Using Analytic QP and Sparseness to Speed Training of Support Vector Machines
香精油中农药残留

Determination of Pesticide Minimum Residue Limits in Essential OilsReport No 3A report for the Rural Industries Research andDevelopment CorporationBy Professor R. C. Menary & Ms S. M. GarlandJune 2004RIRDC Publication No 04/023RIRDC Project No UT-23A© 2004 Rural Industries Research and Development Corporation.All rights reserved.ISBN 0642 58733 7ISSN 1440-6845‘Determination of pesticide minimum residue limits in essential oils’, Report No 3Publication No 04/023Project no.UT-23AThe views expressed and the conclusions reached in this publication are those of the author and not necessarily those of persons consulted. RIRDC shall not be responsible in any way whatsoever to any person who relies in whole or in part on the contents of this report.This publication is copyright. However, RIRDC encourages wide dissemination of its research, providing the Corporation is clearly acknowledged. For any other enquiries concerning reproduction, contact the Publications Manager on phone 02 6272 3186.Researcher Contact DetailsProfessor R. C. Menary & Ms S. M. GarlandSchool of Agricultural ScienceUniversity of TasmaniaGPO Box 252-54HobartTasmania 7001AustraliaPhone: (03) 6226 2723Fax: (03) 6226 7609Email: r.menary@.auIn submitting this report, the researcher has agreed to RIRDC publishing this material in its edited form.RIRDC Contact DetailsRural Industries Research and Development CorporationLevel 1, AMA House42 Macquarie StreetBARTON ACT 2600PO Box 4776KINGSTON ACT 2604Phone: 02 6272 4819Fax: 02 6272 5877Email: rirdc@.auWebsite: .auPublished in June 2004Printed on environmentally friendly paper by Canprint.FOREWORDInternational regulatory authorities are standardising the levels of pesticide residues present in products on the world market which are considered acceptable. The analytical methods to be used to confirm residue levels are also being standardised. To constructively participate in these processes, Australia must have a research base capable of constructively contributing to the establishment of methodologies and must be in a position to assess the levels of contamination within our own products.Methods for the analysis for pesticide residues rarely deal with their detection in the matrix of essential oils. This project is designed to develop and validate analytical methods and apply that methodology to monitor pesticide levels in oils produced from commercial harvests. This will provide an overview of the levels of pesticide residues we can expect in our produce when normal pesticide management programs are adhered to.The proposal to produce a manual which deals with the specific problems associated with detection of pesticide residues in essential oils is intended to benefit the essential oil industry throughout Australia and may prove useful to other horticultural products.This report is the third in a series of four project reports presented to RIRDC on this subject. It is accompanied by a technical manual detailing methodologies appropriate to the analysis for pesticide residues in essential oils.This project was part funded from RIRDC Core Funds which are provided by the Australian Government. Funding was also provided by Essential Oils of Tasmania and Natural Plant Extracts Cooperative Society Ltd.This report, an addition to RIRDC’s diverse range of over 1000 research publications, forms part of our Essential Oils and Plant Extracts R&D program, which aims for an Australian essential oils and plant extracts industry that has established international leadership in production, value adding and marketing.Most of our publications are available for viewing, downloading or purchasing online through our website:•downloads at .au/fullreports/index.html•purchases at .au/eshopSimon HearnManaging DirectorRural Industries Research and Development CorporationAcknowledgementsOur gratitude and recognition is extended to Dr. Noel Davies (Central Science Laboratories, University of Tasmania) who provided considerable expertise in establishing procedures for chromatography mass spectrometry.The contribution to extraction methodologies and experimental work-up of Mr Garth Oliver, Research Assistant, cannot be underestimated and we gratefully acknowledge his enthusiasm and novel approaches.Financial and ‘in kind’ support was provided by Essential Oils Industry of Tasmania, (EOT).AbbreviationsADI Average Daily IntakeAGAL Australian Government Analytical Laboratoriesingredientai activeAPCI Atmospheric Pressure Chemical IonisationBAP Best Agricultural PracticesenergyCE collisionDETA DiethylenetriamineECD Electron Capture DetectorionisationESI ElectrosprayFPD Flame Photometric DetectionChromatographyGC GasResolutionHR HighChromatographyLC LiquidLC MSMS Liquid Chromatography with detection monitoring the fragments of Mass Selected ionsMRL Maximum Residue LimitSpectrometryMS MassNRA National Registration AuthorityR.S.D. Relative Standard DeviationSFE Supercritical Fluid ExtractionSIM Single Ion MonitoringSPE Solid Phase ExtractionTIC Total Ion ChromatogramContents FOREWORD (III)ACKNOWLEDGEMENTS (IV)ABBREVIATIONS (V)CONTENTS (VI)EXECUTIVE SUMMARY (VII)1. INTRODUCTION (1)1.1B ACKGROUND TO THE P ROJECT (1)1.2O BJECTIVES (2)1.3M ETHODOLOGY (2)2. EXPERIMENTAL PROTOCOLS & DETAILED RESULTS (3)2.1M ETHOD D EVELOPMENT (3)2.2M ONITORING OF H ARVESTS (42)2.3P RODUCTION OF M ANUAL (46)3. CONCLUSIONS (47)IMPLICATIONS & RECOMMENDATIONS (50)BIBLIOGRAPHY (50)Executive SummaryThe main objective of this project was to continue method development for the detection of pesticide residues in essential oils, to apply those methodologies to screen oils produced by major growers in the industry and to produce a manual to consolidate and coordinate the results of the research. Method development focussed on the effectiveness of clean-up techniques, validation of existing techniques, the assessment of the application of gas chromatography (GC) with detection using electron capture detectors (ECD), flame photometric detectors (FPD) and high pressure liquid chromatography (HPLC) with ion trap mass selective (MS) detection.The capacity of disposable C18 cartridges to separate components of boronia oil was found to be limited with the majority of boronia components being eluted on the solvent front, with little to no separation achieved. The cartridges were useful, however, in establishing the likely interaction of reverse phases (RP) C18 columns with components of essential oils, using polar mobile phases . The loading of large amounts of oil onto RP HPLC columns presents the risk of permanently contaminating the bonded phases. The lack of retention of components on disposable SPE C18 cartridges, despite the highly polar mobile phase, presented a good indication that essential oils would not accumulate on HPLC RP columns.The removal of non-polar essential oil components by solvent partitioning of distilled oils was minimal, with the recovery of pesticides equivalent to that recorded for the essential oil components. However application of this technique was of advantage in the analysis of solvent extracted essential oils such as those produced from boronia and blackcurrant.ECD was found to be successful in the detection of terbacil, bromacil, haloxyfop ester, propiconazole, tebuconazole and difenaconzole. However, analysis of pesticide residues in essential oils by application of GC ECD is not sufficiently sensitive to allow for a definitive identification of any contaminant. As a screen, ECD will only be effective in establishing that, in the absence of a peak eluting with the correct retention time, no gross contamination of pesticide residues in an essential oil has occurred . In the situation where a peak is recorded with the correct elution characteristics, and which is enhanced when the sample is fortified with the target analyte, a second means of contaminant identification would be required. ECD, then, can only be used to rule out significant contamination and could not in itself be adequate for a positive identification of pesticide contamination.Benchtop GC daughter, daughter mass spectrometry (MSMS) was assessed and was not considered practical for the detection of pesticide residues within the matrix of essential oils without comprehensive clean-up methodologies. The elution of all components into the mass spectrometer would quickly lead to detector contamination.Method validation for the detection of 6 common pesticides in boronia oil using GC high resolution mass spectrometry was completed. An analytical technique for the detection of monocrotophos in essential oils was developed using LC with detection by MSMS. The methodology included an aqueous extraction step which removed many essential oil components from the sample.Further method development of LC MSMS included the assessment of electrospray ionisation (ESI) and atmospheric pressure chemical ionisation (APCI. For the chemicals trialed, ESI has limited application. No response was recorded for some of the most commonly used pesticides in the essential oil industry, such as linuron, oxyflurofen, and bromacil. Overall, there was very little difference between the sensitivity for ESI and APCI. However, APCI was slightly more sensitive for the commonly used pesticides, tebuconazole and propiconazole, and showed a response, though poor, to linuron and oxyflurofen. In addition, APCI was the preferred ionisation method for the following reasons,♦APCI uses less nitrogen gas compared to ESI, making overnight runs less costly;♦APCI does not have the high back pressure associated with ionisation by ESI such that APCI can be run in conjunction with UV-VIS without risk of fracturing the cell, which is pressure sensitive. Analytes that ionised in the negative APCI mode were incorporated into a separate screen which included bromacil, terbacil, and the esters of the fluazifop and haloxyfop acids. Further work using APCI in the positive mode formed the basis for the inclusion of monocrotophos, pirimicarb, propazine and difenaconazole into the standard screen already established. Acephate, carbaryl, dimethoate, ethofumesate and pendimethalin all required further work for enhanced ionisation and / or improved elution profiles. Negative ionisation mode for APCI gave improved characteristics for dicamba, procymidone, MCPA and mecoprop.The thirteen pesticides included in this general screen were monocrotophos, simazine, cyanazine, pirimicarb, propazine, sethoxydim, prometryb, tebuconazole, propiconazole, , difenoconazole and the esters of fluroxypyr, fluazifop and haloxyfop.. Bromacil and terbacil were not included as both require negative ionisation and elute within the same time window as simazine, which requires positive ionisation. Cycling the MS between the two modes was not practical.The method validation was tested against three oils, peppermint, parsley and fennel.Detection limits ranged from 0.1 to 0.5 mgkg-1 within the matrix of the essential oils, with a linear relationship established between pesticide concentration and peak height (r2 greater than 0.997) and repeatabilities, as described by the relative standard deviation (r.s.d), ranging from 3 to 19%. The type of oil analysed had minimal effect on the response function as expressed by slope of the standard curve.The pesticides which have an carboxylic acid moiety such as fluazifop, haloxyfop and fluroxypyr, present several complications in any analytical method development. The commercial preparations usually have the carboxylic acid in the ester form, which is hydrolysed to the active acidic form on contact with soil and vegetation. In addition, the esters may be present in several forms, such as the ethoxy ethyl or butyl esters. Detection using ESI was tested. Preliminary results indicate that ESI is unsuitable for haloxyfop and fluroxypyr ester. Fluazifop possessed good ionisation characteristics using ESI, with responses approximately thirty times that recorded for haxloyfop. Poor chromatography and response necessitated improved mobile phase and the effect of pH on elution characteristics was considered the most critical parameter. The inclusion of acetic acid improved peak resolution.The LC MSMS method for the detection of dicamba, fluroxypyr, MCPA, mecoprop and haloxyfop in peppermint and fennel distilled oils underwent the validation process. Detection limits ranged from 0.01 to 0.1 mgkg-1Extraction protocols and LC MSMS methods for the detection of paraquat and diquat were developed. ESI produced excellent responses for both paraquat and diquat, after some modifications of the mobile phase. Extraction methodology using aqueous phases were developed. Extraction with carbonate buffer proved to be the most effective in terms of recovery and robustness. A total ion chromatogram of the LC run of an aqueous extract of essential oil was recorded and detection using a photodiode array detector confirmed that very little essential oil matrix was co-extracted. The low background noise indicated that samples could be introduced directly into the MS. This presented a most efficient and rapid way for analysis of paraquat and diquat, avoiding the need for specialised columns or modifiers to be included in the mobile phase to instigate ion exchange.The adsorbtion of paraquat and diquat onto glass and other surfaces was reduced by the inclusion of diethylenetriamine (DETA). DETA preferentially accumulates on the surfaces of sample containers, competitively binding to the adsorption sites. All glassware used in the paraquat diquat analysis were washed in a 5% solution of 0.1M DETA, DETA was included in all standard curve preparations, oils were extracted with aqueous DETA and the mobile phase was changed to 50:50 DETA / methanol. The stainless steel tubing on the switching valve was replaced with teflon, further improvingreproducibility. Method validation was undertaken of the analysis of paraquat and diquat using the protocols established. The relationship between analyte concentration and peak area was not linear at low concentrations, with adsorption more pronounced for paraquat, such that the response for this analyte was half that seen for diquat and the 0.1 mgkg-1 level.The development of a method for the detection of the dithiocarbamate, mancozeb was commenced. Disodium N, N'-ethylenebis(dithiocarbamate) was synthesised as a standard for the derivatised final analytical product. An LC method, with detection using MSMS, was successfully completed. The inclusion of a phase transfer reagent, tetrabutylammonium hyrdrogen sulfate, required in the derivatisation step, contaminated the LC MSMS system, such that any signal from the target analyte was masked. Alternatives to the phase transfer reagent are now being investigated.Monitoring of harvests were undertaken for the years spanning 1998 to 2001. Screens were conducted covering a range of solvent extracted and distilled oils. Residues tested for included tebuconazole, simazine, terbacil, bromacil, sethoxydim, prometryn, oxyflurofen, pirimicarb, difenaconazole, the herbicides with acidic moieties and paraquat and diquat. Problems continued for residues of propiconazole in boronia in the 1998 / 1999 year with levels to 1 mgkg-1 still being detected. Prometryn residues were detected in a large number of samples of parsley oil.Finally the information gleaned over years of research was collated into a manual designed to allow intending analysts to determine methodologies and equipment most suited to the type of the pesticide of interest and the applicability of analytical equipment generally available.1. Introduction1.1 Background to the ProjectResearch undertaken by the Horticultural Research Group at the University of Tasmania, into pesticide residues in essential oils has been ongoing for several years and has dealt with the problems specific to the analysis of residues within the matrix of essential oils. Analytical methods for pesticides have been developed exploiting the high degree of specificity and selectivity afforded by high resolution gas chromatography mass spectrometry. Standard curves, reproducibility and detection limits were established for each. Chemicals, otherwise not amenable to gas chromatography, were derivatised and incorporated into a separate screen to cover pesticides with acidic moieties.Research has been conducted into low resolution GC mass selective detectors (MSD and GC ECD. Low resolution GC MSD achieved detection to levels of 1 mgkg-1 in boronia oil, whilst analysis using GC ECD require a clean-up step to effectively detect halogenated chemicals below 1mgkg-1.Dithane (mancozeb) residues were digested using acidified stannous chloride and the carbon disulphide generated from this reaction analysed by GC coupled to FPD in the sulphur mode.Field trials in peppermint crops were established in accordance with the guidelines published by the National Registration Authority (NRA), monitoring the dissipation of Tilt and Folicur residues in peppermint leaves and the co-distillation of these residues with hydro-distilled peppermint oils were assessed.Development of extraction protocols, analytical methods, harvest monitoring and field trials were continued and were detailed in a subsequent report. Solvent-based extractions and supercritical fluid extraction (SFE) was found to have limited application in the clean-up of essential oilsIn conjunction with Essential Oils of Tasmania (EOT), the contamination risk, associated with the introduction of a range of herbicides, was assessed through a series of field trials. This required analytical method development to detect residues in boronia flowers, leaf and oil. The methodology for a further nine pesticides was successful applied. Detection limits for these chemicals ranged from 0.002 mgkg-1 to 0.1 mgkg-1. In addition, methods were developed to analyse for herbicides with active ingredients (ai) whose structure contained acidic functional groups. Two methods of pesticide application were trialed. Directed sprays refer to those directed on the stems and leaves of weeds at the base of boronia trees throughout the trial plot. Cover sprays were applied over the entire canopy. For all herbicides for which significant residues were detected, it was evident that cover sprays resulted in contamination levels ten times those occurring as a result of directed spraying in some instances. Chloropropham, terbacil and simazine presented potentially serious residue problems, with translocation of the chemical from vegetative material to the flower clearly evident.Directed spray applications of diuron and dimethenamid presented only low residue levels in extracted flowers with adequate control of weeds. Oxyflurofen and the mixture of bromacil and diuron (Krovar) presented only low levels of residues when used as a directed spray and were effective as both post and pre-emergent herbicides. Only very low levels of residues of both sethoxydim and norflurazon were detected in boronia oil produced in crops treated with directed spray applications. Sethoxydim was effective as a cover spray for grasses whilst norflurazon showed potential as herbicide to be used in combination with other chemicals such as diuron, paraquat and diquat. Little contamination of boronia oils by herbicides with acidic moieties was found. This advantage, however, appears to be offset by the relatively poor weed control. Both pendimethalin and haloxyfop showed good weed control. Both, however, present problems with chemical residues in boronia oil and should only be used as a directed sprayThe stability of tebuconazole, monocrotophos and propiconazole in boronia under standard storage conditions was investigated. Field trials of tebuconazole and propiconazole were established in commercial boronia crops and the dissipation of both were monitored over time. The amount of pesticide detected in the oils was related to that originally present in the flowers from which the oils were produced.Experiments were conducted to determine whether the accumulation of terbacil residues in peppermint was retarding plant vigour. The level recorded in the peppermint leaves were comparatively low. Itis unlikely that terbacil carry over is the cause for the lack of vigour in young peppermint plants.Boronia oils produced in 1996, 1997 and 1998 were screened for pesticides using the analytical methods developed. High levels of residues of propiconazole were shown to persist in crops harvested up until 1998. Field trials have shown that propiconazole residues should not present problems if the fungicide is used as recommended by the manufacturers.1.2 Objectives♦Provide the industry, including the Standards Association of Australia Committee CH21, with a concise practical reference, immediately relevant to the Australian essential oil industry♦Facilitate the transfer of technology from a research base to practical application in routine monitoring programs♦Continue the development of analytical methods for the detection of metabolites of the active ingredients of pesticide in essential oils.♦Validate the methods developed.♦Provide industry with data supporting assurances of quality for all exported products.♦Provide a benchmark from which Australia may negotiate the setting of a realistic maximum residue limit (MRL)♦Determine whether the rate of uptake is relative to the concentration of active ingredient on the leaf surface may establish the minimum application rates for effective pest control.1.3 MethodologyThree approaches were used to achieve the objectives set out above.♦Continue the development and validation of analytical methods for the detection of pesticide residues in essential oils. Analytical methods were developed using gas chromatography high resolution mass spectrometry (GC HR MS), GC ECD, GC FPD and high pressure liquid chromatography with detection using MSMS.♦Provide industry with data supporting assurances of quality for all exported products.♦Coordinate research results into a comprehensive manual outlining practical approaches to the development of analytical proceduresOne aspect of the commissioning of this project was to provide a cost effective analytical resource to assess the degree of the pesticide contamination already occurring in the essential oils industry using standard pesticide regimens. Oil samples from annual harvests were analysed for the presence of pesticide residues. Data from preceding years were collated to determine the progress or otherwise, in the application of best agricultural practice (BAP).2. Experimental Protocols & Detailed ResultsThe experimental conditions and results are presented under the following headings:♦Method Development♦Monitoring of Commercial Harvests♦Production of a Manual2.1 Method DevelopmentMethod development focussed on the effectiveness of clean-up techniques, validation of existing techniques, the assessment of the application of GC ECD and FPD and high pressure liquid chromatography with ion trap MS, MS detection.2.1.1 Clean-up Methodologies2.1.1.i. Application of Disposable SPE cartridges in the clean-up of pesticide residues in essentialoilsLiterature reviews provided limited information with regards to the separation of contaminants within essential oils. The retention characteristics of disposable C18 cartridges were trialed.Experiment 1;Aim : To assess the capacity of disposable C18 cartridges to the separation of boronia oil components. Experimental : Boronia concrete (49.8 mg) was dissolved in 0.5 mL of acetone and 0.4 mL of chloroform was added. 1mg of octadecane was added as an internal standard. A C18 Sep-Pak Classic cartridge (short body) was pre- conditioned with 1.25 mL of methanol, which was passed through the column at 7.5 mLmin-1, followed by 1.25 mL of acetone, at the same flow rate. The boronia samplewas then applied to the column at 2 mLmin-1 flow and eluted with 1.25 mL of acetone / chloroform (5/ 4) and then eluted with a further 2.5 mL of chloroform. 5 fractions of 25 drops each were collected. The fractions were analysed by GC FID using the following parametersAnalytical parameters6890PackardHewlettGCcolumn: Hewlett Packard 5MS 30m, i.d 0.32µmcarrier gas instrument grade nitrogeninjection volume: 1µL (split)injector temp: 250°Cdetector temp: 280°Cinital temp: 50°C (3 min), 10°Cmin-1 to 270°C (7 mins)head pressure : 10psi.Results : Table 1 record the percentage volatiles detected in the fractions collectedFraction 1 2 3 4 5 % components eluting 18 67 13 2636%monoterpenes 15%sesquiquiterpenes 33 65 2%high M.W components 1 43 47 9Table 1. Percentage volatiles eluting from SPE C18 cartridgesDiscussion : The majority of boronia components eluted on the solvent front, effecting minimal separation. This area of SPE clean-up of essential oils requires a wide ranging investigation, varying parameters such as cartridge type and polarity of mobile phase.Experiment 2.Aim : For the development of methods using LC MSMS without clean-up steps, the potential for oil components to accumulate on the reverse phase (RP) column must be assessed. The retention of essential oil components on SPE C18 cartridges, using the same mobile phase as that to be used in theLC system, would provide a good indication as to the risk of contamination of the LC columns withoil components.Experimental: Parsley oil (20-30 mg) was weighed into a GC vial. 200 µL of a 10 µgmL-1 solution (equivalent to 100mgkg-1 in oil) of each of sethoxydim, simazine, terbacil, prometryn, tebuconazoleand propiconazole were used to spike the oil, which was then dissolved in 1.0 mL of acetonitrile. The solution was then slowly introduced to the C18 cartridge (Waters Sep Pac 'classic' C18 #51910) using a disposable luer lock, 10 mL syringe, under constant manual pressure, and eluted with 9 mLs of acetonitrile. Ten, 1 mL fractions were collected and transferred to GC vials. 1mg of octadecane was added to each vial and the samples were analysed by GC FID under the conditions described in experiment 1.The experiment was repeated using C18 cartridges which had been pre-conditioned with distilled waterfor 15 mins. Again, parsley oil, spiked with pesticides was eluted with acetonitrile and 5 x 1 mL fractions collected.Results: The majority of oil components and pesticides were eluted from the C18 cartridge in the firsttwo fractions. Little to no separation of the target pesticides from the oil matrix was achieved. Table2 lists the distribution of essential oil components in the fractions collected.Fraction 1 2 3 4 5 % components eluting 18 67 13 2663%monoterpenes 15%sesquiquiterpenes 33 65 2%high M.W components 1 43 47 9water conditioned% components eluting 35 56 8 12%monoterpenes 3068%sesquiquiterpenes 60 39 1 0%high M.W components 0 50 42 7Table 2. Percentage volatiles eluting for SPE C18 cartridgesFigure 1 shows a histogram of the percentage distribution of components from the oil in each of the four fractions.Figure 1. Histogram of the percentage of volatiles of distilled oils in each of four fraction elutedon SPE C18 cartridges (non-preconditioned)Figure 2. Histogram of the percentage of volatiles of distilled oils in each of four fraction elutedon SPE C18 cartridges (preconditioned)Discussion : The chemical properties of many of the target pesticides, including polarity, solubility in organic solvents and chromatographic behaviour, are similar to the majority of essential oil components. This precludes the effective separation of analytes from such matrices through the use of standard techniques, where the major focus is pre-concentration of pesticide residues from water or water based vegetative material. However, this experiment served to provide a good indication that under HPLC conditions, where a reverse phase C18 column is used in conjunction with acetonitrile / water based mobile phases, essential oil components do not remain on the column.。
【精】数据库CH(6)(学习资料)

Formal Relational Query LanguagesIn this chapter we study three additional formal relational languages.RelationalAlgebra,tuple relational calculus and domain relational calculus.Of these three formal languages,we suggest placing an emphasis on rela-tional algebra,which is used extensively in the chapters on query processing andoptimization,as well as in several other chapters.The relational calculi generallydo not merit as much emphasis.Our notation for the tuple relational calculus makes it easy to present the concept of a safe query.The concept of safety for the domain relational calcu-lus,though identical to that for the tuple calculus,is much more cumbersomenotationally and requires careful presentation.This consideration may suggestplacing somewhat less emphasis on the domain calculus for classes not focusingon database theory.Exercises6.10Write the following queries in relational algebra,using the universityschema.a.Find the names of all students who have taken at least one Comp.Sci.course.b.Find the ID s and names of all students who have not taken any courseoffering before Spring2009.c.For each department,find the maximum salary of instructors in thatdepartment.You may assume that every department has at least oneinstructor.d.Find the lowest,across all departments,of the per-department maxi-mum salary computed by the preceding query.Answer:4344Chapter6Formal Relational Query Languagesemployee(person name,street,city)works(person name,company name,salary)company(company name,city)manages(person name,manager name)Figure6.22Relational database for Exercises6.2,6.8,6.11,6.13,and6.15a. name(student1takes1 course id(dept name=′Comp.Sci.′(course)))Note that if we join student,takes,and course,only students fromthe Comp.Sci.department would be present in the result;studentsfrom other departments would be eliminated even if they had taken aComp.Sci.course since the attribute dept name appears in both studentand course.b. I D,name(student)− I D,name(year<2009(student1takes)Note thatSpring is thefirst semester of the year,so we do not need to performa comparison on semester.c.dept name G max(salary)(instructor)d.G min(maxsal)(dept name G max(salary)as maxsal(instructor))6.11Consider the relational database of Figure6.22,where the primary keys areunderlined.Give an expression in the relational algebra to express each ofthe following queries:a.Find the names of all employees who work for“First Bank Corpora-tion”.b.Find the names and cities of residence of all employees who work for“First Bank Corporation”.c.Find the names,street addresses,and cities of residence of all em-ployees who work for“First Bank Corporation”and earn more than$10,000.d.Find the names of all employees in this database who live in the samecity as the company for which they work.e.Assume the companies may be located in several cities.Find all com-panies located in every city in which“Small Bank Corporation”islocated.Answer:(w orks))a. person name(company name=“First Bank Corporation”b. person name,city(employee1(w orks)))(company name=“First Bank Corporation”Exercises 45c. person name ,street ,city ((company name =“First Bank Corporation”∧salar y >10000)w orks 1employee )d. person name (employee 1w orks 1company )e.Note:Small Bank Corporation will be included in each answer.company name (company ÷( city (company name =“Small Bank Corporation”(company ))))6.12Using the university example,write relational-algebra queries to find thecourse sections taught by more than one instructor in the following ways:a.Using an aggregate function.b.Without using any aggregate functions.Answer:a.instrcnt >1(course id ,section id ,year ,semester G count (∗)as instrcnt (teaches ))b.course id ,section id ,year ,semester (I D <>ID2(takes 1takes1(ID2,course id ,section id ,year ,semester )(takes )))6.13Consider the relational database of Figure 6.22.Give a relational-algebraexpression for each of the following queries:a.Find the company with the most employees.b.Find the company with the smallest payroll.c.Find those companies whose employees earn a higher salary,on av-erage,than the average salary at First Bank Corporation.Answer:a.t 1←company name G count-distinct (person name )(w orks )t 2←G max (num employees)(company strength (company name ,num employees )(t 1))company name (t 3(company name ,num employees )(t 1)1t 4(num employees )(t 2))b.t 1←company name G sum (salary )(w orks )t 2←G min (payroll )(company payroll (company name ,payroll )(t 1)) company name (t 3(company name ,payroll )(t 1)1t 4(payroll )(t 2))c.t 1←company name G avg (salary )(w orks )t 2←company name =“First Bank Corporation”(t 1) t pany name ((t 3(company name ,a v g salar y )(t 1))1t 3.a v g salar y >f irst bank .a v g salar y (f irst bank (company name ,a v g salar y )(t 2)))6.14Consider the following relational schema for a library:46Chapter6Formal Relational Query Languagesmember(memb no,name,dob)books(isbn,title,authors,publisher)borrowed(memb no,isbn,date)Write the following queries in relational algebra.a.Find the names of members who have borrowed any book publishedby“McGraw-Hill”.b.Find the name of members who have borrowed all books publishedby“McGraw-Hill”.c.Find the name and membership number of members who have bor-rowed more thanfive different books published by“McGraw-Hill”.d.For each publisher,find the name and membership number of mem-bers who have borrowed more thanfive books of that publisher.e.Find the average number of books borrowed per member.Take intoaccount that if an member does not borrow any books,then thatmember does not appear in the borrowed relation at all.Answer:a.t1← isbn(publisher=“McGra w−Hill′′(books))name((member1borro w ed)1t1))b.t1← isbn(publisher=“McGra w−Hill′′(books))name,isbn(member1borro w ed)÷t1c.t1←member1borro w ed1(publisher=“McGra w−Hill′′(books))name(countisbn>5((memb no G count-distinct(isbn)as countisbn(t1))))d.t1←member1borro w ed1bookspublisher,name(countisbn>5((publisher,memb no G count-distinct(isbn)as countisbn(t1)))6.15Consider the employee database of Figure6.22.Give expressions in tuplerelational calculus and domain relational calculus for each of the followingqueries:a.Find the names of all employees who work for“First Bank Corpora-tion”.b.Find the names and cities of residence of all employees who work for“First Bank Corporation”.c.Find the names,street addresses,and cities of residence of all em-ployees who work for“First Bank Corporation”and earn more than$10,000.Exercises47 d.Find all employees who live in the same city as that in which thecompany for which they work is located.e.Find all employees who live in the same city and on the same streetas their managers.f.Find all employees in the database who do not work for“First BankCorporation”.g.Find all employees who earn more than every employee of“SmallBank Corporation”.h.Assume that the companies may be located in several cities.Find allcompanies located in every city in which“Small Bank Corporation”is located.Answer:a.Find the names of all employees who work for First Bank Corporation:i.{t|∃s∈w orks(t[person name]=s[person name]∧s[company name]=“First Bank Corporation”)} ii.{<p>|∃c,s(<p,c,s>∈w orks∧c=“First Bank Corporation”)} b.Find the names and cities of residence of all employees who work forFirst Bank Corporation:i.{t|∃r∈employee∃s∈w orks(t[person name]=r[person name]∧t[city]=r[city]∧r[person name]=s[person name]∧s[company name]=’First Bank Corporation’)} ii.{<p,c>|∃co,sa,st(<p,co,sa>∈w orks∧<p,st,c>∈employee∧co=“First Bank Corporation”)}c.Find the names,street address,and cities of residence of all employeeswho work for First Bank Corporation and earn more than$10,000perannum:i.{t|t∈employee∧(∃s∈w orks(s[person name]=t[person name]∧s[company name]=“First Bank Corporation”∧s[salar y]> 10000))}ii.{<p,s,c>|<p,s,c>∈employee∧∃co,sa(<p,co,sa>∈w orks∧co=’First Bank Corporation’∧sa>10000)}d.Find the names of all employees in this database who live in the samecity as the company for which they work:48Chapter6Formal Relational Query Languagesi.{t|∃e∈employee∃w∈w orks∃c∈company(t[person name]=e[person name]∧e[person name]=w[person name]∧w[company name]=c[company name]∧e[city]=c[city])}ii.{<p>|∃st,c,co,sa(<p,st,c>∈employee∧<p,co,sa>∈w orks∧<co,c>∈company)}e.Find the names of all employees who live in the same city and on thesame street as do their managers:i.{t|∃l∈employee∃m∈manages∃r∈employee(l[person name]=m[person name]∧m[manager name]=r[person name]∧l[street]=r[street]∧l[city]=r[city]∧t[person name]=l[person name])}ii.{<t>|∃s,c,m(<t,s,c>∈employee∧<t,m>∈manages∧<m,s,c>∈employee)}f.Find the names of all employees in this database who do not workfor First Bank Corporation:If one allows people to appear in the database(e.g.in employee)but notappear in works,the problem is more complicated.We give solutionsfor this more realistic case later.i.{t|∃w∈w orks(w[company name]=“First Bank Corporation”∧t[person name]=w[person name])}ii.{<p>|∃c,s(<p,c,s>∈w orks∧c=“First Bank Corporation”)}If people may not work for any company:i.{t|∃e∈employee(t[person name]=e[person name]∧¬∃w∈w orks(w[company name]=“First Bank Corporation”∧w[person name]=t[person name]))}ii.{<p>|∃s,c(<p,s,c>∈employee)∧¬∃x,y(y=“First Bank Corporation”∧<p,y,x>∈w orks)}g.Find the names of all employees who earn more than every employeeof Small Bank Corporation:i.{t|∃w∈w orks(t[person name]=w[person name]∧∀s∈w orks(s[company name]=“Small Bank Corporation”⇒w[salar y]>s[salar y]))}Exercises49 ii.{<p>|∃c,s(<p,c,s>∈w orks∧∀p2,c2,s2(<p2,c2,s2>∈w orks∨c2=“Small Bank Corporation”∨s>s2))}h.Assume the companies may be located in several cities.Find all com-panies located in every city in which Small Bank Corporation is lo-cated.Note:Small Bank Corporation will be included in each answer.i.{t|∀s∈company(s[company name]=“Small Bank Corporation”⇒∃r∈company(t[company name]=r[company name]∧r[city]=s[city]))}ii.{<co>|∀co2,ci2(<co2,ci2>∈company∨co2=“Small Bank Corporation”∨<co,ci2>∈company)} 6.16Let R=(A,B)and S=(A,C),and let r(R)and s(S)be relations.Write relational-algebra expressions equivalent to the following domain-relational-calculus expressions:a.{<a>|∃b(<a,b>∈r∧b=17)}b.{<a,b,c>|<a,b>∈r∧<a,c>∈s}c.{<a>|∃b(<a,b>∈r)∨∀c(∃d(<d,c>∈s)⇒<a,c>∈s)}d.{<a>|∃c(<a,c>∈s∧∃b1,b2(<a,b1>∈r∧<c,b2>∈r∧b1>b2))}Answer:a. A(B=17(r))b.r1sc. A(r)∪(r÷B( C(s)))d. r.A((r1s)1c=r2.A∧r.B>r2.B(r2(r)))It is interesting to note that(d)is an abstraction of the notoriousquery“Find all employees who earn more than their manager.”LetR=(emp,sal),S=(emp,mgr)to observe this.6.17Repeat Exercise6.16,writing SQL queries instead of relational-algebra ex-pressions.Answer:a.select afrom rwhere b=1750Chapter6Formal Relational Query Languagesb.select a,b,cfrom r,swhere r.a=s.ac.(select afrom r)union(select afrom s)d.select afrom r as r1,r as r2,swhere r1.a=s.a and r2.a=s.c and r1.b>r2.b6.18Let R=(A,B)and S=(A,C),and let r(R)and s(S)be relations.Using the special constant null,write tuple-relational-calculus expressionsequivalent to each of the following:a.r1sb.r1sc.r1sAnswer:a.{t|∃r∈R∃s∈S(r[A]=s[A]∧t[A]=r[A]∧t[B]=r[B]∧t[C]=s[C])∨∃s∈S(¬∃r∈R(r[A]=s[A])∧t[A]=s[A]∧t[C]=s[C]∧t[B]=null)}b.{t|∃r∈R∃s∈S(r[A]=s[A]∧t[A]=r[A]∧t[B]=r[B]∧t[C]=s[C])∨∃r∈R(¬∃s∈S(r[A]=s[A])∧t[A]=r[A]∧t[B]=r[B]∧t[C]=null)∨∃s∈S(¬∃r∈R(r[A]=s[A])∧t[A]=s[A]∧t[C]=s[C]∧t[B]=null)}c.{t|∃r∈R∃s∈S(r[A]=s[A]∧t[A]=r[A]∧t[B]=r[B]∧t[C]=s[C])∨∃r∈R(¬∃s∈S(r[A]=s[A])∧t[A]=r[A]∧t[B]=r[B]∧t[C]=null)}6.19Give a tuple-relational-calculus expression tofind the maximum value inrelation r(A).Answer:{<a>|<a>∈r∧∀<b>∈R a>=b}。
-》基于EMD和样本熵的滚动轴承故障SVM识别
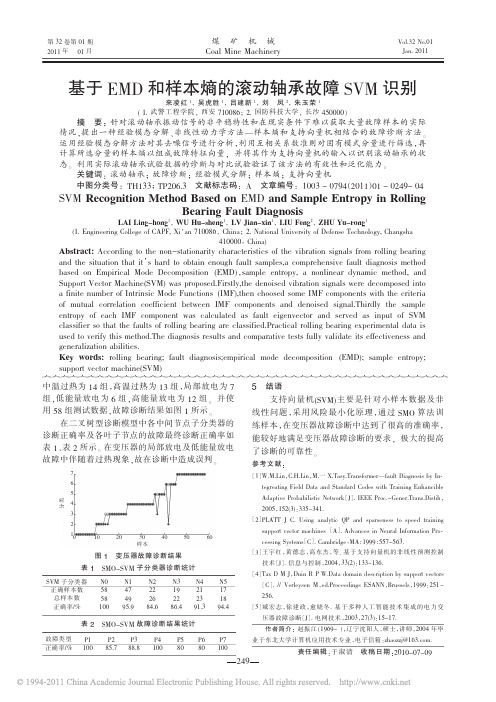
中温过热为14组,高温过热为13组,局部放电为7组,低能量放电为6组,高能量放电为12组。
并使用58组测试数据,故障诊断结果如图1所示。
在二叉树型诊断模型中各中间节点子分类器的诊断正确率及各叶子节点的故障最终诊断正确率如表1、表2所示。
在变压器的局部放电及低能量放电故障中伴随着过热现象,故在诊断中造成误判。
图1变压器故障诊断结果表1SMO-SVM 子分类器诊断统计表2SMO-SVM 故障诊断结果统计5结语支持向量机(SVM)主要是针对小样本数据及非线性问题,采用风险最小化原理,通过SMO 算法训练样本,在变压器故障诊断中达到了很高的准确率,能较好地满足变压器故障诊断的要求,极大的提高了诊断的可靠性。
参考文献:[1]W .M .Lin ,C .H .Lin ,M .一X .Tasy .Transformer —fault Diagnosis by In -tegreating Field Data and Standard Codes with Training Enhancible Adaptive Probabilistic Network [J ].IEEE Proc .-Gener .Trans .Distih ,2005,152(3):335-341.[2]PLATT J C .Using analytic QP and sparseness to speed trainingsupport vector machines [A ].Advances in Neural Information Pro -cessing Systems [C ].Cambridge ,MA :1999:557-563.[3]王宇红,黄德志,高东杰,等.基于支持向量机的非线性预测控制技术[J ].信息与控制,2004,33(2):133-136.[4]Tax D M J ,Duin R P W .Data domain description by support vectors[C ].//Verleysen M ,ed .Proceedings ESANN ,Brussels ,1999:251-256.[5]臧宏志,徐建政,愈晓冬.基于多种人工智能技术集成的电力变压器故障诊断[J ].电网技术,2003,27(3):15-17.作者简介:赵振江(1969-),辽宁沈阳人,硕士,讲师,2004年毕业于东北大学计算机应用技术专业,电子信箱:***************.责任编辑:于淑清收稿日期:2010-07-09SVM 子分类器正确样本数总样本数正确率/%N05858100N1474995.9N2222684.6N3192286.4N4212391.3N5171894.4故障类型正确率/%P1100P285.7P388.8P4100P580P680P7100煤矿机械Coal Mine MachineryVol.32No.01Jan.2011第32卷第01期2011年01月基于EMD 和样本熵的滚动轴承故障SVM 识别来凌红1,吴虎胜1,吕建新1,刘凤2,朱玉荣1(1.武警工程学院,西安710086;2.国防科技大学,长沙450000)摘要:针对滚动轴承振动信号的非平稳特性和在现实条件下难以获取大量故障样本的实际情况,提出一种经验模态分解、非线性动力学方法—样本熵和支持向量机相结合的故障诊断方法。
表面增强拉曼散射
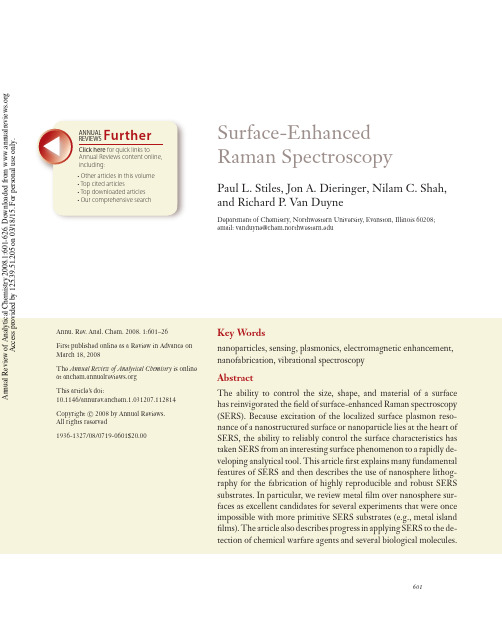
601
1. INTRODUCTION
SERS: surface-enhanced Raman spectroscopy Raman scattering: inelastic scattering of a photon from a molecule in which the frequency change precisely matches the difference in vibrational energy levels LSPR: localized surface plasmon resonance
Surface-Enhanced Raman Spectroscopy
Paul L. Stiles, Jon A. Dieringer, Nilam C. Shah, and Richard P. Van Duyne
Department of Chemistry, Northwestern University, Evanston, Illinois 60208; email: vanduyne@
Annu. Rev. Anal. Chem. 2008. 1:601–26 First published online as a Review in Advance on March 18, 2008 The Annual Review of Analytical Chemistry is online at This article’s doi: 10.1146/annurev.anchem.1.031207.112814 Copyright c 2008 by erved 1936-1327/08/0719-0601$20.00
ANNUAL REVIEWS
Further
A procedural object distribution function
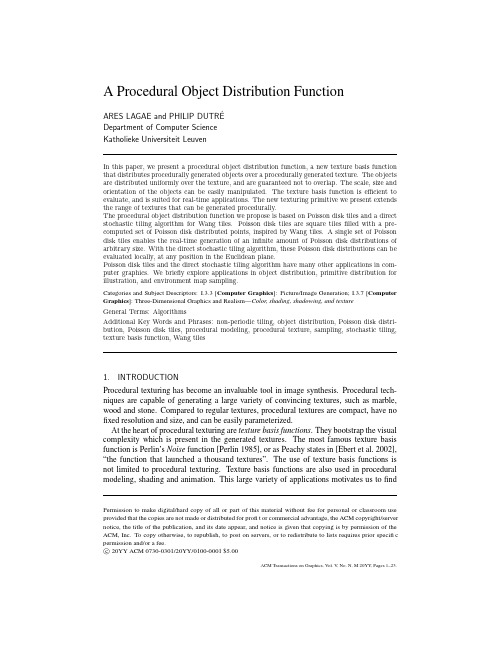
A Procedural Object Distribution FunctionARES LAGAE and PHILIP DUTR´EDepartment of Computer ScienceKatholieke Universiteit LeuvenIn this paper,we present a procedural object distribution function,a new texture basis function that distributes procedurally generated objects over a procedurally generated texture.The objects are distributed uniformly over the texture,and are guaranteed not to overlap.The scale,size and orientation of the objects can be easily manipulated.The texture basis function is efficient to evaluate,and is suited for real-time applications.The new texturing primitive we present extends the range of textures that can be generated procedurally.The procedural object distribution function we propose is based on Poisson disk tiles and a direct stochastic tiling algorithm for Wang tiles.Poisson disk tiles are square tilesfilled with a pre-computed set of Poisson disk distributed points,inspired by Wang tiles.A single set of Poisson disk tiles enables the real-time generation of an infinite amount of Poisson disk distributions of arbitrary size.With the direct stochastic tiling algorithm,these Poisson disk distributions can be evaluated locally,at any position in the Euclidean plane.Poisson disk tiles and the direct stochastic tiling algorithm have many other applications in com-puter graphics.We briefly explore applications in object distribution,primitive distribution for illustration,and environment map sampling.Categories and Subject Descriptors:I.3.3[Computer Graphics]:Picture/Image Generation;I.3.7[Computer Graphics]:Three-Dimensional Graphics and Realism—Color,shading,shadowing,and textureGeneral Terms:AlgorithmsAdditional Key Words and Phrases:non-periodic tiling,object distribution,Poisson disk distri-bution,Poisson disk tiles,procedural modeling,procedural texture,sampling,stochastic tiling, texture basis function,Wang tiles1.INTRODUCTIONProcedural texturing has become an invaluable tool in image synthesis.Procedural tech-niques are capable of generating a large variety of convincing textures,such as marble, wood and pared to regular textures,procedural textures are compact,have no fixed resolution and size,and can be easily parameterized.At the heart of procedural texturing are texture basis functions.They bootstrap the visual complexity which is present in the generated textures.The most famous texture basis function is Perlin’s Noise function[Perlin1985],or as Peachy states in[Ebert et al.2002],“the function that launched a thousand textures”.The use of texture basis functions is not limited to procedural texturing.Texture basis functions are also used in procedural modeling,shading and animation.This large variety of applications motivates us tofind Permission to make digital/hard copy of all or part of this material without fee for personal or classroom use provided that the copies are not made or distributed for profit or commercial advantage,the ACM copyright/server notice,the title of the publication,and its date appear,and notice is given that copying is by permission of the ACM,Inc.To copy otherwise,to republish,to post on servers,or to redistribute to lists requires prior specific permission and/or a fee.c 20YY ACM0730-0301/20YY/0100-0001$5.00ACM Transactions on Graphics,V ol.V,No.N,M20YY,Pages1–23.2· gae and Ph.Dutr´eFig.1.Procedural textures generated with the new texture basis function.new texture basis functions.In this paper,we present a procedural object distribution function.This new texture basis function distributes procedurally generated objects over a procedurally generated texture, which serves as background.Objects are placed uniformly over the texture,and are guar-anteed not to overlap.The texture basis function allows intuitive control over the scale, size and orientation of the objects being distributed,and can be evaluated efficiently.The procedural object distribution function we present complements existing texture ba-sis functions,and extends the range of textures that can be generated procedurally.Figure 1shows several procedural textures generated with the new texturing primitive.Note that none of the existing texture basis functions is capable of generating this kind of textures. The texture basis function we propose builds upon the concept of Poisson disk tiles. Poisson disk tiles are square tiles based on Wang tiles,and arefilled with a precomputed set of Poisson disk distributed points.With a single set of Poisson disk tiles,an infinite amount of high quality Poisson disk distributions of arbitrary size can be generated in real time.We present a method for building the tiles,as well as a direct stochastic tiling algorithm,for non-periodically tiling the infinite Euclidean plane with the tiles.The direct stochastic tiling algorithm allows to locally evaluate a tiled Poisson disk distribution,at any position in the Euclidean plane.The efficient generation and local evaluation of high quality Poisson disk distributions has many other applications in computer graphics.We briefly discuss three such appli-cations:object distribution,primitive distribution for illustration,and environment map sampling.This paper is structured as follows.In section2we discuss related work.Section3 surveys techniques for generating and analyzing Poisson disk distributions.In section4, we present a direct stochastic tiling algorithm for Wang tiles.Section5discusses the construction of Poisson disk tiles.In section6,we combine Poisson disk tiles with the direct stochastic tiling algorithm,and introduce the procedural object distribution function. Section7discusses several other applications of Poisson disk tiles and the direct stochastic algorithm.In section8,we conclude and give some directions for future work.2.RELATED WORKIn this section,we discuss related work in the area of texture basis functions and Poisson disk distributions.We also give an overview of applications of tilings in computer graphics.2.1Texture Basis FunctionsThe introduction of solid texturing[Perlin1985;Peachy1985]was a milestone in thefield of procedural modeling.ACM Transactions on Graphics,V ol.V,No.N,M20YY.A Procedural Object Distribution Function·3 The most popular3D texture basis function is Perlin’s Noise function[Perlin1985;Per-lin and Hoffert1989;Perlin2002].The noise value at each point is determined by com-puting a pseudo-random gradient at each of the eight nearest vertices on the integer cubic lattice,followed by splined interpolation.Perlin’s Noise function has become the standard way to model natural materials such as marble,wood and stone,and natural phenomena such as smoke,water andfire.Another useful3D texture basis function is Worley’s cellular texture basis function [Worley1996].Random feature points are scattered throughout space,and the function returns the distance to the closest feature points.This process is accelerated using space subdivision:feature points are generated on thefly,in the cubes defined by the integer lattice.Worley’s texture basis function is suited for generating rocks,tiled areas,and a variety of organic patterns.There are several other techniques to generate textures procedurally.For example,[Turk 1991]presents a biologically inspired method,called reaction diffusion,that generates interesting mammalian patterns.These methods,however,do not qualify as texture basis functions,because they do not have the semantics of a point evaluation,but require global operations to work.For an excellent overview of thefield of procedural texturing and modeling,we refer the reader to[Ebert et al.2002].2.2Poisson Disk DistributionsIt is generally accepted that the Poisson disk distribution is one of the best sampling pattern for a wide range of applications,because of its blue noise properties[Yellot1982;1983; Dipp´e and Wold1985;Cook1986;Mitchell1987;1991;McCool and Fiume1992;Hiller et al.2001].Poisson disk distributions are traditionally generated using an expensive dart throwing algorithm[Cook1986].Fast methods that generate approximate Poisson disk distribu-tions have been suggested by various authors[Dipp´e and Wold1985;Mitchell1987;1991; Klassen2000].The algorithm mostly used nowadays is due to[McCool and Fiume1992]. It generalizes over the dart throwing approach,and uses Lloyd’s relaxation scheme[Lloyd 1982]to optimize the generated distribution.Fast methods to generate Poisson disk dis-tributions,closely related to our method,are described in[Hiller et al.2001;Cohen et al. 2003]and[Shade et al.2002].They construct points set that can be tiled over the sampling plane,while maintaining blue noise properties.[Ostromoukhov et al.2004]introduce an efficient method to generate sampling patterns with blue noise properties according to a given density.Tools to analyze the spectral properties of point sets are presented in[Ulichney1987].2.3Applications of TilingsOne of thefirst applications of tilings in computer graphics is described in[Stam1997]. Non-repeating textures of arbitrary size are created using the aperiodic set of16Wang tiles [Gr¨u nbaum and Shepard1986].The tiles arefilled with procedurally generated waves and caustics.[Neyret and Cani1999]use triangular tiles to generate a non-periodic texture over a mesh.[Hiller et al.2001]and[Shade et al.2002]use Wang tile sets counting8 tiles.[Cohen et al.2003]present a stochastic tiling procedure for non-periodically tiling the plane with a small set of Wang tiles.They show how tofill the tiles with patterns, and discuss automatic tile design for texture synthesis and object distribution.[Kaplan andACM Transactions on Graphics,V ol.V,No.N,M20YY.moved(d)set is toroidal.Salesin2000]use isohedral tilings to provide a solution to the problem of Escherization: given a closedfigure in the plane,find a new closedfigure that is similar to the original and tiles the plane.Their system creates illustrations much like the ones by the Dutch artist M.C.Escher.[Ostromoukhov et al.2004]use a hierarchical subdivided Penrose tiling [Penrose1974].[Wei2004]present a tile-based texture mapping algorithm for graphics hardware based on Wang tiles.The definite reference on tilings of all kinds is still[Gr¨u nbaum and Shepard1986].A good introductory text on aperiodic tilings is[Glassner1999,chapter12].3.GENERATION AND ANALYSIS OF POISSON DISK DISTRIBUTIONSA Poisson disk distribution is a2D uniform point distribution in which all points are sepa-rated by a minimum distance.Half that distance is called the radius of the distribution.If a disk of that radius is placed at each point,then no two disks overlap.In this section we discuss several algorithms for generating Poisson disk distributions. We also formulate a scale-invariant way to specify the radius of a Poisson disk distribution, and introduce tools to analyze Poisson disk distributions.3.1Dart Throwing,Relaxation Dart Throwing and Lloyd’s RelaxationPoisson disk distributions are traditionally generated with a dart throwing algorithm[Cook 1986].The algorithm generates uniformly distributed points,and rejects points that do not satisfy the minimum separation with already generated points.This process continues until no more points can be added.This algorithm is expensive,and difficult to control:instead of specifying the number of points,the radius of the distribution has to be provided,the final number of points in the distribution is difficult to predict,and if the process is stopped too soon,the density of the points is not uniform.[McCool and Fiume1992]propose an improved version of the dart throwing algorithm, which we call relaxation dart throwing.Points are placed with a large radius initially,and once no more space has been found for a large number of attempts,the radius is reduced by some fraction.This algorithm has several advantages compared to dart throwing:it is faster,it allows to specify the desired number of points rather than the radius,and ter-mination is guaranteed.The Poisson disk distributions generated by dart throwing and relaxation dart throwing are usually toroidal.After a Poisson disk distribution is generated,[McCool and Fiume1992]apply Lloyd’s relaxation[Lloyd1982].Lloyd’s relaxation is an iterative process:in each iteration,the ACM Transactions on Graphics,V ol.V,No.N,M20YY.A Procedural Object Distribution Function·5 V oronoi diagram of the point set is computed,and each point is moved to the centroid of its V oronoi cell.This process is illustrated infigure2.3.2Radius SpecificationExpressing the radius of a Poisson disk distribution as a raw number is impractical.Instead, we propose the following.The densest packing of circles in the plane is a hexagonal lattice.Therefore,the point configuration with maximum disk radius r max is a hexagonal lattice.One can easily verify that,for N points distributed over the unit square,r max= 2√3N −1/2.(1) The radius r of a Poisson disk distribution can now be written asr=αr max,(2) withα∈[0...1].An uniformly distributed point set has anαvalue of0,and theαvalue of a hexagonal lattice equals1.Poisson disk distributions should haveαvalues that are large(α≥0.6),but not too large (α≤0.9),because regular configurations must be avoided.3.3AnalysisThe frequency domain characteristics of point distributions of are analyzed using Fourier techniques[Ulichney1987].The periodogram of a point distribution of N points{x0,...,x N−1}⊂[0,1)2estimates the power spectrum of the distribution,and is given byR f( ω)= F1N N−1∑j=0δ(x−x j)2,(3)where F denotes the Fourier transform andδis Dirac’s delta function.The periodogram of a Poisson disk distribution is radially symmetric.Therefore,two one-dimensional statistics are derived from the periodogram.Thefirst one is the radially averaged power spectrumP i=1S i2πf i+1f iR f(f cosθ,f sinθ)f d f dθ,(4)which measures the mean radial power in a set of concentric annuli defined by the fre-quency intervals[f i,f i+1).S i is the area of annulus i,and is given byπ f2i+1−f2i .The second statistic is the anisotropy A i=s2i/P2i of each ring,wheres2i=1S i2πf i+1f i R f(f cosθ,f sinθ)−P i2f d f dθ.(5)The anisotropy is a measure for the radial symmetry of the distribution of power.Figure10(a)shows the analysis of a Poisson disk distribution generated with relaxation dart throwing and Lloyd’s relaxation.The radially averaged power spectrum of a Poisson disk distribution has a very specific structure.The DC peak is followed by a low-energy region.This region is followed by a sharp transition and a low-frequency cutoff at the frequency corresponding to the Poisson disk radius,followed by aflat high frequencyACM Transactions on Graphics,V ol.V,No.N,M20YY.6· gae and Ph.Dutr´eFig.3.A Wang tile set of16tiles over2colors.The8-tile Wang tile set over two colors used by[Cohen et al. 2003]consists of the tiles with their number circled.Fig.4.A3×5Wang tiling.This tiling uses the tile set shown infigure3.region,in which most energy is contained.Radius statistics and periodograms are the primary means for evaluating the quality of Poisson disk distributions.Note that the values in the periodogram have a range of several orders of magnitude. Therefore the periodogram images are tone mapped,and the radially averaged power spec-trum and anisotropy graphs use a logarithmic scale.4.A DIRECT STOCHASTIC TILING ALGORITHM FOR WANG TILESIn this section we introduce Wang tiles,and we present a direct stochastic tiling algorithm, a new tiling method that allows to evaluate a Wang tiling instantly,at any point in the Euclidean plane.4.1Wang TilesTo tile means to cover the infinite Euclidean plane with a set of polygons so that there are no gaps or overlaps.The set of polygons is called the tile set,and each polygon is called a tile.The resulting composite pattern is called the tiling.Tiling rules describe how tiles are placed next to each other.A tiling is periodic if a translation exists that preserves the tiling.If this is not the case,the tiling is non periodic.An aperiodic tile set is a tile set that does not admit a periodic tiling.Wang tiles are named after Hao Wang,who stated in1961that if a set of tiles tiled the plane,then they could always be arranged to do so periodically[Wang1961].His con-jecture was later refuted by Berger,who constructed thefirst aperiodic tile set,containing 20426tiles[Berger1966].This number was reduced repeatedly,and in1974,Penrose discovered an aperiodic set of only two tiles[Penrose1974].The smallest set of aperiodic Wang tiles counts13tiles[Culik1996;Kari1996].Wang tiles[Wang1961;1965]are unit square tiles with colored edges.A tile set is a ACM Transactions on Graphics,V ol.V,No.N,M20YY.A Procedural Object Distribution Function·7finite set of Wang tiles.We consider tilings of the infinite Euclidean plane using arbitrary many copies of the tiles in the tile set.The tiles are placed with their corners on the integer lattice points.They cannot be rotated nor reflected,and adjoining edges must have matching colors.By convention,the coordinates of a tile are the coordinates of the integer lattice point corresponding to the lower left corner of the tile.Figure3shows a Wang tile set of16tiles over two colors.Horizontal and vertical edges each use exactly two colors.This tile set is complete,it contains all possible tiles that can be created using two colors for horizontal and vertical edges.Figure4shows a3×5tiling created using this tile set.For Wang tiles,aperiodic tile sets are mainly of theoretical interest.Most applications use non-periodic tilings produced by tile sets that are not aperiodic.As we shall see,the tile set is often determined by the tiling procedure.4.2Scanline Stochastic Tiling[Cohen et al.2003]present a stochastic tiling procedure,which we call scanline stochastic tiling.Tiles are placed in scanline order,from West to East,and from North to South.A random tile is selected for the NW corner.Thefirst row is completed by adding tiles for which the color of the W edge corresponds to the color of the E edge of the tile to the left.The leading tile of each new row is selected so that its N edge matches the S edge of the tile above.The row is completed by choosing tiles for which the N and W edges match the S and E edges from the tiles above and to the left.The tile set is constructed such that there are two tiles for each NW combination.Each time a tile has to be selected,the choice is made at random.This ensures a non-periodic tiling.A tile set over K colors will contain2K2tiles,since there are K2NW combinations.4.3Direct Stochastic TilingThe scanline stochastic tiling algorithm works well,but is not sufficient for our needs. Because texture basis functions have the semantics of a point evaluation,we need to be able to evaluate the tiling locally.This means computing which tile is at a given tile coordinate, in constant time,without explicitly constructing the tiling up to that point.The direct stochastic tiling algorithm we propose is based on the observation that it is impossible to obtain a valid tiling of the plane by placing a random tile at each tileACM Transactions on Graphics,V ol.V,No.N,M20YY.8· gae and Ph.Dutr´ecoordinate.However,a valid tiling can be generated by placing randomly colored edges between integer lattice points.For each tile corner,a pair of random colors c h i,c v i ∈Z2K(i∈{NE,SE,SW,NW})is generated.Thefirst color will be used for horizontal edges,the second one for vertical edges.Edge colors are computed as the sum(modulo K)of the random colors associated with their corners.For example,the color of the N edge is computed as c N=c h NW+c h NE. This process is illustrated infigure5.A pair of random colors at a corner is generated by applying a hash function to the integer lattice coordinates of that corner.This tiling procedure results in a valid tiling:adjoining edges have matching colors,since their colors are based on identical hash values.Because edge colors are chosen at random,the tiling is also non periodic.The color of each edge is generated independently of other edges,thus a tile set over K colors will contain K4tiles.The direct stochastic tiling procedure can easily be adapted for tile sets that use a differ-ent number of colors for horizontal and vertical edges,K h and K v.Now,pairs of random colors c h i,c v i ∈Z K h×Z K v are generated.Edge computations for horizontal and vertical edges are executed modulo K h and modulo K v.A tile set over K h and K v colors will now contain K h K v 2tiles.We also give a direct stochastic tiling procedure for Cohen’s8-tile Wang tile set(K=2) [Cohen et al.2003].A single random color in Z2is generated for each corner,and edge colors are computed as the sum(modulo2)of the random colors associated with their corners.Obviously,there are4NW combinations.The E edge brings the total number of combinations to8,because the S edge is completely determined by the other three.The direct stochastic tiling algorithm can be implemented very efficiently,especially if the hash function is based on a permutation table[Perlin2002;Ebert et al.2002],and is suited for real-time applications.A tiling algorithm similar to the one presented here is described in[Wei2004].5.POISSON DISK TILESPoisson disk distributions are clearly expensive to generate.That is why[Dipp´e and Wold 1985]already in1985suggested to replicate a precomputed tile with Poisson disk dis-tributed points across the plane.Tilings are indeed the key to efficient techniques for generating Poisson disk distributions.However,constructing a Poisson disk distribution over a set of tiles is challenging.The difficulty is to generate a Poisson disk distribution in each tile of the tile set such that every tiling of the infinite Euclidean plane results in valid Poisson disk distribution.In this section,we present Poisson disk tiles,our solution to the problem,and discuss previous approaches in detail.5.1Previous ApproachesTwo approaches exist for constructing a Poisson disk distribution over a set of tiles:one based on dart throwing and one based on Lloyd’s relaxation.Atfirst sight,the dart throwing approach seems to extend naturally to Wang tiles:before a point is added to a tile,all possible neighboring tiles are checked,and the point is rejected if the minimum separation criterion is not met.However,as noted by[Shade et al.2002; Cohen et al.2003],this approach isflawed.The constraints of multiple tiles cause less points to be inserted near the edges and corners.This results in a noticeable lower density ACM Transactions on Graphics,V ol.V,No.N,M20YY.(a)(b)(c)(d)Fig.6.The tile regions.(a)The Poisson disk radius determines different regions in a Wang tile.Red points belong to corner regions and blue points belong to edge regions.Green points do not affect points in other tiles.(b)A point in an edge region also constrains points in other edge regions of the same tile.(c)Corner regions are enlarged to eliminate these constraints.(d)Now points in edge regions no longer affect points in other edge regions of the same tile.of points in those regions.The problem of multiple constraints is more severe than it might seem atfirst sight:placing a point near the corner of one tile makes it impossible,for at least one corner of every other tile in the tile set,to have a point nearby![Hiller et al.2001]present an approach based on Lloyd’s relaxation.They use a set of8Wang tiles.An initial point set is generated in every tile.Each tile in the set is then surrounded by all possible configurations of8tiles.For all of these configurations,a V oronoi diagram is constructed.Each V oronoi diagram determines a displacement vector for every point in the tile.All displacement vectors are averaged,and the points in the tile are moved accordingly.This process is iterated,until the point distributions stabilize.Note that this method is also used in[Cohen et al.2003].Although this algorithm is a sensible generalization of Lloyd’s relaxation,it has some limitations.It is more or less a brute force approach,and its convergence properties are not well studied.A tile set of8tiles is rather small to generate tiled Poisson disk distributions with good spectral properties[Lagae and Dutr´e2005],but the algorithm does not handle larger tile sets very well:the displacement vectors tend to average each other out.Also,it seems to be difficult to generate a tile set with a large radius,which is necessary for the procedural object distribution function. 5.2Poisson Disk TilesWe now present Poisson disk tiles,our solution to the problem of constructing a Poisson disk distribution over a set of tiles.A point in a Wang tile,closer to an edge than r,the Poisson disk radius,constrains points in one neighboring tile,and a point closer to a corner than r,affects points in three neighboring tiles.These critical corner regions and edge regions are shown infigures 6(a)and6(b).To minimize the constraints between different regions,we extend the corner regions as illustrated infigures6(c)and6(d).Now points in edge regions only affect points in corner regions and vice versa.When interpreted as markings on a set of16Wang tiles over2colors,the tile regions give rise to a new kind of tiling,illustrated infigure7,which we call the dual Poisson disk tiling.If we discard the whitespace,there are two different kind of tiles:corner tiles and edge tiles.Edge tiles correspond to the union of edge regions of neighboring tiles.Therefore, the tile set contains4edge tiles:a horizontal and vertical one for each color.Corner tiles correspond to the union of the corner regions of four neighboring tiles.Consequently,theACM Transactions on Graphics,V ol.V,No.N,M20YY.10· gae and Ph.Dutr´eFig.7.The dual Poisson disk tiling.This tiling is suggested by the tile regions shown infigure6.As indicated in the center,Poisson disk tiles can be cut out of this tiling.Note that this tiling is based on the Wang tiling shown infigure4.tile set contains16kinds of corner tiles:one for each combination of two horizontal and two vertical edge tiles.A Poisson disk distribution is generated over the dual Poisson disk tiling,and then the Poisson disk tiles are cut from the dual tiling,as illustrated infigure7.We start by choosing N,the number of points per Poisson disk tile,andα,which determines the radius of the Poisson disk distribution.Note that the size of the edge tiles and corner tiles is determined by the radius of the Poisson disk distribution.The edge tiles are constructedfirst(seefigure8(a))For each edge tile,a toroidal Pois-son disk distribution of N points is generated using relaxation dart throwing followed by Lloyd’s relaxation.The edge tile is then cut out of the distribution.If the desired Poisson disk radius is not reached,this process is repeated.The corner tiles can now be constructed by surrounding each corner tile with the corre-sponding edge tiles(seefigure8(c)).Again,a toroidal Poisson disk distribution is gener-ated using relaxation dart throwing followed by Lloyd’s relaxation.The points of the edge tiles are not affected by this process:no new points are added to the edge tiles,and during relaxation,points in the edge tiles arefixed,and other points are prohibited to enter the edge tiles.This is done by clipping displacement vectors of points that are about to enter the edge tiles.Finally,the Poisson disk tiles are constructed(seefigure8(e)).This is done by generat-ing a Poisson disk distribution in the empty space between four corner tiles and edge tiles, and then cutting out the Poisson disk tile.The number of points that is added is chosen to bring the total number of points in the tile to N.Throughout this process,the edge tiles and corner tiles are locked,and to ensure an uniform point density,the tile is embedded in a larger toroidal Poisson disk distribution during relaxation.Figure8illustrates the construction of edge tiles,corner tiles and Poisson disk tiles.It also shows the entire set of edge tiles and corner tiles of a dual Poisson disk tiling based on a complete Wang tile set over two colors,and several Poisson disk tiles.Figure9shows a Poisson disk tiling using this set of Poisson disk tiles.A Poisson disk tile set based on a complete Wang tile set over K colors,will contain K4(K2)4tiles.For K=2,this comes down to4096tiles.Although this might seem like a lot,a tile set with N=32points per tile only occupies about1MB of storage.The time needed to generate a Poisson disk tile set ranges from several minutes to several hours, depending on N andα.However,the construction of a tile set has to be done only once. ACM Transactions on Graphics,V ol.V,No.N,M20YY.。
八年级英语议论文论证方法单选题40题
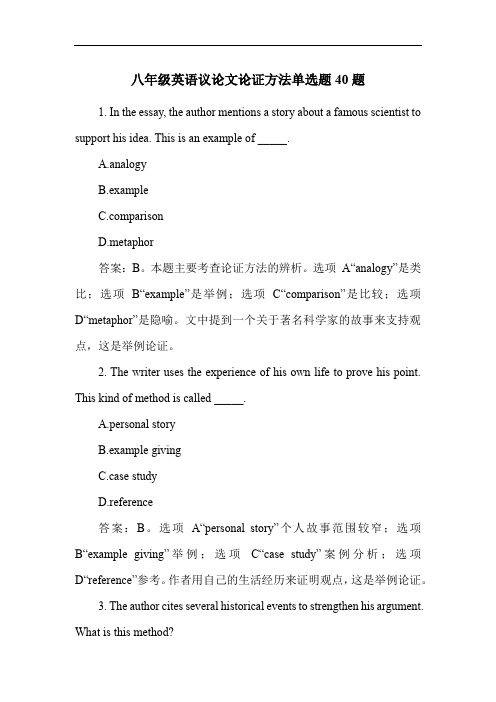
八年级英语议论文论证方法单选题40题1. In the essay, the author mentions a story about a famous scientist to support his idea. This is an example of _____.A.analogyB.exampleparisonD.metaphor答案:B。
本题主要考查论证方法的辨析。
选项A“analogy”是类比;选项B“example”是举例;选项C“comparison”是比较;选项D“metaphor”是隐喻。
文中提到一个关于著名科学家的故事来支持观点,这是举例论证。
2. The writer uses the experience of his own life to prove his point. This kind of method is called _____.A.personal storyB.example givingC.case studyD.reference答案:B。
选项A“personal story”个人故事范围较窄;选项B“example giving”举例;选项C“case study”案例分析;选项D“reference”参考。
作者用自己的生活经历来证明观点,这是举例论证。
3. The author cites several historical events to strengthen his argument. What is this method?A.citing factsB.giving examplesC.making comparisonsing analogies答案:B。
选项A“citing facts”引用事实,历史事件可以作为例子,所以是举例论证;选项B“giving examples”举例;选项C“making comparisons”比较;选项D“using analogies”使用类比。
英语作文科学证据分析
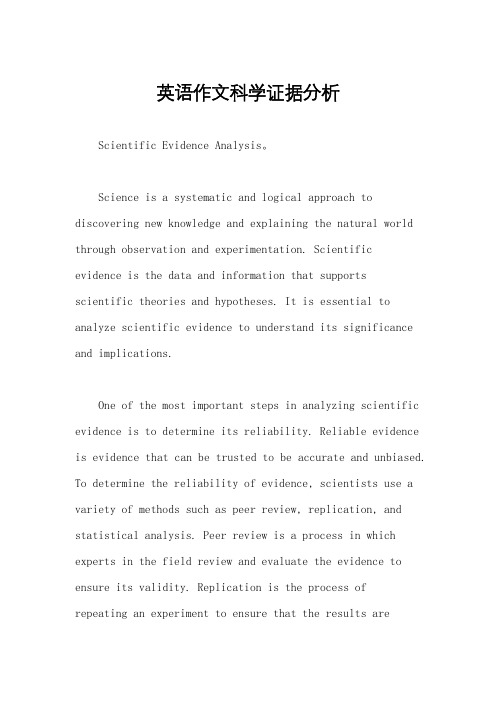
英语作文科学证据分析Scientific Evidence Analysis。
Science is a systematic and logical approach to discovering new knowledge and explaining the natural world through observation and experimentation. Scientificevidence is the data and information that supportsscientific theories and hypotheses. It is essential to analyze scientific evidence to understand its significance and implications.One of the most important steps in analyzing scientific evidence is to determine its reliability. Reliable evidence is evidence that can be trusted to be accurate and unbiased. To determine the reliability of evidence, scientists use a variety of methods such as peer review, replication, and statistical analysis. Peer review is a process in which experts in the field review and evaluate the evidence to ensure its validity. Replication is the process ofrepeating an experiment to ensure that the results areconsistent and reliable. Statistical analysis is used to determine the probability that the evidence is accurate and not due to chance.Another important step in analyzing scientific evidence is to evaluate its significance. Significant evidence is evidence that has a meaningful impact on our understanding of the natural world. To evaluate the significance of evidence, scientists consider factors such as the scope of the evidence, its relevance to current theories, and its potential for future research. They also consider the potential implications of the evidence for society and the environment.Finally, scientists must communicate their findings to the scientific community and the general public. This involves presenting the evidence in a clear and concise manner, using appropriate scientific language and terminology. Scientists must also be transparent abouttheir methods and data, and be open to criticism and feedback.In conclusion, analyzing scientific evidence is acrucial step in the scientific process. It allowsscientists to determine the reliability and significance of their findings, and communicate their discoveries to others. By using rigorous methods and being transparent about their work, scientists can ensure that their evidence is accurate and meaningful, and contributes to our understanding of the natural world.。
gsas 精修

Talk Emphasis
• Solving relevant problems in the various sciences such as the geosciences may require an intimate and non-routine knowledge of possible analytical techniques and their use • You may have to obtain more information that you initially wante to know. • In this case:
Slide 7
Using diffraction methods to solve the problems of the world Lachlan M. D. Cranswick (l.m.d.cranswick@)
Applications of Le Bail fitting
Slide 9 Using diffraction methods to solve the problems of the world Lachlan M. D. Cranswick (l.m.d.cranswick@)
Invention of Le Bail fitting and Le Bail Extraction
"Beyond Classical Rietveld Analysis - using Le Bail fitting of X-ray Powder Diffraction data to help answer the questions of the world:
can the Earth's outer core contain Oxygen?"
杰尼奥公司的Raman光谱仪使用培训课程说明书
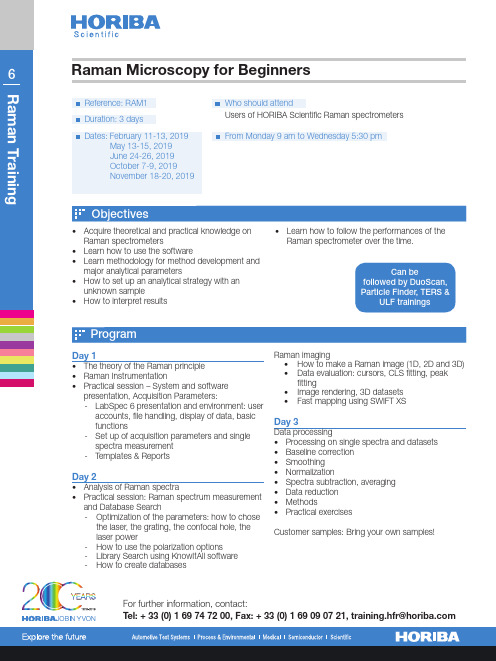
6Who should attendFrom Monday 9 am to Wednesday 5:30 pmDates: February 11-13, 2019 May 13-15, 2019 June 24-26, 2019 October 7-9, 2019November 18-20, 2019Users of HORIBA Scientific Raman spectrometers • A cquire theoretical and practical knowledge on Raman spectrometers • L earn how to use the software • L earn methodology for method development and major analytical parameters • H ow to set up an analytical strategy with an unknown sample • H ow to interpret results• L earn how to follow the performances of theRaman spectrometer over the time.Day 1• The theory of the Raman principle • R aman Instrumentation • P ractical session – System and software presentation, Acquisition Parameters: - L abSpec 6 presentation and environment: useraccounts, file handling, display of data, basic functions - S et up of acquisition parameters and singlespectra measurement - Templates & ReportsDay 2• Analysis of Raman spectra • P ractical session: Raman spectrum measurement and Database Search - O ptimization of the parameters: how to chosethe laser, the grating, the confocal hole, the laser power- How to use the polarization options - Library Search using KnowItAll software - How to create databasesRaman imaging • H ow to make a Raman image (1D, 2D and 3D) • D ata evaluation: cursors, CLS fitting, peakfitting•Image rendering, 3D datasets •Fast mapping using SWIFT XSDay 3Data processing• Processing on single spectra and datasets • Baseline correction • Smoothing • Normalization• Spectra subtraction, averaging • Data reduction • Methods• Practical exercisesCustomer samples: Bring your own samples!Duration: 3 daysReference: RAM1Raman Microscopy for Beginners7Acquire technical skills on DuoScan, Ultra Low Frequency (ULF), Particle Finder or TERS.Users of HORIBA Scientific Raman spectrometers who already understand the fundamentals of Raman spectroscopy and know how to use HORIBA Raman system and LabSpec Software. It is advised to participate in the basic Raman training first (RAM1).Introduction to DuoScan• Principle and hardwareDuoScan Macrospot• Practical examplesDuoScan MacroMapping• Practical examplesDuoScan Stepping Mode• Practical examplesCustomer samples: Bring your own samples!Presentation of the ULF kit• Principle and requirements • Application examplesInstallation of the ULF kitIntroduction to Particle Finder• Principle and requirementsPractical session• Demo with known sample• Customer samples: Bring your own samples!Practical session• Demo with known samplesCustomers samples: Bring your own samples! Presentation of the TERS technique• Principle and requirements • Application examplesDemo TERS• Presentation of the different tips and SPM modes • Laser alignment on the tip • T ERS spectra and TERS imaging on known samplesPractical session• Hands-on on demo samples (AFM mode)• Laser alignment on the tip • T ERS spectra and TERS imaging on known samplesRaman Options: DuoScan, Ultra Low Frequency, Particle Finder, TERS8Users of HORIBA Scientific Raman spectrometers who already understand the fundamentals of Raman spectroscopy and know how to use HORIBA Raman system and labSpec Software. It is adviced to participate in the basic Raman training first.Who should attendDates: February 14, 2019 June 27, 2019November 21, 2019Duration: 1 dayReference: RAM2From 9 am to 5:30 pm• Acquire theoretical and practical knowledge on SERS (Surface Enhanced Raman Spectroscopy)• Know how to select your substrate • Interpret resultsRaman SERSIntroduction to SERSPresentation of the SERS technique • Introduction: Why SERS?• What is SERS?• Surface Enhanced Raman basics • SERS substratesIntroduction to the SERS applications• Examples of SERS applications • Practical advice • SERS limitsDemo on known samplesCustomer samples: Bring your own samples!Raman Multivariate Analysis9Users of HORIBA Scientific Raman spectrometerswho already understand the fundamentals of Ramanspectroscopy and know how to use HORIBA Ramansystem and LabSpec Software. It is advised toparticipate in the basic Raman training first (RAM1).• Understand the Multivariate Analysis module• Learn how to use Multivariate Analysis for data treatment• Perform real case examples of data analysis on demo and customer dataIntroduction to Multivariate Analysis• Univariate vs. Multivariate analysis• Introduction to the main algorithms: decomposition (PCA and MCR), classification and quantification (PLS)Practical work on known datasets (mapping)• CLS, PCA, MCRIntroduction to classification• HCA, k-means• Demo with known datasetsIntroduction to Solo+MIA• Presentation of Solo+MIA Array• Demo with known datasetsData evaluation: cursors, CLS fitting, peak fitting• Fast mapping using SWIFT XSObjective: Being able to select the good parameters for Raman imaging and to perform data processScanning Probe Microscopy (SPM)• Instrumentation• T he different modes (AFM, STM, Tuning Fork) and signals (Topography, Phase, KPFM, C-AFM, MFM,PFM)Practical session• Tips and sample installation• Molecular resolution in AFM tapping mode• M easurements in AC mode, contact mode, I-top mode, KPFM• P resentation of the dedicated tips and additional equipment• O bjective: Being able to use the main AFM modes and optimize the parametersimaging)Practical session• Hands-on on demo samples (AFM mode)• Laser alignment on the tip• T ERS spectra and TERS imaging on known sample Day 3TERS Hands-on• T ERS measurements, from AFM-TERS tip installation to TERS mapping.• TERS measurements on end users samples.• Bring your own samples!28Practical informationCourses range from basic to advanced levels and are taught by application experts. The theoretical sessions aim to provide a thorough background in the basic principles and techniques. The practical sessions are directed at giving you hands-on experience and instructions concerning the use of your instrument, data analysis and software. We encourage users to raise any issues specific to their application. At the end of each course a certificate of participation is awarded.Standard, customized and on-site training courses are available in France, G ermany, USA and also at your location.Dates mentionned here are only available for HORIBA France training center.RegistrationFill in the form and:• Emailitto:***********************• Or Fax it to: +33 (0)1 69 09 07 21• More information: Tel: +33 (0)1 69 74 72 00General InformationThe invoice is sent at the end of the training.A certificate of participation is also given at the end of the training.We can help you book hotel accommodations. Following your registration, you will receive a package including training details and course venue map. We will help with invitation letters for visas, but HORIBA FRANCE is not responsible for any visa refusal. PricingRefreshments, lunches during training and handbook are included.Hotel transportation, accommodation and evening meals are not included.LocationDepending on the technique, there are three locations: Longjumeau (France, 20 km from Paris), Palaiseau (France, 26 km from Paris), Villeneuve d’Ascq (France 220 km from Paris) or at your facility for on-site training courses. Training courses can also take place in subsidiaries in Germany or in the USA.Access to HORIBA FRANCE, Longjumeau HORIBA FRANCE SAS16 - 18 rue du canal91165 Longjumeau - FRANCEDepending on your means of transport, some useful information:- if you are arriving by car, we are situated near the highways A6 and A10 and the main road N20- if you are arriving by plane or train, you can take the train RER B or RER C that will take you not far from our offices. (Around 15 €, 150 € by taxi from Charles de Gaulle airport, 50 € from Orly airport).We remain at your disposal for any information to access to your training place. You can also have a look at our web site at the following link:/scientific/contact-us/france/visi-tors-guide/Access to HORIBA FRANCE, Palaiseau HORIBA FRANCE SASPassage Jobin Yvon, Avenue de la Vauve,91120 Palaiseau - FRANCEFrom Roissy Charles de Gaulle Airport By Train • T ake the train called RER B (direction Saint RemyLes Chevreuse) and stop at Massy-Palaiseaustation• A t Massy-Palaiseau station, take the Bus 91-06C or 91-10 and stop at Fresnel• T he company is a 5 minute walk from the station,on your left, turn around the traffic circle and youwill see the HORIBA building29 Practical InformationAround 150 € by taxi from Charles de Gaulle airport. From Orly Airport By Train• A t Orly airport, take the ORLYVAL, which is ametro line that links the Orly airport to the AntonyRER station• A t Antony station, take the RER B (direction StRemy Les Chevreuse) and stops at Massy-Palai-seau station• A t Massy-Palaiseau station, take the Bus 91-06C, 91-06 B or 91-10 stop at Fresnel• T he company is 5 minutes walk from the station,on your left, turn around the traffic circle and youwill see the HORIBA building• O r at Orly take the Bus 91-10 stop at Fresnel.The company is 5 minutes walk from the station,on your left, turn around the traffic circle and youwill see the HORIBA building. We remain at yourdisposal for any information to access to your trainingplace. You can also have a look at our web site at thefollowing link:/scientific/contact-us/france/visi-tors-guide/Around 50 € by taxi from Orly airport.Access to HORIBA FRANCE, Villeneuve d’Ascq HORIBA Jobin Yvon SAS231 rue de Lille,59650 Villeneuve d’Ascq - FRANCEBy Road from ParisWhen entering Lille, after the exit «Aéroport de Lequin», take the direction «Bruxelles, Gand, Roubaix». Immmediatly take the direction «Gand / Roubaix» (N227) and No «Bruxelles» (A27) Nor «Valenciennes» (A23).You will then arrive on the ringroad around Villeneuve d’Ascq. Take the third exit «Pont de Bois».At the traffic light turn right and follow the road around, (the road will bend left then right). About 20m further on you will see the company on the right hand side where you can enter the car park.By Road from Belgium (GAND - GENT)Once in France, follow the motorway towards Lille. After «Tourcoing / Marcq-en-Baroeul», follow on the right hand side for Villeneuve d’Ascq. Take the exit «Flers Chateau» (This is marked exit 6 and later exit 5 - but it is the same exit). (You will now be following a road parallel to the mo-torway) Stay in the middle lane and go past two sets of traffic lights; at the third set of lighte, move into the left hand lane to turn under the motorway.At the traffic lights under the motorway go straight, (the road shall bend left then right). About 20 m further you shall see the company on the right hand side where you can enter the car park.AeroplaneFrom the airport Charles de Gaulle take the direction ‘Ter-minal 2’ which is also marked TGV (high speed train); where you can take the train to ‘Lille Europe’.Train - SNCFThere are two train stations in Lille - Lille Europe or Lille Flandres. Once you have arrived at the station in Lille you can take a taxi for HORIBA Jobin Yvon S.A.S., or you can take the underground. Please note both train stations have stations for the underground.Follow the signs:1. From the station «Lille Flandres», take line 1, direction «4 Cantons» and get off at the station «Pont de bois».2. From the station «Lille Europe», take line 2, direction «St Philibert» and get off at the following station «Gare Lille Flandres» then take line 1, direction «4 Cantons» and get off at the station «Pont de Bois».BusBus n°43, direction «Hôtel de Ville de Villeneuve d’Ascq», arrêt «Baudoin IX».InformationRegistration: Fill inthe form and send it back by FAX or Email four weeks before beginning of the training.Registration fees: the registration fees include the training courses and documentation. Hotel, transportation and living expenses are not included except lunches which are taken in the HORIBA Scientific Restaurant during the training.Your contact: HORIBA FRANCE SAS, 16-18 rue du Canal, 91165 Longjumeau, FRANCE Tel: + 33 1 64 74 72 00Fax: + 33 1 69 09 07 21E-Mail:***********************Siret Number: 837 150 366 00024Certified ISO 14001 in 2009, HORIBA Scientific is engaged in the monitoring of the environmental impact of its activitiesduring the development, manufacture, sales, installation and service of scientific instruments and optical components. Trainingcourses include safety and environmental precautions for the use of the instrumentsHORIBA Scientific continues contributing to the preservation of theglobal environment through analysis and measuring technologymentisnotcontractuallybindingunderanycircumstances-PrintedinFrance-©HORIBAJobinYvon1/219。
Using Analytic QP and Sparseness to Speed Training of Support VectorMachines
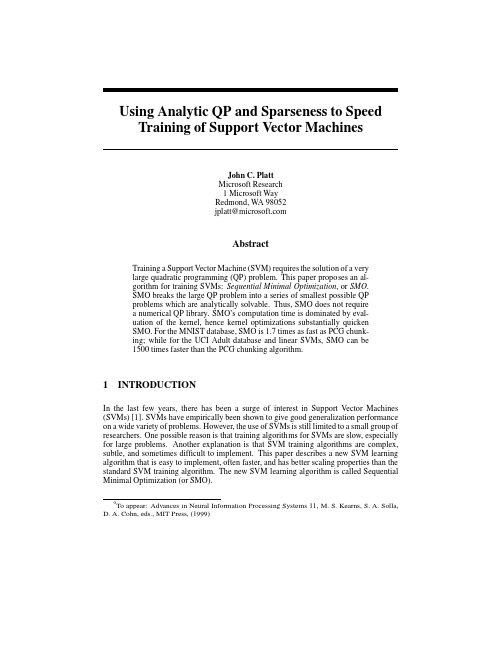
John C.PlattMicrosoft Research1Microsoft WayRedmond,W A98052jplatt@AbstractTraining a Support Vector Machine(SVM)requires the solution of a verylarge quadratic programming(QP)problem.This paper proposes an al-gorithm for training SVMs:Sequential Minimal Optimization,or SMO.SMO breaks the large QP problem into a series of smallest possible QPproblems which are analytically solvable.Thus,SMO does not requirea numerical QP library.SMO’s computation time is dominated by eval-uation of the kernel,hence kernel optimizations substantially quickenSMO.For the MNIST database,SMO is1.7times as fast as PCG chunk-ing;while for the UCI Adult database and linear SVMs,SMO can be1500times faster than the PCG chunking algorithm.1INTRODUCTIONIn the last few years,there has been a surge of interest in Support Vector Machines (SVMs)[1].SVMs have empirically been shown to give good generalization performance on a wide variety of problems.However,the use of SVMs is still limited to a small group of researchers.One possible reason is that training algorithms for SVMs are slow,especially for large problems.Another explanation is that SVM training algorithms are complex, subtle,and sometimes difficult to implement.This paper describes a new SVM learning algorithm that is easy to implement,often faster,and has better scaling properties than the standard SVM training algorithm.The new SVM learning algorithm is called Sequential Minimal Optimization(or SMO).1.1OVERVIEW OF SUPPORT VECTOR MACHINESA general non-linear SVM can be expressed as(1) where is the output of the SVM,is a kernel function which measures the similarity of a stored training example to the input,is the desired output of the classifier,is a threshold,and are weights which blend the different kernels[1].For linear SVMs,the kernel function is linear,hence equation(1)can be expressed as(2) where.Training of an SVM consists offinding the.The training is expressed as a minimization of a dual quadratic form:cannot handle large-scale training problems,because even this reduced matrix cannotfit into memory.Kaufman[3]has described a QP algorithm that does not require the storage of the entire Hessian.The decomposition technique[6]is similar to chunking:decomposition breaks the large QP problem into smaller QP sub-problems.However,Osuna et al.[6]suggest keeping a fixed size matrix for every sub-problem,deleting some examples and adding others which violate the KKT ing afixed-size matrix allows SVMs to be trained on very large training sets.Joachims[2]suggests adding and subtracting examples according to heuristics for rapid convergence.However,until SMO,decomposition required the use of a numerical QP library,which can be costly or slow.2SEQUENTIAL MINIMAL OPTIMIZATIONSequential Minimal Optimization quickly solves the SVM QP problem without using nu-merical QP optimization steps at all.SMO decomposes the overall QP problem intofixed-size QP sub-problems,similar to the decomposition method[7].Unlike previous methods,however,SMO chooses to solve the smallest possible optimiza-tion problem at each step.For the standard SVM,the smallest possible optimization prob-lem involves two elements of because the must obey one linear equality constraint.At each step,SMO chooses two to jointly optimize,finds the optimal values for these, and updates the SVM to reflect these new values.The advantage of SMO lies in the fact that solving for two can be done analytically. Thus,numerical QP optimization is avoided entirely.The inner loop of the algorithm can be expressed in a short amount of C code,rather than invoking an entire QP library routine. By avoiding numerical QP,the computation time is shifted from QP to kernel evaluation. Kernel evaluation time can be dramatically reduced in certain common situations,e.g., when a linear SVM is used,or when the input data is sparse(mostly zero).The result of kernel evaluations can also be cached in memory[1].There are two components to SMO:an analytic method for solving for the two,and a heuristic for choosing which multipliers to optimize.Pseudo-code for the SMO algo-rithm can be found in[8,7],along with the relationship to other optimization and machine learning algorithms.2.1SOLVING FOR TWO LAGRANGE MULTIPLIERSTo solve for the two Lagrange multipliers and,SMOfirst computes the constraints on these multipliers and then solves for the constrained minimum.For convenience,all quan-tities that refer to thefirst multiplier will have a subscript1,while all quantities that refer to the second multiplier will have a subscript2.Because there are only two multipliers, the constraints can easily be displayed in two dimensions(seefigure1).The constrained minimum of the objective function must lie on a diagonal line segment.The ends of the diagonal line segment can be expressed quite simply in terms of.Let .The following bounds apply to:(7) Under normal circumstances,the objective function is positive definite,and there is a min-imum along the direction of the linear equality constraint.In this case,SMO computes the minimum along the direction of the linear equality constraint:where is the error on the th training example.As a next step,the constrained minimum is found by clipping into the interval.The value of is then computed from the new,clipped,:(9) For both linear and non-linear SVMs,the threshold is re-computed after each step,so that the KKT conditions are fulfilled for both optimized examples.2.2HEURISTICS FOR CHOOSING WHICH MULTIPLIERS TO OPTIMIZEIn order to speed convergence,SMO uses heuristics to choose which two Lagrange multi-pliers to jointly optimize.There are two separate choice heuristics:one for and one for.The choice of provides the outer loop of the SMO algorithm.If an example is found to violate the KKT conditions by the outer loop,it is eligible for optimization.The outer loop alternates single passes through the entire training set with multiple passes through the non-bound( ).The multiple passes terminate when all of the non-bound examples obey the KKT conditions within.The entire SMO algorithm terminates when the entire training setobeys the KKT conditions within.Typically,.Thefirst choice heuristic concentrates the CPU time on the examples that are most likely to violate the KKT conditions,i.e.,the non-bound subset.As the SMO algorithm progresses, that are at the bounds are likely to stay at the bounds,while that are not at the bounds will move as other examples are optimized.As a further optimization,SMO uses the shrinking heuristic proposed in[2].After the pass through the entire training set,shrinkingfinds examples which fulfill the KKT conditions more than the worst example failed the KKT conditions.Further passes through the training set ignore these fulfilled conditions until afinal pass at the end of training,which ensures that every example fulfills its KKT condition.Once an is chosen,SMO chooses an to maximize the size of the step taken during joint optimization.SMO approximates the step size by the absolute value of the numerator in equation(8):.SMO keeps a cached error value for every non-bound example in the training set and then chooses an error to approximately maximize the step size.If is positive,SMO chooses an example with minimum error.If is negative,SMO chooses an example with maximum error.Kernel Sparse Kernel Training Number of C%Used Used Size Vectors Inputs AdultLinLinear N mix1122141580.050 WebLinLinear N mix49749172310 AdultGaussKGaussian Y N112214206189 AdultGaussKDGaussian N N11221420610 WebGaussKGaussian Y N497494484596 WebGaussKDGaussian N N49749448450 MNISTTable1:Parameters for various experimentsSMO Chunking SMO Chunking(sec)(sec)(sec)Exponent Exponent Exponent AdultLin21.9n/a21141.1 1.0n/a 3.0339.93980.817164.7 1.6 2.2 2.5 WebLinDAdultGaussK523.3737.5n/a 2.0 2.0n/a1433.0n/a14740.4 2.5n/a 2.8 AdultGaussDWebGaussK2538.06923.5n/a 1.6 1.8n/a23365.3n/a50371.9 2.6n/a 2.0 WebGaussDMNISTTable2:Timings of algorithms on various data sets.tions of two-dimensional sub-problems,while uses numerical QP to solve10-dimensional sub-problems.The difference in timings between the two methods is partly due to the numerical QP overhead,but mostly due to the difference in heuristics and kernel optimizations.For example,SMO is faster than by an order of magnitude on linear problems,due to linear SVM folding.However,can also potentially use linear SVM folding.In these experiments,SMO uses a very simple least-recently-used ker-nel cache of Hessian rows,while uses a more complex kernel cache and modifies its heuristics to utilize the kernel effectively[2].Therefore,SMO does not benefit from the kernel cache at the largest problem sizes,while speeds up by a factor of2.5. Utilizing sparseness to compute kernels yields a large advantage for SMO due to the lack of heavy numerical QP overhead.For the sparse data sets shown,SMO can speed up bya factor of between3and13,while PCG chunking only obtained a maximum speed up of2.1times.The MNIST experiments were performed without a kernel cache,because the MNIST data set takes up most of the memory of the benchmark machine.Due to sparse inputs,SMO is a factor of1.7faster than PCG chunking,even though none of the Lagrange multipliers are at.On a machine with more memory,would be as fast or faster than SMO for MNIST,due to kernel caching.In summary,SMO is a simple method for training support vector machines which does not require a numerical QP library.Because its CPU time is dominated by kernel evaluation, SMO can be dramatically quickened by the use of kernel optimizations,such as linear SVM folding and sparse dot products.SMO can be anywhere from1.7to1500times faster than the standard PCG chunking algorithm,depending on the data set. AcknowledgementsThanks to Chris Burges for running data sets through his projected conjugate gradient code and for various helpful suggestions.References[1]C.J.C.Burges.A tutorial on support vector machines for pattern recognition.DataMining and Knowledge Discovery,2(2),1998.[2]T.Joachims.Making large-scale SVM learning practical.In B.Sch¨o lkopf,C.J.C.Burges,and A.J.Smola,editors,Advances in Kernel Methods—Support Vector Learning,pages169–184.MIT Press,1998.[3]L.Kaufman.Solving the quadratic programming problem arising in support vectorclassification.In B.Sch¨o lkopf,C.J.C.Burges,and A.J.Smola,editors,Advances in Kernel Methods—Support Vector Learning,pages147–168.MIT Press,1998. [4]Y.LeCun.MNIST handwritten digit database.Available on the web at http:///˜yann/ocr/mnist/.[5]C.J.Merz and P.M.Murphy.UCI repository of machine learning databases,1998.[/mlearn/MLRepository.html].Irvine,CA:University of Cali-fornia,Department of Information and Computer Science.[6]E.Osuna,R.Freund,and F.Girosi.Improved training algorithm for support vectormachines.In Proc.IEEE Neural Networks in Signal Processing’97,1997.[7]J.C.Platt.Fast training of SVMs using sequential minimal optimization.InB.Sch¨o lkopf,C.J.C.Burges,and A.J.Smola,editors,Advances in Kernel Meth-ods—Support Vector Learning,pages185–208.MIT Press,1998.[8]J.C.Platt.Sequential minimal optimization:A fast algorithm for training support vec-tor machines.Technical Report MSR–TR–98–14,Microsoft Research,1998.Available at /˜jplatt/smo.html.[9]V.Vapnik.Estimation of Dependences Based on Empirical Data.Springer-Verlag,1982.。
【精品】科技英语4低通滤波器原文和翻译

【关键字】精品Words and Expressionsintegrator n. 积分器amplitude n. 幅值slope n 斜率denominator n. 分母impedance n 阻抗inductor n. 电感capacitor n 电容cascade n. 串联passband n 通带ringing n. 振铃damping n. 阻尼,衰减conjugate adj. 共轭的stage v. 成为low-pass filters 低通滤波器building block 模块linear ramp 线性斜坡log/log coordinates 对数/对数坐标Bode plot 伯德图transfer function 传递函数complex-frequency variable 复变量complex frequency plane 复平面real component 实部frequency response 频率响应complex function 复变函数Laplace transform 拉普拉斯变换real part 实部imaginary part 虚部angular frequency 角频率frequency response 频率响应transient response 瞬态响应decaying-exponential response 衰减指数响应step function input 阶跃(函数)输入time constant 时间常数first-order filters 一阶滤波器second-order low-pass filters 二阶低通滤波器passive circuit 无源电路active circuit 有源电路characteristic frequency 特征频率quality factor n. 品质因子,品质因数circular path 圆弧路径complex conjugate pairs 共轭复数对switched-capacitor 开关电容negative-real half of the complex plane 复平面负半平面Unit 4 Low-pass FiltersFirst-Order FiltersAn integrator (Figure 2. la) is the simplest filter mathematically, and it forms the building block for most modern integrated filters. Consider what we know intuitively about an integrator. If you apply a DC signal at the input (i.e., zero frequency), the output will describe a linear ramp that grows in amplitude until limited by the power supplies. Ignoring that limitation, the response of an integrator at zero frequency is infinite, which means that it has a pole at zero frequency. (A pole exists at any frequency for which the transfer function's value becomes infinite.)(为什么为极点,为什么低通?)Figure A simple RC integratorWe also know that the integrator's gain diminishes with increasing frequency and that at high frequencies the output voltage becomes virtually zero. Gain is inversely proportional to frequency, so it has a slope of -1 when plotted on log/log coordinates (i.e., -20dB/decade on a Bode plot, Figure 2. 1b).Figure 2.1 b A Bode plot of a simple integratorYou can easily derive the transfer function asWhere s is the complex-frequency variable and is 1/RC. If we think of s as frequency, this formula confirms the intuitive feeling that gain is inversely proportional to frequency.The next most complex filter is the simple low-pass RC type (Figure 2. 2a). Its characteristic (transfer function) isWhen, the function reduces to , i.e., 1. When s tends to infinity, the function tends to zero, so this is a low-pass filter. When, the denominator is zero and the function's value is infinite, indicating a pole in the complex frequency plane. The magnitude of the transfer function is plotted against s in Figure 2. 2b, where the real component of s () is toward us and the positive imaginary part () is toward the right. The pole at - is evident. Amplitude is shown logarithmically to emphasize the function's form. For both the integrator and the RC low-pass filter, frequency response tends to zero at infinite frequency; that is, there is a zero at. This single zero surrounds the complex plane.But how does the complex function in s relate to the circuit's response to actual frequencies? When analyzing the response of a circuit to AC signals, we use the expression for impedance of an inductor and for that of a capacitor. When analyzing transient response using Laplace transforms, we use sL and 1/sC for the impedance of these elements. The similarity is apparent immediately. The in AC analysis is in fact the imaginary part of s, which, as mentioned earlier, is composed of a real part and an imaginary part.If we replace s by in any equation so far, we have the circuit's response to an angular frequency. In the complex plot in Figure 2.2b, and hence along the positive j axis. Thus, the function's value along this axis is the frequency response of the filter. We have sliced the function along the axis and emphasized the RC low-pass filter's frequency-response curve by adding a heavy line for function values along the positive j axis. The more familiar Bode plot (Figure 2.2c) looks different in form only because the frequency isexpressed logarithmically.(根据图翻译这两句话)Figure 2.2a A simple RC low-pass filterWhile the complex frequency's imaginary part () helps describe a response to AC signals, the real part() helps describe a circuit's transient response. Looking at Figure 2.2b, we can therefore say something about the RC low-pass filter's response as compared to that of the integrator. The low-pass filter's transient response is more stable, because its pole is in the negative-real half of the complex plane. That is, the low-pass filter makes a decaying-exponential response to a step-function input; the integrator makes an infinite response. For the low-pass filter, pole positions further down the axis mean a higher, a shorter time constant, and therefore a quicker transient response. Conversely, a pole closer to the j axis causes a longer transient response.So far, we have related the mathematical transfer functions of some simple circuits to their associated poles and zeroes in the complex-frequency plane . From these functions, we have derived the circuit ’s frequency response (and hence its Bode plot) and also its transient response. Because both the integrator and the RC filter have only one s in the denominator of their transfer functions, they each have only one pole. That is, they are first-order filters .Figure 2.2b The complex function of an RC low-pass filterFigure 2.2c A Bode plot of a low-pass filterHowever, as we can see from Figure 2.1b, the first-order filter does not provide a very selective frequency response. To tailor a filter more closely to our needs , we must move on to higher orders. From now on, we will describe the transfer function using f(s) rather than the cumbersome IN OUT V V . Second-Order Low-Pass FiltersA second-order filter has 2s in the denominator and two poles in the complex plane. You can obtain such a response by using inductance and capacitance in a passive circuit or by creating an active circuit of resistors, capacitors, and amplifiers. Consider the passive LC filter in Figure 2.3a, for instance. We can show that its transfer function has the formand if we defineLC /120=ωand R L Q /0ω=,then where 0ωis the filter's characteristic frequency and Q is the quality factor (lower R means higher Q).Figure 2.3a An RLC low-pass filterThe poles occur at s values for which the denominator becomes zero; that is,when 0/2002=++ωωQ s s . We can solve this equation by remembering that the roots of 02=++c bx ax are given byIn this case, a = 1, b 0ω=, and 20ω=c .The term (ac b 42-) equals ()4/1220-Q ω, so if Q isless than 0.5 then both roots are real and lie on the negative-real axis. The circuit's behavior is much like that of two first order RC filters in cascade . This case isn't very interesting, so we'll consider only the case where Q > 0.5, which means ()ac b 42-is negative and the roots are complex.Figure 2.3b A pole-zero diagram of an RLC low-pass filterThe real part is therefore a b 2/-, which is Q 2/0ω-, and common to both roots. The roots' imaginary parts will be equal and opposite in signs. Calculating the position of the roots in the complex plane, we find that they lie at a distance of0ωfrom the origin, as shown in Figure 2.3b. Varying 0ω, changes the poles' distance from the origin. Decreasing the Q moves the poles toward each other, whereas increasing the Q moves the poles in a semicircle away from each other and toward the ωj axis. When Q = 0.5, the poles meet at 0ω-on the negative-real axis. In this case, the corresponding circuit is equivalent to two cascaded first-order filters.Now let's examine the second-order function's frequency response and see how it varies with Q. As before, Figure 2.4a shows the function as a curved surface, depicted in the three-dimensional space formed by the complex plane and a vertical magnitude vector . Q =0.707, and you can see immediately that the response is a low-pass filter.The effect of increasing the Q is to move the poles in a circular path toward the ωj axis. Figure2.4b shows the case where Q = 2. Because the poles are closer to the ωj axis, they have a greater effect on the frequency response, causing a peak at the high end of the passband .Figure 2.4a The complex function of a second-order low-pass filter (Q = 0.707)Figure 2.4b The complex function of a second-order low-pass filter (Q = 2)There is also an effect on the filter's transient response. Because the poles' negative-real part is smaller, an input step function will cause ringing at the filter output. Lower values of Q result in less ringing, because the damping is greater. On the other hand, if Q becomes infinite, the poles reach the ωj axis, causing an infinite frequency response (instability and continuous oscillation) at 0ωω=. In the LCR circuit in Figure 2.3a, this condition would be impossible unless R=0. For filters that contain amplifiers, however, the condition is possible and must be considered in the design process.A second-order filter provides the variables 0ωand Q, which allow us to place poles wherever we want in the complex plane. These poles must, however, occur as complex conjugate pairs , in which the real parts are equal and the imaginary parts have opposite signs. This flexibility in pole placement is a powerful tool and one that makes the second-order stage a useful component in many switched-capacitor filters. As in the first-order case, the second-order low-pass transfer function tends to zero as frequency tends to infinity. The second-order function decreases twice as fast, however, because of the 2s factor in the denominator. The result is a double zero (零点) at infinity. 低通滤波器一阶滤波器从数学公式上讲,积分器(见图2.1a )是最简单的滤波器;它是构成大多数现代滤波器的基本模块。
Probability and Stochastic Processes

Probability and Stochastic ProcessesProbability and stochastic processes are important concepts in the field of mathematics and have applications in various areas such as engineering, finance, and computer science. In this response, I will discuss the significance of probability and stochastic processes from multiple perspectives, highlightingtheir practical applications, theoretical foundations, and potential limitations. From a practical perspective, probability and stochastic processes play a crucial role in decision-making under uncertainty. Whether it is predicting the weather, estimating the risk of a financial investment, or designing a reliable communication system, the ability to quantify and analyze uncertainty is essential. Probability theory provides a framework for modeling and analyzing random events, enabling us to make informed decisions based on the likelihood of different outcomes. Stochastic processes, on the other hand, allow us to model systems that evolve over time in a probabilistic manner, providing valuable insights into the behavior of complex systems. In the field of engineering, probability and stochastic processes are used extensively in reliability analysis and system design. By modeling the failure rates of components and the interactions between them, engineers can evaluate the reliability of a system and identify potential weaknesses. This information is crucial for designing robust systems that can withstand uncertainties and minimize the risk of failure. Stochastic processes, such as Markov chains and queuing theory, are also used to model and analyze various engineering systems, including communication networks, manufacturing processes, and transportation systems. From a financial perspective, probability and stochastic processes are essential tools for risk management and investment analysis. Financial markets are inherently uncertain, and understanding the probabilistic nature of asset prices and returns is crucial for making informed investment decisions. By modeling the behavior of financial variables using stochastic processes, such as geometric Brownian motion or jump-diffusion processes, analysts can estimate the probabilities of different market scenarios and assess the risk associated with different investment strategies. This information is invaluable for portfolio management, option pricing, and hedging strategies. From a theoretical perspective, probability theory and stochasticprocesses provide a rigorous mathematical foundation for understanding randomness and uncertainty. Probability theory, with its axioms and theorems, allows us to reason logically about uncertain events and make precise statements about their probabilities. Stochastic processes, as mathematical models for random phenomena, provide a framework for studying the long-term behavior of systems and analyzing their statistical properties. This theoretical understanding is not only important for practical applications but also for advancing our knowledge in various scientific disciplines, including physics, biology, and social sciences. However, it is important to acknowledge the limitations of probability and stochastic processes. Firstly, these concepts are based on assumptions and simplifications that may not always hold in real-world situations. For example, many stochastic models assume that the underlying processes are stationary and independent, which may not be true in practice. Secondly, probability and stochastic processes can only provide probabilistic predictions and estimates, rather than deterministic outcomes. This inherent uncertainty means that even with the best models and data, there will always be a degree of unpredictability. Lastly, the accuracy of probability and stochastic models heavily relies on the availability and quality of data. In situations where data is limited or unreliable, the predictions and estimates obtained from these models may be less accurate or even misleading. In conclusion, probability and stochastic processes are fundamental concepts with wide-ranging applications and theoretical significance. They provide a powerful framework for quantifying and analyzing uncertainty, enabling us to make informed decisions and understand the behavior of complex systems. From practical applications in engineering and finance to theoretical foundations in mathematics and science, probability and stochastic processes play a crucial role in our understanding of the world. However, it is important to recognize theirlimitations and the inherent uncertainties they entail. By embracing uncertainty and using probability and stochastic processes as tools for reasoning anddecision-making, we can navigate the complexities of the world with greater confidence and understanding.。
核磁共振基本原理与实验操作指导说明书
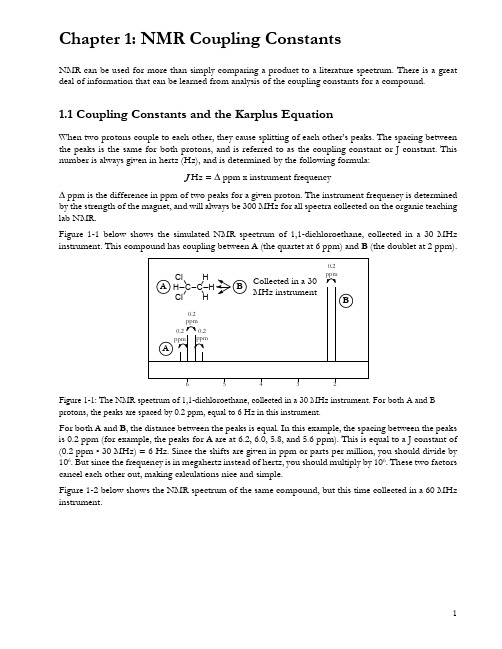
Chapter 1: NMR Coupling ConstantsNMR can be used for more than simply comparing a product to a literature spectrum. There is a great deal of information that can be learned from analysis of the coupling constants for a compound.1.1Coupling Constants and the Karplus EquationWhen two protons couple to each other, they cause splitting of each other’s peaks. The spacing between the peaks is the same for both protons, and is referred to as the coupling constant or J constant. This number is always given in hertz (Hz), and is determined by the following formula:J Hz = ∆ ppm x instrument frequency∆ ppm is the difference in ppm of two peaks for a given proton. The instrument frequency is determined by the strength of the magnet, and will always be 300 MHz for all spectra collected on the organic teaching lab NMR.Figure 1-1 below shows the simulated NMR spectrum of 1,1-dichloroethane, collected in a 30 MHz instrument. This compound has coupling between A (the quartet at 6 ppm) and B (the doublet at 2 ppm).Figure 1-1: The NMR spectrum of 1,1-dichloroethane, collected in a 30 MHz instrument. For both A and B protons, the peaks are spaced by 0.2 ppm, equal to 6 Hz in this instrument.For both A and B, the distance between the peaks is equal. In this example, the spacing between the peaks is 0.2 ppm (for example, the peaks for A are at 6.2, 6.0, 5.8, and 5.6 ppm). This is equal to a J constant of (0.2 ppm • 30 MHz) = 6 Hz. Since the shifts are given in ppm or parts per million, you should divide by 106. But since the frequency is in megahertz instead of hertz, you should multiply by 106. These two factors cancel each other out, making calculations nice and simple.Figure 1-2 below shows the NMR spectrum of the same compound, but this time collected in a 60 MHz instrument.Chapter 1: NMR Coupling ConstantsFigure 1-2: The NMR spectrum of 1,1-dichloroethane, collected in a 60 MHz instrument. For both A and B protons, the peaks are spaced by 0.1 ppm, equal to 6 Hz in this instrument.This time, the peak spacing is 0.1 ppm. This is equal to a J constant of (0.1 ppm • 60 MHz) = 6 Hz, the same as before. This shows that the J constant for any two particular protons will be the same value in hertz, no matter which instrument is used to measure it.The coupling constant provides valuable information about the structure of a compound. Some typical coupling constants are shown here.Figure 1-3: The coupling constants for some typical pairs of protons.In molecules where the rotation of bonds is constrained (for instance, in double bonds or rings), the coupling constant can provide information about stereochemistry. The Karplus equation describes how the coupling constant between two protons is affected by the dihedral angle between them. The equation follows the general format of J = A + B (cos θ) + C (cos 2θ), with the exact values of A, B and C dependent on several different factors. In general, though, a plot of this equation has the shape shown in Figure 1-4. Coupling constants will usually, but not always, fall into the shaded band on this graph.Figure 1-4: The plot of dihedral angle vs. coupling constant described by the Karplus equation.Chapter 1: NMR Coupling ConstantsThe highest coupling constants will occur between protons that have a dihedral angle of either 0° or 180°, and the lowest coupling constants will occur at 90°. This is due to orbital overlap – when the orbitals are at 90°, there is very little overlap between them, so the hydrogens cannot affect each other’s spins very much (Figure 1-5).Figure 1-5: The best orbital overlap occurs at 180° or 0°, which is why the coupling constant is higher for those angles.1.2 Calculating Coupling Constants in MestreNovaTo calculate coupling constants in MestreNova, there are several options. The easiest one is to use the Multiplet Analysis tool. To do this, go to Analysis → Multiplet Analysis → Manual (or just hit the “J” key). Drag a box around each group of equivalent protons. A purple version of the integral bar will appear below each one, along with a purple box above each one describing its splitting pattern and location in ppm. As with normal integrals, you can right-click the integral bar, select “Edit Multiplet”, and set these integrals to whatever makes sense for that particular structure. For example, in Figure 1-6, each peak is from a single proton so each integral should be about 1.00.Figure 1-6: An example NMR spectrum with multiplet analysis.HH H H HHChapter 1: NMR Coupling ConstantsOnce all peaks are labeled, you can go to Analysis → Multiplet Analysis → Report Multiplets. A text box should appear containing information about the peaks in a highly compressed format. You can then copy and paste this text into your lab report as needed. The spectrum shown above has the following multiplets listed:1H NMR (300 MHz, Chloroform-d) δ 5.14 (d, J = 11.7 Hz, 1H), 4.98 (d, J = 11.7 Hz, 1H), 4.75 (d, J = 3.2 Hz, 1H), 3.37 (d, J = 8.5 Hz, 1H), 3.30 (dd, J = 8.5, 3.3 Hz, 1H).The first set of parentheses indicates that the sample was dissolved in Chloroform-d and placed in a 300 MHz instrument. After that, there is a list of numbers. Each number or range indicates the chemical shift of each of the peaks in the spectrum, in order of descending chemical shift. Each number also has a set of parentheses after it, giving information about that peak. These parentheses contain: • A letter or letters to indicate the splitting of a peak (s=singlet, d=doublet, t=triplet, q=quartet); it is also possible to see things like dd for a doublet of doublets or b for broad. If MestreNova can’t identify a uniform splitting pattern, it will name it a multiplet (m).•The coupling constants or J-values for that peak – for example, the peak at 3.30 ppm has J-values of 8.5 and 3.3 ppm.•The integral of the peak, rounded to the nearest whole number of H.Using this information, you can determine which peaks in Figure 1-6 are coupling to each other based on which ones have matching J-values.•Peaks A and B in Figure 1-6 both have J-values of 11.7 Hz, so these two protons are coupling to each other.•Peaks C and E both have J-values of 3.2 or 3.3 Hz (similar enough, within a margin of error), so these two protons are coupling to each other.•Peaks D and E both have J-values of 8.5 Hz, so these two protons are coupling to each other. If the multiplet analysis tool is failing to determine J-values for any reason, you can always calculate them manually. To do this, you will need to get more precise values for your peak locations. Right-click anywhere in the empty space of the spectrum and select Properties, then go to Peaks and increase the decimals to 4 (Figure 1-7).Chapter 1: NMR Coupling ConstantsFigure 1-7: Changing the decimals on peak labeling.Now if you do peak-picking to label the locations of the peaks, you should see them to 4 decimal places. This will allow you to plus these into the equation to find the J-values manually. For example, in Figure 1-8, the peaks around 4.7 ppm have a J-value of (4.7550 ppm – 4.7442 ppm) • 300 MHz = 3.24 Hz. Note that this in in agreement with MestreNova’s determination of 3.2 ppm for this J-value in Figure1-6.Figure 1-8: Peaks labeled with enough precision to allow you to calculate J-values manually.Chapter 1: NMR Coupling Constants1.3 Topicity and Second-Order CouplingDuring the NMR tutorial, you learned about the concept of chemical equivalence: protons in identical chemical environments have identical chemical shifts. However, just because two protons have the same connectivity to the molecule does not mean they are chemically equivalent. This is related to the concept of topicity : the stereochemical relationship between different groups in a molecule. To find the topicity relationship of two groups to each other, you should try replacing first one group, then the other group with a placeholder atom (in the examples in Figure 1-9, a dark circle is used as the placeholder). If the two molecules produced are identical, then the groups are homotopic; if the molecules are enantiomers, then the groups are enantiotopic; and if the molecules are diastereomers, then the groups are diastereotopic. Groups that are diastereotopic are chemically inequivalent, so they will have a different chemical shift from each other in NMR, and will show coupling as if they were neighboring protons instead of on the same carbon atom.Figure 1-9: Some examples of homotopic, enantiotopic, and diastereotopic groups.If two signals are coupled to each other and have very similar (but not identical) chemical shifts, another effect will appear: second-order coupling. This means that the peaks appear to “lean” toward each other – the peaks on the outside of the coupled pair are shorter, and the peaks on the inside are taller. (Figure 1-10).Figure 1-10: As the chemical shifts of H a and H b become more and more similar, the coupling between them becomes more second-order and the peaks lean more.Chapter 1: NMR Coupling Constants This is very common for two diastereotopic protons on the same carbon atom, but it appears in other situations where two protons are almost chemically identical as well. In Figure 1-8, note the two doublets at 4.98 and 5.14 ppm. These happen to be diastereotopic protons – they are attached to the same carbon, but are chemically equivalent.Looking for pairs of leaning peaks is useful, because it allows you to identify which protons are coupled to each other in a complicated spectrum. In Figure 1-11, there are two different pairs of leaning peaks: two 1H peaks with a J = 9 Hz, and two 2H peaks with J = 15 Hz. Recognizing this makes it possible to pick apart the different components of the peaks towards the left of the spectrum: these are two overlapping doublets, not a quartet.Figure 1-11: An NMR spectrum with two different pairs of leaning peaks.The multiplet tool in MestreNova might not work immediately for analyzing overlapping multiplets like this. Instead, you should follow the instructions at /resolving-overlapped-multiplets/ to deal with them.。
毕业论文的实证研究的英文数据分析与结果
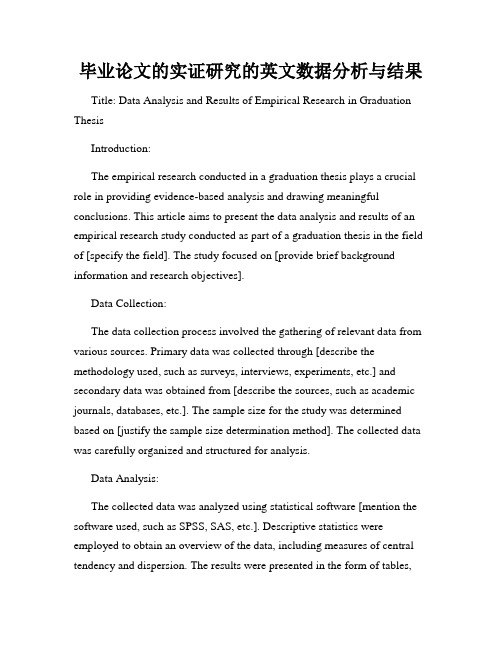
毕业论文的实证研究的英文数据分析与结果Title: Data Analysis and Results of Empirical Research in Graduation ThesisIntroduction:The empirical research conducted in a graduation thesis plays a crucial role in providing evidence-based analysis and drawing meaningful conclusions. This article aims to present the data analysis and results of an empirical research study conducted as part of a graduation thesis in the field of [specify the field]. The study focused on [provide brief background information and research objectives].Data Collection:The data collection process involved the gathering of relevant data from various sources. Primary data was collected through [describe the methodology used, such as surveys, interviews, experiments, etc.] and secondary data was obtained from [describe the sources, such as academic journals, databases, etc.]. The sample size for the study was determined based on [justify the sample size determination method]. The collected data was carefully organized and structured for analysis.Data Analysis:The collected data was analyzed using statistical software [mention the software used, such as SPSS, SAS, etc.]. Descriptive statistics were employed to obtain an overview of the data, including measures of central tendency and dispersion. The results were presented in the form of tables,graphs, and charts for better visualization and understanding. The analysis also involved inferential statistics such as [mention the statistical tests used, such as t-tests, ANOVA, correlation analysis, etc.] to establish relationships, correlations, and significance levels.Results:Based on the data analysis, the following results were obtained:1. Presentation of Descriptive Statistics:- Mean, median, and mode of [mention the variables or factors]- Standard deviation and variance of [mention the variables or factors]- Frequency distribution of [mention the variables or factors]2. Inferential Statistical Analysis:- Correlation analysis revealed a strong positive correlation between [variables A and B], indicating a significant relationship (p < 0.05).- The results of the t-test demonstrated a significant difference (p < 0.01) between [group A] and [group B] in terms of [mention the variable of interest].- ANOVA analysis indicated a significant effect (p < 0.05) of [independent variable] on [dependent variable] across [mention the groups or categories].Discussion and Interpretation:The obtained results were discussed and interpreted in the context of the research objectives and relevant literature. The implications of the findingswere explored, addressing how they contribute to the existing knowledge in the field. Where applicable, the limitations of the study were acknowledged and suggestions for future research were provided. The results were compared and contrasted with previous studies in order to identify similarities or discrepancies and to validate or challenge existing theories.Conclusion:Based on the empirical research conducted and the data analysis performed, the results obtained support the objectives of the graduation thesis and provide valuable insights into [mention the topic or research area]. The findings contribute to the understanding of [mention the field] and provide a foundation for further research in the future. It is important to note that the results are based on the specific sample and methods employed in this study, and therefore, generalization to a broader population should be done with caution.In a nutshell, the data analysis and results of the empirical research study conducted as part of the graduation thesis provide significant evidence and valuable insights pertaining to [mention the field or topic]. The rigorous analysis conducted adheres to academic standards and enhances the overall credibility and reliability of the research.Word Count: [count the final word count based on the provided content, ensure it meets the required limit]。
高中英语学术论文研究方法单选题40题
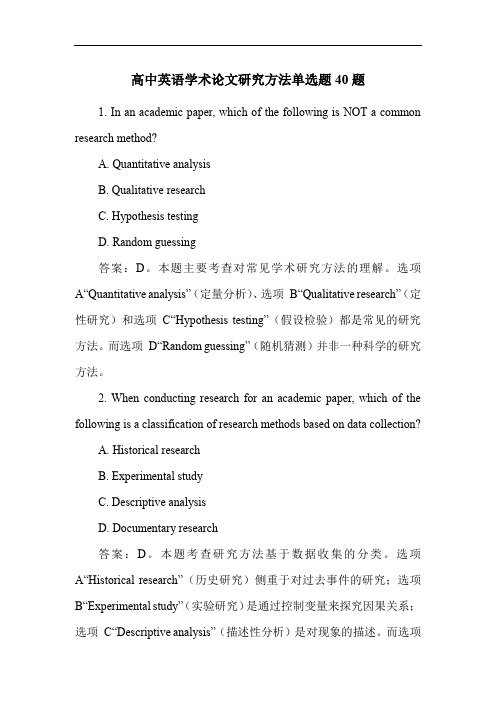
高中英语学术论文研究方法单选题40题1. In an academic paper, which of the following is NOT a common research method?A. Quantitative analysisB. Qualitative researchC. Hypothesis testingD. Random guessing答案:D。
本题主要考查对常见学术研究方法的理解。
选项A“Quantitative analysis”( 定量分析)、选项B“Qualitative research”( 定性研究)和选项C“Hypothesis testing” 假设检验)都是常见的研究方法。
而选项D“Random guessing”( 随机猜测)并非一种科学的研究方法。
2. When conducting research for an academic paper, which of the following is a classification of research methods based on data collection?A. Historical researchB. Experimental studyC. Descriptive analysisD. Documentary research答案:D。
本题考查研究方法基于数据收集的分类。
选项A“Historical research” 历史研究)侧重于对过去事件的研究;选项B“Experimental study”( 实验研究)是通过控制变量来探究因果关系;选项C“Descriptive analysis”( 描述性分析)是对现象的描述。
而选项D“Documentary research” 文献研究)是基于已有的文献资料进行收集和分析,属于基于数据收集的分类。
3. In an academic paper, which research method mainly focuses on understanding the meaning and experience of individuals?A. Empirical studyB. Grounded theoryC. Content analysisD. Case study答案:B。
Advances in
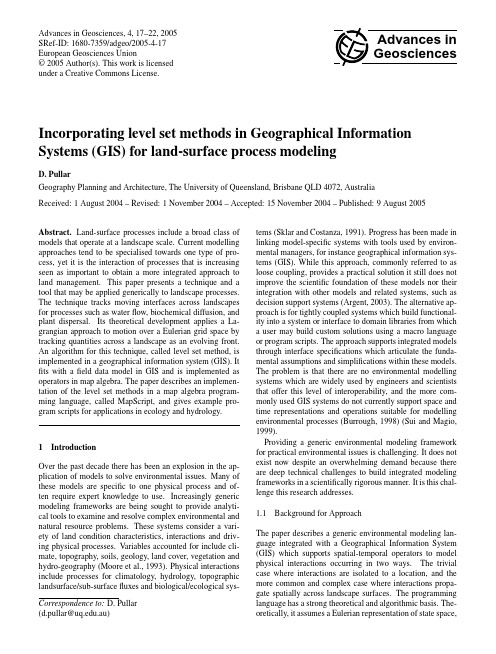
Advances in Geosciences,4,17–22,2005 SRef-ID:1680-7359/adgeo/2005-4-17 European Geosciences Union©2005Author(s).This work is licensed under a Creative CommonsLicense.Advances in GeosciencesIncorporating level set methods in Geographical Information Systems(GIS)for land-surface process modelingD.PullarGeography Planning and Architecture,The University of Queensland,Brisbane QLD4072,Australia Received:1August2004–Revised:1November2004–Accepted:15November2004–Published:9August2005nd-surface processes include a broad class of models that operate at a landscape scale.Current modelling approaches tend to be specialised towards one type of pro-cess,yet it is the interaction of processes that is increasing seen as important to obtain a more integrated approach to land management.This paper presents a technique and a tool that may be applied generically to landscape processes. The technique tracks moving interfaces across landscapes for processes such as waterflow,biochemical diffusion,and plant dispersal.Its theoretical development applies a La-grangian approach to motion over a Eulerian grid space by tracking quantities across a landscape as an evolving front. An algorithm for this technique,called level set method,is implemented in a geographical information system(GIS).It fits with afield data model in GIS and is implemented as operators in map algebra.The paper describes an implemen-tation of the level set methods in a map algebra program-ming language,called MapScript,and gives example pro-gram scripts for applications in ecology and hydrology.1IntroductionOver the past decade there has been an explosion in the ap-plication of models to solve environmental issues.Many of these models are specific to one physical process and of-ten require expert knowledge to use.Increasingly generic modeling frameworks are being sought to provide analyti-cal tools to examine and resolve complex environmental and natural resource problems.These systems consider a vari-ety of land condition characteristics,interactions and driv-ing physical processes.Variables accounted for include cli-mate,topography,soils,geology,land cover,vegetation and hydro-geography(Moore et al.,1993).Physical interactions include processes for climatology,hydrology,topographic landsurface/sub-surfacefluxes and biological/ecological sys-Correspondence to:D.Pullar(d.pullar@.au)tems(Sklar and Costanza,1991).Progress has been made in linking model-specific systems with tools used by environ-mental managers,for instance geographical information sys-tems(GIS).While this approach,commonly referred to as loose coupling,provides a practical solution it still does not improve the scientific foundation of these models nor their integration with other models and related systems,such as decision support systems(Argent,2003).The alternative ap-proach is for tightly coupled systems which build functional-ity into a system or interface to domain libraries from which a user may build custom solutions using a macro language or program scripts.The approach supports integrated models through interface specifications which articulate the funda-mental assumptions and simplifications within these models. The problem is that there are no environmental modelling systems which are widely used by engineers and scientists that offer this level of interoperability,and the more com-monly used GIS systems do not currently support space and time representations and operations suitable for modelling environmental processes(Burrough,1998)(Sui and Magio, 1999).Providing a generic environmental modeling framework for practical environmental issues is challenging.It does not exist now despite an overwhelming demand because there are deep technical challenges to build integrated modeling frameworks in a scientifically rigorous manner.It is this chal-lenge this research addresses.1.1Background for ApproachThe paper describes a generic environmental modeling lan-guage integrated with a Geographical Information System (GIS)which supports spatial-temporal operators to model physical interactions occurring in two ways.The trivial case where interactions are isolated to a location,and the more common and complex case where interactions propa-gate spatially across landscape surfaces.The programming language has a strong theoretical and algorithmic basis.The-oretically,it assumes a Eulerian representation of state space,Fig.1.Shows a)a propagating interface parameterised by differ-ential equations,b)interface fronts have variable intensity and may expand or contract based onfield gradients and driving process. but propagates quantities across landscapes using Lagrangian equations of motion.In physics,a Lagrangian view focuses on how a quantity(water volume or particle)moves through space,whereas an Eulerian view focuses on a localfixed area of space and accounts for quantities moving through it.The benefit of this approach is that an Eulerian perspective is em-inently suited to representing the variation of environmen-tal phenomena across space,but it is difficult to conceptu-alise solutions for the equations of motion and has compu-tational drawbacks(Press et al.,1992).On the other hand, the Lagrangian view is often not favoured because it requires a global solution that makes it difficult to account for local variations,but has the advantage of solving equations of mo-tion in an intuitive and numerically direct way.The research will address this dilemma by adopting a novel approach from the image processing discipline that uses a Lagrangian ap-proach over an Eulerian grid.The approach,called level set methods,provides an efficient algorithm for modeling a natural advancing front in a host of settings(Sethian,1999). The reason the method works well over other approaches is that the advancing front is described by equations of motion (Lagrangian view),but computationally the front propagates over a vectorfield(Eulerian view).Hence,we have a very generic way to describe the motion of quantities,but can ex-plicitly solve their advancing properties locally as propagat-ing zones.The research work will adapt this technique for modeling the motion of environmental variables across time and space.Specifically,it will add new data models and op-erators to a geographical information system(GIS)for envi-ronmental modeling.This is considered to be a significant research imperative in spatial information science and tech-nology(Goodchild,2001).The main focus of this paper is to evaluate if the level set method(Sethian,1999)can:–provide a theoretically and empirically supportable methodology for modeling a range of integral landscape processes,–provide an algorithmic solution that is not sensitive to process timing,is computationally stable and efficient as compared to conventional explicit solutions to diffu-sive processes models,–be developed as part of a generic modelling language in GIS to express integrated models for natural resource and environmental problems?The outline for the paper is as follow.The next section will describe the theory for spatial-temporal processing us-ing level sets.Section3describes how this is implemented in a map algebra programming language.Two application examples are given–an ecological and a hydrological ex-ample–to demonstrate the use of operators for computing reactive-diffusive interactions in landscapes.Section4sum-marises the contribution of this research.2Theory2.1IntroductionLevel set methods(Sethian,1999)have been applied in a large collection of applications including,physics,chemistry,fluid dynamics,combustion,material science,fabrication of microelectronics,and computer vision.Level set methods compute an advancing interface using an Eulerian grid and the Lagrangian equations of motion.They are similar to cost distance modeling used in GIS(Burroughs and McDonnell, 1998)in that they compute the spread of a variable across space,but the motion is based upon partial differential equa-tions related to the physical process.The advancement of the interface is computed through time along a spatial gradient, and it may expand or contract in its extent.See Fig.1.2.2TheoryThe advantage of the level set method is that it models mo-tion along a state-space gradient.Level set methods start with the equation of motion,i.e.an advancing front with velocity F is characterised by an arrival surface T(x,y).Note that F is a velocityfield in a spatial sense.If F was constant this would result in an expanding series of circular fronts,but for different values in a velocityfield the front will have a more contorted appearance as shown in Fig.1b.The motion of thisinterface is always normal to the interface boundary,and its progress is regulated by several factors:F=f(L,G,I)(1)where L=local properties that determine the shape of advanc-ing front,G=global properties related to governing forces for its motion,I=independent properties that regulate and influ-ence the motion.If the advancing front is modeled strictly in terms of the movement of entity particles,then a straightfor-ward velocity equation describes its motion:|∇T|F=1given T0=0(2) where the arrival function T(x,y)is a travel cost surface,and T0is the initial position of the interface.Instead we use level sets to describe the interface as a complex function.The level set functionφis an evolving front consistent with the under-lying viscosity solution defined by partial differential equa-tions.This is expressed by the equation:φt+F|∇φ|=0givenφ(x,y,t=0)(3)whereφt is a complex interface function over time period 0..n,i.e.φ(x,y,t)=t0..tn,∇φis the spatial and temporal derivatives for viscosity equations.The Eulerian view over a spatial domain imposes a discretisation of space,i.e.the raster grid,which records changes in value z.Hence,the level set function becomesφ(x,y,z,t)to describe an evolv-ing surface over time.Further details are given in Sethian (1999)along with efficient algorithms.The next section de-scribes the integration of the level set methods with GIS.3Map algebra modelling3.1Map algebraSpatial models are written in a map algebra programming language.Map algebra is a function-oriented language that operates on four implicit spatial data types:point,neighbour-hood,zonal and whole landscape surfaces.Surfaces are typ-ically represented as a discrete raster where a point is a cell, a neighbourhood is a kernel centred on a cell,and zones are groups of mon examples of raster data include ter-rain models,categorical land cover maps,and scalar temper-ature surfaces.Map algebra is used to program many types of landscape models ranging from land suitability models to mineral exploration in the geosciences(Burrough and Mc-Donnell,1998;Bonham-Carter,1994).The syntax for map algebra follows a mathematical style with statements expressed as equations.These equations use operators to manipulate spatial data types for point and neighbourhoods.Expressions that manipulate a raster sur-face may use a global operation or alternatively iterate over the cells in a raster.For instance the GRID map algebra (Gao et al.,1993)defines an iteration construct,called do-cell,to apply equations on a cell-by-cell basis.This is triv-ially performed on columns and rows in a clockwork manner. However,for environmental phenomena there aresituations Fig.2.Spatial processing orders for raster.where the order of computations has a special significance. For instance,processes that involve spreading or transport acting along environmental gradients within the landscape. Therefore special control needs to be exercised on the order of execution.Burrough(1998)describes two extra control mechanisms for diffusion and directed topology.Figure2 shows the three principle types of processing orders,and they are:–row scan order governed by the clockwork lattice struc-ture,–spread order governed by the spreading or scattering ofa material from a more concentrated region,–flow order governed by advection which is the transport of a material due to velocity.Our implementation of map algebra,called MapScript (Pullar,2001),includes a special iteration construct that sup-ports these processing orders.MapScript is a lightweight lan-guage for processing raster-based GIS data using map alge-bra.The language parser and engine are built as a software component to interoperate with the IDRISI GIS(Eastman, 1997).MapScript is built in C++with a class hierarchy based upon a value type.Variants for value types include numeri-cal,boolean,template,cells,or a grid.MapScript supports combinations of these data types within equations with basic arithmetic and relational comparison operators.Algebra op-erations on templates typically result in an aggregate value assigned to a cell(Pullar,2001);this is similar to the con-volution integral in image algebras(Ritter et al.,1990).The language supports iteration to execute a block of statements in three ways:a)docell construct to process raster in a row scan order,b)dospread construct to process raster in a spreadwhile(time<100)dospreadpop=pop+(diffuse(kernel*pop))pop=pop+(r*pop*dt*(1-(pop/K)) enddoendwhere the diffusive constant is stored in thekernel:Fig.3.Map algebra script and convolution kernel for population dispersion.The variable pop is a raster,r,K and D are constants, dt is the model time step,and the kernel is a3×3template.It is assumed a time step is defined and the script is run in a simulation. Thefirst line contained in the nested cell processing construct(i.e. dospread)is the diffusive term and the second line is the population growth term.order,c)doflow to process raster byflow order.Examples are given in subsequent sections.Process models will also involve a timing loop which may be handled as a general while(<condition>)..end construct in MapScript where the condition expression includes a system time variable.This time variable is used in a specific fashion along with a system time step by certain operators,namely diffuse()andfluxflow() described in the next section,to model diffusion and advec-tion as a time evolving front.The evolving front represents quantities such as vegetation growth or surface runoff.3.2Ecological exampleThis section presents an ecological example based upon plant dispersal in a landscape.The population of a species follows a controlled growth rate and at the same time spreads across landscapes.The theory of the rate of spread of an organism is given in Tilman and Kareiva(1997).The area occupied by a species grows log-linear with time.This may be modelled by coupling a spatial diffusion term with an exponential pop-ulation growth term;the combination produces the familiar reaction-diffusion model.A simple growth population model is used where the reac-tion term considers one population controlled by births and mortalities is:dN dt =r·N1−NK(4)where N is the size of the population,r is the rate of change of population given in terms of the difference between birth and mortality rates,and K is the carrying capacity.Further dis-cussion of population models can be found in Jrgensen and Bendoricchio(2001).The diffusive term spreads a quantity through space at a specified rate:dudt=Dd2udx2(5) where u is the quantity which in our case is population size, and D is the diffusive coefficient.The model is operated as a coupled computation.Over a discretized space,or raster,the diffusive term is estimated using a numerical scheme(Press et al.,1992).The distance over which diffusion takes place in time step dt is minimally constrained by the raster resolution.For a stable computa-tional process the following condition must be satisfied:2Ddtdx2≤1(6) This basically states that to account for the diffusive pro-cess,the term2D·dx is less than the velocity of the advancing front.This would not be difficult to compute if D is constant, but is problematic if D is variable with respect to landscape conditions.This problem may be overcome by progressing along a diffusive front over the discrete raster based upon distance rather than being constrained by the cell resolution.The pro-cessing and diffusive operator is implemented in a map al-gebra programming language.The code fragment in Fig.3 shows a map algebra script for a single time step for the cou-pled reactive-diffusion model for population growth.The operator of interest in the script shown in Fig.3is the diffuse operator.It is assumed that the script is run with a given time step.The operator uses a system time step which is computed to balance the effect of process errors with effi-cient computation.With knowledge of the time step the it-erative construct applies an appropriate distance propagation such that the condition in Eq.(3)is not violated.The level set algorithm(Sethian,1999)is used to do this in a stable and accurate way.As a diffusive front propagates through the raster,a cost distance kernel assigns the proper time to each raster cell.The time assigned to the cell corresponds to the minimal cost it takes to reach that cell.Hence cell pro-cessing is controlled by propagating the kernel outward at a speed adaptive to the local context rather than meeting an arbitrary global constraint.3.3Hydrological exampleThis section presents a hydrological example based upon sur-face dispersal of excess rainfall across the terrain.The move-ment of water is described by the continuity equation:∂h∂t=e t−∇·q t(7) where h is the water depth(m),e t is the rainfall excess(m/s), q is the discharge(m/hr)at time t.Discharge is assumed to have steady uniformflow conditions,and is determined by Manning’s equation:q t=v t h t=1nh5/3ts1/2(8)putation of current cell(x+ x,t,t+ ).where q t is theflow velocity(m/s),h t is water depth,and s is the surface slope(m/m).An explicit method of calcula-tion is used to compute velocity and depth over raster cells, and equations are solved at each time step.A conservative form of afinite difference method solves for q t in Eq.(5). To simplify discussions we describe quasi-one-dimensional equations for theflow problem.The actual numerical com-putations are normally performed on an Eulerian grid(Julien et al.,1995).Finite-element approximations are made to solve the above partial differential equations for the one-dimensional case offlow along a strip of unit width.This leads to a cou-pled model with one term to maintain the continuity offlow and another term to compute theflow.In addition,all calcu-lations must progress from an uphill cell to the down slope cell.This is implemented in map algebra by a iteration con-struct,called doflow,which processes a raster byflow order. Flow distance is measured in cell size x per unit length. One strip is processed during a time interval t(Fig.4).The conservative solution for the continuity term using afirst or-der approximation for Eq.(5)is derived as:h x+ x,t+ t=h x+ x,t−q x+ x,t−q x,txt(9)where the inflow q x,t and outflow q x+x,t are calculated in the second term using Equation6as:q x,t=v x,t·h t(10) The calculations approximate discharge from previous time interval.Discharge is dynamically determined within the continuity equation by water depth.The rate of change in state variables for Equation6needs to satisfy a stability condition where v· t/ x≤1to maintain numerical stabil-ity.The physical interpretation of this is that afinite volume of water wouldflow across and out of a cell within the time step t.Typically the cell resolution isfixed for the raster, and adjusting the time step requires restarting the simulation while(time<120)doflow(dem)fvel=1/n*pow(depth,m)*sqrt(grade)depth=depth+(depth*fluxflow(fvel)) enddoendFig.5.Map algebra script for excess rainfallflow computed over a 120minute event.The variables depth and grade are rasters,fvel is theflow velocity,n and m are constants in Manning’s equation.It is assumed a time step is defined and the script is run in a simulation. Thefirst line in the nested cell processing(i.e.doflow)computes theflow velocity and the second line computes the change in depth from the previous value plus any net change(inflow–outflow)due to velocityflux across the cell.cycle.Flow velocities change dramatically over the course of a storm event,and it is problematic to set an appropriate time step which is efficient and yields a stable result.The hydrological model has been implemented in a map algebra programming language Pullar(2003).To overcome the problem mentioned above we have added high level oper-ators to compute theflow as an advancing front over a land-scape.The time step advances this front adaptively across the landscape based upon theflow velocity.The level set algorithm(Sethian,1999)is used to do this in a stable and accurate way.The map algebra script is given in Fig.5.The important operator is thefluxflow operator.It computes the advancing front for waterflow across a DEM by hydrologi-cal principles,and computes the local drainageflux rate for each cell.Theflux rate is used to compute the net change in a cell in terms offlow depth over an adaptive time step.4ConclusionsThe paper has described an approach to extend the function-ality of tightly coupled environmental models in GIS(Ar-gent,2004).A long standing criticism of GIS has been its in-ability to handle dynamic spatial models.Other researchers have also addressed this issue(Burrough,1998).The con-tribution of this paper is to describe how level set methods are:i)an appropriate scientific basis,and ii)able to perform stable time-space computations for modelling landscape pro-cesses.The level set method provides the following benefits:–it more directly models motion of spatial phenomena and may handle both expanding and contracting inter-faces,–is based upon differential equations related to the spatial dynamics of physical processes.Despite the potential for using level set methods in GIS and land-surface process modeling,there are no commercial or research systems that use this mercial sys-tems such as GRID(Gao et al.,1993),and research systems such as PCRaster(Wesseling et al.,1996)offerflexible andpowerful map algebra programming languages.But opera-tions that involve reaction-diffusive processing are specific to one context,such as groundwaterflow.We believe the level set method offers a more generic approach that allows a user to programflow and diffusive landscape processes for a variety of application contexts.We have shown that it pro-vides an appropriate theoretical underpinning and may be ef-ficiently implemented in a GIS.We have demonstrated its application for two landscape processes–albeit relatively simple examples–but these may be extended to deal with more complex and dynamic circumstances.The validation for improved environmental modeling tools ultimately rests in their uptake and usage by scientists and engineers.The tool may be accessed from the web site .au/projects/mapscript/(version with enhancements available April2005)for use with IDRSIS GIS(Eastman,1997)and in the future with ArcGIS. It is hoped that a larger community of users will make use of the methodology and implementation for a variety of environmental modeling applications.Edited by:P.Krause,S.Kralisch,and W.Fl¨u gelReviewed by:anonymous refereesReferencesArgent,R.:An Overview of Model Integration for Environmental Applications,Environmental Modelling and Software,19,219–234,2004.Bonham-Carter,G.F.:Geographic Information Systems for Geo-scientists,Elsevier Science Inc.,New York,1994. Burrough,P.A.:Dynamic Modelling and Geocomputation,in: Geocomputation:A Primer,edited by:Longley,P.A.,et al., Wiley,England,165-191,1998.Burrough,P.A.and McDonnell,R.:Principles of Geographic In-formation Systems,Oxford University Press,New York,1998. Gao,P.,Zhan,C.,and Menon,S.:An Overview of Cell-Based Mod-eling with GIS,in:Environmental Modeling with GIS,edited by: Goodchild,M.F.,et al.,Oxford University Press,325–331,1993.Goodchild,M.:A Geographer Looks at Spatial Information Theory, in:COSIT–Spatial Information Theory,edited by:Goos,G., Hertmanis,J.,and van Leeuwen,J.,LNCS2205,1–13,2001.Jørgensen,S.and Bendoricchio,G.:Fundamentals of Ecological Modelling,Elsevier,New York,2001.Julien,P.Y.,Saghafian,B.,and Ogden,F.:Raster-Based Hydro-logic Modelling of Spatially-Varied Surface Runoff,Water Re-sources Bulletin,31(3),523–536,1995.Moore,I.D.,Turner,A.,Wilson,J.,Jenson,S.,and Band,L.:GIS and Land-Surface-Subsurface Process Modeling,in:Environ-mental Modeling with GIS,edited by:Goodchild,M.F.,et al., Oxford University Press,New York,1993.Press,W.,Flannery,B.,Teukolsky,S.,and Vetterling,W.:Numeri-cal Recipes in C:The Art of Scientific Computing,2nd Ed.Cam-bridge University Press,Cambridge,1992.Pullar,D.:MapScript:A Map Algebra Programming Language Incorporating Neighborhood Analysis,GeoInformatica,5(2), 145–163,2001.Pullar,D.:Simulation Modelling Applied To Runoff Modelling Us-ing MapScript,Transactions in GIS,7(2),267–283,2003. Ritter,G.,Wilson,J.,and Davidson,J.:Image Algebra:An Overview,Computer Vision,Graphics,and Image Processing, 4,297–331,1990.Sethian,J.A.:Level Set Methods and Fast Marching Methods, Cambridge University Press,Cambridge,1999.Sklar,F.H.and Costanza,R.:The Development of Dynamic Spa-tial Models for Landscape Ecology:A Review and Progress,in: Quantitative Methods in Ecology,Springer-Verlag,New York, 239–288,1991.Sui,D.and R.Maggio:Integrating GIS with Hydrological Mod-eling:Practices,Problems,and Prospects,Computers,Environ-ment and Urban Systems,23(1),33–51,1999.Tilman,D.and P.Kareiva:Spatial Ecology:The Role of Space in Population Dynamics and Interspecific Interactions.Princeton University Press,Princeton,New Jersey,USA,1997. Wesseling C.G.,Karssenberg, D.,Burrough,P. A.,and van Deursen,W.P.:Integrating Dynamic Environmental Models in GIS:The Development of a Dynamic Modelling Language, Transactions in GIS,1(1),40–48,1996.。
七下英语第2单元单词
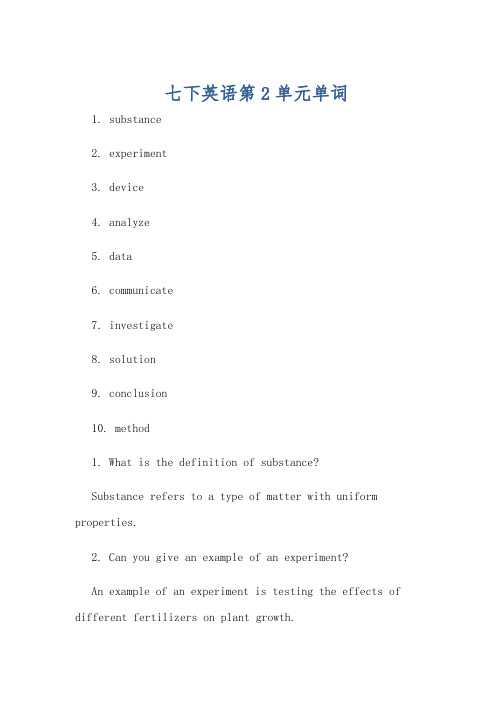
七下英语第2单元单词1. substance2. experiment3. device4. analyze5. data6. communicate7. investigate8. solution9. conclusion10. method1. What is the definition of substance?Substance refers to a type of matter with uniform properties.2. Can you give an example of an experiment?An example of an experiment is testing the effects of different fertilizers on plant growth.3. What is a device used for in scientific research?A device is used to measure or observe a specific aspect of an experiment or study.4. How do scientists analyze data?Scientists analyze data by looking for patterns, trends, and relationships to draw conclusions.5. What is the importance of communicating scientific findings?Communicating scientific findings is important to share knowledge, replicate experiments, and advance research.6. How do scientists investigate natural phenomena?Scientists investigate natural phenomena by conducting experiments, making observations, and analyzing data.7. What is a common step in finding a solution to a problem?A common step in finding a solution is brainstorming different possible approaches and evaluating their effectiveness.8. Why is drawing a conclusion important in the scientific method?Drawing a conclusion allows scientists to summarize their findings and determine if their hypothesis was supported.9. What is a method used in scientific research?A method is a systematic approach or procedure used to conduct experiments and gather data.10. How do scientists ensure accuracy in their research methods?Scientists ensure accuracy in their research methods by following standardized procedures, using precise measurements, and conducting controlled experiments.1. 什么是substance的定义?substance指的是具有统一性质的物质类型。
Employee_Attrition_Classification_Model_Based_on_S
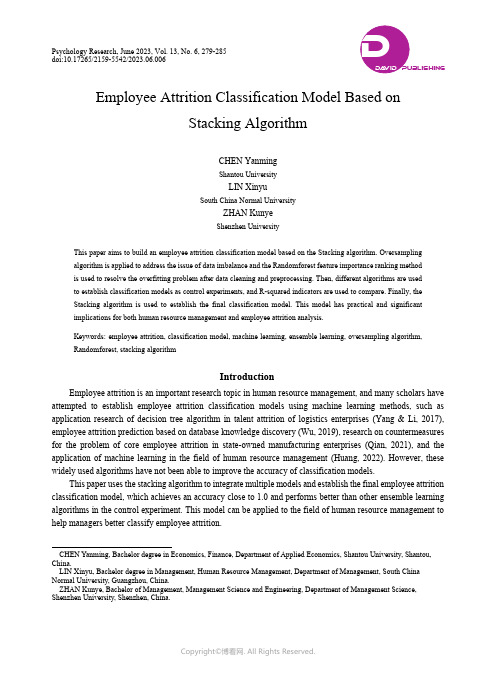
Psychology Research, June 2023, Vol. 13, No. 6, 279-285 doi:10.17265/2159-5542/2023.06.006 Employee Attrition Classification Model Based onStacking AlgorithmCHEN YanmingShantou UniversityLIN XinyuSouth China Normal UniversityZHAN KunyeShenzhen UniversityThis paper aims to build an employee attrition classification model based on the Stacking algorithm. Oversampling algorithm is applied to address the issue of data imbalance and the Randomforest feature importance ranking method is used to resolve the overfitting problem after data cleaning and preprocessing. Then, different algorithms are used to establish classification models as control experiments, and R-squared indicators are used to compare. Finally, the Stacking algorithm is used to establish the final classification model. This model has practical and significant implications for both human resource management and employee attrition analysis.Keywords: employee attrition, classification model, machine learning, ensemble learning, oversampling algorithm, Randomforest, stacking algorithmIntroductionEmployee attrition is an important research topic in human resource management, and many scholars have attempted to establish employee attrition classification models using machine learning methods, such as application research of decision tree algorithm in talent attrition of logistics enterprises (Yang & Li, 2017), employee attrition prediction based on database knowledge discovery (Wu, 2019), research on countermeasures for the problem of core employee attrition in state-owned manufacturing enterprises (Qian, 2021), and the application of machine learning in the field of human resource management (Huang, 2022). However, these widely used algorithms have not been able to improve the accuracy of classification models.This paper uses the stacking algorithm to integrate multiple models and establish the final employee attrition classification model, which achieves an accuracy close to 1.0 and performs better than other ensemble learning algorithms in the control experiment. This model can be applied to the field of human resource management to help managers better classify employee attrition.CHEN Yanming, Bachelor degree in Economics, Finance, Department of Applied Economics, Shantou University, Shantou, China.LIN Xinyu, Bachelor degree in Management, Human Resource Management, Department of Management, South China Normal University, Guangzhou, China.ZHAN Kunye, Bachelor of Management, Management Science and Engineering, Department of Management Science, Shenzhen University, Shenzhen, China.DA VID PUBLISHINGDEMPLOYEE ATTRITION CLASSIFICATION MODEL280Theoretical FoundationMachine learning achieves specific tasks by allowing computers to learn from data and automatically adjust algorithms (Jalil, Hwang, & Dawi, 2019). Machine learning algorithms can classify, predict, cluster, or optimize based on the features and goals of the data.Ensemble learning is an algorithm that combines multiple basic machine learning models into a more powerful model. It can improve the accuracy and robustness of the model by weighting or voting the output of multiple models. Ensemble learning can improve the generalization ability of the model by reducing variance and bias.Materials and MethodsDataset Used in the StudyTo investigate and identify the significant factors responsible for employee attrition, and develop a model for classifying employee attrition, this paper employs a dataset created by IBM data scientists available on . This dataset comprises 1,470 observations, each of which represents whether an employee has resigned or not, and also includes various information about the employee. However, this dataset is considered an imbalanced dataset, because it only consists of 237 resignations and 1,233 non-resignations.Based on the information of the employee, an employee attrition classification model can be established. There are 32 variables in this dataset. We eliminated some variables that were not pertinent to our research, leaving us with 30 variables that were ultimately used. Afterward, the variable named “Attrition” serves as the dependent variable, and the other 29 variables are used as independent variables. The independent variables in this dataset contain both numerical and categorical variables, and the distribution of the numerical and categorical variables is shown in Table 1.Table 1The Proportion of Two Categories of VariablesType Number ProportionCategorical 6 20.69%Numerical 23 79.31%The partial information of numerical and categorical variables is shown in Tables 2 and 3, respectively. Table 2Partial Information About Numerical VariablesCount Min Max MeanAge 1,470 18.00 60.00 36.92Daily rate 1,470 102.00 1499.00 802.49Distance from home 1,470 1.00 29.00 9.19Education 1,470 1.00 5.00 2.91Satisfaction 1,470 1.00 4.00 2.72 Performance rating 1,470 3.00 4.00 3.15Total working years 1,470 0.00 40.00 11.28Job involvement 1,470 1.00 4.00 2.73Job level 1,470 1.00 5.00 2.06EMPLOYEE ATTRITION CLASSIFICATION MODEL 281Table 3Rudimentary Information About Categorical VariablesCount Unique Top FreqBusiness travel 1,470 3 Travel rarely 1043Department 1,470 3 Research & development 961Education field 1,470 6 Life sciences 606Gender 1,470 2 Male 882Job role 1,470 9 Sales executive 326Marital status 1,470 3 Married 673MethodsIn this paper, variables are first divided into numerical variables and categorical variables. After scaling the numerical variables and transforming the categorical variables into dummy variables, an oversampling algorithm was employed to address the issue of data imbalance. Subsequently, feature selection was performed using the Point-biserial algorithm and Randomforest feature importance ranking method. Finally, a Stacking algorithm was utilized to integrate multiple models and establish the ultimate employee attrition classification model.Data cleaning and preprocessing. The dataset under consideration is devoid of missing values and outliers. In order to mitigate the issue of overfitting, this paper utilizes the “min-max rescaling”algorithm to scale numerical variables between 0 and 1. The formula for “min-max rescaling” is as follows Equation (1):X′=X−XminXmax−Xmin(1)where X is the original value, X max and X min are the maximum and minimum values, and X' is the transformed feature value.In this paper, all categorical variables are converted into dummy variables using one-hot encoding, which are 0-1 variables.Oversampling algorithm. In this dataset, the dependent variable “Attrition” is represented by “1” for those who have left resigned and “0” for those who have not. However, the proportion of “1” and “0” is severely imbalanced, which may lead to significant errors if a classification model is directly established. In order to address this issue, this paper has adopted the random oversampling algorithm, which is the quickest and simplest method (Wang & Liu, 2020). The data before and after processing is illustrated in Figure 1.Another commonly used algorithm is SMOTE oversampling. However, through comparison, we found that the random oversampling method yields better results for this dataset. This is due to the fact that the SMOTE algorithm can not effectively address the data distribution issue in imbalanced datasets, which may result in marginalization of the data distribution and increase the difficulty of classification algorithms to accurately classify the data.Point-biserial algorithm for feature analysis. This paper conducts Point-biserial correlation analysis on all variables and the target variable “attrition”. In Point-biserial correlation analysis, the correlation represents the relationship between variables, while the p-value indicates the significance level. Variables with a p-value more than 0.05 are usually considered to have no significant correlation with the target variable. The variables with a p-value more than 0.05 are shown in Table 4.EMPLOYEE ATTRITION CLASSIFICATION MODEL282Figure 1. The data before and after oversampling.Table 4The Variables With a p-Value More Than 0.05 in Point-Biserial AnalysisVariables Correlation p-valueManufacturing director 0.030 0.137Performance rating 0.016 0.409Percent salary hike -0.014 0.472Research scientist 0.010 0.617Sales executive 0.006 0.734Hourly rate -0.003 0.843Randomforest feature importance ranking for feature filtering. The point-biserial correlation algorithm can only be employed for preliminary analysis of the correlation between each feature and employee attrition. However, when building a classification model, it is imperative to also consider the intercorrelation among features. Therefore, this paper employs the Randomforest feature importance ranking technique to select the required features for modeling purposes (Wu & Zhang, 2021).The specific process is as follows: Initially, the dataset is divided into training and testing sets in a 7:3 ratio. Subsequently, all processed variables are inputted into a Randomforest classification model. Then, a Randomforest feature importance ranking can be generated, where the sum of the importance of all features is equal to 1. Finally, features with a feature importance of less than 0.005 are filtered out. This process is shown in Figure 2.The partial feature importance ranking generated by the Randomforest classification model is shown inTable 5.EMPLOYEE ATTRITION CLASSIFICATION MODEL 283Figure 2. Process of feature filtering.Table 5The Top Eight Features of the Adaboosting Feature Importance RankingFeature ImportanceMonthly income 0.068Age 0.063Monthly rate 0.051Years at company 0.048Distance from home 0.047Total working years 0.045Percent salary hike 0.042Satisfaction 0.041Model building. The stacking algorithm is a non-linear ensemble process, which involves performing cross-validation (K-fold validation) on each base learner (first-layer model), and training a meta-learner (second-layer model) using the results of the base learners as features (Ni, Tang, & Wang, 2022). Typically, a relatively simple model is selected as the second-layer model. The main process of Stacking algorithm is shown in Figure 3.Figure 3. The main process of Stacking algorithm.EMPLOYEE ATTRITION CLASSIFICATION MODEL284This paper employs random forest and Adaboosting as the control experiments, which are two ensemble learning algorithms. Then, we employ a stacking ensemble approach, with random forest classification model, KNN model, Adaboosting classification model, decision tree model, extreme decision tree model, and logistic regression model as the first-layer models. For the second-layer model, we select a decision tree model.Experiments & ResultsExperiment EnvironmentThe dataset comes from a public database named . This experiment was done in python 3.8.0, and the configuration of the computer is shown in Table 6.Table 6The Configuration of the ComputerHardware Hardware modelCPU Intel core i7 CPU 2.90 GHZRAM 40.0 GBExperiments and ResultsFirstly, comparative experiments are conducted using random forest classification model and Adaboosting model. The random forest classification model is based on decision tree as the base model, and we select the support vector machine (SVM) model as the base model for the Adaboosting model. The results are shown in Table 7.Table 7Experimental Results of Two Classification ModelsTraining set (accuracy) Testing set (accuracy) Testing set (F1-score)Randomforest 1.0 0.985 0.984Adaboosting 1.0 0.982 0.982 Secondly, we conducted experiments using the Stacking algorithm, and the result is shown in Table 8.Table 8Experimental Result of the Stacking AlgorithmTraining set (accuracy) Testing set (accuracy) Testing set (F1-score)The ROC curve of the stacking model is shown in Figure 4.EMPLOYEE ATTRITION CLASSIFICATION MODEL285Figure 4. The ROC curve of the stacking model.From the experimental results, the stacking algorithm can improve the accuracy and F1 score and reduce the risk of overfitting to a certain extent on this dataset.ConclusionsIn this paper, we use oversampling algorithm to address the issue of data imbalance, and employe Stacking algorithm to establish the final employee attrition classification model. Through experimental comparisons, we find that it demonstrated better performance on both the training set and the testing set. However, there are still some flaws. When using the Stacking algorithm, due to the numerous models involved, the process of tuning the model parameters may become more challenging when dealing with more complex datasets.ReferencesHuang, H. L. (2022). The application of machine learning in the field of human resource management. Human ResourcesDevelopment, 23, 92-93.Jalil, N. A., Hwang, H. J., & Dawi, N. M. (2019). Machines learning trends, perspectives and prospects in education sector. InProceedings of the 2019 3rd international conference on education and multimedia technology (pp. 201-205). doi:10.1145/3345120.3345147Ni, P., Tang, K., & Wang, Z. Y. (2022). Research on sentinel-1 sea ice classification based on stacking integrated machine learningmethod. Mine Surveying, 1, 70-77.Qian, J. (2021). Research on countermeasures for the problem of core employee attrition in state-owned manufacturing enterprises.China Market, 32, 19-21.Wang, D., & Liu, Y. (2020). Denoise-based over-sampling for imbalanced data classification. In Proceedings of 2020 19thinternational symposium on distributed computing and applications for business engineering and science (DCABES 2020). Wu, D. (2019). Employee attrition prediction based on database knowledge discovery. Science and Technology & Innovation, 14,16-19.Wu, W. J., & Zhang, J. X. (2021). Feature selection algorithm of random forest based on fusion of classification information andits application. Computer Engineering and Applications, 57, 147-156.Yang, J., & Li, Y. H. (2017). Application research of decision tree algorithm in talent attrition of logistics enterprises. LogisticsEngineering and Management, 8, 154-156.。
- 1、下载文档前请自行甄别文档内容的完整性,平台不提供额外的编辑、内容补充、找答案等附加服务。
- 2、"仅部分预览"的文档,不可在线预览部分如存在完整性等问题,可反馈申请退款(可完整预览的文档不适用该条件!)。
- 3、如文档侵犯您的权益,请联系客服反馈,我们会尽快为您处理(人工客服工作时间:9:00-18:30)。
John C.PlattMicrosoft Research1Microsoft WayRedmond,W A98052jplatt@AbstractTraining a Support Vector Machine(SVM)requires the solution of a verylarge quadratic programming(QP)problem.This paper proposes an al-gorithm for training SVMs:Sequential Minimal Optimization,or SMO.SMO breaks the large QP problem into a series of smallest possible QPproblems which are analytically solvable.Thus,SMO does not requirea numerical QP library.SMO’s computation time is dominated by eval-uation of the kernel,hence kernel optimizations substantially quickenSMO.For the MNIST database,SMO is1.7times as fast as PCG chunk-ing;while for the UCI Adult database and linear SVMs,SMO can be1500times faster than the PCG chunking algorithm.1INTRODUCTIONIn the last few years,there has been a surge of interest in Support Vector Machines (SVMs)[1].SVMs have empirically been shown to give good generalization performance on a wide variety of problems.However,the use of SVMs is still limited to a small group of researchers.One possible reason is that training algorithms for SVMs are slow,especially for large problems.Another explanation is that SVM training algorithms are complex, subtle,and sometimes difficult to implement.This paper describes a new SVM learning algorithm that is easy to implement,often faster,and has better scaling properties than the standard SVM training algorithm.The new SVM learning algorithm is called Sequential Minimal Optimization(or SMO).1.1OVERVIEW OF SUPPORT VECTOR MACHINESA general non-linear SVM can be expressed as(1) where is the output of the SVM,is a kernel function which measures the similarity of a stored training example to the input,is the desired output of the classifier,is a threshold,and are weights which blend the different kernels[1].For linear SVMs,the kernel function is linear,hence equation(1)can be expressed as(2) where.Training of an SVM consists offinding the.The training is expressed as a minimization of a dual quadratic form:cannot handle large-scale training problems,because even this reduced matrix cannotfit into memory.Kaufman[3]has described a QP algorithm that does not require the storage of the entire Hessian.The decomposition technique[6]is similar to chunking:decomposition breaks the large QP problem into smaller QP sub-problems.However,Osuna et al.[6]suggest keeping a fixed size matrix for every sub-problem,deleting some examples and adding others which violate the KKT ing afixed-size matrix allows SVMs to be trained on very large training sets.Joachims[2]suggests adding and subtracting examples according to heuristics for rapid convergence.However,until SMO,decomposition required the use of a numerical QP library,which can be costly or slow.2SEQUENTIAL MINIMAL OPTIMIZATIONSequential Minimal Optimization quickly solves the SVM QP problem without using nu-merical QP optimization steps at all.SMO decomposes the overall QP problem intofixed-size QP sub-problems,similar to the decomposition method[7].Unlike previous methods,however,SMO chooses to solve the smallest possible optimiza-tion problem at each step.For the standard SVM,the smallest possible optimization prob-lem involves two elements of because the must obey one linear equality constraint.At each step,SMO chooses two to jointly optimize,finds the optimal values for these, and updates the SVM to reflect these new values.The advantage of SMO lies in the fact that solving for two can be done analytically. Thus,numerical QP optimization is avoided entirely.The inner loop of the algorithm can be expressed in a short amount of C code,rather than invoking an entire QP library routine. By avoiding numerical QP,the computation time is shifted from QP to kernel evaluation. Kernel evaluation time can be dramatically reduced in certain common situations,e.g., when a linear SVM is used,or when the input data is sparse(mostly zero).The result of kernel evaluations can also be cached in memory[1].There are two components to SMO:an analytic method for solving for the two,and a heuristic for choosing which multipliers to optimize.Pseudo-code for the SMO algo-rithm can be found in[8,7],along with the relationship to other optimization and machine learning algorithms.2.1SOLVING FOR TWO LAGRANGE MULTIPLIERSTo solve for the two Lagrange multipliers and,SMOfirst computes the constraints on these multipliers and then solves for the constrained minimum.For convenience,all quan-tities that refer to thefirst multiplier will have a subscript1,while all quantities that refer to the second multiplier will have a subscript2.Because there are only two multipliers, the constraints can easily be displayed in two dimensions(seefigure1).The constrained minimum of the objective function must lie on a diagonal line segment.The ends of the diagonal line segment can be expressed quite simply in terms of.Let .The following bounds apply to:(7) Under normal circumstances,the objective function is positive definite,and there is a min-imum along the direction of the linear equality constraint.In this case,SMO computes the minimum along the direction of the linear equality constraint:where is the error on the th training example.As a next step,the constrainedminimum is found by clippinginto the interval .The value of is then computed from the new,clipped,:(9)For both linear and non-linear SVMs,the threshold is re-computed after each step,so that the KKT conditions are fulfilled for both optimized examples.2.2HEURISTICS FOR CHOOSING WHICH MULTIPLIERS TO OPTIMIZE In order to speed convergence,SMO uses heuristics to choose which two Lagrange multi-pliers to jointly optimize.There are two separate choice heuristics:one forand one for .The choice of provides the outer loop of the SMO algorithm.If an example is found to violate the KKT conditions by the outer loop,it is eligible for optimization.The outer loop alternates single passes through the entire training set with multiple passes through the non-bound ().The multiple passes terminate when all of the non-bound examples obey the KKT conditions within .The entire SMO algorithm terminates when the entire training setobeys the KKT conditions within .Typically,.The first choice heuristic concentrates the CPU time on the examples that are most likely to violate the KKT conditions,i.e.,the non-bound subset.As the SMO algorithm progresses,that are at the bounds are likely to stay at the bounds,while that are not at the bounds will move as other examples are optimized.As a further optimization,SMO uses the shrinking heuristic proposed in [2].After the pass through the entire training set,shrinking finds examples which fulfill the KKT conditions more than the worst example failed the KKT conditions.Further passes through the training set ignore these fulfilled conditions until a final pass at the end of training,which ensures that every example fulfills its KKT condition.Once anis chosen,SMO chooses an to maximize the size of the step taken during joint optimization.SMO approximates the step size by the absolute value of the numerator in equation (8):.SMO keeps a cached error value for every non-bound example in the training set and then chooses an error to approximately maximize the step size.If is positive,SMO chooses an example with minimum error .If is negative,SMO chooses an example with maximum error .More documents and datum download websiteLu Zhenbo's Blog: /luzhenbo2Communication & Cooperation: luzhenbo@Kernel Sparse Kernel Training Number of C%Used Used Size Vectors Inputs AdultLinLinear N mix1122141580.050 WebLinLinear N mix49749172310 AdultGaussKGaussian Y N112214206189 AdultGaussKDGaussian N N11221420610 WebGaussKGaussian Y N497494484596 WebGaussKDGaussian N N49749448450 MNISTTable1:Parameters for various experimentsSMO Chunking SMO Chunking(sec)(sec)(sec)Exponent Exponent Exponent AdultLin21.9n/a21141.1 1.0n/a 3.0339.93980.817164.7 1.6 2.2 2.5 WebLinDAdultGaussK523.3737.5n/a 2.0 2.0n/a1433.0n/a14740.4 2.5n/a 2.8 AdultGaussDWebGaussK2538.06923.5n/a 1.6 1.8n/a23365.3n/a50371.9 2.6n/a 2.0 WebGaussDMNISTTable2:Timings of algorithms on various data sets.tions of two-dimensional sub-problems,while uses numerical QP to solve 10-dimensional sub-problems.The difference in timings between the two methods is partlydue to the numerical QP overhead,but mostly due to the difference in heuristics and kerneloptimizations.For example,SMO is faster thanby an order of magnitude on linear problems,due to linear SVM folding.However,can also potentially use linear SVM folding.In these experiments,SMO uses a very simple least-recently-used ker-nel cache of Hessian rows,while uses a more complex kernel cache and modifies its heuristics to utilize the kernel effectively [2].Therefore,SMO does not benefit from thekernel cache at the largest problem sizes,whilespeeds up by a factor of 2.5.Utilizing sparseness to compute kernels yields a large advantage for SMO due to the lack of heavy numerical QP overhead.For the sparse data sets shown,SMO can speed up by a factor of between 3and 13,while PCG chunking only obtained a maximum speed up of2.1times.The MNIST experiments were performed without a kernel cache,because the MNIST data set takes up most of the memory of the benchmark machine.Due to sparse inputs,SMO is a factor of 1.7faster than PCG chunking,even though none of the Lagrange multipliers areat .On a machine with more memory,would be as fast or faster than SMO for MNIST,due to kernel caching.In summary,SMO is a simple method for training support vector machines which does not require a numerical QP library.Because its CPU time is dominated by kernel evaluation,SMO can be dramatically quickened by the use of kernel optimizations,such as linear SVM folding and sparse dot products.SMO can be anywhere from 1.7to 1500times faster than the standard PCG chunking algorithm,depending on the data set.AcknowledgementsThanks to Chris Burges for running data sets through his projected conjugate gradient code and for various helpful suggestions.References[1]C.J.C.Burges.A tutorial on support vector machines for pattern recognition.DataMining and Knowledge Discovery ,2(2),1998.[2]T.Joachims.Making large-scale SVM learning practical.In B.Sch¨o lkopf,C.J.C.Burges,and A.J.Smola,editors,Advances in Kernel Methods —Support Vector Learning ,pages 169–184.MIT Press,1998.[3]L.Kaufman.Solving the quadratic programming problem arising in support vectorclassification.In B.Sch¨o lkopf,C.J.C.Burges,and A.J.Smola,editors,Advances in Kernel Methods —Support Vector Learning ,pages 147–168.MIT Press,1998.[4]Y .LeCun.MNIST handwritten digit database.Available on the web at http:///˜yann/ocr/mnist/.[5]C.J.Merz and P.M.Murphy.UCI repository of machine learning databases,1998.[/mlearn/MLRepository.html].Irvine,CA:University of Cali-fornia,Department of Information and Computer Science.[6]E.Osuna,R.Freund,and F.Girosi.Improved training algorithm for support vectormachines.In Proc.IEEE Neural Networks in Signal Processing ’97,1997.[7]J.C.Platt.Fast training of SVMs using sequential minimal optimization.InB.Sch¨o lkopf,C.J.C.Burges,and A.J.Smola,editors,Advances in Kernel Meth-ods —Support Vector Learning ,pages 185–208.MIT Press,1998.More documents and datum download website Lu Zhenbo's Blog: /luzhenbo2Communication & Cooperation: luzhenbo@[8]J.C.Platt.Sequential minimal optimization:A fast algorithm for training support vec-tor machines.Technical Report MSR–TR–98–14,Microsoft Research,1998.Availableat /˜jplatt/smo.html.[9]V .Vapnik.Estimation of Dependences Based on Empirical Data .Springer-Verlag,1982.More documents and datum download website Lu Zhenbo's Blog: /luzhenbo2Communication & Cooperation: luzhenbo@。