简单,可以使用各种编程语言和软件包来实现。
用MATLAB的时重要MATLAB tools
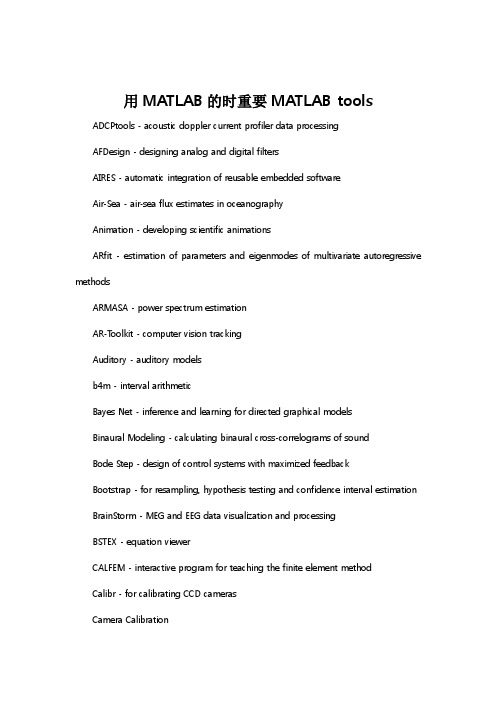
用MATLAB的时重要MATLAB toolsADCPtools - acoustic doppler current profiler data processingAFDesign - designing analog and digital filtersAIRES - automatic integration of reusable embedded softwareAir-Sea - air-sea flux estimates in oceanographyAnimation - developing scientific animationsARfit - estimation of parameters and eigenmodes of multivariate autoregressive methodsARMASA - power spectrum estimationAR-Toolkit - computer vision trackingAuditory - auditory modelsb4m - interval arithmeticBayes Net - inference and learning for directed graphical modelsBinaural Modeling - calculating binaural cross-correlograms of soundBode Step - design of control systems with maximized feedbackBootstrap - for resampling, hypothesis testing and confidence interval estimationBrainStorm - MEG and EEG data visualization and processingBSTEX - equation viewerCALFEM - interactive program for teaching the finite element methodCalibr - for calibrating CCD camerasCamera CalibrationCaptain - non-stationary time series analysis and forecastingCHMMBOX - for coupled hidden Markov modeling using maximum likelihood EM Classification - supervised and unsupervised classification algorithmsCLOSIDCluster - for analysis of Gaussian mixture models for data set clusteringClustering - cluster analysisClusterPack - cluster analysisCOLEA - speech analysisCompEcon - solving problems in economics and financeComplex - for estimating temporal and spatial signal complexitiesComputational StatisticsCoral - seismic waveform analysisDACE - kriging approximations to computer modelsDAIHM - data assimilation in hydrological and hydrodynamic modelsData VisualizationDBT - radar array processingDDE-BIFTOOL - bifurcation analysis of delay differential equationsDenoise - for removing noise from signalsDiffMan - solving differential equations on manifoldsDimensional Analysis -DIPimage - scientific image processingDirect - Laplace transform inversion via the direct integration methodDirectSD - analysis and design of computer controlled systems with process-oriented modelsDMsuite - differentiation matrix suiteDMTTEQ - design and test time domain equalizer design methodsDrawFilt - drawing digital and analog filtersDSFWAV - spline interpolation with Dean wave solutionsDWT - discrete wavelet transformsEasyKrigEconometricsEEGLABEigTool - graphical tool for nonsymmetric eigenproblemsEMSC - separating light scattering and absorbance by extended multiplicative signal correctionEngineering VibrationFastICA - fixed-point algorithm for ICA and projection pursuitFDC - flight dynamics and controlFDtools - fractional delay filter designFlexICA - for independent components analysisFMBPC - fuzzy model-based predictive controlForWaRD - Fourier-wavelet regularized deconvolutionFracLab - fractal analysis for signal processingFSBOX - stepwise forward and backward selection of features using linear regressionGABLE - geometric algebra tutorialGAOT - genetic algorithm optimizationGarch - estimating and diagnosing heteroskedasticity in time series modelsGCE Data - managing, analyzing and displaying data and metadata stored using the GCE data structure specificationGCSV - growing cell structure visualizationGEMANOVA - fitting multilinear ANOVA modelsGenetic AlgorithmGeodetic - geodetic calculationsGHSOM - growing hierarchical self-organizing mapglmlab - general linear modelsGPIB - wrapper for GPIB library from National InstrumentGTM - generative topographic mapping, a model for density modeling and data visualizationGVF - gradient vector flow for finding 3-D object boundariesHFRadarmap - converts HF radar data from radial current vectors to total vectorsHFRC - importing, processing and manipulating HF radar dataHilbert - Hilbert transform by the rational eigenfunction expansion methodHMM - hidden Markov modelsHMMBOX - for hidden Markov modeling using maximum likelihood EMHUTear - auditory modelingICALAB - signal and image processing using ICA and higher order statisticsImputation - analysis of incomplete datasetsIPEM - perception based musical analysisJMatLink - Matlab Java classesKalman - Bayesian Kalman filterKalman Filter - filtering, smoothing and parameter estimation (using EM) for linear dynamical systemsKALMTOOL - state estimation of nonlinear systemsKautz - Kautz filter designKrigingLDestimate - estimation of scaling exponentsLDPC - low density parity check codesLISQ - wavelet lifting scheme on quincunx gridsLKER - Laguerre kernel estimation toolLMAM-OLMAM - Levenberg Marquardt with Adaptive Momentum algorithm for training feedforward neural networksLow-Field NMR - for exponential fitting, phase correction of quadrature data and slicingLPSVM - Newton method for LP support vector machine for machine learning problemsLSDPTOOL - robust control system design using the loop shaping design procedure LS-SVMlabLSVM - Lagrangian support vector machine for machine learning problemsLyngby - functional neuroimagingMARBOX - for multivariate autogressive modeling and cross-spectral estimationMatArray - analysis of microarray dataMatrix Computation - constructing test matrices, computing matrix factorizations, visualizing matrices, and direct search optimizationMCAT - Monte Carlo analysisMDP - Markov decision processesMESHPART - graph and mesh partioning methodsMILES - maximum likelihood fitting using ordinary least squares algorithmsMIMO - multidimensional code synthesisMissing - functions for handling missing data valuesM_Map - geographic mapping toolsMODCONS - multi-objective control system designMOEA - multi-objective evolutionary algorithmsMS - estimation of multiscaling exponentsMultiblock - analysis and regression on several data blocks simultaneouslyMultiscale Shape AnalysisMusic Analysis - feature extraction from raw audio signals for content-based music retrievalMWM - multifractal wavelet modelNetCDFNetlab - neural network algorithmsNiDAQ - data acquisition using the NiDAQ libraryNEDM - nonlinear economic dynamic modelsNMM - numerical methods in Matlab textNNCTRL - design and simulation of control systems based on neural networksNNSYSID - neural net based identification of nonlinear dynamic systemsNSVM - newton support vector machine for solving machine learning problemsNURBS - non-uniform rational B-splinesN-way - analysis of multiway data with multilinear modelsOpenFEM - finite element developmentPCNN - pulse coupled neural networksPeruna - signal processing and analysisPhiVis - probabilistic hierarchical interactive visualization, i.e. functions for visual analysis of multivariate continuous dataPlanar Manipulator - simulation of n-DOF planar manipulatorsPRTools - pattern recognitionpsignifit - testing hyptheses about psychometric functionsPSVM - proximal support vector machine for solving machine learning problemsPsychophysics - vision researchPyrTools - multi-scale image processingRBF - radial basis function neural networksRBN - simulation of synchronous and asynchronous random boolean networksReBEL - sigma-point Kalman filtersRegression - basic multivariate data analysis and regressionRegularization ToolsRegularization Tools XPRestore ToolsRobot - robotics functions, e.g. kinematics, dynamics and trajectory generationRobust Calibration - robust calibration in statsRRMT - rainfall-runoff modellingSAM - structure and motionSchwarz-Christoffel - computation of conformal maps to polygonally bounded regions SDH - smoothed data histogramSeaGrid - orthogonal grid makerSEA-MAT - oceanographic analysisSLS - sparse least squaresSolvOpt - solver for local optimization problemsSOM - self-organizing mapSOSTOOLS - solving sums of squares (SOS) optimization problemsSpatial and Geometric AnalysisSpatial RegressionSpatial StatisticsSpectral MethodsSPM - statistical parametric mappingSSVM - smooth support vector machine for solving machine learning problemsSTATBAG - for linear regression, feature selection, generation of data, and significance testingStatBox - statistical routinesStatistical Pattern Recognition - pattern recognition methodsStixbox - statisticsSVM - implements support vector machinesSVM ClassifierSymbolic Robot DynamicsTEMPLAR - wavelet-based template learning and pattern classificationTextClust - model-based document clusteringTextureSynth - analyzing and synthesizing visual texturesTfMin - continous 3-D minimum time orbit transfer around EarthTime-Frequency - analyzing non-stationary signals using time-frequency distributions Tree-Ring - tasks in tree-ring analysisTSA - uni- and multivariate, stationary and non-stationary time series analysisTSTOOL - nonlinear time series analysisT_Tide - harmonic analysis of tidesUTVtools - computing and modifying rank-revealing URV and UTV decompositions Uvi_Wave - wavelet analysisvarimax - orthogonal rotation of EOFsVBHMM - variation Bayesian hidden Markov modelsVBMFA - variational Bayesian mixtures of factor analyzersVMT - VRML Molecule Toolbox, for animating results from molecular dynamics experimentsVOICEBOXVRMLplot - generates interactive VRML 2.0 graphs and animationsVSVtools - computing and modifying symmetric rank-revealing decompositionsWAFO - wave analysis for fatique and oceanographyWarpTB - frequency-warped signal processingWAVEKIT - wavelet analysisWaveLab - wavelet analysisWeeks - Laplace transform inversion via the Weeks methodWetCDF - NetCDF interfaceWHMT - wavelet-domain hidden Markov tree modelsWInHD - Wavelet-based inverse halftoning via deconvolutionWSCT - weighted sequences clustering toolkitXMLTree - XML parserYAADA - analyze single particle mass spectrum dataZMAP - quantitative seismicity analysis。
重点抽样下拔靴法计算风险值

Journal of Financial Studies Vol.12 No.1 April 2004
83
Glasserman, 2003). That is, it can reduce the sample size to save the computational time with a certain accuracy. The main idea behind the importance sampling is the change of measure. That is, we do simulation from the original probability measure to an importance tilting measure, to obtain the accurate estimator for the portfolio VaR. Glasserman (2002, 2003) considered the idea of combining it with stratified sampling to have a further reduction. The idea of the importance sampling can also be used to price the path-dependent and early exercise option (cf. Glasserman et al., 1999a, 1999b, and Glasserman, 2003). The bootstrap algorithm is a statistical resampling method, and has been applied to finance and risk management (cf. Efron, 1979, Efron and Tibshirani, 1993, Horowitz, 2001, and Jorion, 2000). The main idea of the bootstrap algorithm is to approximate the distribution of the portfolio VaR by its bootstrap analogue, and then use it to approximate the portfolio VaR. There are two approaches in bootstrap algorithm: parametric bootstrap and nonparametric bootstrap (cf. Efron and Tibshirani, 1993). The parametric bootstrap is that one uses the observed data to estimate the unknown parameters of the given distribution, then construct the sampling distribution of the VaR by the bootstrap algorithm from the estimated distribution. The nonparametric bootstrap is that one uses the observed data to construct the sampling distribution of the VaR without any assumption of the underlying distribution. There are two main contributions of this paper. First, we provide the parametric bootstrap method to evaluate VaR in financial assets. Next, we introduce an importance resampling technique to have more efficient simulation in bootstrap replications, and gain the accuracy for the portfolio VaR estimation. Further extension from the parametric bootstrap to the nonparametric bootstrap with importance resampling can be seen in Fuh and Hu (2004). The rest of this paper is organized as follows. In Section II, we provide the asset returns distributions, and introduce a parametric bootstrap method to estimate the tail probability. An importance resampling within the bootstrap procedure is proposed in Section III, to evaluate the value-at-risk. In section IV, we first report the simulation results, which discuss the relative efficiency for the naive Monte Carlo and importance sampling. Then, we provide the comparison of the default bootstrap algorithm and the bootstrap algorithm with importance resampling. The sensitivity analysis of the tail probability and the quantile are also given. As an illustration of our proposed methods, in Section V, we give an empirical study based on two stock index returns in Taiwan, the Chang Hwa Bank and the China Steel Corporation. Conclusion remarks and further researches are presented in Section VI.
A Study of Cross-Validation and Bootstrap for Accuracy Estimation and Model Selection
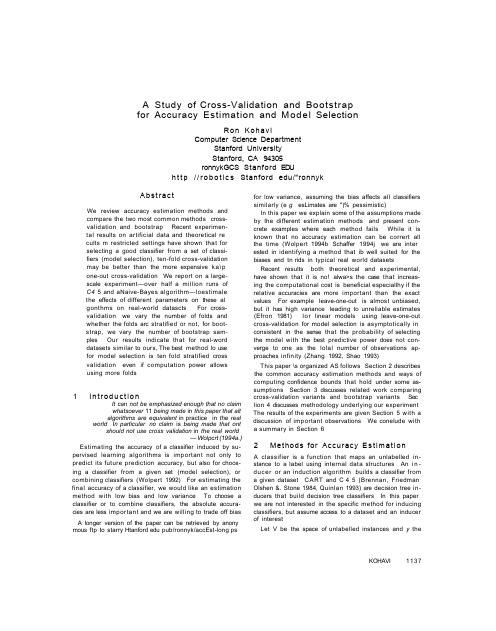
A Study of Cross-Validation and Bootstrap for Accuracy E stimation and Model SelectionRon KohaviComputer Science DepartmentStanford UniversityStanford, CA 94305ronnykGCS Stanford E D Uh t t p //r o b o t i c s Stanford edu/"ronnykA b s t r a c tWe review accuracy estimation methods andcompare the two most common methods cross-validation and bootstrap Recent experimen-tal results on artificial data and theoretical recults m restricted settings have shown that forselecting a good classifier from a set of classi-fiers (model selection), ten-fold cross-validationmay be better than the more expensive ka\pone-out cross-validation We report on a large-scale experiment—over half a million runs ofC4 5 and aNaive-Bayes algorithm—loestimalethe effects of different parameters on these algonthms on real-world datascts For cross-validation we vary the number of folds andwhether the folds arc stratified or not, for boot-strap, we vary the number of bootstrap sam-ples Our results indicate that for real-worddatasets similar to ours, The best method lo usefor model selection is ten fold stratified crossvalidation even if computation power allowsusing more folds1 I n t r o d u c t i o nIt can not be emphasized enough that no claimwhatsoever 11 being made in this paper that altalgorithms a re equiva lent in practice in the rea l world In pa rticula r no cla im is being ma de tha t ont should not use cross va lida tion in the real world— Wolpcrt (1994a.) Estimating the accuracy of a classifier induced by su-pervised learning algorithms is important not only to predict its future prediction accuracy, but also for choos-ing a classifier from a given set (model selection), or combining classifiers (Wolpert 1992) For estimating the final accuracy of a classifier, we would like an estimation method with low bias and low variance To choose a classifier or to combine classifiers, the absolute accura-cies are less important and we are willing to trade off biasA longer version of the paper can be retrieved by anony mous ftp to starry Htanford edu pub/ronnyk/accEst-long ps for low variance, assuming the bias affects all classifiers similarly (e g esLimates are ")% pessimistic)In this paper we explain some of the assumptions madeby Ihe different estimation methods and present con-crete examples where each method fails While it is known that no accuracy estimation can be corrert allthe time (Wolpert 1994b Schaffer 1994j we are inter ested in identifying a method that ib well suited for the biases and tn rids in typical real world datasetsRecent results both theoretical and experimental, have shown that it is no! alwa>s the case that increas-ing the computational cost is beneficial especiallhy if the relative accuracies are more important than the exact values For example leave-one-out is almost unbiased,but it has high variance leading to unreliable estimates (Efron 1981) l o r linear models using leave-one-out cross-validation for model selection is asymptotically in consistent in the sense that the probability of selectingthe model with the best predictive power does not con-verge to one as the lolal number of observations ap-proaches infinity (Zhang 1992, Shao 1993)This paper \s organized AS follows Section 2 describesthe common accuracy estimation methods and ways of computing confidence bounds that hold under some as-sumptions Section 3 discusses related work comparing cross-validation variants and bootstrap variants Sec lion 4 discusses methodology underlying our experimentThe results of the experiments are given Section 5 with a discussion of important observations We conelude witha summary in Section 62 Methods for Accuracy E s t i m a t i o nA classifier is a function that maps an unlabelled in-stance to a label using internal data structures An i n-ducer or an induction algorithm builds a classifier froma given dataset CART and C 4 5 (Brennan, Friedman Olshen &. Stone 1984, Quinlan 1993) are decision tree in-ducers that build decision tree classifiers In this paperwe are not interested in the specific method for inducing classifiers, but assume access to a dataset and an inducerof interestLet V be the space of unlabelled instances and y theKOHAVI 1137set of possible labels be the space of labelled instances and ,i n ) be a dataset (possibly a multiset) consisting of n labelled instances, where A classifier C maps an unla-beled instance ' 10 a l a b e l a n d an inducer maps a given dataset D into a classifier CThe notationwill denote the label assigned to an unlabelled in-stance v by the classifier built, by inducer X on dataset D tWe assume that there exists adistribution on the set of labelled instances and that our dataset consists of 1 1 d (independently and identically distributed) instances We consider equal misclassifica-lion costs using a 0/1 loss function, but the accuracy estimation methods can easily be extended to other loss functionsThe accuracy of a classifier C is the probability ofcorrectly clasaifying a randoml\ selected instance, i efor a randomly selected instancewhere the probability distribution over theinstance space 15 the same as the distribution that was used to select instances for the inducers training set Given a finite dataset we would like to custimate the fu-ture performance of a classifier induced by the given in-ducer and dataset A single accuracy estimate is usually meaningless without a confidence interval, thus we will consider how to approximate such an interval when pos-sible In order to identify weaknesses, we also attempt o identify cases where the estimates fail2 1 Holdout The holdout method sometimes called test sample esti-mation partitions the data into two mutually exclusivesubsets called a training set and a test set or holdout setIt is Lommon to designate 2/ 3 of the data as the trainingset and the remaining 1/3 as the test set The trainingset is given to the inducer, and the induced classifier istested on the test set Formally, let , the holdout set,be a subset of D of size h, and let Theholdout estimated accuracy is defined aswhere otherwise Assummg that the inducer s accuracy increases as more instances are seen, the holdout method is a pessimistic estimator because only a portion of the data is given to the inducer for training The more instances we leave for the test set, the higher the bias of our estimate however, fewer test set instances means that the confidence interval for the accuracy will be wider as shown belowEach test instance can be viewed as a Bernoulli trialcorrect or incorrect prediction Let S be the numberof correct classifications on the test set, then s is dis-tributed bmomially (sum of Bernoulli trials) For rea-sonably large holdout sets, the distribution of S/h is ap-proximately normal with mean ace (the true accuracy of the classifier) and a variance of ace * (1 — acc)hi Thus, by De Moivre-Laplace limit theorem, we havewhere z is the quanl lie point of the standard normal distribution To get a IOO7 percent confidence interval, one determines 2 and inverts the inequalities Inversion of the inequalities leads to a quadratic equation in ace, the roots of which are the low and high confidence pointsThe above equation is not conditioned on the dataset D , if more information is available about the probability of the given dataset it must be taken into accountThe holdout estimate is a random number that de-pends on the division into a training set and a test set In r a n d o m sub s a m p l i n g the holdout method is re-peated k times and the eslimated accuracy is derived by averaging the runs Th( slandard deviation can be estimated as the standard dewation of the accuracy es-timations from each holdout runThe mam assumption that is violated in random sub-sampling is the independence of instances m the test set from those in the training set If the training and testset are formed by a split of an original dalaset, thenan over-represented class in one subset will be a under represented in the other To demonstrate the issue we simulated a 2/3, 1 /3 split of Fisher's famous ins dataset and used a majority inducer that builds a classifier pre dieting the prevalent class in the training set The iris dataset describes ins plants using four continuous fea-tures, and the task is to classify each instance (an ins) as Ins Setosa Ins Versicolour or Ins Virginica For each class label there are exactly one third of the instances with that label (50 instances of each class from a to-tal of 150 instances) thus we expect 33 3% prediction accuracy However, because the test set will always con-tain less than 1/3 of the instances of the class that wasprevalent in the training set, the accuracy predicted by the holdout method is 21 68% with a standard deviation of 0 13% (estimated by averaging 500 holdouts) In practice, the dataset size is always finite, and usu-ally smaller than we would like it to be The holdout method makes inefficient use of the data a third of dataset is not used for training the inducer 2 2 Cross-Validation, Leave-one-out, and Stratification In fc-fold cross-validation, sometimes called rotation esti-mation, the dataset V is randomly split into k mutuallyexclusive subsets (the folds) , of approx-imately equal size The inducer is trained and tested1138 LEARNINGThe cross-validation estimate is a random number that depends on the division into folds C o m p l e t ec r o s s -v a l id a t i o n is the average of all possibil ities for choosing m/k instances out of m, but it is usually too expensive Exrept for leave-one-one (rc-fold cross-validation), which is always complete, fc-foM cross-validation is estimating complete K-foId cross-validationusing a single split of the data into the folds Repeat-ing cross-validation multiple limes using different spillsinto folds provides a better M onte C arlo estimate to 1 hecomplele cross-validation at an added cost In s t r a t i -fied c r o s s -v a l i d a t i o n the folds are stratified so thaitlicy contain approximately the same proportions of la-bels as the original dataset An inducer is stable for a given dataset and a set of perturbal ions if it induces classifiers thai make the same predictions when it is given the perturbed datasets P r o p o s i t i o n 1 (V a r i a n c e in A>fold C V )Given a dataset and an inducer If the inductr isstable under the pei tur bations caused by deleting theinstances f o r thr folds in k fold cross-validatwn thecross validation < stnnate will be unbiastd and the t a i lance of the estimated accuracy will be approximatelyaccrv (1—)/n when n is the number of instancesin the datasi t Proof If we assume that the k classifiers produced makethe same predictions, then the estimated accuracy has a binomial distribution with n trials and probabihly of success equal to (he accuracy of the classifier | For large enough n a confidence interval may be com-puted using Equation 3 with h equal to n, the number of instancesIn reality a complex inducer is unlikely to be stable for large perturbations unless it has reached its maximal learning capacity We expect the perturbations induced by leave-one-out to be small and therefore the classifier should be very stable As we increase the size of the perturbations, stability is less likely to hold we expect stability to hold more in 20-fold cross-validation than in 10-fold cross-validation and both should be more stable than holdout of 1/3 The proposition does not apply to the resubstitution estimate because it requires the in-ducer to be stable when no instances are given in the datasetThe above proposition helps, understand one possible assumption that is made when using cross-validation if an inducer is unstable for a particular dataset under a set of perturbations introduced by cross-validation, the ac-curacy estimate is likely to be unreliable If the inducer is almost stable on a given dataset, we should expect a reliable estimate The next corollary takes the idea slightly further and shows a result that we have observed empirically there is almost no change in the variance of the cross validation estimate when the number of folds is variedC o r o l l a r y 2 (Variance m cross-validation)Given a dataset and an inductr If the inducer is sta-ble undfi the }>tituibuhoris (aused by deleting the test instances foi the folds in k-fold cross-validation for var-ious valuts of k then tht vartanct of the estimates will be the sameProof The variance of A-fold cross-validation in Propo-sition 1 does not depend on k |While some inducers are liktly to be inherently more stable the following example shows that one must also take into account the dalaset and the actual perturba (ions E x a m p l e 1 (Failure of leave-one-out)lusher s ins dataset contains 50 instances of each class leading one to expect that a majority indu<er should have acruraov about j \% However the eombmation ofthis dataset with a majority inducer is unstable for thesmall perturbations performed by leave-one-out Whenan instance is deleted from the dalaset, its label is a mi-nority in the training set, thus the majority inducer pre-dicts one of the other two classes and always errs in clas-sifying the test instance The leave-one-out estimatedaccuracy for a majont> inducer on the ins dataset istherefore 0% M oreover all folds have this estimated ac-curacy, thus the standard deviation of the folds is again0 %giving the unjustified assurance that 'he estimate is stable | The example shows an inherent problem with cross-validation th-t applies to more than just a majority in-ducer In a no-infornirition dataset where the label val-ues are completely random, the best an induction algo-rithm can do is predict majority Leave-one-out on such a dataset with 50% of the labels for each class and a majontv ind'-cer (the best, possible inducer) would still predict 0% accuracy 2 3 B o o t s t r a pThe bootstrap family was introduced by Efron and is fully described in Efron &. Tibshirani (1993) Given a dataset of size n a b o o t s t r a p s a m p l e is created by sampling n instances uniformly from the data (with re-placement) Since the dataset is sampled with replace-ment, the probability of any given instance not beingchosen after n samples is theKOHAVI 1139expected number of distinct instances from the original dataset appearing in the teat set is thus 0 632n The eO accuracy estimate is derived by using the bootstrap sam-ple for training and the rest of the instances for testing Given a number b, the number of bootstrap samples, let e0, be the accuracy estimate for bootstrap sample i The632 bootstrap estimate is defined as(5)where ace, is the resubstitution accuracy estimate on the full dataset (i e , the accuracy on the training set) The variance of the estimate can be determined by com puting the variance of the estimates for the samples The assumptions made by bootstrap are basically the same as that of cross-validation, i e , stability of the al-gorithm on the dataset the 'bootstrap world" should closely approximate the real world The b32 bootstrap fails (o give the expected result when the classifier is a perfect memonzer (e g an unpruned decision tree or a one nearest neighbor classifier) and the dataset is com-pletely random, say with two classes The resubstitution accuracy is 100%, and the eO accuracy is about 50% Plugging these into the bootstrap formula, one gets an estimated accuracy of about 68 4%, far from the real ac-curacy of 50% Bootstrap can be shown to fail if we add a memonzer module to any given inducer and adjust its predictions If the memonzer remembers the training set and makes the predictions when the test instance was a training instances, adjusting its predictions can make the resubstitution accuracy change from 0% to 100% and can thus bias the overall estimated accuracy in any direction we want3 Related W o r kSome experimental studies comparing different accuracy estimation methods have been previously done but most of them were on artificial or small datasets We now describe some of these effortsEfron (1983) conducted five sampling experiments and compared leave-one-out cross-validation, several variants of bootstrap, and several other methods The purpose of the experiments was to 'investigate some related es-timators, which seem to offer considerably improved es-timation in small samples ' The results indicate that leave-one-out cross-validation gives nearly unbiased esti-mates of the accuracy, but often with unacceptably high variability, particularly for small samples, and that the 632 bootstrap performed bestBreiman et al (1984) conducted experiments using cross-validation for decision tree pruning They chose ten-fold cross-validation for the CART program and claimed it was satisfactory for choosing the correct tree They claimed that "the difference in the cross-validation estimates of the risks of two rules tends to be much more accurate than the two estimates themselves "Jain, Dubes fa Chen (1987) compared the performance of the t0 bootstrap and leave-one-out cross-validation on nearest neighbor classifiers Using artificial data and claimed that the confidence interval of the bootstrap estimator is smaller than that of leave-one-out Weiss (1991) followed similar lines and compared stratified cross-validation and two bootstrap methods with near-est neighbor classifiers His results were that stratified two-fold cross validation is relatively low variance and superior to leave-one-outBreiman fa Spector (1992) conducted a feature sub-set selection experiments for regression, and compared leave-one-out cross-validation, A:-fold cross-validation for various k, stratified K-fold cross-validation, bias-corrected bootstrap, and partial cross-validation (not discussed here) Tests were done on artificial datasets with 60 and 160 instances The behavior observed was (1) the leave-one-out has low bias and RMS (root mean square) error whereas two-fold and five-fold cross-validation have larger bias and RMS error only at models with many features, (2) the pessimistic bias of ten-fold cross-validation at small samples was significantly re-duced for the samples of size 160 (3) for model selection, ten-fold cross-validation is better than leave-one-out Bailey fa E lkan (1993) compared leave-one-out cross-ahdation to 632 bootstrap using the FOIL inducer and four synthetic datasets involving Boolean concepts They observed high variability and little bias in the leave-one-out estimates, and low variability but large bias in the 632 estimatesWeiss and Indurkyha (Weiss fa Indurkhya 1994) con-ducted experiments on real world data Lo determine the applicability of cross-validation to decision tree pruning Their results were that for samples at least of size 200 using stratified ten-fold cross-validation to choose the amount of pruning yields unbiased trees (with respect to their optimal size) 4 M e t h o d o l o g yIn order to conduct a large-scale experiment we decided to use 04 5 and a Naive Bayesian classifier The C4 5 algorithm (Quinlan 1993) is a descendent of ID3 that builds decision trees top-down The Naive-Bayesian clas-sifier (Langley, Iba fa Thompson 1992) used was the one implemented in (Kohavi, John, Long, Manley fa Pfleger 1994) that uses the observed ratios for nominal features and assumes a Gaussian distribution for contin-uous features The exact details are not crucial for this paper because we are interested in the behavior of the accuracy estimation methods more than the internals of the induction algorithms The underlying hypothe-sis spaces—decision trees for C4 5 and summary statis-tics for Naive-Bayes—are different enough that we hope conclusions based on these two induction algorithms will apply to other induction algorithmsBecause the target concept is unknown for real-world1140 LEARNINGconcepts, we used the holdout method to estimate the quality of the cross-validation and bootstrap estimates To choose & set of datasets, we looked at the learning curves for C4 5 and Najve-Bayes for most of the super-vised classification dataaets at the UC Irvine repository (Murphy & Aha 1994) that contained more than 500 instances (about 25 such datasets) We felt that a min-imum of 500 instances were required for testing While the true accuracies of a real dataset cannot be computed because we do not know the target concept, we can esti mate the true accuracies using the holdout method The "true' accuracy estimates in Table 1 were computed by taking a random sample of the given size computing the accuracy using the rest of the dataset as a test set, and repeating 500 timesWe chose six datasets from a wide variety of domains, such that the learning curve for both algorithms did not flatten out too early that is, before one hundred instances We also added a no inform a tion d l stt, rand, with 20 Boolean features and a Boolean random label On one dataset vehicle, the generalization accu-racy of the Naive-Bayes algorithm deteriorated hy morethan 4% as more instances were g;iven A similar phenomenon was observed on the shuttle dataset Such a phenomenon was predicted by Srhaffer and Wolpert (Schaffer 1994, Wolpert 1994), but we were surprised that it was observed on two real world datasetsTo see how well an Accuracy estimation method per forms we sampled instances from the dataset (uniformly without replacement) and created a training set of the desired size We then ran the induction algorihm on the training set and tested the classifier on the rest of the instances L E I the dataset This was repeated 50 times at points where the lea rning curve wa s sloping up The same folds in cross-validation and the same samples m bootstrap were used for both algorithms compared5 Results and DiscussionWe now show the experimental results and discuss their significance We begin with a discussion of the bias in the estimation methods and follow with a discussion of the variance Due to lack of space, we omit some graphs for the Naive-Bayes algorithm when the behavior is ap-proximately the same as that of C 4 5 5 1 T h e B i a sThe bias of a method to estimate a parameter 0 is de-fined as the expected value minus the estimated value An unbiased estimation method is a method that has zero bias Figure 1 shows the bias and variance of k-fold cross-validation on several datasets (the breast cancer dataset is not shown)The diagrams clearly show that k-fold cross-validation is pessimistically biased, especially for two and five folds For the learning curves that have a large derivative at the measurement point the pessimism in k-fold cross-Figure ] C'4 5 The bias of cross-validation with varying folds A negative K folds stands for leave k-out E rror bars are 95% confidence intervals for (he mean The gray regions indicate 95 % confidence intervals for the true ac curaries Note the different ranges for the accuracy axis validation for small k s is apparent Most of the esti-mates are reasonably good at 10 folds and at 20 folds they art almost unbiasedStratified cross validation (not shown) had similar be-havior, except for lower pessimism The estimated accu-racy for soybe an at 2 fold was 7% higher and at five-fold, 1 1% higher for vehicle at 2-fold, the accuracy was 2 8% higher and at five-fold 1 9% higher Thus stratification seems to be a less biased estimation methodFigure 2 shows the bias and variance for the b32 boot-strap accuracy estimation method Although the 632 bootstrap is almost unbiased for chess hypothyroid, and mushroom for both inducers it is highly biased for soy-bean with C'A 5, vehicle with both inducers and rand with both inducers The bias with C4 5 and vehicle is 9 8%5 2 The VarianceWhile a given method may have low bias, its perfor-mance (accuracy estimation in our case) may be poor due to high variance In the experiments above, we have formed confidence intervals by using the standard de-viation of the mea n a ccura cy We now switch to the standard deviation of the population i e , the expected standard deviation of a single accuracy estimation run In practice, if one dots a single cross-validation run the expected accuracy will be the mean reported above, but the standard deviation will be higher by a factor of V50, the number of runs we averaged in the experimentsKOHAVI 1141Table 1 True accuracy estimates for the datasets using C4 5 and Naive-Bayes classifiers at the chosen sample sizesFigure 2 C4 5 The bias of bootstrap with varying sam-ples Estimates are good for mushroom hypothyroid, and chess, but are extremely biased (optimistically) for vehicle and rand, and somewhat biased for soybeanIn what follows, all figures for standard deviation will be drawn with the same range for the standard devi-ation 0 to 7 5% Figure 3 shows the standard devia-tions for C4 5 and Naive Bayes using varying number of folds for cross-validation The results for stratified cross-validation were similar with slightly lower variance Figure 4 shows the same information for 632 bootstrap Cross-validation has high variance at 2-folds on both C4 5 and Naive-Bayes On C4 5, there is high variance at the high-ends too—at leave-one-out and leave-two-out—for three files out of the seven datasets Stratifica-tion reduces the variance slightly, and thus seems to be uniformly better than cross-validation, both for bias and vananceFigure 3 Cross-validation standard deviation of accu-racy (population) Different, line styles are used to help differentiate between curves6 S u m m a r yWe reviewed common accuracy estimation methods in-cluding holdout, cross-validation, and bootstrap, and showed examples where each one fails to produce a good estimate We have compared the latter two approaches on a variety of real-world datasets with differing charac-teristicsProposition 1 shows that if the induction algorithm is stable for a given dataset, the variance of the cross-validation estimates should be approximately the same, independent of the number of folds Although the induc-tion algorithms are not stable, they are approximately stable it fold cross-validation with moderate k values (10-20) reduces the variance while increasing the bias As k decreases (2-5) and the sample sizes get smaller, there is variance due to the instability of the training1142 LEARNING1 igure 4 632 Bootstrap standard deviation in acc-rat y (population)sets themselves leading to an increase in variance this is most apparent for datasets with many categories, such as soybean In these situations) stratification seems to help, but -epeated runs may be a better approach Our results indicate that stratification is generally a better scheme both in terms of bias and variance whencompared to regular cross-validation Bootstrap has low,variance but extremely large bias on some problems We recommend using stratified Len fold cross-validation for model selection A c k n o w l e d g m e n t s We thank David Wolpert for a thorough reading of this paper and many interesting dis-cussions We thank Tom Bylander Brad E fron Jerry Friedman, Rob Holte George John Pat Langley Hob Tibshiram and Sholom Weiss for their helpful com nients and suggestions Dan Sommcrfield implemented Lhe bootstrap method in WLC++ All experiments were conducted using M L C ++ partly partly funded by ONR grant N00014-94-1-0448 and NSF grants IRI 9116399 and IRI-941306ReferencesBailey, T L & E lkan C (1993) stimating the atcuracy of learned concepts, in Proceedings of In ternational Joint Conference on Artificial Intelli-gence , Morgan Kaufmann Publishers, pp 895 900 Breiman, L & Spector, P (1992) Submodel selectionand evaluation in regression the x random case Inttrnational St atistic al Review 60(3), 291-319 Breiman, L , Friedman, J H , Olshen, R A & StoneC J (1984), Cl a ssific ation a nd Regression Trets Wadsworth International GroupEfron, B (1983), 'E stimating the error rate of a pre-diction rule improvement on cross-validation",Journal of the Americ an St atistic al Associ ation 78(382), 316-330 Efron, B & Tibshiram, R (1993) An introduction tothe bootstra p, Chapman & HallJam, A K Dubes R C & Chen, C (1987), "Boot-strap techniques lor error estimation", IEEE tra ns-actions on p a ttern a n a lysis a nd m a chine intelli-gence P A M I -9(5), 628-633 Kohavi, R , John, G , Long, R , Manley, D &Pfleger K (1994), M L C ++ A machine learn-ing library in C ++ in 'Tools with Artifi-cial Intelligence I E E EComputer Society Press, pp 740-743 Available by anonymous ftp from s t a r r y Stanford E DU pub/ronnyk/mlc/ toolsmlc psLangley, P Tba, W & Thompson, K (1992), An anal-ysis of bayesian classifiers in Proceedings of the tenth national conference on artificial intelligence",A A A I Press and M I T Press, pp 223-228Murph' P M & Aha D W (1994), V( I repository of machine learning databases, For information con-tact ml-repository (Ui(,s uci edu Quinlan I R (1993) C4 5 Progra ms for Ma chine Learning Morgan Kaufmann Los Altos CaliforniaSchaffcr C (19941 A conservation law for generalization performance, in Maehinc Learning Proceedings of Lhe E leventh International conference Morgan Kaufmann, pp 259-265Shao, J (1993), Linear model seletion via cross-validation Journ a l of the America n sta tistica l As-sociation 88(422) 486-494 Weiss S M (1991), Small sample error rate estimationfor k nearest neighbor classifiers' I E EE Tr a ns ac tions on Pa ttern An alysis a nd Ma chine Intelligence 13(3), 285-289 Weiss, S M & lndurkhya N (1994) Decision Lreepruning Biased or optimal, in Proceedings of the twelfth national conference on artificial intel-ligence A A A I Press and M I T Press pp 626-632 Wolpert D H (1992), Stacked generalization , Neura lNetworks 5 241-259 Wolpert D H (1994a) Off training set error and a pri-ori distinctions between learning algorithms, tech-mcal Report SFI TR 94-12-121, The Sante Fe ln-stituteWolpert D II {1994b), The relationship between PAC, the statistical physics framework the Bayesian framework, and the VC framework Technical re-port, The Santa Fe Institute Santa Fe, NMZhang, P (1992), 'On the distributional properties of model selection criteria' Journ al of the America nStatistical Associa tion 87(419), 732-737 KOHAVI 1143。
bootstrapping method
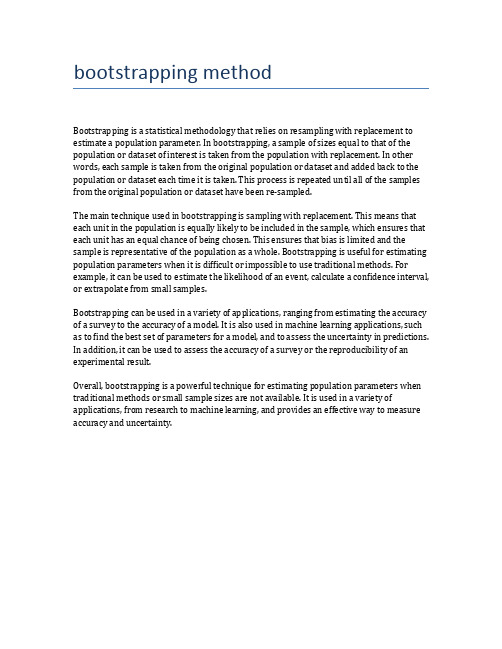
bootstrapping methodBootstrapping is a statistical methodology that relies on resampling with replacement to estimate a population parameter. In bootstrapping, a sample of sizes equal to that of the population or dataset of interest is taken from the population with replacement. In other words, each sample is taken from the original population or dataset and added back to the population or dataset each time it is taken. This process is repeated until all of the samples from the original population or dataset have been re-sampled.The main technique used in bootstrapping is sampling with replacement. This means that each unit in the population is equally likely to be included in the sample, which ensures that each unit has an equal chance of being chosen. This ensures that bias is limited and the sample is representative of the population as a whole. Bootstrapping is useful for estimating population parameters when it is difficult or impossible to use traditional methods. For example, it can be used to estimate the likelihood of an event, calculate a confidence interval, or extrapolate from small samples.Bootstrapping can be used in a variety of applications, ranging from estimating the accuracy of a survey to the accuracy of a model. It is also used in machine learning applications, such as to find the best set of parameters for a model, and to assess the uncertainty in predictions. In addition, it can be used to assess the accuracy of a survey or the reproducibility of an experimental result.Overall, bootstrapping is a powerful technique for estimating population parameters when traditional methods or small sample sizes are not available. It is used in a variety of applications, from research to machine learning, and provides an effective way to measure accuracy and uncertainty.。
Statistics Toolbox

An Introduction to Dataset Arrays 5:31Improve productivity with statistical arrays.Statistics ToolboxPerform statistical modeling and analysisStatistics Toolbox™provides algorithms and tools for organizing, analyzing, and modeling data. You can use regression or classification for predictive modeling, generate random numbers for Monte Carlo simulations, use statistical plots for exploratory data analysis, and perform hypothesis tests.For analyzing multidimensional data, Statistics Toolbox includes algorithms that let you identify key variables that impact your model with sequential feature selection, transform your data with principal component analysis,apply regularization and shrinkage, or use partial least squares regression.Statistics Toolbox includes specialized data types for organizing and accessing heterogeneous data. Dataset arrays store numeric data, text, and metadata in a single data container. Built-in methods enable you to merge datasets using a common key (join), calculate summary statistics on grouped data, and convert between tall and wide data representations. Categorical arrays provide a memory-efficient data container for storing information drawn from a finite, discrete set of categories.Statistics Toolbox is included in MATLAB and Simulink Student Version .Key Features▪Statistical arrays for storing heterogeneous and categorical data▪Regression techniques, including linear, nonlinear, robust, and ridge, and nonlinear mixed-effects models ▪Classification algorithms, including boosted and bagged decision trees, k-Nearest Neighbor, and linear discriminant analysis▪Analysis of variance (ANOV A)▪Probability distributions, including copulas and Gaussian mixtures▪Random number generation▪Hypothesis testing▪Design of experiments and statistical process controlData Organization and ManagementStatistics Toolbox provides two specialized arrays for storing and managing statistical data: dataset arrays and categorical arrays.Dataset ArraysDataset arrays enable convenient organization and analysis of heterogeneous statistical data and metadata. Dataset arrays provide columns to represent measured variables and rows to represent observations. With dataset arrays,you can:▪Store different types of data in a single container.An Introduction to Joins 5:01Combine fields from two dataset arrays using a variable that is present in both.▪Label rows and columns of data and reference that data using recognizable names.▪Display and edit data in an intuitive tabular format.▪Use metadata to define units, describe data, and store information.Dataset array displayed in the MATLAB Variable Editor. This dataset array includes a mixture of cell strings and numeric information, with selected columns available in the Plot Selector Tool.Statistics Toolbox provides specialized functions to operate on dataset arrays. With these specialized functions,you can:▪Merge datasets by combining fields using common keys.▪Export data into standard file formats, including Microsoft ®Excel ®and comma-separated value (CSV).▪Calculate summary statistics on grouped data.▪Convert data between tall and wide representations.Categorical ArraysCategorical arrays enable you to organize and process nominal and ordinal data that uses values from a finite set of discrete levels or categories. With categorical arrays, you can:▪Decrease memory footprint by replacing repetitive text strings with categorical labels.▪Store nominal data using descriptive labels, such as red ,green , and blue for an unordered set of colors.▪Store ordinal data using descriptive labels, such as cold ,warm , and hot for an ordered set of temperature measurements.▪Manipulate categorical data using familiar array operations and indexing methods.▪Create logical indexes based on categorical data.▪Group observations by category.Exploratory Data AnalysisStatistics Toolbox provides multiple ways to explore data: statistical plotting with interactive graphics, algorithms for cluster analysis, and descriptive statistics for large datasets.Statistical Plotting and Interactive GraphicsStatistics Toolbox includes graphs and charts to explore your data visually. The toolbox augments MATLAB®plot types with probability plots, box plots, histograms, scatter histograms, 3D histograms, control charts, and quantile-quantile plots. The toolbox also includes specialized plots for multivariate analysis, including dendograms, biplots, parallel coordinate charts, and Andrews plots.Group scatter plot matrix showing interactions between five variables.Compact box plot with whiskers providing a five-number summary of a dataset.Scatter histogram using a combination of scatter plots and histograms to describe the relationship between variables.Plot comparing the empirical CDF for a sample from an extreme value distribution with a plot of the CDF for the sampling distribution.Cluster AnalysisStatistics Toolbox offers multiple algorithms to analyze data using hierarchical clustering, k-means clustering, and Gaussian mixtures.Two-component Gaussian mixture model fit to a mixture of bivariate Gaussians.Output from applying a clustering algorithm to the same example.Dendrogram plot showing a model with four clusters.Cluster Analysis(Example)Use k-means and hierarchical clustering to discover natural groupings in data.Descriptive StatisticsDescriptive statistics enable you to understand and describe potentially large sets of data quickly. Statistics Toolbox includes functions for calculating:▪Measures of central tendency (measures of location), including average, median, and various means▪Measures of dispersion (measures of spread), including range, variance, standard deviation, and mean or median absolute deviation▪Linear and rank correlation (partial and full)▪Results based on data with missing values▪Percentile and quartile estimates▪Density estimates using a kernel-smoothing functionThese functions help you summarize values in a data sample using a few highly relevant numbers.In some cases, estimating summary statistics using parametric methods is not possible. To deal with these cases, Statistics Toolbox provides resampling techniques, including:▪Generalized bootstrap function for estimating sample statistics using resampling▪Jackknife function for estimating sample statistics using subsets of the data▪bootci function for estimating confidence intervals4:07Develop a predictive model without specifying a function that describes the relationship between variables.Regression, Classification, and ANOV ARegressionWith regression, you can model a continuous response variable as a function of one or more predictors. Statistics Toolbox offers a wide variety of regression algorithms, including:▪Linear regression▪Nonlinear regression▪Robust regression▪Logistic regression and other generalized linear modelsFitting with MATLAB: Statistics, Optimization, and Curve Fitting (Webinar)Apply regression algorithms with MATLAB.You can evaluate goodness of fit using a variety of metrics, including:▪R 2and adjusted R 2▪Cross-validated mean squared error▪Akaike information criterion (AIC) and Bayesian information criterion (BIC)With the toolbox, you can calculate confidence intervals for both regression coefficients and predicted values.Statistics Toolbox supports more advanced techniques to improve predictive accuracy when the dataset includes large numbers of correlated variables. The toolbox supports:▪Subset selection techniques, including sequential features selection and stepwise regression▪Regularization methods, including ridge regression, lasso, and elastic netComputational Statistics: Feature Selection, Regularization, and Shrinkage with MATLAB(Webinar)Learn how to generate accurate fits in the presence of correlated data.Statistics Toolbox also supports nonparametric regression techniques for generating an accurate fit without specifying a model that describes the relationship between the predictor and the response. Nonparametric regression techniques include decision trees as well as boosted and bagged regression trees.Additionally, Statistics Toolbox supports nonlinear mixed-effect (NLME) models in which some parameters of a nonlinear function vary across individuals or groups.An Introduction to Classification9:00Develop predictive models for classifying data.Nonlinear mixed-effects model of drug absorption and elimination showing intrasubject concentration-versus-time profiles. The nlmefit function in Statistics Toolbox generates a population model using fixed and random effects.ClassificationClassification algorithms enable you to model a categorical response variable as a function of one or more predictors. Statistics Toolbox offers a wide variety of parametric and nonparametric classification algorithms,such as:▪Boosted and bagged classification trees, including AdaBoost, LogitBoost, GentleBoost, and RobustBoost ▪Naïve Bayes classification▪k-Nearest Neighbor (kNN) classification▪Linear discriminant analysisYou can evaluate goodness of fit for the resulting classification models using techniques such as:▪Cross-validated loss▪Confusion matrices▪Performance curves/receiver operating characteristic (ROC) curvesANOV AAnalysis of variance (ANOV A) enables you to assign sample variance to different sources and determine whether the variation arises within or among different population groups. Statistics Toolbox includes these ANOV A algorithms and related techniques:One-way ANOV A▪Two-way ANOV A for balanced data▪Multiway ANOV A for balanced and unbalanced data▪Multivariate ANOV A (MANOV A)▪Nonparametric one-way and two-way ANOVA (Kruskal-Wallis and Friedman)▪Analysis of covariance (ANOCOVA)▪Multiple comparison of group means, slopes, and interceptsMultivariate StatisticsMultivariate statistics provide algorithms and functions to analyze multiple variables. Typical applications include:▪Transforming correlated data into a set of uncorrelated components using rotation and centering (principal component analysis)▪Exploring relationships between variables using visualization techniques, such as scatter plot matrices and classical multidimensional scaling▪Segmenting data with cluster analysisFitting an Orthogonal Regression Using Principal Component Analysis(Example)Implement Deming regression (total least squares).Feature TransformationFeature transformation techniques enable dimensionality reduction when transformed features can be more easily ordered than original features. Statistics Toolbox offers three classes of feature transformation algorithms:▪Principal component analysis for summarizing data in fewer dimensions▪Nonnegative matrix factorization when model terms must represent nonnegative quantities▪Factor analysis for building explanatory models of data correlationPartial Least Squares Regression and Principal Component Regression(Example)Model a response variable in the presence of highly correlated predictors.Multivariate VisualizationStatistics Toolbox provides graphs and charts to explore multivariate data visually, including:▪Scatter plot matrices▪Dendograms▪Biplots▪Parallel coordinate charts▪Andrews plots▪Glyph plotsGroup scatter plot matrix showing how model year impacts different variables.Biplot showing the first three loadings from a principal component analysis.Andrews plot showing how country of original impacts the variables.Cluster AnalysisStatistics Toolbox offers multiple algorithms for cluster analysis, including:▪Hierarchical clustering, which creates an agglomerative cluster typically represented as a tree.▪K-means clustering, which assigns data points to the cluster with the closest mean.▪Gaussian mixtures, which are formed by combining multivariate normal density components. Clusters areassigned by selecting the component that maximizes posterior probability.Two-component Gaussian mixture model fit to a mixture of bivariate Gaussians.Output from applying a clustering algorithm to the same example.Dendrogram plot showing a model with four clusters.Probability DistributionsStatistics Toolbox provides functions and graphical tools to work with parametric and nonparametric probability distributions. With these tools, you can:▪Fit distributions to data.▪Use statistical plots to evaluate goodness of fit.▪Compute key functions such as probability density functions and cumulative distribution functions.▪Generate random and quasi-random number streams from probability distributions.8:15Fit distributions to empirical data, and visually explore the effects of changing parameters onthe shape of a distribution.The Distribution Fitting Tool in the toolbox enables you to fit data using predefined univariate probability distributions, a nonparametric (kernel-smoothing) estimator, or a custom distribution that you define. This tool supports both complete data and censored (reliability) data. You can exclude data, save and load sessions, and generate MATLAB code.Visual plot of distribution data (left) and summary statistics (right). Using the Distribution Fitting Tool, you can estimate a normal distribution with mean and variance values (16.9 and 8.7, respectively, in this example).You can estimate distribution parameters at the command line or construct probability distributions that correspond to the governing parameters.Additionally, you can create multivariate probability distributions, including Gaussian mixtures and multivariate normal, multivariate t, and Wishart distributions. You can use copulas to create multivariate distributions by joining arbitrary marginal distributions using correlation structures.See the complete list of supported distributions.Simulating Dependent Random Numbers Using Copulas(Example)Create distributions that model correlated multivariate data.With the toolbox, you can specify custom distributions and fit these distributions using maximum likelihood estimation.Fitting Custom Univariate Distributions(Example)Perform maximum likelihood estimation on truncated, weighted, or bimodal data.Evaluating Goodness of FitStatistics Toolbox provides statistical plots to evaluate how well a dataset matches a specific distribution. The toolbox includes probability plots for a variety of standard distributions, including normal, exponential, extreme value, lognormal, Rayleigh, and Weibull. You can generate probability plots from complete datasets and censored datasets. Additionally, you can use quantile-quantile plots to evaluate how well a given distribution matches a standard normal distribution.Statistics Toolbox also provides hypothesis tests to determine whether a dataset is consistent with different probability distributions. Specific tests include:▪Chi-Square goodness-of-fit tests▪One-sided and two-sided Kolmogorov-Smirnov tests▪Lilliefors tests▪Ansari-Bradley tests▪Jarque-Bera testsAnalyzing Probability DistributionsStatistics Toolbox provides functions for analyzing probability distributions, including:▪Probability density functions▪Cumulative density functions▪Inverse cumulative density functions▪Negative log-likelihood functionsGenerating Random NumbersStatistics Toolbox provides functions for generating pseudo-random and quasi-random number streams from probability distributions. You can generate random numbers from either a fitted or constructed probability distribution by applying the random method.MATLAB code for constructing a Poisson distribution with a specific mean and generating a vector of random numbers that match the distribution.Statistics Toolbox also provides functions for:▪Generating random samples from multivariate distributions, such as t, normal, copulas, and Wishart▪Sampling from finite populations▪Performing Latin hypercube sampling▪Generating samples from Pearson and Johnson systems of distributionsYou can also generate quasi-random number streams. Quasi-random number streams produce highly uniform samples from the unit hypercube. Quasi-random number streams can often accelerate Monte Carlo simulations because fewer samples are required to achieve complete coverage.Hypothesis TestingRandom variation can make it difficult to determine whether samples taken under different conditions are different. Hypothesis testing is an effective tool for analyzing whether sample-to-sample differences are significant and require further evaluation or are consistent with random and expected data variation. Statistics Toolbox supports widely used parametric and nonparametric hypothesis testing procedures, including:▪One-sample and two-sample t-tests▪Nonparametric tests for one sample, paired samples, and two independent samples▪Distribution tests (Chi-square, Jarque-Bera, Lillifors, and Kolmogorov-Smirnov)Comparison of distributions (two-sample Kolmogorov-Smirnov)▪Tests for autocorrelation and randomness▪Linear hypothesis tests on regression coefficientsSelecting a Sample Size(Example)Calculate the sample size necessary for a hypothesis test.Design of Experiments and Statistical Process ControlDesign of ExperimentsFunctions for design of experiments (DOE) enable you to create and test practical plans to gather data for statistical modeling. These plans show how to manipulate data inputs in tandem to generate information about their effect on data outputs. Supported design types include:▪Full factorial▪Fractional factorial▪Response surface (central composite and Box-Behnken)▪D-optimal▪Latin hypercubeYou can use Statistics Toolbox to define, analyze, and visualize a customized DOE. For example, you can estimate input effects and input interactions using ANOVA, linear regression, and response surface modeling, then visualize results through main effect plots, interaction plots, and multi-vari charts.Fitting a decision tree to data. The fitting capabilities in Statistics Toolbox enable you to visualize a decision tree by drawing a diagram of the decision rule and group assignments.Model of a chemical reaction for an experiment using the design-of-experiments (DOE) and surface-fitting capabilities of Statistics Toolbox.Statistical Process ControlStatistics Toolbox provides a set of functions that support Statistical Process Control (SPC). These functions enable you to monitor and improve products or processes by evaluating process variability. With SPC functions, you can:▪Perform gage repeatability and reproducibility studies.▪Estimate process capability.▪Create control charts.▪Apply Western Electric and Nelson control rules to control chart data.Control charts showing process data and violations of Western Electric control rules. Statistics Toolbox provides a variety of control charts and control rules for monitoring and evaluating products or processes.Product Details, Examples, and System Requirements/products/statisticsTrial Software/trialrequestSales/contactsalesTechnical Support/support ResourcesOnline User Community /matlabcentral Training Services /training Third-Party Products and Services /connections Worldwide Contacts /contact。
时间温度超位数Master Curve估计:时间温度超位数分析应用于频率修饰数据的动态 mechan

Package‘TTS’February24,2023Type PackageTitle Master Curve Estimates Corresponding to Time-TemperatureSuperpositionVersion1.1Date2023-02-24Author Antonio Meneses<********************************>,Salvador Naya<************>,Javier Tarrio-Saavedra<**************>Maintainer Antonio Meneses<********************************>Depends R(>=3.0.1),mgcv,sfsmisc,splinesDescription Time-Temperature Superposition analysis is often applied to frequency modu-lated data obtained by DynamicMechanic Analysis(DMA)and Rheometry in the analytical chemistry and physicsareas.These techniques provide estimates of material mechanical properties(such as moduli)at different temperatures in a wider range of time.Thispackage provides the Time-Temperature superposition Master Curve at a referredtemperature by the three methods:the two wider used methods,Arrhenius basedmethods and WLF,and the newer methodology based on derivatives procedure.The Master Curve is smoothed by B-splines basis.The package output is composedof plots of experimental data,horizontal and vertical shifts,TTS data,and TTSdatafitted using B-splines with bootstrap confidence intervals.License GPL(>=2)Encoding UTF-8RoxygenNote7.2.1LazyData trueNeedsCompilation noRepository CRANDate/Publication2023-02-2417:50:02UTCR topics documented:TTS-package (2)12TTS-package Epoxy (3)PC (4)PLOT.TTS (5)SBS (7)TTS (8)TTS_10 (10)Index13 TTS-package Estimates of material properties by Time-Temperature Superposition(TTS)analysisDescriptionTTS analysis is often applied to frequency modulated data obtained by Dynamic Mechanic Analy-sis(DMA)and Rheometry in the analytical chemistry and physics areas.These techniques provide estimates of material mechanical properties(such as moduli)at different temperatures in a wider range of time.This package provides the Time-Temperature superposition Master Curve at a re-ferred temperature by the three methods:the two wider used methods,Arrhenius based methods and WLF,and the newer methodology based on derivatives procedure.The Master Curve is smoothed by B-splines basis.The package output is composed of plots of experimental data,horizontal and vertical shifts,TTS data,and TTS datafitted using B-splines with bootstrap confidence intervals.DetailsPackage:TTSType:PackageVersion: 1.1Date:2023-02-24License:GPL>=2The main functions and data frame are TTS,TTS_10,PLOT.TTS,Epoxy,SBS,and PCAuthor(s)Antonio Meneses<********************************>,Salvador Naya<************>and Javier Tarrio-Saavedra<**************>Maintainer:Antonio Meneses<********************************>ReferencesNaya,S.,Meneses A.,Tarrio-Saavedra,J.,Artiaga R.,Lopez-Beceiro,J.and Gracia-Fernandez C.(2013)New method for estimating shift factors in time-temperatura superposition models.JournalEpoxy3 of Thermal Analysis and Calorimetry.ISSN1388-6150.DOI10.1007/s10973-013-3193-1.Williams,M.L.(1964)Structural analysis of Viscoelastic materials.AIAA Journal,785-808.Zou,J.,You F.,Su L.,Yang Z.,Chen G.and Guo S.(2011).Failure Mechanism of Time-Temperature Superposition for Poly(vinyl chloride)/Dioctylphthalate(100/70)System.DOI10.1002/app.35113.Ferry J.D.(1980)Viscoelastic Properties of Polymers,Wiley:New York.Artiaga R.,Garcia A.Fundamentals of DMA.In:’Thermal analysis.Fundamentals and applica-tions to material characterization’(ed.:Artiaga R.)Publicaciones de la Universidade da Coruna,ACoruna,Spain,183-206(2005).Chartoff R.P.,Menczel J.D.,Dillman S.H.Dynamic mechanical analysis(DMA).In:’Thermalanalysis of polymers.Fundamentals and applications’(eds.:Menczel J.D.,Prime R.B.)Wiley,SanJose,387-496(2009).Epoxy Dataset obtained from creep tests of an epoxy based composite by us-ing Dynamic Mechanical Thermal Analysis(DMTA)DescriptionEpoxy is a dataset composed of273rows and3columns that describes the performance of an epoxyresin based composite in a creep type test.This type of laboratory experimental procedure is definedby the application of a constant stress,σ,and the measuring of the strain,ε(t),or compliance,J(t)=ε(t)/σ(in the present case)as the response variable.The experimental tests are made byDynamic Mechanical Thermal Analysis(DMTA)technique,using a3point bending configurationwith the following features:Clamp,3-Point Bending;Geometry,Rectangular;Size:length of20mm,width of5.55mm,and thickness of about0.85mm.FormatThis data frame is composed of the following columns:Log10_time It accounts for39different times from2s to3599s,in logarithmic scale for each one of the7studied temperatures(overall273observations).Log10_Compliance It accounts for39different values for the compliance,J(MPa-1),for each one of the7studied temperatures,in base-ten logarithmic scale.Temperature It is the variable that shows the temperature at which the measurements of compli-ance are experimentally obtained,namely30,40,50,60,70,80,and90Celsius degrees.4PCDetailsThe dataset includes the measurements of the compliance property depending on the time and cor-responding to different specimens of an epoxy resin based composite.All the observations were obtained by the application of the DMTA experimental technique.The application of the TTS prin-ciple to creep tests is becoming more and more common.Creep test provides information about the deformation of a material subjected to a constant load.This test is in accordance with many real applications such as the performance of shoe insoles,structural materials,asphalt,etc.In this framework,TTS provides the degree of the deformation of the material at an extended range of times,when this material is subjected to a constant load.Therefore,TTS is becoming increasingly useful in studies of material degradation and lifetime estimation.The use of the TTS principle with creep tests usually provides smoother master curves since each curve is usually defined by a larger number of experimental observations than,for example,modulus curves as a function of frequency. SourceJaneiro-Arocas,J.,Tarrío-Saavedra,J.,López-Beceiro,J.,Naya,S.,López-Canosa,A.,Heredia-García,N.,and Artiaga,R.(2016).Creep analysis of silicone for podiatry applications.Journal of the Mechanical Behavior of Biomedical Materials,63,456-469.DOI10.1016/j.jmbbm.2016.07.014.Naya,S.,Meneses A,.Tarrio-Saavedra,J,.Artiaga R.,Lopez-Beceiro,J.and Gracia-Fernandez C.(2013)New method for estimating shift factors in time-temperatura superposition models.Journal of Thermal Analysis and Calorimetry.ISSN1388-6150.DOI10.1007/s10973-013-3193-1. Examplesdata(Epoxy)PC Dataset obtained from polycarbonate(polymer)tests using DynamicMechanical Analysis(DMA)DescriptionPC contains49rows and3columns.FormatThis data frame is composed of the following columns:A data frame with49observations on the following3variables:log10.frequency It accounts for seven different frequencies(rad/s)in logarithmic scale for each temperature(overall49).log10.module It accounts for seven different storage modulus,E’(Pa),in base-ten logarithmic scale for each temperature(overall49).temperature Seven different temperatures:147,148,149,150,151,152,153degrees celsius,each one with the corresponding seven values of frequency and storage modulus(overall49).DetailsThe dataset corresponds to the storage modulus viscoelastic property of different specimens of polycarbonate(PC)and obtained by DMA using TA Instruments Q800(Naya et al.,2013). SourceNaya,S.,Meneses A,.Tarrio-Saavedra,J,.Artiaga R.,Lopez-Beceiro,J.and Gracia-Fernandez C.(2013)New method for estimating shift factors in time-temperatura superposition models.Journal of Thermal Analysis and Calorimetry.ISSN1388-6150.DOI10.1007/s10973-013-3193-1. Examplesdata(PC)PLOT.TTS Time-Temperature Superposition(TTS)plotsDescriptionPlots of TTS results:experimental data,horizontal and vertical shifts,TTS data,TTS Master Curve fitting with B-Splines and bootstrap confidence intervals are deployed.UsagePLOT.TTS(x)Argumentsx TTS object.DetailsTTS plots are performed from the outputs of TTS function:data,aT,bT,TTS.data,TTS.gam y residuals.ValueThe following values are returned:PLOT.data()Generic function to plot the experimental data.By default log10.module versus log10.frequency.PLOT.aT()Generic plot of the horizontal shifts corresponding to each curve(modulus ver-sus frequency)obtained on temperature.PLOT.bT()Generic plot of the vertical shifts corresponding to each curve(modulus versus frequency)obtained on temperature.PLOT.TTS.data()Generic plot of the experimental data horizontally and vertically shifted withrespect to a the curve corresponding to the reference temperature.PLOT.TTS.gam()Generic plot of the Master Curve B-splines estimation with bootstrap confidence intervals at95per cent.PLOT.res()Generic plot of the residuals of Master Curve B-splinesfitting.Author(s)Antonio Meneses<********************************>,Salvador Naya<************>and Javier Tarrio-Saavedra<**************>ReferencesNaya,S.,Meneses A.,Tarrio-Saavedra,J.,Artiaga R.,Lopez-Beceiro,J.and Gracia-Fernandez C.(2013)New method for estimating shift factors in time-temperatura superposition models.Journal of Thermal Analysis and Calorimetry.ISSN1388-6150.DOI10.1007/s10973-013-3193-1.Williams,M.L.(1964)Structural analysis of Viscoelastic materials.AIAA Journal,785-808.Artiaga R.,Garcia A.Fundamentals of DMA.In:’Thermal analysis.Fundamentals and applica-tions to material characterization’(ed.:Artiaga R.)Publicaciones de la Universidade da Coruna,A Coruna,Spain,183-206(2005).Examples##TTS object applied to PC dataset.data(PC)Derive<-TTS(PC)x<-Derive##Generic plots for TTS analysisPLOT<-PLOT.TTS(x)names(PLOT)##[1]"PLOT.data""PLOT.aT""PLOT.bT""PLOT.TTS.data"##[5]"PLOT.TTS.gam""PLOT.res"##Generic plots of:data,aT,bT,TTS.data,TTS.gam and resPLOT$PLOT.data(main="PLOT:Data",xlab="log10.Frequency(rad/s)",ylab="log10.E (Pa)") PLOT$PLOT.aT(main="PLOT:horizontal translation factors",xlab="Temperature",ylab="aT") PLOT$PLOT.bT(main="PLOT:vertical translation factors",xlab="Temperature",ylab="bT") PLOT$PLOT.TTS.data(xlab="log10.Frequency(rad/s)",ylab="log10.E (Pa)")PLOT$PLOT.TTS.gam(xlab="log10.Frequency(rad/s)",ylab="log10.E (Pa)",main="Fitted gam,Bootstrap confidence intervals",sub="Reference temperature=150degrees celsius")PLOT$PLOT.res(main="TTS:gam residual",xlab="Fitted",ylab="Standardized residuals")SBS7SBS Dataset obtained from creep tests of styrene-butadiene-styrene(SBS)composite by using Dynamic Mechanical Thermal Analysis(DMTA)DescriptionSBS is a dataset composed of195rows and3columns that describes the performance in the frame-work of a creep test of a styrene-butadiene-styrene(SBS)composite.The creep tests are defined by the application of a constant stress,σ,and the measuring of the strain,ε(t),or compliance, J(t)=ε(t)/σ(in the present case),as the response variable.The experimental tests are made by Dynamic Mechanical Thermal Analysis(DMTA)technique,using a3point bending configuration with the following features:Clamp,3-Point Bending;Geometry,Rectangular;Size:length of20 mm,width of5.54mm,and thickness of about3.87mmm.FormatThis data frame is composed of the following columns:Log10_time It accounts for39different times from2s to3600s,in logarithmic scale for each one of the5studied temperatures(overall195observations).Log10_Compliance It accounts for39different values for the compliance,J(MPa-1),for each one of the5studied temperatures,in base-ten logarithmic scale.Temperature It is the variable that shows the temperature at which the measurements of compli-ance are experimentally obtained,namely40,50,60,70,and80Celsius degrees.DetailsThe dataset corresponds to the measure of the compliance property(with respect to the time)of different specimens of a SBS composite.The measurements were obtained by DMTA experimental technique.The application of the TTS principle to creep tests is becoming more and more common.Creep test provides information about the deformation of a material subjected to a constant load.This test is in accordance with many real applications such as the performance of shoe insoles,structural ma-terials,asphalt,etc.In this framework,TTS provides the degree of the deformation of the material at an extended range of times,when this material is subjected to a constant load.Therefore,TTS is becoming increasingly useful in studies of material degradation and lifetime estimation.The use of the TTS principle with creep tests usually provides smoother master curves since each curve is usually defined by a larger number of experimental observations than,for example,modulus curves as a function of frequency.SourceJaneiro-Arocas,J.,Tarrío-Saavedra,J.,López-Beceiro,J.,Naya,S.,López-Canosa,A.,Heredia-García,N.,and Artiaga,R.(2016).Creep analysis of silicone for podiatry applications.Journal of the Mechanical Behavior of Biomedical Materials,63,456-469.DOI10.1016/j.jmbbm.2016.07.014.8TTSNaya,S.,Meneses A,.Tarrio-Saavedra,J,.Artiaga R.,Lopez-Beceiro,J.and Gracia-Fernandez C.(2013)New method for estimating shift factors in time-temperatura superposition models.Journal of Thermal Analysis and Calorimetry.ISSN1388-6150.DOI10.1007/s10973-013-3193-1. Examplesdata(SBS)TTS Time-Temperature Superposition(TTS)analysisDescriptionThe Master Curve at a specific temperature is estimated using Time-Temperature Superposition (TTS)procedures.The Master Curve means the variation of a specific viscoelastic property of the selected material depending on time or frequency.TTS procedures provide the viscoelastic property variation at the selected temperature in a wider interval of time or frequency than in the experimental case.The Master Curve is estimated for each selected reference temperaure using TTS procedures.Three TTS methodologies are implemented in this package:the two wider used methods,Arrhenius based methods and WLF,and the newer methodology based on derivatives procedure.The Master Curve is smoothed by B-splines basis.UsageTTS(x,reference.temperature=150,n=100,nB=100,method=c("derived","WLF","Arrhenius"))Argumentsx Matrix or data frame composed of three columns:a numeric column vector with the experimental frequencies(in logarithmic scale,base-ten),the modulus(E’or G’)base-ten logarithm vector and,finally the corresponding temperaturesvector.reference.temperatureValue of the selected reference temperatura at which the Master Curve of mod-ulus will be obtained,the default value of temperature is150.n Number of partitions in the frequency domain in order tofit the B-spline basis.The default value is100.nB Number of bootstrap replicates to estimate confidence intervals of master curve fitting.The default is100.method A string vector composed of one of the following options:"derived"(by default), "WLF"and"Arrhenius".TTS9DetailsEl New method for estimating shift factors in time-temperatura superposition models(Naya et al., 2013)opens the possibility to perform the TTS function.The horizontal and vertical shifts are ly,the different methods are differenciated due to the expression for estimating the horizontal shifts,aT.The"derivated"method is based on the application of horizontal shifts to the moduli derivatives(depending on the frequency)and thus obtaining the Master Curve at the selected temperature:(dE’)/dx(x+aT)->(dE’)/dx(x)WLF method is defined by the parametric expression:Log10(aT)=-C1*(T-To)/(C2+(T-To))Where C1and C2are constants to be estimated,T is the temperature and To the reference tempera-ture.Arrhenius method is defined by the parametric expression:Log10(aT)=Ea*((1/T)-(1/To))*log10(2.718282)/RWhere Ea is the activation energy,R=8.314J/mol is the universal gas constant,T is the absolute temperature(Kelvin degrees),and To the reference temperature(Celsius degrees).The vertical shifts,bT,are calculated taking into account the vertical distance between the moduli curves.ValueThe function returns a list composed of the following outputs:data Input experimental data.aT Numerical vector of horizontal shifts between the modulus curves.bT Numerical vector of vertical shifts between the modulus curves.TTS.data Master Curve Data frame defined by three columns:log10frequency,log10module and temperature.ref.temp Reference temperature value.TTS.gam Data frame of the Generalized Additive Model with B-splines(GAM)estimate of the Master Curve.It contains two columns:frequency and Prediction.I.lower Lower limit of bootstrap confidence interval corresponding to the estimated B-splines Master Curve.I.upper Upper limit of bootstrap confidence interval corresponding to the estimated B-splines Master Curve.residuals Residuals corresponding to the GAM with B-splines Master Curvefitting.10TTS_10 Author(s)Antonio Meneses<********************************>,Salvador Naya<************>and Javier Tarrio-Saavedra<**************>ReferencesNaya,S.,Meneses A.,Tarrio-Saavedra,J.,Artiaga R.,Lopez-Beceiro,J.and Gracia-Fernandez C.(2013)New method for estimating shift factors in time-temperatura superposition models.Journal of Thermal Analysis and Calorimetry.ISSN1388-6150.DOI10.1007/s10973-013-3193-1.Williams,M.L.(1964)Structural analysis of Viscoelastic materials.AIAA Journal,785-808.Artiaga R.,Garcia A.Fundamentals of DMA.In:’Thermal analysis.Fundamentals and applica-tions to material characterization’(ed.:Artiaga R.)Publicaciones de la Universidade da Coruna,A Coruna,Spain,183-206(2005).Examples##Polycarbonate datasetdata(PC)x=PC##TTS function applied to polycarbonate.derive=TTS(x,reference.temperature=150,method=c("derived","WLF","Arrhenius"))names(derive)##[1]"data""aT""bT""TTS.data""ref.temp""TTS.gam"##[7]"I.lower""I.upper""residuals"##Horizontal shifts vector of modulus versus frequency curves.derive$aT##Reference temperaturederive$ref.tempTTS_10Time-Temperature Superposition(TTS)analysisDescriptionThis function provides an estimate of the Master Curve in a similar way to the TTS function,with the difference that,in this case,a thin plate splinefit is performed(instead the B-splines smoothing), within the framework of the application of generalized additive models(GAM),as implemented in the mgcv package.TTS_1011UsageTTS_10(x,reference.temperature=40,n=100,nB=100,method=c("derived","WLF","Arrhenius"))Argumentsx Matrix or data frame composed of three columns:a numeric column vectorwith the experimental frequencies(in logarithmic scale,base-ten),the responsevariable(E’or G’modulus,compliance,etc.)base-ten logarithm vector and,finally the corresponding temperatures vector.reference.temperatureValue of the selected reference temperature at which the Master Curve of theresponse variable(E’or G’modulus,compliance,etc.)will be obtained,thedefault value of temperature is40.n Number of partitions in the frequency domain in order tofit the B-spline basis.The default value is100.nB Number of bootstrap replicates to estimate confidence intervals of Master Curvefitting.The default is100.method A string vector composed of one of the following options:"derived"(by default),"WLF"and"Arrhenius".ValueThe function returns a list composed of the following outputs:data Input experimental data.aT Numerical vector of horizontal shifts between the modulus curves.bT Numerical vector of vertical shifts between the modulus curves.TTS.data Master Curve Data frame defined by three columns:Log10_time,Log10_Compliance and Temperature.ref.temp Reference temperature value.TTS.gam Data frame of the Generalized Additive Model with B-splines(GAM)estimateof the Master Curve.It contains two columns:time and Prediction.I.lower Lower limit of bootstrap confidence interval corresponding to the estimated B-splines Master Curve.I.upper Upper limit of bootstrap confidence interval corresponding to the estimated B-splines Master Curve.residuals Residuals corresponding to the GAM with B-splines Master Curvefitting.12TTS_10Author(s)Antonio Meneses<********************************>,Salvador Naya<************>and Javier Tarrio-Saavedra<**************>ReferencesNaya,S.,Meneses A.,Tarrio-Saavedra,J.,Artiaga R.,Lopez-Beceiro,J.and Gracia-Fernandez C.(2013)New method for estimating shift factors in time-temperatura superposition models.Journal of Thermal Analysis and Calorimetry.ISSN1388-6150.DOI10.1007/s10973-013-3193-1.Williams,M.L.(1964)Structural analysis of Viscoelastic materials.AIAA Journal,785-808.Artiaga R.,Garcia A.Fundamentals of DMA.In:’Thermal analysis.Fundamentals and applica-tions to material characterization’(ed.:Artiaga R.)Publicaciones de la Universidade da Coruna,A Coruna,Spain,183-206(2005).Wood,S.N.(2017)Generalized Additive Models:An Introduction with R(2nd edition).Chapman and Hall/CRC.Examples##Epoxydata(Epoxy)x=Epoxy##TTS_10function applied to Epoxy.Q=TTS_10(x,reference.temperature=40,method=c("derived","WLF","Arrhenius"))names(Q)##Horizontal shifts vector of compliance versus time curves.Q$aT##Reference temperatureQ$ref.tempPLOT<-PLOT.TTS(Q)##Generic plots of:data,aT,bT,TTS.data and TTS.gamPLOT$PLOT.data(main="PLOT:Data",xlab="Log_time",ylab="Log_Compliance")PLOT$PLOT.aT(main="PLOT:horizontal shift factors",xlab="Temperature",ylab="aT")PLOT$PLOT.bT(main="PLOT:vertical shift factors",xlab="Temperature",ylab="bT")PLOT$PLOT.TTS.data(xlab="Log_time",ylab="Log_Compliance")PLOT$PLOT.TTS.gam(xlab="Log_time",ylab="Log_Compliance",main="Fitted gam,Bootstrap confidence intervals",sub="Reference temperature=40Celsius degrees")Index∗TTS.PLOT()PLOT.TTS,5∗TTS_10TTS_10,10∗TTSTTS,8∗datasetsEpoxy,3PC,4SBS,7∗packageTTS-package,2Epoxy,3PC,4PLOT.TTS,5SBS,7TTS,8TTS-package,2TTS_10,1013。
[转载]Bootstrapping
![[转载]Bootstrapping](https://img.taocdn.com/s3/m/a914606b178884868762caaedd3383c4bb4cb471.png)
[转载]Bootstrapping关于Bootstrap的专门介绍,很全⾯。
如果能对Bias-corrected bootstrap给予更深⼊的分析对⽐就更好了。
原⽂地址:Bootstrapping作者:招展如桦BootstrappingBootstrapping is the practice of estimating properties of an estimator (such as its variance) by measuring those properties when sampling from an approximating distribution. One standard choice for an approximating distribution is the empirical distribution of the observed data. In the case where a set of observations can be assumed to be from an independent and identically distributed population, this can be implemented by constructing a number of resamples of the observed dataset (and of equal size to the observed dataset), each of which is obtained by random sampling with replacement from the original dataset.It may also be used for constructing hypothesis tests. It is often used as an alternative to inference based on parametric assumptions when those assumptions are in doubt, or where parametric inference is impossible or requires very complicated formulas for the calculation of standard errors.Informal descriptionThe basic idea of bootstrapping is that the sample we have collected is often the best guess we have as to the shape of the population from which the sample was taken. For instance, a sample of observations with two peaks in its histogram would not be well approximated by a Gaussian or normal bell curve, which has only one peak. Therefore, instead of assuming a mathematical shape (like the normal curve or some other) for the population, we instead use the shape of the sample.As an example, assume we are interested in the average (or mean) height of people worldwide. We cannot measure all the people in the global population, so instead we sample only a tiny part of it, and measure that. Assume the sample is of size N; that is, we measure the heights of N individuals. From that single sample, only one value of the mean can be obtained. In order to reason about the population, we need some sense of the variability of the mean that we have computed.To use the simplest bootstrap technique, we take our original data set of N heights, and, using a computer, make a new sample (called a bootstrap sample) that is also of size N. This new sample is taken from the original using sampling with replacement so it is not identical with the original "real" sample. We repeat this a lot (maybe 1000 or 10,000 times), and for each of these bootstrap samples we compute its mean (each of these are called bootstrap estimates). We now have a histogram of bootstrap means. This provides an estimate of the shape of the distribution of the mean from which we can answer questions about how much the mean varies. (The method here, described for the mean, can be applied to almost any other statistic or estimator.)The key principle of the bootstrap is to provide a way to simulate repeated observations from an unknown population using the obtained sample as a basis.Situations where bootstrapping is usefulAdèr et al.[4] recommend the bootstrap procedure for the following situations:When the theoretical distribution of a statistic of interest is complicated or unknown. Since the bootstrapping procedure is distribution-independent it provides an indirect method to assess the properties of the distributionunderlying the sample and the parameters of interest that are derived from this distribution.When the sample size is insufficient for straightforward statistical inference. If the underlying distribution is well-known, bootstrapping provides a way to account for the distortions caused by the specific sample that may not be fully representative of the population.When power calculations have to be performed, and a small pilot sample is available. Most power and sample size calculations are heavily dependent on the standard deviation of the statistic of interest. If the estimate used is[edit ][edit ][edit ][edit ]incorrect, the required sample size will also be wrong. One method to get an impression of the variation of the statistic is to use a small pilot sample and perform bootstrapping on it to get impression of the variance. DiscussionAdvantagesA great advantage of bootstrap is its simplicity. It is straightforward way to derive estimates of standard errors and confidence intervals for complex estimators of complex parameters of the distribution, such as percentile points, proportions, odds ratio,and correlation coefficients. Moreover, it is an appropriate way to control and check the stability of the results.Disadvantages Although bootstrapping is (under some conditions) asymptotically consistent, it does not provide general finite-sampleguarantees. Furthermore, it has a tendency to be overly optimistic.[citation needed ] The apparent simplicity may conceal the fact that important assumptions are being made when undertaking the bootstrap analysis (e.g. independence of samples) where these would be more formally stated in other approaches.Types of bootstrap scheme In univariate problems, it is usually acceptable to resample the individual observations with replacement ("case resampling" below). In small samples, a parametric bootstrap approach might be preferred. For other problems, a smooth bootstrap will likely be preferred.For regression problems, various other alternatives are available.[citation needed ]Case resampling Bootstrap is generally useful for estimating the distribution of a statistic (e.g. mean, variance) without using normal theory (e.g. z-statistic, t-statistic). Bootstrap comes in handy when there is no analytical form or normal theory to help estimate the distribution of the statistics of interest, since bootstrap method can apply to most random quantities, e.g., the ratio of variance and mean. There are at least two ways of performing case resampling.1. The Monte Carlo algorithm for case resampling is quite simple. First, we resample the data with replacement, and the size of the resample must be equal to the size of the original data set. Then the statistic of interest is computed from the resample from the first step. We repeat this routine many times to get a more precise estimate of the Bootstrapdistribution of the statistic.2. The 'exact' version for case resampling is similar, but we exhaustively enumerate every possible resample of the data set. This can be computationally expensive as there are a total of different resamples, where n is the size of the data set.Estimating the distribution of sample mean Consider a coin-flipping experiment. We flip the coin and record whether it lands heads or tails. (Assume for simplicity that there are only two outcomes) Let X = x 1, x 2, …, x 10 be 10 observations from the experiment. x i = 1 if the i th flip lands heads,and 0 otherwise. From normal theory, we can use t-statistic to estimate the distribution of the sample mean, .Instead, we use bootstrap, specifically case resampling, to derive the distribution of . We first resample the data to obtain a bootstrap resample . An example of the first resample might look like this X 1* = x 2, x 1, x 10, x 10, x 3, x 4, x 6, x 7, x 1, x 9. Note that there are some duplicates since a bootstrap resample comes from sampling with replacement from the data. Note also that the number of data points in a bootstrap resample is equal to the number of data points in our original observations. Then we compute the mean of this resample and obtain the first bootstrap mean : µ1*. We repeat this process to obtain the second resample X 2* and compute the second bootstrap mean µ2*. If we repeat this 100 times, then we have µ1*, µ2*, …, µ100*. This represents an empirical bootstrap distribution of sample mean. From this empirical distribution, one can derive a bootstrap confidence interval for the purpose of hypothesis testing.Regression In regression problems, case resampling refers to the simple scheme of resampling individual cases - often rows of a data set . For regression problems, so long as the data set is fairly large, this simple scheme is often acceptable. However, the method is open to criticism [citation needed ].In regression problems, the explanatory variables are often fixed, or at least observed with more control than the response[edit ][edit ][edit ][edit ][edit ][edit ][edit ][edit ]variable. Also, the range of the explanatory variables defines the information available from them. Therefore, to resample cases means that each bootstrap sample will lose some information. As such, alternative bootstrap procedures should be considered.Bayesian bootstrap Bootstrapping can be interpreted in a Bayesian framework using a scheme that creates new datasets through reweighting the initial data. Given a set of data points, the weighting assigned to data point in a new dataset is , where is a low-to-high ordered list of uniformly distributed random numbers on , preceded by 0 and succeeded by 1. The distributions of aparameter inferred from considering many such datasets are then interpretable as posterior distributions on that parameter.[5]Smooth bootstrapUnder this scheme, a small amount of (usually normally distributed) zero-centered random noise is added on to each resampled observation. This is equivalent to sampling from a kernel density estimate of the data.Parametric bootstrap In this case a parametric model is fitted to the data, often by maximum likelihood , and samples of random numbers are drawn from this fitted model. Usually the sample drawn has the same sample size as the original data. Then the quantity, or estimate, of interest is calculated from these data. This sampling process is repeated many times as for other bootstrapmethods. The use of a parametric model at the sampling stage of the bootstrap methodology leads to procedures which are different from those obtained by applying basic statistical theory to inference for the same model.Resampling residualsAnother approach to bootstrapping in regression problems is to resample residuals . The method proceeds as follows.1. Fit the model and retain the fitted values and the residuals .2. For each pair, (x i , y i ), in which x i is the (possibly multivariate) explanatory variable, add a randomly resampledresidual, , to the response variable y i . In other words create synthetic response variables where j is selectedrandomly from the list (1, …, n ) for every i .3. Refit the model using the fictitious response variables y*i , and retain the quantities of interest (often the parameters, ,estimated from the synthetic y*i ).4. Repeat steps 2 and 3 a statistically significant number of times.This scheme has the advantage that it retains the information in the explanatory variables. However, a question arises as to which residuals to resample. Raw residuals are one option; another is studentized residuals (in linear regression). Whilst there are arguments in favour of using studentized residuals; in practice, it often makes little difference and it is easy to run both schemes and compare the results against each other.Gaussian process regression bootstrap When data are temporally correlated, straightforward bootstrapping destroys the inherent correlations. This method uses Gaussian process regression to fit a probabilistic model from which replicates may then be drawn. Gaussian processes are methods from Bayesian non-parametric statistics but are here used to construct a parametric bootstrap approach, which implicitly allows the time-dependence of the data to be taken into account.Wild bootstrap Each residual is randomly multiplied by a random variable with mean 0 and variance 1. This method assumes that the 'true'residual distribution is symmetric and can offer advantages over simple residual sampling for smaller sample sizes.[6]Moving block bootstrap In the moving block bootstrap, n-b+1 overlapping blocks of length b will be created in the following way: Observation 1 to b will be block 1, observation 2 to b+1 will be block 2 etc. Then from these n-b+1 blocks, n/b blocks will be drawn at random with replacement. Then aligning these n/b blocks in the order they were picked, will give the bootstrap observations. This bootstrap works with dependent data, however, the bootstrapped observations will not be stationary anymore byconstruction. But, it was shown that varying the block length can avoid this problem.[7]Choice of statistic[edit ][edit ][edit ][edit ][edit ]The bootstrap distribution of a point estimator of a population parameter has been used to produce a bootstrapped confidence interval for the parameter's true value, if the parameter can be written as a function of the population's distribution .Population parameters are estimated with many point estimators . Popular families of point-estimators include mean-unbiased minimum-variance estimators , median-unbiased estimators , Bayesian estimators (for example, the posterior distribution 's mode , median , mean ), and maximum-likelihood estimators .A Bayesian point estimator and a maximum-likelihood estimator have good performance when the sample size is infinite,according to asymptotic theory . For practical problems with finite samples, other estimators may be preferable. Asymptotic theory suggests techniques that often improve the performance of bootstrapped estimators; the bootstrapping of a maximum-likelihood estimator may often be improved using transformations related to pivotal quantities .[8]Deriving confidence intervals from the bootstrap distribution The bootstrap distribution of a parameter-estimator has been used to calculate confidence intervals for its population-parameter.[citation needed ]Effect of bias and the lack of symmetry on bootstrap confidence intervalsBias : The bootstrap distribution and the sample may disagree systematically, in which case bias may occur.If the bootstrap distribution of an estimator is symmetric, then percentile confidence-interval are often used; such intervals are appropriate especially for median-unbiased estimators of minimum risk (with respect to an absolute loss function ). Bias in the bootstrap distribution will lead to bias in the confidence-interval.Otherwise, if the bootstrap distribution is non-symmetric, then percentile confidence-intervals are often inappropriate.Methods for bootstrap confidence intervalsThere are several methods for constructing confidence intervals from the bootstrap distribution of a real parameter:Percentile Bootstrap . It is derived by using the 2.5 and the 97.5 percentiles of the bootstrap distribution as the limits of the 95% confidence interval. This method can be applied to any statistic. It will work well in cases where the bootstrap distribution is symmetrical and centered on the observed statistic [9] and where the sample statistic is median-unbiased and has maximum concentration (or minimum risk with respect to an absolute value loss function). In other cases, the percentile bootstrap can be too narrow. When working with small sample sizes (i.e., less than 50), the percentile confidence intervals for (for example) the variance statistic will be too narrow. So that with a sample of 20 points, 90%confidence interval will include the true variance only 78% of the time, according to Schenker.[citation needed ]Bias-Corrected Bootstrap - adjusts for bias in the bootstrap distribution.Accelerated Bootstrap - The bootstrap bias-corrected and accelerated (BCa) bootstrap, by Efron (1987),[10] adjusts for both bias and skewness in the bootstrap distribution. This approach is accurate in a wide variety of settings, hasreasonable computation requirements, and produces reasonably narrow intervals.[citation needed ]Basic Bootstrap .Studentized Bootstrap .Example applicationsSmoothed bootstrap In 1878, Simon Newcomb took observations on the speed of light .[11] The data set contains two outliers , which greatly influence the sample mean . (Note that the sample mean need not be a consistent estimator for any population mean ,because no mean need exist for a heavy-tailed distributions .) A well-defined and robust statistic for central tendency is the sample median, which is consistent and median-unbiased for the population median.The bootstrap distribution for Newcomb's data appears below. A convolution-method of regularization reduces thediscreteness of the bootstrap distribution, by adding a small amount of N (0, σ2) random noise to each bootstrap sample. Aconventional choice is for sample size n .[citation needed ]Histograms of the bootstrap distribution and the smooth bootstrap distribution appear below[edit ][edit ][edit ]. The bootstrap distribution of the sample-median has only a small number of values. The smoothed bootstrap distribution has a richer support .In this example, the bootstrapped 95% (percentile) confidence-interval for the population median is (26, 28.5), which is close to the interval for (25.98, 28.46) for the smoothed bootstrap.Relation to other approaches to inference Relationship to other resampling methods The bootstrap is distinguished from :the jackknife procedure, used to estimate biases of sample statistics and to estimate variances, and cross-validation , in which the parameters (e.g., regression weights, factor loadings) that are estimated in one subsample are applied to another subsample.For more details see bootstrap resampling .Bootstrap aggregating (bagging) is a meta-algorithm based on averaging the results of multiple bootstrap samples.U-statisticsMain article: U-statistic In situations where an obvious statistic can be devised to measure a required characteristic using only a small number, r , of data items, a corresponding statistic based on the entire sample can be formulated. Given an r -sample statistic, one can create an n -sample statistic by something similar to bootstrapping (taking the average of the statistic over all subsamples of size r ). This procedure is known to have certain good properties and the result is a U-statistic . The sample mean and sample variance are of this form, for r =1 and r =2.。
Matlab的第三方工具箱全套整合(强烈推荐)

Complex- for estimating temporal and spatial signal complexities
Computational Statistics
Coral- seismic waveform analysis
2007-8-2915:04
#1
littleboy
助理工程师
精华0
积分49
帖子76
水位164
技术分0
JMatLink- Matlab Java classes
Kalman- Bayesian Kalman filter
Kalman Filter- filtering, smoothing and parameter estimation (using EM) for linear dynamical systems
DMsuite- differentiation matrix suite
DMTTEQ- design and test time domain equalizer design methods
DrawFilt- drawing digitaland analog filters
DSFWAV- spline interpolation with Dean wave solutions
FSBOX- stepwise forward and backward selection of features using linear regression
GABLE- geometric algebra tutorial
GAOT- genetic algorithm optimization
bootstrap算法流程

bootstrap算法流程英文回答:Bootstrap Algorithm Procedure.The bootstrap algorithm is a resampling technique used to estimate the sampling distribution of a statistic. It involves repeatedly drawing samples from the original dataset with replacement, calculating the statistic of interest for each sample, and then using these values to estimate the sampling distribution.Procedure:1. Collect data. Obtain a sample of data from the population of interest.2. Resample the data. Draw a new sample of the same size as the original sample from the original sample with replacement. This means that some data points may beselected multiple times, while others may not be selectedat all.3. Calculate the statistic. Compute the statistic of interest for the resampled data.4. Repeat steps 2 and 3. Repeat the resampling and statistic calculation steps B times to obtain B bootstrap samples and B corresponding statistic values.5. Create the bootstrap distribution. The distributionof the B statistic values from the bootstrap samples provides an estimate of the sampling distribution of the statistic.6. Estimate the standard error and confidence intervals. The standard error of the statistic can be estimated from the standard deviation of the bootstrap distribution. Confidence intervals for the true value of the statisticcan be constructed using the percentiles of the bootstrap distribution.中文回答:Bootstrap算法流程。
Bootstrap方法及其在生物学研究中的应用

收稿日期:2009-10-12接受日期:2009-12-06基金项目:安徽省教育厅重点项目(KJ2009A052Z );宿州学院人才基金(2007YSS10)作者简介:赵亮,男,博士,副教授,研究方向:保护遗传学,E-mail :zhaoliang@ioz.ac.cn *通讯作者Corresponding author ,E-mail :lim@ioz.ac.cn Bootstrap 方法及其在生物学研究中的应用赵亮1,3,程锦秀2,许木启3,李明3*(1.安徽宿州学院化学与生命科学系,安徽宿州234000;2.安徽宿州学院继续教育学院,安徽宿州234000;3.中国科学院动物研究所,动物生态与保护遗传学重点实验室,北京100101)摘要:Bootstrap 方法是以原始数据为基础的模拟抽样统计推断法,特别适用于那些难以用常规方法导出的参数的区间估计、假设检验等问题。
本文介绍了该方法的基本思想及具体步骤,并附有生物学研究中应用的实例。
生物科学中有许多数据总体分布信息往往很难确定,难以用常规的方法进行统计分析,因此Bootstrap 方法在生物科学研究中具有很大的应用价值。
关键词:Bootstrap 方法;区间估计;假设检验中图分类号:O212文献标识码:B文章编号:1000-7083(2010)04-0638-04Bootstrap Method and its Application in BiologyZHAO Liang 1,3,CHENG Jin-xiu 2,XU Mu-qi 3,LI Ming 3*(1.Department of Biology ,Suzhou College ,Suzhou ,Anhui Province 234000,China ;2.Department of Continuing Education ,Suzhou College ,Suzhou ,Anhui Province 234000,China ;3.Key Laboratory of Animal Ecology andConservation Biology ,Institute of Zoology ,Chinese Academy of Sciences ,Beijing 100101,China )Abstract :The bootstrap method is a data-based simulation to carry out familiar statistical calculations ,such as confidence intervals estimated ,statistic inference ,et al .By purely computation means rather than using of statistical formulas ,it is useful especially when the statistical formulas are hard to be got.This article introduced the bootstrap method ,including bootstrap basic ideas and procedures and illustrated its application in biology with some examples.Along with the quick de-velopment of computer techniques ,this method is now surging into widely practical use in biological studies.Key words :bootstrap ;confidence intervals estimated ;statistic inference统计推断是从样本资料推断相应的总体特征,包括参数估计和假设检验。
使用Bootstrap方法计算认知诊断评估中的信度
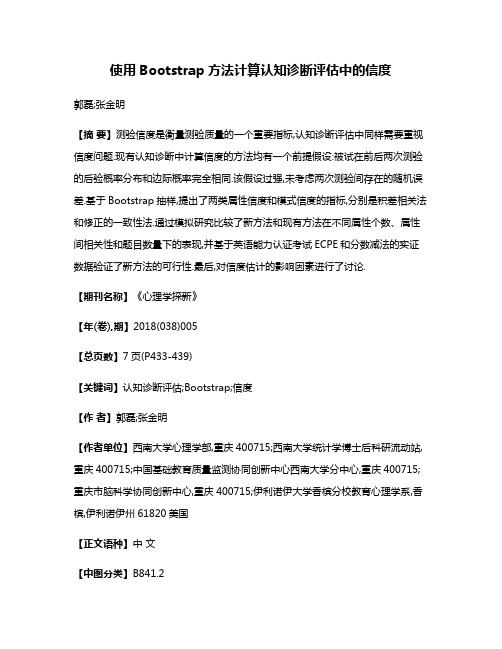
使用Bootstrap方法计算认知诊断评估中的信度郭磊;张金明【摘要】测验信度是衡量测验质量的一个重要指标,认知诊断评估中同样需要重视信度问题.现有认知诊断中计算信度的方法均有一个前提假设:被试在前后两次测验的后验概率分布和边际概率完全相同.该假设过强,未考虑两次测验间存在的随机误差.基于Bootstrap抽样,提出了两类属性信度和模式信度的指标,分别是积差相关法和修正的一致性法.通过模拟研究比较了新方法和现有方法在不同属性个数、属性间相关性和题目数量下的表现,并基于英语能力认证考试ECPE和分数减法的实证数据验证了新方法的可行性.最后,对信度估计的影响因素进行了讨论.【期刊名称】《心理学探新》【年(卷),期】2018(038)005【总页数】7页(P433-439)【关键词】认知诊断评估;Bootstrap;信度【作者】郭磊;张金明【作者单位】西南大学心理学部,重庆400715;西南大学统计学博士后科研流动站,重庆400715;中国基础教育质量监测协同创新中心西南大学分中心,重庆400715;重庆市脑科学协同创新中心,重庆400715;伊利诺伊大学香槟分校教育心理学系,香槟,伊利诺伊州61820美国【正文语种】中文【中图分类】B841.21 引言认知诊断评估(cognitive diagnostic assessment,CDA)已成为国内外测量学研究的关注热点。
CDA优势为不仅能获得被试能力水平,还能诊断其在知识点上的掌握情况。
通过对知识状态的估计,可知晓强项与弱项,指导教师开展针对性的教学补救,实现个性化教学。
由此,认知诊断被视为新一代心理测量理论的核心(涂冬波,蔡艳,丁树良,2012)。
CDA依赖测验进行评估,因此,测验质量决定了评估质量。
测验信度是衡量测验质量的一个重要指标(温忠麟,叶宝娟,2011)。
一个良好的测验,首先应该保证在评价同一批被试时,在不同时间或场合得到的测量结果是一致的。
- 1、下载文档前请自行甄别文档内容的完整性,平台不提供额外的编辑、内容补充、找答案等附加服务。
- 2、"仅部分预览"的文档,不可在线预览部分如存在完整性等问题,可反馈申请退款(可完整预览的文档不适用该条件!)。
- 3、如文档侵犯您的权益,请联系客服反馈,我们会尽快为您处理(人工客服工作时间:9:00-18:30)。
Department of Statistics, University of Ibadan, Ibadan, Nigeria.
* Corresponding author. Address: Department of Statistics, University of Ibadan, Ibadan, Nigeria; E-Mail: eiolamide@ Received: December 26, 2012/ Accepted: April 16, 2013/ Published: April 30, 2013
Progress in Applied Mathematics Vol. 5, No. 2, 2013, pp. [55–63] DOI: 10.3968/j.pam.1925252820130502.1942
ISSN 1925-251X [Print] ISSN 1925-2528 [Online]
Abstract: The Seemingly Unrelated Regressions (SUR) model proposed in 1962 by Arnold Zellner has gained a wide acceptability and its practical use is enormous. In this research, two methods of estimation techniques were examined in the presence of varying degrees of first order Autoregressive [AR(1)] coefficients in the error terms of the model. Data was simulated using bootstrapping approach for sample sizes of 20, 50, 100, 500 and 1000. Performances of Ordinary Least Squares (OLS) and Generalized Least Squares (GLS) estimators were examined under a definite form of the variance-covariance matrix used for estimation in all the sample sizes considered. The results revealed that the GLS estimator was efficient both in small and large sample sizes. Comparative performances of the estimators were studied with 0.3 and 0.5 as assumed coefficients of AR(1) in the first and second regressions and these coefficients were further interchanged for each regression equation, it was deduced that standard errors of the parameters decreased with increase in the coefficients of AR(1) for both estimators with the SUR estimator performing better as sample size increased. Examining the performances of the SUR estimator with varying degrees of AR(1) using Mean Square Error (MSE), the SUR estimator performed better with autocorrelation coefficient of 0.3 than that of 0.5 in both regression equations with best MSE obtained to be 0.8185 using ρ = 0.3 in the second regression equation for sample size of 50. Key words: Autocorrelation; Bootstrapping; Generalized least squares; Ordinary least squares; Seemingly unrelated regressions
55
Bootstrap Approach for Estimating Seemingly Unrelated Regressions with Varying Degrees of Autocorrelated Disturbances
Ebukuyo, O. B., Adepoju, A. A., & Olamide, E. I. (2013). Bootstrap Approach for Estimating Seemingly Unrelated Regressions with Varying Degrees of Autocorrelated Disturbances. Progress in Applied Mathematics, 5 (2), 55–63. Available from http://www. /index.php/pam/article/view/j.pam.1925252820130502.1942 DOI: 10.3968/j. pam.1925252820130502.1942
1. INTRODUCTION
Seemingly Unrelated Regression (SUR) is a system of regression equations which consists of a set of M regression equations, each of which contains different explanatory variables and satisfies the assumptions of the Classical Linear Regression Model (CLRM). The SUR estimation technique which allows for an efficient joint estimation of all the regression parameters was first reported by Zellner [21] which involves the application of Aitken’s Generalised Least Squares (AGLS) [2] to the whole system of equations. Several scholars have also developed other estimators for diverse SUR models to address different situations being examined. Dwivedi and Srivastava [6], Zellner [21] cited in William [18] have shown that the estimation procedure of SUR model was based on Generalized Least Squares (GLS) approach. In answering how much efficiency is gained by using GLS instead of OLS, Zellner [21] has shown in his two-stage approach the gain in efficiency of SUR model over separate equation by equation, that efficiency would be attained when contemporaneous correlation between the disturbances is high and explanatory variables in different equations are uncorrelated. Youssef [19,20] studied the properties of seemingly unrelated regression equation estimators. In an additional paper, he considered a general distribution function for the coefficients of seemingly unrelated regression equations (SURE) model when we unrestricted regression (SUUR) equations. Viraswami [17] presented a working paper on some efficiency results on SURE model. In his work, he considered a two equation seemingly unrelated regressions model in which the equations have some common independent variables and obtained the asymptotic efficiency of the OLS estimator of a parameter of interest relative to its FGLS estimator. He also provided the small-sample relative efficiency of the ordinary least squares estimator and the seemingly unrelated residuals estimator. Alaba et al. [3] recently examined the efficiency gain of the GLS estimator over the Ordinary Least Squares (OLS) estimator. This paper thus examines the performances of OLS and GLS estimators when the disturbances are both autoregressively and contemporaneously correlated. The remainder of the paper is organized as follows. In section 2, the parametric SUR framework is presented while the simulation studies carried out in the work is discussed in Section 3. Results and detailed discussions are presented in Section 4 while Section 5 gives some concluding remarks.