Hierarchical overlapped neural gas network with application to pattern classification
基于深度残差网络的医学图像鲁棒可逆水印算法
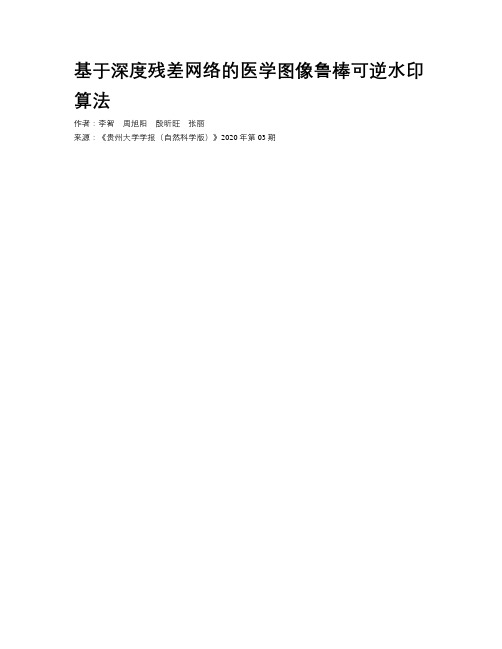
基于深度残差网络的医学图像鲁棒可逆水印算法作者:李智周旭阳殷昕旺张丽来源:《贵州大学学报(自然科学版)》2020年第03期摘要:醫学图像作为医生诊断的重要依据,其版权保护一直是研究重点。
在对医学图像进行版权保护时,必须保证图像修改后能无损恢复。
但是,当前大多数应用于医学图像的可逆水印算法并未考虑鲁棒性。
因此,本文针对具有一定鲁棒性的可逆水印算法进行研究,提出一种基于深度残差网络ResNet的鲁棒可逆医学图像水印算法。
首先,算法利用深度残差模型提取医学图像的深度特征信息,自适应确定最优嵌入强度,均衡水印的不可见性和鲁棒性;此外,结合遗传算法和模糊C-均值的聚类算法对水印区域动态划分,根据聚类结果提取水印信息,有效克服信号攻击对含水印图像的影响,提高可逆水印算法的鲁棒性。
实验结果表明:在嵌入水印后,含水印医学图像的PSNR值都可以达到36 dB以上,具有较好的图像质量;在提取水印后,算法可以完全无损恢复医学图像;在受到高斯噪声、椒盐噪声、JPEG压缩等常见信号攻击后,算法仍然可以准确提取水印信息,表现出较强的鲁棒性。
关键词:医学图像;版权保护;深度残差网络;聚类算法;鲁棒可逆水印中图分类号:TP309文献标识码: A随着网络技术的快速发展,智能医学和远程诊断技术日趋成熟。
大量医学图像经常在网络上进行传输和使用,未授权者可轻易通过网络获取、存储、使用和篡改网络上的医学图像[1]。
因而,保护医学图像的版权信息显得十分重要。
数字水印算法是一种常用的信息隐藏技术,可用于医学图像的版权保护[2]。
在对医学图像进行保护时,为了不影响医生的诊断,不可破坏原始医学图像的信息,因此,基于医学图像的可逆水印算法成为研究者们关注的重点。
郑洪英等[3]提出了基于位平面的可逆信息隐藏算法。
首先,将医学图像分解为八位平面,通过压缩最高的四位平面获得对空间进行像素填充后的重建图像;其次,分别对重建图像的头部、中间、尾部进行加密;再次,利用直方图移位的方法将水印信息嵌入图像中。
几类高斯过程Karhunen-Loève展开及再生核希尔伯特空间
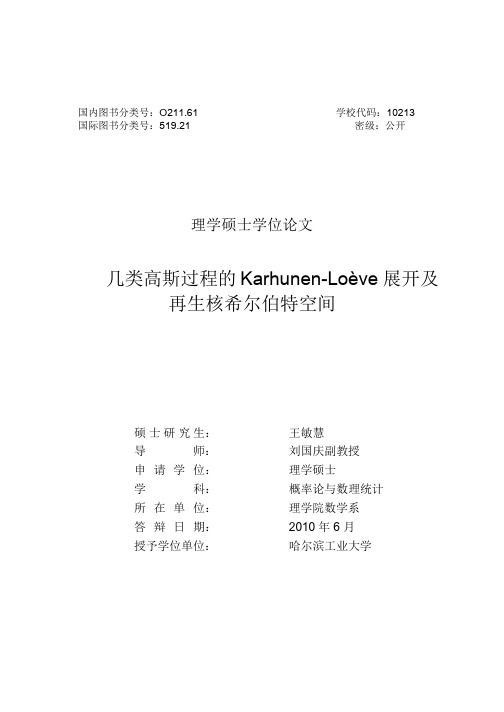
哈尔滨工业大学理学硕士学位论文
(2)ThereproducingkernelHilbertspaceofGaussianprocess:Firstlywe
expandandcompletethesectionofthereproducingkerneltoconstructthe
reproducingkernelHilbertspace;SecondlyweuseMercer’stheoremandhave
topicofGaussianrandomprocessandthereproducingkernelHilbertspace,and
mainlyforzeromeanGaussianprocess,byusingMercer’stheoremandthe
reproducingkernelHilbertspacetheoryweyieldKarhunen-Loèveexpansionsand
1.1.1理论的产生与发展..............................................................................1
1.1.2本文研究的目的和意义.......................................................................1
decomposition,kernelfunction.TheresearchofKarhunen-Loèveexpansionand
reproducingkernelHilbertspaceofGaussianprocessallowsus tograspexactlythe
seriesexpansionsofsomeGaussianprocessesandthecoefficientsoftheseriesis
研究NLP100篇必读的论文---已整理可直接下载
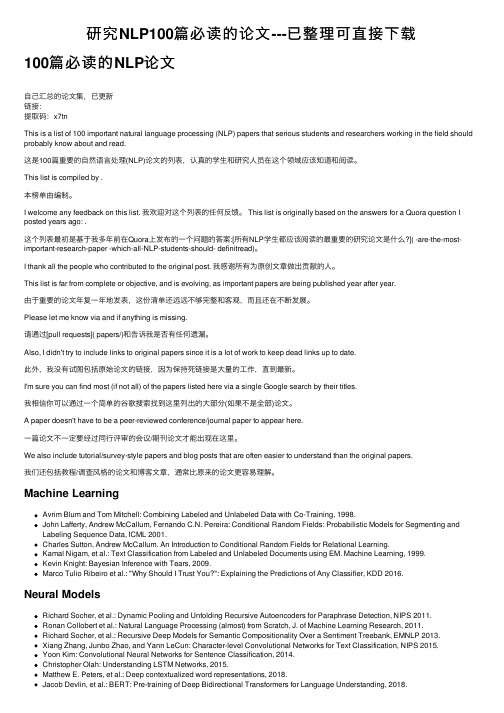
研究NLP100篇必读的论⽂---已整理可直接下载100篇必读的NLP论⽂⾃⼰汇总的论⽂集,已更新链接:提取码:x7tnThis is a list of 100 important natural language processing (NLP) papers that serious students and researchers working in the field should probably know about and read.这是100篇重要的⾃然语⾔处理(NLP)论⽂的列表,认真的学⽣和研究⼈员在这个领域应该知道和阅读。
This list is compiled by .本榜单由编制。
I welcome any feedback on this list. 我欢迎对这个列表的任何反馈。
This list is originally based on the answers for a Quora question I posted years ago: .这个列表最初是基于我多年前在Quora上发布的⼀个问题的答案:[所有NLP学⽣都应该阅读的最重要的研究论⽂是什么?]( -are-the-most-important-research-paper -which-all-NLP-students-should- definitread)。
I thank all the people who contributed to the original post. 我感谢所有为原创⽂章做出贡献的⼈。
This list is far from complete or objective, and is evolving, as important papers are being published year after year.由于重要的论⽂年复⼀年地发表,这份清单还远远不够完整和客观,⽽且还在不断发展。
基于深度学习的磁共振图像重建和波谱相位校正方法

基于生成对抗网络的图像重建方法
数据生成
利用GAN生成高质量的磁共振图 像,作为训练集的一部分。
网络结构设计
设计生成对抗网络结构,包括生 成器和判别器,实现对图像特征
的提取和重建。
模型训练
利用训练集对模型进行训练,通 过反向传播算法调整参数,提高 模型的重建效果。同时,通过博 弈过程优化生成器的生成能力和
基于深度学习的磁共振图像重建 和波谱相位校正方法
汇报人: 2023-11-26
目录
• 引言 • 基于深度学习的磁共振图像重建方法 • 基于深度学习的磁共振波谱相位校正方法 • 实验结果和分析 • 研究结论和展望 • 参考文献
01
引言
研究背景和意义
01
磁共振成像技术的优势
磁共振成像技术具有无辐射、高分辨率、对软组织对比度高等优点,在
判别器的鉴别能力。
03
基于深度学习的磁共振波谱相 位校正方法
磁共振波谱相位校正原理和方法
磁共振波谱相位校正原理
磁共振波谱相位是反映样本内部磁性粒子分布的重要参数, 通过对接收到的磁共振信号进行相位校正,可以获得样本内 部磁性粒子的空间分布情况。
磁共振波谱相位校正方法
磁共振波谱相位校正方法主要包括去噪、寻峰和相位校正三 个步骤。去噪主要是去除背景噪声和固定频率的干扰,寻峰 主要是寻找信号中的主峰,相位校正则是根据主峰位置和其 他信息计算出磁性粒子的空间分布。
基于循环神经网络的波谱相位校正方法
数据预处理
网络模型训练
利用预处理后的磁共振信号作为输入,通过反向传 播算法训练RNN模型,以获得最佳的权重参数。
对接收到的磁共振信号进行预处理,包括去 噪、寻峰等步骤,以减少干扰和噪声对模型 训练的影响。
云南大中山黑颈长尾雉取食生境模拟分析英文
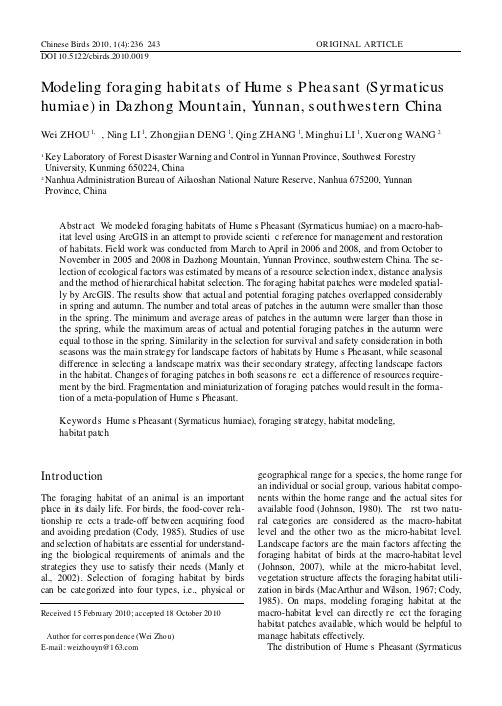
Chinese Birds 2010,1(4):236–243DOI 10.5122/cbirds.2010.0019ORIGINAL ARTICLEReceived 15February 2010;accepted 18October 2010f (W Z )z y @63Modeling foraging habitats of Hume ’s P heasant (Syrmaticus humiae)in Dazhong Mountain,Y unnan,southwestern ChinaWei ZHOU 1,,Ning LI 1,Zhongjia n DENG 1,Qing ZHANG 1,Minghui LI 1,Xuer ong WANG 21Key Laboratory of Forest Disaster Warning and Control in Y unnan Province,Southwest Forestry University,Kunming 650224,China 2Nanhua Administration Bureau of Ailaoshan National Nature Reserve,Nanhua 675200,Y unnan Province,ChinaAbstr act W e modeled foraging habitats of Hume ’s Pheasant (Syrmaticus humiae)on a macro-hab-itat level using ArcGIS in an attempt to provide scienti c reference for management and restoration of habitats.Field work was conducted from March to April in 2006and 2008,and from October to November in 2005and 2008in Dazhong Mountain,Y unnan Province,southwestern China.The se-lection of ecological factors was estimated by means of a resource selection index,distance analysis and the method of hierarchical habitat selection.The foraging habitat patches were modeled spatial-ly by ArcGIS.The results show that actual and potential foraging patches overlapped considerably in spring and autumn.The number and total areas of patches in the autumn were smaller than those in the spring.The minimum and average areas of patches in the autumn were larger than those in the spring,while the maximum areas of actual and potential foraging patches in the autumn were equal to those in the spring.Similarity in the selection for survival and safety consideration in both seasons was the main strategy for landscape factors of habitats by Hume ’s Pheasant,while seasonal difference in selecting a landscape matrix was their secondary strategy,affecting landscape factors in the habitat.Changes of foraging patches in both seasons re ect a difference of resources require-ment by the bird.Fragmentation and miniaturization of foraging patches would result in the forma-tion of a meta-population of Hume ’s Pheasant.Keywords Hume ’s Pheasant (Syrmaticus humiae),foraging strategy,habitat modeling,habitat patchIntroductionThe foraging habitat of an animal is an important place in its daily life.For birds,the food-cover rela-tionship re ects a trade-off between acquiring food and avoiding predation (Cody,1985).Studies of use and selection of habitats are essential for understand-ing the biological requirements of animals and the strategies they use to satisfy their needs (Manly et al.,2002).Selection of foraging habitat by birds can be categorized into four types,i.e.,physical orgeographical range for a species,the home range for an individual or social group,various habitat compo-nents within the home range and the actual sites for available food (Johnson,1980).The rst two natu-ral categories are considered as the macro-habitat level and the other two as the micro-habitat ndscape factors are the main factors affecting the foraging habitat of birds at the macro-habitat level (Johnson,2007),while at the micro-habitat level,vegetation structure affects the foraging habitat utili-zation in birds (MacArthur and Wilson,1967;Cody,1985).On maps,modeling foraging habitat at the macro-habitat level can directly re ect the foraging habitat patches available,which would be helpful to ff y T f ’(Sy Author or correspon dence ei ho u E-mail:wei ho u n manage habitats e ectivel .he distribution o Hume s Pheasant rmaticusW ei Zhou et al.Modeling foraging habitat of Hume’s Pheasant237humiae)is restricted to China,India,Myanmar and Thailand.The species has been listed as“globally near-threatened”(BirdLife International,2010).Its reproduction,feeding and habitat selection were studied in terms of food,water and human distur-bance(Jiang et al.,2006;Li et al.,2006;Iamsiri and Gale,2008).Based on these earlier studies and com-bining eld data with ArcGIS analyses,we modeled the foraging habitat patches of Hume’s Pheasant in Dazhong Mountain,Y unnan Province,China,which can have important implications for future manage-ment of Hume’s Pheasant in dealing with its core habitat and establishing foraging corridors. MethodsStudy areaDazhong Mountain(24°43′32″–25°01′10″N,100°44′28″–100°57′42″E)is located in the southwestern part of Nanhua County,Chuxiong Prefecture in cen-tral Y unnan,China.This area lies at the con uence of the central Y unnan Plateau,Hengduan mountains and the southern tip of the Qinghai-Tibetan Plateau. The study area consists of mid-alpine mountains and valleys caused by age-old movements in the Earth’s crust.These upward movements of the Earth resulted in modi ed soils and climate regimes which in turn have affected the vegetation and species diversity and distribution.P inus yunnanensis and scrub forests dominate in areas below1500m elevation,semi-moist evergreen broad-leaved forests and deciduous broad-leaved forests are found at elevations between 1500–2400m,while the vegetation above2400m comprises mid-alpine evergreen broad-leaved and Pinus armandii forests.A total of356bird species were recorded in the area,including nine species of Galliformes,such as Syrmaticus humiae,Povo muti-cus and Lophura nycthemera(W ang,2000).Basic map digit alizationA topographic map(1:50000)was matched with re-mote sensing images.Some factors such as terrain, rivers,small roads and residential areas,which are of obvious biological importance for Hume’s Pheasant (Liu et al.,1999),were digitalized.The vector cover-age for these factors was formed and transformed to the grid-layer on a GRID background.R f yy f3per(TM)data.According to Wu and Zhu(1987), the vegetation was divided into ten types:mountain-top mossy coppice,semi-humid evergreen broad-leaved forests,mid-mountain moist evergreen broad-leaved forests,deciduous broad-leaved forests,Pinus a rmandii forests,P.yunna nensis forests,shrub-grassland with sparse trees,A lbizzia julibrissin-Rho-dodendron forests,economic forests and farmland. The remote sensing images were superimposed on the topographic map to form a base map of Hume’s Pheasant habitat.A confusion matrix was construct-ed using GPS point-site data,by which the general interpretation precision to the vegetation was83.5%. Observat ion of foraging habitatMicroscopic analysis of feces indicated that the available food sources for Hume’s Pheasant in the spring include Fagaceae and Theaceae plants and ferns(Li et al.,2009),which are abundant in the study area(Wang,2000).Ecological factors were collected from March to April in2006and2008and from October to No-vember in2005and2008.Our earlier studies found that Hume’s Pheasants are found at2200–2500m elevations and were actively foraging between08:00–11:00and17:00–19:00hours(Li et al.,2006).W e set four5m wide and4–6km long transects at this elevation in our study area,frequented by pheas-ants.Two investigators walked along the lines dur-ing08:00–11:00and17:00–19:00hours and sought foraging traces of this pheasant,which consist of long,narrow pits,approximately4–6cm deep(Li et al.,2006).Once a foraging trace was located,it was marked as the center of a20m×20m plot,es-tablished for investigating ecological factors within it.Factors could be categorized into two types:1) habitat factors which include slope,aspect,vegeta-tion type and distance from plot center to water and 2)disturbance factors which comprise the distance from plot center to residential areas and small roads (small roads are often used by village farmers for grazing their cattle).Assessment of foraging habitatResource selection functionResource selection functions are standard methods f yz f(I,6),T fem ote sensing im ages o vegetation t pes in the stud area originated rom the200thematic map-or anal ing habitat actors vlev191which we used in our assessment.he value o each ecologicalChinese Birds 2010,1(4):236–243238factor changed in each range and could be dividedinto two equal parts,where the utilization and avail-ability of factors at either level for this bird were estimated (Garshelis,2000).Resource utilization (R i )refers to the frequency of actual utilization by animals (birds in this case)us-ing resource i in a period.R i =U i /Uwhere U i represents the utilization times of resource i,and U the total amount of resource i.Resource availability (N i )refers to the availability of animals using resource i.N i =S i /Swhere S i is the available area of resource i and S the total area of this type of resource.A resource selection function (E)is an index,used for comparing resource utilization with resource availability,in order to identify the resources select-ed by the bird (Manly et al.,2002).E i =(R iN i )/(R i +N i )If E i =0,the birds do not select resource i and given the value “0”;E i <0implies that birds avoid resource i,expressed as “–”and E i >0means that the birds prefer resource i,expressed as “+”(Ivlev,1961).Analysis of disturbance factorsDistance analysis was used to evaluate human dis-turbance of the pheasant,following the method of Hebblewhite and Merrill (2008).Human activities,such as grazing cattle,harvesting medicinal herbs and non-timber products,disturb wildlife and theirhabitats (Liu et al.,1999).For human activities,birds often keep a minimum warning distance.Birds will escape and/or hide when people are close.Within this minimum warning distance,few activities of birds are found (Geist et al.,2005).W e took small roads and residential areas as disturbance centers in our study.Disturbance factors were evaluated using the following procedures.First,a minimum distance from the disturbance center to traces of bird activity was measured on the map and the distance divided into two equal ranks.Second,the level of anthropo-genic activity was estimated according to the number of traces of bird activities:disturbance was regarded as “intense ”if no traces of bird activity could be f ,“”–5,“”6–“”f larger than 10.Third,the disturbance distance for the level referred to as “none ”was considered the minimum warning distance for the bird and a map of the disturbance range was drawn using the point of a road or residential area as its center and the mini-mum warning distance as its radius.Assessment of foraging habitatMethods of hierarchical habitat selection are excel-lent means for estimating the requirements of ani-mals at different habitat levels (Johnson,1980).W e used the following procedures.First,we estimated the requirements for habitat by the pheasant,given the combined results of a resource selection function and an analysis of the disturbance factors.Second,a multi-scale foraging habitat classification was established according to the habitat requirements of Hume ’s Pheasant,both at a macro-habitat and a micro-habitat level.Modeling of foraging habitatAn ecological niche is a multiple resource space required by animals.If in this space,one resource cannot satisfy the basic requirement of animals,i.e.,if the tness of this resource is zero,then the tness of the entire ecological niche would be zero.By overlapping several single-resource image layers inArcGIS,a comprehensive,superimposed layer isgenerated to re ect the multiple requirements for resources by animals (Liu et al.,1999).In our case,modeling foraging habitats were established as fol-lows.1)Modeling of habitat factors.First,the tness characteristics of individual habitat factors were ana-lyzed.Then the tness characteristics for all habitat factors were analyzed according to the characteris-tics of each factor,used to model the potential for-aging habitat of Hume ’s Pheasant.In modeling,the threshold of a minimum habitat area is an important index for estimating the smallest amount in terms of requirement for resources in different seasons.There have been no such reports of S.humiae so far in China.Therefore,the spring data of S.ellioti from Zhejiang Province,China (Cai et al.,2007)and the autumn data of S.humiae from Doi Chinang Dao,northern Thailand (Iamsiri and Gale,2008)were taken as reference for determination of a threshold S f S y ound moderate with 1traces weak with 10traces and none when the number o traces was value..ellioti is a congeneric close relative o .humiae with similar morpholog and behavior .W ei Zhou et al.Modeling foraging habitat of Hume ’s Pheasant 239Garshelis (2000)once used the data of closely relat-ed species as reference for his own study in a habitat model.2)Modeling of disturbance factors.The range of disturbance was estimated according to characteris-tics of each disturbance factor,such as small roads and residential areas and a map of these factors was drawn.3)Modeling of foraging habitat.Habitat and dis-turbance factors were evaluated.A map of the actual foraging habitat of this pheasant was modeled by overlapping layers.Our data were analyzed by ArcView 3.3and Arc-GIS 9.2.ResultsDuring the collection of spring data,from March to April in 2006and 2008,a total of 76foraging traces of Hume ’s Pheasant were identi ed,while in the au-tumn data collection,from October to November in 2005and 2008,we identi ed 52traces.Analysis of the resource selection function showed that selectivity for habitat factors by this bird was consistent during the spring and autumn,but only deciduous broad-leaved forests were used randomly in the spring (Table 1).Analysis of disturbance fac-tors showed that effects of anthropogenic activities were consistent during spring and autumn (Table 2).Based on the results of a resource selection index,we acquired a multi-scale foraging habitat system ofHume ’s Pheasant in Dazhong Mountain.Spring:Forests in the southwestSlopes of 25–50°in the east and northMiddle-mountain,moist evergreen broad-leaved forestDistance to water:0–200m;distur-bance radius from small roads:10–100m;disturbance radius from resi-dential areas:500mMinimum patch area:<2.40ha (minimum habitat area require-ment for Syrmaticus in spring)(Cai et al.,2007)Autumn:Forests in the southwestSlopes of 25–50°in the east and northMiddle-mountain,moist evergreen broad-leaved forestDistance to water:0–200m;distur-bance radius from small roads:10–100m;disturbance radius from resi-dential areas:500mMinimum patch area:<4.90ha (minimum habitat area require-ment for Syrmaticus in autumn)(Iamsiri and Gale,2008)Comparing the habitat characteristics with the multi-scale foraging habitat system of our pheasant,patches of potential and actual foraging habitats in the autumn overlapped with those in the spring.The number of patches and areas of actual autumn habitat were smaller than those in the spring.The minimumT a ble 1Resource selection function of foraging habitat factors of Hume ’s Pheasant in spring and autumn Factori R iN iE iSelective SpringAutumn Spring Autumn Spring Autumn Spring Autumn SpringAutumn ASEast East 0.570.670.280.280.340.31++SouthSouth 0.0300.240.240.78 1.00W est W est 0.070.010.240.240.710.92NorthNorth 0.330.320.240.240.130.12++SL (°)0–250–250.380.120.530.530.160.6325–5025–500.620.880.470.470.140.30++VTMEBF MEBF 0.87 1.000.490.490.280.34++DBF DBF 0.0500.050.050 1.000YPFYPF0.0800.460.460.70 1.00DSW (m)0–2000–2000.550.700.520.520.030.15++200–400200–4000.450.300.480.480.030.23AS:aspect;DBF:deciduous broad –leaved forest;DSW:distance to water;MEBF:middle mountain moist evergreen broad-f ;SL ;VT y ;Y F Y (y )f ;+yx ;y x ;y leaved orest :slope :vegetation t pe P :unnan pine Pinus unnanensis orest :observed usage signi cantl higher than e pected :observed usage signi cantl lower than e pected 0:observed usage in proportion to its availabilit .Chinese Birds2010,1(4):236–243 240number and mean area of patches in the autumn were larger than those in the spring.Only the maximum areas of potential and actual habitats in the autumn were similar with those in the spring.If smaller than 4.90ha,the patch would not be repeatedly used by this bird in the autumn.There were a number of more or less isolated patches of potential and actual habitats(Table3;Figs.1and2).DiscussionSelect ion st rategy for landscape habitat factor s The similarity for survival and safety requirements in both seasons was the main selection strategy for landscape habitat factors of Hume’s Pheasant.Habi-tat and disturbance factors in the landscape made up its survival and safety conditions.There were no clear differences in selection of habitat and distur-bance factors between spring and autumn(Table3), so that patches of potential and actual spring habitats overlapped considerably with those in the autumn (Figs.1and2).It may be an effective strategy for use of space by this bird.When it selected similar foraging patches among seasons,the bird would not spend much time learning new environmental patch-es and could therefore spend more time breeding and feeding(Cody,1985).Hence,the use of overlapping foraging patches by the pheasant in different seasons is an effective strategy for the use of space.The selection of a different landscape matrix among seasons is a secondary strategy affecting the choice of habitat landscape factors by this bird.V egetation can often be divided into suitable vegeta-tion and a landscape matrix used by birds(Carroll and Fox,2008).Suitable vegetation is a re ection of the nutritional and safety conditions provided by the environment(Cody,1985).In the spring,this pheas-ant randomly used deciduous broad-leaved forests but not during the autumn(Table2).It may be that this type of forest provides the pheasant with a cor-ridor to move among several patches for mating. Food was also one of the main factors that limited dispersion of Syrmaticus according to Liu and Zhang (2008).Deciduous broad-leaved forests can provide a suf cient supply of food when the bird disperses. Therefore,deciduous broad-leaved forests can be used at will.In the autumn,individual pheasants form groups and accumulate energy for wintering, where the abundance of food resources becomes a precondition for energy accumulation to live safely through the winter.Therefore,this bird prefers to use patches where resources are more abundant and the area larger,rather than the earlier dispersion corri-dors.So the difference in landscape matrix selection during the seasons can be considered an intermittent strategic use of a habitat corridor in both seasons. Of course,other possibilities cannot readily be ruled out,since there has been a severe habitat fragmenta-tion in the study area.The repeated use of a foraging habitat by this pheasant in both seasons has presum-ably become an adaptive form of behavior,a forced behavior of selecting and utilizing foraging habitats (W atson et al.,2005),which needs attention in future research.T a ble2Effect of anthropogenic factors on foraging habitat of Hume’s Pheasant in spring and autumnfactorsAnthropogenic effect(m)Strong Moderate Weak None Spring Autumn Spring Autumn Spring Autumn Spring AutumnResidential area0–2500–250––250–500250–500>500>500Small road10–5510–5555–10055–100–>100or0–10>100or0–10T a ble3Comparison between potential and actual foraging habitats of Hume’s Pheasant in spring and autumntypeNumber ofpatchesPatch area(ha)Max.area(ha)Min.area(ha)Mean patcharea(ha)Per cent of totalpatch area(%) Spring Autumn Spring Autumn Spring Autumn Spring Autumn Spring Autumn Spring AutumnPotential habitat5629588.48497.2286.9086.90 2.51 5.4810.5117.14 2.32 1.96 3353333335353 Actual habitat9202.12 4.94 1.4 1.4 2.49.178.411.7 1.280.9W ei Zhou et al.Modeling foraging habitat of Hume ’s Pheasant 241Fragment ation of foraging patches and met a-populat ionFragmentation and small areas of foraging patches fy y f ’D z Mountain.According to a theory of island biogeog-raphy,a large habitat area can provide large amountsof resources and support a great number of species.The amount of resources often determines the size f (M W ,6)G,Fig.1Potential and actual foraging habitat patches of Hume ’s Pheasant in spring and autumn at Dazhong Mountain,Y unnan.(a )Foraging habitat patches in spring;(b)Foraging habitat patches inautumn.Fig.2Part of potential and actual foraging habitat of Hume ’s Pheasant in spring and autumn at Dazhong Mountain,Y unnan.(a)Foraging habitat in spring;(b)Foraging habitat in autumn.were the main reasons leading to the reduction o carr ing capacit o Hume s Pheasant in a hongo a bird population acArthur and ilson 197.iv en human disturbances the total number andChinese Birds2010,1(4):236–243 242area as well as the mean area of actual foraging habi-tats could be greatly reduced and would be less than those of potential foraging habitats(Table3).Using the total area of habitats to divide the requirement of the minimum habitat area,we can obtain an ideal population size of47.9–245.2in space.The spatial carrying capacity of Hume’s Pheasant is rather low. Therefore,most potential habitats are not suitable for their presence,considering human disturbances. Fragmentation and small areas of foraging patches would result in the formation of meta-populations of Hume’s Pheasants(Fig.2)and likely result in an increase in the rate of extinction of this bird in this area.This probability has two causes.On the one hand,the actual foraging patches are more or less isolated,without any individual exchanges of birds among patches when the extent of isolation was beyond the capacity of the pheasant to disperse;as a result,its population would decline due to inbreed-ing,in the end giving rise to a meta-population. On the other hand,fragmentation and small areas of foraging patches directly cause a decline in the spatial carrying capacity at Dazhong Mountain for the pheasant and again,a meta-population would be formed.A study of the rate of extinction of species in fragmented forests in an area of the Amazon Basin also showed that over the long term,the reduction of the number and areas of habitat patches would lead to meta-populations,which obviously causes an in-crease in the rate of extinction of species in the area (Ferraz et al.,2003).Therefore,in the future,atten-tion should be paid to the effect of fragmentation and small areas of foraging patches for Hume’s Pheasant in Dazhong Mountain.Acknowledgements W e express our thanks to Mr.Paul But-ler,Senior Vice President of the Global Programs of RARE, an inspiring conservationist and to Prof.Zhengwang Zhang from Beijing Normal University,who reviewed our manu-script and provided some constructive suggestions.The eld work was nanced by the Wildlife Conservation Program in 2009,administered by the State Forestry Administration of China and,as well,supported as a key subject by the Wildlife Conservation and Utilization Program in Yunnan Province (No.XKZ200904).ReferencesBirdLife International.2010.Hume’s Pheasant(Sy r maticus )f z f ?=6O Cai LY,Xu YP,Jiang PP,Ding P,Y ao XH,Xu XY,W ang GB.2007.Home range and daily moving distance of Elliot’s pheasant.J Zhejiang Univ(Sci Edit),34(6):679–683.(in Chinese with English abstract)Carroll SP,Fox CW.2008.Conservation Biology:Evolution in Action.Oxford University Press,New Y ork.Cody ML.1985.Habitats Selection in Birds.Academic Press, London.Ferraz G,Russell GJ,Stouffer PC,Blerregaard RO,Plmm SL, Lovejoy TE.2003.Rates of species loss from Amazonian forest fragments.Proceed Natl Acad Sci,100(24):14069–14073.Garshelis DL.2000.Delusions in habitat evaluation:measur-ing use,selection,and importance.In:Boitani L,Fuller TK (eds)Research T echniques in Animal Ecology:Controver-sies and Consequences.Columbia University Press,New Y ork,pp111–164.Geist C,Liao J,Libby S,Blumstein DT.2005.Does intruder group size and orientation affect ight initiation distance in birds?Animal Biodiv Conserv,28(1):69–73.Hebblewhite M,Merrill E.2008.Modeling wildlife-human relationships for social species with mixed-effects resource selection models.J Appl Ecol,45(3):834–844.Iamsiri A,Gale GA.2008.Breeding season habitat use by Hume’s pheasant Syramticus humiae in the Doi Chinang Dao wildlife sanctuary,northern Thailand.Zool Stud, 47(2):138–145.Ivlev VS.1961.Experimental Ecology of the Feeding of Fishes.Yale University Press,New Haven.Jiang A W,Zhou F,Lu Z,Han XJ,Sun RJ,Li XL.2006.Roost-site Selection of Mrs Hume’s Pheasant(Syrmaticus humiae) in Guangxi,China.Zool Res,27(3):249–254.(in Chinese with English abstract)Johnson DH.1980.The comparison of usage and availability measurements for evaluations of resource preference.Ecol-ogy,61:65–71.Johnson DM.2007.Measuring habitat quality:a review.Con-dor,109:489–504.Li N,Zhou W,Y ang YY,Zhang Q,Liu Z.2009.Microcopic analysis on winter plant food of Syrmaticus humiae(Hume’s pheasant)in Dazhongshan,Yunnan.J Zhejiang Forest Coll, 26(3):363–367.(in Chinese with English abstract)Li W,Zhou W,Zhang XY,Cao M,Zhang RG.2006.Spring foraging sites of three pheasa nts at Nanhua Pa rt in Ailaoshan National Nature Reserve.Zool Res,27(5):495–504.(in Chinese with English abstract)Liu JG,Ouyang ZY,Taylor WW,Groop R,Tan YC,Zhang HM.1999.A framework for evaluating the effects of hu-man factors on wildlife habitat:the case of Giant Pandas.B,336–3L Y,Z ZW Rhumiae.http://www.birdli /data one/species actsheet. php id28.Accessed12ctober2010.Conserv iol1:10170.iu hang.2008.esearch progress in avian dispersalW ei Zhou et al.Modeling foraging habitat of Hume’s Pheasant243behavior.Acta Ecol Sin,28(4):1354–1365.MacArthur RH,Wilson EO.1967.The Theory of Island Bio-geography.Princeton University Press,Princeton.Manly BFJ,McDonald LL,Tomas DL,McDonald TL,Erick-son WP.2002.Resource Selection by Animals:Statistical Design and Analysis for Field Studies.Kluwer Academic Press,London.W ang YK.2000.Integrated Investigation Report on Dazhong-shan Provincial Natural Reserve,Nanhua County.Nanhua Forest Bureau,Nanhua.(in Chinese)Watson JEM,Whittaker RJ,Freudenberger D.2005.Bird community responses to habitat fragmentation:how consis-tent are they across landscape?J Biogeogr,32:1353–1370. Wu ZY,Zhu CY.1987.Y unnan V egetation.Science Press, Beijing.(in Chinese)云南大中山黑颈长尾雉取食生境模拟分析周伟1,李宁1,邓忠坚1,张庆1,李明会1,王学荣2(1西南林业大学,云南省森林灾害预警与控制重点实验室,昆明,650224;2哀牢山国家级自然保护区南华管理局,南华,675200)摘要:我们用ArcGIS从宏生境尺度模拟黑颈长尾雉(Syrmaticus humiae)的取食生境,期望为该物种的科学管理和生境恢复提供科学指导。
基于改进随机游走的复杂网络节点重要性评估
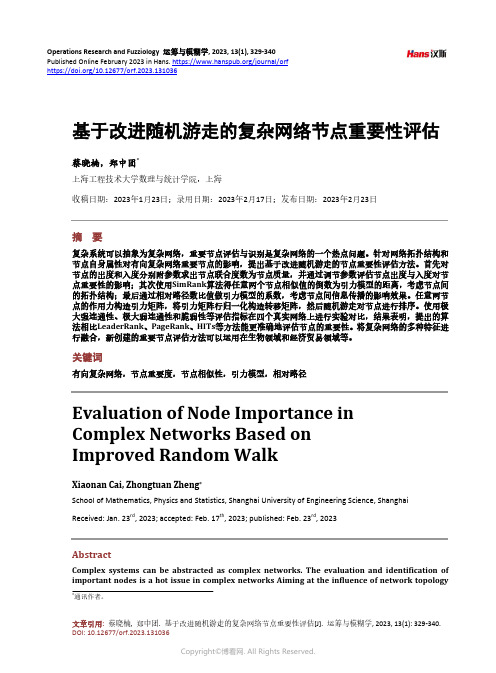
Operations Research and Fuzziology 运筹与模糊学, 2023, 13(1), 329-340 Published Online February 2023 in Hans. https:///journal/orf https:///10.12677/orf.2023.131036基于改进随机游走的复杂网络节点重要性评估蔡晓楠,郑中团*上海工程技术大学数理与统计学院,上海收稿日期:2023年1月23日;录用日期:2023年2月17日;发布日期:2023年2月23日摘要复杂系统可以抽象为复杂网络,重要节点评估与识别是复杂网络的一个热点问题。
针对网络拓扑结构和节点自身属性对有向复杂网络重要节点的影响,提出基于改进随机游走的节点重要性评估方法。
首先对节点的出度和入度分别附参数求出节点联合度数为节点质量,并通过调节参数评估节点出度与入度对节点重要性的影响;其次使用SimRank 算法得任意两个节点相似值的倒数为引力模型的距离,考虑节点间的拓扑结构;最后通过相对路径数比值做引力模型的系数,考虑节点间信息传播的影响效果。
任意两节点的作用力构造引力矩阵,将引力矩阵行归一化构造转移矩阵,然后随机游走对节点进行排序。
使用极大强连通性、极大弱连通性和脆弱性等评估指标在四个真实网络上进行实验对比,结果表明,提出的算法相比LeaderRank 、PageRank 、HITs 等方法能更准确地评估节点的重要性。
将复杂网络的多种特征进行融合,新创建的重要节点评估方法可以运用在生物领域和经济贸易领域等。
关键词有向复杂网络,节点重要度,节点相似性,引力模型,相对路径Evaluation of Node Importance in Complex Networks Based on Improved Random WalkXiaonan Cai, Zhongtuan Zheng *School of Mathematics, Physics and Statistics, Shanghai University of Engineering Science, Shanghai Received: Jan. 23rd, 2023; accepted: Feb. 17th, 2023; published: Feb. 23rd, 2023AbstractComplex systems can be abstracted as complex networks. The evaluation and identification of important nodes is a hot issue in complex networks Aiming at the influence of network topology*通讯作者。
基于多级全局信息传递模型的视觉显著性检测
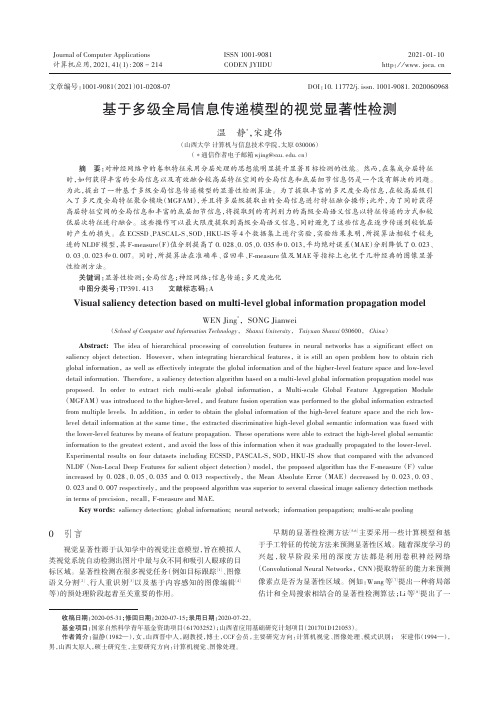
2021⁃01⁃10计算机应用,Journal of Computer Applications 2021,41(1):208-214ISSN 1001⁃9081CODEN JYIIDU http ://基于多级全局信息传递模型的视觉显著性检测温静*,宋建伟(山西大学计算机与信息技术学院,太原030006)(∗通信作者电子邮箱wjing@ )摘要:对神经网络中的卷积特征采用分层处理的思想能明显提升显著目标检测的性能。
然而,在集成分层特征时,如何获得丰富的全局信息以及有效融合较高层特征空间的全局信息和底层细节信息仍是一个没有解决的问题。
为此,提出了一种基于多级全局信息传递模型的显著性检测算法。
为了提取丰富的多尺度全局信息,在较高层级引入了多尺度全局特征聚合模块(MGFAM ),并且将多层级提取出的全局信息进行特征融合操作;此外,为了同时获得高层特征空间的全局信息和丰富的底层细节信息,将提取到的有判别力的高级全局语义信息以特征传递的方式和较低层次特征进行融合。
这些操作可以最大限度提取到高级全局语义信息,同时避免了这些信息在逐步传递到较低层时产生的损失。
在ECSSD 、PASCAL -S 、SOD 、HKU -IS 等4个数据集上进行实验,实验结果表明,所提算法相较于较先进的NLDF 模型,其F -measure (F )值分别提高了0.028、0.05、0.035和0.013,平均绝对误差(MAE )分别降低了0.023、0.03、0.023和0.007。
同时,所提算法在准确率、召回率、F -measure 值及MAE 等指标上也优于几种经典的图像显著性检测方法。
关键词:显著性检测;全局信息;神经网络;信息传递;多尺度池化中图分类号:TP391.413文献标志码:AVisual saliency detection based on multi -level global information propagation modelWEN Jing *,SONG Jianwei(School of Computer and Information Technology ,Shanxi University ,Taiyuan Shanxi 030600,China )Abstract:The idea of hierarchical processing of convolution features in neural networks has a significant effect onsaliency object detection.However ,when integrating hierarchical features ,it is still an open problem how to obtain rich global information ,as well as effectively integrate the global information and of the higher -level feature space and low -leveldetail information.Therefore ,a saliency detection algorithm based on a multi -level global information propagation model was proposed.In order to extract rich multi -scale global information ,a Multi -scale Global Feature Aggregation Module(MGFAM )was introduced to the higher -level ,and feature fusion operation was performed to the global information extracted from multiple levels.In addition ,in order to obtain the global information of the high -level feature space and the rich low -level detail information at the same time ,the extracted discriminative high -level global semantic information was fused with the lower -level features by means of feature propagation.These operations were able to extract the high -level global semantic information to the greatest extent ,and avoid the loss of this information when it was gradually propagated to the lower -level.Experimental results on four datasets including ECSSD ,PASCAL -S ,SOD ,HKU -IS show that compared with the advanced NLDF (Non -Local Deep Features for salient object detection )model ,the proposed algorithm has the F -measure (F )valueincreased by 0.028、0.05、0.035and 0.013respectively ,the Mean Absolute Error (MAE )decreased by 0.023、0.03、0.023and 0.007respectively ,and the proposed algorithm was superior to several classical image saliency detection methods in terms of precision ,recall ,F -measure and MAE.Key words:saliency detection;global information;neural network;information propagation;multi -scale pooling引言视觉显著性源于认知学中的视觉注意模型,旨在模拟人类视觉系统自动检测出图片中最与众不同和吸引人眼球的目标区域。
基于复杂网络理论的大型换热网络节点重要性评价
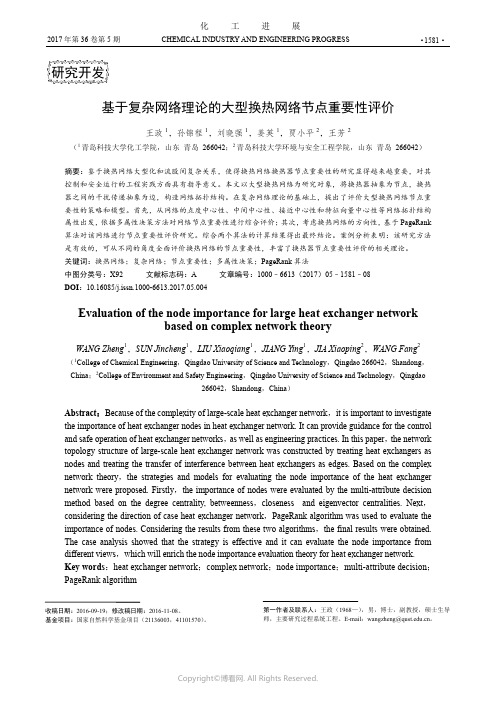
2017年第36卷第5期 CHEMICAL INDUSTRY AND ENGINEERING PROGRESS·1581·化 工 进展基于复杂网络理论的大型换热网络节点重要性评价王政1,孙锦程1,刘晓强1,姜英1,贾小平2,王芳2(1青岛科技大学化工学院,山东 青岛 266042;2青岛科技大学环境与安全工程学院,山东 青岛 266042) 摘要:鉴于换热网络大型化和流股间复杂关系,使得换热网络换热器节点重要性的研究显得越来越重要,对其控制和安全运行的工程实践方面具有指导意义。
本文以大型换热网络为研究对象,将换热器抽象为节点,换热器之间的干扰传递抽象为边,构造网络拓扑结构。
在复杂网络理论的基础上,提出了评价大型换热网络节点重要性的策略和模型。
首先,从网络的点度中心性、中间中心性、接近中心性和特征向量中心性等网络拓扑结构属性出发,依据多属性决策方法对网络节点重要性进行综合评价;其次,考虑换热网络的方向性,基于PageRank 算法对该网络进行节点重要性评价研究。
综合两个算法的计算结果得出最终结论。
案例分析表明:该研究方法是有效的,可从不同的角度全面评价换热网络的节点重要性,丰富了换热器节点重要性评价的相关理论。
关键词:换热网络;复杂网络;节点重要性;多属性决策;PageRank 算法中图分类号:X92 文献标志码:A 文章编号:1000–6613(2017)05–1581–08 DOI :10.16085/j.issn.1000-6613.2017.05.004Evaluation of the node importance for large heat exchanger networkbased on complex network theoryWANG Zheng 1,SUN Jincheng 1,LIU Xiaoqiang 1,JIANG Ying 1,JIA Xiaoping 2,WANG Fang 2(1College of Chemical Engineering ,Qingdao University of Science and Technology ,Qingdao 266042,Shandong ,China ;2College of Environment and Safety Engineering ,Qingdao University of Science and Technology ,Qingdao266042,Shandong ,China )Abstract :Because of the complexity of large-scale heat exchanger network ,it is important to investigate the importance of heat exchanger nodes in heat exchanger network. It can provide guidance for the control and safe operation of heat exchanger networks ,as well as engineering practices. In this paper ,the network topology structure of large-scale heat exchanger network was constructed by treating heat exchangers as nodes and treating the transfer of interference between heat exchangers as edges. Based on the complex network theory ,the strategies and models for evaluating the node importance of the heat exchanger network were proposed. Firstly ,the importance of nodes were evaluated by the multi-attribute decision method based on the degree centrality, betweenness ,closeness and eigenvector centralities. Next ,considering the direction of case heat exchanger network ,PageRank algorithm was used to evaluate the importance of nodes. Considering the results from these two algorithms ,the final results were obtained. The case analysis showed that the strategy is effective and it can evaluate the node importance from different views ,which will enrich the node importance evaluation theory for heat exchanger network.Key words :heat exchanger network ;complex network ;node importance ;multi-attribute decision ;PageRank algorithm第一作者及联系人:王政(1968—),男,博士,副教授,硕士生导师,主要研究过程系统工程。
基于 HESSIAN 增强和形态学尺度空间的视网膜血管分割
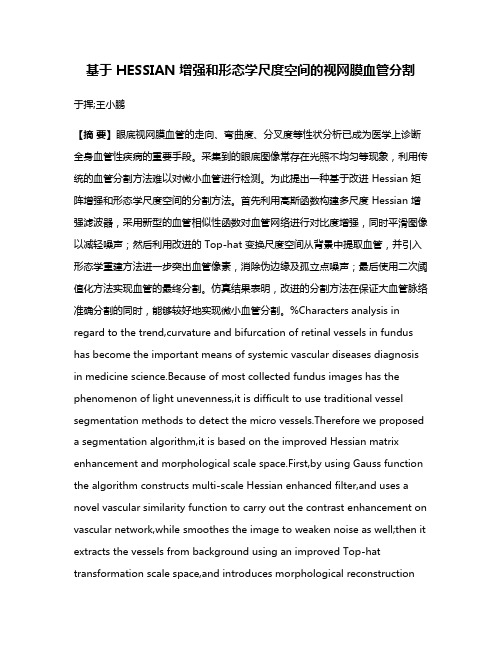
基于 HESSIAN 增强和形态学尺度空间的视网膜血管分割于挥;王小鹏【摘要】眼底视网膜血管的走向、弯曲度、分叉度等性状分析已成为医学上诊断全身血管性疾病的重要手段。
采集到的眼底图像常存在光照不均匀等现象,利用传统的血管分割方法难以对微小血管进行检测。
为此提出一种基于改进 Hessian 矩阵增强和形态学尺度空间的分割方法。
首先利用高斯函数构建多尺度 Hessian 增强滤波器,采用新型的血管相似性函数对血管网络进行对比度增强,同时平滑图像以减轻噪声;然后利用改进的 Top-hat 变换尺度空间从背景中提取血管,并引入形态学重建方法进一步突出血管像素,消除伪边缘及孤立点噪声;最后使用二次阈值化方法实现血管的最终分割。
仿真结果表明,改进的分割方法在保证大血管脉络准确分割的同时,能够较好地实现微小血管分割。
%Characters analysis in regard to the trend,curvature and bifurcation of retinal vessels in fundus has become the important means of systemic vascular diseases diagnosis in medicine science.Because of most collected fundus images has the phenomenon of light unevenness,it is difficult to use traditional vessel segmentation methods to detect the micro vessels.Therefore we proposed a segmentation algorithm,it is based on the improved Hessian matrix enhancement and morphological scale space.First,by using Gauss function the algorithm constructs multi-scale Hessian enhanced filter,and uses a novel vascular similarity function to carry out the contrast enhancement on vascular network,while smoothes the image to weaken noise as well;then it extracts the vessels from background using an improved Top-hat transformation scale space,and introduces morphological reconstructionmethod to further highlight the vascular pixels and to eliminate the pseudo-edges and the noise of outliers;finally the algorithm uses secondary thresholding approach to realise final vessel segmentation. Simulation experimental results showed that while ensuring the accurate segmentation of great vessels and choroid,the improved segmentation method can better realise the segmentation of micro vessels.【期刊名称】《计算机应用与软件》【年(卷),期】2016(033)008【总页数】6页(P200-205)【关键词】视网膜血管;Hessian 增强;尺度空间;形态学分割【作者】于挥;王小鹏【作者单位】兰州交通大学电子与信息工程学院甘肃兰州 730070;兰州交通大学电子与信息工程学院甘肃兰州 730070【正文语种】中文【中图分类】TP391.4近年来,由于临床诊断全身血管类疾病的需要,国内外学者针对视网膜血管的增强和分割提取进行了大量研究。
核磁高级谱的作图及解析应用
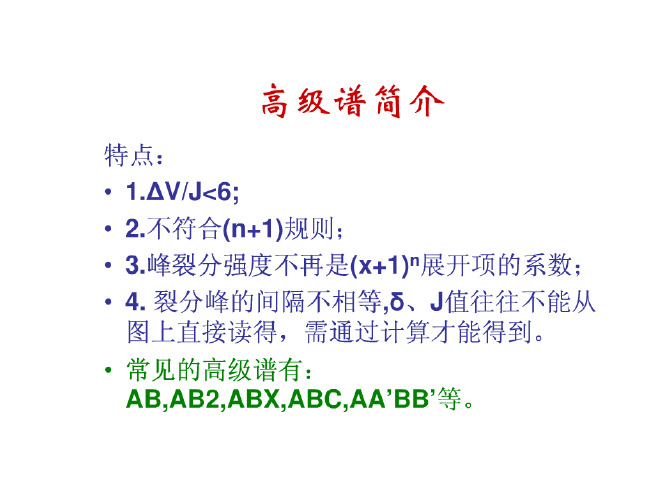
例化合物C9H12S的1H-NMR谱如图,推其结构式。 CH3-CH-CH3 Ph
=4, 1.4ppm双峰,6个H,峰间距与 3.3ppm处七 个峰(1个H)的间距相同,表明互相耦合,故有基 团:CH3-CH-CH3。 7~7.5ppm有5个H,可能有 苯环,而且是单取代苯。故结构式如上。
例3. 根据下面谱图推出分子C9H10O的结构式。
乙烯型的J值 3J =11.6Hz 不饱和度 =6 顺 3J =19.1Hz 7.2ppm附近单峰(5个H): 单取代苯。 反 10.9ppm处单峰 (1个H), 且分子式中又有2个O: -COOH 还剩C2H2及一个不饱和度。 5.5 (1H)与 7.8 (1H) 两组双峰, 间距相同, AX系统,C2H2是 烯,且反式(根据乙烯型的J值判断)。
本资料只限于学习交流,严禁用于商业用途!!! 分析化学,测试技术交流,学习,由微信公众号“分析测试圈”[I D : i fenxi ceshi ]制作, 欢迎关注,获取更多资料
高级图谱的简化方法: • 加大仪器的磁场强度 • 去偶法 • 加入位移试剂等
本资料只限于学习交流,严禁用于商业用途!!! 分析化学,测试技术交流,学习,由微信公众号“分析测试圈”[I D : i fenxi ceshi ]制作, 欢迎关注,获取更多资料
本资料只限于学习交流,严禁用于商业用途!!! 分析化学,测试技术交流,学习,由微信公众号“分析测试圈”[I D : i fenxi ceshi ]制作, 欢迎关注,获取更多资料
芳香质子耦合系统
大部分芳香质子偶合系统的图谱是高级谱。但 从大量实验数据中可归纳出一些经验规则 : (1)单取代苯的取代基没有强烈的屏蔽或去屏 蔽效应时,出现五个芳氢的单峰。 (2)对位取代时,若是相同的取代基,不论取 代基的电负性强弱如何,由于分子的对称性,在 苯环上的四个芳氢为磁等价,出现的是单峰。
欧洲药典7.5版
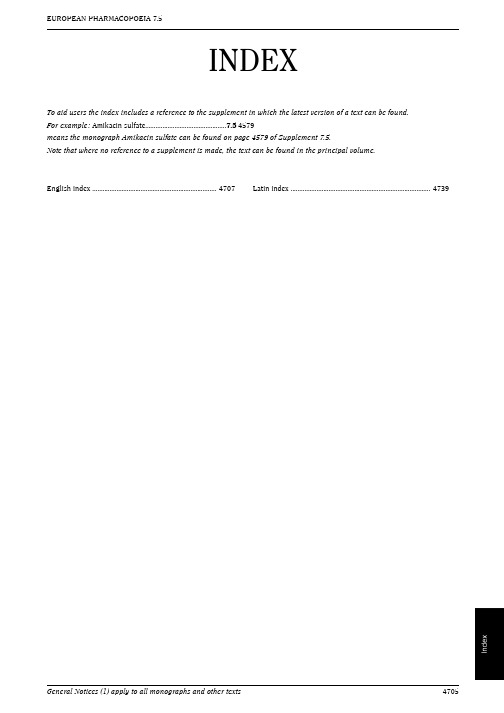
INDEX
To aid users the index includes a reference to the supplement in which the latest version of a text can be found. For example : Amikacin sulfate...............................................7.5-4579 means the monograph Amikacin sulfate can be found on page 4579 of Supplement 7.5. Note that where no reference to a supplement is made, the text can be found in the principal volume.
English index ........................................................................ 4707
Latin index ................................................................................. 4739
EUROPEAN PHARMACOPபைடு நூலகம்EIA 7.5
Index
Numerics 1. General notices ................................................................... 7.5-4453 2.1.1. Droppers...................
融合注意力机制的孪生网络目标跟踪算法研究

2021578近年来,目标跟踪逐渐成为计算机视觉领域的热点课题,它被广泛地应用在视频监控、自动驾驶、人机交互和医学诊疗等众多领域。
目前,目标跟踪面临诸多挑战,比如跟踪目标的尺度变化、跟踪漂移以及背景杂乱等。
因此,设计一个准确率和稳健性较高的算法成为目标跟踪研究的重点。
主流的跟踪算法分为相关滤波类算法和深度学习类算法。
在相关滤波算法中,KCF (Kernelized Correla-tion Filter )[1]使用循环矩阵扩大样本容量,提高分类器的准确性。
成悦等[2]提出使用加权方法融合多种特征,增强算法的鲁棒性。
在深度学习算法中,Bertinetto 等[3]提出基于相似度匹配的目标跟踪算法SiamFC (Fully-Convolutional Siamese Networks )。
SiamFC 使用离线训练的网络模型,通过比较输入图像和模板图像的相似度大小来得到跟踪结果。
以SiamFC 为基础,RepresentationLearning for Correlation Filter (CFNet )[4]将相关滤波器作为一个网络层嵌入到孪生网络中,加强对深度特征的学习。
在SiamFC 中,模板图像由简单裁剪得到,背景也被当作正样本来和检测图像匹配。
这可能会导致:(1)当模板图像中背景与前景外观较为相似的时候,背景可能获得更大的相似度评分从而导致跟踪漂移。
(2)在目标运动过程中背景发生多次变化时,算法可能会跟踪到与模板中背景相似的目标上。
此外,SiamFC 使用最深层的卷积特征,缺乏对目标底层颜色及纹理信息的学习。
最近,注意力思想被融合到目标跟踪中,用来提高算法的准确率。
其中,CSR-DCF (Discriminative Corre-融合注意力机制的孪生网络目标跟踪算法研究王玲,王家沛,王鹏,孙爽滋长春理工大学计算机科学技术学院,长春130022摘要:在全卷积孪生网络跟踪算法(SiamFC )的基础上,提出一种融合注意力机制的孪生网络目标跟踪算法。
基于混沌和细胞自动机的图像加密算法
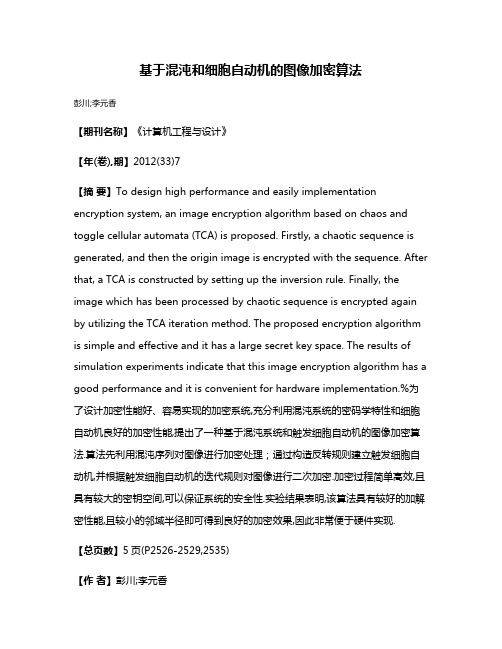
基于混沌和细胞自动机的图像加密算法彭川;李元香【期刊名称】《计算机工程与设计》【年(卷),期】2012(33)7【摘要】To design high performance and easily implementation encryption system, an image encryption algorithm based on chaos and toggle cellular automata (TCA) is proposed. Firstly, a chaotic sequence is generated, and then the origin image is encrypted with the sequence. After that, a TCA is constructed by setting up the inversion rule. Finally, the image which has been processed by chaotic sequence is encrypted again by utilizing the TCA iteration method. The proposed encryption algorithm is simple and effective and it has a large secret key space. The results of simulation experiments indicate that this image encryption algorithm has a good performance and it is convenient for hardware implementation.%为了设计加密性能好、容易实现的加密系统,充分利用混沌系统的密码学特性和细胞自动机良好的加密性能,提出了一种基于混沌系统和触发细胞自动机的图像加密算法.算法先利用混沌序列对图像进行加密处理;通过构造反转规则建立触发细胞自动机,并根据触发细胞自动机的迭代规则对图像进行二次加密.加密过程简单高效,且具有较大的密钥空间,可以保证系统的安全性.实验结果表明,该算法具有较好的加解密性能,且较小的邻域半径即可得到良好的加密效果,因此非常便于硬件实现.【总页数】5页(P2526-2529,2535)【作者】彭川;李元香【作者单位】武汉大学软件工程国家重点实验室,湖北武汉430072;中南民族大学计算中心,湖北武汉430074;武汉大学软件工程国家重点实验室,湖北武汉430072【正文语种】中文【中图分类】TP309【相关文献】1.基于耦合混沌系统和细胞自动机的图像加密算法 [J], 彭川;李元香2.基于混沌细胞自动机的图像加密算法 [J], 张统权;何建农3.基于2D Arnold混沌映射和初等细胞自动机的图像加密算法 [J], 成建宏;朱从旭;牛梦佳;张天然4.基于分数阶超混沌的混沌细胞自动机图像加密算法 [J], 梁晏慧; 李国东5.OFDM-PON系统中基于混沌的细胞自动机加密算法 [J], 卓先好;楼丁溧;毕美华;胡志蕊因版权原因,仅展示原文概要,查看原文内容请购买。
多层密度界面的拟BP神经网络反演方法

多层密度界面的拟BP神经网络反演方法刘展1赵文举2相鹏1(1.中国石油大学(华东)地球资源与信息学院,东营,257061 (2.东方地球物理勘探有限责任公司综合物化探事业部,涿州,072751)摘要提出一种根据重力异常反演三维密度界面分布的反演模式。
该模式将拟BP神经网络与重力反演理论结合,与传统神经网络不同的是拟BP神经网络不需要进行训练,而是直接求取隐层中的物性值。
该模式应用于合成数据集可以发现三维密度界面能够被很好的复原。
最后,利用该方法反演了冲绳海槽南部第三系底与莫霍面深度。
关键词三维重力反演,密度界面,拟BP神经网络,冲绳海槽南,莫霍面,第三系基底1、引言根据重力异常求取三维密度界面的几何形态是重力数据解释工作的一个主要目标。
目前存在很多种不同的算法,例如,Oldenburg(1974)对Parker(1973)提出的非均匀层状介质正演公式重新推导得到根据已知重力异常求取密度界面深度的反演公式。
Rao等(1999)利用邻接直立棱柱体模型根据重力异常或者基底构造求取三维密度界面深度。
人工神经网络已经被成功地应用于地球物理数据处理和反演问题当中。
例如,测井数据解释(Wiener等,1991;Huang等1996),反射地震数据处理(Ashida,1996),近地表电磁成像模式识别(Poulton等,1992),密度界面反演(Taylor,Vasco,1991;朱自强,1995)等。
尽管取得了进步,但是众所周知的是神经网络的反演结果很大程度上取决于训练数据,所以训练数据的选择是决定神经网络性能的关键。
当训练数据与观测数据的模式存在很大差异时,神经网络会求出不合理的结果。
管志宁(1998)将BP神经网络与重磁异常反演相结合提出了一种新的反演算法—拟BP神经网络。
隐层中的物性值可以直接求出而不需要传统神经网络的训练过程。
本文提出一种迭代拟BP神经网络三维密度界面反演算法。
首先,简要回顾一下三维密度界面的正演模型;然后详细介绍拟BP神经网络三维密度界面反演算法;接着利用合成数据分析算法的性能;最后用该方法求取南冲绳海槽盆地的第三系基底和莫霍面深度。
基于混合双层自组织径向基函数神经网络的优化学习算法

基于混合双层自组织径向基函数神经网络的优化学习算法杨彦霞;王普;高学金;高慧慧;齐泽洋【期刊名称】《北京工业大学学报》【年(卷),期】2024(50)1【摘要】针对传统方法采用先训练后测试两阶段学习机制极易导致的过拟合或欠拟合问题,提出一种基于混合双层自组织径向基函数神经网络的优化学习(hybrid bilevel self-organizing radial basis function neural network optimization learning,Hb-SRBFNN-OL)算法。
首先,将训练过程和测试过程集成到一个统一的框架中,规避过拟合或欠拟合问题。
其次,基于进化学习机制,提出上下2层的交互式优化学习算法,上层基于网络复杂度和测试误差自组织调整网络结构,下层采用列文伯格-马夸尔特(Levenberg Marquardt,LM)算法作为优化器对自组织径向基函数神经网络(self-organizing radial basis function neural network,SO-RBFNN)的连接权值进行优化。
最后,利用来自多个子网络的综合信息生成模型的最终输出,加速网络全局收敛。
为验证所提方法的可行性,分别在多个分类和预测任务中进行了测试实验。
结果表明,在与传统神经网络结构相似甚至更好的测试和分类精度下,该方法不仅能实现更快的训练收敛,而且能进化成更精简紧凑的径向基函数神经网络(radial basis function neural network,RBFNN)模型。
尤其在污水处理过程中总磷的质量浓度预测实验中,测试集中均方根误差(root mean squared error,RMSE)最高可降低48.90%,实际场景实验结果验证了所提算法的精确性更佳且泛化能力更强。
【总页数】12页(P38-49)【作者】杨彦霞;王普;高学金;高慧慧;齐泽洋【作者单位】北京工业大学信息学部;北京工业大学计算智能与智能系统北京市重点实验室【正文语种】中文【中图分类】TP273【相关文献】1.基于径向基函数神经网络和NLJ优化算法的精馏塔控制2.径向基函数神经网络的新型混合递推学习算法3.基于混合学习算法的径向基函数神经网络设计4.一种基于径向基函数神经网络参数优化的ICA-RBF神经网络算法因版权原因,仅展示原文概要,查看原文内容请购买。
基于残差注意力融合和门控信息蒸馏的图像修复
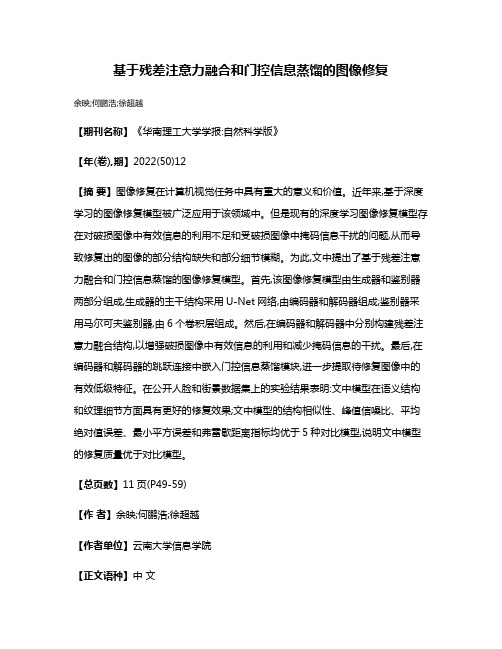
基于残差注意力融合和门控信息蒸馏的图像修复余映;何鹏浩;徐超越【期刊名称】《华南理工大学学报:自然科学版》【年(卷),期】2022(50)12【摘要】图像修复在计算机视觉任务中具有重大的意义和价值。
近年来,基于深度学习的图像修复模型被广泛应用于该领域中。
但是现有的深度学习图像修复模型存在对破损图像中有效信息的利用不足和受破损图像中掩码信息干扰的问题,从而导致修复出的图像的部分结构缺失和部分细节模糊。
为此,文中提出了基于残差注意力融合和门控信息蒸馏的图像修复模型。
首先,该图像修复模型由生成器和鉴别器两部分组成,生成器的主干结构采用U-Net网络,由编码器和解码器组成;鉴别器采用马尔可夫鉴别器,由6个卷积层组成。
然后,在编码器和解码器中分别构建残差注意力融合结构,以增强破损图像中有效信息的利用和减少掩码信息的干扰。
最后,在编码器和解码器的跳跃连接中嵌入门控信息蒸馏模块,进一步提取待修复图像中的有效低级特征。
在公开人脸和街景数据集上的实验结果表明:文中模型在语义结构和纹理细节方面具有更好的修复效果;文中模型的结构相似性、峰值信噪比、平均绝对值误差、最小平方误差和弗雷歇距离指标均优于5种对比模型,说明文中模型的修复质量优于对比模型。
【总页数】11页(P49-59)【作者】余映;何鹏浩;徐超越【作者单位】云南大学信息学院【正文语种】中文【中图分类】TP391.4【相关文献】1.基于注意力与残差级联的红外与可见光图像融合方法2.基于残差门控循环卷积和注意力机制的端到端光学乐谱识别方法3.基于融合残差与注意力机制的图像去雨网络4.基于注意力机制的残差密集网络红外/可见光图像融合5.基于拆分注意力残差网络的红外和可见光图像融合算法因版权原因,仅展示原文概要,查看原文内容请购买。
采用核Rayleigh商二次相关滤波器的星图自适应杂波抑制
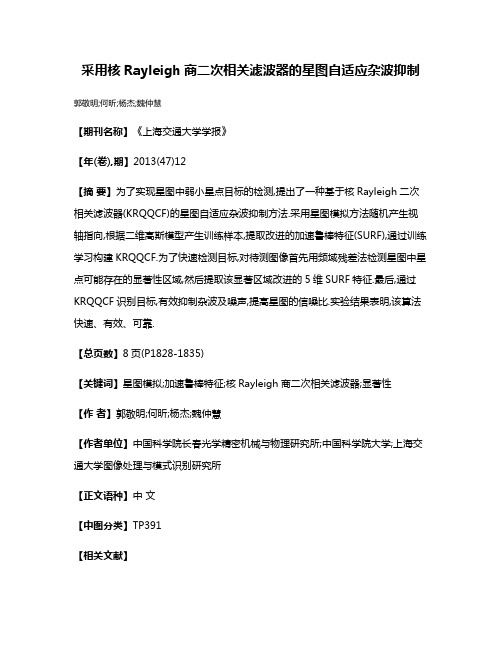
采用核Rayleigh商二次相关滤波器的星图自适应杂波抑制郭敬明;何昕;杨杰;魏仲慧
【期刊名称】《上海交通大学学报》
【年(卷),期】2013(47)12
【摘要】为了实现星图中弱小星点目标的检测,提出了一种基于核Rayleigh二次相关滤波器(KRQQCF)的星图自适应杂波抑制方法.采用星图模拟方法随机产生视轴指向,根据二维高斯模型产生训练样本,提取改进的加速鲁棒特征(SURF),通过训练学习构建KRQQCF.为了快速检测目标,对待测图像首先用频域残差法检测星图中星点可能存在的显著性区域,然后提取该显著区域改进的5维SURF特征.最后,通过KRQQCF识别目标,有效抑制杂波及噪声,提高星图的信噪比.实验结果表明,该算法快速、有效、可靠.
【总页数】8页(P1828-1835)
【关键词】星图模拟;加速鲁棒特征;核Rayleigh商二次相关滤波器;显著性
【作者】郭敬明;何昕;杨杰;魏仲慧
【作者单位】中国科学院长春光学精密机械与物理研究所;中国科学院大学;上海交通大学图像处理与模式识别研究所
【正文语种】中文
【中图分类】TP391
【相关文献】
1.采用核相关滤波器的自适应尺度目标跟踪 [J], 张雷;王延杰;孙宏海;姚志军;吴培
2.基于核Rayleigh商二次相关滤波器的红外目标检测 [J], 吴燕茹;程咏梅;赵永强;高仕博
3.采用自适应杂波白化滤波器和多卜勒滤波器组的雷达... [J], 金永汉;沈桂明
4.基于载波域自适应迭代滤波器的无源雷达多径杂波抑制方法 [J], 赵志欣;周新华;洪升;翁涛;王玉皞;
5.基于载波域自适应迭代滤波器的无源雷达多径杂波抑制方法 [J], 赵志欣;周新华;洪升;翁涛;王玉皞
因版权原因,仅展示原文概要,查看原文内容请购买。
一种基于混沌序列的灰度级盲水印算法
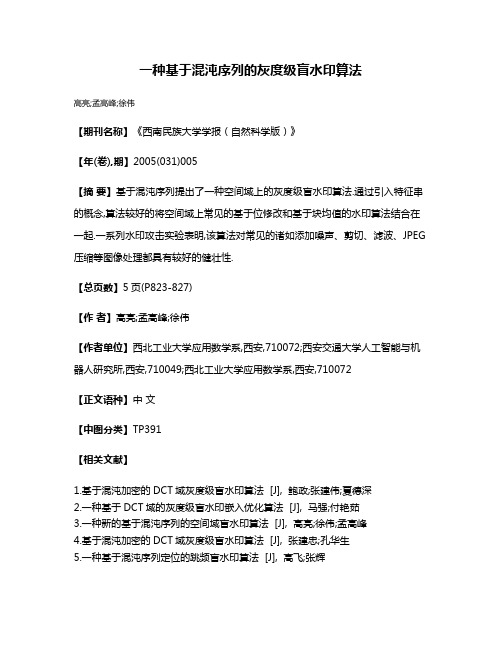
一种基于混沌序列的灰度级盲水印算法
高亮;孟高峰;徐伟
【期刊名称】《西南民族大学学报(自然科学版)》
【年(卷),期】2005(031)005
【摘要】基于混沌序列提出了一种空间域上的灰度级盲水印算法.通过引入特征串的概念,算法较好的将空间域上常见的基于位修改和基于块均值的水印算法结合在一起.一系列水印攻击实验表明,该算法对常见的诸如添加噪声、剪切、滤波、JPEG 压缩等图像处理都具有较好的健壮性.
【总页数】5页(P823-827)
【作者】高亮;孟高峰;徐伟
【作者单位】西北工业大学应用数学系,西安,710072;西安交通大学人工智能与机器人研究所,西安,710049;西北工业大学应用数学系,西安,710072
【正文语种】中文
【中图分类】TP391
【相关文献】
1.基于混沌加密的DCT域灰度级盲水印算法 [J], 鲍政;张建伟;夏德深
2.一种基于DCT域的灰度级盲水印嵌入优化算法 [J], 马强;付艳茹
3.一种新的基于混沌序列的空间域盲水印算法 [J], 高亮;徐伟;孟高峰
4.基于混沌加密的DCT域灰度级盲水印算法 [J], 张建忠;孔华生
5.一种基于混沌序列定位的跳频盲水印算法 [J], 高飞;张辉
因版权原因,仅展示原文概要,查看原文内容请购买。
基于超混沌系统及有限域的图像加密算法
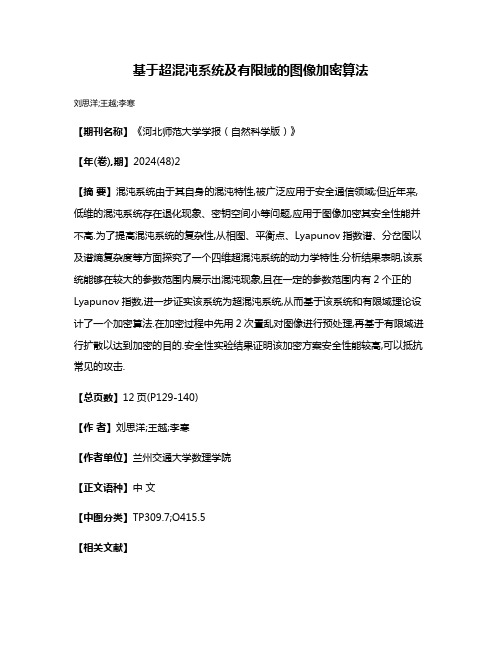
基于超混沌系统及有限域的图像加密算法
刘思洋;王越;李寒
【期刊名称】《河北师范大学学报(自然科学版)》
【年(卷),期】2024(48)2
【摘要】混沌系统由于其自身的混沌特性,被广泛应用于安全通信领域;但近年来,低维的混沌系统存在退化现象、密钥空间小等问题,应用于图像加密其安全性能并不高.为了提高混沌系统的复杂性,从相图、平衡点、Lyapunov指数谱、分岔图以及谱熵复杂度等方面探究了一个四维超混沌系统的动力学特性.分析结果表明,该系统能够在较大的参数范围内展示出混沌现象,且在一定的参数范围内有2个正的Lyapunov指数,进一步证实该系统为超混沌系统,从而基于该系统和有限域理论设计了一个加密算法.在加密过程中先用2次置乱对图像进行预处理,再基于有限域进行扩散以达到加密的目的.安全性实验结果证明该加密方案安全性能较高,可以抵抗常见的攻击.
【总页数】12页(P129-140)
【作者】刘思洋;王越;李寒
【作者单位】兰州交通大学数理学院
【正文语种】中文
【中图分类】TP309.7;O415.5
【相关文献】
1.基于超混沌理论的小波域分块图像加密算法
2.基于超混沌系统和有限域理论的图像加密算法
3.基于超混沌映射的医学图像小波域加密算法
4.基于混沌系统的彩色图像小波域加密算法
5.基于Chen系统与超混沌Lorenz系统的彩色图像\r加密算法
因版权原因,仅展示原文概要,查看原文内容请购买。
- 1、下载文档前请自行甄别文档内容的完整性,平台不提供额外的编辑、内容补充、找答案等附加服务。
- 2、"仅部分预览"的文档,不可在线预览部分如存在完整性等问题,可反馈申请退款(可完整预览的文档不适用该条件!)。
- 3、如文档侵犯您的权益,请联系客服反馈,我们会尽快为您处理(人工客服工作时间:9:00-18:30)。
*Corresponding author.Tel.:#61-7-3365-3984;fax:#61-7-3365-4999.E-mail address:ajantha @.au (A.S.Atukorale).Neurocomputing 35(2000)165}176Hierarchical overlapped neural gas network withapplication to pattern classi "cationAjantha S.Atukorale *,P.N.SuganthanDepartment of Computer Science and Electrical Engineering,Uni v ersity of Queensland,Brisbane,Qld.4072,AustraliaSchool of Electrical and Electronic Engineering,Nanyang Technological Uni v ersity,639798,Republic of SingaporeReceived 12June 1999;accepted 13April 2000AbstractThis paper describes our investigations into the neural gas (NG)network.The original neural gas network is computationally expensive,as an explicit ordering of all distances between synaptic weights and the training sample is necessary.This has a time complexity of O(N log N )in its sequential implementation.An alternative scheme was proposed for the above explicit ordering where it is carried out implicitly.In addition,a truncated weight updating rule was used similar to Choy and Siu (IEEE munications 46(3)(1998)301}304).By implementing the above modi "cations,the NG algorithm was made to run faster in its sequential implementation.A hierarchical overlapped neural gas architecture was developed on top of the above modi "ed NG algorithm for the classi "cation of real world handwritten numerals with high variations.This allowed us to obtain multiple classi "cations for each sample presented,and the "nal classi "cation was made by fusing the individual classi "cations.An excellent recognition rate for the NIST SD3database was consequently obtained. 2000Elsevier Science B.V.All rights reserved.Keywords:Character recognition;Hierarchical overlapped architecture;Multiple classi "er fusion;Neural gas network;Self-organizing maps1.IntroductionNeural network models have been intensively studied for many years in an e !ort to obtain superior performance compared to classical approaches.The self-organizing 0925-2312/00/$-see front matter 2000Elsevier Science B.V.All rights reserved.PII:S 0925-2312(00)00315-5166 A.S.Atukorale,P.N.Suganthan/Neurocomputing35(2000)165}176feature map(SOFM)proposed by Kohonen[12]is one of the network paradigms widely used for solving complex problems such as vector quantization,speech recog-nition,combinatorial optimization,pattern recognition and modeling the structure of the visual cortex.Kohonen's feature map is a special way of conserving the topologi-cal relationships in input data,which also bears some limitations.In this model the neighborhood relations between neural units have to be de"ned in advance.The topology of the input space has to match the topology of the output space which is to be represented.In addition,the dynamics of the SOFM algorithm cannot be described as a stochastic gradient descent on any energy function.A set of energy functions,one for each weight vector,seems to be the best description of the dynamics of the algorithm[5].Martinetz et al.[13]and Martinetz and Schulten[14]proposed the neural gas (NG)network algorithm for vector quantization,prediction and topology representa-tion a few years ago.The NG network model:(1)converges quickly to low-distortion errors,(2)reaches a distortion error lower than that resulting from K-means cluster-ing,maximum-entropy clustering and Kohonen's SOFM,(3)obeys a gradient descent on an energy surface.Similar to the SOFM algorithm the NG algorithm uses a soft-max adaptation rule(i.e.,not only adjust the winner reference vector,but also a!ects all the cluster centers depending on their proximity to the input signal).This is mainly to generate a topographic map and also to avoid con"nement to local minima during the adaptation procedure.Despite all those advantages,the NG network algorithm su!ers from a high time complexity problem in its sequential implementa-tion[4].In this paper,we discuss how such a time complexity problem associated with the NG algorithm can be reduced e$ciently.In addition,by de"ning an hierarchical overlapped structure[15]on top of the standard NG network,we obtained a hier-archical overlapped neural gas(HONG)network model for the classi"cation of real-world handwritten digits with high variations.The paper is organized as follows.After a brief review of the NG network algorithm in Section2,the proposed speed-up technique is presented in Section3.In Section4, the functionality of the HONG network architecture is discussed,and in Section5,the experimental results are presented.The paper is concluded with a brief discussion in Section6.2.The neural gas algorithmIn the neural gas algorithm,the synaptic weights w G are adapted without any"xed topological arrangement of the neural units within the network.Instead,it utilizes a neighborhood-ranking of the synaptic weights w G for given data vector*.The synaptic weight changes w G are not determined by the relative distances between the neural units within a topologically prestructured lattice,but by the relative distances between the neural units within the input space:hence the name neural gas network. Information about the arrangement of the receptive"elds within the input space is implicitly given by a set of distortions,D T"+""*!w G"",i"1,2,N,,associated witheach *,where N is the number of units in the network [14].Each time an input signal *is presented,the ordering of the elements of the set D T is necessary,to determine the adjustment of the synaptic weights w G .This ordering has a time complexity of O(N log N )in its sequential implementation.The resulting adaptation rule can be described as a winner-take-most instead of winner-take-all rule.The presented input signal *is received by each neural unit i and induces excitations f G (D T )which depend on the set of distortions D T .Assuming a Hebb-like rule,the adaptation step for w G is given by adjusting w G " f G (D T )(*!w G),i "1,2,N .(1)The step size 3[0,1]describes the overall extent of the modi "cation (learning rate)and f G (D T )3[0,1]accounts for the topological arrangement of the w G within the input space.Martinetz and Schullen [14]reported that an exponential function exp(!k G / )should give the best overall result,compared to other choices like Gaussians for the excitation function f G (D T ),where determines the number of neural units signi "cantly changing their synaptic weights with the adaptation step (1).The rank index k G "0,2,(N !1),describes the neighborhood-ranking of the neural units,with k G "0being the closest synaptic weight (w G )to the input signal *,k G "1being the second closest synaptic weight (w G )to *,and so on.That is,the set +w G ,w G ,2,w G ,\ ,is the neighborhood-ranking of w G relative to the given input vector *.The neighborhood-ranking index k G depends on *and the whole set of synaptic weights W "+w ,w ,2,w ,,and we denote this as k G (*,W ).The original NG network algorithm is summarized below.NG1:Initialize the synaptic weights w G randomly and the training parameter values ( G , D , G , D ),where G , G are initial values of (t ), (t )and D , D are "nal values of (t ), (t ).NG2:Present an input vector *and compute the distortions D T .NG3:Order the distortion set D T in ascending order.NG4:Adapt weight vectors according tow G " (t )h H (k G (*,W ))(*!w G ),i "1,2,N ,(2)where the parameters have the following time dependencies:(t )" G ( D / G)R R , (t )" G ( D / G )R R ,h H (k G )"exp(!k G / (t )).NG5:Increment the time parameter t by 1.NG6:Repeat NG2}NG5until the maximum iteration t is reached.3.Implicit ranking schemeThe NG network algorithm su !ers from a high time complexity problem in its sequential implementation [14,4].In the original neural gas network,an explicit ordering of all distances between synaptic weights and the training sample was necessary.This has a time complexity of O(N log N )in a sequential implementation.Recently some work has been done on speeding-up procedures for the sequential A.S.Atukorale,P.N.Suganthan /Neurocomputing 35(2000)165}176167implementation of the NG algorithm.Ancona et al.[1]discussed the questions of sorting accuracy and sorting completeness.With theoretical analysis and experi-mental evidence,they have concluded that partial,exact sorting(i.e.,ordering the top few winning units correctly and keeping all other units in the list una!ected)performs better than complete but noisy sorting(i.e.,ordering the top few winning units correctly and subjecting all other remaining units to inexact sorting).Also,they have concluded that even a few units in partial sorting is su$cient to attain a"nal distortion equivalent to that attained by the original NG algorithm.Moreover,they have concluded that correct identi"cation of the best-matching unit becomes more and more important while training proceeds.This is obvious,because as training proceeds,the adaptation step(1)becomes equivalent to the K-means adaptation rule. Choy and Siu[4]have also applied a partial distance elimination(PDE)method to speed-up the NG algorithm in the above context.In our investigations,we eliminated the explicit ordering(NG3in the above summary)by employing the following implicit ordering metric:m G"(d G!d )(d !d ),(3)where d and d are the minimum and maximum distances between the training sample and all reference units in the network respectively,and d G3D T,i"1,2,N. The best matching winner unit will then have an index of0,the worst matching unit will have an index of1,and the other units will take values between0and1(i.e., m G3[0,1]).By employing the above modi"cation to the original NG algorithm discussed earlier,we modi"ed the two entries NG3and NG4as follows.NG3:Find d ,d from the distortion set D T.NG4:Adapt weight vectors according tow G" (t)h H Y(m G(*,W))(*!w G),i"1,2,N,(4)where h H Y(m G)"exp(!m G/ (t))and (t)" (t)/(N!1).The modi"cation in(3)sped up the running time of the sequential implementation of the NG algorithm by about17%on average(see Table1).Further modi"cation to the weight update rule in(4)is done by rewriting it as w G" (t)(*!w G),where (t)" (t)exp(!m G/ (t)).We now update only those neurons with a non-negligible e!ective overall learning rate (t)as in[14,4].Given a threshold for (t)(say (t)"10\ ),the weight update rule in(4)is modi"ed by updating neurons in the following way:(t)510\ N exp(!m G/ (t))510\ / (t).168 A.S.Atukorale,P.N.Suganthan/Neurocomputing35(2000)165}176A.S.Atukorale,P.N.Suganthan/Neurocomputing35(2000)165}176169Table1Comparison of the processing time,number of updates and recognition rate for the originalNG algorithm(2),the implicit ranking metric(4),and the truncated weight update rule(6)Original NG Implicit ranking Truncated update Processing time(s)152.9327.72N/ANo.of updates106,152,000106,152,000707,957Recognition rate(%)96.8396.8196.98Thus m G/ (t)45log(10)#log( (t)).If we let r(t)"5log(10)#log( (t)),since (t)" G( D/ G)R R then it follows that:r(t)"5log(10)#log( G)#log( D/ G)t/t .(5) That is,update the weight vectors according to the following truncated weight update rule:w G" (t)exp(!m G/ (t))(*!w G)if m G4r(t) (t),(6)0otherwise,where r(t)is a parameter which depends only on t.Because of the above truncation, the weight update rule(4)will update those weights with non-zero values of w G. These modi"cations were able to eliminate the explicit ranking mechanism com-pletely,and reduce the number of weight updates by about99%on average(see Table 1).This also sped up the sequential implementation of the NG algorithm by about 96%on average(see Table1).4.Hierarchical overlapped neural gas architectureBy retaining the essence of the original NG algorithm and our modi"cation,we have developed a hierarchical overlapped neural gas(HONG)network algorithm for labeled pattern recognition.The structure of the HONG network architecture is an adaptation of the hierarchi-cal overlapped architecture developed for SOMs by Suganthan[15].First,the network is initialized with just one layer which is called the base layer.The number of neurons in the base layer has to be chosen appropriately.In labeled pattern recogni-tion applications,the number of distinct classes and the number of training samples may be considered in the selection of the initial size of the network.Similar to the SOM architecture,in the HONG network every neuron has an associated synaptic weight vector which has the same dimension as the input feature vector.Once we have selected the number of neurons in the base layer,we applied our modi"ed version of the NG algorithm to adapt the synaptic weights of the neurons in the base network. Having completed the unsupervised NG learning,the neurons in the base layer were labeled using a simple voting mechanism.In order to"ne tune the labeled network,weFig.1.Hierarchical overlapped architecture showing three second layer NG networks grown from units,A,B and C of the base NG network.applied the supervised learning vector quantization (LVQ)algorithm [12].The overlaps for each neuron in the base layer were then obtained.For instance,if we had 100neurons in the base layer network,then we have 100separate second layer NG networks grown from each neuron in the base layer network (see Fig.1).The overlapping is achieved by duplicating every training sample to train several second layer NG networks.That is,the winning neuron as well as a number of runners-up neurons will make use of the same training sample to train second layer NG networks grown from those neurons in the base layer NG network.In Fig.1for example,the overlapped NG network grown from neuron A is trained on samples where the neuron A is the winner or one of the "rst few runners-up for all the training samples presented to the base layer network.That is,if we have an overlap of 5(i.e.,the winner and 4runners-up)for the training samples then each training sample is being used to train "ve di !erent second layer NG networks.Fig.1also shows the overlap in the feature space of the two overlapped second layer NG networks conceptually assuming that the nodes A and B are adjacent to each other in the170 A.S.Atukorale,P.N.Suganthan /Neurocomputing 35(2000)165}176A.S.Atukorale,P.N.Suganthan/Neurocomputing35(2000)165}176171 feature space.Once the partially overlapping training samples were obtained for each of the second layer NG networks,we trained each of them as we trained the base layer NG network earlier.The second layer NG networks were then labeled using a simple voting mechanism.The testing samples were also duplicated,but to a lesser degree (e.g.,3times).Hence the testing samples"t well inside the feature space spanned by the winner and several runners-up in the training data.In addition,this duplication of the samples assists each HONG network to generate"ve independent classi"cations for every training sample and three independent classi"cations for every testing sample. In order to combine the outputs[3,11]of the second layer NG networks,we employed the idea of con"dence values.That is,we obtained a con"dence value for every sample's membership in every class(j)of each overlapped second layer NG network(i.e.,"ve for training and,three for testing)using the following:c H" 1!d H d ,(7)where d H is the minimum distance for every class j,d " H d H and j"0,2,9for numeral classi"cation.This will de"ne a con"dence value(c H)for the input pattern belonging to the j th class of an overlapped second layer NG network.The class which has the global minimum distance yields a con"dence value closer to one(in case of a perfect match,i.e.,d H"0,the con"dence value for that class becomes one).That is, the higher the con"dence value for a class,more likely a sample belongs to that class. We can also consider the above function as a basic probability assignment,because 04c H41.We can de"ne C G,the collection of all ten con"dence values of a second layer NG network asC G"+c H"j"0,1,2,9,,(8) where i"1,2,2,n and n is the number of overlaps considered(e.g.,n"5for training and n"3for testing).Here onwards we refer to this as the con x dence v ector of that second layer NG network.For example,let us assume that we are considering three overlaps for testing data.Then we get three con x dence v ectors from the corresponding second layer NG networks.Given the individual con x dence v ectors we can calculate the o v erall con x dence v ector of the HONG architecture by adding the individual con"dence values according to their class label(see Fig.1).We can then assign the class label of the test data according to the o v erall con x dence v ector(i.e.,select the index of the maximum con"dence value from the vector).The summary of the HONG network algorithm is given below.HONG1:Initialize the synaptic weights and training parameters of the base NG network.HONG2:Train the base NG network using the modi"ed NG algorithm as ex-plained in(4)using the neighborhood function de"ned in(6).As we train up to5second layer NG networks using the same training sample,we claim that there is a partial overlap between several second layer NG networks.172 A.S.Atukorale,P.N.Suganthan/Neurocomputing35(2000)165}176HONG3:Label the base NG network using a simple voting scheme.HONG4:Fine tune the base map with the supervised LVQ algorithm.HONG5:Obtain the overlaps for each unit in the base layer.HONG6:Initialize the synaptic weights of the second level NG networks around their base layer unit's(i.e.,root unit)value.HONG7:Train each second layer NG network as in HONG2using the overlap-ped samples obtained in HONG5.HONG8:Label each of the second layer NG networks accordingly as in HONG3, and"ne tune them as in HONG4.HONG9:Obtain the"nal recognition rates by combining the con"dence values generated by each of the second layer overlapped NG networks.5.Experimental results5.1.Implicit rankingTable1compares the corresponding processing times,number of updates and the recognition rates using our proposed implicit ranking metric with those obtained in the original NG algorithm.The simulations were performed using the parameters given in Subsection5.2on a Pentium II,350MHz personal computer.The overall processing time of the NG algorithm results from two distinct phases. First,it consists of the distance calculation phase and,second,the sorting or the ranking phase.Note that the distance calculation time is common to both explicit sorting and implicit ranking algorithms.In Table1,the processing time for`Original NG a(t )refers to the time taken by the sorting procedure(using qsort(),the C library routine with time complexity O(N log N)).The common distance calculation time(t )taken by the given parameters is154.51s.The percentage of improvement of time(or the speed-up)for the implicit ranking metric over the sorting algorithm is given byspeed up"(t !t /t );100%(9) where t ,t are as shown in Fig.2.In Table1,the`No.of updates a refers to the total number of updates performed in the training phase.This is given by the total number of training samples multiplied by the total number of neurons in the base network multiplied by the total number of iterations.The`Recognition Rate a refers to the training rate of the base network using the given samples.The results in the second and third columns of Table1compare the original NG algorithm against the implicit ranking metric de"ned in(3).This is a comparison between equations(2)and(4).We have achieved a speed up of81.87%with the proposed implicit ranking metric.The results in the second and fourth columns of Table1compare the original NG algorithm against the truncated weight update rule.This is a comparison between equations(2)and(6).The truncated weight update rule has reduced the number of weight updates vastly}more than99%.Since the processing time for this involvesFig.2.Processing time of the NG algorithm.weight update time,we did not report its processing time.Also,this modi "cation has increased the recognition rate slightly.This is due to the fact that,very small weight updates are generally noisy and,eliminating them would improve recognition accuracy.5.2.Character recognitionWe performed experiments on handwritten numerals to test our proposed HONG classi "er.These handwritten numeral samples were extracted from the NIST SD3database (which contains 223,124isolated handwritten numerals written by 2100writers and scanned at 300dots per inch)provided by the American National Institute of Standards and Technology (NIST)[7].We partitioned the NIST SD3database into non-overlapping sets shown in Table 2.The test set comprises samples from 600writers not used in the training and validation sets.We restricted the number of upper layers of the overlapped NG networks to two.The base layer consisted of 250neurons.The number of neurons for each overlapped NG network (second layer)were determined empirically by considering the available training samples for each of them.We found experimentally that min +300,max +35,(training }samples )/8,,was a good estimate for determining the number of neurons for the second layer.We used "ve overlaps for the training set and three overlaps for the testing set.Through trial and error,we discovered empirically G "0.7, D "0.05, G "0.01, D "0.0001and t "4;training }samples ,gave the best results for the proposed ing the above parameters in equation (5),we calculated the parameter r (t )"11.156!6.2;10\ t which was used to truncate the weight update rule as described in (6).The feature extraction algorithm is brie #y summarized below.E Prior to the feature extraction operation,pre-processing operations to the isolated numerals were performed.This involved removing isolated blobs from the binary image based on a ratio test.E The pre-processed digit was then centered and only the numeral part was extracted from the 128;128binary image.E The extracted binary image was rescaled to an 88;72pixel resolution.E Finally,each such binary image was subsampled into 8;8blocks and the result was an 11;9grey scale image with pixel values in the range [0,64].A.S.Atukorale,P.N.Suganthan /Neurocomputing 35(2000)165}176173174 A.S.Atukorale,P.N.Suganthan/Neurocomputing35(2000)165}176Table2Partitions of SD3data set used in our experimentsPartition(s)hsf}+0,1,2,hsf}+0,1,2,hsf}3Size106,15253,07663,896Use Training Validation TestingTable3Recognition ratesTraining Validation TestingBase network99.31%98.60%98.84%HONG99.90%99.30%99.30%As a result of the above feature extraction method,we were left with a feature vector of 99elements.The recognition rates obtained using the above parameters are illustrated in Table3.As can be seen,the HONG architecture improves further on the high classi"cation rate provided by the base NG network.To the best of our knowledge, the most successful results obtained for the NIST SD3database were by Ha and Bunke[8].They used a total of223,124samples and obtained a recognition rate of 99.54%from a test set of173,124samples.They designed two recognition systems based on two distinct feature extraction methods and used a fully connected feed-forward three layer perceptron as the classi"er for both feature extraction methods.In addition,if the best score in the combined classi"er was less than a"xed prede"ned threshold,they replaced the normalization operation prior to feature extraction bya set of perturbation processes which modeled the writing habits and instruments.6.ConclusionsIn this paper,we have proposed an implicit ranking scheme to speed-up the sequential implementation of the original NG algorithm.In contrast to Kohonen's SOFM algorithm,the NG algorithm takes a smaller number of learning steps to converge,does not require any prior knowledge about the structure of the network, and its dynamics can be characterized by a global cost function.We have also developed the HONG network architecture to obtain a better classi"cation on con#icting data.This is particularly important in totally uncon-strained handwritten data,since they contain con#icting information within the same class due to the various writing styles and instruments used.The HONG network architecture systematically partitions the input space to avoid such situations by projecting the input data to di!erent upper level NG networks(see Fig.1).Since the training and the testing samples were duplicated in the upper layers,we obtained multiple decision classi"cations for every sample.The"nal classi"cation was obtainedA.S.Atukorale,P.N.Suganthan/Neurocomputing35(2000)165}176175 by combining the individual classi"cations generated by the second level networks. We employed the idea of con"dence values in obtaining the"nal classi"cation.The proposed architecture was tested on handwritten numerals extracted from the NIST 19database.Compared to the number of applications for Kohonen's SOFM,there are relatively few for NG in the literature[2,6,9,10,16,17].We hope,due to the speeding up method that we have introduced for the sequential implementation,that there will be more applications of the NG algorithm in the future.AcknowledgementsThe authors would like to thank Marcus Gallagher,Ian Wood and Hugo Navone of the Neural Network Laboratory,University of Queensland,Australia,for their invaluable support and comments.The authors would also like to thank the two reviewers for their comments and suggestions that improved the quality of this manuscript.References[1]F.Ancona,S.Ridella,S.Rovetta,R.Zunino,On the importance of sorting in neural gas training ofvector quantizers,Proceedings of the ICNN-97,1997,pp.1804}1808.[2]E.Ardizzone,A.Chella,R.Rizzo,Color image segmentation based on a neural gas network,in:M.Marinaro and P.G.Morsso(Eds.),International Conference on Arti"cial Neural Networks, 1994,pp.1161}1164.[3]S.-B.Cho,J.H.Kim,Multiple network fusion using fuzzy logic,IEEE Trans.Neural Networks6(2)(1995)497}501.[4]C.S.-T.Choy,W.-C.Siu,Fast sequential implementation of neural gas network for vector quantiz-ation,IEEE mun.46(3)(1998)301}304.[5]E.Erwin,K.Obermayer,K.Schulten,Self-organizing maps:ordering,convergence properties andenergy functions,Biol.Cybernet.67(1992)47}55.[6]M.Fontana,N.Borghese,S.Ferrari,Image reconstruction using improved neural gas,in:M.Marinaro and R.Tagliaferri(Eds.),Proceedings of the Seventh Italian Workshop on Neural Nets,1996,pp.260}265.[7]M.D.Garris,Design,collection and analysis of handwriting sample image databases,Encyclo.Comput.Sci.Technol.31(16)(1994)189}213.[8]T.M.Ha,H.Bunke,O!-line handwritten numeral recognition by perturbation method,IEEE Trans.Pattern Anal.Mach.Intell.19(5)(1997)535}539.[9]T.Hofmann,J.M.Buhmann,An annealed neural gas network for robust vector quantization,in:C.von der Malsburg,W.von Seelen,J.Vorbruggen,B.Sendho!(Eds.),Arti"cial Neural Networks,Springer,Germany,1996,pp.151}156.[10]K.Kishida,H.Miyajima,M.Maeda,Destructive fuzzy modeling using neural gas network,IEICEput.Sci.E80-A(9)(1997)1578}1584.[11]J.Kittler,Combining classi"ers:A Theoretical Framework,Pattern Anal.Appl.1(1)(1998)18}27.[12]T.Kohonen,Self-Organizing Maps,Springer,Berlin,1995.[13]T.Martinetz,S.G.Berkovich,K.Schulten,Neural gas network for vector quantization and itsapplication to times-series prediction,IEEE Trans.Neural Networks4(4)(1993)218}226.。