An equation involving the Smarandache function
Fredholm Determinants, Differential Equations and Matrix Models
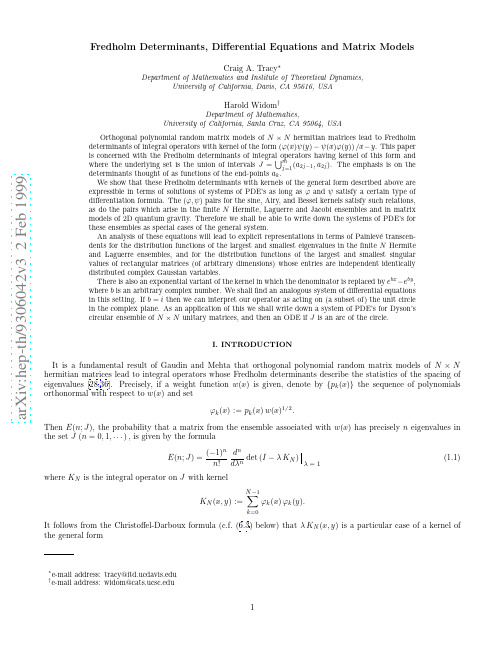
(1.1)
where KN is the integral operator on J with kernel
N −1
KN (x, y ) :=
k=0
ϕk (x) ϕk (y ).
It follows from the Christoffel-Darboux formula (c.f. (6.3) below) that λ KN (x, y ) is a particular case of a kernel of the g=
j =1
(a2j −1 , a2j ).
The emphasis is on the determinants thought of as functions of the end-points ak . If we denote the operator itself by K then it is well known that ∂ log det (I − K ) = (−1)k−1 R(ak , ak ) (k = 1, · · · , 2m) ∂ak (1.3)
∗ †
e-mail address: tracy@ e-mail address: widom@
1
K (x, y ) :=
ϕ(x)ψ (y ) − ψ (x)ϕ(y ) . x−y
(1.2)
This paper is concerned with the Fredholm determinants of integral operators having kernel of this form and where the underlying set is the union of intervals
Harold Widom†
关于一些数论函数的性质研究
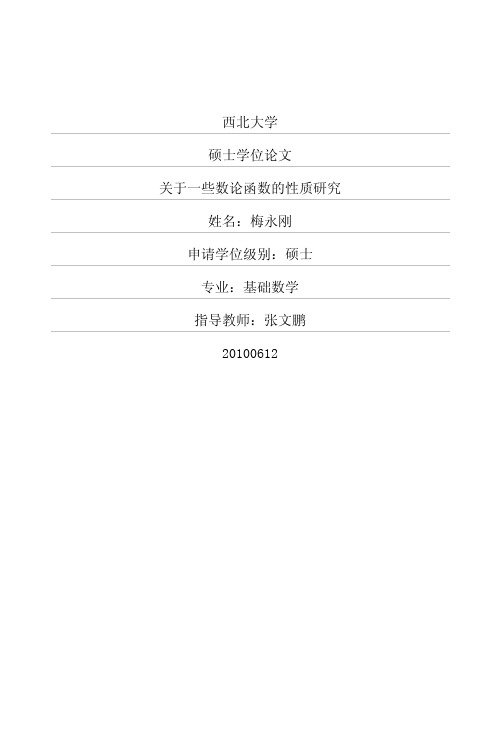
this paper,to the random Dirichlet source characteristic X,砂,we have given double L-and expresses c(七,l,d;x,矽)simple formula.Compare with Terhune double L-and the formula,when x(一1)砂(一1)=(一1)七+“-d+1,we obtained seal form value of c(七,l,d;X,砂).
2
两北大学硕士学位论文
第 弟一 二早 章 数 鳅T论匕间简7I介’
§2.1数论的发展简史
人类从使用数字开始,生活中就伴随着自然数.随着实践的需要,数的概念 被进一步扩充,自然数被叫做正整数,而把它们的相反数叫做负整数,介于正整 数和负整数中间的中性数叫做0.它们合起来叫做整数(注:现在自然数的概 念有了改变,包括正整数和0).对于整数可以施行加、减、乘、除四种运算, 叫做四则运算.随着人们在对整数进行运算的应用和研究中,逐步熟悉了整数 的特性.如,整数可分为两大类:奇数和偶数.利用整数的一些基本性质,可以 进一步探索许多有趣和复杂的数学规律,正是这些特性的魅力,吸引了古往今 来许多的数学家和学者不断地进行研究和探索.
ll
the methods of Elementary number theory and Analytic number theory,and several asymptotic formulae are obtained.
涉及非体积功的热力学基本方程
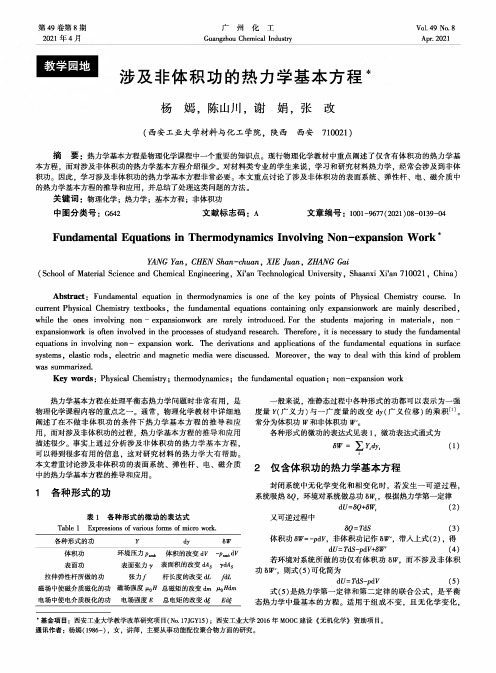
第49卷第8期2021年4月广州化工Guangzhou Chemical IndustryVol.49No.8Apr.2021涉及非体积功的热力学基本方程杨嫣,陈山川,谢娟,张改(西安工业大学材料与化工学院,陕西西安710021)摘要:热力学基本方程是物理化学课程中一个重要的知识点。
现行物理化学教材中重点阐述了仅含有体积功的热力学基本方程,而对涉及非体积功的热力学基本方程介绍很少。
对材料类专业的学生来说,学习和研究材料热力学,经常会涉及到非体积功。
因此,学习涉及非体积功的热力学基本方程非常必要。
本文重点讨论了涉及非体积功的表面系统、弹性杆、电、磁介质中的热力学基本方程的推导和应用,并总结了处理这类问题的方法。
关键词:物理化学;热力学;基本方程;非体积功中图分类号:G642文献标志码:A文章编号:1001-9677(2021)08-0139-04 Fundamental Equations in Thermodynamics Involving Non-expansion Work*YANG Yan,CHEN Shan-chuan,XIE Juan,ZHANG Gai(School of Material Science and Chemical Engineering,Xi9an Technological University,Shaanxi Xi'an710021,China) Abstract:Fundamental equation in thermodynamics is one of the key points of Physical Chemistry course・In current Physical Chemistry textbooks,the fundamental equations containing only expansionwork are mainly described, while the ones involving non-expansionwork are rarely introduced.For the students majoring in materials,non-expansionwork is often involved in the processes of studyand research.Therefore,it is necessary to study the fundamental equations in involving non-expansion work.The derivations and applications of the fundamental equations in surface systems,elastic rods,electric and magnetic media were discussed.Moreover,the way to deal with this kind of problem was summarized.Key words:Physical Chemistry;thermodynamics;the fundamental equation;non-expansion work热力学基本方程在处理平衡态热力学问题时非常有用,是物理化学课程内容的重点之一。
Eyring equation - Wikipedia
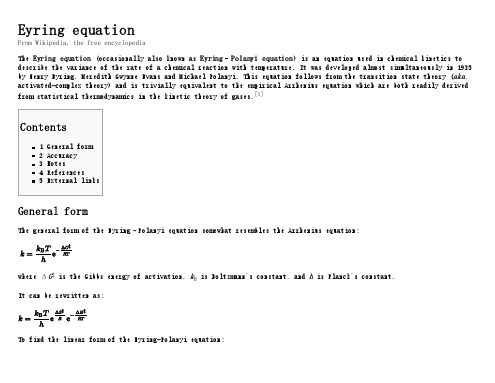
Notes
References
1. Chapman & Enskog 1939
Evans, M.G.; Polanyi M. (1935). "Some applications of the transition state method to the calculation of reaction velocities, especially in solution". . : 875–894. doi:10.1039/tf935vation
entropy of activation
A certain chemical reaction is performed at different temperatures and the reaction rate is determined. The plot of versus gives a straight line with slope from which the enthalpy of activation can be derived and with intercept from which the entropy of activation is derived.
Eyring–Polanyi equation
aka
from statistical thermodynamics in the kinetic theory of gases.[1]
Contents
1 2 3 4 5 General form Accuracy Notes References External links
General form
The general form of the Eyring–Polanyi equation somewhat resembles the Arrhenius equation: where Δ ‡ is the Gibbs energy of activation, It can be rewritten as:
üèDòáò×ù für Mathematik in den Naturwissenschaften Leipzig
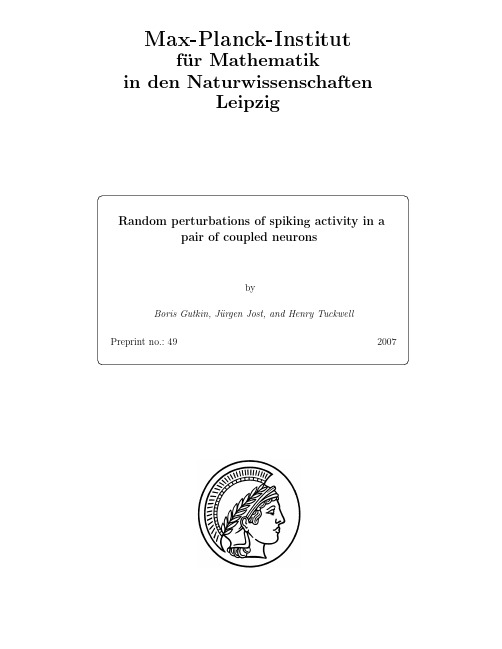
f¨u r Mathematikin den NaturwissenschaftenLeipzigRandom perturbations of spiking activity in apair of coupled neuronsbyBoris Gutkin,J¨u rgen Jost,and Henry TuckwellPreprint no.:492007Random perturbations of spiking activity in apair of coupled neuronsBoris Gutkin∗,J¨u rgen Jost and Henry C.Tuckwell†May14,2007AbstractWe examine the effects of stochastic input currents on thefiring be-haviour of two coupled Type1or Type2neurons.In Hodgkin-Huxleymodel neurons with standard parameters,which are Type2,in the bistableregime,synaptic transmission can initiate oscillatory joint spiking,butwhite noise can terminate it.In Type1cells(models),typified by aquadratic integrate andfire model,synaptic coupling can cause oscilla-tory behaviour in excitatory cells,but Gaussian white noise can againterminate it.We locally determine an approximate basin of attraction,A,of the periodic orbit and explain thefiring behaviour in terms of theeffects of noise on the probability of escape of trajectories from A.1IntroductionHodgkin(1948)found that various squid axon preparations responded in quali-tatively different ways to applied currents.Some preparations gave a frequency offiring which rose smoothly from zero as the current increased whereas oth-ers manifested the sudden appearance of a train of spikes at a particular input current.Cells that responded in thefirst manner were called Class1(which we refer to as Type1)whereas cells with a discontinuous frequency-current curve were called Class2(Type2).Mathematical explanations for the two types are found in the bifurcation which accompanies the transition from rest state to a periodicfiring mode.For Type1behaviour,a resting potential vanishes via a saddle-node bifurcation whereas for Type2behaviour the instability of the rest point is due to an Andronov-Hopf bifurcation,see Rinzel and Ermentrout (1989).Stochastic effects in thefiring behaviour of neurons have been widely reported, discussed and analyzed since their discovery in the1940’s.One of thefirst reports for the central nervous system was by Frank and Fuortes(1955)for catX1X3X2X4X1X2TIMEFigure1:On the left are shown the solutions of(1)-(4)for two coupled QIF model neurons with the standard parameters.X1and X2are the potential variables of neurons1and2and X3and X4are the inputs to neurons1and2, respectively.On the right is shown the periodic orbit in the(x1,x2)-plane.The square marked P was explored in detail in reference to the extent of the basin of attraction of the periodic orbit.spinal neurons.Although there have been many single neuron studies,the effect of noise on systems of coupled neurons have not been extensively investigated. Some preliminary studies are those of Gutkin,Hely and Jost(2004)and Casado and Baltan´a s(2003).2The quadratic integrate andfire modelA relatively simple neural model which exhibits Type1firing behaviour is the quadratic integrate andfire(QIF)model.We couple two model neurons in the following manner(Gutkin,Hely and Jost,2004).Let{X1(t),X2(t),t≥0}be the depolarizations of neurons1and2,where t is the time index.Then the model equations are,for subthreshold states of two identical neurons,dX1=[(X1−x R)2+β+g s X3]dt+σdW1(1)dX2=[(X2−x R)2+β+g s X4]dt+σdW2(2)dX3=−X3τ+F(X1)(4)2where X3is the synaptic input to neuron1from neuron2and X4is the synaptic input to neuron2from neuron1.The quantity x R is a resting value.g s is the coupling strength.βis the mean background input.W1and W2are independent standard Wiener processes which enter with strengthσ.This term may model variations in nonspecific inputs to the circuit as well as possibly intrinsic membrane and channel noise.By construction,we take this term to be much weaker than the mutual coupling between the cells in our circuit.The function F is given byF(x)=1+tanh(α(x−θ))whereθcharacterizes the threshold effect of synaptic activation.Since when a QIF neuron is excited and it receives no inhibition,its potential reaches an infinite value in afinite time,for numerical simulations a cutoffvalue x max is introduced so that the above model equations for the potential apply only if X1 or X2are below x max.To complete a“spike”in any neuron,taken as occurring when its potential reaches x max,its potential is instantaneously reset to some value x reset which may be taken as−x max.At the bifurcation point g s=g∗s, two heteroclinic orbits between unstable rest points turn into a periodic orbit of antiphase oscillations.3Results and theoryIn the numerical work,the following constants are employed throughout.x R= 0,x max=20,θ=10,α=1,β=−1,g s=100andτ=0.25.The initial values of the neural potentials are X1(0)=1.1,X2(0)=0and the initial values of the synaptic variables are X3(0)=X4(0)=0.When there is no noise,σ=0,the results of Figure1are obtained.The spike trains of the two coupled neurons and their synaptic inputs are shown on the left.Thefiring settles down to be quite regular and the periodic orbit,S,is shown on the right.The patch marked P is the location of the region explored in detail below.The effects of a small amount of noise are shown in Figure2.The neural excitation variables are shown on the left and the corresponding trajectories in the(x1,x2)-plane are shown on the right.In the top portion an example of the trajectory forσ=0.1is shown.Here three spikes arise in neuron1and two in neuron2,but the time between spikes increases and eventually the orbit collapses away from the periodic orbit.In the example(lower part)forσ=0.2 there are no spikes in either neuron.In10trials,the average numbers of spikes obtained for the pair of neurons were(2.5,2.2)forσ=0.1,(1.4,1.1)forσ=0.2 and(1.3,0.9)forσ=0.3;these may be compared with(5,5)for zero noise. 3.1Exit-time and orbit stabilityIf a basin of attraction for a periodic orbit can be found,then the probabil-ity that the process with noise escapes from the region of attraction gives the probability,in the present context,that spiking will cease.Since the system3TIMEX1X21 X2Figure2:On the left are shown examples of the neuronal potentials for neurons 1and2(QIF model)for two values of the noise,σ=0.1andσ=0.2.On the right are shown the trajectories corresponding to the results on the left,showing how noise pushes or keeps the trajectories out of the basin of attraction of the periodic orbit.(1)-(4)is Markovian,we may apply standardfirst-exit time theory(Tuckwell, 1989).Letting A be a set in R4and letting x=(x1,x2,x3,x4)∈A be a values of X1,X2,X3,X4)at some given time,the probability p(x1,x2,x3,x4)that the process ever escapes from A is given byL p≡σ2∂x21+σ2∂x22(5)+[(x1−x R)2+β+g s x3]∂p∂x2+ F(x2)−x3∂x3+ F(x1)−x4∂x4=0,x∈Awith boundary condition that p=1on the boundary of A(since the process is continuous).If one also adds an arbitrarily small amount of noise for X3and X4(or considers those solutions of(5)that arise from the limit of vanishing noise for X3,X4),the solution of the linear elliptic partial differential equation (5)is unique and≡1,that is,the process will eventually excape from A with probability1.Hence,the expected time f(x)of exit of the process from A satisfies L f=−1,x∈A with boundary condition f=0on the boundary of A.In fact,for small noise,the logarithm of the expected exit time from A,that4is,the time at whichfiring stops,behaves like the inverse of the square of the noise amplitude(Freidlin and Wentzell,1998).These linear partial differential equations can be solved numerically,for example by Monte-Carlo techniques.The basin of attraction A must be found in order to identify the domain of(5).We have done this approximately for the square P in Figure1.The effects of perturbations of the periodic orbit S within P on the spiking activity were found by solving(1)-(4)with various initial conditions in the absence of noise.The values of x1were from−0.43to1.57in steps of0.2and the values of x2were from-4to2also in steps of0.2.For this particular region, as expected from geometrical considerations,the system responded sensitively to to variations in x1but not x2.For example,to the left of S there tended to be no spiking activity whereas just to the right there was a full complement of spikes and further to the right(but still inside P)one spike.4Coupled Hodgkin-Huxley neuronsAs an example of a Type2neuron,we use the standard Hodgkin-Huxley(HH) model augmented with synaptic input variables as in the model for coupled QIF neurons given by equations(3)and(4),but with different parameter values. It has been long known that additive noise has a facilitative effect on single HH neurons(Yu and Lewis,1989).Coupled pairs of HH neurons have been employed with a different approach using conductance noise in order to analyze synchronization properties(e.g.Casado and Balt´a nas,2003).For the present approach,with X1and X2as the depolarizations of the two cells,we putdX1=1g K n4(V K−X1)+it was found that transient synchronization can terminate sustained activity. For Type2neurons,we have investigated coupled Hodgkin-Huxley neurons and found that in the bistable regime,noise can again terminate sustained spiking activity initiated by synaptic connections.We have investigated a minimal cir-cuit model of sustained neural activity.Such sustained activity in the prefrontal cortex has been proposed as a neural correlate of working memory(Fuster and Alexander,1973).ReferencesCasado,J.M.,Balt´a nas,J.P.(2003).Phase switching in a system of two noisy Hodgkin-Huxley neurons coupled by a diffusive interaction.Phys.Rev.E68,061917,Frank,K.,Fuortes,M.G.(1955).Potentials recorded from the spinal cord with microelectrodes,J.Physiol.130,625-654.Freidlin,M.I.,Wentzell,A.D.(1998),Random Perturbations of Dynamical Sys-tems,2nd ed.,Springer,New York Fuster,J.M.and Alexander,G.E.(1971),Neuron activity related to short-term memory.Science652-654 Gutkin,B.,Ermentrout,G.B.(1998).Dynamics of membrane excitability de-termine interval variability:a link between spike generation mechanismsand cortical spike train statistics.Neural Comp.10,1047-1065. Gutkin,B.S.et al.(2001)Turning on and offwith p.Neurosc.11:2,121-134Gutkin,B.,Hely,T.,Jost,J.(2004).Noise delays onset of sustainedfiring in a minimal model of persistent activity.Neurocomputing58-60,753-760. Hodgkin,A.L.(1948).The local changes associated with repetitive action in a non-medullated axon.J.Physiol.107,165-181.Rinzel,J.,Ermentrout,G.B.(1989).Analysis of neural excitability and oscilla-tions;in:Koch C.&Segev I.,eds.MIT Press.Tateno,T.,Harsch,A.,Robinson,H.P.C.(2004).Thresholdfiring frequency-current relationships of neurons in rat somatosensory cortex:Type1and Type2dynamics.J.Neurophysiol.92,2283-2294.Tuckwell,H.C.(1989).Stochastic Processes in the Neurosciences.SIAM,Philadel-phia.Yu,X.,Lewis,E.R.(1989).Studies with spike initiators:linearization by noise allows continuous signal modulation in neural networks.IEEE Trans.Biomed.Eng.36,36-43.6。
Arrhenius equation
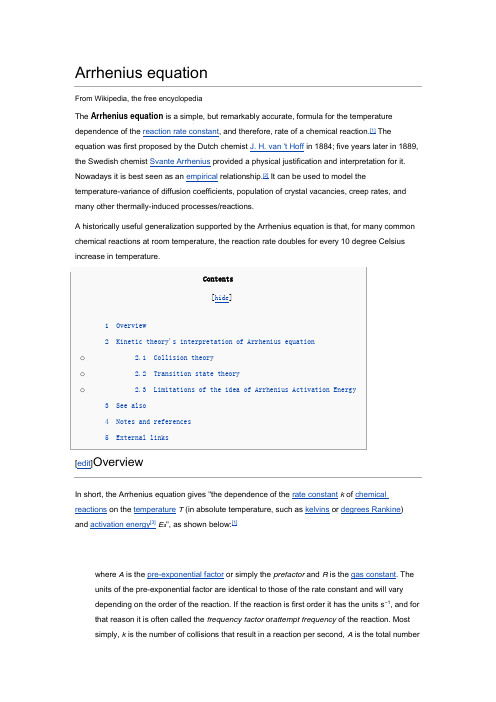
Arrhenius equationFrom Wikipedia, the free encyclopediaThe Arrhenius equation is a simple, but remarkably accurate, formula for the temperaturedependence of the reaction rate constant, and therefore, rate of a chemical reaction.[1] Theequation was first proposed by the Dutch chemist J. H. van 't Hoff in 1884; five years later in 1889, the Swedish chemist Svante Arrhenius provided a physical justification and interpretation for it.Nowadays it is best seen as an empirical relationship.[2] It can be used to model thetemperature-variance of diffusion coefficients, population of crystal vacancies, creep rates, and many other thermally-induced processes/reactions.A historically useful generalization supported by the Arrhenius equation is that, for many commonchemical reactions at room temperature, the reaction rate doubles for every 10 degree Celsius increase in temperature.∙∙∙∙∙[edit]OverviewIn short, the Arrhenius equation gives "the dependence of the rate constant k of chemicalreactions on the temperature T (in absolute temperature, such as kelvins or degrees Rankine) and activation energy[3]E a", as shown below:[1]where A is the pre-exponential factor or simply the prefactor and R is the gas constant. Theunits of the pre-exponential factor are identical to those of the rate constant and will varydepending on the order of the reaction. If the reaction is first order it has the units s−1, and for that reason it is often called the frequency factor or attempt frequency of the reaction. Mostsimply, k is the number of collisions that result in a reaction per second, A is the total numberof collisions (leading to a reaction or not) per second and is the probability that any given collision will result in a reaction. When the activation energy is given in molecular units instead of molar units, e.g., joules per molecule instead of joules per mole, the Boltzmann constant is used instead of the gas constant. It can be seen that either increasing the temperature or decreasing the activation energy (for example through the use of catalysts) will result in an increase in rate of reaction.Given the small temperature range kinetic studies occur in, it is reasonable to approximate the activation energy as being independent of the temperature. Similarly, under a wide range of practical conditions, the weak temperature dependence of the pre-exponential factor isnegligible compared to the temperature dependence of the factor; except in the case of "barrierless" diffusion-limited reactions, in which case the pre-exponential factor is dominant and is directly observable.Some authors define a modified Arrhenius equation,[4] that makes explicit the temperature dependence of the pre-exponential factor. If one allows arbitrary temperature dependence of the prefactor, the Arrhenius description becomes overcomplete, and the inverse problem (i.e., determining the prefactor and activation energy from experimental data) becomes singular. The modified equation is usually of the formwhere T0 is a reference temperature and allows n to be a unitless power. Clearly the original Arrhenius expression above corresponds to n = 0. Fitted rate constants typically lie in the range -1<n<1. Theoretical analyses yield various predictions for n. It has been pointed out that "it is not feasible to establish, on the basis of temperature studies of the rate constant, whether the predicted T½ dependence of the pre-exponential factor isobserved experimentally."[2] However, if additional evidence is available, from theory and/or from experiment (such as density dependence), there is no obstacle to incisive tests of the Arrhenius law.Another common modification is the stretched exponential formwhere β is a unitless number of order 1. This is typically regarded as a fudgefactor to make the model fit the data, but can have theoretical meaning, forexample showing the presence of a range of activation energies or in specialcases like the Mott variable range hopping.Taking the natural logarithm of the Arrhenius equation yields:So, when a reaction has a rate constant that obeys the Arrhenius equation, a plot of ln(k) versus T−1 gives a straight line, whose slope and intercept can be used to determine E a and A. This procedure has become so common in experimental chemical kinetics that practitioners have taken to using itto define the activation energy for a reaction. That is the activation energy is defined to be (-R) times the slope of a plot of ln(k) vs. (1/T ):[edit]Kinetic theory's interpretation of Arrhenius equationArrhenius argued that for reactants to transform into products, theymust first acquire a minimum amount of energy, called the activationenergy E a. At an absolute temperature T, the fraction of molecules that have a kinetic energy greater than E a can be calculated fromthe Maxwell-Boltzmann distribution of statistical mechanics, and turns out to be proportional to . The concept of activationenergy explains the exponential nature of the relationship, and in one way or another, it is present in all kinetic theories.[edit]Collision theoryMain article: Collision theoryOne example comes from the "collision theory" of chemical reactions, developed by Max Trautz and William Lewis in the years 1916-18. In this theory, molecules are supposed to react if they collide with arelative kinetic energy along their lines-of-center that exceeds E a. This leads to an expression very similar to the Arrhenius equation.[edit]Transition state theoryAnother Arrhenius-like expression appears in the "transition statetheory" of chemical reactions, formulatedby Wigner, Eyring, Polanyi and Evans in the 1930s. This takes various forms, but one of the most common iswhere ΔG‡ is the Gibbs free energy ofactivation, k B is Boltzmann's constant, and h is Planck's constant.At first sight this looks like an exponential multiplied by a factor that is linear in temperature. However, one must remember that free energy is itself a temperature dependent quantity. The free energy of activation is the difference of an enthalpy term and an entropy term multiplied by the absolute temperature. When all of the details are worked out one ends up with an expression that again takes the form of an Arrhenius exponential multiplied by a slowly varying function of T. The precise form of the temperature dependence depends upon the reaction, and can be calculated using formulas from statistical mechanics involving the partition functions of the reactants and of the activated complex.[edit]Limitations of the idea of Arrhenius Activation EnergyBoth the Arrhenius activation energy and the rate constant k are experimentally determined, and represent macroscopic reaction-specific parameters that are not simply related to threshold energies and the success of individual collisions at the molecular level. Consider a particular collision (an elementary reaction) between molecules A and B. The collision angle, the relative translational energy, the internal (particularly vibrational) energy will all determine the chance that the collision will produce a product molecule AB. Macroscopic measurements of E and k are the result of many individual collisions with differing collision parameters. To probe reaction rates at molecular level, experiments have to be conducted under near-collisional conditions and this subject is often called molecular reaction dynamics (see Levine).'[edit]See also▪Accelerated aging▪Arrhenius plot▪Eyring equation▪Q10 (temperature coefficient)[edit]Notes and references1. ^ a b Arrhenius equation - IUPAC Goldbook definition2. ^ a b Kenneth Connors, Chemical Kinetics, 1990, VCHPublishers3. ^Arrhenius activation energy - IUPAC Goldbook definition4. ^IUPAC Goldbook definition of modified Arrheniusequation▪Laidler, K. J. (1997) Chemical Kinetics,Third Edition, Benjamin-Cummings▪Laidler, K. J. (1993) The World of Physical Chemistry, Oxford University Press▪Levine, R.D. (2005) Molecular Reaction Dynamics, Cambridge University Press[edit]External links▪Carbon Dioxide solubility in Polyethylene - Using Arrhenius equation for calculating species solubility in polymersArrhenius plotFrom Wikipedia, the free encyclopediaAn Arrhenius plot displays the logarithm of kinetic constants (ln(k), ordinate axis) plotted against inverse temperature (1 / T, abscissa). Arrhenius plots are often used to analyze the effect of temperature on the rates of chemical reactions. For a single rate-limited thermally activated process, an Arrhenius plot gives a straight line, from which the activation energy and the pre-exponential factor can both be determined.Conventional plot:k against TArrhenius plot:ln(k) against 1/TThe Arrhenius equation given in the form: can be written equivalently as:Where:k = Rate constantA = Pre-exponential factorE a = Activation energyR = Gas constantT = Absolute temperature, KWhen plotted in the manner described above, the value of the "y-intercept" will correspond to ln(A), and the gradient of the line will be equal to −E a / R.The pre-exponential factor, A, is a constant of proportionality that takes into account a number of factors such as the frequency of collision between and the orientation of the reacting particles.The expression −E a / RT represents the fraction of the molecules present in a gas which have energies equal to or in excess of activation energy at a particular temperature.[edit]See also▪Arrhenius equation。
两个关于Smarandache函数的方程
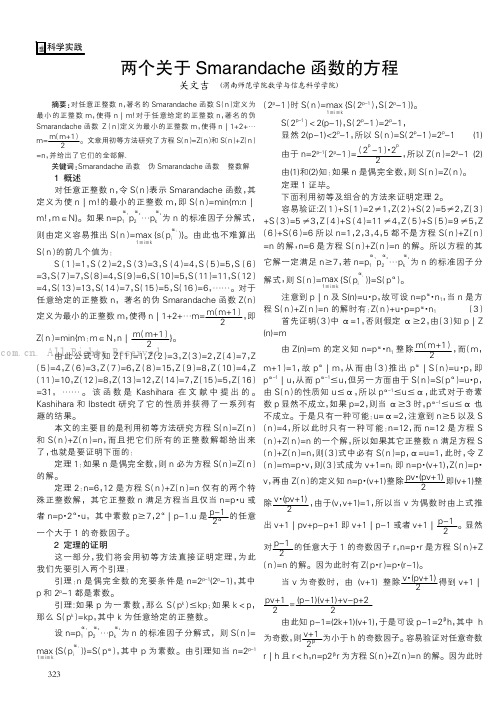
摘要:对任意正整数n,著名的Smarandache 函数S (n )定义为最小的正整数m,使得n|m!对于任意给定的正整数n,著名的伪Smarandache 函数Z(n )定义为最小的正整数m,使得n|1+2+…m=m (m+1)2。
文章用初等方法研究了方程S (n )=Z (n )和S (n )+Z (n )=n,并给出了它们的全部解.关键词:Smarandache 函数伪Smarandache 函数整数解1概述对任意正整数n,令S (n )表示Smarandache 函数,其定义为使n|m!的最小的正整数m,即S (n )=min{m:n|m!,m∈N}。
如果n=p 1α1p 2α2…p k αk为n 的标准因子分解式,则由定义容易推出S (n )=max 1⩽i ⩽k{s(p i αi)}。
由此也不难算出S (n )的前几个值为:S (1)=1,S (2)=2,S (3)=3,S (4)=4,S (5)=5,S (6)=3,S (7)=7,S (8)=4,S (9)=6,S (10)=5,S (11)=11,S (12)=4,S (13)=13,S (14)=7,S (15)=5,S (16)=6,……。
对于任意给定的正整数n,著名的伪Smarandache 函数Z (n )定义为最小的正整数m,使得n|1+2+…m=m (m+1)2,即Z (n )=min{m:m∈N,n|m(m+1)2}。
由此公式可知Z (1)=1,Z (2)=3,Z (3)=2,Z (4)=7,Z(5)=4,Z (6)=3,Z (7)=6,Z (8)=15,Z (9)=8,Z (10)=4,Z (11)=10,Z (12)=8,Z (13)=12,Z (14)=7,Z (15)=5,Z (16)=31,……。
该函数是Kashihara 在文献中提出的。
Kashihara 和Ibstedt 研究了它的性质并获得了一系列有趣的结果。
薛定谔—麦克斯韦尔方程径向解的存在性和多重性(英文)
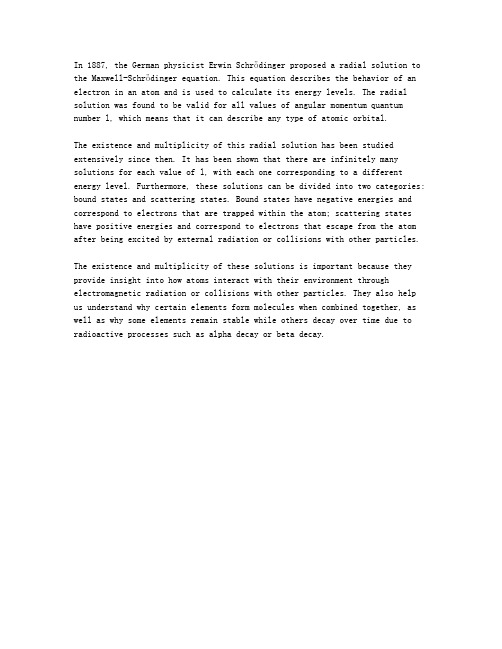
In 1887, the German physicist Erwin Schrödinger proposed a radial solution to the Maxwell-Schrödinger equation. This equation describes the behavior of an electron in an atom and is used to calculate its energy levels. The radial solution was found to be valid for all values of angular momentum quantum number l, which means that it can describe any type of atomic orbital.The existence and multiplicity of this radial solution has been studied extensively since then. It has been shown that there are infinitely many solutions for each value of l, with each one corresponding to a different energy level. Furthermore, these solutions can be divided into two categories: bound states and scattering states. Bound states have negative energies and correspond to electrons that are trapped within the atom; scattering states have positive energies and correspond to electrons that escape from the atom after being excited by external radiation or collisions with other particles.The existence and multiplicity of these solutions is important because they provide insight into how atoms interact with their environment through electromagnetic radiation or collisions with other particles. They also help us understand why certain elements form molecules when combined together, as well as why some elements remain stable while others decay over time due to radioactive processes such as alpha decay or beta decay.。
frobenius method
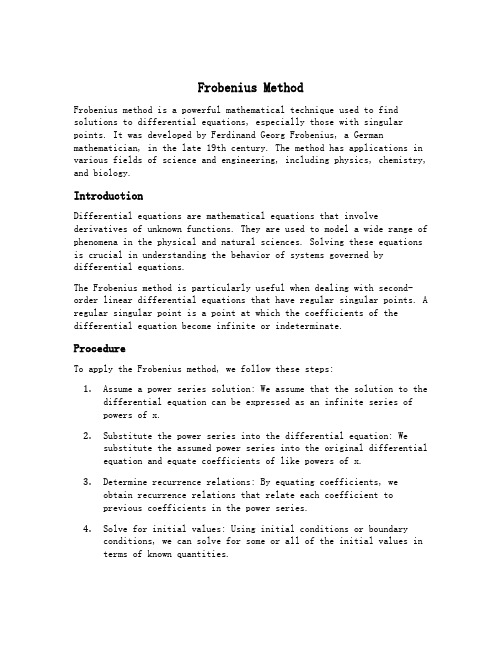
Frobenius MethodFrobenius method is a powerful mathematical technique used to find solutions to differential equations, especially those with singular points. It was developed by Ferdinand Georg Frobenius, a German mathematician, in the late 19th century. The method has applications in various fields of science and engineering, including physics, chemistry, and biology.IntroductionDifferential equations are mathematical equations that involve derivatives of unknown functions. They are used to model a wide range of phenomena in the physical and natural sciences. Solving these equations is crucial in understanding the behavior of systems governed by differential equations.The Frobenius method is particularly useful when dealing with second-order linear differential equations that have regular singular points. A regular singular point is a point at which the coefficients of the differential equation become infinite or indeterminate.ProcedureTo apply the Frobenius method, we follow these steps:1.Assume a power series solution: We assume that the solution to thedifferential equation can be expressed as an infinite series ofpowers of x.2.Substitute the power series into the differential equation: Wesubstitute the assumed power series into the original differential equation and equate coefficients of like powers of x.3.Determine recurrence relations: By equating coefficients, weobtain recurrence relations that relate each coefficient toprevious coefficients in the power series.4.Solve for initial values: Using initial conditions or boundaryconditions, we can solve for some or all of the initial values in terms of known quantities.5.Determine convergence: We analyze the radius and interval ofconvergence for the power series solution to ensure its validitywithin a certain domain.6.Construct the general solution: Once we have determined allnecessary coefficients, we can construct the general solution as a linear combination of terms involving these coefficients.ExampleLet’s consider an example to illustrate how to apply the Frob enius method:We want to solve Bessel’s equation:x^2 y’’ + x y’ + (x^2 - n^2) y = 0where n is a constant.1.Assume a power series solution:We assume that the solution can be expressed as a power series:y(x) = Σ(a_n * x^(n+r))where r is an arbitrary constant.2.Substitute the power series into the differential equation: Substituting the power series into Bessel’s equation, we obtain:Σ[(n+r)(n+r-1) * a_n * x^(n+r-2) + (n+r) * a_n * x^(n+r-1) + (x^2 - n^2) * a_n * x^(n+r)] = 03.Determine recurrence relations:By equating coefficients of like powers of x, we obtain the following recurrence relation:a_(n+2) = -((n+r)^2 - n^2)/(n+1)(n+2) * a_n4.Solve for initial values:To solve for initial values, we need to consider the behavior of the coefficients as n approaches negative infinity. We can chooseappropriate values for r to ensure convergence and determine all necessary initial values.5.Determine convergence:We analyze the recurrence relation to determine the radius and interval of convergence for the power series solution.6.Construct the general solution:Once we have determined all necessary coefficients, we can construct the general solution by combining terms involving these coefficients.ApplicationsThe Frobenius method has various applications in science and engineering. Some examples include:1.Quantum mechanics: The Frobenius method is used to solveSchrödinger’s equation in atomic physics and quantum mechanics.2.Heat conduction: The Frobenius method can be applied to solvepartial differential equations governing heat conduction invarious materials.3.Fluid dynamics: The Frobenius method is used to solve differentialequations describing fluid flow and turbulence in fluid dynamics.4.Electromagnetism: The Frobenius method is used to solve Maxwell’sequations, which describe the behavior of electric and magneticfields.ConclusionThe Frobenius method provides a systematic approach for findingsolutions to differential equations with regular singular points. By assuming a power series solution and equating coefficients, we can determine the necessary recurrence relations and initial values to construct the general solution. This method has wide-rangingapplications in various scientific and engineering disciplines, makingit an essential tool for researchers and practitioners alike.。
1 Introduction to the Galois Theory of Linear Differential Equations
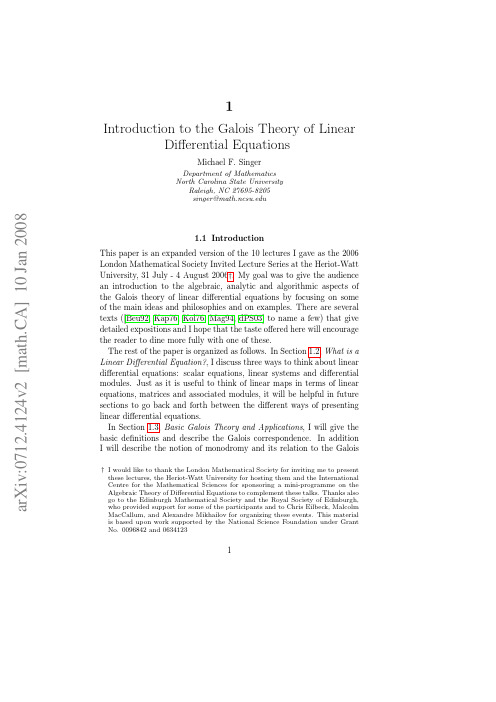
1.2 What is a Linear Differential Equation? I will develop an algebraic setting for the study of linear differential equations. Although there are many interesting questions concerning differential equations in characteristic p [MvdP03a, MvdP03b, dP95, dP96], we will restrict ourselves throughout this paper, without further mention, to fields of characteristic 0. I begin with some basic definitions.
麦克斯韦方程组 英文

麦克斯韦方程组英文English: The Maxwell's equations are a set of fundamental equations in classical electromagnetism that describe how electric and magnetic fields interact with each other and with electric charges and currents. They were formulated by the physicist James Clerk Maxwell in the 19th century and played a crucial role in the development of electromagnetic theory. The equations consist of four equations: Gauss's law for electric fields, Gauss's law for magnetic fields, Faraday's law of electromagnetic induction, and Ampère's law with Maxwell's addition. These equations are mainly concerned with the spatial and temporal changes of electric and magnetic fields, and they are usually written in differential form or integral form. In the differential form, the equations express how the fields change at each point in space, while in the integral form, they describe the flux of the fields through closed surfaces or the circulation of the fields along closed paths. The Maxwell's equations have important implications in many areas of physics and engineering, as they govern the behavior of electromagnetic waves, the propagation of signals through transmission lines, the behavior of antennas, the operation of electric motors and generators, andvarious other electromagnetic phenomena. In addition, the Maxwell's equations also played a crucial role in the development of the theory of relativity, as they led to the realization that electric and magnetic fields are two different manifestations of a single electromagnetic field, and they can transform into each other under certain conditions. Overall, the Maxwell's equations are of fundamental importance in understanding the behavior of electric and magnetic fields and their interactions with matter, and they have paved the way for numerous technological advancements and scientific discoveries.中文翻译: 麦克斯韦方程组是经典电磁学中描述电场、磁场与电荷电流相互作用的一组基本方程。
Numerical solution of SDE

f (t) dWt = lim
c
∆t→0
f (ti−1 )∆Wi
i=1
where ∆Wi = Wti − Wti−1 , a step of Brownian motion across the interval. Note a major difference: while the ti in the Riemann integral may be chosen at any point in the interval (ti−1 , ti ), the corresponding point for the Ito integral is required to be the left endpoint of that interval. Because f and Wt are random variables, so is the Ito integral I = d f (t) dWt . The differential dI is a notational convenience; thus c
t t
X (t) = X (0) +
0
a(s, y ) ds +
0
b(s, y ) dWs ,
where the meaning of the last integral, called an Ito integral, will be defined next. Let c = t0 < t1 < . . . < tn−1 < tn = d be a grid of points on the interval [c, d]. The Riemann integral is defined as a limit
Bernoulli‘s equation
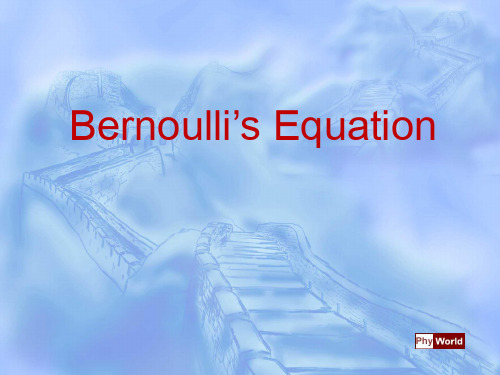
Bernoulli’s equation
Bernoulli desses this principle quantitatively:
This is Bernoulli’s equation.
Bernoulli’s equation can be written as:
Bernoulli’s Equation
Bernoulli’s principle
Where the velocity of a fluid is high, the pressure is low, and where the velocity is low, the pressure is high.
To derive Bernoulli’s equation, we assume the flow is steady and laminar, the fluid is incompressible, and the viscosity is small enough to be ignored.
Lift on an airplane wing. We are in the reference frame of the wing, seeing the air flow by.
Looking down on a pitched baseball heading toward home plate. We are in the reference frame of the baseball, with the air flowing by.
To be general, we assume the fluid is flowing in a tube of nonuniform cross section that varies in height above some reference level.
河北省2024-2025学年高三上学期9月月考英语试题

河北省2024-2025学年高三上学期9月月考英语试题一、听力选择题1.What will the man probably do next?A.Make a cake.B.Take part in a race.C.Stop at the supermarket. 2.What does the man advise the woman to do?A.Take a few risks.B.Watch out for potential dangers.C.Avoid harming the natural system.3.What does the man intend to do?A.Buy a house.B.Expand his house.C.Advertise his house. 4.What are the speakers talking about?A.Drink orders.B.Items on the menu.C.Their favorite fruit. 5.Who is Elle most likely to be?A.Elena’s sister.B.John’s daughter.C.John’s elder sister.听下面一段较长对话,回答以下小题。
6.What do we know about Rob Brown?A.He will graduate next year.B.He takes an interest in cooking.C.He’s dissatisfied with Stacy’s service.7.What problem does Stacy find out?A.Rob clicked the wrong birth date.B.Rob selected the wrong year for his class.C.Rob didn’t know how to register for the course.听下面一段较长对话,回答以下小题。
Level set methods An overview and some recent results
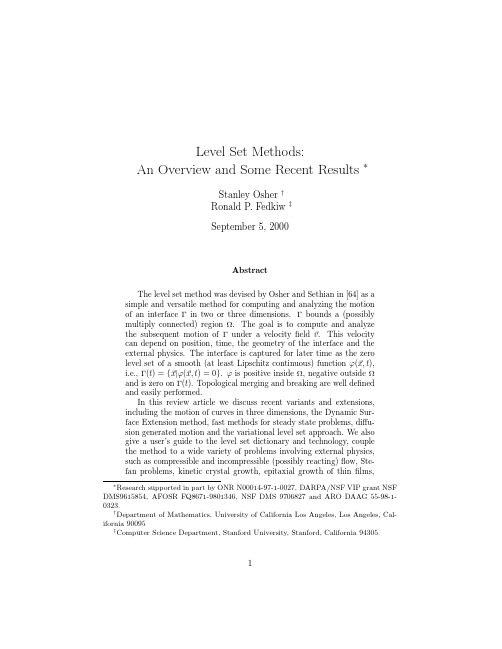
Level Set Methods:An Overview and Some Recent Results∗Stanley Osher†Ronald P.Fedkiw‡September5,2000AbstractThe level set method was devised by Osher and Sethian in[64]as a simple and versatile method for computing and analyzing the motion of an interfaceΓin two or three dimensions.Γbounds a(possibly multiply connected)regionΩ.The goal is to compute and analyze the subsequent motion ofΓunder a velocityfield v.This velocity can depend on position,time,the geometry of the interface and the external physics.The interface is captured for later time as the zero level set of a smooth(at least Lipschitz continuous)functionϕ( x,t), i.e.,Γ(t)={ x|ϕ( x,t)=0}.ϕis positive insideΩ,negative outsideΩand is zero onΓ(t).Topological merging and breaking are well defined and easily performed.In this review article we discuss recent variants and extensions, including the motion of curves in three dimensions,the Dynamic Sur-face Extension method,fast methods for steady state problems,diffu-sion generated motion and the variational level set approach.We also give a user’s guide to the level set dictionary and technology,couple the method to a wide variety of problems involving external physics, such as compressible and incompressible(possibly reacting)flow,Ste-fan problems,kinetic crystal growth,epitaxial growth of thinfilms,vortex dominatedflows and extensions to multiphase motion.We con-clude with a discussion of applications to computer vision and image processing.21IntroductionThe original idea behind the level set method was a simple one.Given an interfaceΓin R n of codimension one,bounding a(perhaps multiply connected)open regionΩ,we wish to analyze and compute its subsequent motion under a velocityfield v.This velocity can depend on position,time, the geometry of the interface(e.g.its normal or its mean curvature)and the external physics.The idea,as devised in1987by S.Osher and J.A. Sethian[64]is merely to define a smooth(at least Lipschitz continuous) functionϕ(x,t),that represents the interface as the set whereϕ(x,t)=0. Here x=x(x1,...,x n)εR n.The level set functionϕhas the following propertiesϕ(x,t)>0for x∈Ωϕ(x,t)<0for x∈¯Ωϕ(x,t)=0for x∈∂Ω=Γ(t)Thus,the interface is to be captured for all later time,by merely locat-ing the setΓ(t)for whichϕvanishes.This deceptively trivial statement is of great significance for numerical computation,primarily because topolog-ical changes such as breaking and merging are well defined and performed “without emotional involvement”.The motion is analyzed by convecting theϕvalues(levels)with the velocityfield v.This elementary equation is∂ϕ|∇ϕ|,so(1)becomes∂ϕWe emphasize that all this is easy to implement in the presence of bound-ary singularities,topological changes,and in2or3dimensions.Moreover, in the case which v N is a function of the direction of the unit normal(as in kinetic crystal growth[62],and Uniform Density Island Dynamics[15],[36]) then equation(2)becomes thefirst order Hamilton-Jacobi equation∂ϕ|∇ϕ|.High order accurate,essentially non-oscillatory discretizations to general Hamilton-Jacobi equations including(3)were obtained in[64],see also[65] and[43].Theoretical justification of this method for geometric based motion came through the theory of viscosity solutions for scalar time dependent partial differential equations[23],[30].The notion of viscosity solution(see e.g.[8, 27])–which applies to a very wide class of these equations,including those derived from geometric based motions–enables users to have confidence that their computer simulations give accurate,unique solutions.A particularly interesting result is in[29]where motion by mean curvature,as defined by Osher and Sethian in[64],is shown to be essentially the same motion as is obtained from the asymptotics in the phasefield reaction diffusion equation. The motion in the level set method involves no superfluous stiffness as is required in phasefield models.As was proven in[53],this stiffness due to a singular perturbation involving a small parameter will lead to incorrect answers as in[48],without the use of adaptive grids[59].This is not an issue in the level set approach.The outline of this paper is as follows:In section2we present recent vari-ants,extensions and a rather interesting selection of related fast numerical methods.This section might be skipped atfirst,especially by newcomers to this subject.Section3contains the key definitions and basic level set tech-nology,as well as a few words about the numerical implementation.Section 4describes applications in which the moving interfaces are coupled to ex-ternal physics.Section5concerns the variational level set approach with applications to multiphase(as opposed to two phase)problems.Section6 gives a very brief introduction to the ever-increasing use of level set method and related methods in image analysis.42Recent Variants,Extensions and Related Fast Methods2.1Motion of Curves in Three Spatial DimensionsIn this section we discuss several new and related techniques and fast nu-merical methods for a class of Hamilton-Jacobi equations.These are all relatively recent developments and less experienced readers might skip this section atfirst.As mentioned above,the level set method was originally developed for curves in R2and surfaces in R3.Attempts have been made to modify it to handle objects of high codimension.Ambrosio and Soner[5]were interested in moving a curve in R3by curvature.They used the squared distance to the curve as the level set function,thusfixing the curve as the zero level set, and evolved the curve by solving a PDE for the level set function.The main problem with this approach is that one of the most significant advantages of level set method,the ability to easily handle merging and pinching,does not carry over.A phenomenon called“thickening”emerges,where the curve develops an interior.Attempts have also been made in other directions,front tracking,e.g. see[41].This is where the curve is parameterized and then numerically rep-resented by discrete points.The problem with this approach lies infinding when merging and pinching will occur and in reparameterizing the curve when it does.The representation we derived in[13]makes use of two level set functions to model a curve in R3,an approach Ambrosio and Soner also suggested but did not pursue because the theoretical aspects become very difficult.In this formulation,a curve is represented by the intersection between the zero level sets of two level set functionsφandψ,i.e.,where φ=ψ=0.From this,many properties of the curve can be derived such as the tangent vectors, T=∇ψ×∇φA simple example involves moving the curve according to its curvature vec-tors,for which v=κ N.We have shown that this system can also be obtained by applying a gradient descent algorithm minimizing the length ofthe curve,L(φ,ψ)=R3|∇ψ×∇φ|δ(ψ)δ(φ)d x.This follows the general procedure derived in[88]for the variational level set method for codimension one motion,also described in[90].Numerical simulations performed in[13]on this system of PDE’s,and shown infigures 1and2,show that merging and pinching offare handled automatically and follow curve shortening principles.We repeat the observation made above that makes this sort of motion easily accessible to this vector valued level set ly all geometric properties of a curveΓwhich is expressed as the zero level set of the vector equationφ(x,y,z,t)=0ψ(x,y,z,t)=0can easily be obtained numerically by computing discrete gradients and higher derivatives of the functionsφandψrestricted to their common zero level set.This method will be used to simulate the dynamics of defect lines as they arise in heteroepitaxy of non-lattice notched materials,see[79]and[80]for Lagrangian calculations.An interesting variant of the level set method for geometry based mo-tion was introduced in[53]as diffusion generated motion,and has now been generalized to forms known as convolution generated motion or threshold dy-namics.This method splits the reaction diffusion approach into two highly simplified steps.Remarkably,a vector valued generalization of this ap-proach,as in the vector valued level set method described above gives an alternative approach[74]to easily compute the motion(and merging)of curves moving normal to themselves in three dimensions with velocity equal to their curvature.2.2Dynamic Surface Extension(DSE)Anotherfixed grid method for capturing the motion of self-intersecting in-terfaces was obtained in[73].This is afixed grid,interface capturing formu-lation based on the Dynamic Surface Extension(DSE)method of Steinhoff6et.al.[82].The latter method was devised as an alternative to the level set method of Osher and Sethian[64]which is needed to evolve wavefronts according to geometric optics.The problem is that the wavefronts in this case are supposed to pass through each other–not merge as in the viscos-ity solution case.Ray-tracing can be used but the markers tend to diverge which leads to loss of resolution and aliasing.The original(ingenious)DSE method was not well suited to certain fundamental self intersection problems such as formation of swallowtails.In [73]we extended the basic DSE scheme to handle this fundamental problem, as well as all other complex intersections.The method is designed to track moving setsΓof points of arbitrary (perhaps changing)codimension,moreover there is no concept of“inside”or“outside”.The method is,in some sense,dual to the level set method. In the latter,the distance representation is constant tangential to a surface. In the DSE method,the closest point to a surface is constant in directions orthogonal to the surface.The version of DSE presented in[73]can be described as follows:For each point in R n,set the tracked pointed T P( x)equal to CP( x)the closest point(to x)on the initial surfaceΓ0.Set N equal to the surface normal at the tracked point T P( x).Let v(T P( x))be the velocity of the tracked point.Repeat for all steps:(1)Evolve the tracked point T P( x)according to the local dynamics T P( x)t=v(T P( x)).(2)Extend the surface representation by resetting each tracked point T P( x)equal to the true closest point CP( x)on the updated surfaceΓ,where Γis defined to be the locus of all tracked points,i.e.Γ={T P( x)| xεR n}.Replace each N( x)by the normal at the updated T P( x).This method treats self intersection by letting moving sets pass through each other.This is one of its main virtues in the ray tracing case.However, it has other virtues–namely the generality of the moving set–curves can end or change dimension.An important extension is motivated by consideringfirst arrival times. This enables us to easily compute swallowtails,for example,and other sin-gular points.We actually use a combination of distance and direction of7motion.One interesting choice arises when nodal values of T P ( x )are set equal to the “Minimizing Point”MP ( x )=min y εInterfaceβ|( x − y )· N ⊥( y )|+ x − y 2for β>0(rather than CP ( x )),since a good agreement with the minimal arrival time representation is found near the surface.Recall that the minimal arrival time at a point x is the shortest time it takes a ray emanating from the surface to reach x .Using this idea gives a very uniform approximation and naturally treats the prototype swallowtail problem.For the special case of curvature dependent motion we may use an elegant observation of DeGiorgi [28].Namely the vector mean curvature for a surface of arbitrary codimension is given by κ N=−∆∇ d 2as in[66]for the level set method would be of great practical importance. It would be particularly interesting to determine if surfaces fatten(or de-velop interiors)when mergers occur.See[9]for a detailed discussion of this phenomenon.Additionally in[73]we successfully calculated a geometric optics ex-pansion by retaining the wave front curvature.Thus this method has the possibility of being quite useful in electromagnetic calculations.We hope to investigate its three dimensional performance and include the effects of diffraction.2.3A Class of Fast Hamilton-Jacobi SolversAnother important set of numerical algorithms involves the fast solution of steady(time independent)Hamilton-Jacobi equations.We also seek meth-ods which are faster than the globally defined schemes originally used to solve equation2.The level set method of Osher and Sethian[64]for time dependent problems can be localized.This means that the problemϕt+ v·∇ϕ=0withΓ(t)={ x|ϕ( x,t)=0}as the evolving front,can be solved locally near Γ(t).Several algorithms exist for doing this,see[66]and[2].These both report an O(N)algorithm where N is the total number of grid points on or near the front.However,the algorithm in[66]has O(N log(N))complexity because a partial differential equation based reinitialization step requires log(1then we proved in[63]that the t level set{ x|ψ( x)=t}=Γ (t)is the same as the zero level setΓ(t)ofϕ( x,t),for t>0whereϕsatisfies˜Hx,−∇ϕc( x)=a( x)>0.So wefindfirst arrival times instead of zero level sets.In[86]J.N.Tsitsiklis devised a fast algorithm for the eikonal equation. He obtained the viscosity solution using ideas involving Dijkstra’s algorithm, adapted to the eikonal equation,heap sort and control theory.From a nu-merical PDE point of view,however,Tsitsiklis had an apparently nonstan-dard approximation to|∇ψ|on a uniform Cartesian grid.In(1995)Sethian[76]and Helmsen et.al.[40]independently published what appeared to be a simpler algorithm making use of the Rouy-Tourin al-gorithm to approximate|∇ϕ|.This has become known as the“fast marching method”.However,together with Helmsen[61]we have proven that Tsit-siklis’approximation is the usual Rouy-Tourin[69]version of Godunov’s monotone upwind scheme.That is,the algorithm in[76]and[40]is simply Tsitsiklis’algorithm with a different(simpler)exposition.Our goal here is to extend the applicability of this idea from the eikonal equation to any geometrically based Hamiltonian.By this we mean a Hamil-tonian satisfying the properties:H( x,∇ψ)>0,if∇ψ= 0(4) andH( x,∇ψ)is homogeneous of degree one in∇ψ(5)10We wish to obtain a fast algorithm to approximate the viscosity solution of˜H ( x ,∇ψ)=H ( x ,∇ψ)−a ( x )=0.(6)The first step is to set up a monotone upwind scheme to approximatethis problem.Such a scheme is based on the idea of Godunov used in the approximation of conservation laws.In Bardi and Osher [7],see also [65],the following was obtained (for simplicity we exemplify using two space dimensions and ignore the explicit x dependence in the Hamiltonian)H (ψx ,ψy )≈H G (D x +ψ,D x−ψj ;D y +ψ,D y −ψ)=ext uεI (u −,u +)ext v εI (v −,v +)H (u,v )whereI (a,b )=[min(a,b ),max(a,b )]ext u I (a,b )=min a ≤u ≤b if a ≤bmax b ≤u ≤a if a >bu ±=D x±ψij =±(ψi ±1,j −ψij )∆y.(Note,the order may be reversed in the ext operations above –we always obtain a monotone upwind scheme which is often,but not always,order invariant [65]).This is a monotone upwind scheme which is obtained through the Go-dunov procedure involving Riemann problems,extended to general Hamilton-Jacobi equations [7],[65].If we approximateH (∇ϕ)=a (x,y )byH G (D x +ϕ,D x −ϕ;D x+ψ;D y +ψ,D y −ψ)(7)for Hamiltonians satisfying (4),(5)above,then there exists a unique solutionfor ψi,j in terms of ψi ±1,j ,ψi,j ±1and ψi,j .Furthermore ψi,j is a nondecreas-ing function of all these variables.However,the fast algorithm needs to have property F :The solution to (7)depends on the neighboring ψµ,νonly for ψµ,ν<ψi,j .This gives us a hint as to how to proceed.11For special Hamiltonians of the form:H(u,v)=F(u2,v2),with F non-decreasing in these variables,then we have the following result[61]H G(u+,u−;v+,v−)=F(max((u−+)2,(u+−)2);max((v−+)2,(v+−)2))(8) where x+=max(x,0),x−=min(x,0).It is easy to see that this numerical Hamiltonian has property F described above.This formula,as well as the one obtained in equation10below enables us to extend the fast marching method algorithm to a much wider class than was done before.For example, using this observation we were able to solve an etching problem,also consid-ered in[3]where the authors did not use a fast marching method algorithm, but instead used a local narrow band approach and schemes devised in[64]. The Hamiltonian wasH(ϕx,ϕy,ϕz)=ϕ2x+ϕ2y+ϕ2z.We are able to use the same heap sort technology as for the eikonal equation,for problems of this type.Seefigures3and4.Thesefigures represent the level contours of an etching process whose normal velocity is a function of the direction of the normal.The process moves down infigure 3and up infigure4.More generally,for H(u,v)having the propertyuH1≥0,vH2≥0(9) then we also proved[61]H G(u+,u−;v+,v−)=max[H(u−+,v−+),H(u+−,v−+),H(u−+,v+−),H(u+−,v+−)](10) and property F is again satisfied.Again in[61],we were able to solve a somewhat interesting and very anisotropic etching problem with this new fast algorithm.Here we tookH(ϕx,ϕy)=|ϕy|(1−a(ϕy)ϕy/(ϕ2x+ϕ2y))wherea=0ifϕy<0a=.8ifϕy>012and observed merging of two fronts.Seefigures5and6.Thesefigures show a two dimensional etching process resulting in a merger.The fast method originating in[86]is a variant of Dijkstra’s algorithm and,as such involves the tree like heap sort algorithm in order to compute the smallest of a set of numbers.Recently Bou´e and Dupuis[11]have pro-posed an extremely simple fast algorithm for a class of convex Hamiltonians including those which satisfy(4)and(5)above.Basically,their statement is that the standard Gauss-Seidel algorithm,with a simple ordering,con-verges in afinite number of iterations for equation(7).This would give an O(N),not O(N log N)operations,with an extremely simple to program algorithm–no heap sort is needed.Moreover,for the eikonal equation with a(x,y)=1,the algorithm would seem to converge in2d N iterations in R d,d=1,2,3,which is quite fast.This gives a very simple and fast re-distancing algorithm.For more complicated problems we have found more iterations to be necessary,but still obtained promising results,together with some theoretical justification.See[85]for details,which also include results for a number of nonconvex Hamiltonians.We call this technique the“fast sweeping method”in[85].We refer to it in section3when we discuss the basic distance reinitialization algorithm.131415Figure3:Three dimensional etching using a fast algorithm.Reprinted from [61].16Figure4:Three dimensional etching using a fast algorithm.Reprinted from [61].17Figure5:Two dimensional etching with merging using a fast algorithm. Reprinted from[61].18Figure6:Two dimensional etching with merging using a fast algorithm. Reprinted from[61].193Level Set Dictionary,Technology and Numerical ImplementationWe list key terms and define them by their level set representation.1.The interface boundaryΓ(t)is defined by:{ x|ϕ( x,t)=0}.The regionΩ(t)is bounded byΓ(t):{ x|ϕ( x,t)>0}and its exterior is defined by:{ x|ϕ( x,t)<0}2.The unit normal N toΓ(t)is given byN=−∇ϕ|∇ϕ| .4.The Dirac delta function concentrated on an interface is:δ(ϕ)|∇ϕ|,whereδ(x)is a one dimensional delta function.5.The characteristic functionχof a regionΩ(t):χ=H(ϕ)whereH(x)≡1if x>0H(x)≡0if x<0.is a one dimensional Heaviside function.6.The surface(or line)integral of a quantity p( x,t)overΓ:R np( x,t)δ(ϕ)|∇ϕ|d x.7.The volume(or area)integral of p( x,t)overΩR np( x,t)H(ϕ)d x.20Next we describe three key technological advances which are important in many,if not most,level set calculations.8.The distance reinitialization procedure replaces a general level set func-tionϕ( x,t)by d( x,t)which is the value of the distance from x to Γ(t),positive outside and negative inside.This assures us thatϕdoes not become tooflat or too steep nearΓ(t).Let d( x,t),be signed dis-tance of x to the closest point onΓ.The quantity d( x,t)satisfies |∇d|=1,d>0inΩ,d<0in(¯Ω)c and is the steady state solution (asτ→∞)to∂ψ∂τ+sgn(ϕ)∇ϕThis wasfirst suggested and implemented in[24],analyzed carefully in[88],and further discussed and implemented in both[32]and[66].Acomputationally efficient algorithm based on heap sort technology and fast marching methods was devised in[1].There are many reasons to extend a quantity offofΓ,one of which is to obtain a well conditioned normal velocity for level contours ofϕclose toϕ=0[24].Others involve implementation of the Ghost Fluid Method of[32]discussed in the next section.10.The basic level set method concerns a functionϕ( x,t)which is definedthroughout space.Clearly this is wasteful if one only cares about information near the zero level set.The local level set method defines ϕonly near the zero level set.We may solve(2)in a neighborhood of Γof width m∆x,where m is typically5or6.Points outside of this neighborhood need not be updated by this motion.This algorithm works in“ϕ”space–so not too much intricate computer science is used.For details see[66].Thus this local method works easily in the presence of topological changes and for multiphaseflow.An earlier local level set approach called“narrow banding”was devised in[2].Finally,we repeat that,in the important special case where v N in equa-tion2is a function only of x,t and∇ϕ(e.g.v N=1),then equation 2becomes a Hamilton-Jacobi equation whose solutions generally develop kinks(jumps in derivatives).We seek the unique viscosity solution.Many good references exist for this important subject,see e.g.[8,27].The appear-ance of these singularities in the solution means that special,but not terribly complicated,numerical methods have to be used,usually on uniform Carte-sian grids.This wasfirst discussed in[64]and numerical schemes developed there were generalized in[65]and[43].The key ideas involve monotonicity, upwind differencing,essentially nonoscillatory(ENO)schemes and weighted essentially nonoscillatory(WENO)schemes.See[64],[65]amd[43]for more details.224Coupling of the Level Set Method with External PhysicsInterface problems involving external physics arise in various areas of science. The computation of such problems has a very long history.Methods of choice include front tracking,see e.g.[87]and[41],phase-field methods,see e.g.[48]and[59],and the volume offluid(VOF)approach,see e.g.[60]and [12].The level set method has had major successes in this area.Much of the level set technology discussed in the previous two sections was developed with such applications in mind.Here,we shall describe level set approaches to problems in compressible flow,incompressibleflow,flows having singular vorticity,Stefan problems, kinetic crystal growth and a relatively new island dynamics model for epitax-ial growth of thinfilms.We shall also discuss a recently developed technique, the ghostfluid method(GFM),which can be used(1)to remove numerical smearing and nonphysical oscillations inflow variables near the interface and (2)to simplify the numerical linear algebra arising in some of the problems in this section and elsewhere.4.1Compressible FlowChronologically,thefirst attempt to use the level set method in this area came in two phase inviscid compressibleflow,[55].There,to the equations of conservation of mass,momentum and energy,we appended equation(1), which we rewrote in conservation form as(ρϕ)t+∇·(ρϕ v)=0(12) using the density of thefluidρ.The sign ofϕis used to identify which gas occupied which region,so it determines the local equation of state.This(naive)method suffered from spurious pressure oscillations at the interface,as shown in[46]and[45]. These papers proposed a new method which reduced these errors by using a nonconservative formulation near the interface.However,[46]and[45]still smear out the density across the interface,leading to terminal oscillations for many equations of state.A major breakthrough in this area came in the development of the ghost fluid method(GFM)in[32].This enables us to couple the level set repre-sentation of discontinuities tofinite difference calculations of compressible23flows.The approach was based on using the jump relations for discontinu-ities which are tracked using equation(1)(for two phase compressibleflow). What the method amounts to(in any number of space dimensions)is to pop-ulate cells next to the interface with“ghost values”,which,for two phase compressibleflow retain their usual values of pressure and normal veloc-ity(quantities which are continuous across the interface),with extrapolated values of entropy and tangential velocity(which jump across the interface). These quantities are used in the numericalflux when“crossing”an interface.An important aspect of the method is its simplicity.There is no need to solve a Riemann problem normal to the interface,consider the Rankine-Hugoniot jump conditions,or solve an initial-boundary value problem.An-other important aspect is its generality.The philosophy appears to be:at a phase boundary,use afinite difference scheme which takes only values which are continuous across the interface,using the natural values when-ever possible.Of course,this implies that the tangential velocity is treated in the same fashion as the normal velocity and the pressure when viscosity is present.The same holds true for the temperature in the presence of thermal conductivity.Figure7shows results obtained for two phase compressibleflow using the GFM together with the level set method.Air with density around 1kgm3 is to the right of the interface.Note that there is no numerical smearing of the density at the interface itself which is fortunate as water cavitates at a density above999kgG-equation forflame discontinuities which was originally proposed in[50]. The G-equation represents theflame front as a discontinuity in the same fashion as the level set method so that one can easily consult the abundant literature on the G-equation to obtain deflagration speeds for the Ghost Fluid Method.Figure10shows two initially circular deflagration fronts that have just recently merged together.Note that the light colored region surrounding the deflagration fronts is a precursor shock wave that causes the initially circular deflagration waves to deform as they attempt to merge.The GFM was extended in[34]in order to treat the two phase compress-ible viscous Navier Stokes equations in a manner that allows for a large jump in viscosity across the interface.This paper spawned the technology needed to extend the GFM to multiphase incompressibleflow including the effects of viscosity,surface tension and gravity as discussed in the next subsection.4.2Incompressible FlowThe earliest real success in the coupling of the level set method to prob-lems involving external physics came in computing two-phase Navier-Stokes incompressibleflow[84],[22].The equations can be written as:u t+ u·∇ u+∇pρ+δ(ϕ)σκ Nρterm is used to approximate the surface tension forces which are lost when using a continuous pressure[84].Successful computations using this model were performed in[84]and elsewhere[22].Problems involving area loss were observed and significant improvements were made in[83].As mentioned above,the technology from[34]motivated the extension of the Ghost Fluid Method to this two phase incompressibleflow problem.25First,a new boundary condition capturing approach was devised and applied to the variable coefficient Poisson equation to solve problems of the form∇1ρ∇p· N]=h are given andρisdiscontinuous across the interface.This was accomplished in[49].A sample calculation from[49]is shown infigure11where one can see that both the solution,p,and itsfirst derivatives are sharp across the interface without numerical smearing.Next,this new technique was applied to multiphase incompressibleflow in[44].Here,since one can model the jumps in pressure directly,there is no need to add theσκ Nm3while the water has density near 1000kg。
On the middle convolution
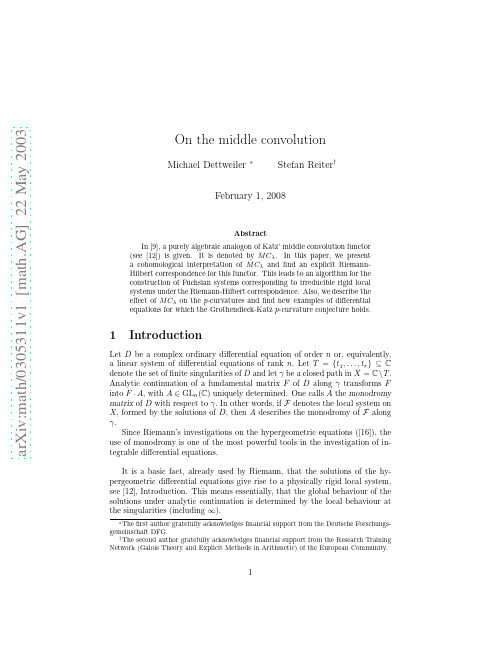
1
Introduction
Let D be a complex ordinary differential equation of order n or, equivalently, a linear system of differential equations of rank n. Let T = {t1 , . . . , tr } ⊆ C denote the set of finite singularities of D and let γ be a closed path in X = C \ T. Analytic continuation of a fundamental matrix F of D along γ transforms F into F · A, with A ∈ GLn (C) uniquely determined. One calls A the monodromy matrix of D with respect to γ. In other words, if F denotes the local system on X, formed by the solutions of D, then A describes the monodromy of F along γ. Since Riemann’s investigations on the hypergeometric equations ([16]), the use of monodromy is one of the most powerful tools in the investigation of integrable differential equations. It is a basic fact, already used by Riemann, that the solutions of the hypergeometric differential equations give rise to a physically rigid local system, see [12], Introduction. This means essentially, that the global behaviour of the solutions under analytic continuation is determined by the local behaviour at the singularities (including ∞).
The Andante Regime of Scalar Field Dynamics
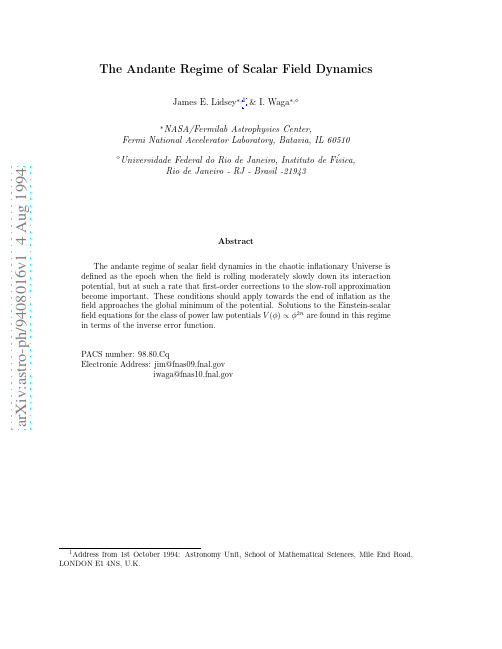
arXiv:astro-ph/9408016v1 4 Aug 1994
Federal do Rio de Janeiro, Instituto de F´ isica, Rio de Janeiro - RJ - Brasil -21943
2 where g ≡ detgµν , κ2 ≡ 8πm− P and mP is the Planck mass. We choose units such that c = h ¯ = 1. If the Universe is spatially isotropic, closed and flat with a world-interval ds2 = −N 2 (t)dt2 + e2α(t) [dx2 + dy 2 + dz 2 ] and lapse function N (t), the Arnowitt-DeserMisner (ADM) action is
1
Introduction
A study of the evolution of self-interacting scalar fields in the early Universe is important for a number of reasons. Firstly, in addition to offering a possible resolution to some of the fundamental problems of the hot big bang model, the inflationary scenario provides a causal mechanism for generating adiabatic density perturbations [1]. These may produce anisotropies in the cosmic microwave background and act as a seed for galaxy formation via gravitational instability. During inflation the Universe is dominated by the potential energy V (φ) associated with the self-interactions of a quantum scalar field φ. If the field is initially displaced from the global minimum of the potential and the potential is sufficiently flat, the scalar field will evolve very slowly towards the true vacuum state. The potential therefore behaves as an effective cosmological constant and introduces a negative pressure into the Universe that drives the accelerated expansion. Secondly, many natural extensions to General Relativity may be expressed in terms of Einstein gravity minimally coupled to a self-interacting scalar field after a suitable conformal transformation on the metric tensor. Two classes of theory that have received much attention in recent years are scalartensor theories of gravity and higher-order theories, where the gravitational lagrangian is an analytic function of the Ricci scalar R. However, only a limited number of exact solutions to the isotropic Einstein-scalar field equations have been found to date. These have recently been classified by Barrow in terms of the potential V = V0 φN exp(AφM ), where {V0 , A, M, N } are constants [2]. Special cases include V = constant corresponding to exponential expansion, power-law inflation from an exponential potential (N = 0, M = 1) [3, 5, 6, 7] and intermediate inflation from a combination of power-law potentials (N < 0, M = 0) [4, 8]. Solutions corresponding to potentials leading to hybrid inflation [9] have been presented in Refs. [10, 11] and exact solutions have also been found for hyperbolic and trigonometric potentials [12, 13]. Consequently, it is common practice to invoke the slow-roll approximation. This assumes that the kinetic energy of the scalar field is negligible relative to its potential energy and that the dominant term in the scalar field equation is due to friction arising from the expansion of the Universe. The system is therefore reduced to a set of coupled, first-order differential equations. The slow-roll approximation is usually valid during the initial stages of the inflationary expansion, but as the field rolls towards the minimum, the approximation inevitably breaks down at some point. Moreover, inflation arises whenever the strong energy condition is violated and this does not necessarily require the slow-roll approximation to be valid. Therefore, it is important to develop analytical techniques that allow deviations from the slow-roll regime to be accounted for and in this paper we discuss how this may be achieved. After summarizing the general features of scalar field dynamics in Sec. II, we illustrate how corrections to the slow-roll approximation may be included in Sec. III and we derive the corresponding field equations. In Sec. IV these equations are solved in parametric form for the class of power law potentials V (φ) ∝ φ2n in terms of the inverse error function. The rapid increase in the scale factor during inflation is naturally explained by using the properties of this function.
数论函数方程φ(x)=S(xk)的非平凡解
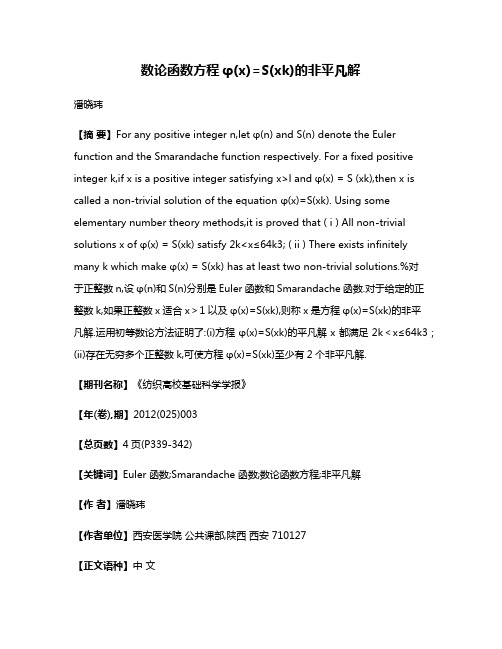
数论函数方程φ(x)=S(xk)的非平凡解潘晓玮【摘要】For any positive integer n,let φ(n) and S(n) denote the Euler function and the Smarandache function respectively. For a fixed positive integer k,if x is a positive integer satisfying x>l and φ(x) = S (xk),then x is called a non-trivial solution of the equation φ(x)=S(xk). Using some elementary number theory methods,it is proved that ( i ) All non-trivial solutions x of φ(x) = S(xk) satisfy 2k<x≤64k3; ( ii ) There exists infinitely many k which make φ(x) = S(xk) has at least t wo non-trivial solutions.%对于正整数n,设φ(n)和S(n)分别是Euler函数和Smarandache函数.对于给定的正整数k,如果正整数x适合x>1以及φ(x)=S(xk),则称x是方程φ(x)=S(xk)的非平凡解.运用初等数论方法证明了:(i)方程φ(x)=S(xk)的平凡解x都满足2k<x≤64k3;(ii)存在无穷多个正整数k,可使方程φ(x)=S(xk)至少有2个非平凡解.【期刊名称】《纺织高校基础科学学报》【年(卷),期】2012(025)003【总页数】4页(P339-342)【关键词】Euler 函数;Smarandache 函数;数论函数方程;非平凡解【作者】潘晓玮【作者单位】西安医学院公共课部,陕西西安 710127【正文语种】中文【中图分类】O156.71 引言与主要结论∀n∈N+,设φ(n)是Euler函数.这是一类经典的数论函数,有关它的各种性质一直是数论中引人关注的研究课题[1].又设S(n)是Smarandache函数,其定义为这是一类近年来讨论较多的数论函数[2-8].设k是给定的正整数.本文将讨论函数方程的求解问题.对此,从文献[9-12]中的结果可知,当1≤k≤7时,方程(2)的解已经全部找出.同时,文献[10]指出,对于任何给定的k,方程(2)仅有有限多个解x;文献[13]算出,方程(2)的解x都满足x<k 16log k.显然,对于任何的正整数k,方程(2)都有解x=1,该解称为方程(2)的平凡解;方程(2)适合x>1的解称为它的非平凡解.本文运用初等数论方法给出了方程(2)非平凡解较为精确的上下界,即证明了:定理1 方程(2)的非平凡解x都满足2k<x≤64k 3.另外,关于非平凡解的存在性,本文证明了以下结果:定理2 设p和q是不同的奇素数.如果k适合则方程(2)至少有2个非平凡解x=p q和2p q.从定理1,2可知,存在无穷多个正整数k,可使方程(2)有非平凡解.因此,本文还进一步提出了以下问题:问题1 是否仅有有限多个正整数k,可使方程(2)无非平凡解?2 引理引理1 对于任何正整数r,必有证明当1≤r≤7时,不等式(4)显然成立.当r≥8,因为所以式(4)也成立.引理1证毕.对于给定的正整数n和素数p,设or d pn表示p在n中的次数,即p ord p n‖n.引理2 对于正整数a,ord pa其中[a/p l]是a/p l 的整数部分.证明可参见文献[14]的定理1.11.1.引理3 Euler函数φ(n)是积性函数.如果n=p r 11 p r22…p rtt是n的标准分解式,则证明可参见文献[14]的定理2.5.3和2.5.4.引理4 当n≠2时,必有φ3(n)≥n.证明当n≤100时,引理4显然成立.当n>100时,由于从文献[13]的引理2可知φ(n)>(n/4)log n,所以若引理4不成立,则从φ3(n)<n可得然而,式(5)在n>100时显然不成立,故结论成立,引理4证毕.引理5 设p是素数.如果正整数n可表成则必有证明假如式(7)不成立,则从式(6)可知当m=2时,因为p≥2且φ(2)=1,故从式(8)可得然而,根据引理1可知式(9)不成立,所以此时式(7)成立.当m≠2时,由于从引理4可知φ3(m)≥m,所以从式(8)可得这一矛盾.引理5证毕.引理6 设p是素数,l是正整数.Smarandache函数S(n)有下列性质:(ⅰ)如果n=p r 11 p r22 …p rtt 是n的标准分解式,则S(n)= max{S(p r11 ),…,S(p rtt)}.(ⅱ)p|S(p l).(ⅲ)S(p l)≤pl.(ⅳ)S (p l)>(p-1)l.(ⅴ)当l 1,l 2是适合l 1<l 2的正整数时,S(p l1)≤S(p l2).(ⅵ)当p 1,p 2 是适合p 1 <p 2 的素数时,S(p l1)<S(pl2).证明根据文献[13]的引理3和4分别可知引理6的性质(ⅰ),(ⅱ)和(ⅲ)成立.设a=S(p l).根据Smarandache函数的定义式(1)可知根据引理2,从式(10)可得从式(11)可知性质(ⅳ)成立.从(1)直接可知性质(ⅴ)成立.当p 1<p 2时,根据已经证明的性质(ⅲ)和(ⅳ)分别可得以及因为p 2≥p 1+1,故从式(12)和(13)可知性质(ⅵ)成立.引理6证毕.3 定理1的证明设x是方程(2)的非平凡解,又设是x的标准分解式.根据引理6的性质(ⅰ),从式(14)可得同时,从式(14)可知因此,根据引理3,从式(16)可得结合方程(2),式(15)和(17)即得因为从引理6的性质(ⅱ)可知p j|S(p rjkj),所以当r j=1时,从式(18)可知又因从式(16)可知p j↾m,所以根据引理3,从式(14)和(19)可知x必有素因数p i (i≠j)适合从式(20)可知p i>p j.然而,因为r j=1,所以此时根据引理6的性质(ⅴ)和(ⅵ),从式(15)和p i>p j可得这一矛盾.由此可知r j≥2.因为以及m ≥φ(m),所以从式(16)和式(18)可得又从引理6的性质(ⅳ)可知S(p rjkj )>(p j-1)r jk,故从r j>2和式(21)可得另一方面,根据引理6的性质(ⅲ)可知S(p rjkj)≤p jr jk,故从式(18)和r j>2可得由于根据引理5,从式(16)可知故从式(23)和式(24)可得于是,从式(22)和(25)即得定理1.证毕.4 定理2的证明由于p≠q,所以根据文献[14]的定理2.3.3可知适合式(3)的k必为正整数.当x=p q时,从引理3可知同时,根据引理2可知从方程(1)和式(27)可知又因q是奇素数,所以q≥3,故从引理2可得因此从方程(1)和式(29)可知结合式(28)和(30)立得S(x k)=p q-p q-1,故从式(26)可知x=p q是方程(2)在k适合式(3)时的非平凡解.当x=2p q时,从引理3可知又从引理6的性质(ⅰ),(ⅴ)和(ⅵ)可知因此,从式(28),(30),(31)和(32)可知x=2p q 也是方程(2)在k适合式(3)时的非平凡解.于是完成了定理2的证明.【相关文献】[1] GUY R K.Unsolved problems in number theory[M].3rd ed.北京:科学出版社,2007.[2]徐哲峰.Smarandache幂函数的均值[J].数学学报,2006,49(1):77-80.[3]徐哲峰.Smarandache函数的值分布性质[J].数学学报,2006,49(5):1 009-1 012.[4]杨倩丽,王涛,李海龙.Smarandache双阶乘函数性质的研究[J].西安工程大学学报,2006,19(4):494-496.[5]李洁.一个包含Smarandache原函数的方程[J].数学学报,2007,50(2):333-336.[6]马金萍,刘宝利.一个包含Smarandache函数的方程[J].数学学报,2007,50(5):1 185-1 190.[7]贺艳峰,潘晓玮.一个包含Smarandache LCM 函数的方程[J].数学学报,2008,51(4):779-786.[8]路玉麟.一个包含Smarandache函数的方程[J].纺织高校基础科学学报,2008,21(2):253-254.[9] MA J P.An equation involving the Smarandache function[J].Scientia Magna,2005,1(2):89-90.[10] YI Y.An equation involving the Euler function and Smarandache function [J].Scientia Magna,2005,1(2):173-175.[11]黄寿生,陈锡庚.关于数论函数方程φ(n)=S(n 5)[J].华南师范大学学报:自然科学版,2007(4):41-43.[12]曹楠,高丽.关于数论函数方程φ(n)=S(n 7)[J].西南民族大学学报:自然科学版,2009,39(5):992-994.[13]廖思泉.关于数论函数方程φ(n)=S(n k)[J].福州大学学报:自然科学版,2009,37(2):302-304.[14]华罗庚.数论导引[M].北京:科学出版社,1979.。
Stefan-Maxwell Equations
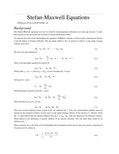
There are several important point to note when we examine Eq. 7. Firs,t the concentration gradient does not depend on the reference velocity (in this case it is the molar average velocity of the mixture v* ). Second, From * Eq. 7 it also follows that the species diffusive flux JA =- AB “ xA does not depend on the reference velocity. What matters is the difference in species velocity. If the species velocities were the same there would be no diffusive flux. These concepts are at the heart of the Stefan-Maxwell formulation which for an ideal gas mixture consisting of species can be written as “ xi = ‚
We can also write the fluxes as a function of the concentration gradients.
Substituting this expression into Eq. 2 gives AB “ xA = - xA vA - vB + Then solving for AB “ xA we get AB x2 “ xA (6)
- 1、下载文档前请自行甄别文档内容的完整性,平台不提供额外的编辑、内容补充、找答案等附加服务。
- 2、"仅部分预览"的文档,不可在线预览部分如存在完整性等问题,可反馈申请退款(可完整预览的文档不适用该条件!)。
- 3、如文档侵犯您的权益,请联系客服反馈,我们会尽快为您处理(人工客服工作时间:9:00-18:30)。
4786
Progress in Industrial and Civil Engineering
Lemma 2. For any given integer number n ≥ 1 , n = p1α1 p2
of n .we have : ϕ ( n ) = ∏ piαi−1 ( pi − 1) .
Applied Mechanics and Materials Vols. 204-208 (2012) pp 4785-4788 © (2012) Trans Tech Publications, Switzerland doi:10.4028//AMM.204-208.4785
9α 2 S ( n9 ) = max S ( p19α1 ) , S ( p2 ),
{
9α k , S pk
(
)} = S ( p )
9r
(2)
From the Lemma 1,
ϕ ( n ) = ϕ ( p r )ϕ
n r p n r −1 = p ( p − 1) ϕ r p
p − 1 ϕ ( n ) ,so, 2 ϕ ( n ) .
(2) if n not have the odd divisor p ,when n > 2 ,we must have n = 2k ,where k ≥ 1, k ∈ N . With the Lemma 2 that, ϕ ( n ) = 2k −1 ( 2 − 1) = 2k −1 , So we also have 2 ϕ ( n ) . From the (1)and(2),we proofed the lemma 3. α Lemma 4. For any given integer number n ≥ 1 , n = p1α1 p2 2
solutions.
Introduction
For any given integer number n ≥ 1 , the well-known Smarandache function is defined as the integer m which is smallest number that can make n m ! [1], that is, S ( n ) = min {m : m ∈ N , n m !}
let y = p
p .
( p − 1) − α p ,then we have y is increasing. Proof: Because y′ = ( p − 1)(α − 2 ) pα −3 − p ,if α > 3 ,we immediately have
α −2
y′ > 0 .
So we complete the proof of the lemma 6.
Some lemmas
To complete the proof of the problem, we need some simple lemmas. Lemma 1. For any given integer number m, n ,we have : ϕ ( mn ) = ϕ ( m ) ϕ ( n )
(3)
Combine the (1)to(3),we have,
n p r −1 ( p − 1) ϕ r = S ( p9 r ) p
(4)
Applied Mechanics and Materials Vols. 204-208
4787
n First,when r = 1 and p = 2 ,From the Lemma 4, S ( 29 ) = 12 = ϕ ,that is n = 13, 26 , 2 9 9 but S (13 ) = 117 ≠ 12 and S ( 26 ) = 117 ≠ 12 ,so n = 13, 26 is not the solution of equation (1).
The Euler function is defined as the positive integer number which is no more than n and coprime n ′ with n . that is, ϕ ( n ) = ∑1 .
k =1
The sign ' means the summation with all the coprime positive integer of n [2]. The problems about Smarandache function and Euler function equation ϕ ( n ) = S ( n k ) in elementary number theory are
Online: 2012-10-26
An equation involving the Smarandache function Bin Chen
Department of Mathematics, Weinan Normal University, Weinan Shaanxi, P.R. China ccbb@ Keywords:Euler function; Smarandache function; The solutions of the equation.
n = 1,8,9,12,18 . In reference [4], Yi Yuan had gave all the solutions of the equation S ( n ) = ϕ ( nk ) when
k = 2,3, 4 . In reference [5], Huang Shousheng found the solutions of the equation S ( n ) = ϕ ( n5 ) ,that is n = 1, 64 . In reference [6], Zheng Tao got the solutions of the equation S ( n ) = ϕ ( n6 ) ,that is n = 1,81,96,169,338 .Gao Nan discussed the solutions of the equation S ( n ) = ϕ ( nk ) when k = 7 . The author had obtained the olutions of the equation
Abstract. For any positive integer n , let ϕ ( n ) and S ( n ) denote the Euler function and the Smarandache function of the integer n . In this paper, we use the elementary number theory methods to get the solutions of the equation ϕ ( n ) = S ( n k ) if the k = 9 , and give its all positive integer
Proof: See the m of the text [2]. we immediately complete the proof of the lemma 1.
All rights reserved. No part of contents of this paper may be reproduced or transmitted in any form or by any means without the written permission of Trans Tech Publications, . (ID: 141.211.4.224, University of Michigan Library, Media Union Library, Ann Arbor, USA-04/07/15,13:19:05)
α1 α2 of n .we have : S ( n ) = max S p1 , S p2 ,
{( ) ( )
αk , S pk
( )}
prα r is the prime power factorization
Proof: See the theorem of the text [7]. we immediately complete the proof of the lemma 4. Lemma 5. For any given integer number k ≥ 1 and the prime number p .we have : S ( p k ) ≤ kp ,
i =1 k
α2
prα r is the prime power factorization
Proof: See the theorem of the text [2]. we immediately complete the proof of the lemma 2. Lemma 3. let n > 2 ,we have 2 ϕ ( n ) . Proof: (1) if n have the odd divisor p , that is p > 2 and 2 p − 1 ,from the Lemma2 we have,
S ( n ) = ϕ ( nk ) when
k = 8 ,that
is
n = 1,125, 250, 289,578 . The main purpose of this paper is to study the problem S ( n ) = ϕ ( nk ) when k = 9 by using the new elementary methods, and solved it thoroughly. That is the equation S ( n ) = ϕ ( n9 ) holds if and only if n = 1,361, 722 .