sysAnalysis-cp04-1.0l
PetroMod_2012_2_Installation_Guide
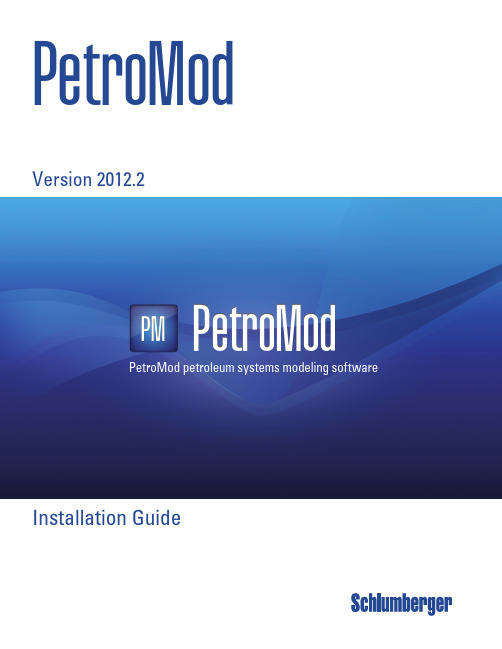
Installation GuideVersion 2012.2PetroModPetroMod petroleum systems modeling software PetroModPM*Mark of SchlumbergerCopyright © 2012 Schlumberger. All rights reserved.Copyright © 2012 Schlumberger. All rights reserved.This work contains the confidential and proprietary trade secrets of Schlumberger and may not be copied or stored in an information retrieval system, transferred, used, distributed, translated or retransmitted in any form or by any means, electronic or mechanical, in whole or in part, without the express written permission of the copyright owner.Trademarks & Service MarksSchlumberger, the Schlumberger logotype, and other words or symbols used to identify the products and services described herein are either trademarks, trade names or service marks of Schlumberger and its licensors, or are the property of their respective owners. These marks may not be copied, imitated or used, in whole or in part, without the express prior written permission of Schlumberger. In addition, covers, page headers, custom graphics, icons, and other design elements may be service marks, trademarks, and/or trade dress of Schlumberger, and may not be copied, imitated, or used, in whole or in part, without the express prior written permission of Schlumberger. Other company, product, and service names are the properties of their respective owners.An asterisk (*) is used throughout this document to designate a mark of Schlumberger.iv PetroMod 2012.2 Installation GuideContents1 Information Resources . . . . . . . . . . . . . . . . . . . . . . . . . . . . . . . . . . . . . . . . . . . . . . . . . . . . .1-1Schlumberger Product Documentation . . . . . . . . . . . . . . . . . . . . . . . . . . . . . . . . . . . . . . . . . . .1-2 About Schlumberger . . . . . . . . . . . . . . . . . . . . . . . . . . . . . . . . . . . . . . . . . . . . . . . . . . . .1-2 Documentation . . . . . . . . . . . . . . . . . . . . . . . . . . . . . . . . . . . . . . . . . . . . . . . . . . . . . . . .1-2 Typestyle Conventions . . . . . . . . . . . . . . . . . . . . . . . . . . . . . . . . . . . . . . . . . . . . . . . . . . .1-2 Alert Statements . . . . . . . . . . . . . . . . . . . . . . . . . . . . . . . . . . . . . . . . . . . . . . . . . . . . . . .1-2 Contacting Schlumberger . . . . . . . . . . . . . . . . . . . . . . . . . . . . . . . . . . . . . . . . . . . . . . . . . . . .1-3 Technical Support . . . . . . . . . . . . . . . . . . . . . . . . . . . . . . . . . . . . . . . . . . . . . . . . . . . . . .1-32 Getting Started . . . . . . . . . . . . . . . . . . . . . . . . . . . . . . . . . . . . . . . . . . . . . . . . . . . . . . . . . . .2-1Overview . . . . . . . . . . . . . . . . . . . . . . . . . . . . . . . . . . . . . . . . . . . . . . . . . . . . . . . . . . . . . . .2-2 Introduction . . . . . . . . . . . . . . . . . . . . . . . . . . . . . . . . . . . . . . . . . . . . . . . . . . . . . . . . . .2-2 Audience . . . . . . . . . . . . . . . . . . . . . . . . . . . . . . . . . . . . . . . . . . . . . . . . . . . . . . . . . . . .2-2 System Requirements . . . . . . . . . . . . . . . . . . . . . . . . . . . . . . . . . . . . . . . . . . . . . . . . . . .2-2 Licensing . . . . . . . . . . . . . . . . . . . . . . . . . . . . . . . . . . . . . . . . . . . . . . . . . . . . . . . . . . . .2-43 Installation (Windows) . . . . . . . . . . . . . . . . . . . . . . . . . . . . . . . . . . . . . . . . . . . . . . . . . . . . .3-1Downloading the Installation Package . . . . . . . . . . . . . . . . . . . . . . . . . . . . . . . . . . . . . . . . . . .3-2 Installing PetroMod . . . . . . . . . . . . . . . . . . . . . . . . . . . . . . . . . . . . . . . . . . . . . . . . . . . . . . . .3-3 Installing PetroMod . . . . . . . . . . . . . . . . . . . . . . . . . . . . . . . . . . . . . . . . . . . . . . . . . . . . .3-3 Files Installed During Installation . . . . . . . . . . . . . . . . . . . . . . . . . . . . . . . . . . . . . . . . . . .3-6 Optional: Installing Runtime Environment for Parallel Processing . . . . . . . . . . . . . . . . . . . . . . . .3-7 Installing Runtime Environment . . . . . . . . . . . . . . . . . . . . . . . . . . . . . . . . . . . . . . . . . . . .3-7 Activating Parallel Processing in the PetroMod Simulation Interface . . . . . . . . . . . . . . . . . . .3-7 Installing the Licensing Tool . . . . . . . . . . . . . . . . . . . . . . . . . . . . . . . . . . . . . . . . . . . . . . . . . .3-9 CodeMeter . . . . . . . . . . . . . . . . . . . . . . . . . . . . . . . . . . . . . . . . . . . . . . . . . . . . . . . . . . .3-9 Schlumberger Licensing Tool . . . . . . . . . . . . . . . . . . . . . . . . . . . . . . . . . . . . . . . . . . . . . 3-10 Configuring the PetroMod License . . . . . . . . . . . . . . . . . . . . . . . . . . . . . . . . . . . . . . . . . . . . . 3-13 Before you start . . . . . . . . . . . . . . . . . . . . . . . . . . . . . . . . . . . . . . . . . . . . . . . . . . . . . . 3-13 Obtaining the license . . . . . . . . . . . . . . . . . . . . . . . . . . . . . . . . . . . . . . . . . . . . . . . . . . . 3-13 Activating a local license . . . . . . . . . . . . . . . . . . . . . . . . . . . . . . . . . . . . . . . . . . . . . . . . 3-14 Activating an external license server . . . . . . . . . . . . . . . . . . . . . . . . . . . . . . . . . . . . . . . . 3-15 Checking the License Status . . . . . . . . . . . . . . . . . . . . . . . . . . . . . . . . . . . . . . . . . . . . . . 3-16Contents v4 Uninstalling PetroMod (Windows) . . . . . . . . . . . . . . . . . . . . . . . . . . . . . . . . . . . . . . . . . . . .4-1Uninstalling PetroMod (Windows) . . . . . . . . . . . . . . . . . . . . . . . . . . . . . . . . . . . . . . . . . . . . . .4-2 Before You Begin . . . . . . . . . . . . . . . . . . . . . . . . . . . . . . . . . . . . . . . . . . . . . . . . . . . . . . .4-2 Uninstalling PetroMod . . . . . . . . . . . . . . . . . . . . . . . . . . . . . . . . . . . . . . . . . . . . . . . . . . .4-2 Results of the Uninstallation Process . . . . . . . . . . . . . . . . . . . . . . . . . . . . . . . . . . . . . . . . .4-25 Installation (Unix) . . . . . . . . . . . . . . . . . . . . . . . . . . . . . . . . . . . . . . . . . . . . . . . . . . . . . . . . .5-1Downloading the Installation Package . . . . . . . . . . . . . . . . . . . . . . . . . . . . . . . . . . . . . . . . . . .5-2 Installing PetroMod . . . . . . . . . . . . . . . . . . . . . . . . . . . . . . . . . . . . . . . . . . . . . . . . . . . . . . . .5-3 Installing PetroMod . . . . . . . . . . . . . . . . . . . . . . . . . . . . . . . . . . . . . . . . . . . . . . . . . . . . .5-3 Files Installed During Installation . . . . . . . . . . . . . . . . . . . . . . . . . . . . . . . . . . . . . . . . . . .5-4 Parallel Processing Set-up (Systems Admin) . . . . . . . . . . . . . . . . . . . . . . . . . . . . . . . . . . . . . . .5-6 Intel MPI runtime . . . . . . . . . . . . . . . . . . . . . . . . . . . . . . . . . . . . . . . . . . . . . . . . . . . . . .5-6 PetroMod Machine Files . . . . . . . . . . . . . . . . . . . . . . . . . . . . . . . . . . . . . . . . . . . . . . . . . .5-6 Configuring users for ssh . . . . . . . . . . . . . . . . . . . . . . . . . . . . . . . . . . . . . . . . . . . . . . . . .5-7 Configuring users for rsh . . . . . . . . . . . . . . . . . . . . . . . . . . . . . . . . . . . . . . . . . . . . . . . . .5-7 Activating Parallel Processing in PetroMod . . . . . . . . . . . . . . . . . . . . . . . . . . . . . . . . . . . . .5-8 Load Sharing Facility (LSF) in Conjunction with Parallel PetroMod . . . . . . . . . . . . . . . . . . . . . . .5-9 Editing the MPI location . . . . . . . . . . . . . . . . . . . . . . . . . . . . . . . . . . . . . . . . . . . . . . . . . .5-9 Intel MPI Settings . . . . . . . . . . . . . . . . . . . . . . . . . . . . . . . . . . . . . . . . . . . . . . . . . . . . . .5-9 Running Parallel PetroMod with Queuing Systems . . . . . . . . . . . . . . . . . . . . . . . . . . . . . . . 5-10 Running PetroMod Software with LSF . . . . . . . . . . . . . . . . . . . . . . . . . . . . . . . . . . . . . . . 5-10 Configuring the License . . . . . . . . . . . . . . . . . . . . . . . . . . . . . . . . . . . . . . . . . . . . . . . . . . . . 5-12 Setting the Environment . . . . . . . . . . . . . . . . . . . . . . . . . . . . . . . . . . . . . . . . . . . . . . . . . 5-12 Obtaining a License Key . . . . . . . . . . . . . . . . . . . . . . . . . . . . . . . . . . . . . . . . . . . . . . . . . 5-12 Setting up the License Server (Systems Administrator) . . . . . . . . . . . . . . . . . . . . . . . . . . . 5-136 Uninstalling PetroMod (Unix) . . . . . . . . . . . . . . . . . . . . . . . . . . . . . . . . . . . . . . . . . . . . . . . .6-1Uninstalling PetroMod (Unix) . . . . . . . . . . . . . . . . . . . . . . . . . . . . . . . . . . . . . . . . . . . . . . . . .6-2 Before You Begin . . . . . . . . . . . . . . . . . . . . . . . . . . . . . . . . . . . . . . . . . . . . . . . . . . . . . . .6-2 Uninstalling PetroMod . . . . . . . . . . . . . . . . . . . . . . . . . . . . . . . . . . . . . . . . . . . . . . . . . . .6-2 Files Removed During Uninstallation . . . . . . . . . . . . . . . . . . . . . . . . . . . . . . . . . . . . . . . . .6-2vi PetroMod 2012.2 Installation Guide1Information ResourcesIn This SectionSchlumberger Product Documentation.........................................................1-2About Schlumberger.............................................................................1-2Documentation.....................................................................................1-2Typestyle Conventions..........................................................................1-2Alert Statements..................................................................................1-2 Contacting Schlumberger............................................................................1-3Technical Support.................................................................................1-3Information Resources 1-1Schlumberger Product Documentation1-2PetroMod 2012.2 Installation GuideSchlumberger Product DocumentationAbout Schlumberger Schlumberger is the leading oilfield services provider, trusted to deliver superiorresults and improved E&P performance for oil and gas companies around the world.Through our well site operations and in our research and engineering facilities, wedevelop products, services, and solutions that optimize customer performance in asafe and environmentally sound manner.Documentation Documentation is provided in the following electronic formats via the listed location:•PetroMod 2012.2 Installation Guide (Adobe ® Acrobat ® PDF file):https://•PetroMod 2012.2 User Guides (Adobe ® Acrobat ® PDF files):https://•Online help for some applications: PetroMod -> HelpYou must have Adobe ® Reader ® installed to read the PDF files. Adobe Readerinstallation programs for common operating systems are available for a freedownload from the Adobe Web site at .Typestyle Conventions The following conventions are observed throughout this guide:•Bold text is used to designate file and folder names, dialog titles, names ofbuttons, icons, and menus, and terms that are objects of a user selection.•Italic text is used for word emphasis, defined terms, and manual titles.•Monospace text (Courier ) is used to show literal text as you would enter it, or asit would appear onscreen.Alert Statements The alerting statements are Notes, Cautions, and Warnings. These statements areformatted in the following style:• • • • • •Note:Information that is incidental to the main text flow, or to an important pointor tip provided in addition to the previous statement or instruction.• • • • • •Caution:Advises of machine or data error that could occur should the user fail totake or avoid a specified action.• • • • • •Warning:Requires immediate action by the user to prevent actual loss of data orwhere an action is irreversible, or when physical damage to themachine or devices is possible.Contacting SchlumbergerInformation Resources 1-3Contacting SchlumbergerTechnical Support Schlumberger has sales and support offices around the world. For information oncontacting Schlumberger, please refer to the information below.For Technical Support for PetroMod software please contact the Customer CareCenter via the Schlumberger Support Portal at https://Internet Postal Mail SchlumbergerAachen Technology Center (AaTC)Ritterstr . 2352072 Aachen - GermanyContacting Schlumberger1-4PetroMod 2012.2 Installation Guide2Getting StartedIn This ChapterOverview...................................................................................................2-2Introduction.........................................................................................2-2System Requirements...........................................................................2-2System Requirements...........................................................................2-2Licensing.............................................................................................2-4Getting Started 2-1Overview2-2PetroMod 2012.2 Installation GuideOverviewIntroduction This document describes the steps necessary to install PetroMod* 2012.2. Theinstaller includes a full PetroMod installation.•Installing on a workstation using a local license•Installing on a workstation using a license on a central license serverThis guide also explains the procedures required after installation:•Defining your license environmentThis module has been designed by the Schlumberger Aachen Technology Center(AaTC), Germany.Note:The screen shots in this document show PetroMod 2012.1.Audience This guide is useful for the following people:•PetroMod users who install PetroMod on their workstations•System Administrator who installs PetroMod on a network shared diskSystem Requirements Before you install PetroMod 2012.2 your machine must meet the followingrequirements:Hardware Requirements Table 2-1Hardware Requirements for workstation (e.g. Dell T7500)Table 2-2Hardware Requirements for laptop (e.g. Dell M4600)Computer CPU 2 x Intel X5667 (quad core) or X5675 (hexa core)Physical Memory12 GB RAM Hard Disk Space 5 GB of free disk space Graphic CardNvidia Quadro 5000 Network Card 1000 Mbit NicComputerIntel Core i7-2860Q 2.5 GHz Physical Memory 16 GB RAMOverview Getting Started 2-3Table 2-3Hardware Requirements for Linux Cluster • • • • • •Warning:Linux Users - Due to known issues concerning the instability ofOpenGL graphics, PetroMod only supports local rendering on 3Dgraphic cards with stable graphic drivers. Rendering via a networkcould cause stability issues. In particular, we observed problems withthe Mesa OpenGL package that is delivered with RHEL5 and works asa fall-back when no other driver is installed.Software Requirements Table 2-4Software Requirements• • • • • •Caution:It is possible that graphics do not display correctly when using older ATIgraphics cards and drivers. Thus, we recommend the use of Nvidiagraphics cards. We also recommend to install the latest graphicsdrivers available from Nvidia to avoid OpenGL graphic display errors.The driver version that comes with the OS in most cases is quite old oreven generic drivers are used if the graphics hardware is notrecognized correctly during the installation of the OS. These driversonly support basic functionalities and do not offer the OpenGL featuresrequired by PetroMod. Please be aware that most onboard graphicshardware does not support OpenGL at all. A dedicated graphics card isrequired for PetroMod.Hard Disk Space5 GB of free disk space Graphic Card Nvidia Quadro 2000M Computer CPU 2 x Intel X5667 (quad core) or X5675 (hexa core)Physical Memory48GB RAM Network Card1000 Mbit Nic Hard Disk Space 5 GB of free disk spaceMicrosoft Vista64-bit Microsoft Windows 7(recommended)64-bit RedHat EnterpriseLinux 5.3(recommended)64 bit Framework 2.0Overview••••••Note:Software applications created under older operating system versions willrun under newer operating system versions, but not vice versa. Licensing Licenses are required to access PetroMod. Certain functionalities or modules willonly be available with the respective licenses. Contact your Schlumberger SISCustomer Support representative to obtain the necessary licensesMaintenance contracts are usually yearly contracts, renewed at any time during theyear. Prior to 2012.1, PetroMod licenses allowed you to step up to a new PetroModversion based on the PetroMod license expiration date without having a validmaintenance contract. Beginning with 2012.1, upgrades are based on yourmaintenance contract expiration date. This is how you read the new licensingformat:FEATURE petrobuilder3D slbsls <yyyy.mm> <dd-mmm-yyyy> <#>Where• <yyyy.mm> is the maintenance expiration year and month• <dd-mmm-yyyy> is the license expiration day, month, year• <#> is the number of licensesMaintenance renewal is required to run any PetroMod version released after yourmaintenance expiration date. You will be automatically contacted by SchlumbergerInformation Systems before your maintenance expires.2-4PetroMod 2012.2 Installation Guide3Installation (Windows)In This ChapterDownloading the Installation Package..........................................................3-2Installing PetroMod.....................................................................................3-3Installing PetroMod...............................................................................3-3Files Installed During Installation...........................................................3-6 Optional: Installing Runtime Environment for Parallel Processing....................3-7Installing Runtime Environment.............................................................3-7Activating Parallel Processing in the PetroMod Simulation Interface..........3-7 Installing the Licensing Tool........................................................................3-9CodeMeter...........................................................................................3-9Schlumberger Licensing Tool................................................................3-10 Configuring the PetroMod License...............................................................3-13Before you start..................................................................................3-13Obtaining the license...........................................................................3-13Activating a local license......................................................................3-14Activating an external license server.....................................................3-15Checking the License Status.................................................................3-16Installation (Windows)3-1Downloading the Installation PackageDownloading the Installation PackageTo install PetroMod, you need the installation package. If you have a DVD, you canuse it. Otherwise, download PetroMod from the Software Download Center.••••••Note:If you are a new user of the Software Download Center, you must registerbefore you can download PetroMod.To download PetroMod 2012 from the Software Download Center1Go to .2Click SIS Software download center.3Log in to the site.4On the Welcome Message page, click Continue.5In the Product Group Name list (in the upper-left corner), click Geology &Geophysics.6In the table on the right, click PetroMod.7In the table of PetroMod downloads, click the Download icon for the PetroMod2012.2 file you need.You are ready to install PetroMod 2012.3-2PetroMod 2012.2 Installation GuideInstalling PetroModInstallation (Windows)3-3Installing PetroModPerform the following tasks prior to beginning the installation:•Ensure that you have admin privileges on the machine on which you are installing PetroMod and/or install the software together with your systems administrator since superuser passwords are required.•Ensure that the “System Requirements” on page 2-2 are met.PetroMod 2012 is a full installation. If you are already using an earlier PetroMod release, copy the new release into a new directory! Do not install the new version ‘over’ the old version to ensure that all programs and files can be updated and will then be compatible.Installing PetroMod The installation ensures that the files required to run PetroMod are installed on yourcomputer.To Install PetroMod 20121Insert the DVD or navigate to the location where you downloaded theinstallation files.2Double-click PetroMod2012.2.exe to start the installation. The folderPetroMod2012.2.msi will be unpacked, then the InstallShield Wizard will open, see Fig. 3-1. Click Next .Fig. 3-1PetroMod InstallShield Wizard3Fill in your User Name and Organization , see Fig. 3-2, then click Next.Installing PetroMod3-4PetroMod 2012.2 Installation GuideFig. 3-2Filling in user name and organization 4Determine the location of the files, see Fig. 3-3. Default is a folder calledSchlumberger in your Program Files folder. If this is not what you want you need to change that manually by clicking the Change button.When you are content with the location, click Next.Fig. 3-3Determining the location of the files 5 A summary of the settings will be displayed, see Fig. 3-4. Click Install .Installing PetroModInstallation (Windows)3-5Fig. 3-4Summary of settings 6You can follow the progress of the installation in the InstallShield Wizard, seeFig. 3-5.Fig. 3-5Installation progress 7Once the installation is complete the InstallShield Wizard will display the finaldialog, see Fig. 3-6. Click Finish .Installing PetroMod3-6PetroMod 2012.2 Installation Guide Fig. 3-6Installation complete 8The PetroMod 2012.2 icon will appear on your desktop . PetroMod 2012.2 willalso be added to the Schlumberger folder in the Progams list of your Start menu.Proceed with the installation of the .NETruntime environment, the MS HPC runtime for parallel processing and / or with installing Flexnet.Files Installed During Installation The following files / folders are installed during the installation of PetroMod:•one folder: PetroMod 2012.2:-client folder incl. sub folders/files-cult folder incl. sub folders/files-def folder incl. sub folders/files-doc folder incl. sub folders/files-geo folder incl. sub folders/files-well folder incl. sub folders/files-WIN64 folder incl. sub folders/files-PetroMod2012.2.batOptional: Installing Runtime Environment for Parallel Processing Optional: Installing Runtime Environment for Parallel ProcessingPetroMod 2012.2 supports parallel processing on Windows platforms usingMicrosoft HPC Pack 2008 R2 SP3. Using parallel processing from the SimulationInterface Microsoft requires the previous installation of Microsoft HPC runtimeenvironment.Installing Runtime Environment You can find the files in the installation package in the RuntimeEnvironment/ Windows folder:•mpi_x64.exe - MS MPI runtime•HpcClient_x64.exe - MS HPC web interface (optional)Once you have installed the files you need to obtain and activate the necessary licenses.••••••Note:If you want to consolidate your existing MS HPC runtime environments (installed with PM 11 or PetroMod 2011) to the latest version that shipswith PetroMod 2012.2, then you have to uninstall the MS HPC Pack 2008SDK on your system and manually set the required environment variable(called CCP_SDK) to C:\Program Files|Microsoft HPC Pack 2008 R2.Otherwise, PetroMod 2011.1 or PetroMod 11 SP4 will complain about themissing MPI runtime environment.Activating Parallel Processing in the PetroMod Simulation Interface 1After the licenses have been activated open the PetroMod Simulation Interface and select Processors for Parallel Run, see Fig. 3-7.Fig. 3-7Activating parallel processing in the PetroMod Simulation InterfaceOptional: Installing Runtime Environment for Parallel Processing2Increase the number of processors in the Processors Selection dialog.••••••Caution:Parallel processing is only supported on your local machine. Youcannot run a simulation on several nodes (as you could on Linuxclusters).Installing the Licensing Tool Installing the Licensing ToolPetroMod 2012 supports the use of the CodeMeter dongle as well as HOSTIDs forlicense authentication.•If you use CodeMeter, you must install CodeMeter software prior to installing theSchlumberger Licensing tool.•If you use HOSTIDs, you can continue by installing the Schlumberger Licensingtool.CodeMeter PetroMod 2012 uses the CodeMeter dongle for license authentication. Before youinsert your CodeMeter dongle into a USB port on your local workstation or on acentral license server, you must install the CodeMeter software. You should useCodeMeter 4.40 in the following circumstances:•If you use a local license (that is, your local workstation is your local licenseserver), install the CodeMeter dongle, appropriate CodeMeter driver, and theSchlumberger Licensing tool on your computer.•If you use a license on a central license server, the Administrator installs theCodeMeter dongle, CodeMeter driver, and the Schlumberger Licensing tool on theserver. Individual users do not need to install any licensing hardware or softwareon their computers.Installing CodeMeter Follow the steps in this section to uninstall previous versions of CodeMeter, andinstall the version required for this PetroMod release.◆To uninstall previous versions of CodeMeterIf you have an older version of CodeMeter installed on your computer, uninstall itand then install the latest version.1If you want to check the version of CodeMeter you are currently using beforeuninstalling it, on the Windows toolbar right-click the CodeMeter icon and selectAbout to open the About CodeMeter window.2Remove your CodeMeter dongle from the USB port.3Select Start > Control Panel > Programs and Features.4On the list of currently installed programs, select CodeMeter Runtime Kit vx.x.5Click Remove.You are ready to install the latest CodeMeter version.◆To install Codemeter••••••Note:CodeMeter automatically installs in your default Program Files directory:%program files%/Codemeter (usually C:/Program Files).1From the Licensing folder in the PetroMod installation package run the correctprogram (.exe) file:Installing the Licensing Tool•For PetroMod 64-bit, run CodeMeterRuntime64.exeAlternatively, go to the CodeMeter website () anddownload CodeMeter 4.40 from their website.The CodeMeter installation wizard opens.2On the Welcome panel, click Next.3On the License Agreement panel, read the agreement, select I accept thelicense agreement, and then click Next.4On the User Information panel, enter your name, your company’s name, andwho will use CodeMeter on your computer, and then click Next.5On the Select Features panel, select the features you want to install and thenclick Next.Schlumberger recommends that you accept the default settings.6On the Ready to Install panel, click Next to begin the installation.7When the installation is complete, on the last panel click Finish.8Restart your computer.The CodeMeter icon appears in your Windows taskbar. When you insert yourCodeMeter dongle into the USB port, the icon changes to show that your computerrecognizes the dongle. If you double-click the icon, you can view information aboutthe dongle.You are ready to install the Schlumberger Licensing tool.Schlumberger Licensing Tool PetroMod uses FlexNet for licensing. The Schlumberger Licensing tool is a simple interface for FlexNet. Using the Schlumberger Licensing 2012 tool to configure and manage your PetroMod license is recommended, but you may use FlexNet tools instead.If you do not already have the Schlumberger licensing tool on your computer, install the Licensing tool as follows:•If you use a local license (that is, your local workstation is your local license server), install the licensing tool on your computer.•If you use a license on a central license server, the Administrator installs the licensing tool on the server. The Schlumberger Licensing tool is not required for the Administrator to install, configure, and manage the PetroMod license. The Administrator may choose to manage PetroMod licenses with FlexNet native tools.The computer that you use to run the Schlumberger licensing tool is the license server for your PetroMod 2012 installaton.Installing the Schlumberger Licensing Tool Follow the steps in this section to install the Schlumberger Licensing tool. If you have a previous version of the licensing tool and want the latest version (2012), uninstall the older version first, and then install the latest version.。
Analyst software软件操作指南2A-1Tutorial forManual MS_MS
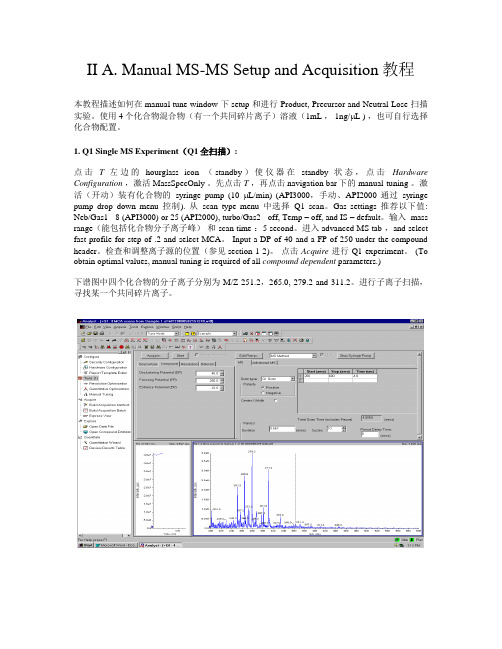
II A. Manual MS-MS Setup and Acquisition教程本教程描述如何在manual tune window 下setup 和进行 Product, Precursor and Neutral Lose 扫描实验。
使用4个化合物混合物(有一个共同碎片离子)溶液(1mL , 1ng/μL ) ,也可自行选择化合物配置。
1. Q1 Single MS Experiment(Q1全扫描):点击T左边的hourglass icon (standby)使仪器在standby状态,点击Hardware Configuration,激活MassSpecOnly 。
先点击T,再点击navigation bar下的 manual-tuning 。
激活(开动)装有化合物的syringe pump (10 μL/min) (API3000,手动、API2000 通过syringe pump drop down menu控制). 从scan type menu中选择Q1 scan。
Gas settings 推荐以下值: Neb/Gas1 - 8 (API3000) or 25 (API2000), turbo/Gas2 - off, Temp – off, and IS – default。
输入 mass range(能包括化合物分子离子峰)和 scan time :5 second。
进入advanced MS tab ,and select fast profile for step of .2 and select MCA。
Input a DP of 40 and a FP of 250 under the compound header。
检查和调整离子源的位置(参见section 1-2)。
点击Acquire进行 Q1 experiment。
(To obtain optimal values, manual tuning is required of all compound dependent parameters.)下谱图中四个化合物的分子离子分别为 M/Z 251.2,265.0, 279.2 and 311.2。
broadcom 驱动 linux 释放说明 14.0.326.25-14.0.326.14说明书
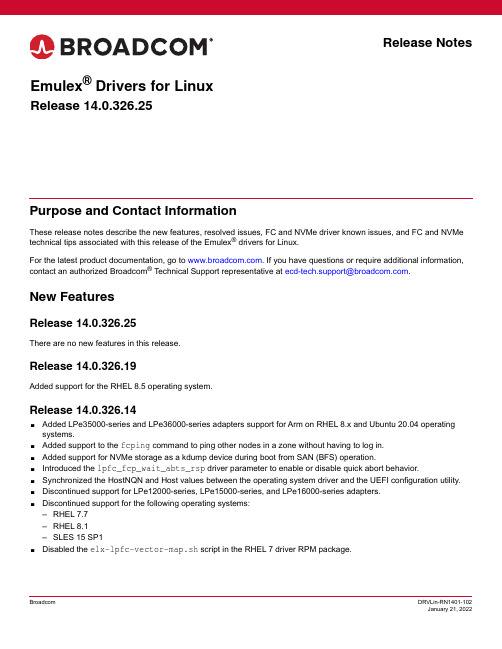
Release NotesPurpose and Contact InformationThese release notes describe the new features, resolved issues, FC and NVMe driver known issues, and FC and NVMe technical tips associated with this release of the Emulex ® drivers for Linux.For the latest product documentation, go to . If you have questions or require additional information, contact an authorized Broadcom ® Technical Support representative at *****************************.New FeaturesRelease 14.0.326.25There are no new features in this release.Release 14.0.326.19Added support for the RHEL 8.5 operating system.Release 14.0.326.14⏹Added LPe35000-series and LPe36000-series adapters support for Arm on RHEL 8.x and Ubuntu 20.04 operating systems. ⏹Added support to the fcping command to ping other nodes in a zone without having to log in. ⏹Added support for NVMe storage as a kdump device during boot from SAN (BFS) operation. ⏹Introduced the lpfc_fcp_wait_abts_rsp driver parameter to enable or disable quick abort behavior. ⏹Synchronized the HostNQN and Host values between the operating system driver and the UEFI configuration utility. ⏹Discontinued support for LPe12000-series, LPe15000-series, and LPe16000-series adapters. ⏹Discontinued support for the following operating systems: –RHEL 7.7–RHEL 8.1 –SLES 15 SP1⏹Disabled the elx-lpfc-vector-map.sh script in the RHEL 7 driver RPM package.Emulex ® Drivers for LinuxRelease 14.0.326.25⏹The following changes were made to NVMe:–Added support to create the HostNQN and HostID using the system UUID.–Discontinued support for creating a HostNQN based on the adapter WWPN.–Added support for reading, writing, and dumping EFI variables NvmeHostNQN and NvmeHostID.–Added support for the NVMe BFS kit on SLES 15 SP3 and RHEL 8.4 operating systems.NOTE:Since the nvmefc-dm features are now inbox in SLES 15 SP3, you do not need to use the NVMe BFS kit on SLES15 SP3 to BFS. However, if the operating system installer determines that the system UUID is not random enoughand silently generates a new UUID, you must use the NVMe BFS kit to resolve the issue.Resolved IssuesRelease 14.0.326.25On the SLES 12 SP2 operating system with a multiport HBA, using FC inbox driver version 12.8.0.10 or FC out-of-box driver 14.0.326.x, the issue of targets being discovered on only one port is resolved.Release 14.0.326.19There are no resolved issues in this release.Release 14.0.326.14⏹On the CentOS 8 operating system, the driver installer script no longer fails to determine the distribution and version.⏹HBA reset no longer causes the congestion buffer to be reset. Both congestion buffer information and statistics arepreserved across reset.FC Driver Known Issues1.On Red Hat Virtualization (RHV) 4.4, dracut does not add the lpfc driver configuration file to initramfs.Workarounda.Edit the /usr/lib/dracut/dracut.conf.d/02-generic-image.conf file.b.Add a # before the hostonly=no string to comment out the entry.c.Rebuild the initramfs using dracut.2.On RHEL 8.4 inbox drivers, diagnostic loopback tests always fail and might result in a timeout error.WorkaroundInstall any RHEL 8.4 supported out-of-box driver version.3.There is a change to the value specified for the driver parameter lpfc_log_verbose in Step 5 in Section 2.5 Updatingthe Firmware. For details, refer to the Emulex Drivers for Linux User Guide4.An unrecoverable operating system fault might occur when generating a crash dump after successful installation of theSLES 15 SP2 operating system on a local device or an FC BFS LUN.WorkaroundInstall the SUSE maintenance update dracut-049.1+suse.183.g7282fe92-3.18.2.x86_64.rpm orlater.Release 14.0.326.25Release 14.0.326.25NVMe Driver Known Issues1.Unloading the Fibre Channel Protocol (FCP) driver using the modprobe -r command might cause issues on the initiatorbefore NVMe devices are disconnected.WorkaroundUnload the driver with rmmod lpfc, or if modprobe –r lpfc is required, wait for the device loss period of 60 seconds before unloading the driver.2.On RHEL 7.8 inbox drivers with NVMe targets connected to the port, when the firmware is upgraded, the No host rebootrequired to activate new firmware feature might not work as intended.WorkaroundIf the new firmware is not activated, reload the driver or reboot the system.3.On the Oracle Linux 7.7 unbreakable enterprise kernel (UEK) R6 operating system, unloading a Linux FCP driver withNVMe connections might fail.WorkaroundDisconnect the NVMe connections before unloading the Linux FCP driver.4.When performing a clean operating system installation of RHEL 8.2 and loading the nvme-cli RPM from the ISO, thedefault hostid and hostnqn values are the same on multiple servers.WorkaroundUpdate the nvme-cli utility to version 1.10.1-1.el8 or later.5.On an NVMe controller, reconnectivity issues may occur during switch port toggles. This issue is an operating systemissue.WorkaroundContact Red Hat or SUSE technical support.6.In the fstab file, when an NVMe namespace mount point entry is included and the defaults option is specified, if theoperating system is rebooted, the operating system might go into emergency mode.WorkaroundsFor SLES15 SP3:Specify nofail as the mount option when creating the NVMe namespace fstab mount point entry.For RHEL7.x, SLES 12 SP5, SLES 15 SP2, and RHEL8.x:–Specify nofail as the mount option when creating the NVMe namespace fstab mount point entry.–Install the nvmf boot dracut module provided in the nvmefc-dm kit.7.The SLES 15 SP3 installer might not discover the NVMe namespace to install the operating system.WorkaroundUse the nvmefc-dm kit to discover the NVMe namespace.8.During RHEL 7.x and RHEL 8.2 installation, the operating system reports an error while attempting to add an UEFI bootentry. This issue is an operating system issue and has been reported to Red Hat.NOTE:This issue is specific to NVMe BFS.WorkaroundIgnore the warning prompt and continue the installation. On the first reboot, use the server UEFI menu to select the adapter port that is attached to the fabric to boot the operating system.9.On SLES 12 SP5, the nvmefc-boot-connections systemd unit is disabled after the operating system is installedusing NVMe BFS. This issue leads to the operating system failing to discover all NVMe subsystems when a large number of NVMe subsystems exist.WorkaroundManually enable the nvmefc-boot-connections systemd unit. See NVMe Driver Technical Tips, Item6, for more information.10.An unrecoverable operating system fault might occur when generating a crash dump after successful installation of theoperating system in the NVMe BFS namespace.WorkaroundFrom the NVMe BFS kit, extract and install the nvmefc-dm RPM that matches the installed operating system.# tar zxf elx-nvmefc-dm-<version>-<release>/nvmefc-dm-<version>-<release>.<OS>.tar.gz11.If the operating system was installed using NVMe over FC BFS, and you are updating the firmware from version12.8.xxx.xx to version 14.0.xxx.xx, NVMe storage might not be found, and the UEFI boot menu might not boot theoperating system.WorkaroundIn the UEFI configuration utility, enable the Generate and use a Port Based HostNQN option. Refer to the Emulex Boot for the Fibre Channel and NVMe over FC Protocols User Guide for details.12.If the operating system was installed using NVMe over FC BFS, and you are updating the firmware from version14.0.xxx.xx to version 12.8.xxx.xx, NVMe storage might not be found, and the UEFI boot menu might not boot theoperating system.WorkaroundUpdate the SAN storage configuration and add an adapter WWPN-based HostNQN to the storage array.FC Driver Technical Tips1.For secure boot of the RHEL operating system, download the public key file for the RHEL operating system from, and place it in the systems key ring before installing the signed lpfc driver for the RHEL operating system. Refer to the Red Hat documentation for instructions on adding a kernel module to the system.2.On Red Hat operating systems, the installer automatically searches for a driver update disk (DUD) on a storage volumelabeled OEMDRV. For details, refer to the Red Hat documentation.3.Out-of-box Linux driver builds are built on, and are intended to be run on, general availability (GA) kernels for theirrespective distributions. If you need an out-of-box Linux driver build for a subsequent distribution’s errata kernel, you might need to recompile the driver from the Linux driver source file on the errata kernel.NVMe Driver Technical Tips1.Creation of N_Port ID Virtualization (NPIV) connections on initiator ports that are configured for NVMe over FC is notsupported. However, initiator ports can connect to FCP and NVMe targets simultaneously.2.NVMe disks might not reconnect after a device timeout greater than 60 seconds has occurred.WorkaroundYou must perform a manual scan or connect using the nvme connect-all CLI command.3.To manually scan for targets or dynamically added subsystems, type the following command (all on one line):nvme connect-all --transport=fc --host-traddr=nn-<initiator_WWNN>:pn-<initiator_WWPN>--traddr=nn-<target_WWNN>:pn-<target_WWPN>where:–<initiator_WWNN> is the WWNN of the initiator, in hexadecimal.–<initiator_WWPN> is the WWPN of the initiator, in hexadecimal.–<target_WWNN> is the WWNN of the target, in hexadecimal.–<target_WWPN> is the WWPN of the target, in hexadecimal.For example:# nvme connect-all --transport=fc --host-traddr=nn-0x20000090fa942779:pn-0x10000090fa942779 --traddr=nn-0x20000090fae39706:pn-0x10000090fae397064.To enable autoconnect on the following operating systems, install nvme-cli from the operating system distributionmedia:–RHEL 8.3–RHEL 8.2–SLES 15 SP2–SLES 12 SP55.If the following files are present on the system after the operating system is installed, the operating system has alreadyinstalled NVMe over FC autoconnect facilities. Do not install the Emulex autoconnect script file for inbox NVMe over FC drivers.–/usr/lib/systemd/system/nvmefc-boot-connections.service–/usr/lib/systemd/system/nvmefc-connect@.service–/usr/lib/udev/rules.d/70-nvmefc-autoconnect.rules6.Enable nvmefc-boot-connections to start during boot.Use systemctl enable to enable the nvmefc-boot-connections systemd unit to start during boot.# systemctl enable nvmefc-boot-connectionsCreated symlink /etc/systemd/system/default.target.wants/nvmefc-boot-connections.service ? /usr/ lib/systemd/system/nvmefc-boot-connections.service.7.Back up the initial ramdisk before upgrading the operating system.During an operating system upgrade, the kernel or nvme-cli might be upgraded. Back up the initial ramdisk before upgrading the operating system to ensure that the operating system is still bootable in the event that NVMe BFS fails due to a change in either the kernel or nvme-cli.Change the directory to /boot, and make a copy of the initial ramdisk.Example for SUSE operating systems:# cd /boot# cp initrd-$(uname -r) initrd-$(uname -r).bakExample for Red Hat operating systems:# cd /boot# cp initramfs-$(uname -r).img initramfs-$(uname -r).img.origCopyright © 2022 Broadcom. All Rights Reserved. The term “Broadcom” refers to Broadcom Inc. and/or its subsidiaries. For more information, go to . All trademarks, trade names, service marks, and logos referenced herein belong to their respective companies.Broadcom reserves the right to make changes without further notice to any products or data herein to improve reliability, function, or design. Information furnished by Broadcom is believed to be accurate and reliable. However, Broadcom does not assume any liability arising out of the application or use of this information, nor the application or use of any product or circuit described herein, neither does it convey any license under its patent rights nor the rights of others.。
岛津LCsolution软件快速参考说明书

8.1 查看和使用 [ 数据管理器 ]................................................................................. 32 8.2 查看数据 ........................................................................................................... 34 8.3 使用 CLASS-Agent 查看数据 ........................................................................... 36
岛津 LCsolution
色谱数据系统 快速参考说明书
使用产品前请仔细阅读本说明书。 请妥善保管本说明书以备今后参考。
岛津企业管理(中国)有限公司 分析仪器事业部 分析中心
1
本页空白。
目录
1 2
3 4 5
6 7 8
索引
在阅读此文档前....................................................................................................1
对羟基苯甲酸酯混合物
1.2
LCsolution 的数据结构
本节简要描述了 LCsolution 的数据结构。 各种 LCsolution 数据参数保存在数据文件中,例如在数据采集和数据分析 (元数据)中的仪器和分析条 件。由于可以从数据文件本身参照分析条件和参数,这样就确保了数据的可跟踪性。此外,仅使用数据文 件即可重新分析数据。
Moxa ioLogik 4000 Series模块化远程I O系统说明书

ioLogik4000SeriesModular remote I/OFeatures and Benefits•I/O expansion without a backplane•Active communication with MX-AOPC UA Server•Supports SNMP v1/v2c•Easy configuration with Modular ioAdmin utility•Friendly configuration via web browser•Simplifies I/O management with MXIO library for Windows or LinuxCertificationsIntroductionThe ioLogik4000Series is suitable for remote monitoring and alarm systems,such as those used for water treatment systems,water supply systems,wastewater treatment systems,and power monitoring systems.These kinds of applications need more I/O points and a variety of I/O types,including temperature sensors,gas detectors,and water quality detectors,all of which can benefit from the versatile mixture of I/O features supported by the ioLogik4000Series.Slice Form Factor and Flexible I/O VarietyThe unique modular construction of the ioLogik4000Series allows for mixing and matching of modules to achieve the best combination of I/O modules to meet the needs of a wide range of remote automation applications.An industrial modular housing enables I/O modules to be added to the base unit without a backplane.The ioLogik4000Series is perfect for space-limited applications,and high-density I/O points are provided for greater flexibility and expandability.Modules can connect to virtually any type of sensor,including but not limited to those for temperature, pressure,flow,voltage,current,and contact closure.Easy MaintenanceThe ioLogik4000Series comes with removable spring-type terminal blocks(RTBs)that allow you to conserve field wiring for future use.SpecificationsInput/Output InterfaceButtons Reset buttonControl LogicLanguage ioLogik E4200:Click&Go,NA-4010/4020/4021:NoneEthernet Interface10/100BaseT(X)Ports(RJ45connector)NA-4010:1ioLogik E4200:2,2MAC addressesEthernet Software FeaturesConfiguration Options NA-4010/ioLogik E4200:Web Console(HTTP)NA-4010/4020/4021:Windows Utility(ioAdmin)ioLogik E4200:Windows Utility(Modular-ioAdmin)Industrial Protocols NA-4010:Modbus TCP Server(Slave),MXIO LibraryNA-4020/4021:MXIO LibraryioLogik E4200:Modbus TCP Server(Slave),Moxa AOPC(Active Tag),MXIO Library,SNMPv1/v2c,SNMPv1TrapManagement NA-4010/ioLogik E4200:DHCP Client,HTTP,IPv4,TCP/IP,UDPNA-4010/4020/4021:ioAdminioLogik E4200:Modular-ioAdminSerial InterfaceConnector NA-4021:DB9femaleNA-4020:Terminal blockData Bits7,8No.of Ports1Parity None,Even,OddSerial Standards NA-4021:RS-232NA-4020:RS-485Stop Bits1,2Baudrate NA-4020/4021:1200,2400,4800,9600,19200,38400,57600,115200bpsSerial Software FeaturesIndustrial Protocols NA-4020/4021:Modbus RTU/ASCII SlaveSerial SignalsRS-232NA-4021:TxD,RxD,GNDRS-485-2w NA-4020:Data+,Data-,GNDDIP Switch ConfigurationSerial Interface Fixed baudrateModbus TCPMax.No.of Client Connections8Mode Client(Master)Power ParametersNo.of Power Inputs1System Power ParametersPower Connector Spring-type Euroblock terminalNo.of Power Inputs1Input Voltage11to28.8VDCPower Consumption NA-4010:60mA@24VDCNA-4020/4021:70mA@24VDCioLogik E4200:175mA@24VDCField Power ParametersPower Connector Spring-type Euroblock terminalNo.of Power Inputs1Input Voltage11to28.8VDCCurrent in Field Power Contact10A(max.)Physical CharacteristicsHousing PlasticDimensions45x99x70mm(1.77x3.90x2.76in)Weight NA-4010/4020/4021:150g(0.33lb)ioLogik E4200:180g(0.396lb)Installation DIN-rail mountingEnvironmental LimitsOperating Temperature-10to60°C(14to140°F)Storage Temperature(package included)-40to85°C(-40to185°F)Ambient Relative Humidity5to95%(non-condensing)Altitude2000m1Standards and CertificationsEMC EN61000-6-2/-6-4EMI CISPR22,FCC Part15B Class AEMS IEC61000-4-2ESD:Contact:4kV;Air:8kVIEC61000-4-3RS:80MHz to1GHz:10V/mIEC61000-4-4EFT:Power:2kV;Signal:1kVIEC61000-4-5Surge:Power:2kV;Signal:1kVIEC61000-4-6CS:10VIEC61000-4-8PFMFSafety UL508Shock IEC60068-2-27Vibration IEC60068-2-6DeclarationGreen Product RoHS,CRoHS,WEEEMTBFTime NA-4010:4,739,300hrsNA-4020:4,721,640hrsNA-4021:4,695,360hrsioLogik E4200:357,000hrsStandards Telcordia SR332WarrantyWarranty Period2yearsDetails See /warranty1.Please contact Moxa if you require products guaranteed to function properly at higher altitudes.Package ContentsDevice1x ioLogik4000Series remote I/OInstallation Kit1x terminal block,8-pin,3.81mmDocumentation1x quick installation guide(ioLogik E4200)1x warranty cardNote This product requires additional modules(sold separately)to function.DimensionsI/O Network Adapter I/O ModuleOrdering InformationModel Name Control Logic Ethernet Interface Serial Interface No.of Support I/O Modules ioLogik E4200Click&Go2x RJ45–16NA-4010–1x RJ45–32NA-4020––RS-48532NA-4021––RS-23232 Accessories(sold separately)I/O ModulesM-1450For the ioLogik4000Series,4DIs,110VACM-1451For the ioLogik4000Series,4DIs,220VACM-1600For the ioLogik4000Series,16DIs,24VDC,sink typeM-1601For the ioLogik4000Series,16DIs,24VDC,source typeM-1800For the ioLogik4000Series,8DIs,24VDC,sink typeM-1801For the ioLogik4000Series,8DIs,24VDC,source typeM-2450For the ioLogik4000Series,4relays,24VDC/230VAC,2AM-2600For the ioLogik4000Series,16DOs,24VDC,0.5A,sink typeM-2601For the ioLogik4000Series,16DOs,24VDC,0.5A,source typeM-2800For the ioLogik4000Series,8DOs,24VDC,0.5A,sink typeM-2801For the ioLogik4000Series,8DOs,24VDC,0.5A,source typeM-3802For the ioLogik4000Series,8AIs,4to20mA,12bitsM-3810For the ioLogik4000Series,8AIs,0to10V,12bitsM-4402For the ioLogik4000Series,4AOs,4to20mA,12bitsM-4410For the ioLogik4000Series,4AOs,0to10V,12bitsM-6200For the ioLogik4000Series,2AIs,RTD:PT100,JPT100M-6201For the ioLogik4000Series,2AIs,TCPower ModulesM-7001For the ioLogik4000Series,system power moduleM-7002For the ioLogik4000Series,field power moduleM-7804For the ioLogik4000Series,potential distributor module,0VDC8channelM-7805For the ioLogik4000Series,potential distributor module,24VDC,8channelSoftwareMX-AOPC UA Server OPC UA Server software for converting fieldbus to the OPC UA standard©Moxa Inc.All rights reserved.Updated Dec09,2020.This document and any portion thereof may not be reproduced or used in any manner whatsoever without the express written permission of Moxa Inc.Product specifications subject to change without notice.Visit our website for the most up-to-date product information.。
patran错误日志及解决方法

2. USER WARNING MESSAGE 4124 (IFS3P)
THE SPCADD OR MPCADD UNION CONSISTS OF A SINGLE SET 在图中用了 RB3 的 MPC,其中 dependent node (ux,uy,uz), independent(ux,uy,uz,rx,ry,rz),有可 能是这里的问题。不过这个倒不影响计算结果。
^^^ RUN TERMINATED DUE TO EXCESSIVE PIVOT RATIOS IN MATRIX KLL. ^^^ USER ACTION: CONSTRAIN MECHANISMS WITH SPCI OR SUPORTI ENTRIES OR SPECIFY PARAM,BAILOUT,-1 TO CONTINUE THE RUN WITH MECHANISMS. 以前也遇到这种情况,这次遇到后,又在一节点加载了位移约束,就解决了。看来这 种错误主要是由于约束不够,线性方程组无解造成的。 还有可能是没有 equivalence,This should solve your problem or reduce the number of failed ratios.有一次就遇到此情况。
7. 常用材料定义对比表
MAT1 MAT2 MAT3 MAT8 MAT9
isotropic anisotropic(2) orthotropic(3) orthotropic(2) anisotropic(3) • Use a FORCE entry if you want to define a static, concentrated force at a grid point by 一个点的力 specifying a vector. • Use a FORCE1 entry if the direction is determined by a vector connecting two grid points. 两个点的力 • Use a FORCE2 entry if the direction is specified by the cross product of two such vectors. 以上的乘积
_NCCP1H010_MoveAbsolute_REAL
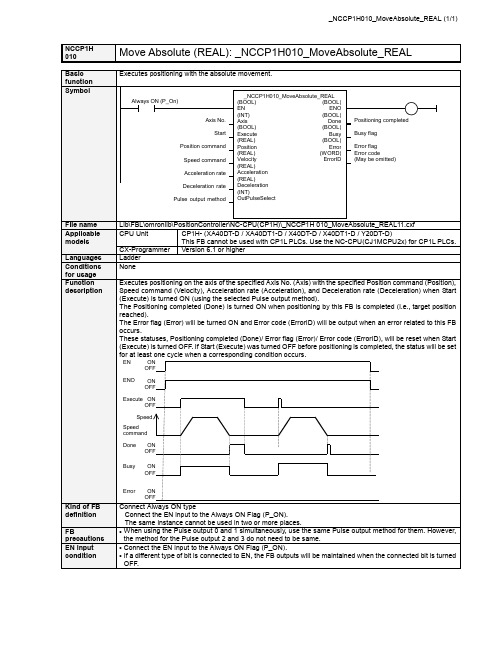
NCCP1HMove Absolute (REAL): _NCCP1H010_MoveAbsolute_REAL 010■ Variable TableOutput Variables Name Variable name Data type Range DescriptionENO ENO BOOL 1(ON): FB operating normally0(OFF): FB not operating normallyPositioning completed Done BOOL 1 (ON) indicates that positioning is completed.Busy flag Busy BOOL 1 (ON) indicates that the FB is in pregress.Error flag Error BOOL 1 (ON) indicates that an error has occurred in the FB. Error code(May be omitted)ErrorIDWORDThe error code of the error occurred in the FB will be output. For details of the errors, refer to the sections of the manual listed in the Related manuals above. When Unit No. or Axis. No. is out of the range, #0000 will be output.Limitation of Function block by Combination of CPU type and Unit Version CPU Type Unit Version Axis No. Range of Frequency Description 1.1 &0 to &3 +1.0 to +100000.0 &0 to &1 +1.0 to +100000.0XA / X 1.0 &2 to &3 +1.0 to +30000.0Please use Function Block Version1.10 or higher whenyou set values that are larger than 30000.0Hz to Axis. No.2 or No.3. &0 to &1 +1.0 to +1000000.0 Y 1.1 &2 to &3 +1.0 to +100000.0Please use Function Block Version1.10 or higher forCP1H-Y20DT-D.■ Revision History Version Date Contents 1.10 2006.5. Addition of CP1H CPU Unit unit version 1.1 1.00 2005.9. Original productionNoteThis document explains the function of the function block.It does not provide information of restrictions on the use of Units and Components or combination of them. For actual applications, make sure to read the operation manuals of the applicable products.。
CPX-4AE-T CPX-4AE-I 分析模块说明书
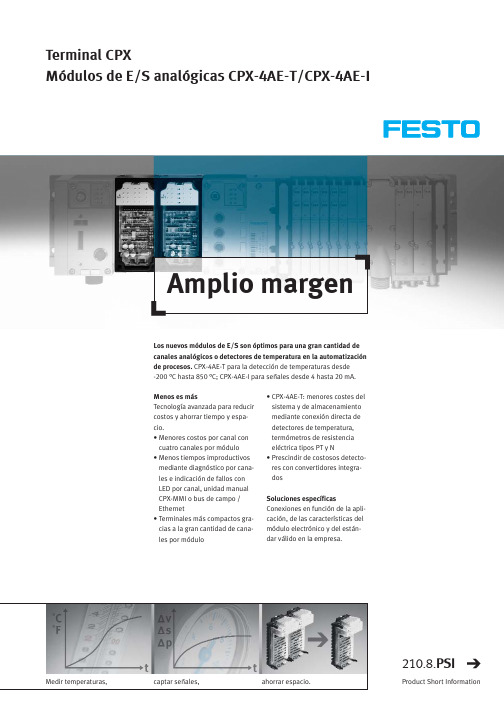
Terminal CPXMódulos de E/S analógicas CPX-4AE-T/CPX-4AE-IAmplio margen•CPX-4AE-T: menores costes del sistema y de almacenamiento mediante conexión directa de detectores de temperatura,termómetros de resistencia eléctrica tipos PT y N•Prescindir de costosos detecto-res con convertidores integra-dosSoluciones específicasConexiones en función de la apli-cación, de las características del módulo electrónico y del están-dar válido en la empresa.Menos es másTecnología avanzada para reducir costos y ahorrar tiempo y espa-cio.•Menores costos por canal con cuatro canales por módulo •Menos tiempos improductivos mediante diagnóstico por cana-les e indicación de fallos con LED por canal, unidad manual CPX-MMI o bus de campo /Ethernet•Terminales más compactos gra-cias a la gran cantidad de cana-les por móduloLos nuevos módulos de E/S son óptimos para una gran cantidad de canales analógicos o detectores de temperatura en la automatización de procesos. CPX-4AE-T para la detección de temperaturas desde -200°C hasta 850 °C; CPX-4AE-I para señales desde 4 hasta 20 mA.Medir temperaturas,captar señales,ahorrar espacio.210.8.PSIProduct Short InformationFesto AG &Co.KGRuiter Strasse 8273734 EsslingenInternet Tel. ++49 (0)711 347-0 Fax ++49 (0)711 347-2144E-mail service_international@270406R e s e r v a d o e l d e r e c h o d e m o d i f i c a c i ónTerminal CPXMódulos de E/S analógicas CPX-4AE-T / CPX-4AE-IAmplia modularidadMás economía mediante la utili-zación de módulos electrónicos.Medir temperaturas, caudales,presiones y distancias.CPX: diversidad de conexiones Gran cantidad de funciones eléc-tricas. El encadenamiento de la placa de alimentación, módulo electrónico y placa de alimenta-ción permite numerosas combi-naciones. Elección rápida y man-tenimiento sencillo gracias a la sustitución de módulos electróni-cos sin modificar el cableado.TipoCPX-4AE-ICPX-4AE-TMódulos de entradas analógicas Captación de señales Detección de temperaturas Cantidad de entradas 44Cantidad de salidas ––Línea característica ––Resolución12 Bit16 BitNivel de conm./Margen señales 0 ... 20 mA, 4 ... 20 mA Fuente de intensidad constante Alim. máx. de corr. por canal 40 mA En función del detector Alim. máx. de corr. por módulo 0,7 A0,7 A Detectores P . ej. sensor de presión y vacío SDE–DetectoresSensores de presión, detectores de caudal PT 100, PT 200, PT 500, PT 1000y medidores de distancias Ni 100, Ni 120, Ni 500, Ni 1000Margen de temperatura –PT estándar: -200 °C ... 850 °C DetectoresPT entorno: -120 °C ... 130 °C NI estándar: -60 °C ... 180 °CConexiones para detectores 2, 3, 4 hilos 2, 3, 4 hilos ParametrizaciónFormato de datos, valores límite, factor de escala Formato de datos, valores límite, factor de escala,detector de temperatura, comportamiento de la fuente de corriente en caso de sobrecargaDiagnóstico 4 LED para errores de canal y 1 LED para error de móduloFuncionesParametrización y diagnóstico por canal, señales del canal defectuoso a través de la red o en CPX-MMIMPA1 y MPA2Bloque de enlace CPX-GE-7/8" 5 cont.CPX-4AE-ISensor de presión SDE1Medidor de distancias SOELDetector de caudal SFE1Módulo electrónico CPX-4AE-TDetector de temperatura PT 100Datos técnicos。
SAP中常用SE系列TCODE汇总
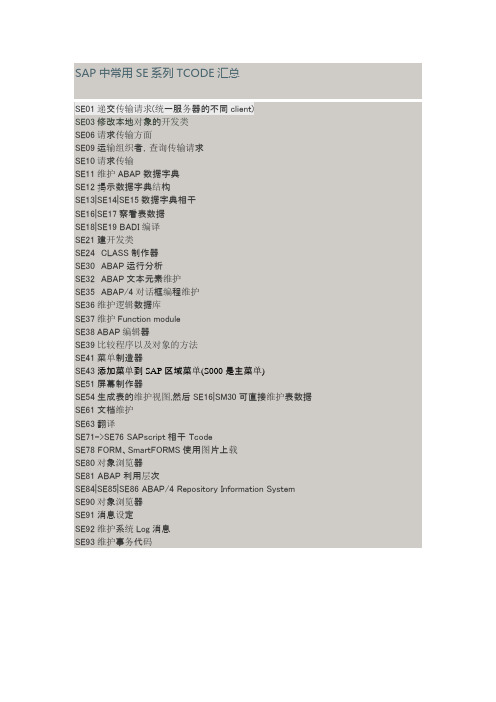
SAP中常用SE系列TCODE汇总SE01递交传输请求(统一服务器的不同client)SE03修改本地对象的开发类SE06请求传输方面SE09运输组织者,查询传输请求SE10请求传输SE11维护ABAP数据字典SE12揭示数据字典结构SE13|SE14|SE15数据字典相干SE16|SE17察看表数据SE18|SE19 BADI编译SE21建开发类SE24 CLASS制作器SE30 ABAP运行分析SE32 ABAP文本元素维护SE35 ABAP/4对话框编程维护SE36维护逻辑数据库SE37维护Function moduleSE38ABAP编辑器SE39比较程序以及对象的方法SE41菜单制造器SE43添加菜单到SAP区域菜单(S000是主菜单)SE51屏幕制作器SE54生成表的维护视图,然后SE16|SM30可直接维护表数据SE61文档维护SE63翻译SE71->SE76 SAPscript相干 TcodeSE78 FORM、SmartFORMS使用图片上载SE80对象浏览器SE81 ABAP利用层次SE84|SE85|SE86 ABAP/4 Repository Information SystemSE90对象浏览器SE91消息设定SE92维护系统Log消息SE93维护事务代码一句话概括SAPSD1,谁来卖?既是执行销售动作的组织结构及此结构下的人员。
SAP术语有:公司代码、销售组织、分销渠道、产品组、销售办公室、销售小组、销售雇员、装运点等。
2,卖什么?广义销售概念中的销售内容包括实物和非实物的服务。
SAP术语中,我们称之为物料(Material)。
有实物产品物料,也有服务物料。
3,卖给谁?卖给客户。
这里,客户的外延与内涵较多。
如一次性客户、大客户、集团内部客户、海外客户;售达方、送达方、收票方、付款方等。
如果对某客户做赊销业务,相应的,该客户还有用于控制应收风险的信用数据。
4,什么样的价格?价格是销售的技术核心,定价功能也是SAP SD的核心所在。
性能测试执行之使用Analysis分析测试结果
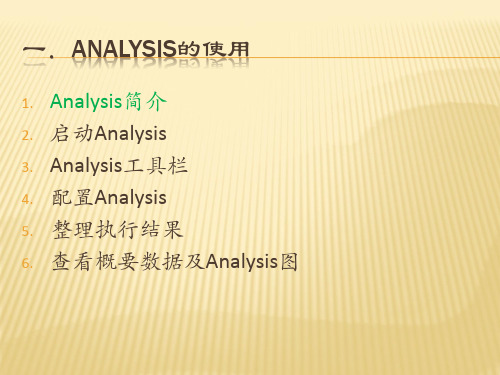
一.ANALYSIS的使用——配置ANALYSIS
② 选择 “数据源”、“数据聚合”、“数据时 间范围”和 “输出消息”选项。
③ 要配置自定义聚合,请单击聚合配置,然后 设置选项。
④ 单击确定。
一.ANALYSIS的使用
1. Analysis简介 2. 启动Analysis 3. Analysis工具栏 4. 配置Analysis 5. 整理执行结果 6. 查看概要数据及Analysis图
一. ANALYSIS的使用——查看概要数据及 ANALYSIS图
2. Analysis 图 ① Vuser 图。提供 Vuser 状态信息和其他 Vuser 统计信息。 ② 错误图。提供有关负载测试场景中所发生错误的信息。 ③ 事务图。提供有关事务性能和响应时间的信息。 ④ Web 资源图。提供有关 Web Vuser 吞吐量、每秒点击次数、
一.ANALYSIS的使用——配置ANALYSIS
要配置用户定义的聚合和设置: 1 选择工具 > 选项,然后选择结果集合选项卡。 2 在 “数据聚合”区域,选择应用用户定义的
聚合。 3 单击聚合配置。这时将打开 “数据聚合配置”
对话框。
一.ANALYSIS的使用——配置ANALYSIS
一.ANALYSIS的使用——配置ANALYSIS
一.ANALYSIS的使用
1. Analysis简介 2. 启动Analysis 3. Analysis工具栏 4. 配置Analysis 5. 整理执行结果 6. 查看概要数据及Analysis图
一.ANALYSIS的使用——启动ANALYSIS
1. 启动Analysis Analysis 可以作为独立的应用程序打开,也可以直接从
从 窗口 > 会话浏览器可以访问 Analysis 图。
CP1H操作手册中文
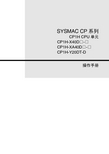
关于在国外的使用
当出口(或提供给非居住者)本产品中属于外汇及外国贸易管理法所规定的出口许可、 承认对象货物(或技术)范围的产品时,必须有以相关法律为基准的出口许可、承认(或 官方交易许可)。
4
关于 CP 系列的「单元版本」
关于 CP 系列的「单元版本」
单元版本是指
在 SYSMAC CP 系列中,为了管理由于版本升级等引起的 CPU 单元配置功能的差异,引 入了「单元版本」这个概念。
W451
CP1H-X40D□-□ CP1H-XA40D□-□ CP1H-Y20DT-D CS1G/H-CPU□□H CS1G/H-CPU□□-V1 CS1D-CPU□□H CS1D-CPU□□S CS1W-SCU21 CS1W-SCB21-V1/41-V1 CJ1G/H-CPU□□H CJ1G-CPU□□P CP1H-CPU□□ CJ1G-CPU□□ CJ1W-SCU21-V1/41-V1 WS02-CXPC1-EV6 WS02-CXPC1-EV6
6
关于 CP 系列的「单元版本」
3)通过单元版本标签进行识别
单元版本标签(下图)附带在产品中。
Ver. Ver.
1.0 1.0
Ver. Ver.
为了管理由于版本升级等引起的 CPU 单元配置功能的差异的标 签。
请根据需要贴在产品的正面。 These Labels can be used to manage differences in the available functions among the Units. Place the appropriate label on the front of the Unit to show what Unit version is actually being used.
PAM4 Transmitter Analysis软件应用说明书
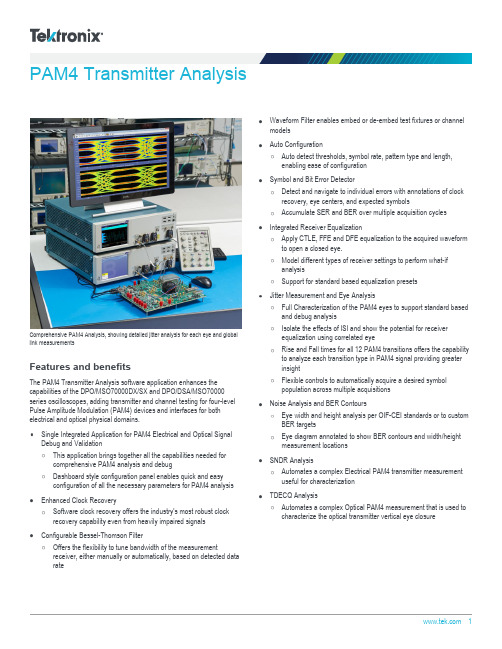
PAM4 Transmitter AnalysisComprehensive PAM4 Analysis, showing detailed jitter analysis for each eye and global link measurementsFeatures and benefitsThe PAM4 Transmitter Analysis software application enhances the capabilities of the DPO/MSO70000DX/SX and DPO/DSA/MSO70000series oscilloscopes, adding transmitter and channel testing for four-level Pulse Amplitude Modulation (PAM4) devices and interfaces for bothelectrical and optical physical domains.Single Integrated Application for PAM4 Electrical and Optical SignalDebug and ValidationThis application brings together all the capabilities needed forcomprehensive PAM4 analysis and debugDashboard style configuration panel enables quick and easyconfiguration of all the necessary parameters for PAM4 analysisEnhanced Clock RecoverySoftware clock recovery offers the industry's most robust clockrecovery capability even from heavily impaired signalsConfigurable Bessel-Thomson FilterOffers the flexibility to tune bandwidth of the measurementreceiver, either manually or automatically, based on detected datarateWaveform Filter enables embed or de-embed test fixtures or channelmodelsAuto ConfigurationAuto detect thresholds, symbol rate, pattern type and length,enabling ease of configurationSymbol and Bit Error DetectorDetect and navigate to individual errors with annotations of clockrecovery, eye centers, and expected symbolsAccumulate SER and BER over multiple acquisition cyclesIntegrated Receiver EqualizationApply CTLE, FFE and DFE equalization to the acquired waveformto open a closed eye.Model different types of receiver settings to perform what-ifanalysisSupport for standard based equalization presetsJitter Measurement and Eye AnalysisFull Characterization of the PAM4 eyes to support standard basedand debug analysisIsolate the effects of ISI and show the potential for receiverequalization using correlated eyeRise and Fall times for all 12 PAM4 transitions offers the capability to analyze each transition type in PAM4 signal providing greaterinsightFlexible controls to automatically acquire a desired symbolpopulation across multiple acquisitionsNoise Analysis and BER ContoursEye width and height analysis per OIF-CEI standards or to customBER targetsEye diagram annotated to show BER contours and width/heightmeasurement locationsSNDR AnalysisAutomates a complex Electrical PAM4 transmitter measurementuseful for characterizationTDECQ AnalysisAutomates a complex Optical PAM4 measurement that is used tocharacterize the optical transmitter vertical eye closurePlots and reportsComprehensively interact with plots for measurement visualizationand deep analysisHTML report captures all the relevant setup configuration,measurement test results, and plot in single file that is easy to readand shareMeasurement results across multiple acquisitions can be exported to a consolidated CSV file useful for additional analysisApplicationsDebug, Analysis, and Characterization of Electrical and Optical PAM4signalsCharacterization of OIF-CEI and IEEE based PAM4 standards; such as OIFCEI-VSR-56G-PAM4, 802.3bs, and CDAUI-8.PAM4 overviewThe frequency content of the NRZ signal increases linearly with bit-rate.PAM4 signaling needs half the bandwidth as NRZ for the same data rate.400G Ethernet standards, both electrical and optical interfaces, adopted PAM4 signaling to support the forecasted growth in the datacenter andnetwork traffic.Assumes linear coding for illustration. In practice, gray coding is frequently used.The 4 levels of PAM4 introduce additional complexity in signaling and place new demands on the test methodology. The PAM4 analysis tool offers several measurement and visualization capabilities aimed at making the task of validating PAM4 designs more efficient.PAM4 Measurement configurationThe configuration panel is a dashboard within the PAM4 analysis tool that enables you to configure most elements for a PAM4 analysis run. The panel includes; measurement source selection, Clock recovery, Threshold,and Bessel-Thomson filter and Equalization configuration. It also has theability to embed or de-embed a channel using a waveform filter.Clock recoveryConfigurable PLL (phase-locked loop) clock recovery reliably extracts the symbol clock, even with highly impaired signals, and exports thereconstructed clock waveform to a reference channel where it may be viewed.Channel Embedding / De-embeddingThe waveform filter option offers the ability to embed or de-embed differentchannel elements. For example:The effects of a test fixture can be de-embedded to gain visibility of thesignal at the transmitter output.A channel can be embedded to gain visibility of the signal at the receiver input.DatasheetEqualizationIt is often necessary to apply receiver equalization to open the eyes before measurements can be performed. In most cases the lack of physical access makes it impossible to verify the receiver circuit behavior and monitor the effects of clock recovery and equalization.A comprehensive equalizer in the PAM4 analysis tool offers the ability to dothe following:Apply CTLE either using custom poles and zeros or standards basedpresets.Apply configurable length FFE and / or DFE with auto-adapted tapvalues.Observe the tap values that have been chosen.Measurement SelectionThe new Select panel enables you to select between electrical and optical PAM4 measurements.The selection list allows you to choose window measurements andconfigure the display for ease of use and execution speed.Auto Configure CapabilityThe PAM4 analysis application can automatically detect the signal’s symbol rate and pattern, and choose the appropriate decision thresholds based on analysis of the eye diagram. This allows quick and error-free set-up, as well as, verifying your signal’s key characteristics.PAM4 MeasurementsPAM4 analysis package provides a comprehensive set of measurements that offer greater insight into signal characteristics, speeding up validation or characterization of PAM4 designs.The supported list includes IEEE (802.3bs/cd) and OIF-CEI Standards based measurements or SNDR and TDECQ when enable characterized of electrical and optical PAM4 Transmitter.PAM4 Transmitter AnalysisFull Waveform and Correlated WaveformanalysisA full waveform analysis can be performed by overlaying all the unitintervals on the acquired PAM4 signal. A jitter analysis is done on theindividual eyes within the link and the BER eye contours. Both tests cangive insight into eye closure at all timing phases and reference levelssimultaneously.The correlated waveform and eye show how much additional eye openingis theoretically obtainable through equalization. The correlated waveformcan be analyzed by tools and techniques similar to those found onEquivalent Time Oscilloscopes. Many performance communicationsstandards assume access to correlated data. The PAM4 Analysisapplication can effectively model correlated and composite eye diagrams.1Supports Average Launch Power of Off Transmitter as per IEEE 802.3bs/cd specifications.2This measurement not available until December 2018.DatasheetRise and Fall Time analysisAnalysis of the individual transitions rise and fall times helps separate linear impairments (bandwidth, ISI) from nonlinear (slew-rate limiting, clipping).The rise and fall times also support advanced tuning of equalizationalgorithms. The PAM-4 analysis software provides the max, min, and meanrise and fall time for each of the six transition types within the PAM4 eye.VisualizationA comprehensive set of plots can be used to visualize measurement data.The plots provide additional insight into the signal characteristics and are useful for debugging.The plot toolset enables interaction with the plots and can focus in on anarea of interest for closer examination and further analysis.Error DetectorThe PAM4 analysis tool comes with a built in error detector that can identify individual symbol errors in the current source waveform. The identified errorcan be viewed in a dedicated error navigator window.The error navigator has several capabilities that makes it easy to quickly navigate and zoom into the error location. The additional information for thefollowing detected errors offer help debugging symbol errors on the link:Location of recovered clockLocation of symbol error reference thresholdsExpected symbol displayed Actual symbol displayedPAM4 Transmitter AnalysisComprehensive test report and data exportThe measurement results can be saved in the form of a test report. The report includes; the configuration of the oscilloscope, applicationconfiguration, measurement results, and plots all available in an easy to read or share format.The measurement results across multiple acquisitions can also be exportedto a single CSV file for further analysis.Full waveform resultsFull waveform eye diagramDPO7OE Series Optical ProbesThe DPO7OE Series Optical probes can be used as an Optical Reference Receiver for high speed serial data signals (using selectable Bessel-Thomson ORR filters), or can be used as a conventional O/E converter for general wide-bandwidth optical signal acquisition. The DPO7OE Series is compatible with DPO/MSO70000 C/DX/SX models. Connected to TekConnect channels for up to 33 GHz bandwidth. Connected to ATI channels, the DPO7OE1 provides up to 42 GHz electrical response; theDPO7OE2 provides up to 59 GHz electrical bandwidth response.DPO7OE1 33 GHz Optical ProbeDatasheetOrdering informationThe PAM4 Transmitter analysis software for Tektronix DPO/MSO70000 Win7 Series oscilloscopesFor new DPO/MSO70000 Series oscilloscopesFor users with existing DPO/DSA/MSO70000 Series oscilloscopesRequired optionsDJA DPOJET Eye and Jitter Analysis DJAN DPOJET Noise AnalysisSDLA64SDLA Visualizer channel de-embedding, embedding, and equalizationRecommended ProbesDPO7OE133 GHz optical probe DPO7OE259 GHz optical probeTektronix is registered to ISO 9001 and ISO 14001 by SRI Quality System Registrar.Product(s) complies with IEEE Standard 488.1-1987, RS-232-C, and with Tektronix Standard Codes and Formats.PAM4 Transmitter AnalysisDatasheetASEAN / Australasia (65) 6356 3900 Austria 00800 2255 4835*Balkans, Israel, South Africa and other ISE Countries +41 52 675 3777 Belgium 00800 2255 4835*Brazil +55 (11) 3759 7627 Canada180****9200Central East Europe and the Baltics +41 52 675 3777 Central Europe & Greece +41 52 675 3777 Denmark +45 80 88 1401Finland +41 52 675 3777 France 00800 2255 4835*Germany 00800 2255 4835*Hong Kong 400 820 5835 India 000 800 650 1835 Italy 00800 2255 4835*Japan 81 (3) 6714 3086 Luxembourg +41 52 675 3777 Mexico, Central/South America & Caribbean 52 (55) 56 04 50 90Middle East, Asia, and North Africa +41 52 675 3777 The Netherlands 00800 2255 4835*Norway 800 16098People's Republic of China 400 820 5835 Poland +41 52 675 3777 Portugal 80 08 12370Republic of Korea +822 6917 5084, 822 6917 5080 Russia & CIS +7 (495) 6647564 South Africa +41 52 675 3777Spain 00800 2255 4835*Sweden 00800 2255 4835*Switzerland 00800 2255 4835*Taiwan 886 (2) 2656 6688 United Kingdom & Ireland 00800 2255 4835*USA180****9200* European toll-free number. If not accessible, call: +41 52 675 3777For Further Information. Tektronix maintains a comprehensive, constantly expanding collection of application notes, technical briefs and other resources to help engineers working on the cutting edge of technology. Please visit . Copyright © Tektronix, Inc. All rights reserved. Tektronix products are covered by U.S. and foreign patents, issued and pending. Information in this publication supersedes that in all previously published material. Specification andprice change privileges reserved. TEKTRONIX and TEK are registered trademarks of Tektronix, Inc. All other trade names referenced are the service marks, trademarks, or registered trademarks of their respective companies.06 Feb 2019 55W-60239-8 。
用SYS软件分析压电换能器入门
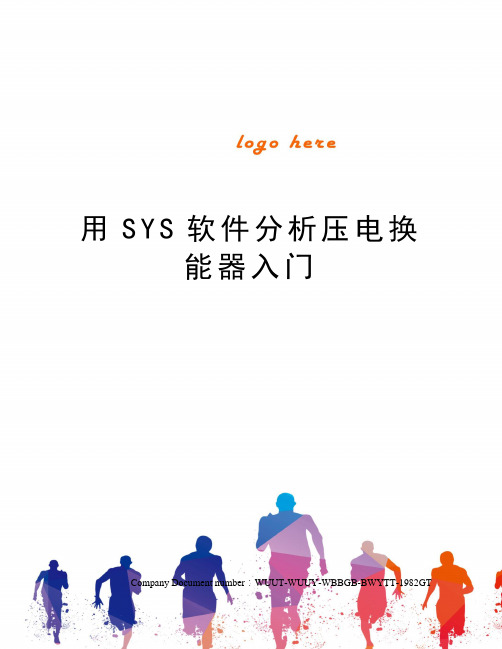
用S Y S软件分析压电换能器入门Company Document number:WUUT-WUUY-WBBGB-BWYTT-1982GT用ANSYS软件分析压电换能器入门A:分析过程基本步骤一:问题描述(草稿纸上完成)1:画出换能器几何模型,包括尺寸2:选定材料3:查材料手册确定材料参数二:建立模型1:根据对称性确定待建模型的维数2:根据画出的几何模型确定关键点坐标,给关键点编好号码3:建立一个文件夹用于当前分析4:启动ANSYS软件,指定路径到建立的文件夹,5:定义单元类型压电换能器分析使用的单元类型:solid5:8个节点3D六面体耦合场单元(也可缩减为三角柱形单元或四面体单元)。
无实常数。
plane13:4个节点2D四边形耦合场单元(也可缩减为三角形单元)。
无实常数。
solid98:10个节点3D四面体耦合场单元。
无实常数。
Fluid30:8个节点3D六面体声学流体单元(也可缩减为三角柱形单元或四面体单元)。
应用于近场水和远场水。
实常数为参考声压,可缺省。
Fluid130:4个节点面无穷吸收水声学流体单元(也可缩减为三角形面单元)。
实常数:半径,球心X,Y,Z坐标值。
6:定义材料参数对一般均匀各向同性材料要给出材料密度,杨氏模量,泊松系数。
(静态分析不用密度)对压电材料:一般使用的压电方程:e型压电方程,因此输入的常数为注意!一般顺序为:XX,YY,ZZ,YZ,XZ,XY。
在ANSYS中为XX,YY,ZZ,XY,YZ,XZ。
因此,前两矩后三行和后三列要做相应变化。
7:建立关键点8:把关键点连成线9:把线段围成面10:通过适当的方法生成体11:指定单元类型和材料参数12:划分线段13:划分体单元14:坐标转换,(转换到柱坐标系下)15:节点转换三:加载约束条件1:加载边界约束条件2:电极上加电压四:求解1:模态分析2:谐响应分析五:查看结果1:查看模态分析结果,计算导纳。
2:各模态的动态演示3:查看谐响应分析结果,计算导纳、发射与接收响应。
paco 0.4.2 软件说明书
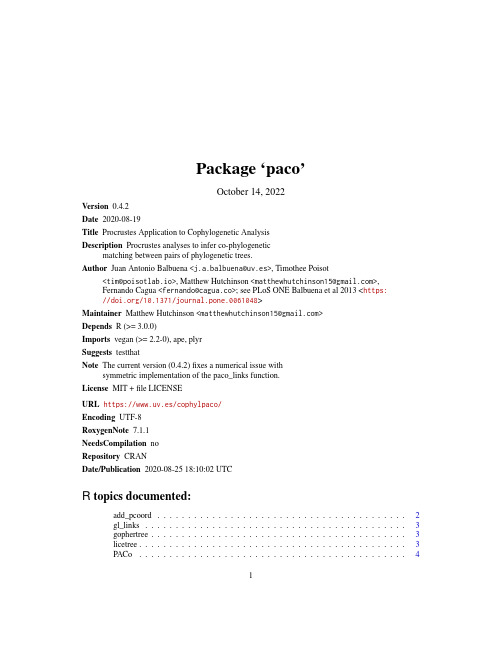
Package‘paco’October14,2022Version0.4.2Date2020-08-19Title Procrustes Application to Cophylogenetic AnalysisDescription Procrustes analyses to infer co-phylogeneticmatching between pairs of phylogenetic trees.Author Juan Antonio Balbuena<******************>,Timothee Poisot<****************>,Matthew Hutchinson<*****************************>,Fernando Cagua<*****************>;see PLoS ONE Balbuena et al2013<https:///10.1371/journal.pone.0061048>Maintainer Matthew Hutchinson<*****************************>Depends R(>=3.0.0)Imports vegan(>=2.2-0),ape,plyrSuggests testthatNote The current version(0.4.2)fixes a numerical issue withsymmetric implementation of the paco_links function.License MIT+file LICENSEURL https://www.uv.es/cophylpaco/Encoding UTF-8RoxygenNote7.1.1NeedsCompilation noRepository CRANDate/Publication2020-08-2518:10:02UTCR topics documented:add_pcoord (2)gl_links (3)gophertree (3)licetree (3)PACo (4)12add_pcoord paco_links (5)prepare_paco_data (6)residuals_paco (7)Index8 add_pcoord Principal Coordinates analysis of phylogenetic distance matricesDescriptionTranslates the distance matrices of’host’and’parasite’phylogenies into Principal Coordinates,as needed for Procrustes superimposition.Usageadd_pcoord(D,correction="none")ArgumentsD A list with objects H,P,and HP,as returned by paco::prepare_paco_data.correction In some cases,phylogenetic distance matrices are non-Euclidean which gen-erates negative eigenvalues when those matrices are translated into PrincipalCoordinates.There are several methods to correct negative eigenvalues.Cor-rection options available here are"cailliez","lingoes",and"none".The"cail-liez"and"lingoes"corrections add a constant to the eigenvalues to make themnon-negative.Default is"none".ValueThe list that was input as the argument‘D’with four new elements;the Principal Coordinates of the‘host’distance matrix and the Principal Coordinates of the‘parasite’distance matrix,as well as, a‘correction’object stating the correction used for negative eigenvalues and a‘note’object stating whether or not negative eigenvalues were present and therefore corrected.NoteTofind the Principal Coordinates of each distance matrix,we internally a modified version of the function ape::pcoa that uses vegan::eigenvals and zapsmallExamplesdata(gopherlice)library(ape)gdist<-cophenetic(gophertree)ldist<-cophenetic(licetree)D<-prepare_paco_data(gdist,ldist,gl_links)D<-add_pcoord(D)gl_links3 gl_links Gopher-lice interactionsDescriptionOne part of example data.The associations between pocket gophers and their chewing lice ectopar-asites.Usagedata(gopherlice)gophertree Gopher phylogenyDescriptionOne part of example data.The phylogeny of pocket gophers.Usagedata(gopherlice)licetree Lice phylogenyDescriptionOne part of example data.The phylogeny of chewing lice.Usagedata(gopherlice)4PACo PACo Performs PACo analysis.DescriptionTwo sets of Principal Coordinates are superimposed by Procrustes superimposition.The sum of squared residuals of this superimposition give an indication of how congruent the two datasets are.For example,in a biological system the two sets of Principal Coordinates can be composed from the phylogenetic distance matrices of two interacting groups.The congruence measured by PACo indicates how concordant the two phylogenies are based on observed ecological interactions between them.UsagePACo(D,nperm=1000,seed=NA,method="r0",symmetric=FALSE,proc.warnings=TRUE,shuffled=FALSE)ArgumentsD A list of class paco as returned by paco::add_pcoord which includes PrincipalCoordinates for both phylogenetic distance matrices.nperm The number of permutations to run.In each permutation,the network is ran-domized following the method argument and phylogenetic congruence betweenphylogenies is reassessed.seed An integer with which to begin the randomizations.If the same seed is used the randomizations will be the same and results reproducible.If NA a random seedis chosen.method The method with which to permute association matrices:"r0","r1","r2","c0", "swap","quasiswap","backtrack","tswap","r00".Briefly,"r00"produces theleast conservative null model as it only maintains totalfill(i.e.,total numberof interactions)."r0"and"c0"maintain the row sums and column sums,re-spectively,as well as the total number of interactions."backtracking"and anyof the"swap"algorithms conserve the total number of interactions in the ma-trix,as well as both row and column sums.Finally,"r1"and"r2"conserve therow sums,the total number of interactions,and randomize based on observedinteraction frequency.See vegan::commsim for more details.symmetric Logical.Whether or not to use the symmetric Procrustes statistic,or not.When TRUE,the symmetric statistic is used.When FALSE,the asymmetric is used.Adecision on which to use is based on whether one group is assumed to track theevolution of the other,or not.paco_links5 proc.warnings Logical.Make any warnings from the Procrustes superimposition callable.If TRUE,any warnings are viewable with the warnings()command.If FALSE,warnings are internally suppressed.Default is TRUEshuffled Logical.Return the Procrustes sum of squared residuals for every permutation of the network.When TRUE,the Procrustes statistic of all permutations is returnedas a vector.When FALSE,they are not returned.ValueA paco object that now includes(alongside the Principal Coordinates and input distance matrices)the PACo sum of sqaured residuals,a p-value for this statistic,and the PACo statistics for each randomisation of the network if shuffled=TRUE in the PACo call.NoteAny call of PACo in which the distance matrices have differing dimensions(i.e.,different num-bers of tips of the two phylogenies)will produce warnings from the vegan::procrustes function.These warnings require no action by the user but are merely letting the user know that,as the distance matrices had differing dimensions,their Principal Coordinates have differing numbers of columns.vegan::procrustes deals with this internally by adding columns of zeros to the smaller of the two until the are the same size.Examplesdata(gopherlice)require(ape)gdist<-cophenetic(gophertree)ldist<-cophenetic(licetree)D<-prepare_paco_data(gdist,ldist,gl_links)D<-add_pcoord(D)D<-PACo(D,nperm=10,seed=42,method="r0")print(D$gof)paco_links Contribution of individual linksDescriptionUses a jackknife procedure to perform bias correction on procrustes residuals(i.e.interactions)that are indicative of the degree to which individual interactions are more supportive of a hypothesis of phylogenetic congruence than others.Interactions are iteratively removed,the globalfit of the two phylogenies is reassessed and bias in observed residuals calculated and corrected.Usagepaco_links(D,.parallel=FALSE,proc.warnings=TRUE)6prepare_paco_dataArgumentsD A list of class paco as returned by paco::PACo..parallel If TRUE,calculate the jackknife contribution in parallel using the backend pro-vided by foreach.proc.warnings As in PACo.If TRUE,any warnings produced by internal calls of paco::PACo will be available for the user to view.If FALSE,warnings are internally sup-pressed.ValueThe input list of class paco with the added object jackknife which contains the bias-corrected resid-ual for each link.Examplesdata(gopherlice)require(ape)gdist<-cophenetic(gophertree)ldist<-cophenetic(licetree)D<-prepare_paco_data(gdist,ldist,gl_links)D<-add_pcoord(D)D<-PACo(D,nperm=10,seed=42,method="r0")D<-paco_links(D)prepare_paco_data Prepares the data(distance matrices and association matrix)for PACoanalysisDescriptionSimple wrapper to make sure that the matrices are sorted accordingly and to group them together into a paco object(effectively a list)that is then passed to the remaining steps of PACo analysis.Usageprepare_paco_data(H,P,HP)ArgumentsH Host distance matrix.This is the distance matrix upon which the other will besuperimposed.We term this the host matrix in reference to the original cophy-logeny studies between parasites and their hosts,where parasite evolution wasthought to track host evolution hence why the parasite matrix is superimposedon the host.P Parasite distance matrix.The distance matrix that will be superimposed on the host matrix.As mentioned above,this is the group that is assumed to track theevolution of the other.HP Host-parasite association matrix,hosts in rows.This should be a binary matrix.If host species aren’t in the rows,the matrix will be translated internally.residuals_paco7ValueA list with objects H,P,HP to be passed to further functions for PACo analysis.Examplesdata(gopherlice)library(ape)gdist<-cophenetic(gophertree)ldist<-cophenetic(licetree)D<-prepare_paco_data(gdist,ldist,gl_links)residuals_paco Return Procrustes residuals from a paco objectDescriptionTakes the Procrustes object from vegan::procrustes of the global superimpostion and pulls outeither the residual matrix of superimposition or the residual of each individual interaction(linkbetween host and parasite).Usageresiduals_paco(object,type="interaction")Argumentsobject An obejct of class procrustes as returned from PACo(and internally the vegan::procrustes function).In a PACo output this is D\$proc.type Character string.Whether the whole residual matrix(matrix)or the residualsper interaction(interaction)is desired.ValueIf type=interaction,a named vector of the Procrustes residuals is returned where names are theinteractions.If type=matrix,a matrix of residuals from Procrustes superimposition is returned.Examplesdata(gopherlice)library(ape)gdist<-cophenetic(gophertree)ldist<-cophenetic(licetree)D<-prepare_paco_data(gdist,ldist,gl_links)D<-add_pcoord(D,correction= cailliez )D<-PACo(D,nperm=100,seed=42,method= r0 )residuals_paco(D$proc)Index∗datasetsgl_links,3gophertree,3licetree,3add_pcoord,2gl_links,3gophertree,3licetree,3PACo,4paco_links,5prepare_paco_data,6residuals_paco,78。
The learning-curve sampling method applied to model-based clustering
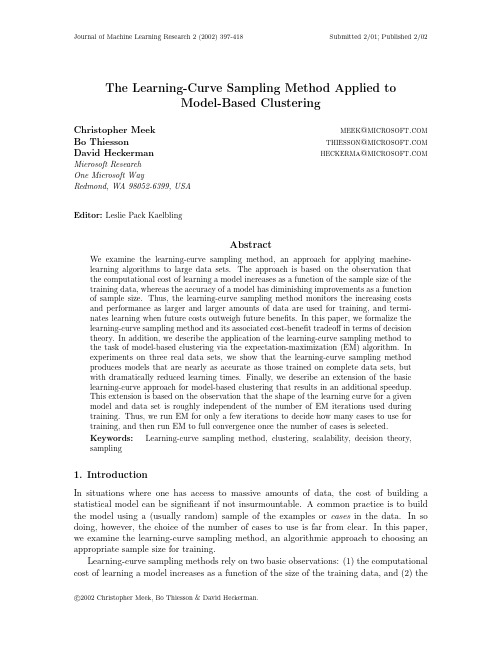
Journal of Machine Learning Research2(2002)397-418Submitted2/01;Published2/02 The Learning-Curve Sampling Method Applied toModel-Based ClusteringChristopher Meek meek@ Bo Thiesson thiesson@ David Heckerman heckerma@ Microsoft ResearchOne Microsoft WayRedmond,WA98052-6399,USAEditor:Leslie Pack KaelblingAbstractWe examine the learning-curve sampling method,an approach for applying machine-learning algorithms to large data sets.The approach is based on the observation that the computational cost of learning a model increases as a function of the sample size of the training data,whereas the accuracy of a model has diminishing improvements as a function of sample size.Thus,the learning-curve sampling method monitors the increasing costs and performance as larger and larger amounts of data are used for training,and termi-nates learning when future costs outweigh future benefits.In this paper,we formalize the learning-curve sampling method and its associated cost-benefit tradeoffin terms of decision theory.In addition,we describe the application of the learning-curve sampling method to the task of model-based clustering via the expectation-maximization(EM)algorithm.In experiments on three real data sets,we show that the learning-curve sampling method produces models that are nearly as accurate as those trained on complete data sets,but with dramatically reduced learning times.Finally,we describe an extension of the basic learning-curve approach for model-based clustering that results in an additional speedup.This extension is based on the observation that the shape of the learning curve for a given model and data set is roughly independent of the number of EM iterations used during training.Thus,we run EM for only a few iterations to decide how many cases to use for training,and then run EM to full convergence once the number of cases is selected.Keywords:Learning-curve sampling method,clustering,scalability,decision theory, sampling1.IntroductionIn situations where one has access to massive amounts of data,the cost of building a statistical model can be significant if not insurmountable.A common practice is to build the model using a(usually random)sample of the examples or cases in the data.In so doing,however,the choice of the number of cases to use is far from clear.In this paper, we examine the learning-curve sampling method,an algorithmic approach to choosing an appropriate sample size for training.Learning-curve sampling methods rely on two basic observations:(1)the computational cost of learning a model increases as a function of the size of the training data,and(2)theMeek,Thiesson and Heckermanperformance/accuracy of a model has diminishing improvements as a function of the size ofthe training data.The curve describing the performance as a function of the sample size ofthe training data is often called the learningcurve.The typical shape of a learning curveis concave(down)with performance approaching some limiting behavior.Thus,a learning-curve sampling method monitors the increasing costs and performance as larger and largeramounts of data are used for training,and terminates learning when the increasing costsoutweigh the benefit of increasing performance.In this paper,we formalize the learning-curve approach to sampling in terms of decisiontheory.In particular,we describe the use of utility as an overall measure of the qualityof a learning method,and derive algorithms for selecting sample size from the principle ofmaximum expected utility.In addition,we describe several high-utility learning-curve sampling methods for theparticular task of model-based clustering.We investigate a simple but widely used class ofmodels for clustering—namely,finite mixture models—with afixed,pre-specified numberof components and use the Expectation–Maximization(EM)algorithm to learn the modelparameters.In experiments on three real data sets,we show that the learning-curve sam-pling method produces models of high utility—ones that are nearly as accurate as thosetrained on complete data sets,but with dramatically reduced run times.Also in this paper,we describe an extension of the basic learning-curve approach formodel-based clustering that results in higher utilities by further reducing run time withoutloss in accuracy.Our approach is based on the observation that the shape of the learningcurve for a given model and data set is roughly independent of the number of EM iterationsused during training.Thus,we run EM for only a few iterations to decide how many casesto use for training,and then run EM to full convergence once the number of cases is selected.The paper is organized as follows.In Section2,we present our decision-theoretic for-mulation of the learning-curve sampling method.In Section3,we describe a model-basedapproach to clustering that uses the EM algorithm.In Section4,we apply the learning-curvesampling method to the task of model-based clustering and,in Section5,provide extensionsfor improving the method.In Section6,we present an empirical study that demonstratesthat our learning-curve sampling methods provide order-of-magnitude speedups on real dataand have higher utilities than alternative methods.Finally,in Section7we describe relatedwork and,in Section8,we provide a summary and directions for future work.2.A Decision-Theoretic Formulation of the Learning-Curve Sampling MethodAs we have discussed,the basic idea of a learning-curve sampling method is to iterativelyapply a training algorithm to larger and larger subsets of the data,until the future expectedcosts outweigh the future expected benefits associated with the training.In this section,wepresent a decision-theoretic formulation of this general approach.Given a data set D,let D1,D2,...,D n=D denote the sequence of data sets that are examined in the process of finding the appropriate sample size,denoted N lc.We shall assume that the data sets arenested—that is,D i⊂D i+1—so that|D i|<|D i+1|,where|D i|is the number of cases in data set D i.We shall also assume that,apart from this nesting,the cases in each D i arerandomly selected from D.The Learning-Curve Sampling MethodWhen we examine data set D i,we apply some trainingmethod to it,gather informationabout that application—for example,the accuracy of the resulting model and the time ittook to learn that model—and then determine whether to examine additional data sets.Expressed in decision-theoretic terms,our determination of when to stop is a sequentialdecision problem.At stage i in this decision problem,we have just run the training methodon data subset D i.At this point,we have two alternatives:(1)stop—that is,output thelearned model,or(2)continue.The choice to continue brings us to stage i+1of the decisionproblem.Decision theory(e.g.,Howard,1966)tells us that we should choose the alternativewith the maximum expected utility(MEU),where expectation is taken with respect to ouruncertainty(encoded in terms of probability)of the possible outcomes that followfrom thealternatives.Thus,once we have identified the possible sequences of data sets,the utilitiesassociated with the possible outcomes,and the uncertainties of those outcomes,our decisionis determined.Let us consider each of these ingredients.Thefirst ingredient,the sequence of data sets,may befixed—that is,chosen in advance—or chosen adaptively as data sets are examined.Two types offixed sequences have beenconsidered.John&Langley(1996)consider incrementally adding a constant number ofcases.Provost,Jensen&Oates(1999)consider incrementally adding a geometrically in-creasing number of cases.As argued by Provost et al.,when one does not have an accurateguess as to the“correct”number of cases to achieve the proper cost/benefit tradeoff,themethod of incrementally adding afixed number of cases can require an unreasonable numberof iterations when a large number of cases is needed.In contrast,when using a geometricschedule,one can quickly reach an appropriate sample size.For instance,if the cost of train-ing is roughly linear in the number of cases,then using a geometric schedule to train on datasets of size k·20,k·21,...,k·2i,until we reach some data set of size k·2i(N≤k·2i<2N), will require only a constant factor more computation than simply applying the trainingmethod to the data set of N cases.In situations where the sequence is adaptively selected,we can use points along thelearning curve for the smallest data sets D1,...,D i,in conjunction with a model for the shape of the learning curve(e.g.,Kadie,1995),to estimate future points along the curve. We can then use these estimates to expand the number of alternatives in our sequential decision problem to include a choice about the number of cases in D i+1.In this paper,we use thefixed geometric sequence and do not elaborate further on adaptive strategies.The second ingredient is the utility or“goodness”of stopping with data set D i.Wedecompose this utility into a benefit and cost.Let m i denote the model learned with thisdata set.A natural measure of cost is proportional to the total time it takes to producemodel m i.A natural measure of benefit is proportional to the accuracy of m i on the taskto which that model will be applied.Of course,the measure of accuracy will depend on thetask at hand.In the remainder of the paper,we shall consider a measure of accuracy thatis used commonly in practice:the log-likelihood of the model on holdout data D ho,denoted l(D ho|m i).Note that the learning-curve sampling method is applied in situations where the full data set D is extremely large.Consequently,there will be ample data to hold out.To combine these measures to produce an overall measure of utility,we need to scalebenefit and cost appropriately.One possibility is to determine both scales on a problem-by-problem basis.In this paper,we consider an approach that can be applied across a widerange of problems.In particular,we measure the benefit of stopping with model m i inMeek,Thiesson and Heckermanterms of the accuracy of that model relative to a model trained with all the data(m D)and a baseline model m base:benefit(m i)=l(D ho|m i)−l(D ho|m base)l(D ho|m D)−l(D ho|m base)(1)One simple choice for the baseline model is one in which all of the features are mutually independent and trained with a relatively small fraction of the data.We use this baseline model in our empirical study.The choice of this baseline model yields a measure of benefit that lies between0and1for a wide range of problems.(When the data set that produces m i is extremely small,this measure may be negative.Such occurrences,however,are rare.) Note that this approach is appropriate for density-estimation models and can be extended to classification/regression models in a straightforward fashion.To scale cost,we simply writecost(m i)=α·runtime i(2) where runtime i is total time required to produce model m i,andαis the relative importance of benefit to run time.This quantity,which depends on the preferences of the decision maker—the person who is controlling the execution of the algorithm—should be assessed on a problem-by-problem basis.We discuss this assessment later in this bining these two measures,we setutility(m i)=benefit(m i)−cost(m i)(3)The third ingredient for our decision problem are the uncertainties associated with the possible outcomes.These uncertainties are mostly problem specific,but commonly share an important ly,imagine we are at stage i of the decision,and want to decide whether to continue with larger data sets.As we look forward in time,the uncertainties associated with the benefits and costs of examining data set D k,k>i,increase with k. In particular,it will be extremely difficult to estimate these uncertainties for large k.This observation suggests that we may want to replace strict adherence to the MEU principle, which requires uncertainty estimates for all k,with an approximate procedure that uses only uncertainty estimates at the next stage.Let us consider a procedure with this property.At stage i of this procedure,we incor-rectly assume that there are only two alternatives:(1)stop now,and(2)learn model m i+1 and stop.We then choose the alternative among these two that maximizes our expected utility.If we choose alternative1,we indeed stop,returning model m i and its accuracy score.If we choose alternative2,we evaluate another decision problem,now at stage i+1. This strategy is often referred to as a“myopic”strategy,because it only looks one step into the future.According to this myopic strategy,we stop at stage i if and only if the expected utility of stopping at stage i+1is less than or equal to the expected utility of stopping at stage i—or,equivalently,if the expected increase in utility of moving from stage i to i+1is less than zero.In particular,according to Equations1through3,we stop if and only ifE i(benefit(m i+1)−benefit(m i))E i(runtime i+1−runtime i)≤α(4)wherehandvalueand its“Howmodelincremental benefits decrease and incremental costs increase as we progress through the stages of our decision problem,it makes sense to stop when the ratio of these two quantities falls belowα,the relative importance of benefit to cost.To understand this observation more precisely,consider Figure1,which shows a hypothetical plot of the expected benefit E i(benefit(m i+1))versus the expected run time E i(runtime i+1)as a function of stage i. For purposes of argument,the curve is shown to be continuous under the(temporary) assumption that the sample sizes of successive stages are closely spaced.The more important aspect of the curve is that it is concave down and non-decreasing so as to represent the progressive decrease in incremental-benefit-to-incremental-cost ratio.In general,the curve will have this shape when(1)the“expected learning curve”—the plot of expected benefit versus sample size—is concave down and non-decreasing,and(2)the rate of change of expected run time with respect to sample size is non-decreasing in sample size.Both conditions are satisfied often in practice,and are satisfied(roughly)for the specific problems we consider in the remainder of the paper.Now,consider the difference between the height of the curve and the height of a line with slopeαthrough the origin(also shown in thefigure)as a function of expected run time. This difference represents expected utility as a function of expected run time.Because theexpected-benefit-versus-expected-run-time curve is concave down and non-decreasing,thisMeek,Thiesson and Heckermandifference will be a maximum when the tangent to the curve has slopeα.Thus,according to the MEU principle,we should stop at a stage corresponding to point B in thefigure.Just beyond this point,the ratio of expected incremental benefit to expected incremental run time falls belowα—precisely the inequality in Equation4.(Arguments similar to this one are common in the discipline of cost-benefit analysis—for example,Pearce,1983.)When we relax our assumption that the sample sizes are closely spaced,wefind that the criterion expressed by Equation4may be non-optimal.For example,suppose points A and C in thefigure correspond to stages D i and D i+1.In this case,although our criterion yields a stopping point at stage i+1,the expected utility of stopping at stage i is slightly greater. In general,we may stop one step late,but never early—that is,our stopping criterion is conservative.We consider this approximate stopping criterion throughout the remainder of the paper. As we shall see,it can be computed efficiently and is amenable to improvement.3.Model-Based ClusteringIn this section,we describe the model-based approach to clustering usingfinite mixture models and howone can use the expectation maximization(EM)algorithm to learn such models.We focus on details important for the application of our learning-curve sampling method to model-based clustering described in Sections4and5.3.1Mixture ModelsLet X={X1,...,X L}be a multivariate random variable taking on values corresponding to observations of individual objects or cases.The goal of clustering is to form groups of objects that share similar values for X.In a model-based approach to clustering,we assume that our data is generated in the following fashion:1.An object is assigned to one of K(hidden)clusters with some probability,and2.Given that an object is in a cluster,its value for X is generated from some statisticalmodel specific to that cluster.More formally,let C be a discrete-valued variable taking on values c1,...,c K.The value of C corresponds to the unknown cluster assignment for an object.Then,we havep(x|θ)=Kk=1p(c k|θ)p k(X=x|c k,θk)=Kk=1πk p k(X=x|c k,θk)whereπk=p(c k|θ)is the marginal probability of the k th cluster(kπk=1),p k(x|c k,θk)isthe statistical model describing the distribution over the variables for an object in the k th cluster,andθ={π1,...,πk,θ1,...,θK}are the parameters of the model.The statistical model p(x|θ)is called afinite mixture model.For additional information onfinite mixture models see(e.g.)Titterington,Smith&Makov(1985)and McLachlan&Basford(1988).The Learning-Curve Sampling MethodIn model-based clustering,one specifies the form of the cluster-specific parametric mod-els p k(X=x|c k,θk),and then uses a data set to learn the specific cluster probabilities and the cluster-specific model parameters.The approach is called model-based clustering because a separate statistical model is used for each cluster.In our experiments,we concentrate on a simple but widely used class offinite mixture models where each component is described by a product of multinomials model:p k(x|c k,θk)=Li=1p(x i|θi k),where p(x i|θi k)is a multinomial distribution over the values for variable X i andθi k is the set of parameters.Here,we assume that,in each component,the variables X1,...,X L are mutually independent—that is,we assume that the statistical model for each component is a log-linear model with only main effects.This mixture model can alternatively be viewed as naive-Bayes models with a hidden class variable,also known as AutoClass models (Cheeseman&Stutz,1995).We further restrict our attention to mixture models with a fixed,pre-specified number of components.Given the parameters of a mixture model,we can assign an object(or case)to a cluster as follows.For an object with X=x,we use Bayes’rule to compute the probability distribution over the hidden variable C:p(c k|x,θ)=πk p k(x|c k,θk)Kj=1πj p j(x|c j,θj)(5)The probabilities p(c k|x,θ)are sometimes called membership probabilities and correspond to the object’s(fractional)cluster assignment.Once we have computed these probabilities,we can either assign the object to the cluster with highest probability—a hard assignment—or assign the object fractionally to the set of clusters according to this distribution—a soft assignment.3.2Learning Mixture Models from DataNoww e describe howto learn the parameters of afinite mixture model w ith know n number of components K,given training data D=(x1,...,x N).One possible criterion for doing so is to identify those parameter values forθthat maximize the likelihood of the training data:θML=argmaxθp(D|θ)=argmaxθNi=1p(x i|θ)This value is often referred to as the maximum likelihood(ML)estimate and p(D|θ)is the likelihood of the training data.Alternatively,one may have prior knowledge about the domain and can encode this information in the form of a prior probability distribution over the parameters,denoted p(θ).In this situation,a criterion for learning the parameters is to identify the parameter value ofθthat maximize the posterior probability ofθgiven our training data:θMAP=argmaxθp(θ|D)=argmaxθp(D|θ)p(θ)/p(D)Meek,Thiesson and Heckermanwhere p(θ|D)is the posterior on the parameters and the second identity follows by Bayes’rule.This value is often referred to as the maximum a posteriori(MAP)estimate.When used in conjunction with vague or non-informative priors,MAP estimates are smoothed (i.e.,less extreme)versions of ML estimates.In the work described in this paper we learn MAP estimates for the parametersθusing the diffuse Dirichlet prior described in Cooper &Herskovits(1992).In situations in which the a statistical model has an unobserved variable—as is the case withfinite mixture models—one can use the well-known expectation maximization (EM)algorithm to obtain ML and MAP estimates(Dempster,Laird&Rubin1977).The EM algorithm is given starting values for the parameters,and then iterates between an Expectation or E step and a Maximization or M step until successive parameter values are stable.In the E step of the algorithm,given a current value of the parametersθ,w e fractionally assign an object with X=x to cluster c k using the membership probabilities given by Equation5.In the M step of the algorithm,we pretend that these fractional assignments correspond to real data,and reassignθto be the MAP estimate given this fictitious data.By iteratively applying the E step and M step,we monotonically improve the estimates of the model parametersθ,ensuring convergence(under fairly general conditions) to a local maximum of the posterior distribution(or a maximum likelihood estimate)forθ.In our experiments,we initialize the parameters of the EM algorithm as follows.We choose the parametersπ1,...,πK to be equal,and we set the parameters of our component modelsθk by estimating the parameters for a single-component cluster model and then randomly perturbing the parameter values by a small amount to obtain K sets of param-eters.This approach is described in detail in Thiesson,Meek,Chickering&Heckerman, 1999.The convergence criterion that we use to terminate the EM algorithm is one that is commonly ly,we converge when the relative improvement in log-posterior (or log-likelihood)of the training data between successive EM iterations relative to the total improvement in log-posterior(or log-likelihood)over the initial model is less than a convergence thresholdγ.4.A Learning-Curve Sampling Method for ClusteringIn this section,we describe how to apply the learning curve sampling method described in Section2to the problem of model-based clustering.We call this method the standard learning-curve sampling methodIn applying the learning curve approach to model-based clustering,we make several approximations.To help illustrate the approximate validity of the assumptions,we consider three real-world data sets.We shall also use these data sets in our experiments.We note that the only criterion we used to select these data sets was large sample size,and that our method was developed prior to the examination of any of these data sets.The three data sets that we consider are the MSNBC,,and USCensus1990data sets.The MSNBC data set is derived from web logs for one day in1998for the website.It records which of the303most popular stories on that day were read by each of the visitors.The data set is derived from the web logs for one day in2000for the web site.It records which of the775most popular areas or“vroots”of the site were visited by each person.In each of these data sets,cases correspond to people,The Learning-Curve Sampling Methodand variables correspond to possibly viewed items.Both of these data sets are sparse in the sense that—on average—a person only views a few items.The USCensus1990data set, available from the UC Irvine KDD Archive,is derived from the one percent sample of the Public Use Microdata Samples(PUMS)person records from the full1990census sample (allfifty states and the District of Columbia but not including“PUMA Cross State Lines One Percent Persons Record”).Each case corresponds to a person and has68categorical variables.The MSNBC,,and USCensus1990data sets contain597,971,1,938,877, and2,458,284cases,respectively.Nowlet us examine the expectations in our stopping criterion for stage i,Equation4. An examination of the EM algorithm shows that E i(runtime i+1)is given byE i(runtime i+1)∼=runtime i+c1·E i(I i+1)·|D i+1|+c2·E i(I i+1)+c3where c1,c2,and c3are constants,and E i(I i+1)is the expected number of times the EM algorithm iterates(when applied to D i+1)before reaching convergence.Thefirst term in the sum is simply the known time to reach stage i.The second and third terms correspond to the time spent by the EM algorithm in the E and M steps,respectively.The fourth term corresponds to the time spent evaluating the accuracy of the model on the holdout set.(The time to load the clustering algorithm and initialize data structures in memory is insignificant.)The constants c1,c2,and c3are known once thefirst data set in the sequence has been evaluated.Furthermore,in our experience,we have found that the number of EM iterations is roughly constant for afixed convergence thresholdγ.Figure2shows the number of iterations versus sample size for the MSNBC, and USCensus1990 domains.Consequently,we use the approximationE i(I i+1)∼=1iij=1I j≡¯I i(6)Note that,at stage i,the quantities I1,...,I i are known with certainty.The remaining quantity that we need at stage i is the expected incremental benefit E i(benefit(m i+1)−benefit(m i)).Using Equation1and an approximation in which we take expectations of the numerator and denominator separately,we obtainE i(benefit(m i+1)−benefit(m i))∼=E i(l(D ho|m i+1)−l(D ho|m i))E i(l(D ho|m D))−l(D ho|m base).(7)In addition,we can approximate both the numerator and denominator in this expression. To illustrate the approximation for the numerator,consider the learning curves for the MSNBC,,and USCensus1990data sets shown in Figure3.Thisfigure provides two plots of the learning curves for each of the data sets;one where the x-axis is linear in the sample size and the other logarithmic.In particular,the learning curves are linear or slightly concave down when sample size is plotted on the logarithmic scale.Consequently, because we are using a sequence of data sets in which data set size increases geometrically, it follows thatE i(l(D ho|m i+1)−l(D ho|m i))<∼l(D ho|m i)−l(D ho|m i−1)(8)Meek,Thiesson andHeckermanFigure2:Number of iterations to convergence as a function of convergence thresholdγand sample size.The Learning-Curve Sampling MethodIn this work,we shall use the right-hand side of Equation8as an approximation for the left-hand side.The approximation is conservative in that it will tend to over-estimate the expected change in benefit,and thus bias the stopping decision toward late stopping.Also, because we are computing differences,this approximation requires that we stop no sooner than stage i=2.For the denominator of Equation7,we use the simple approximationE i(l(D ho|m D))∼=l(D ho|m i)(9) If the learning curve is noisy,a better approximation is the maximum over l(D ho|m1),..., l(D ho|m i)).In our experiments,however,the better approximation is not needed.In either case,the approximation is conservative.Given the approximations associated with Equations4through9,we can re-write the stopping criterion for stage i,given in Equation4,asl(D ho|m i)−l(D ho|m i−1)/(c1·¯I i·|D i+1|+c2·¯I i+c3)≤α(10) l(D ho|m i)−l(D ho|m base)We determine l(D ho|m base)before considering any data sets in the sequence.Consequently, at stage i,all the quantities in this expression are known.Finally,recall the conditions from Section2needed for the myopic stopping criterion Equation4to be near optimal:(1)the“expected learning curve”—the plot of expected benefit versus sample size—should be concave down and non-decreasing,and(2)the rate of change of expected run time with respect to sample size should be non-decreasing in sample size.We note that,in our approach using EM for model-based clustering,both conditions are roughly satisfied.In particular,with a few exceptions due to small amounts of noise,the plots on the left-hand-size of Figure3are concave down and non-decreasing Furthermore, assuming E i(I i+1)does not decrease substantially with i,expected run time(Equation4) satisfies the second condition.5.Speeding up the Learning-Curve Sampling Method for ClusteringIn this section,we consider an extension that obtains higher utilities than the standard learning-curve sampling method applied to model-based clustering.The extension substan-tially reduces training time without significantly affecting model accuracy.To understand this extension,consider again the learning curves in Figure3.Here,we see that learning curves for different EM convergence levels(γ)are roughly parallel for each of the data sets.We have found that this is a common property for data sets.This property suggests the following extension.When determining the expected benefit for a given data set D i,use an ab b reviated trainingmethod—EM run to a high convergence threshold or run for only a relatively fewiterations—to obtain an approximate value.Then,make the decision to stop or continue based on this approximation.Finally,once the decision has been made to stop,learn a model on the selected data set by running EM to full convergence.In the following section,we show that this extension can increase the utilities of the methods—that is,increase computational efficiency without sacrificing much accuracy.In the remainder of this section,we examine the details of the approach.。
Agilent 4294A精确阻抗分析操作手册
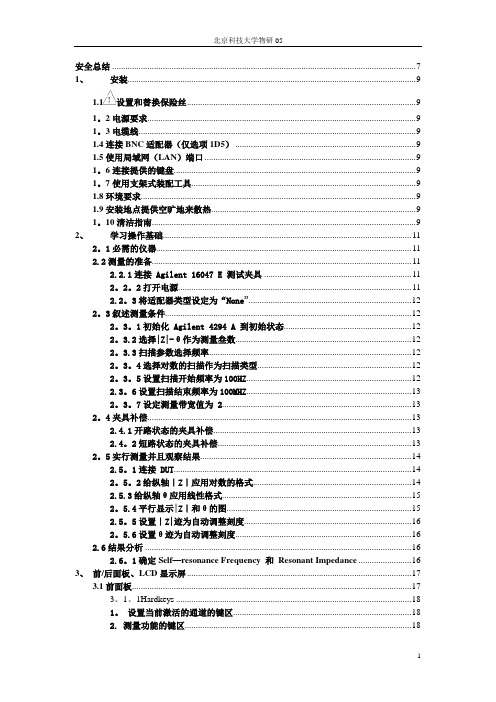
安全总结 (7)1、安装 (9)1.1设置和替换保险丝 (9)1。
2电源要求 (9)1。
3电缆线 (9)1.4连接BNC适配器(仅选项1D5) (9)1.5使用局域网(LAN)端口 (9)1。
6连接提供的键盘 (9)1。
7使用支架式装配工具 (9)1.8环境要求 (9)1.9安装地点提供空旷地来散热 (9)1。
10清洁指南 (9)2、学习操作基础 (11)2。
1必需的仪器 (11)2.2测量的准备 (11)2.2.1连接 Agilent 16047 E 测试夹具 (11)2。
2。
2打开电源 (11)2.2。
3将适配器类型设定为“None” (12)2。
3叙述测量条件 (12)2。
3。
1初始化 Agilent 4294 A 到初始状态 (12)2。
3.2选择|Z|-θ作为测量叁数 (12)2。
3.3扫描参数选择频率 (12)2。
3。
4选择对数的扫描作为扫描类型 (12)2。
3。
5设置扫描开始频率为100HZ (12)2.3。
6设置扫描结束频率为100MHZ (13)2。
3。
7设定测量带宽值为 2 (13)2。
4夹具补偿 (13)2.4.1开路状态的夹具补偿 (13)2.4。
2短路状态的夹具补偿 (13)2。
5实行测量并且观察结果 (14)2.5。
1连接 DUT (14)2。
5。
2给纵轴|Z|应用对数的格式 (14)2.5.3给纵轴θ应用线性格式 (15)2。
5.4平行显示|Z|和θ的图 (15)2.5。
5设置|Z|迹为自动调整刻度 (16)2。
5.6设置θ迹为自动调整刻度 (16)2.6结果分析 (16)2.6。
1确定Self—resonance Frequency 和Resonant Impedance (16)3、前/后面板、LCD显示屏 (17)3.1前面板 (17)3。
1。
1Hardkeys (18)1。
设置当前激活的通道的键区 (18)2. 测量功能的键区 (18)3. 与激励有关的键区 (19)4. 数字输入键区 (19)5. 关于定位记号功能的键区 (19)6. 关于仪器状态的键区 (20)7。
simplis教程
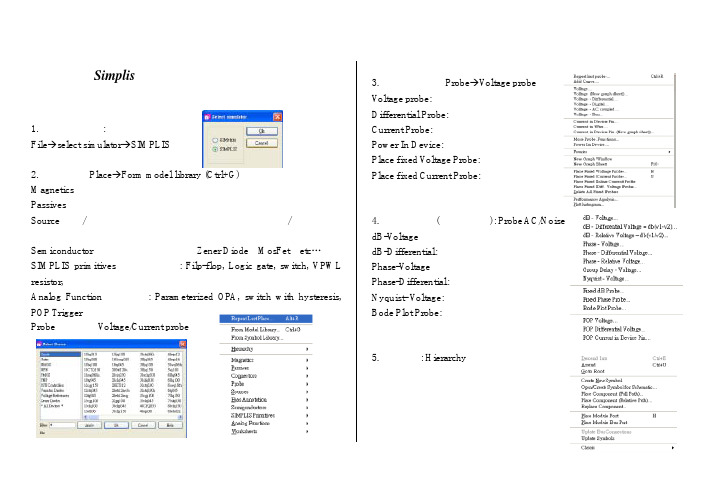
C1 330u
1 R1
AC 1 V2
Transient response
S1
12 V1
D1
10u
Vout
L1
100n C2
C1 330u
32c t q030
I(o)
I1
U1
X1
100k R4
10k R2
N I / T U O =
N I T U O
X2
22n C2
V5
2.5 V3
5 V4
100n C3
SIMPLIS Mode – Buck Converter Close Loop
S1
10u
Vout
I(S1) 12 V1
D1
L1
C1 330u
1 R1
32ctq030
AC 1 V2
T U N I / O T N = I
U O
U1 Comparator
100n C2
U1
X2
V5 waveform Generator
S1
I (S 1 ) 12 V1
10u
Vout
L1
D1 32c tq030
C1 330u
1 R1
AC 1 V2
T U O N I
N I / T U O =
A / ) 1 S ( I
100n C2
U1
X1
100k R4
10k R2
X2
V5
2.5 V3
5 V4
100n C3
10k R3
1k R5
time/uSecs
Critical Parts:
1. I1 Current waveform generator: One pulse
SysmeS i血液分析仪作业指导书
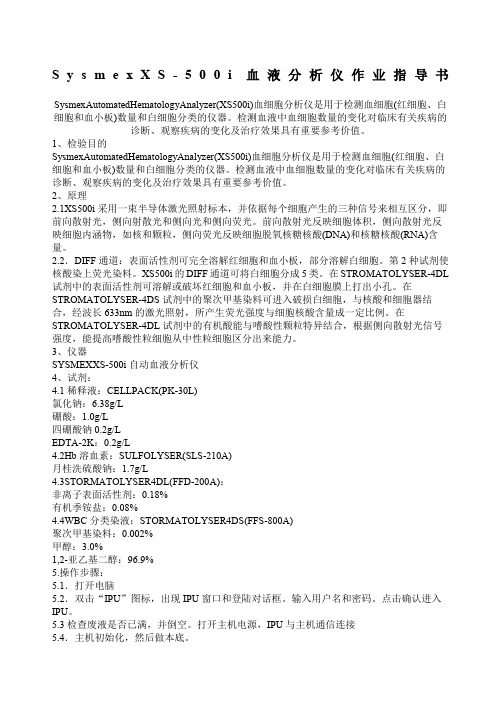
S y s m e x X S-500i血液分析仪作业指导书SysmexAutomatedHematologyAnalyzer(XS500i)血细胞分析仪是用于检测血细胞(红细胞、白细胞和血小板)数量和白细胞分类的仪器。
检测血液中血细胞数量的变化对临床有关疾病的诊断、观察疾病的变化及治疗效果具有重要参考价值。
1、检验目的SysmexAutomatedHematologyAnalyzer(XS500i)血细胞分析仪是用于检测血细胞(红细胞、白细胞和血小板)数量和白细胞分类的仪器。
检测血液中血细胞数量的变化对临床有关疾病的诊断、观察疾病的变化及治疗效果具有重要参考价值。
2、原理2.1XS500i采用一束半导体激光照射标本,并依据每个细胞产生的三种信号来相互区分,即前向散射光,侧向射散光和侧向光和侧向荧光。
前向散射光反映细胞体积,侧向散射光反映细胞内涵物,如核和颗粒,侧向荧光反映细胞脱氧核糖核酸(DNA)和核糖核酸(RNA)含量。
2.2.DIFF通道:表面活性剂可完全溶解红细胞和血小板,部分溶解白细胞。
第2种试剂使核酸染上荧光染料。
XS500i的DIFF通道可将白细胞分成5类。
在STROMATOLYSER-4DL 试剂中的表面活性剂可溶解或破坏红细胞和血小板,并在白细胞膜上打出小孔。
在STROMATOLYSER-4DS试剂中的聚次甲基染料可进入破损白细胞,与核酸和细胞器结合,经波长633nm的激光照射,所产生荧光强度与细胞核酸含量成一定比例。
在STROMATOLYSER-4DL试剂中的有机酸能与嗜酸性颗粒特异结合,根据侧向散射光信号强度,能提高嗜酸性粒细胞从中性粒细胞区分出来能力。
3、仪器SYSMEXXS-500i自动血液分析仪4、试剂:4.1稀释液:CELLPACK(PK-30L)氯化钠:6.38g/L硼酸:1.0g/L四硼酸钠0.2g/LEDTA-2K:0.2g/L4.2Hb溶血素:SULFOLYSER(SLS-210A)月桂洗硫酸钠:1.7g/L4.3STORMATOLYSER4DL(FFD-200A):非离子表面活性剂:0.18%有机季铵盐:0.08%4.4WBC分类染液:STORMATOLYSER4DS(FFS-800A)聚次甲基染料:0.002%甲醇:3.0%1,2-亚乙基二醇:96.9%5.操作步骤:5.1.打开电脑5.2.双击“IPU”图标,出现IPU窗口和登陆对话框。
- 1、下载文档前请自行甄别文档内容的完整性,平台不提供额外的编辑、内容补充、找答案等附加服务。
- 2、"仅部分预览"的文档,不可在线预览部分如存在完整性等问题,可反馈申请退款(可完整预览的文档不适用该条件!)。
- 3、如文档侵犯您的权益,请联系客服反馈,我们会尽快为您处理(人工客服工作时间:9:00-18:30)。
系统分析与设计第4章:信息收集-交互式方法2信息需求一系列问题意见运用技术1. 面谈41. 面谈在和别人面谈之前,必须先同自己进行有效的面谈。
不仅需要知道自己的倾向,还要知道如何影响自己的理解。
教育,智力,素养,情感和道德标准都会对面谈时听到的内容起着强大的过滤作用。
5发现问题:系统输出的结果?丰富多彩更多的专业知识发现问题:系统内部人员状态?外部环境对系统的影响?定义问题陈述:议题(当前情况):目标(期望情况):1. 面谈为什么要进行面谈?要提什么问题?如何才能使面谈成功?如何是面谈对象也感到满意?大连理工大学软件学院9“大学生校园生活辅助系统”为什么要进行面谈?发现需求明确功能改善要提什么问题?系统输出要提什么问题?系统内部状态外部环境如何才能使面谈成功?如何是面谈对象也感到满意?面谈要求:目标明确,一般采用问与答的形式。
获取的信息:获取面谈对象的观点当前系统的状态组织和个人的目标非正规过程的感受大连理工大学软件学院141. 调查面谈对象的观点观点有时比事实更重要,更有启发性。
2. 获悉面谈对象的感受3. 目标是面谈时可以收集的重要信息。
从硬数据中得到的事实可以解释过去的成绩。
目标能够反映组织的未来。
4. 考察关键的HCI(人-机交互)问题人类工程系统的可用性系统的令人满意性系统在支持个人任务上有多大用处151. 阅读背景材料2.4.5. 决定问题的类型和结构大连理工大学软件学院161. 阅读背景材料通过公司Web站点,年度报告,事实通信,介绍组织的出版物。
特别留意组织成员用来描述自己及组织的语言:设法统一一种共同词汇。
大连理工大学软件学院172. 确定面谈目标提出的问题,4 -6个问题涉及HCI,信息处理,决策行为。
涉及:HCI方面:系统的有用性和可用性如何适应物理方面的如何适应用户的认知能力是否迷人的,美观的外表使用该系统是否得到了回报,预期的结果信息资源,信息格式,信息的性质决策频度决策样式大连理工大学软件学院18“大学生校园生活辅助系统”面谈目标1.1 面谈准备的5个步骤3. 决定面谈对象所有层次上载某些方面受到系统影响的关键人物。
力争尽可能多地收集用户的需求。
4. 准备面谈的对象提前通知,使面谈人有时间去思考面谈事宜。
面谈要控制在45分钟之内,最多不要超过1小时。
大连理工大学软件学院20“大学生校园生活辅助系统”N = 2 X 5 X 6 X 2 X 20 = 2400 人1.1 面谈准备的5个步骤5. 决定问题的类型和结构写下在确定面谈目标是发现的涵盖HCI和决策等关键领域的问题。
正确的提问技巧是面谈的核心。
问题的基本形式:开放式问题,封闭式问题。
面谈的3种组织模式:金字塔结构,漏斗结构,菱形结构。
大连理工大学软件学院221.2 问题类型开放式问题:面谈对象答复问题的选择不受限制。
答复可以是2个词,也可以是2段话。
有何看法?目标是什么?如何处理?最大的挫折是什么?大连理工大学软件学院23“大学生校园生活辅助系统”开放式问题的优势:让面谈对象感到自在可以收集面谈对象的词汇,放映他们的教育程度,价值标准,态度和信念提供丰富的细节对未采用的进一步提问方法有启迪作用让面谈对象更感兴趣允许更多的自发性会见者更容易措词会见者没有准备的紧急关头采用这种类型大连理工大学软件学院25开放式问题的缺点:提此类问题可能会产生太多不相干的细节面谈可能失控开放式的回答会花费大量的时间才能获得有用的信息量可能会使会见者看上去没有准备可能产生这样的现象:会见者在对面谈做没有实际目标的调查大连理工大学软件学院26封闭式问题面谈对象可能的回答是受到限制的。
回答只能用限定的数字:0,1或者n类似“多选题”有多少个XXX?是否XXX?谁能够XXX?大连理工大学软件学院27“大学生校园生活辅助系统”封闭式问题的优势节省时间容易对比面谈切中要点保持对面谈的控制权快速探讨大范围的问题可到贴切的数据大连理工大学软件学院29封闭式问题的缺点是面谈对象厌烦得不到丰富的细节处于上述原因,失去主要思想不能建立会见者和面谈对象的友好关系大连理工大学软件学院30大连理工大学软件学院31时间的使用效率数据的精度广度和深度需要面谈的技能分析的难易度数据的可靠性开放式问题封闭式问题底底底广多难高高高窄少易调查问题目的是深究答复得到面谈对象要表达的更多意思澄清面谈对象的观点既可以是开放问题,也可以是封闭式问题为什么?举个例子?更详细的说明?大连理工大学软件学院32“大学生校园生活辅助系统”调查问题1.3 按逻辑顺序安排问题1. 使用金字塔结构采用归纳组织方式。
会见者以很具体的问题(封闭式问题)开始然后,使用开放式问题允许面谈对象用更一般的回答来扩展这个话题。
面谈对象需要对这个话题预热,就采用金字塔结构。
想结束谈话的时候,使用:请概括一下你的想法。
大连理工大学软件学院34“大学生校园生活辅助系统”你参加学校的社团了么?你们社团主要在做什么?你期望你们社团应该在什么方面有所改进?你认为社团对你们的大学生活的重要性?351.3 按逻辑顺序安排问题2. 使用漏斗结构采用演绎的方法以一般的开放式问题开始然后,用封闭式问题缩小可能的答复。
开始一场面谈提供了一种容易而轻松的途径当面谈对象对这个话题有情绪,需要自由表达这些情绪的时候,才能采用漏斗形提问顺序大连理工大学软件学院36“大学生校园生活辅助系统”如果提供学习这些知识的条件,你能够很好的自学这些知识么?在学习方面,除了学校所授的课程外,你还想学些什么?你认为什么样的大学生活才是丰富多彩的?1.3 按逻辑顺序安排问题3. 使用菱形结构金字塔结构和漏斗结构的结合以一种非常明确的问题开始然后,考察一般的问题最后,得出一个明确的结论首先提出一些简单的封闭式问题,为面谈过程做好铺垫。
中间阶段,询问对明显没有“正确”答案的一般话题的看法。
然后,会见者再次限制问题以获得明确的答案。
缺点:花费的时间比其他2个要长。
大连理工大学软件学院38“大学生校园生活辅助系统”你最近一次去开发区是什么时候?你经常去开发区么去开发区一般要做些什么?大连理工大学软件学院在购物方面,什么东西你觉得需要到开发区来买?你需要到开发区购物是价格的原因,还是因为样式的原因?你觉得如果网站可以提供代买你想要的商品,你会选择在网上购买么?1.4 撰写面谈报告面谈本身结束了,但是处理面谈的数据工作才刚刚开始。
撰写面谈报告来获取面谈的实质。
尽可能在面谈结束后马上写出面谈报告。
保证面谈数据质量初步总结后,就要深究细节标出面谈的要点,和自己的观点与面谈对象一起评审面谈报告让面谈对象知道,我们非常愿意花时间了解他们的观点和感受。
大连理工大学软件学院40“大学生校园生活辅助系统”观点感觉目标2. 联合应用程序设计422. 联合应用程序设计面谈:不可避免出现没有想象中有用的情况。
不仅耗时,容易出错,数据容易被误解。
联合应用程序设计:Joint Application Design: JAD节省个人面谈的时间(减少成本)改善信息需求评估结果的质量通过多方一起参加的过程,获得用户对新系统的更多认可大连理工大学软件学院432.1 支持使用JAD的条件用户想有新东西,不希望用常规的方法解决典型的问题。
公司文化支持不同层次的职员间联合问题求解。
分析员预测,通过一对一面谈获得的观点没有通过广泛的分组练习获得的丰富。
组织工作流允许关键职员离职2 –4 天。
大连理工大学软件学院44主办者:以为地位较高的人来主办JAD会议负责开始和结束JAD会议这个人将是组织对系统项目承诺的重要的,显著的象征。
大连理工大学软件学院45系统分析员通常是被动角色全程参加JAD会议,倾听用户谈话和他们的需求。
如果JAD会议中提出了与成本不相称的解决方法,应给出内行的观点。
避免事后因成本过高而舍弃系统开发。
大连理工大学软件学院46会议主持人不应该是系统分析员一个具有优秀沟通能力的人以便会议顺利的进展他能够使小组集中关注重要的系统问题,能够使小组满意的协商,解决冲突帮助小组达成一致意见大连理工大学软件学院478 –12 位不同阶层的用户他们清楚工作需要的信息在新的,或改进的系统中,他们想要什么。
1 –2 位系统观察员来自其他职能单位的分析员,技术专家提供技术上的解释,和忠告。
1位抄写员正式记下已完成的所有事情。
大连理工大学软件学院482.3 召开JAD会议地点建议召开2 –4 天的脱产会议选择一个远离单位的,舒服的环境不让参与者分心,使参与者日常工作的责任最少会议室应该很大,足以容纳邀请人。
安排好JAD会议日程确保所有被邀人都能够出席JAD会议提前一个星期左右,用半天时间召开研究会议大连理工大学软件学院492.4 完成项目活动的结构化分析检查系统中如下要点:计划,接受,收据处理/跟踪,监视和指派,处理,记录,发送和评估每一个主题:一下方式提问和回答:对谁,什么,怎样,何处,为什么JAD会议的分析员应该得到抄写员的笔记准备一份基于会议上发生的事情的规范文档提出管理目标,项目范围和边界包括系统特性大连理工大学软件学院50。