Extending the Limits of Supertree Methods c ○ Birkhuser Verlag, Basel, 2006 Annals of Comb
九年级英语地理常识单选题60题

九年级英语地理常识单选题60题1. Which country is known as the "Land of the Rising Sun"?A. ChinaB. JapanC. IndiaD. South Korea答案:B。
日本被称为“日出之国”,中国是“The People's Republic of China”,印度是“India”,韩国是“South Korea”,所以选B。
2. Where is the Amazon Rainforest located?A. North AmericaB. South AmericaC. AfricaD. Australia答案:B。
亚马逊雨林位于南美洲,北美洲没有如此大面积的雨林,非洲主要是热带草原和沙漠,澳大利亚以独特的生态环境为主,故选B。
3. Which country is famous for the Pyramids of Giza?A. EgyptB. IraqC. IranD. Israel答案:A。
吉萨金字塔位于埃及,伊拉克、伊朗和以色列没有著名的吉萨金字塔,所以选A。
4. In which continent is the Alps mountain range?A. AsiaB. EuropeC. AfricaD. North America答案:B。
阿尔卑斯山脉在欧洲,亚洲、非洲和北美洲没有阿尔卑斯山脉,故答案是B。
5. Which country is the largest in area in the world?A. ChinaB. RussiaC. United StatesD. Canada答案:B。
世界上面积最大的国家是俄罗斯,中国面积居世界第三,美国面积第四,加拿大面积第二,所以选B。
6. Which river is the longest in the world?A. The Yangtze RiverB. The Nile RiverC. The Amazon RiverD. The Yellow River答案:B。
树的变化过程英语作文初中

The process of a trees growth is a fascinating journey that begins with a tiny seed and culminates in a towering presence in the forest. Here is a brief essay on the changes a tree undergoes from its inception to maturity.The Transformation of a Tree: A Journey from Seed to CanopyIn the vast expanse of nature, the life cycle of a tree is a testament to resilience and growth. It starts with a humble seed, often no larger than a pinhead, yet carrying within it the potential to become a mighty tree.Stage One: GerminationThe first stage of a trees life is germination. When the conditions are rightadequate moisture, warmth, and darknessthe seed begins to sprout. The seeds outer shell cracks, and a tiny root emerges, seeking nourishment from the soil. Simultaneously, a shoot pushes its way towards the light, breaking through the earths surface.Stage Two: SaplingAs the young plant, now called a sapling, grows, it develops leaves that photosynthesize sunlight, converting it into energy for growth. The roots delve deeper into the ground, anchoring the tree and absorbing water and minerals. During this stage, the sapling is vulnerable to various threats, including pests, diseases, and harsh weather conditions.Stage Three: Juvenile GrowthThe juvenile phase is characterized by rapid growth. The trees trunk thickens, and its branches extend outwards, reaching for more sunlight. This is a critical period where the tree establishes its place in the ecosystem, competing with other plants for resources. Stage Four: MaturityMaturity brings a tree to its prime. It reaches its full height, and its canopy spreads wide, providing shade and habitat for various species of birds and insects. The tree now plays a vital role in the forest ecosystem, contributing to nutrient cycling, oxygen production, and carbon sequestration.Stage Five: Senescence and DecayAs the tree ages, it enters the phase of senescence. Its growth slows, and it may start to lose branches. Eventually, the tree reaches the end of its life cycle and begins to decay. This natural process is essential, as it recycles nutrients back into the soil, enriching it for new life to sprout.ConclusionThe life of a tree is a beautiful and complex process, mirroring the stages of growth, maturity, and decline that all living things experience. Each tree, from the smallest sapling to the grandest oak, contributes uniquely to the tapestry of life on Earth. Understanding this process not only deepens our appreciation for nature but also reminds us of our interconnectedness with the environment.。
文献 (10)Semi-supervised and unsupervised extreme learning
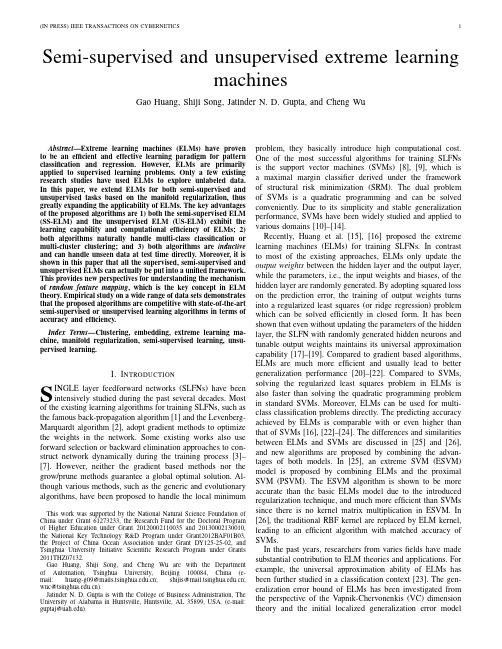
Semi-supervised and unsupervised extreme learningmachinesGao Huang,Shiji Song,Jatinder N.D.Gupta,and Cheng WuAbstract—Extreme learning machines(ELMs)have proven to be an efficient and effective learning paradigm for pattern classification and regression.However,ELMs are primarily applied to supervised learning problems.Only a few existing research studies have used ELMs to explore unlabeled data. In this paper,we extend ELMs for both semi-supervised and unsupervised tasks based on the manifold regularization,thus greatly expanding the applicability of ELMs.The key advantages of the proposed algorithms are1)both the semi-supervised ELM (SS-ELM)and the unsupervised ELM(US-ELM)exhibit the learning capability and computational efficiency of ELMs;2) both algorithms naturally handle multi-class classification or multi-cluster clustering;and3)both algorithms are inductive and can handle unseen data at test time directly.Moreover,it is shown in this paper that all the supervised,semi-supervised and unsupervised ELMs can actually be put into a unified framework. This provides new perspectives for understanding the mechanism of random feature mapping,which is the key concept in ELM theory.Empirical study on a wide range of data sets demonstrates that the proposed algorithms are competitive with state-of-the-art semi-supervised or unsupervised learning algorithms in terms of accuracy and efficiency.Index Terms—Clustering,embedding,extreme learning ma-chine,manifold regularization,semi-supervised learning,unsu-pervised learning.I.I NTRODUCTIONS INGLE layer feedforward networks(SLFNs)have been intensively studied during the past several decades.Most of the existing learning algorithms for training SLFNs,such as the famous back-propagation algorithm[1]and the Levenberg-Marquardt algorithm[2],adopt gradient methods to optimize the weights in the network.Some existing works also use forward selection or backward elimination approaches to con-struct network dynamically during the training process[3]–[7].However,neither the gradient based methods nor the grow/prune methods guarantee a global optimal solution.Al-though various methods,such as the generic and evolutionary algorithms,have been proposed to handle the local minimum This work was supported by the National Natural Science Foundation of China under Grant61273233,the Research Fund for the Doctoral Program of Higher Education under Grant20120002110035and20130002130010, the National Key Technology R&D Program under Grant2012BAF01B03, the Project of China Ocean Association under Grant DY125-25-02,and Tsinghua University Initiative Scientific Research Program under Grants 2011THZ07132.Gao Huang,Shiji Song,and Cheng Wu are with the Department of Automation,Tsinghua University,Beijing100084,China(e-mail:huang-g09@;shijis@; wuc@).Jatinder N.D.Gupta is with the College of Business Administration,The University of Alabama in Huntsville,Huntsville,AL35899,USA.(e-mail: guptaj@).problem,they basically introduce high computational cost. One of the most successful algorithms for training SLFNs is the support vector machines(SVMs)[8],[9],which is a maximal margin classifier derived under the framework of structural risk minimization(SRM).The dual problem of SVMs is a quadratic programming and can be solved conveniently.Due to its simplicity and stable generalization performance,SVMs have been widely studied and applied to various domains[10]–[14].Recently,Huang et al.[15],[16]proposed the extreme learning machines(ELMs)for training SLFNs.In contrast to most of the existing approaches,ELMs only update the output weights between the hidden layer and the output layer, while the parameters,i.e.,the input weights and biases,of the hidden layer are randomly generated.By adopting squared loss on the prediction error,the training of output weights turns into a regularized least squares(or ridge regression)problem which can be solved efficiently in closed form.It has been shown that even without updating the parameters of the hidden layer,the SLFN with randomly generated hidden neurons and tunable output weights maintains its universal approximation capability[17]–[19].Compared to gradient based algorithms, ELMs are much more efficient and usually lead to better generalization performance[20]–[22].Compared to SVMs, solving the regularized least squares problem in ELMs is also faster than solving the quadratic programming problem in standard SVMs.Moreover,ELMs can be used for multi-class classification problems directly.The predicting accuracy achieved by ELMs is comparable with or even higher than that of SVMs[16],[22]–[24].The differences and similarities between ELMs and SVMs are discussed in[25]and[26], and new algorithms are proposed by combining the advan-tages of both models.In[25],an extreme SVM(ESVM) model is proposed by combining ELMs and the proximal SVM(PSVM).The ESVM algorithm is shown to be more accurate than the basic ELMs model due to the introduced regularization technique,and much more efficient than SVMs since there is no kernel matrix multiplication in ESVM.In [26],the traditional RBF kernel are replaced by ELM kernel, leading to an efficient algorithm with matched accuracy of SVMs.In the past years,researchers from variesfields have made substantial contribution to ELM theories and applications.For example,the universal approximation ability of ELMs has been further studied in a classification context[23].The gen-eralization error bound of ELMs has been investigated from the perspective of the Vapnik-Chervonenkis(VC)dimension theory and the initial localized generalization error model(LGEM)[27],[28].Varies extensions have been made to the basic ELMs to make it more efficient and more suitable for specific problems,such as ELMs for online sequential data [29]–[31],ELMs for noisy/missing data[32]–[34],ELMs for imbalanced data[35],etc.From the implementation aspect, ELMs has recently been implemented using parallel tech-niques[36],[37],and realized on hardware[38],which made ELMs feasible for large data sets and real time reasoning. Though ELMs have become popular in a wide range of domains,they are primarily used for supervised learning tasks such as classification and regression,which greatly limits their applicability.In some cases,such as text classification, information retrieval and fault diagnosis,obtaining labels for fully supervised learning is time consuming and expensive, while a multitude of unlabeled data are easy and cheap to collect.To overcome the disadvantage of supervised learning al-gorithms that they cannot make use of unlabeled data,semi-supervised learning(SSL)has been proposed to leverage both labeled and unlabeled data[39],[40].The SSL algorithms assume that the input patterns from both labeled and unlabeled data are drawn from the same marginal distribution.Therefore, the unlabeled data naturally provide useful information for exploring the data structure in the input space.By assuming that the input data follows some cluster structure or manifold in the input space,SSL algorithms can incorporate both la-beled and unlabeled data into the learning process.Since SSL requires less effort to collect labeled data and can offer higher accuracy,it has been applied to various domains[41]–[43].In some other cases where no labeled data are available,people may be interested in exploring the underlying structure of the data.To this end,unsupervised learning(USL)techniques, such as clustering,dimension reduction or data representation, are widely used to fulfill these tasks.In this paper,we extend ELMs to handle both semi-supervised and unsupervised learning problems by introducing the manifold regularization framework.Both the proposed semi-supervised ELM(SS-ELM)and unsupervised ELM(US-ELM)inherit the computational efficiency and the learn-ing capability of traditional pared with existing algorithms,SS-ELM and US-ELM are not only inductive (straightforward extension for out-of-sample examples at test time),but also can be used for multi-class classification or multi-cluster clustering directly.We test our algorithms on a variety of data sets,and make comparisons with other related algorithms.The results show that the proposed algorithms are competitive with state-of-the-art algorithms in terms of accuracy and efficiency.It is worth to mention that all the supervised,semi-supervised and unsupervised ELMs can actually be put into a unified framework,that is all the algorithms consist of two stages:1)random feature mapping;and2)output weights solving.Thefirst stage is to construct the hidden layer using randomly generated hidden neurons.This is the key concept in the ELM theory,which differs it from many existing feature learning methods.Generating feature mapping randomly en-ables ELMs for fast nonlinear feature learning and alleviates the problem of over-fitting.The second stage is to solve the weights between the hidden layer and the output layer, and this is where the main difference of supervised,semi-supervised and unsupervised ELMs lies.We believe that the unified framework for the three types of ELMs might provide us a new perspective to understand the underlying behavior of the random feature mapping in ELMs.The rest of the paper is organized as follows.In Section II,we give a brief review of related existing literature on semi-supervised and unsupervised learning.Section III and IV introduce the basic formulation of ELMs and the man-ifold regularization framework,respectively.We present the proposed SS-ELM and US-ELM algorithms in Sections V and VI.Experiment results are given in Section VII,and Section VIII concludes the paper.II.R ELATED WORKSOnly a few existing research studies on ELMs have dealt with the problem of semi-supervised learning or unsupervised learning.In[44]and[45],the manifold regularization frame-work was introduce into the ELMs model to leverage both labeled and unlabeled data,thus extended ELMs for semi-supervised learning.However,both of these two works are limited to binary classification problems,thus they haven’t explore the full power of ELMs.Moreover,both algorithms are only effective when the number of training patterns is more than the number of hidden neurons.Unfortunately,this condition is usually violated in semi-supervised learning since the training data is relatively scarce compared to the hidden neurons,whose number is commonly set to several hundreds or several thousands.Recently,a co-training approach have been proposed to train ELMs in a semi-supervised setting [46].In this algorithm,the labeled training sets are augmented gradually by moving a small set of most confidently predicted unlabeled data to the labeled set at each loop,and ELMs are trained repeatedly on the pseudo-labeled set.Since the algo-rithm need to train ELMs repeatedly,it introduces considerable extra computational cost.The proposed SS-ELM is related to a few other mani-fold assumption based semi-supervised learning algorithms, such as the Laplacian support vector machines(LapSVMs) [47],the Laplacian regularized least squares(LapRLS)[47], semi-supervised neural networks(SSNNs)[48],and semi-supervised deep embedding[49].It has been shown in these works that manifold regularization is effective in a wide range of domains and often leads to a state-of-the-art performance in terms of accuracy and efficiency.The US-ELM proposed in this paper are related to the Laplacian Eigenmaps(LE)[50]and spectral clustering(SC) [51]in that they both use spectral techniques for embedding and clustering.In all these algorithms,an affinity matrix is first built from the input patterns.The SC performs eigen-decomposition on the normalized affinity matrix,and then embeds the original data into a d-dimensional space using the first d eigenvectors(each row is normalized to have unit length and represents a point in the embedded space)corresponding to the d largest eigenvalues.The LE algorithm performs generalized eigen-decomposition on the graph Laplacian,anduses the d eigenvectors corresponding to the second through the(d+1)th smallest eigenvalues for embedding.When LE and SC are used for clustering,then k-means is adopted to cluster the data in the embedded space.Similar to LE and SC,the US-ELM are also based on the affinity matrix,and it is converted to solving a generalized eigen-decomposition problem.However,the eigenvectors obtained in US-ELM are not used for data representation directly,but are used as the parameters of the network,i.e.,the output weights.Note that once the US-ELM model is trained,it can be applied to any presented data in the original input space.In this way,US-ELM provide a straightforward way for handling new patterns without recomputing eigenvectors as in LE and SC.III.E XTREME LEARNING MACHINES Consider a supervised learning problem where we have a training set with N samples,{X,Y}={x i,y i}N i=1.Herex i∈R n i,y i is a n o-dimensional binary vector with only one entry(correspond to the class that x i belongs to)equal to one for multi-classification tasks,or y i∈R n o for regression tasks,where n i and n o are the dimensions of input and output respectively.ELMs aim to learn a decision rule or an approximation function based on the training data. Generally,the training of ELMs consists of two stages.The first stage is to construct the hidden layer using afixed number of randomly generated mapping neurons,which can be any nonlinear piecewise continuous functions,such as the Sigmoid function and Gaussian function given below.1)Sigmoid functiong(x;θ)=11+exp(−(a T x+b));(1)2)Gaussian functiong(x;θ)=exp(−b∥x−a∥);(2) whereθ={a,b}are the parameters of the mapping function and∥·∥denotes the Euclidean norm.A notable feature of ELMs is that the parameters of the hidden mapping functions can be randomly generated ac-cording to any continuous probability distribution,e.g.,the uniform distribution on(-1,1).This makes ELMs distinct from the traditional feedforward neural networks and SVMs. The only free parameters that need to be optimized in the training process are the output weights between the hidden neurons and the output nodes.By doing so,training ELMs is equivalent to solving a regularized least squares problem which is considerately more efficient than the training of SVMs or backpropagation algorithms.In thefirst stage,a number of hidden neurons which map the data from the input space into a n h-dimensional feature space (n h is the number of hidden neurons)are randomly generated. We denote by h(x i)∈R1×n h the output vector of the hidden layer with respect to x i,andβ∈R n h×n o the output weights that connect the hidden layer with the output layer.Then,the outputs of the network are given byf(x i)=h(x i)β,i=1,...,N.(3)In the second stage,ELMs aim to solve the output weights by minimizing the sum of the squared losses of the prediction errors,which leads to the following formulationminβ∈R n h×n o12∥β∥2+C2N∑i=1∥e i∥2s.t.h(x i)β=y T i−e T i,i=1,...,N,(4)where thefirst term in the objective function is a regularization term which controls the complexity of the model,e i∈R n o is the error vector with respect to the i th training pattern,and C is a penalty coefficient on the training errors.By substituting the constraints into the objective function, we obtain the following equivalent unconstrained optimization problem:minβ∈R n h×n oL ELM=12∥β∥2+C2∥Y−Hβ∥2(5)where H=[h(x1)T,...,h(x N)T]T∈R N×n h.The above problem is widely known as the ridge regression or regularized least squares.By setting the gradient of L ELM with respect toβto zero,we have∇L ELM=β+CH H T(Y−Hβ)=0(6) If H has more rows than columns and is of full column rank,which is usually the case where the number of training patterns are more than the number of the hidden neurons,the above equation is overdetermined,and we have the following closed form solution for(5):β∗=(H T H+I nhC)−1H T Y,(7)where I nhis an identity matrix of dimension n h.Note that in practice,rather than explicitly inverting the n h×n h matrix in the above expression,we can use Gaussian elimination to directly solve a set of linear equations in a more efficient and numerically stable manner.If the number of training patterns are less than the number of hidden neurons,then H will have more columns than rows, which often leads to an underdetermined least squares prob-lem.In this case,βmay have infinite number of solutions.To handle this problem,we restrictβto be a linear combination of the rows of H:β=H Tα(α∈R N×n o).Notice that when H has more columns than rows and is of full row rank,then H H T is invertible.Multiplying both side of(6) by(H H T)−1H,we getα+C(Y−H H Tα)=0,(8) This yieldsβ∗=H Tα∗=H T(H H T+I NC)−1Y(9)where I N is an identity matrix of dimension N. Therefore,in the case where training patterns are plentiful compared to the hidden neurons,we use(7)to compute the output weights,otherwise we use(9).IV.T HE MANIFOLD REGULARIZATION FRAMEWORK Semi-supervised learning is built on the following two assumptions:(1)both the label data X l and the unlabeled data X u are drawn from the same marginal distribution P X ;and (2)if two points x 1and x 2are close to each other,then the conditional probabilities P (y |x 1)and P (y |x 2)should be similar as well.The latter assumption is widely known as the smoothness assumption in machine learning.To enforce this assumption on the data,the manifold regularization framework proposes to minimize the following cost functionL m=12∑i,jw ij ∥P (y |x i )−P (y |x j )∥2,(10)where w ij is the pair-wise similarity between two patterns x iand x j .Note that the similarity matrix W =[w ij ]is usually sparse,since we only place a nonzero weight between two patterns x i and x j if they are close,e.g.,x i is among the k nearest neighbors of x j or x j is among the k nearest neighbors of x i .The nonzero weights are usually computed using Gaussian function exp (−∥x i −x j ∥2/2σ2),or simply fixed to 1.Intuitively,the formulation (10)penalizes large variation in the conditional probability P (y |x )when x has a small change.This requires that P (y |x )vary smoothly along the geodesics of P (x ).Since it is difficult to compute the conditional probability,we can approximate (10)with the following expression:ˆLm =12∑i,jw ij ∥ˆyi −ˆy j ∥2,(11)where ˆyi and ˆy j are the predictions with respect to pattern x i and x j ,respectively.It is straightforward to simplify the above expression in a matrix form:ˆL m =Tr (ˆY T L ˆY ),(12)where Tr (·)denotes the trace of a matrix,L =D −W isknown as the graph Laplacian ,and D is a diagonal matrixwith its diagonal elements D ii =l +u∑j =1w i,j .As discussed in [52],instead of using L directly,we can normalize it byD −12L D −12or replace it by L p (p is an integer),based on some prior knowledge.V.S EMI -SUPERVISED ELMIn the semi-supervised setting,we have few labeled data and plenty of unlabeled data.We denote the labeled data in the training set as {X l ,Y l }={x i ,y i }l i =1,and unlabeled dataas X u ={x i }ui =1,where l and u are the number of labeled and unlabeled data,respectively.The proposed SS-ELM incorporates the manifold regular-ization to leverage unlabeled data to improve the classification accuracy when labeled data are scarce.By modifying the ordinary ELM formulation (4),we give the formulation ofSS-ELM as:minβ∈R n h ×n o12∥β∥2+12l∑i =1C i ∥e i ∥2+λ2Tr (F T L F )s.t.h (x i )β=y T i −e T i ,i =1,...,l,f i =h (x i )β,i =1,...,l +u(13)where L ∈R (l +u )×(l +u )is the graph Laplacian built fromboth labeled and unlabeled data,and F ∈R (l +u )×n o is the output matrix of the network with its i th row equal to f (x i ),λis a tradeoff parameter.Note that similar to the weighted ELM algorithm (W-ELM)introduced in [35],here we associate different penalty coeffi-cient C i on the prediction errors with respect to patterns from different classes.This is because we found that when the data is skewed,i.e.,some classes have significantly more training patterns than other classes,traditional ELMs tend to fit the classes that having the majority of patterns quite well but fits other classes poorly.This usually leads to poor generalization performance on the testing set (while the prediction accuracy may be high,but the some classes are neglected).Therefore,we propose to alleviate this problem by re-weighting instances from different classes.Suppose that x i belongs to class t i ,which has N t i training patterns,then we associate e i with a penalty ofC i =C 0N t i.(14)where C 0is a user defined parameter as in traditional ELMs.In this way,the patterns from the dominant classes will not be over fitted by the algorithm,and the patterns from a class with less samples will not be neglected.We substitute the constraints into the objective function,and rewrite the above formulation in a matrix form:min β∈R n h×n o 12∥β∥2+12∥C 12( Y −Hβ)∥2+λ2Tr (βT H TL Hβ)(15)where Y∈R (l +u )×n o is the training target with its first l rows equal to Y l and the rest equal to 0,C is a (l +u )×(l +u )diagonal matrix with its first l diagonal elements [C ]ii =C i ,i =1,...,l and the rest equal to 0.Again,we compute the gradient of the objective function with respect to β:∇L SS −ELM =β+H T C ( Y−H β)+λH H T L H β.(16)By setting the gradient to zero,we obtain the solution tothe SS-ELM:β∗=(I n h +H T C H +λH H T L H )−1H TC Y .(17)As in Section III,if the number of labeled data is fewer thanthe number of hidden neurons,which is common in SSL,we have the following alternative solution:β∗=H T (I l +u +C H H T +λL L H H T )−1C Y .(18)where I l +u is an identity matrix of dimension l +u .Note that by settingλto be zero and the diagonal elements of C i(i=1,...,l)to be the same constant,(17)and (18)reduce to the solutions of traditional ELMs(7)and(9), respectively.Based on the above discussion,the SS-ELM algorithm is summarized as Algorithm1.Algorithm1The SS-ELM algorithmInput:The labeled patterns,{X l,Y l}={x i,y i}l i=1;The unlabeled patterns,X u={x i}u i=1;Output:The mapping function of SS-ELM:f:R n i→R n oStep1:Construct the graph Laplacian L from both X l and X u.Step2:Initiate an ELM network of n h hidden neurons with random input weights and biases,and calculate the output matrix of the hidden neurons H∈R(l+u)×n h.Step3:Choose the tradeoff parameter C0andλ.Step4:•If n h≤NCompute the output weightsβusing(17)•ElseCompute the output weightsβusing(18)return The mapping function f(x)=h(x)β.VI.U NSUPERVISED ELMIn this section,we introduce the US-ELM algorithm for unsupervised learning.In an unsupervised setting,the entire training data X={x i}N i=1are unlabeled(N is the number of training patterns)and our target is tofind the underlying structure of the original data.The formulation of US-ELM follows from the formulation of SS-ELM.When there is no labeled data,(15)is reduced tomin β∈R n h×n o ∥β∥2+λTr(βT H T L Hβ)(19)Notice that the above formulation always attains its mini-mum atβ=0.As suggested in[50],we have to introduce addtional constraints to avoid a degenerated solution.Specifi-cally,the formulation of US-ELM is given bymin β∈R n h×n o ∥β∥2+λTr(βT H T L Hβ)s.t.(Hβ)T Hβ=I no(20)Theorem1:An optimal solution to problem(20)is given by choosingβas the matrix whose columns are the eigenvectors (normalized to satisfy the constraint)corresponding to thefirst n o smallest eigenvalues of the generalized eigenvalue problem:(I nh +λH H T L H)v=γH H T H v.(21)Proof:We can rewrite the problem(20)asminβ∈R n h×n o,ββT Bβ=I no Tr(βT Aβ),(22)Algorithm2The US-ELM algorithmInput:The training data:X∈R N×n i;Output:•For embedding task:The embedding in a n o-dimensional space:E∈R N×n o;•For clustering task:The label vector of cluster index:y∈N N×1+.Step1:Construct the graph Laplacian L from X.Step2:Initiate an ELM network of n h hidden neurons withrandom input weights,and calculate the output matrix of thehidden neurons H∈R N×n h.Step3:•If n h≤NFind the generalized eigenvectors v2,v3,...,v no+1of(21)corresponding to the second through the n o+1smallest eigenvalues.Letβ=[ v2, v3,..., v no+1],where v i=v i/∥H v i∥,i=2,...,n o+1.•ElseFind the generalized eigenvectors u2,u3,...,u no+1of(24)corresponding to the second through the n o+1smallest eigenvalues.Letβ=H T[ u2, u3,..., u no+1],where u i=u i/∥H H T u i∥,i=2,...,n o+1.Step4:Calculate the embedding matrix:E=Hβ.Step5(For clustering only):Treat each row of E as a point,and cluster the N points into K clusters using the k-meansalgorithm.Let y be the label vector of cluster index for allthe points.return E(for embedding task)or y(for clustering task);where A=I nh+λH H T L H and B=H T H.It is easy to verify that both A and B are Hermitianmatrices.Thus,according to the Rayleigh-Ritz theorem[53],the above trace minimization problem attains its optimum ifand only if the column span ofβis the minimum span ofthe eigenspace corresponding to the smallest n o eigenvaluesof(21).Therefore,by stacking the normalized eigenvectors of(21)corresponding to the smallest n o generalized eigenvalues,we obtain an optimal solution to(20).In the algorithm of Laplacian eigenmaps,thefirst eigenvec-tor is discarded since it is always a constant vector proportionalto1(corresponding to the smallest eigenvalue0)[50].In theUS-ELM algorithm,thefirst eigenvector of(21)also leadsto small variations in embedding and is not useful for datarepresentation.Therefore,we suggest to discard this trivialsolution as well.Letγ1,γ2,...,γno+1(γ1≤γ2≤...≤γn o+1)be the(n o+1)smallest eigenvalues of(21)and v1,v2,...,v no+1be their corresponding eigenvectors.Then,the solution to theoutput weightsβis given byβ∗=[ v2, v3,..., v no+1],(23)where v i=v i/∥H v i∥,i=2,...,n o+1are the normalizedeigenvectors.If the number of labeled data is fewer than the numberTABLE ID ETAILS OF THE DATA SETS USED FOR SEMI-SUPERVISED LEARNINGData set Class Dimension|L||U||V||T|G50C2505031450136COIL20(B)2102440100040360USPST(B)225650140950498COIL2020102440100040360USPST1025650140950498of hidden neurons,problem(21)is underdetermined.In this case,we have the following alternative formulation by using the same trick as in previous sections:(I u+λL L H H T )u=γH H H T u.(24)Again,let u1,u2,...,u no +1be generalized eigenvectorscorresponding to the(n o+1)smallest eigenvalues of(24), then thefinal solution is given byβ∗=H T[ u2, u3,..., u no +1],(25)where u i=u i/∥H H T u i∥,i=2,...,n o+1are the normal-ized eigenvectors.If our task is clustering,then we can adopt the k-means algorithm to perform clustering in the embedded space.We summarize the proposed US-ELM in Algorithm2. Remark:Comparing the supervised ELM,the semi-supervised ELM and the unsupervised ELM,we can observe that all the algorithms have two similar stages in the training process,that is the random feature learning stage and the out-put weights learning stage.Under this two-stage framework,it is easy tofind the differences and similarities between the three algorithms.Actually,all the algorithms share the same stage of random feature learning,and this is the essence of the ELM theory.This also means that no matter the task is a supervised, semi-supervised or unsupervised learning problem,we can always follow the same step to generate the hidden layer. The differences of the three types of ELMs lie in the second stage on how the output weights are computed.In supervised ELM and SS-ELM,the output weights are trained by solving a regularized least squares problem;while the output weights in the US-ELM are obtained by solving a generalized eigenvalue problem.The unified framework for the three types of ELMs might provide new perspectives to further develop the ELM theory.VII.E XPERIMENTAL RESULTSWe evaluated our algorithms on wide range of semi-supervised and unsupervised parisons were made with related state-of-the-art algorithms, e.g.,Transductive SVM(TSVM)[54],LapSVM[47]and LapRLS[47]for semi-supervised learning;and Laplacian Eigenmap(LE)[50], spectral clustering(SC)[51]and deep autoencoder(DA)[55] for unsupervised learning.All algorithms were implemented using Matlab R2012a on a2.60GHz machine with4GB of memory.TABLE IIIT RAINING TIME(IN SECONDS)COMPARISON OF TSVM,L AP RLS,L AP SVM AND SS-ELMData set TSVM LapRLS LapSVM SS-ELMG50C0.3240.0410.0450.035COIL20(B)16.820.5120.4590.516USPST(B)68.440.9210.947 1.029COIL2018.43 5.841 4.9460.814USPST68.147.1217.259 1.373A.Semi-supervised learning results1)Data sets:We tested the SS-ELM onfive popular semi-supervised learning benchmarks,which have been widely usedfor evaluating semi-supervised algorithms[52],[56],[57].•The G50C is a binary classification data set of which each class is generated by a50-dimensional multivariate Gaus-sian distribution.This classification problem is explicitlydesigned so that the true Bayes error is5%.•The Columbia Object Image Library(COIL20)is a multi-class image classification data set which consists1440 gray-scale images of20objects.Each pattern is a32×32 gray scale image of one object taken from a specific view.The COIL20(B)data set is a binary classification taskobtained from COIL20by grouping thefirst10objectsas Class1,and the last10objects as Class2.•The USPST data set is a subset(the testing set)of the well known handwritten digit recognition data set USPS.The USPST(B)data set is a binary classification task obtained from USPST by grouping thefirst5digits as Class1and the last5digits as Class2.2)Experimental setup:We followed the experimental setup in[57]to evaluate the semi-supervised algorithms.Specifi-cally,each of the data sets is split into4folds,one of which was used for testing(denoted by T)and the rest3folds for training.Each of the folds was used as the testing set once(4-fold cross-validation).As in[57],this random fold generation process were repeated3times,resulted in12different splits in total.Every training set was further partitioned into a labeled set L,a validation set V,and an unlabeled set U.When we train a semi-supervised learning algorithm,the labeled data from L and the unlabeled data from U were used.The validation set which consists of labeled data was only used for model selection,i.e.,finding the optimal hyperparameters C0andλin the SS-ELM algorithm.The characteristics of the data sets used in our experiment are summarized in Table I. The training of SS-ELM consists of two stages:1)generat-ing the random hidden layer;and2)training the output weights using(17)or(18).In thefirst stage,we adopted the Sigmoid function for nonlinear mapping,and the input weights and biases were generated according to the uniform distribution on(-1,1).The number of hidden neurons n h wasfixed to 1000for G50C,and2000for the rest four data sets.In the second stage,wefirst need to build the graph Laplacian L.We followed the methods discussed in[52]and[57]to compute L,and the hyperparameter settings can be found in[47],[52] and[57].The trade off parameters C andλwere selected from。
tpo32三篇托福阅读TOEFL原文译文题目答案译文背景知识

tpo32三篇托福阅读TOEFL原文译文题目答案译文背景知识阅读-1 (2)原文 (2)译文 (5)题目 (7)答案 (16)背景知识 (16)阅读-2 (25)原文 (25)译文 (28)题目 (31)答案 (40)背景知识 (41)阅读-3 (49)原文 (49)译文 (53)题目 (55)答案 (63)背景知识 (64)阅读-1原文Plant Colonization①Colonization is one way in which plants can change the ecology of a site.Colonization is a process with two components:invasion and survival.The rate at which a site is colonized by plants depends on both the rate at which individual organisms(seeds,spores,immature or mature individuals)arrive at the site and their success at becoming established and surviving.Success in colonization depends to a great extent on there being a site available for colonization–a safe site where disturbance by fire or by cutting down of trees has either removed competing species or reduced levels of competition and other negative interactions to a level at which the invading species can become established.For a given rate of invasion,colonization of a moist,fertile site is likely to be much more rapid than that of a dry, infertile site because of poor survival on the latter.A fertile,plowed field is rapidly invaded by a large variety of weeds,whereas a neighboring construction site from which the soil has been compacted or removed to expose a coarse,infertile parent material may remain virtually free of vegetation for many months or even years despite receiving the same input of seeds as the plowed field.②Both the rate of invasion and the rate of extinction vary greatly among different plant species.Pioneer species-those that occur only in the earliest stages of colonization-tend to have high rates of invasion because they produce very large numbers of reproductive propagules(seeds,spores,and so on)and because they have an efficient means of dispersal(normally,wind).③If colonizers produce short-lived reproductive propagules,they must produce very large numbers unless they have an efficient means of dispersal to suitable new habitats.Many plants depend on wind for dispersal and produce abundant quantities of small,relatively short-lived seeds to compensate for the fact that wind is not always a reliable means If reaching the appropriate type of habitat.Alternative strategies have evolved in some plants,such as those that produce fewer but larger seeds that are dispersed to suitable sites by birds or small mammals or those that produce long-lived seeds.Many forest plants seem to exhibit the latter adaptation,and viable seeds of pioneer species can be found in large numbers on some forest floors. For example,as many as1,125viable seeds per square meter were found in a100-year-old Douglas fir/western hemlock forest in coastal British Columbia.Nearly all the seeds that had germinated from this seed bank were from pioneer species.The rapid colonization of such sites after disturbance is undoubtedly in part a reflection of the largeseed band on the forest floor.④An adaptation that is well developed in colonizing species is a high degree of variation in germination(the beginning of a seed’s growth). Seeds of a given species exhibit a wide range of germination dates, increasing the probability that at least some of the seeds will germinate during a period of favorable environmental conditions.This is particularly important for species that colonize an environment where there is no existing vegetation to ameliorate climatic extremes and in which there may be great climatic diversity.⑤Species succession in plant communities,i.e.,the temporal sequence of appearance and disappearance of species is dependent on events occurring at different stages in the life history of a species. Variation in rates of invasion and growth plays an important role in determining patterns of succession,especially secondary succession. The species that are first to colonize a site are those that produce abundant seed that is distributed successfully to new sites.Such species generally grow rapidly and quickly dominate new sites, excluding other species with lower invasion and growth rates.The first community that occupies a disturbed area therefore may be composed of specie with the highest rate of invasion,whereas the community of the subsequent stage may consist of plants with similar survival ratesbut lower invasion rates.译文植物定居①定居是植物改变一个地点生态环境的一种方式。
GRE阅读材料练习:摩天大楼向更高处伸展

GRE阅读材料练习:摩天大楼向更高处伸展A new lightweight lift cable will let buildings soar ever upward.WHEN Elisha Otis stood on a platform at the 1854 World Fair in New York and ordered an axeman to cut the rope used to hoist him aloft, he changed cityscapes for ever.To the amazement of the crowd his new safety lift dropped only a few inches before being held by an automatic braking system.This gave people the confidence to use what Americans insist on calling elevators.That confidence allowed buildings to rise higher and higher.一种新型的轻型升降梯将会让建筑连续向更高处进展.当艾利沙·奥的斯在1854年纽约世界博览会上站在一个高楼的阳台下,命令一个持斧的人砍断那个把他带到高空的绳索时,他彻底转变了人们对城市景观的印象。
为了吸引人群的眼光,在新的自动制动系统起动前,他只让他的新安全电梯降落了几英寸。
这让人们在使用电梯时-美国人坚持这个称呼有了足够的信念,也正是缘于这种信念,后来的建筑造得越来越高。
They could soon go higher still, as a result of anotherbreakthrough inlift technology.This week Kone, a Finnish liftmaker, announced that after a decade of development at its laboratory in Lohja, which sits above a 333-metre-deep mineshaft which the firm uses as a test bed, it has devised a system that should be able to raise an elevator a kilometre or more.This is twice as far as the things can go at present.Since the effectiveness of lifts is one of the main constraints on the height of buildings, Kone”s technology—which replaces the steel cables from which lift cars are currently suspended with ones made of carbon fibres—could result in buildings truly worthy of the name “skyscraper”.当另一种升降技术取得突破后,很快,高楼大厦将会连续往更高处进展。
新核心大学英语阶梯阅读3段落信息匹配题原文及翻译
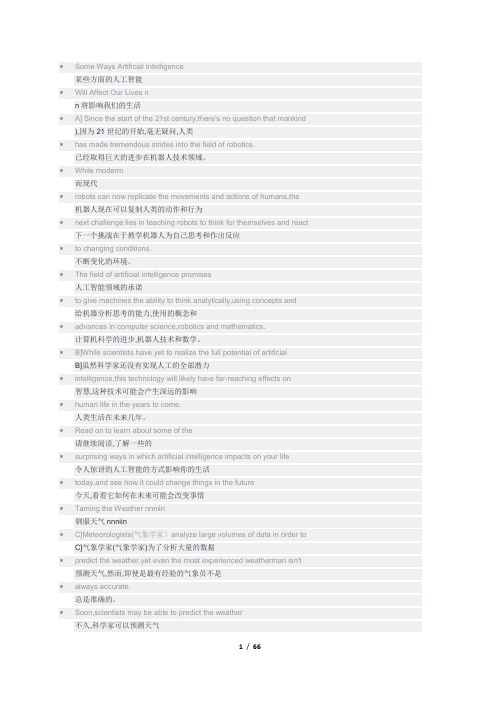
∙Some Ways Artificial Intelligence某些方面的人工智能∙Will Affect Our Lives nn将影响我们的生活∙A] Since the start of the 21st century,there's no question that mankind ),因为21世纪的开始,毫无疑问,人类∙has made tremendous strides into the field of robotics.已经取得巨大的进步在机器人技术领域。
∙While moderm而现代∙robots can now replicate the movements and actions of humans,the 机器人现在可以复制人类的动作和行为∙next challenge lies in teaching robots to think for themselves and react 下一个挑战在于教学机器人为自己思考和作出反应∙to changing conditions.不断变化的环境。
∙The field of artificial intelligence promises人工智能领域的承诺∙to give machines the ability to think analytically,using concepts and 给机器分析思考的能力,使用的概念和∙advances in computer science,robotics and mathematics.计算机科学的进步,机器人技术和数学。
∙B]While scientists have yet to realize the full potential of artificial B]虽然科学家还没有实现人工的全部潜力∙intelligence,this technology will likely have far-reaching effects on 智慧,这种技术可能会产生深远的影响∙human life in the years to come.人类生活在未来几年。
A Systematic Approach to Confinement in N=1 Supersymmetric Gauge Theories
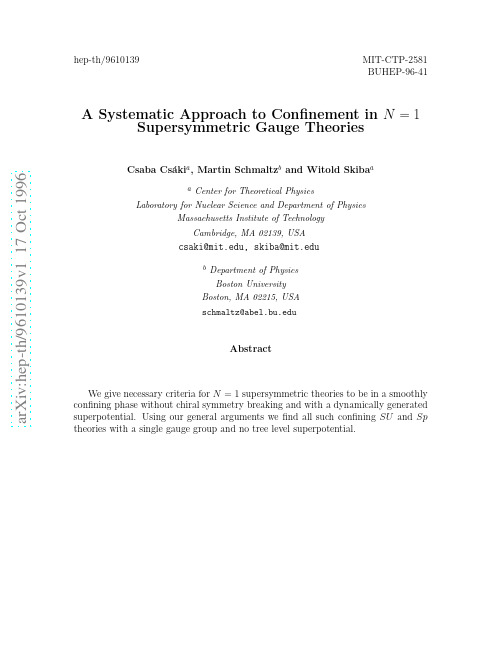
a r X i v :h e p -t h /9610139v 1 17 O c t 1996hep-th/9610139MIT-CTP-2581BUHEP-96-41A Systematic Approach to Confinement in N =1Supersymmetric Gauge TheoriesCsaba Cs´a ki a ,Martin Schmaltz b and Witold Skiba aaCenter for Theoretical Physics Laboratory for Nuclear Science and Department of Physics Massachusetts Institute of Technology Cambridge,MA 02139,USA csaki@,skiba@ b Department of Physics Boston University Boston,MA 02215,USA schmaltz@ Abstract We give necessary criteria for N =1supersymmetric theories to be in a smoothly confining phase without chiral symmetry breaking and with a dynamically generated ing our general arguments we find all such confining SU and Sptheories with a single gauge group and no tree level superpotential.Following the initial breakthrough in the works of Seiberg on exact results in N=1supersymmetric QCD(SQCD)[1],much progress has been made in extending these results to other theories with different gauge and matterfields[2-11].We now have a whole zoo of examples of supersymmetric theories for which we know results about the vacuum structure and the infrared spectrum.A number of theories are known to have dual descriptions,others are known to confine with or without chiral symmetry breaking,and some theories do not possess a stable ground state.Unfortunately,we are still lacking a systematic and general approach that allows one to determine the infrared properties of a given theory.The results in the literature have mostly been obtained by an ingenious guess of the infrared spectrum.This guess is then justified by performing a number of non-trivial consistency checks which include matching of the global anomalies,detailed study of the moduli space of vacua, and the behavior of the theory under perturbations.In this letter,we will depart from the customary trial and error procedure and give some general arguments which allow us to classify a subset of supersymmetric theories. To be specific,we intend to answer the general question of which supersymmetricfield theories may be confining without chiral symmetry breaking and with a confining superpotential.We present a few simple arguments which allow us to rule out most theories as possible candidates for confinement without chiral symmetry breaking.For the most part,these arguments already exist in the literature but our systematic way of putting them to use is new.As a demonstration of the power of our arguments we give a complete list of all SU(N)and Sp(N)gauge theories with no tree level superpotential which confine without chiral symmetry breaking,and we determine the confined degrees of freedom and the superpotential describing their interactions (“confining superpotential”).To begin,let usfirst explain what we mean by“smooth confinement without chiral symmetry breaking and with a non-vanishing confining superpotential”,which,from now on,we will abbreviate by s-confinement.We will call a theory confining when its infrared physics can be described exactly in terms of gauge invariant composites and their interactions.This description has to be valid everywhere on the moduli space of vacua.Our definition of s-confinement also requires that the theory dynamically generates a confining superpotential,which excludes models of the type presented in Ref.[11].Furthermore,the phrase“without chiral symmetry breaking”implies that the origin of the classical moduli space is also a vacuum in the quantum theory.In this vacuum,all the global symmetries of the ultraviolet remain unbroken.Finally,the confining superpotential is a holomorphic function of the confined degrees of freedom and couplings,which describes all the interactions in the extreme infrared.Note that this definition excludes theories which are in a Coulomb phase on a submanifold of the moduli space[2],or theories which have distinct Higgs and confining phases with associated phase boundaries on the moduli space.Our prototype example for an s-confining theory is Seiberg’s SQCD[1]with the number offlavors F chosen to equal N+1,where N is the number of colors,and a“flavor”is a pair of matterfields in the fundamental and antifundamental represen-tations of SU(N).Seiberg argued that the matterfields Q and¯Q are confined into “mesons”M=Q¯Q and“baryons”B=Q N,¯B=¯Q N.At the origin of moduli space all components of the mesons and baryons are massless and interact via the confining superpotential1W=1We normalize the index of the fundamental representation to1.integers.Therefore2, jµj−µ(G)=1or2,and for SU and Sp theories anomaly cancellation further constrainsjµj−µ(G)=2.(3)This formula constitutes a necessary condition for s-confinement,it enables us to rule out most theories immediately.For example,for SQCD wefind that the only candidate theory is the theory with F=N+1.Unfortunately,Eq.3is not a sufficient condition.An example for a theory which satisfies Eq.3but does not s-confine is SU(N)with an adjoint superfield and oneflavor.This theory is easily seen to be in an Abelian Coulomb phase for generic VEVs of the adjoint scalars and vanishing VEVs for the fundamentals.We could now simply examine all theories that satisfy Eq.3byfinding all in-dependent gauge invariants and checking if this ansatz for the confining spectrum matches the anomalies.Apart from being very cumbersome,this method is also not very useful to demonstrate that a given theory satisfying Eq.3is not s-confining.A better strategy relies on our second observation.An s-confining theory with a smooth description in terms of gauge invariants at the origin must also be s-confining everywhere on its moduli space.This is because the confining superpotential at the origin which is a simple polynomial in thefields is analytical everywhere,and no additional massless states are present anywhere on the moduli space.Therefore,the theory restricted to a particularflat direction must have a smooth description as well. This observation has two very useful applications.First,if we have a theory that s-confines and we know its confined spectrum and superpotential,we can easilyfind new s-confining theories by going to different points on moduli space.In the ultraviolet description,the gauge group is broken to a sub-group of the original group,some matterfields are eaten by the Higgs mechanism, and the remaining ones decompose under the unbroken subgroup.The corresponding confined description is obtained by simplyfinding the corresponding point on the moduli space of the confined theory.The global symmetries will be broken in the same way,and somefields may be massive and can be integrated out.This newly found confined theory is guaranteed to pass all the standard consistency checks be-cause they are a subset of the consistency checks for the original theory.For example, the anomalies of the new s-confining theory are guaranteed to match:the unbroken global symmetries are a subgroup of original global symmetries,and the anomalies under the subgroup are left unchanged–both in the infrared and ultraviolet descrip-tions–because the fermions which obtain masses give cancelling contributions to the anomalies.Second,the above observation can be turned around to provide another necessary condition for s-confinement.If anywhere on the moduli space of a given theory we find a theory which is not s-confining or completely higgsed,we know that the original theory cannot be s-confining either.Let us study some examples.Suppose we knew that SU(N)with N+1flavors for some large N is s-confining,then we could immediately conclude that the theories with n<N also s-confine.We simply need to give a VEV to some of the quark-antiquark pairs to break SU(N)to any SU(n)subgroup.The quarks with vevs are eaten,leaving n+1flavors and some singlets.We remove these singlets by adding “mirror”superfields with opposite global charges and giving them a mass.We now identify the corresponding point on the moduli space of the confined SU(N)theory. Somefields obtain masses from the superpotential of Eq.1when we expand around the new point in moduli space.After integrating the massivefields and removing the fields corresponding to the singlets in the ultraviolet theory via masses with mirror partners,we obtain the correct confined description of SU(n).A non-trivial example of a theory which can be shown to not s-confine is SU(4) with three antisymmetric tensors and twoflavors.This theory satisfies Eq.3and is therefore a candidate for s-confinement.By giving a VEV to an antisymmetric tensor we canflow from this theory to Sp(4)with two antisymmetric tensors and four fundamentals.VEVs for the other antisymmetric tensors let usflow further to SU(2) with eight fundamentals which is known to be at an interactingfixed point in the infrared.We conclude that the SU(4)and Sp(4)theories and all theories thatflow to them cannot be s-confining either.This allows us to rule out the following chain of theories,all of which are gauge anomaly free and satisfy Eq.3SU(7)→SU(6)→SU(5)→SU(4)→Sp(4)(4)432232Note that a VEV for one of the quarkflavors of the SU(4)theory lets usflow to an SU(3)theory with fourflavors which is s-confining.We must therefore be careful, when wefind aflow to an s-confining theory,it does not follow that the original theory is s-confining as well.Theflow is only a necessary condition.However,we suspect that a theory with a single gauge group and no tree-level superpotential is s-confining if it is found toflow to s-confining theories in all directions of its moduli space.We do not know of any counter examples.Armed with formula in Eq.3and our observation onflows of s-confining theories, we were able tofind all s-confining SU and Sp gauge theories with a single gauge group and no tree-level superpotential for arbitrary tensor representations.To achieve this, wefirst found all possible matter contents satisfying Eq.3.We list all these theories in Table1.We then studied the possibleflows of these theories and discarded all those withflows to theories which do not s-confine.This process eliminated all except about a dozen theories for which we then explicitly determined the independent gaugeinvariants and matched anomalies tofind the confining spectra.These results are summarized in Table1.Six of the ten theories which s-confine are new3:SU(N)with++3, SU(7)with2+6,SU(6)with2++4+4,and SU(5)with3+3.For the theories which do not s-confine we indicated the method by which we obtained this result:either by noting that the theory has a branch with only unbroken U(1)gauge groups,or else byflowing along aflat direction to a theory with smaller non-Abelian gauge group which does not s-confine.Detailed results on the new theories including the confining spectra,superpoten-tials,variousflows,and consistency checks will be reported elsewhere[14].Here,we just point out a few salient features.Most of the new s-confining theories contain vector-like matter.Perturbing these theories by adding mass terms for some of the vector-like matter,we easily obtain exact results on the theories with the matter integrated out.Among the theories that wefind in this way are new theories which confine with chiral symmetry breaking, theories with runaway vacua,and theories which confine without chiral symmetry breaking and vanishing superpotentials.Since many of the new theories presented here are chiral,they can be used tofind models of dynamical supersymmetry breaking along the lines of Refs.[15].Examples for such supersymmetry breaking theories will also be included in the detailed paper[14].Our s-confining theories might be used for building extensions of the standard model with composite quarks and leptons[16].Finally,we comment on possible exceptions and generalizations of our arguments.A possible exception to our condition in Eq.3arises,when allµi andµ(G)have a common divisor.Then the superpotential Eq.2can be holomorphic even when jµj−µ(G)= 2.However,whereas Eq.3is preserved under mostflows,the property that allµ’s have a common divisor is not.Therefore,such theoriesflow to theories which are not s-confining,and by our second necessary condition the original theory is not s-confining either.Another possibility is that the confining superpotential vanishes,and the confined degrees of freedom are free in the infrared.This can only happen if there are no clas-sical constraints among the basic gauge invariant operators which satisfy the’t Hooft anomaly matching conditions,otherwise the quantum solution would not have the correct classical limit.Examples of theories which are believed to confine in this way can be found in the literature[7,11,14].Generalizations to SO(N)groups are not completely straightforward because in the case of SO(N)theories“exotic composites”containing the chiral superfield Wαmight appear in the infrared spectrum and superpotential,thus modifying our argu-ment and result of Eq.3.SU(N)(N+1)(s-confiningSU(N)+N+4s-confiningSU(N)++)+SU(4)Adj++)SU(4)4+SU(2):+4Coulomb branchSU(5)3(+)+4)SU(5)2++) SU(6)2+5+s-confiningSU(6)2+SU(4):3+2(+4(s-confiningSU(6)+2+ ++Sp(6):SU(6)2+)SU(7)2(+3)+4+2SU(6):SU(7)+Sp(6):Sp(2N)(2N+4)s-confiningSp(2N)+6s-confiningSp(2N)+2Coulomb branchSp(4)3+2SU(2):+4SU(2):2Sp(6)2+2Sp(4):2+4+5Sp(4):2+4++SU(2):+4Sp(8)2Generalizations to theories with more than one gauge group or tree level super-potentials are more difficult.The additional interactions break some of the global symmetries which are now not sufficient to completely determine the functional form of the confining superpotential.Another complication is that in these theories theflat directions of the quantum theory are sometimes difficult to identify.Since our second argument only applies toflows in directions which are on the quantum moduli space, incorrect conclusions would be obtained fromflows along classicalflat directions which are notflat in the quantum theory.In summary,we have discussed general criteria for s-confinement and used them tofind all s-confining theories with SU(N)or Sp(2N)gauge groups.It is a pleasure to thank P.Cho,A.Cohen,N.Evans,L.Randall,and R.Sundrum for useful discussions.We also thank B.Dobrescu,A.Nelson,and J.Terning for comments on the manuscript. C.C.and W.S.are supported in part by the U.S. Department of Energy under cooperative agreement#DE-FC02-94ER40818.M.S.is supported by the U.S.Department of Energy under grant#DE-FG02-91ER40676. References[1]N.Seiberg,Phys.Rev.D49,6857(1994),hep-th/9402044;Nucl.Phys.B435,129(1995),hep-th/9411149.[2]K.Intriligator and N.Seiberg,Nucl.Phys.B431,551(1994),hep-th/9408155.[3]E.Poppitz and S.Trivedi,Phys.Lett.365B,125(1996),hep-th/9507169;P.Pouliot,Phys.Lett.367B,151(1996),hep-th/9510148.[4]K.Intriligator and P.Pouliot,Phys.Lett.353B,471(1995),hep-th/9505006.[5]P.Cho and P.Kraus,hep-th/9607200.[6]C.Cs´a ki,W.Skiba and M.Schmaltz,hep-th/9607210.[7]K.Intriligator and N.Seiberg,Nucl.Phys.B444,125(1995),hep-th/9503179.[8]P.Pouliot,Phys.Lett.359B,108(1995),hep-th/9507018;P.Pouliot and M.Strassler,Phys.Lett.370B,76(1996),hep-th/9510228;Phys.Lett.375B,175 (1996),hep-th/9602031.[9]I.Pesando,Mod.Phys.Lett.A10,1871(1995),hep-th/9506139;S.Giddingsand J.Pierre Phys.Rev.D52,6065(1995),hep-th/9506196.[10]K.Intriligator,R.G.Leigh,and M.J.Strassler,Nucl.Phys.B456,567(1995),hep-th/9506148;K.Intriligator,R.Leigh,and N.Seiberg,Phys.Rev.D50,1092 (1994),hep-th/9403198;D.Kutasov,Phys.Lett.351B,230(1995);D.Kutasovand A.Schwimmer,Phys.Lett.354B,315(1995);D.Kutasov,A.Schwimmer, and N.Seiberg,Nucl.Phys.B459,455(1996),hep-th/9510222;M.Luty,M.Schmaltz and J.Terning,hep-th/9603034;N.Evans and M.Schmaltz,hep-th/9609183.[11]K.Intriligator,N.Seiberg and S.Shenker,Phys.Lett.342B,152(1995),hep-th/9410203.[12]I.Affleck,M.Dine,and N.Seiberg,Nucl.Phys.B256,557(1985).[13]A.Nelson,private communication.[14]C.Cs´a ki,M.Schmaltz,and W.Skiba,to appear.[15]M.Dine,A.Nelson,Y.Nir and Y.Shirman,Phys.Rev.D53,2658(1996),hep-ph/9507378;K.Intriligator and S.Thomas,Nucl.Phys.B473,121(1996), hep-th/9603158;E.Poppitz,Y.Shadmi and S.Trivedi,hep-th/9605113,hep-th/9606184;C.Cs´a ki,L.Randall and W.Skiba,hep-th/9605108;C.Cs´a ki,L.Randall,W.Skiba and R.Leigh,hep-th/9607021.[16]A.Nelson and M.Strassler,hep-ph/9607362;A.Cohen,D.Kaplan and A.Nel-son,hep-ph/9607394.。
攀科学高树,摘创新之果作文
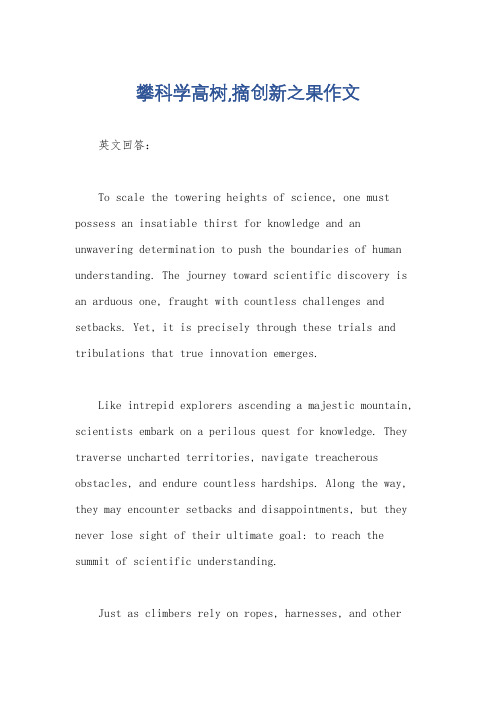
攀科学高树,摘创新之果作文英文回答:To scale the towering heights of science, one must possess an insatiable thirst for knowledge and an unwavering determination to push the boundaries of human understanding. The journey toward scientific discovery is an arduous one, fraught with countless challenges and setbacks. Yet, it is precisely through these trials and tribulations that true innovation emerges.Like intrepid explorers ascending a majestic mountain, scientists embark on a perilous quest for knowledge. They traverse uncharted territories, navigate treacherous obstacles, and endure countless hardships. Along the way, they may encounter setbacks and disappointments, but they never lose sight of their ultimate goal: to reach the summit of scientific understanding.Just as climbers rely on ropes, harnesses, and othertools to ascend a mountain, scientists utilize a variety of methods to conquer scientific challenges. They conduct rigorous experiments, analyze data, formulate hypotheses, and test their theories. Through a process of trial and error, they gradually chip away at the unknown, revealing the secrets of nature one step at a time.As scientists ascend the ladder of knowledge, they encounter new and exciting discoveries that illuminate our understanding of the world. These discoveries can take many forms, from groundbreaking theories to practical inventions that improve our lives. They have the power to transform our societies, cure diseases, and unlock the potential of the human spirit.However, the pursuit of scientific innovation is not without its risks. Scientists often venture into uncharted territory, where the potential for error and even danger is high. They may face skepticism or opposition from those who cling to outdated beliefs or vested interests. But true innovators press on, driven by an unstoppable desire to unveil the mysteries that lie ahead.In the annals of science, countless individuals have scaled the heights of their disciplines and plucked thefruits of innovation. From Albert Einstein's groundbreaking theories of relativity to Marie Curie's discovery of radium, scientific discoveries have shaped the course of human history and continue to inspire generations to come.Today, the challenges facing our planet are more pressing than ever before. Climate change, environmental degradation, and disease pose serious threats to our future. It is up to the next generation of scientists to climb the ladder of knowledge and develop innovative solutions that will ensure a sustainable and prosperous world for all.The path to scientific innovation is not an easy one, but it is a journey worth taking. By embracing challenges, persevering through setbacks, and never losing sight of the ultimate goal, we can scale the towering heights of science and pluck the fruits of a brighter future.中文回答:攀登科学的高峰,摘取创新的果实是一段艰辛而漫长的旅程,需要我们付出巨大的努力和不懈的坚持。
- 1、下载文档前请自行甄别文档内容的完整性,平台不提供额外的编辑、内容补充、找答案等附加服务。
- 2、"仅部分预览"的文档,不可在线预览部分如存在完整性等问题,可反馈申请退款(可完整预览的文档不适用该条件!)。
- 3、如文档侵犯您的权益,请联系客服反馈,我们会尽快为您处理(人工客服工作时间:9:00-18:30)。
Annals of Combinatorics10(2006)31-51 0218-0006/06/010031-21
DOI10.1007/s00026-006-0272-z
c Birkh¨a user Verlag,Basel,2006 Annals of Combinatorics
Extending the Limits of Supertree Methods
Magnus Bordewich1∗,Gareth Evans2,and Charles Semple2†
1School of Computing,University of Leeds,Leeds,United Kingdom
magnusb@
2Biomathematics Research Centre,Department of Mathematics and Statistics,University of Can-terbury,Christchurch,New Zealand
gee16@,c.semple@
Received June14,2004
AMS Subject Classification:05C05;92D15 Abstract.Recently,two exact polynomial-time supertree methods have been developed in which the traditional input of rooted leaf-labelled trees has been extended in two separate ways.The first method,called R ANKED T REE,allows for the inclusion of relative divergence dates and the second method,called A NCESTRAL B UILD,allows for the inclusion of rooted trees in which some of the interior vertices as well as the leaves are labelled.The latter is particular useful for when one has information that includes nested taxa.In this paper,we present two supertree methods that unite and generalise R ANKED T REE and A NCESTRAL B UILD.Thefirst method is polynomial time and combines the allowable inputs of R ANKED T REE and A NCESTRAL B UILD. It determines if the original input is compatible,in which case it outputs an appropriate‘ranked semi-labelled tree’.The second method lists all‘ranked semi-labelled trees’that are consistent with the original input.While there may be an exponential number of such trees,the second method outputs the next such tree in the list in polynomial time.
Keywords:Supertree methods,nested taxa,AncestralBuild,RankedTree
References
1. A.V.Aho,S.Yehoshua,T.G.Szymanski,and J.D.Ullman,Inferring a tree from lowest
common ancestors with an application to the optimization of relational expressions,SIAM put.10(1981)405–421.
2.M.Bordewich and C.Semple,Counting consistent phylogenetic trees is#P-complete,Adv.
in Appl.Math.33(2004)416–430.
3. D.Bryant,C.Semple,and M.Steel,Supertree methods for ancestral divergence dates and
other applications,In:Phylogenetic Supertrees:Combining Information to Reveal the Tree of Life,O.Bininda-Emonds,Ed.,Computational Biology Series,Kluwer,(2004)pp.129–150.
∗Supported by the New Zealand Institute of Mathematics and its Applications funded programme Phyloge-netic Genomics and the work was conducted while at the University of Canterbury.
†Supported by the New Zealand Marsden Fund(UOC301).
31
32M.Bordewich,G.Evans,and C.Semple 4.M.Constantinescu and D.Sankoff,An efficient algorithm for supertrees,J.Classification12
(1995)101–112.
5.P.Daniel,W.Hordijk,R.D.M.Page,C.Semple,and M.Steel,Supertree algorithms for
ancestral divergence dates and nested taxa,Bioinformatics20(2004)2355–2360.
6.P.Daniel and C.Semple,Supertree algorithms for nested taxa,In:Phylogenetic Supertrees:
Combining Information to Reveal the Tree of Life,O.Bininda-Emonds,Ed.,Computational Biology Series,Kluwer,(2004)pp.151–171.
7.P.Daniel and C.Semple,A class of general supertree methods for nested taxa,SIAM J.
Discrete Math.,to appear.
8.M.P.Ng and N.C.Wormald,Reconstruction of rooted trees from subtrees,Discrete Appl.
Math.69(1996)19–31.
9. C.Semple,Reconstructing minimal rooted trees,Discrete Appl.Math.127(2003)489–503.
10. C.Semple and M.Steel,Phylogenetics,Oxford University Press,2003.。