eigenfaces for recognition
人脸表情识别英文参考资料
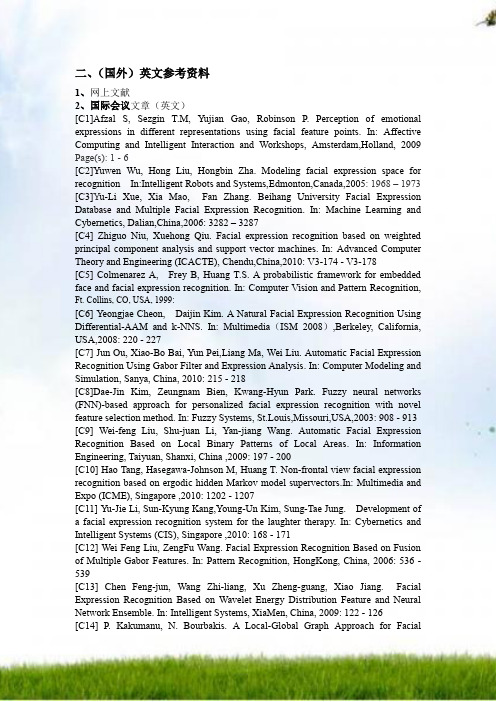
二、(国外)英文参考资料1、网上文献2、国际会议文章(英文)[C1]Afzal S, Sezgin T.M, Yujian Gao, Robinson P. Perception of emotional expressions in different representations using facial feature points. In: Affective Computing and Intelligent Interaction and Workshops, Amsterdam,Holland, 2009 Page(s): 1 - 6[C2]Yuwen Wu, Hong Liu, Hongbin Zha. Modeling facial expression space for recognition In:Intelligent Robots and Systems,Edmonton,Canada,2005: 1968 – 1973 [C3]Y u-Li Xue, Xia Mao, Fan Zhang. Beihang University Facial Expression Database and Multiple Facial Expression Recognition. In: Machine Learning and Cybernetics, Dalian,China,2006: 3282 – 3287[C4] Zhiguo Niu, Xuehong Qiu. Facial expression recognition based on weighted principal component analysis and support vector machines. In: Advanced Computer Theory and Engineering (ICACTE), Chendu,China,2010: V3-174 - V3-178[C5] Colmenarez A, Frey B, Huang T.S. A probabilistic framework for embedded face and facial expression recognition. In: Computer Vision and Pattern Recognition, Ft. Collins, CO, USA, 1999:[C6] Yeongjae Cheon, Daijin Kim. A Natural Facial Expression Recognition Using Differential-AAM and k-NNS. In: Multimedia(ISM 2008),Berkeley, California, USA,2008: 220 - 227[C7]Jun Ou, Xiao-Bo Bai, Yun Pei,Liang Ma, Wei Liu. Automatic Facial Expression Recognition Using Gabor Filter and Expression Analysis. In: Computer Modeling and Simulation, Sanya, China, 2010: 215 - 218[C8]Dae-Jin Kim, Zeungnam Bien, Kwang-Hyun Park. Fuzzy neural networks (FNN)-based approach for personalized facial expression recognition with novel feature selection method. In: Fuzzy Systems, St.Louis,Missouri,USA,2003: 908 - 913 [C9] Wei-feng Liu, Shu-juan Li, Yan-jiang Wang. Automatic Facial Expression Recognition Based on Local Binary Patterns of Local Areas. In: Information Engineering, Taiyuan, Shanxi, China ,2009: 197 - 200[C10] Hao Tang, Hasegawa-Johnson M, Huang T. Non-frontal view facial expression recognition based on ergodic hidden Markov model supervectors.In: Multimedia and Expo (ICME), Singapore ,2010: 1202 - 1207[C11] Yu-Jie Li, Sun-Kyung Kang,Young-Un Kim, Sung-Tae Jung. Development of a facial expression recognition system for the laughter therapy. In: Cybernetics and Intelligent Systems (CIS), Singapore ,2010: 168 - 171[C12] Wei Feng Liu, ZengFu Wang. Facial Expression Recognition Based on Fusion of Multiple Gabor Features. In: Pattern Recognition, HongKong, China, 2006: 536 - 539[C13] Chen Feng-jun, Wang Zhi-liang, Xu Zheng-guang, Xiao Jiang. Facial Expression Recognition Based on Wavelet Energy Distribution Feature and Neural Network Ensemble. In: Intelligent Systems, XiaMen, China, 2009: 122 - 126[C14] P. Kakumanu, N. Bourbakis. A Local-Global Graph Approach for FacialExpression Recognition. In: Tools with Artificial Intelligence, Arlington, Virginia, USA,2006: 685 - 692[C15] Mingwei Huang, Zhen Wang, Zilu Ying. Facial expression recognition using Stochastic Neighbor Embedding and SVMs. In: System Science and Engineering (ICSSE), Macao, China, 2011: 671 - 674[C16] Junhua Li, Li Peng. Feature difference matrix and QNNs for facial expression recognition. In: Control and Decision Conference, Yantai, China, 2008: 3445 - 3449 [C17] Yuxiao Hu, Zhihong Zeng, Lijun Yin,Xiaozhou Wei, Jilin Tu, Huang, T.S. A study of non-frontal-view facial expressions recognition. In: Pattern Recognition, Tampa, FL, USA,2008: 1 - 4[C18] Balasubramani A, Kalaivanan K, Karpagalakshmi R.C, Monikandan R. Automatic facial expression recognition system. In: Computing, Communication and Networking, St. Thomas,USA, 2008: 1 - 5[C19] Hui Zhao, Zhiliang Wang, Jihui Men. Facial Complex Expression Recognition Based on Fuzzy Kernel Clustering and Support Vector Machines. In: Natural Computation, Haikou,Hainan,China,2007: 562 - 566[C20] Khanam A, Shafiq M.Z, Akram M.U. Fuzzy Based Facial Expression Recognition. In: Image and Signal Processing, Sanya, Hainan, China,2008: 598 - 602 [C21] Sako H, Smith A.V.W. Real-time facial expression recognition based on features' positions and dimensions. In: Pattern Recognition, Vienna,Austria, 1996: 643 - 648 vol.3[C22] Huang M.W, Wang Z.W, Ying Z.L. A novel method of facial expression recognition based on GPLVM Plus SVM. In: Signal Processing (ICSP), Beijing, China, 2010: 916 - 919[C23] Xianxing Wu; Jieyu Zhao; Curvelet feature extraction for face recognition and facial expression recognition. In: Natural Computation (ICNC), Yantai, China, 2010: 1212 - 1216[C24]Xu Q Z, Zhang P Z, Yang L X, et al.A facial expression recognition approach based on novel support vector machine tree. In Proceedings of the 4th International Symposium on Neural Networks, Nanjing, China, 2007: 374-381.[C25] Wang Y B, Ai H Z, Wu B, et al. Real time facial expression recognition with adaboost.In: Proceedings of the 17th International Conference on Pattern Recognition , Cambridge,UK, 2004: 926-929.[C26] Guo G, Dyer C R. Simultaneous feature selection and classifier training via linear programming: a case study for face expression recognition. In: Proceedings of the 2003 IEEE Computer Society Conference on Computer Vision and Pattern Recognition, Madison, W isconsin, USA, 2003,1: 346-352.[C27] Bourel F, Chibelushi C C, Low A A. Robust facial expression recognition using a state-based model of spatially-localised facial dynamics. In: Proceedings of the 5th IEEE International Conference on Automatic Face and Gesture Recognition, Washington, DC, USA, 2002: 113-118·[C28] Buciu I, Kotsia I, Pitas I. Facial expression analysis under partial occlusion. In: Proceedings of the 2005 IEEE International Conference on Acoustics, Speech, and Signal Processing, Philadelphia, PA, USA, 2005,V: 453-456.[C29] ZHAN Yong-zhao,YE Jing-fu,NIU De-jiao,et al.Facial expression recognition based on Gabor wavelet transformation and elastic templates matching. Proc of the 3rd International Conference on Image and Graphics.Washington DC, USA,2004:254-257.[C30] PRASEEDA L V,KUMAR S,VIDYADHARAN D S,et al.Analysis of facial expressions using PCA on half and full faces. Proc of ICALIP2008.2008:1379-1383.[C31] LEE J J,UDDIN M Z,KIM T S.Spatiotemporal human facial expression recognition using Fisher independent component analysis and Hidden Markov model [C]//Proc of the 30th Annual International Conference of IEEE Engineering in Medicine and Biology Society.2008:2546-2549.[C32] LITTLEWORT G,BARTLETT M,FASELL. Dynamics of facial expression extracted automatically from video. In: Proceedings of IEEE Conference on Computer Vision and Pattern Recognition, Workshop on Face Processing inVideo, Washington DC,USA,2006:80-81.[C33] Kotsia I, Nikolaidis N, Pitas I. Facial Expression Recognition in Videos using a Novel Multi-Class Support Vector Machines Variant. In: Acoustics, Speech and Signal Processing, Honolulu, Hawaii, USA, 2007: II-585 - II-588[C34] Ruo Du, Qiang Wu, Xiangjian He, Wenjing Jia, Daming Wei. Facial expression recognition using histogram variances faces. In: Applications of Computer Vision (WACV), Snowbird, Utah, USA, 2009: 1 - 7[C35] Kobayashi H, Tange K, Hara F. Real-time recognition of six basic facial expressions. In: Robot and Human Communication, Tokyo , Japan,1995: 179 - 186 [C36] Hao Tang, Huang T.S. 3D facial expression recognition based on properties of line segments connecting facial feature points. In: Automatic Face & Gesture Recognition, Amsterdam, The Netherlands, 2008: 1 - 6[C37] Fengjun Chen, Zhiliang Wang, Zhengguang Xu, Donglin Wang. Research on a method of facial expression recognition.in: Electronic Measurement & Instruments, Beijing,China, 2009: 1-225 - 1-229[C38] Hui Zhao, Tingting Xue, Linfeng Han. Facial complex expression recognition based on Latent DirichletAllocation. In: Natural Computation (ICNC), Yantai, Shandong, China, 2010: 1958 - 1960[C39] Qinzhen Xu, Pinzheng Zhang, Wenjiang Pei, Luxi Yang, Zhenya He. An Automatic Facial Expression Recognition Approach Based on Confusion-Crossed Support Vector Machine Tree. In: Acoustics, Speech and Signal Processing, Honolulu, Hawaii, USA, 2007: I-625 - I-628[C40] Sung Uk Jung, Do Hyoung Kim, Kwang Ho An, Myung Jin Chung. Efficient rectangle feature extraction for real-time facial expression recognition based on AdaBoost.In: Intelligent Robots and Systems, Edmonton,Canada, 2005: 1941 - 1946[C41] Patil K.K, Giripunje S.D, Bajaj P.R. Facial Expression Recognition and Head Tracking in Video Using Gabor Filter .In: Emerging Trends in Engineering and Technology (ICETET), Goa, India, 2010: 152 - 157[C42] Jun Wang, Lijun Yin, Xiaozhou Wei, Yi Sun. 3D Facial Expression Recognition Based on Primitive Surface Feature Distribution.In: Computer Vision and PatternRecognition, New York, USA,2006: 1399 - 1406[C43] Shi Dongcheng, Jiang Jieqing. The method of facial expression recognition based on DWT-PCA/LDA.IN: Image and Signal Processing (CISP), Yantai,China, 2010: 1970 - 1974[C44] Asthana A, Saragih J, Wagner M, Goecke R. Evaluating AAM fitting methods for facial expression recognition. In: Affective Computing and Intelligent Interaction and Workshops, Amsterdam,Holland, 2009:1-8[C45] Geng Xue, Zhang Youwei. Facial Expression Recognition Based on the Difference of Statistical Features.In: Signal Processing, Guilin, China, 2006[C46] Metaxas D. Facial Features Tracking for Gross Head Movement analysis and Expression Recognition.In: Multimedia Signal Processing, Chania,Crete,GREECE, 2007:2[C47] Xia Mao, YuLi Xue, Zheng Li, Kang Huang, ShanWei Lv. Robust facial expression recognition based on RPCA and AdaBoost.In: Image Analysis for Multimedia Interactive Services, London, UK, 2009: 113 - 116[C48] Kun Lu, Xin Zhang. Facial Expression Recognition from Image Sequences Based on Feature Points and Canonical Correlations.In: Artificial Intelligence and Computational Intelligence (AICI), Sanya,China, 2010: 219 - 223[C49] Peng Zhao-yi, Wen Zhi-qiang, Zhou Yu. Application of Mean Shift Algorithm in Real-Time Facial Expression Recognition.In: Computer Network and Multimedia Technology, Wuhan,China, 2009: 1 - 4[C50] Xu Chao, Feng Zhiyong, Facial Expression Recognition and Synthesis on Affective Emotions Composition.In: Future BioMedical Information Engineering, Wuhan,China, 2008: 144 - 147[C51] Zi-lu Ying, Lin-bo Cai. Facial Expression Recognition with Marginal Fisher Analysis on Local Binary Patterns.In: Information Science and Engineering (ICISE), Nanjing,China, 2009: 1250 - 1253[C52] Chuang Yu, Yuning Hua, Kun Zhao. The Method of Human Facial Expression Recognition Based on Wavelet Transformation Reducing the Dimension and Improved Fisher Discrimination.In: Intelligent Networks and Intelligent Systems (ICINIS), Shenyang,China, 2010: 43 - 47[C53] Stratou G, Ghosh A, Debevec P, Morency L.-P. Effect of illumination on automatic expression recognition: A novel 3D relightable facial database .In: Automatic Face & Gesture Recognition and Workshops (FG 2011), Santa Barbara, California,USA, 2011: 611 - 618[C54] Jung-Wei Hong, Kai-Tai Song. Facial expression recognition under illumination variation.In: Advanced Robotics and Its Social Impacts, Hsinchu, Taiwan,2007: 1 - 6[C55] Ryan A, Cohn J.F, Lucey S, Saragih J, Lucey P, De la Torre F, Rossi A. Automated Facial Expression Recognition System.In: Security Technology, Zurich, Switzerland, 2009: 172 - 177[C56] Gokturk S.B, Bouguet J.-Y, Tomasi C, Girod B. Model-based face tracking for view-independent facial expression recognition.In: Automatic Face and Gesture Recognition, Washington, D.C., USA, 2002: 287 - 293[C57] Guo S.M, Pan Y.A, Liao Y.C, Hsu C.Y, Tsai J.S.H, Chang C.I. A Key Frame Selection-Based Facial Expression Recognition System.In: Innovative Computing, Information and Control, Beijing,China, 2006: 341 - 344[C58] Ying Zilu, Li Jingwen, Zhang Youwei. Facial expression recognition based on two dimensional feature extraction.In: Signal Processing, Leipzig, Germany, 2008: 1440 - 1444[C59] Fengjun Chen, Zhiliang Wang, Zhengguang Xu, Jiang Xiao, Guojiang Wang. Facial Expression Recognition Using Wavelet Transform and Neural Network Ensemble.In: Intelligent Information Technology Application, Shanghai,China,2008: 871 - 875[C60] Chuan-Yu Chang, Yan-Chiang Huang, Chi-Lu Yang. Personalized Facial Expression Recognition in Color Image.In: Innovative Computing, Information and Control (ICICIC), Kaohsiung,Taiwan, 2009: 1164 - 1167[C61] Bourel F, Chibelushi C.C, Low A.A. Robust facial expression recognition using a state-based model of spatially-localised facial dynamics. In: Automatic Face and Gesture Recognition, Washington, D.C., USA, 2002: 106 - 111[C62] Chen Juanjuan, Zhao Zheng, Sun Han, Zhang Gang. Facial expression recognition based on PCA reconstruction.In: Computer Science and Education (ICCSE), Hefei,China, 2010: 195 - 198[C63] Guotai Jiang, Xuemin Song, Fuhui Zheng, Peipei Wang, Omer A.M. Facial Expression Recognition Using Thermal Image.In: Engineering in Medicine and Biology Society, Shanghai,China, 2005: 631 - 633[C64] Zhan Yong-zhao, Ye Jing-fu, Niu De-jiao, Cao Peng. Facial expression recognition based on Gabor wavelet transformation and elastic templates matching.In: Image and Graphics, Hongkong,China, 2004: 254 - 257[C65] Ying Zilu, Zhang Guoyi. Facial Expression Recognition Based on NMF and SVM. In: Information Technology and Applications, Chengdu,China, 2009: 612 - 615 [C66] Xinghua Sun, Hongxia Xu, Chunxia Zhao, Jingyu Yang. Facial expression recognition based on histogram sequence of local Gabor binary patterns. In: Cybernetics and Intelligent Systems, Chengdu,China, 2008: 158 - 163[C67] Zisheng Li, Jun-ichi Imai, Kaneko M. Facial-component-based bag of words and PHOG descriptor for facial expression recognition.In: Systems, Man and Cybernetics, San Antonio,TX,USA,2009: 1353 - 1358[C68] Chuan-Yu Chang, Yan-Chiang Huang. Personalized facial expression recognition in indoor environments.In: Neural Networks (IJCNN), Barcelona, Spain, 2010: 1 - 8[C69] Ying Zilu, Fang Xieyan. Combining LBP and Adaboost for facial expression recognition.In: Signal Processing, Leipzig, Germany, 2008: 1461 - 1464[C70] Peng Yang, Qingshan Liu, Metaxas, D.N. RankBoost with l1 regularization for facial expression recognition and intensity estimation.In: Computer Vision, Kyoto,Japan, 2009: 1018 - 1025[C71] Patil R.A, Sahula V, Mandal A.S. Automatic recognition of facial expressions in image sequences: A review.In: Industrial and Information Systems (ICIIS), Mangalore, India, 2010: 408 - 413[C72] Iraj Hosseini, Nasim Shams, Pooyan Amini, Mohammad S. Sadri, Masih Rahmaty, Sara Rahmaty. Facial Expression Recognition using Wavelet-Based Salient Points and Subspace Analysis Methods.In: Electrical and Computer Engineering, Ottawa, Canada, 2006: 1992 - 1995[C73][C74][C75][C76][C77][C78][C79]3、英文期刊文章[J1] Aleksic P.S., Katsaggelos A.K. Automatic facial expression recognition using facial animation parameters and multistream HMMs.IEEE Transactions on Information Forensics and Security, 2006, 1(1):3-11[J2] Kotsia I,Pitas I. Facial Expression Recognition in Image Sequences Using Geometric Deformation Features and Support Vector Machines. IEEE Transactions on Image Processing, 2007, 16(1):172 – 187[J3] Mpiperis I, Malassiotis S, Strintzis M.G. Bilinear Models for 3-D Face and Facial Expression Recognition. IEEE Transactions on Information Forensics and Security, 2008,3(3) : 498 - 511[J4] Sung J, Kim D. Pose-Robust Facial Expression Recognition Using View-Based 2D+3D AAM. IEEE Transactions on Systems and Humans, 2008 , 38 (4): 852 - 866 [J5]Yeasin M, Bullot B, Sharma R. Recognition of facial expressions and measurement of levels of interest from video. IEEE Transactions on Multimedia, 2006, 8(3): 500 - 508[J6] Wenming Zheng, Xiaoyan Zhou, Cairong Zou, Li Zhao. Facial expression recognition using kernel canonical correlation analysis (KCCA). IEEE Transactions on Neural Networks, 2006, 17(1): 233 - 238[J7]Pantic M, Patras I. Dynamics of facial expression: recognition of facial actions and their temporal segments from face profile image sequences. IEEE Transactions on Systems, Man, and Cybernetics, Part B: Cybernetics, 2006, 36(2): 433 - 449[J8] Mingli Song, Dacheng Tao, Zicheng Liu, Xuelong Li, Mengchu Zhou. Image Ratio Features for Facial Expression Recognition Application. IEEE Transactions on Systems, Man, and Cybernetics, Part B: Cybernetics, 2010, 40(3): 779 - 788[J9] Dae Jin Kim, Zeungnam Bien. Design of “Personalized” Classifier Using Soft Computing Techniques for “Personalized” Facial Expression Recognition. IEEE Transactions on Fuzzy Systems, 2008, 16(4): 874 - 885[J10] Uddin M.Z, Lee J.J, Kim T.-S. An enhanced independent component-based human facial expression recognition from video. IEEE Transactions on Consumer Electronics, 2009, 55(4): 2216 - 2224[J11] Ruicong Zhi, Flierl M, Ruan Q, Kleijn W.B. Graph-Preserving Sparse Nonnegative Matrix Factorization With Application to Facial Expression Recognition.IEEE Transactions on Systems, Man, and Cybernetics, Part B: Cybernetics, 2011, 41(1): 38 - 52[J12] Chibelushi C.C, Bourel F. Hierarchical multistream recognition of facial expressions. IEE Proceedings - Vision, Image and Signal Processing, 2004, 151(4): 307 - 313[J13] Yongsheng Gao, Leung M.K.H, Siu Cheung Hui, Tananda M.W. Facial expression recognition from line-based caricatures. IEEE Transactions on Systems, Man and Cybernetics, Part A: Systems and Humans, 2003, 33(3): 407 - 412[J14] Ma L, Khorasani K. Facial expression recognition using constructive feedforward neural networks. IEEE Transactions on Systems, Man, and Cybernetics, Part B: Cybernetics, 2004, 34(3): 1588 - 1595[J15] Essa I.A, Pentland A.P. Coding, analysis, interpretation, and recognition of facial expressions. IEEE Transactions on Pattern Analysis and Machine Intelligence, 1997, 19(7): 757 - 763[J16] Anderson K, McOwan P.W. A real-time automated system for the recognition of human facial expressions. IEEE Transactions on Systems, Man, and Cybernetics, Part B: Cybernetics, 2006, 36(1): 96 - 105[J17] Soyel H, Demirel H. Facial expression recognition based on discriminative scale invariant feature transform. Electronics Letters 2010, 46(5): 343 - 345[J18] Fei Cheng, Jiangsheng Yu, Huilin Xiong. Facial Expression Recognition in JAFFE Dataset Based on Gaussian Process Classification. IEEE Transactions on Neural Networks, 2010, 21(10): 1685 – 1690[J19] Shangfei Wang, Zhilei Liu, Siliang Lv, Yanpeng Lv, Guobing Wu, Peng Peng, Fei Chen, Xufa Wang. A Natural Visible and Infrared Facial Expression Database for Expression Recognition and Emotion Inference. IEEE Transactions on Multimedia, 2010, 12(7): 682 - 691[J20] Lajevardi S.M, Hussain Z.M. Novel higher-order local autocorrelation-like feature extraction methodology for facial expression recognition. IET Image Processing, 2010, 4(2): 114 - 119[J21] Yizhen Huang, Ying Li, Na Fan. Robust Symbolic Dual-View Facial Expression Recognition With Skin Wrinkles: Local Versus Global Approach. IEEE Transactions on Multimedia, 2010, 12(6): 536 - 543[J22] Lu H.-C, Huang Y.-J, Chen Y.-W. Real-time facial expression recognition based on pixel-pattern-based texture feature. Electronics Letters 2007, 43(17): 916 - 918[J23]Zhang L, Tjondronegoro D. Facial Expression Recognition Using Facial Movement Features. IEEE Transactions on Affective Computing, 2011, pp(99): 1[J24] Zafeiriou S, Pitas I. Discriminant Graph Structures for Facial Expression Recognition. Multimedia, IEEE Transactions on 2008,10(8): 1528 - 1540[J25]Oliveira L, Mansano M, Koerich A, de Souza Britto Jr. A. Selecting 2DPCA Coefficients for Face and Facial Expression Recognition. Computing in Science & Engineering, 2011, pp(99): 1[J26] Chang K.I, Bowyer W, Flynn P.J. Multiple Nose Region Matching for 3D Face Recognition under Varying Facial Expression. Pattern Analysis and Machine Intelligence, IEEE Transactions on2006, 28(10): 1695 - 1700[J27] Kakadiaris I.A, Passalis G, Toderici G, Murtuza M.N, Yunliang Lu, Karampatziakis N, Theoharis T. Three-Dimensional Face Recognition in the Presence of Facial Expressions: An Annotated Deformable Model Approach.IEEE Transactions on Pattern Analysis and Machine Intelligence, 2007, 29(4): 640 - 649[J28] Guoying Zhao, Pietikainen M. Dynamic Texture Recognition Using Local Binary Patterns with an Application to Facial Expressions. IEEE Transactions on Pattern Analysis and Machine Intelligence, 2007, 29(6): 915 - 928[J29] Chakraborty A, Konar A, Chakraborty U.K, Chatterjee A. Emotion Recognition From Facial Expressions and Its Control Using Fuzzy Logic. IEEE Transactions on Systems, Man and Cybernetics, Part A: Systems and Humans, 2009, 39(4): 726 - 743 [J30] Pantic M, RothkrantzL J.M. Facial action recognition for facial expression analysis from static face images. IEEE Transactions on Systems, Man, and Cybernetics, Part B: Cybernetics, 2004, 34(3): 1449 - 1461[J31] Calix R.A, Mallepudi S.A, Bin Chen, Knapp G.M. Emotion Recognition in Text for 3-D Facial Expression Rendering. IEEE Transactions on Multimedia, 2010, 12(6): 544 - 551[J32]Kotsia I, Pitas I, Zafeiriou S, Zafeiriou S. Novel Multiclass Classifiers Based on the Minimization of the Within-Class Variance. IEEE Transactions on Neural Networks, 2009, 20(1): 14 - 34[J33]Cohen I, Cozman F.G, Sebe N, Cirelo M.C, Huang T.S. Semisupervised learning of classifiers: theory, algorithms, and their application to human-computer interaction. IEEE Transactions on Pattern Analysis and Machine Intelligence, 2004, 26(12): 1553 - 1566[J34] Zafeiriou S. Discriminant Nonnegative Tensor Factorization Algorithms. IEEE Transactions on Neural Networks, 2009, 20(2): 217 - 235[J35] Zafeiriou S, Petrou M. Nonlinear Non-Negative Component Analysis Algorithms. IEEE Transactions on Image Processing, 2010, 19(4): 1050 - 1066[J36] Kotsia I, Zafeiriou S, Pitas I. A Novel Discriminant Non-Negative Matrix Factorization Algorithm With Applications to Facial Image Characterization Problems. IEEE Transactions on Information Forensics and Security, 2007, 2(3): 588 - 595[J37] Irene Kotsia, Stefanos Zafeiriou, Ioannis Pitas. Texture and shape information fusion for facial expression and facial action unit recognition . Pattern Recognition, 2008, 41(3): 833-851[J38]Wenfei Gu, Cheng Xiang, Y.V. Venkatesh, Dong Huang, Hai Lin. Facial expression recognition using radial encoding of local Gabor features and classifier synthesis. Pattern Recognition, In Press, Corrected Proof, Available online 27 May 2011[J39] F Dornaika, E Lazkano, B Sierra. Improving dynamic facial expression recognition with feature subset selection. Pattern Recognition Letters, 2011, 32(5): 740-748[J40] Te-Hsun Wang, Jenn-Jier James Lien. Facial expression recognition system based on rigid and non-rigid motion separation and 3D pose estimation. Pattern Recognition, 2009, 42(5): 962-977[J41] Hyung-Soo Lee, Daijin Kim. Expression-invariant face recognition by facialexpression transformations. Pattern Recognition Letters, 2008, 29(13): 1797-1805[J42] Guoying Zhao, Matti Pietikäinen. Boosted multi-resolution spatiotemporal descriptors for facial expression recognition . Pattern Recognition Letters, 2009, 30(12): 1117-1127[J43] Xudong Xie, Kin-Man Lam. Facial expression recognition based on shape and texture. Pattern Recognition, 2009, 42(5):1003-1011[J44] Peng Yang, Qingshan Liu, Dimitris N. Metaxas Boosting encoded dynamic features for facial expression recognition . Pattern Recognition Letters, 2009,30(2): 132-139[J45] Sungsoo Park, Daijin Kim. Subtle facial expression recognition using motion magnification. Pattern Recognition Letters, 2009, 30(7): 708-716[J46] Chathura R. De Silva, Surendra Ranganath, Liyanage C. De Silva. Cloud basis function neural network: A modified RBF network architecture for holistic facial expression recognition. Pattern Recognition, 2008, 41(4): 1241-1253[J47] Do Hyoung Kim, Sung Uk Jung, Myung Jin Chung. Extension of cascaded simple feature based face detection to facial expression recognition. Pattern Recognition Letters, 2008, 29(11): 1621-1631[J48] Y. Zhu, L.C. De Silva, C.C. Ko. Using moment invariants and HMM in facial expression recognition. Pattern Recognition Letters, 2002, 23(1-3): 83-91[J49] Jun Wang, Lijun Yin. Static topographic modeling for facial expression recognition and analysis. Computer Vision and Image Understanding, 2007, 108(1-2): 19-34[J50] Caifeng Shan, Shaogang Gong, Peter W. McOwan. Facial expression recognition based on Local Binary Patterns: A comprehensive study. Image and Vision Computing, 2009, 27(6): 803-816[J51] Xue-wen Chen, Thomas Huang. Facial expression recognition: A clustering-based approach. Pattern Recognition Letters, 2003, 24(9-10): 1295-1302 [J52] Irene Kotsia, Ioan Buciu, Ioannis Pitas. An analysis of facial expression recognition under partial facial image occlusion. Image and Vision Computing, 2008, 26(7): 1052-1067[J53] Shuai Liu, Qiuqi Ruan. Orthogonal Tensor Neighborhood Preserving Embedding for facial expression recognition. Pattern Recognition, 2011, 44(7):1497-1513[J54] Eszter Székely, Henning Tiemeier, Lidia R. Arends, Vincent W.V. Jaddoe, Albert Hofman, Frank C. Verhulst, Catherine M. Herba. Recognition of Facial Expressions of Emotions by 3-Year-Olds. Emotion, 2011, 11(2): 425-435[J55] Kathleen M. Corcoran, Sheila R. Woody, David F. Tolin. Recognition of facial expressions in obsessive–compulsive disorder. Journal of Anxiety Disorders, 2008, 22(1): 56-66[J56] Bouchra Abboud, Franck Davoine, Mô Dang. Facial expression recognition and synthesis based on an appearance model. Signal Processing: Image Communication, 2004, 19(8): 723-740[J57] Teng Sha, Mingli Song, Jiajun Bu, Chun Chen, Dacheng Tao. Feature level analysis for 3D facial expression recognition. Neurocomputing, 2011,74(12-13) :2135-2141[J58] S. Moore, R. Bowden. Local binary patterns for multi-view facial expression recognition . Computer Vision and Image Understanding, 2011, 15(4):541-558[J59] Rui Xiao, Qijun Zhao, David Zhang, Pengfei Shi. Facial expression recognition on multiple manifolds. Pattern Recognition, 2011, 44(1):107-116[J60] Shyi-Chyi Cheng, Ming-Yao Chen, Hong-Yi Chang, Tzu-Chuan Chou. Semantic-based facial expression recognition using analytical hierarchy process. Expert Systems with Applications, 2007, 33(1): 86-95[J71] Carlos E. Thomaz, Duncan F. Gillies, Raul Q. Feitosa. Using mixture covariance matrices to improve face and facial expression recognitions. Pattern Recognition Letters, 2003, 24(13): 2159-2165[J72]Wen G,Bo C,Shan Shi-guang,et al. The CAS-PEAL large-scale Chinese face database and baseline evaluations.IEEE Transactions on Systems,Man and Cybernetics,part A:Systems and Hu-mans,2008,38(1):149-161.[J73] Yongsheng Gao,Leung ,M.K.H. Face recognition using line edge map.IEEE Transactions on Pattern Analysis and Machine Intelligence,2002,24:764-779. [J74] Hanouz M,Kittler J,Kamarainen J K,et al. Feature-based affine-invariant localization of faces.IEEE Transactions on Pat-tern Analysis and Machine Intelligence,2005,27:1490-1495.[J75] WISKOTT L,FELLOUS J M,KRUGER N,et al.Face recognition by elastic bunch graph matching.IEEE Trans on Pattern Analysis and Machine Intelligence,1997,19(7):775-779.[J76] Belhumeur P.N, Hespanha J.P, Kriegman D.J. Eigenfaces vs. fischerfaces: recognition using class specific linear projection.IEEE Trans on Pattern Analysis and Machine Intelligence,1997,15(7):711-720[J77] MA L,KHORASANI K.Facial Expression Recognition Using Constructive Feedforward Neural Networks. IEEE Transactions on Systems, Man and Cybernetics, Part B,2007,34(3):1588-1595.[J78][J79][J80][J81][J82][J83][J84][J85][J86][J87][J88][J89][J90]4、英文学位论文[D1]Hu Yuxiao. Three-dimensional face processing and its applications in biometrics:[Ph.D dissertation]. USA,Urbana-Champaign: University of Illinois, 2008。
人脸识别外文文献
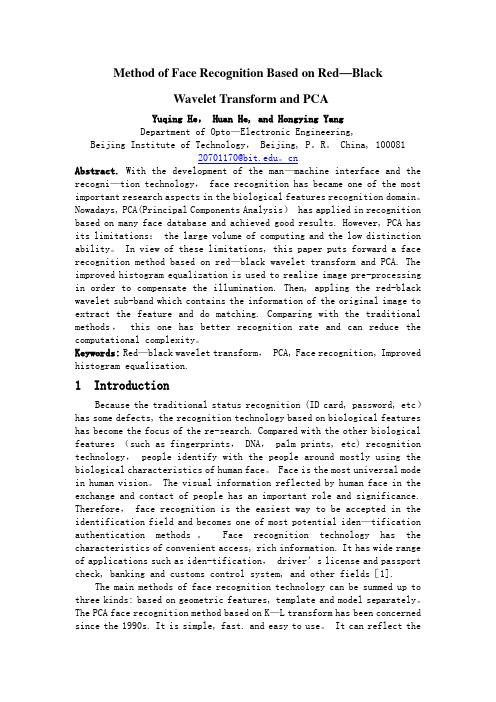
Method of Face Recognition Based on Red—BlackWavelet Transform and PCAYuqing He, Huan He, and Hongying YangDepartment of Opto—Electronic Engineering, Beijing Institute of Technology, Beijing, P。
R。
China, 10008120701170@。
cnAbstract. With the development of the man—machine interface and the recogni—tion technology, face recognition has became one of the most important research aspects in the biological features recognition domain。
Nowadays, PCA(Principal Components Analysis) has applied in recognition based on many face database and achieved good results. However, PCA has its limitations: the large volume of computing and the low distinction ability。
In view of these limitations, this paper puts forward a face recognition method based on red—black wavelet transform and PCA. The improved histogram equalization is used to realize image pre-processing in order to compensate the illumination. Then, appling the red-black wavelet sub-band which contains the information of the original image to extract the feature and do matching. Comparing with the traditional methods,this one has better recognition rate and can reduce the computational complexity。
cv人物表

CV人物4:Matthew Turk毕业于MIT,最有影响力的研究成果:人脸识别。其和Alex Pentland在1991年发表了"Eigenfaces for Face Recognition".该论文首次将PCA(Principal Component Analysis)引入到人脸识别中,是人脸识别最早期最经典的方法,且被人实现,开源在OpenCV了。主页:/~mturk/
CV人物16: William T.Freeman毕业于MIT;研究领域:应用于CV的ML、可视化感知的贝叶斯模型、计算摄影学;最有影响力的研究成果:图像纹理合成;Alex Efros和Freeman在2001年SIGGRAPH上发表了"Image quilting for texture synthesis and transfer",其思想是从已知图像中获得小块,然后将这些小块拼接mosaic一起,形成新的图像。该算法是图像纹理合成中经典中的经典。主页:/billf/
CV人物17: Feifei Li李菲菲,毕业于Caltech;导师:Pietro Perona;研究领域:Object Bank、Scene Classification、ImageNet等;最有影响力的研究成果:图像识别;她建立了图像识别领域的标准测试库Caltech101/256。是词包方法的推动者。主页:/~feifeili/
CV人物8:Michal Irani毕业于Hebrew大学,最有影响力的研究成果:超分辨率。她和Peleg于1991年在Graphical Models and Image Processing发表了"Improving resolution by image registration",提出了用迭代的、反向投影的方法来解决图像放大的问题,是图像超分辨率最经典的算法。我在公司实现的产品化清晰化增强算法就参考了该算法思想哈哈。主页:http://www.wisdom.weizmann.ac.il/~irani/
基于eigenfaces的人脸识别算法实现大学论文

河北农业大学本科毕业论文(设计)题目:基于Eigenfaces的人脸识别算法实现摘要随着科技的快速发展,视频监控技术在我们生活中有着越来越丰富的应用。
在这些视频监控领域迫切需要一种远距离,非配合状态下的快速身份识别,以求能够快速识别所需要的人员信息,提前智能预警。
人脸识别无疑是最佳的选择。
可以通过人脸检测从视频监控中快速提取人脸,并与人脸数据库对比从而快速识别身份。
这项技术可以广泛应用于国防,社会安全,银行电子商务,行政办公,还有家庭安全防务等多领域。
本文按照完整人脸识别流程来分析基于PCA(Principal Component Analysis)的人脸识别算法实现的性能。
首先使用常用的人脸图像的获取方法获取人脸图像。
本文为了更好的分析基于PCA人脸识别系统的性能选用了ORL人脸数据库。
然后对人脸数据库的图像进行了简单的预处理。
由于ORL人脸图像质量较好,所以本文中只使用灰度处理。
接着使用PCA提取人脸特征,使用奇异值分解定理计算协方差矩阵的特征值和特征向量以及使用最近邻法分类器欧几里得距离来进行人脸判别分类。
关键词:人脸识别PCA算法奇异值分解定理欧几里得距离ABSTRACTWith the rapid development of technology, video surveillance technology has become increasingly diverse applications in our lives. In these video surveillance urgent need for a long-range, with rapid identification of non-state, in order to be able to quickly identify people the information they need, advance intelligence warning. Face recognition is undoubtedly the best choice. Face detection can quickly extract human faces from video surveillance, and contrast with the face database to quickly identify identity. This technology can be widely used in national defense, social security, bank e-commerce, administrative offices, as well as home security and defense and other areas.In accordance with the full recognition process to analyze the performance of PCA-based face recognition algorithm. The first to use the method of access to commonly used face images for face images. In order to better analysis is based on the performance of the PCA face recognition system selected ORL face database. Then the image face database for a simple pretreatment. Because ORL face image quality is better, so this article uses only gray scale processing. Then use the PCA for face feature extraction using singular value decomposition theorem to calculate the covariance matrix of the eigenvalues and eigenvectors, and use the Euclidean distance of the nearest neighbor classifier to the classification of human face discrimination.KEYWORDS: face recognition PCA algorithm SVD Euclidean distance目录摘要 (1)ABSTRACT (2)1 人脸识别概述 (4)1.1 人脸识别的研究概况和发展趋势 (4)1.1.1 人脸识别的研究概况 (4)1.1.2 人脸识别的发展趋势 (5)1.2 人脸识别的主要难点 (6)1.3 人脸识别的流程 (6)1.3.1 人脸图像采集 (7)1.3.2 预处理 (7)1.3.3 特征提取 (7)1.4 本章小结 (8)2 人脸图像 (9)2.1 人脸图像获取 (9)2.2 人脸图像数据库 (9)2.3 人脸图像预处理 (10)2.3.1 灰度变化 (10)2.3.2 二值化 (11)2.3.3 图像锐化 (11)2.4 本章小结 (12)3 人脸识别 (13)3.1 PCA算法理论 (13)3.2 PCA人脸识别算法的实现 (14)3.2.1 K-L变换 (14)3.2.2 SVD 定理 (14)3.2.3 PCA算法 (15)3.2.4 人脸识别过程 (16)3.3 程序运行效果 (16)3.4 程序代码 (17)3.4.1 代码类关系 (17)3.4.2 代码的OpenCV相关 (18)3.4.3 关键函数代码 (18)3.5 本章小结 (22)结论 (23)致谢 (24)参考文献 (25)1人脸识别概述1.1 人脸识别的研究概况和发展趋势1.1.1 人脸识别的研究概况人脸识别的研究开始于上世纪七十年代,当时的研究主要是基于人脸外部轮廓的方法。
基于pca算法的eigenfaces人脸识别算法大学论文
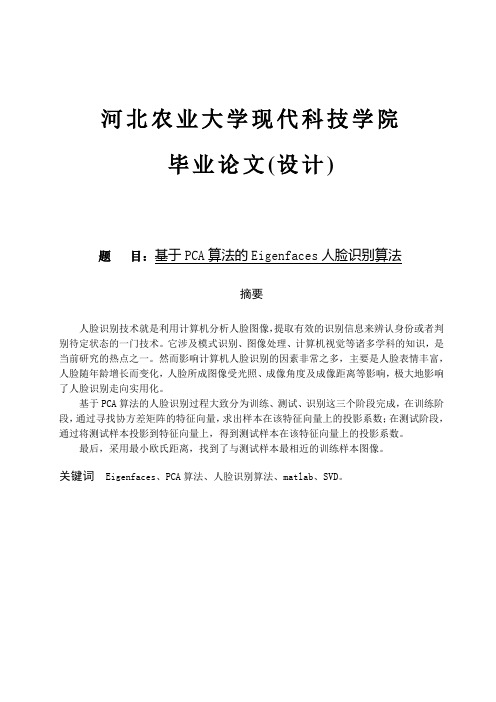
河北农业大学现代科技学院毕业论文(设计)题目:基于PCA算法的Eigenfaces人脸识别算法摘要人脸识别技术就是利用计算机分析人脸图像,提取有效的识别信息来辨认身份或者判别待定状态的一门技术。
它涉及模式识别、图像处理、计算机视觉等诸多学科的知识,是当前研究的热点之一。
然而影响计算机人脸识别的因素非常之多,主要是人脸表情丰富,人脸随年龄增长而变化,人脸所成图像受光照、成像角度及成像距离等影响,极大地影响了人脸识别走向实用化。
基于PCA算法的人脸识别过程大致分为训练、测试、识别这三个阶段完成,在训练阶段,通过寻找协方差矩阵的特征向量,求出样本在该特征向量上的投影系数;在测试阶段,通过将测试样本投影到特征向量上,得到测试样本在该特征向量上的投影系数。
最后,采用最小欧氏距离,找到了与测试样本最相近的训练样本图像。
关键词Eigenfaces、PCA算法、人脸识别算法、matlab、SVD。
AbstractFace recognition technology is the use of computer analysis of facial images to extract valid identification information to identify or determine the identity of a technology Pending state. It involves knowledge of pattern recognition, image processing, computer vision, and many other disciplines, is one of the hotspots of current research. However, factors affecting the computer face recognition very much, mainly rich facial expression, face changes with age, face a picture of the affected light, imaging and imaging distance, angle, greatly influenced the Face to practical use.PCA algorithm based recognition process is roughly divided into training and testing, the identification of these three stages, in the training phase, to find the eigenvectors of the covariance matrix is obtained on the sample feature vector projection coefficient; in the test phase by the test feature vector is projected onto the sample to obtain a test sample on the projection of the feature vector of coefficients.Finally, the minimum Euclidean distance, the test sample to find the closest sample images.Keywords Eigenfaces PCA Algorithm、Face Recognition Algorithm、matlab、SVD.目录1 绪论---------------------------------------------------------------------- 11.1计算机人脸识别技术及应用--------------------------------------------- 11.2常用的人脸识别方法简介----------------------------------------------- 11.3本论文内容安排------------------------------------------------------- 12 PCA ----------------------------------------------------------------------- 32.1 PCA简介------------------------------------------------------------- 32.2 PCA的实质----------------------------------------------------------- 32.3 PCA理论基础--------------------------------------------------------- 32.3.1投影----------------------------------------------------------- 32.3.2最小平方误差理论----------------------------------------------- 42.3.3 PCA几何解释--------------------------------------------------- 82.4 PCA降维计算--------------------------------------------------------- 83 PCA在人脸识别中的应用--------------------------------------------------- 113.1 人脸识别技术简介--------------------------------------------------- 113.2 图片归一化--------------------------------------------------------- 113.3 基于PCA的人脸识别------------------------------------------------- 113.3.1 人脸数据特征提取---------------------------------------------- 113.3.2计算均值------------------------------------------------------ 123.3.3计算协方差矩阵C ----------------------------------------------- 123.3.4求出协方差C的特征值和特征向量-------------------------------- 123.4奇异值分解定理------------------------------------------------------ 123.5 基于PCA的人脸识别的训练------------------------------------------- 133.5.1 训练集的主成分计算-------------------------------------------- 133.5.2 训练集图片重建------------------------------------------------ 133.6 识别--------------------------------------------------------------- 144 实验--------------------------------------------------------------------- 154.1 实验环境----------------------------------------------------------- 154.2 PCA人脸识别实验过程------------------------------------------------ 154.2.1 训练阶段------------------------------------------------------ 154.2.2 测试阶段------------------------------------------------------ 224.2.3 采用欧氏最小距离识别------------------------------------------ 234.3实验结果------------------------------------------------------------ 245 总结--------------------------------------------------------------------- 265.1.1内容总结:---------------------------------------------------- 265.1.2工作总结:---------------------------------------------------- 26 6致谢--------------------------------------------------------------------- 27 参考文献------------------------------------------------------------------- 281 绪论1.1计算机人脸识别技术及应用计算机人脸识别技术就是利用计算机分析人脸图像,进而从中提取出有效的识别信息,用来“辨认”身份的一门技术,它涉及图像处理、模式识别、计算机视觉、神经网络、生理学、心理学等诸多学科领域的知识。
人脸识别算法详解(最全)
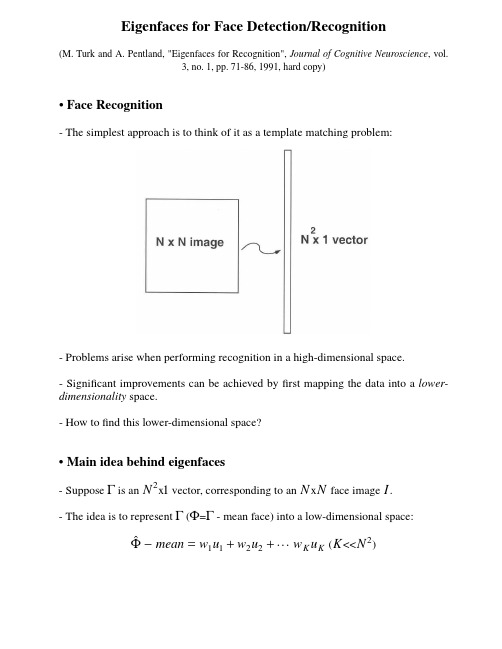
ˆ − mean = w1 u1 + w2 u2 + . . . w K u K ( K << N 2 ) Φ
-2-
Computation of the eigenfaces
Step 1: obtain face images I1 , I2 , ..., I M (training faces) (very important: the face images must be centered and of the same size)
英文翻译

2D-LDA:A statistical linear discriminant analysisfor image matrixMing Li *,Baozong YuanInstitute of Information Science,Beijing Jiaotong University,Beijing 100044,ChinaReceived 19August 2004Available online 18October 2004AbstractThis paper proposes an innovative algorithm named 2D-LDA,which directly extracts the proper features from image matrices based on Fisher Õs Linear Discriminant Analysis.We experimentally compare 2D-LDA to other feature extraction methods,such as 2D-PCA,Eigenfaces and Fisherfaces.And 2D-LDA achieves the best performance.Ó2004Elsevier B.V.All rights reserved.Keywords:Feature extraction;Image representation;Linear discriminant analysis;Subspace techniques;Face recognition1.IntroductionFeature extraction is the key to face recogni-tion,as it is to any pattern classification task.The aim of feature extraction is reducing the dimensionality of face image so that the extracted features are as representative as possible.The class of image analysis methods called appearance-based approach has been of wide concern,which relies on statistical analysis and machine learning.Turk and Pentland (1991)presented the well-known Eigenfaces method for face recognition,which uses principal component analysis (PCA)for dimensionality reduction.However,the base physical similarity of the represented images to originals does not provide the best measure of use-ful information for distinguishing faces from one another (O ÕToole,1993).Belhumeur et al.(1997)proposed Fisherfaces method,which is based on Fisher Õs Linear Discriminant and produces well separated classes in a low-dimensional subspace.His method is insensitive to large variation in lighting direction and facial expression.Recently,Yang (2002)investigated the Kernel PCA for learning low dimensional representations for face recognition and found that the Kernel methods provide better representations and achieve lower error rates for face recognition.Bartlett et al.(2002)proposed using ICA for face representation,which is sensitive to the high-order0167-8655/$-see front matter Ó2004Elsevier B.V.All rights reserved.doi:10.1016/j.patrec.2004.09.007*Corresponding author.Tel.:+861051683149;fax:+516861688616.E-mail address:liming@ (M.Li).Pattern Recognition Letters 26(2005)527–532statistics.This method is superior to representa-tions based on PCA for recognizing faces across days and changes in expression.However,Kernel PCA and ICA are both computationally more expensive than PCA.Weng et al.(2003)presented a fast method,called candid covariance-free IPCA (CCIPCA),to obtain the principal components of high-dimensional image vectors.Moghaddan (2002)compared the Bayesian subspace method with several PCA-related methods(PCA,ICA, and Kernel PCA).The experimental results dem-onstrated its superiority over PCA,ICA and Ker-nel PCA.All the PCA-related methods discussed above are based on the analysis of vectors.When dealing with images,we shouldfirstly transform the image matrixes into image vectors.Then based on these vectors the covariance matrix is calculated and the optimal projection is obtained.However,face images are high-dimensional patterns.For exam-ple,an image of112·92will form a10304-dimen-sional vector.It is difficult to evaluate the covariance matrix in such a high-dimensional vector space.To overcome the drawback,Yang proposed a straightforward image projection tech-nique,named as image principal component analy-sis(IMPCA)(Yang et al.,2004),which is directly based on analysis of original image matrices.Dif-ferent to traditional PCA,2D-PCA is based on 2D matrices rather than1D vectors.This means that the image matrix does not need to be con-verted into a vector.As a result,2D-PCA has two advantages:easier to evaluate the covariance matrix accurately and lower time-consuming.Liu et al.(1993)proposed an iterative method to calcu-late the Foley-Sammon optimal discriminant vec-tors from image matrixes.And he proposed to substitute D t=D b+D w for D w to overcome the sin-gularity problem.LiuÕs method was complicate and didnÕt resolve the singularity problem well.In this paper,a statistical linear discriminant analysis for image matrix is discussed.Our method proposes to use Fisher linear projection criterion tofind out a good projection.This crite-rion is based on two parameters:the between-class scatter matrix and the within-class scatter matrix. Because the dimension of between-class and within-class scatter matrix is much low(compara-tive to number of training samples).So,the prob-lem,that the within-class scatter matrix maybe singular,will be handled.At the same time,the compute-costing is lower than traditional Fisher-faces.Moreover,we discuss about image recon-struction and conduct a series of experiments on the ORL face database.The organization of this paper is as follows:In Section2,we propose the idea and describe the algorithm in detail.In Section3,we compare 2D-LDA with Eigenfaces,Fisherfaces and2D-PCA on the ORL face database.Finally,the paper concludes with some discussions in Section4.2.Two-dimensional linear discriminant analysis 2.1.Principle:The construction of Fisher projection axisLet A denotes a m·n image,and x denotes an n-dimensional column vector.A is projected onto x by the following linear transformationy¼Ax:ð1ÞThus,we get an m-dimensional projected vector y,which is called the feature vector of the image A.Suppose there are L known pattern classes in the training set,and M denotes the size of the training set.The j th training image is denoted by an m·n matrix A j(j=1,2,...,M),and the mean image of all training sample is denoted by A and A iði¼1;2;...;LÞdenoted the mean image of class T i and N i is the number of samples in class T i,the projected class is P i.After the projection of training image onto x,we get the projected fea-ture vectoryj¼A j x;j¼1;2;...;M:ð2ÞHow do we judge a projection vector x is good? In fact,the total scatter of the projected samples can be characterized by the trace of the covariance matrix of the projected feature vectors(Turk and Pentland,1991).From this point of view,we intro-duced a criterion atfirst,JðxÞ¼P BP W:ð3Þ528M.Li,B.Yuan/Pattern Recognition Letters26(2005)527–532There were two parametersP B¼trðTS BÞ;ð4ÞP W¼trðTS WÞ;ð5Þwhere TS B denotes the between-class scatter matrix of projected feature vectors of training images,and TS W denotes the within-class scatter matrix of projected feature vectors of training images.So,TS B¼X Li¼1N ið y iÀ yÞð y iÀ yÞT¼X Li¼1N i½ðA iÀAÞx ½ðA iÀAÞx T;ð6ÞTS W¼X Li¼1Xy k2P iðy kÀ y iÞðy kÀy iÞT¼X Li¼1Xy k2P i½ðA kÀA iÞx ½ðA kÀA iÞx T:ð7ÞSotrðTS BÞ¼x TX Li¼1N iðA iÀAÞTðA iÀAÞ!x¼x T S B x;ð8ÞtrðTS WÞ¼x TX Li¼1XA k2T iðA kÀA iÞTðA kÀA iÞ!x¼x T S W x:ð9ÞWe could evaluate TS B and TS W directly using the training image samples.So,the criterion could be expressed byJðxÞ¼x T S B xx T S W x;ð10Þwhere x is a unitary column vector.This criterion is called Fisher linear projection criterion.The unitary vector x that maximizes J(x)is called the Fisher optimal projection axis.The optimal projection x opt is chosen when the criterion is maximized,i.e.,x opt¼arg maxxJðxÞ:ð11ÞIf S W is nonsingular,the solution to above opti-mization problem is to solve the generalized eigen-value problem(Turk and Pentland,1991):S B x opt¼k S W x opt:ð12ÞIn the above equation,k is the maximal eigenvalue of SÀ1WS B.The traditional LDA must face to the singularity problem.However,2D-LDA overcomes this prob-lem successfully.This is because:For each training image,A j(j=1,2,...,M),we have rank(A j)= min(m,n).From(9),we haverankðS WÞ¼rankX Li¼1XA k2T iðA kÀA iÞTðA kÀA iÞ!6ðMÀLÞÁminðm;nÞ:ð13ÞSo,in2D-LDA,S W is nonsingular whenM P Lþnminðm;nÞ:ð14ÞIn real situation,(14)is always satisfied.So,S W is always nonsingular.In general,it is not enough to have only one Fisher optimal projection axis.We usually need to select a set of projection axis,x1,...,x d,subject to the orthonormal constraints.That is,f x1;...;x d g¼arg max JðxÞx Tix j¼0;i¼j;i;j¼1;...;d:ð15ÞIn fact,the optimal projection axes,x1,...,x d,are the orthonormal eigenvectors of SÀ1WS B corre-sponding to thefirst d largest eigenvalues.Using these projection axes,we could form a new Fisher projection matrix X,which is a n·d matrix,X¼½x1x2ÁÁÁx d :ð16Þ2.2.Feature extractionWe will use the optimal projection vectors of 2D-LDA,x1,...,x d,for feature extraction.For a given image A,we haveyk¼Ax k;k¼1;2;...;d:ð17ÞThen,we have a family of Fisher feature vectors y1,...,y d,which formed a m·d matrix Y=[y1,...,y d].We called this matrix Y as the Fisher feature matrix of the image A.M.Li,B.Yuan/Pattern Recognition Letters26(2005)527–5325292.3.ReconstructionIn the2D-LDA method,we can use the Fisher feature matrixes and Fisher optimal projection axes to reconstruct a image by following steps.For a given image A,the Fisher feature matrix is Y=[y1,...,y d]and the Fisher optimal projection axes X=[x1,...,x d],then we haveY¼AX:ð18ÞBecause x1,...,x d are orthonormal,it is easy to obtain the reconstructed image of A:e A¼YX T¼X dk¼1ykx Tk:ð19ÞWe called e A k¼y k x T k as a reconstructed subimage of A,which have the same size as image A.This means that we use a set of2D Fisherfaces to reconstruct the original image.If we select d=n,then we can completely reconstruct the images in the training set:e A¼A.If d<n,the reconstructed image e A is an approximation for A.2.4.ClassificationGiven two images A1,A2represented by2D-LDA feature matrix Y1¼½y11;...;y1dandY2¼½y21;...;y2d.So the similarity d(Y1,Y2)is de-fined asdðY1;Y2Þ¼X dk¼1k y1kÀy2kk2;ð20Þwhere k y1k Ày2kk2denotes the Euclidean distancebetween the two Fisher feature vectors y1k and y2k.If the Fisher feature matrix of training images are Y1,Y2,...,Y M(M is the total number of train-ing images),and each image is assigned to a class T i.Then,for a given test image Y,if d(Y,Y l)=min j d(Y1,Y j)and Y l2T i,then the resulting deci-sion is Y2T i.3.Experiment and analysisWe evaluated our2D-LDA algorithm on the ORL face image database.The ORL database ()contains images of40 individuals,each person have10different images. For some individuals,the images were taken at different times.The facial expression(open or closed eyes,smiling or nonsmiling)and facial de-tails(glasses or no glasses)also vary.All the images were taken against a dark homogeneous background with the subjects in an upright,frontal position(with tolerance for some side movement). The images were taken with a tolerance for some titling and rotation of the face of up to20°.More-over,there is also some variation in the scale of up to about10%.The size of each image is92·112 pixels,with256grey levels per pixel.Five samples of one person in ORL database are shown in Fig.1.So,we could use the ORL:database to evaluate2D-LDAÕs performance under conditions where pose and the size of sample are varied.Using2D-LDA,we could project the test face image onto the Fisher optimal projection axis, then we could use the Fisher feature vectors set to reconstruct the image.In Fig.2,some recon-structed images and the original image of one per-son were given out.In Fig.2,the variance d denotes the number of dimension used to map and reconstruct the face image.As observed these images,we couldfind that the reconstructed images are very like obtained by sample the origi-nal image on the spacing vertical scanning line. The reconstructed image e A is more and more like to the original image A as the value of d increased.We have done an experiment on the ORL data-base to evaluate performance of2D-LDA,2D-Fig.1.Five images in ORL database.530M.Li,B.Yuan/Pattern Recognition Letters26(2005)527–532PCA (Yang et al.,2004),Eigenfaces (Turk and Pentland,1991),Fisherfaces (Belhumeur et al.,1997).To evaluate the pure ability of these four in the fair environment,we did not do any prepro-cess on the face images,and we did not utilize any optimized algorithm.We just realized the algo-rithm appeared in the literature (Turk and Pent-land,1991;Belhumeur et al.,1997and Yang et al.,2004)without any modification.In our experiment,we select first five images samples per person for training,and the left five images samples for testing.So,in our experiment,the size of training set and testing set were both 200.So in the 2D-LDA,the size of between-class scatter ma-trix S B and within-class scatter matrix S W are both 92·92.Fig.3shows the classification result.From Fig.3,we find that the recognition rate of 2D-LDA have achieved the best performance in the four methods.And the best result of 2D-LDA 94.0%is much better than the best result of 2D-PCA 92.5%.And from Fig.3,we could find that the two 2D feature extraction methods have outstanding performance in the low-dimensioncondition,but the conventional ones Õability is very poor.Table 1showed out the comparison of the train-ing time of the four algorithms (CPU:Pentium IV 2.66GHz,RAM:256M).The four algorithms are realized in the Matlab environment.We could see that the 2D-LDA and 2D-PCA Õs computing-cost is very low compared with Eigenfaces and Fisher-faces.This is because in the 2D condition,we only need to handle a 92·92matrix.But using the Eigenfaces and Fisherfaces,we must face to a 10304·10304matrix.It is a hard work.At last,It must be mentioned that when used the Fisher-faces,we must reduced the dimension of image data to avoid that S W is singular (Belhumeur et al.,1997).Mapping the original data onto how many dimensions space is a hard problem.We must select the proper number of dimension through experiment.Considering this situation,Fisherfaces is very time-costing.Table 2showed that the memory cost of 2D fea-ture extraction is much larger than the 1D ones.This is because 2D methods used a n ·d matrix to present a face image.At the same time the 1D techniques reconstructed face images by a d -dimensionvector.Fig. parison of 2D-LDA and 2D-PCA on ORLDatabase.Fig.2.Some reconstructed images of one person.Table 2Comparison of memory cost (bytes)to present a 92·112image using different techniques (15dimensions)2D-LDA 2D-PCA Eigenfaces Fisherfaces 672067206060Table 1Comparison of CPU Time (s)for feature extraction using ORL database (15dimensions)2D-LDA 2D-PCA Eigenfaces Fisherfaces 0.42100.421028.500032.5310M.Li,B.Yuan /Pattern Recognition Letters 26(2005)527–5325314.ConclusionIn this paper,a new algorithm for image feature extraction and selection was proposed.This meth-od uses the Fisher Linear Discriminant Analysis to enhance the effect of variation caused by different individuals,other than by illumination,expres-sion,orientation,etc.2D-LDA uses the image ma-trix instead of the image vector to compute the between-class scatter matrix and the within-class scatter matrix.From our experiments,we can see that the2D-LDA have many advantages over other methods. 2D-LDA achieves the best recognition accuracy in the four algorithms.And this techniqueÕs com-puting cost is very low compared with Eigenfaces and Fisherfaces,and close to2D-PCA.At the same time,this method shows powerful perfor-mance in the low dimension.From Fig.2,we can see that this new projection method is very like to select spacing vertical scanning lines to present a image.Maybe this is the reason that this algorithm is so effective in image classification.2D-LDA still has its shortcoming.It needs more memory to store a image than the Eigenfaces and Fisherfaces.AcknowledgementThis work was supported by the National Nat-ural Science Foundation of China(No.60441002)and the University Key Research Project(No. 2003SZ002).ReferencesBartlett,M.S.,Movellan,J.R.,Sejnowski,T.J.,2002.Face recognition by independent component analysis.IEEE Trans.Neural Networks13(6),1450–1464. Belhumeur,P.N.,Hespanha,J.P.,Kriegman, D.J.,1997.Eigenfaces vs.Fisherfaces:Recognition using class specific linear projection.IEEE Trans.Pattern Anal.Machine Intell.19(7),711–720.Lui,K.,Cheng,Y.,Yang,J.,1993.Algebraic feature extraction for image recognition based on an optimal discriminant criterion.Pattern Recognition26(6),903–911.Moghaddan,B.,2002.Principal manifolds and probabilistic subspaces for visual recognition.IEEE Trans.Pattern Anal.Machine Intell.24(6),780–788.OÕToole,A.,1993.Low-dimensional representation of faces in higher dimensions of the face space.J.Opt.Soc.Amer.10(3).Turk,M.,Pentland,A.,1991.Eigenfaces for recognition.J.Cognitive Neurosci.3(1),71–86.Weng,J.,Zhang,Y.,Hwang,W.,2003.Candid covariance-free incremental principal component analysis.IEEE Trans.Pattern Anal.Machine Intell.25(8),1034–1040.Yang,M.H.,2002.Kernel eigenfaces vs.Kernel Fisherfaces: Face recognition using Kernel methods.In:Proc.5th Internat.Conf.on Automatic Face and Gesture Recogni-tion(RGRÕ02).pp.215–220.Yang,J.,Zhang,D.,Frangi,A.F.,Yang,J.-y.,2004.Two-dimensional PCA:A new approach to appearance-based face representation and recognition.IEEE Trans.Pattern Anal.Machine Intell.26(1),131–137.532M.Li,B.Yuan/Pattern Recognition Letters26(2005)527–532。
基于PCA和神经网络的人脸识别算法研究

基于PCA和神经网络的人脸识别算法研究作者:唐赫来源:《软件导刊》2013年第06期摘要:在MATLAB环境下,取ORL人脸数据库的部分人脸样本集,基于PCA方法提取人脸特征,形成特征脸空间,然后将每个人脸样本投影到该空间得到一投影系数向量,该投影系数向量在一个低维空间表述了一个人脸样本,这样就得到了训练样本集。
同时将另一部分ORL人脸数据库的人脸作同样处理得到测试样本集。
然后基于最近邻算法进行分类,得到识别率,接下来使用BP神经网络算法进行人脸识别,最后通过基于神经网络算法和最近邻算法进行综合决策,对待识别的人脸进行分类。
关键词:人脸识别;主成分;BP神经网络;最近邻算法中图分类号:TP311文献标识码:A文章编号:1672-7800(2013)006-0033-02作者简介:唐赫(1989-),女,武汉理工大学理学院统计系硕士研究生,研究方向为人脸图像识别、遥感图像、统计预测决策。
0引言特征脸方法就是将人脸的图像域看作是一组随机向量,可以从训练图像中,通过主元分析得到一组特征脸图像,任意给定的人脸图像都可以近似为这组特征脸图像的线性组合,用组合的系数作为人脸的特征向量。
识别过程就是将人脸图像映射到由特征脸组成的子空间上,比较其与已知人脸在特征脸空间中的位置。
经典的特征脸方法是采用基于欧氏距离的最近中心分类器,比较常用的是基于欧氏距离的最近邻。
1算法流程(1)读入人脸库。
每个人取前5张作为训练样本,后5张为测试样本,共40人,则训练样本和测试样本数分别为N=200。
人脸图像为92×112维,按列相连就构成N=10 304维矢量x-j,可视为N维空间中的一个点。
(2)构造平均脸和偏差矩阵。
(3)计算通(4)计算训练样本在特征脸子空间上的投影系数向量,生成训练集的人脸图像主分量allcoor-200×71。
(5)计算测试样本在特征脸子空间上的投影系数向量,生成测试集的人脸图像主分量tcoor-200×71。
频域下稀疏表示的大数据库人脸分类算法
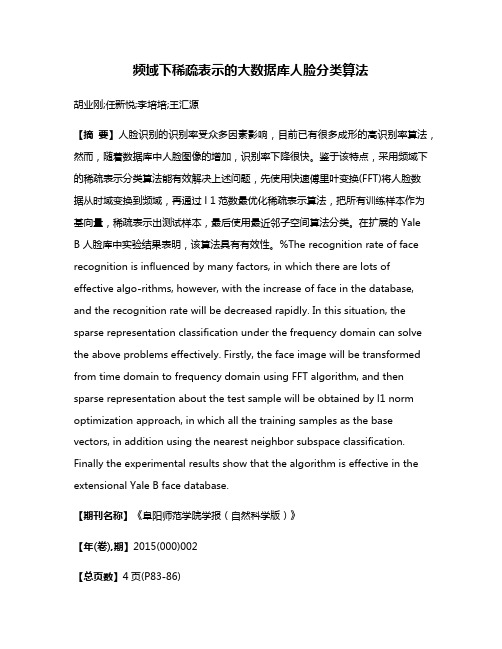
频域下稀疏表示的大数据库人脸分类算法胡业刚;任新悦;李培培;王汇源【摘要】人脸识别的识别率受众多因素影响,目前已有很多成形的高识别率算法,然而,随着数据库中人脸图像的增加,识别率下降很快。
鉴于该特点,采用频域下的稀疏表示分类算法能有效解决上述问题,先使用快速傅里叶变换(FFT)将人脸数据从时域变换到频域,再通过 l 1范数最优化稀疏表示算法,把所有训练样本作为基向量,稀疏表示出测试样本,最后使用最近邻子空间算法分类。
在扩展的 YaleB 人脸库中实验结果表明,该算法具有有效性。
%The recognition rate of face recognition is influenced by many factors, in which there are lots of effective algo-rithms, however, with the increase of face in the database, and the recognition rate will be decreased rapidly. In this situation, the sparse representation classification under the frequency domain can solve the above problems effectively. Firstly, the face image will be transformed from time domain to frequency domain using FFT algorithm, and then sparse representation about the test sample will be obtained by l1 norm optimization approach, in which all the training samples as the base vectors, in addition using the nearest neighbor subspace classification. Finally the experimental results show that the algorithm is effective in the extensional Yale B face database.【期刊名称】《阜阳师范学院学报(自然科学版)》【年(卷),期】2015(000)002【总页数】4页(P83-86)【关键词】稀疏表示;快速傅里叶变换;人脸识别【作者】胡业刚;任新悦;李培培;王汇源【作者单位】阜阳师范学院数学与统计学院,安徽阜阳 236037;阜阳师范学院数学与统计学院,安徽阜阳 236037;阜阳师范学院数学与统计学院,安徽阜阳236037;阜阳师范学院数学与统计学院,安徽阜阳 236037【正文语种】中文【中图分类】TP391.41 引言近年来,人脸识别已成为经典的模式识别研究问题之一。
CAS-PEAL大规模中国人脸图象数据库及其基本评测介绍
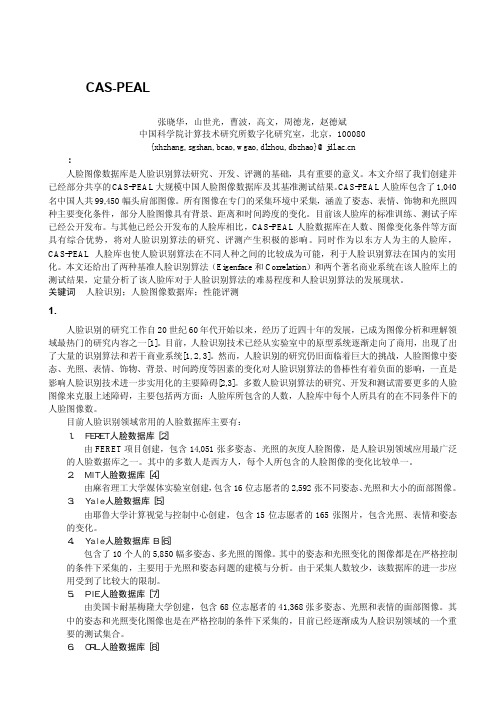
CAS-PEAL大规模中国人脸图像数据库及其基本评测介绍张晓华,山世光,曹波,高文,周德龙,赵德斌中国科学院计算技术研究所数字化研究室,北京,100080{xhzhang, sgshan, bcao, wgao, dlzhou, dbzhao}@摘要:人脸图像数据库是人脸识别算法研究、开发、评测的基础,具有重要的意义。
本文介绍了我们创建并已经部分共享的CAS-PEAL大规模中国人脸图像数据库及其基准测试结果。
CAS-PEAL人脸库包含了1,040名中国人共99,450幅头肩部图像。
所有图像在专门的采集环境中采集,涵盖了姿态、表情、饰物和光照四种主要变化条件,部分人脸图像具有背景、距离和时间跨度的变化。
目前该人脸库的标准训练、测试子库已经公开发布。
与其他已经公开发布的人脸库相比,CAS-PEAL人脸数据库在人数、图像变化条件等方面具有综合优势,将对人脸识别算法的研究、评测产生积极的影响。
同时作为以东方人为主的人脸库,CAS-PEAL人脸库也使人脸识别算法在不同人种之间的比较成为可能,利于人脸识别算法在国内的实用化。
本文还给出了两种基准人脸识别算法(Eigenface和Correlation)和两个著名商业系统在该人脸库上的测试结果,定量分析了该人脸库对于人脸识别算法的难易程度和人脸识别算法的发展现状。
关键词:人脸识别;人脸图像数据库;性能评测1. 引言人脸识别的研究工作自20世纪60年代开始以来,经历了近四十年的发展,已成为图像分析和理解领域最热门的研究内容之一[1]。
目前,人脸识别技术已经从实验室中的原型系统逐渐走向了商用,出现了出了大量的识别算法和若干商业系统[1, 2, 3]。
然而,人脸识别的研究仍旧面临着巨大的挑战,人脸图像中姿态、光照、表情、饰物、背景、时间跨度等因素的变化对人脸识别算法的鲁棒性有着负面的影响,一直是影响人脸识别技术进一步实用化的主要障碍[2,3]。
多数人脸识别算法的研究、开发和测试需要更多的人脸图像来克服上述障碍,主要包括两方面:人脸库所包含的人数,人脸库中每个人所具有的在不同条件下的人脸图像数。
翻译0

二维线性统计判别图像矩阵的分析摘要本文提出的这种创新性的算法是2D –LDA,它是依据Fisher的线性判别分析而建立起来的一种从图像矩阵中直接提取合适的特性。
我们实验上对它和其他的特性提取方法(如2D-PCA, Eigenfaces 和 Fisherfaces方法)进行了比较,结果2D – LDA的性能最佳。
1、引言因为特征提取是任何模式分类的特征提取的主要任务,因此特征提取是人脸识别的关键。
特征提取的目的是减少人脸图像的维数,使提取的特征是尽可能具有代表性。
所谓外观基础的图像分析方法现在已经引起广泛的注意,它是依赖统计分析和机器的学习。
Turk和Pentland(1991年)针对人脸识别发布了著名的Eigenfaces方法,它是使用主要成分分析的方法(PCA)来降维。
然而这种使用基本物理相似代表图像并没有提供一个利用有用信息去区分人脸的最好的方法。
Belhumeur等人(1997年)提出了Fisherfaces的方法,它是基于Fisher的线性辨别和低维子空间所产生的分隔类。
这种方法的最大好处是对灯光和面部表情的变化并不敏感。
最近,Yang(2002年)研究了Kernel的 PCA方法中关于人脸识别的低维代表,发现Kernel的方法提取的特征更具代表性和错误率很低。
Bartlett等人(2002年)提出了使用ICA去代表人脸,这种方法是对高阶统计区很敏感,因此这种方法在要比基于PCA的代表特征更有优势。
然而Kernel PCA和ICA的计算量都要比PCA大的多。
Weng 等人(2003年)提出了一种快速方法(CCIPCA),以获得高维图像矢量的主要组成部分。
Moghaddan(2002年)对贝氏子空间法和几种PCA 相关方法(PCA, ICA, 和Kernel PCA),实验结果表明这种方法比PCA, ICA, 和Kernel PCA 都要优越。
上述所有PCA 相关的方法都是基于向量分析。
故在图像处理时,首先要把图像矩阵转化成图像向量。
非负矩阵分解算法

应用于寻找局部最小值。
4
梯度下降法4可能是实现起来最简单的技术,但其收敛速度可能 很慢。其他方法如共轭梯度具有更快的收敛(至少在局部最小值附 近),但是比梯度下降更复杂[8]。并且,基于梯度的方法的收敛具有 对步长选择非常敏感的缺点,这对于大型应用非常不方便。
四.乘法矫正规则
我们发现,以下“乘法矫正规则”是解决问题 1 和 2 的速度和
1
3(������3 −
T ������3TℎT)1
(15)
证明:因为显然������ ℎ, ℎ ≥ ������ ℎ ,我们只需要证明������ ℎ, ℎd ≥ ������ ℎ ,
为了证明需要,我们对比
������ ℎ = ������ ℎe + ℎ − ℎe X∇������ ℎe + g ℎ − ℎe X ������X������ ℎ − ℎe
������TU
=
Z[\ (]^]Z)[\
(7)
那么我们获得在定理 1 中给出的 H 的矫正规则。注意,该重新
调整会得出乘子因子(分母中的梯度的正分量和因子的分子中的负
分量的绝对值)。
对于散度,对角线重新调整梯度下降采取以下显示:
������TU ← ������TU + ������TU[ 3 ������3T������3U/(������������)3U − 3 ������3T] (8)
非负矩阵分解算法1
摘 要:非负矩阵分解(NMF)是一种处理多变量数据分解极为有效的方
法。这里分析了两种不同的 NMF 多重算法。它们只在矫正规则2中使用 的乘法因子上略有不同。一种算法可以最小化传统的最小二乘误差,而 另一种算法则能将广义的 Kullback-Leibler 发散度最小化。两种算法 的单调收敛性均可使用类似于用于证明期望最大化算法收敛的辅助函 数来证明。 这些算法采用对角比例梯度下降的方式,重新调整因子被 最优选择以确保收敛。
机器学习白皮书系列之二:无监督学习的方法介绍及金融领域应用实例
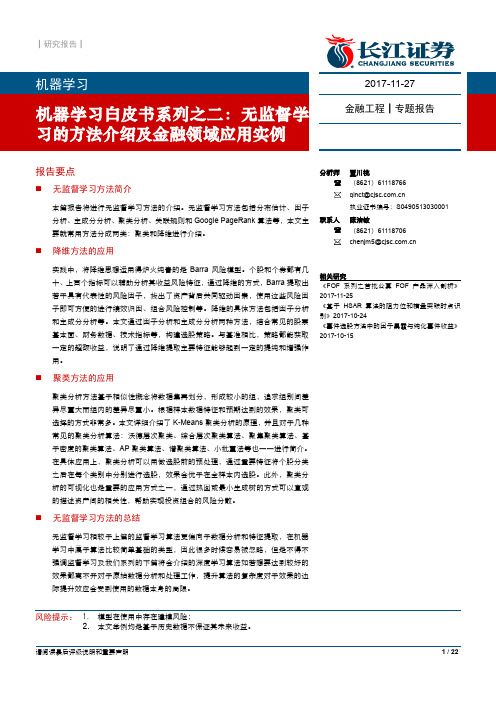
┃研究报告┃2017-11-27金融工程┃专题报告报告要点⏹无监督学习方法简介本篇报告将进行无监督学习方法的介绍。
无监督学习方法包括分布估计、因子分析、主成分分析、聚类分析、关联规则和Google PageRank算法等,本文主要就常用方法分成两类:聚类和降维进行介绍。
⏹降维方法的应用实践中,将降维思想运用得炉火纯青的是Barra风险模型。
个股和个券都有几十、上百个指标可以辅助分析其收益风险特征,通过降维的方式,Barra提取出若干具有代表性的风险因子,找出了资产背后共同驱动因素,使用这些风险因子即可方便的进行绩效归因、组合风险控制等。
降维的具体方法包括因子分析和主成分分析等。
本文通过因子分析和主成分分析两种方法,结合常见的股票基本面、财务数据、技术指标等,构建选股策略。
与基准相比,策略都能获取一定的超额收益,说明了通过降维提取主要特征能够起到一定的提纯和增强作用。
⏹聚类方法的应用聚类分析方法基于相似性概念将数据集再划分,形成较小的组,追求组别间差异尽量大而组内的差异尽量小。
根据样本数据特征和预期达到的效果,聚类可选择的方式非常多。
本文详细介绍了K-Means聚类分析的原理,并且对于几种常见的聚类分析算法:沃德层次聚类、综合层次聚类算法、聚集聚类算法、基于密度的聚类算法、AP聚类算法、谱聚类算法、小批量法等也一一进行简介。
在具体应用上,聚类分析可以用做选股前的预处理,通过重要特征将个股分类之后在每个类别中分别进行选股,效果会优于在全样本内选股。
此外,聚类分析的可视化也是重要的应用方式之一,通过热图或最小生成树的方式可以直观的描述资产间的相关性,帮助实现投资组合的风险分散。
⏹无监督学习方法的总结无监督学习相较于上篇的监督学习算法更偏向于数据分析和特征提取,在机器学习中属于算法比较简单基础的类型,因此很多时候容易被忽略,但是不得不强调监督学习及我们系列的下篇将会介绍的深度学习算法如若想要达到较好的效果都离不开对于原始数据分析和处理工作,提升算法的复杂度对于效果的边际提升效应会受到使用的数据本身的局限。
生成对抗网络人脸生成及其检测技术研究
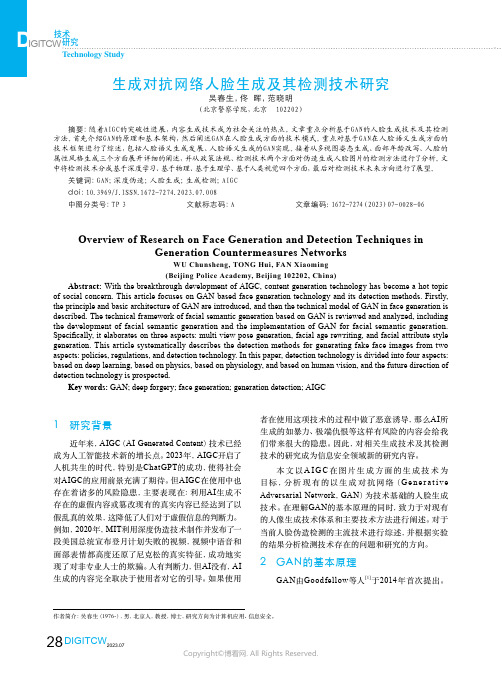
1 研究背景近年来,AIGC(AI Generated Content)技术已经成为人工智能技术新的增长点。
2023年,AIGC开启了人机共生的时代,特别是ChatGPT的成功,使得社会对AIGC的应用前景充满了期待。
但AIGC在使用中也存在着诸多的风险隐患,主要表现在:利用AI生成不存在的虚假内容或篡改现有的真实内容已经达到了以假乱真的效果,这降低了人们对于虚假信息的判断力。
例如,2020年,MIT利用深度伪造技术制作并发布了一段美国总统宣布登月计划失败的视频,视频中语音和面部表情都高度还原了尼克松的真实特征,成功地实现了对非专业人士的欺骗。
人有判断力,但AI没有,AI 生成的内容完全取决于使用者对它的引导。
如果使用者在使用这项技术的过程中做了恶意诱导,那么AI所生成的如暴力、极端仇恨等这样有风险的内容会给我们带来很大的隐患。
因此,对相关生成技术及其检测技术的研究成为信息安全领域新的研究内容。
本文以A I G C在图片生成方面的生成技术为目标,分析现有的以生成对抗网络(G e n e r a t i v e Adversarial Network,GAN)为技术基础的人脸生成技术。
在理解GAN的基本原理的同时,致力于对现有的人像生成技术体系和主要技术方法进行阐述。
对于当前人脸伪造检测的主流技术进行综述,并根据实验的结果分析检测技术存在的问题和研究的方向。
2 GAN的基本原理GAN由Goodfellow等人[1]于2014年首次提出。
生成对抗网络人脸生成及其检测技术研究吴春生,佟 晖,范晓明(北京警察学院,北京 102202)摘要:随着AIGC的突破性进展,内容生成技术成为社会关注的热点。
文章重点分析基于GAN的人脸生成技术及其检测方法。
首先介绍GAN的原理和基本架构,然后阐述GAN在人脸生成方面的技术模式。
重点对基于GAN在人脸语义生成方面的技术框架进行了综述,包括人脸语义生成发展、人脸语义生成的GAN实现。
FR_ICA1
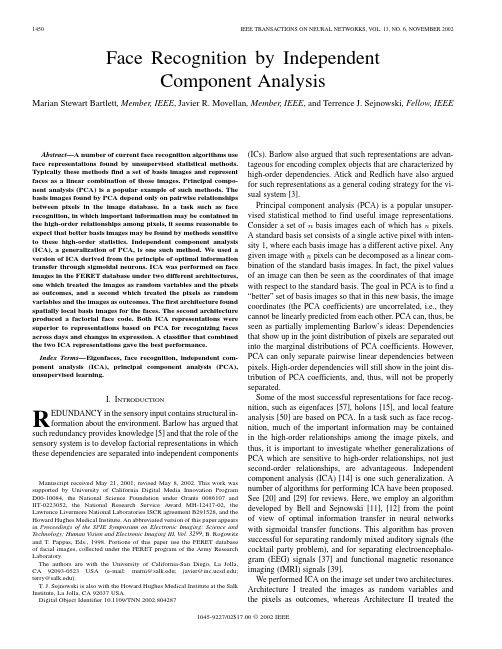
Face Recognition by IndependentComponent AnalysisMarian Stewart Bartlett,Member,IEEE,Javier R.Movellan,Member,IEEE,and Terrence J.Sejnowski,Fellow,IEEEAbstract—A number of current face recognition algorithms use face representations found by unsupervised statistical methods. Typically these methods find a set of basis images and represent faces as a linear combination of those images.Principal compo-nent analysis(PCA)is a popular example of such methods.The basis images found by PCA depend only on pairwise relationships between pixels in the image database.In a task such as face recognition,in which important information may be contained in the high-order relationships among pixels,it seems reasonable to expect that better basis images may be found by methods sensitive to these high-order statistics.Independent component analysis (ICA),a generalization of PCA,is one such method.We used a version of ICA derived from the principle of optimal information transfer through sigmoidal neurons.ICA was performed on face images in the FERET database under two different architectures, one which treated the images as random variables and the pixels as outcomes,and a second which treated the pixels as random variables and the images as outcomes.The first architecture found spatially local basis images for the faces.The second architecture produced a factorial face code.Both ICA representations were superior to representations based on PCA for recognizing faces across days and changes in expression.A classifier that combined the two ICA representations gave the best performance.Index Terms—Eigenfaces,face recognition,independent com-ponent analysis(ICA),principal component analysis(PCA), unsupervised learning.I.I NTRODUCTIONR EDUNDANCY in the sensory input contains structural in-formation about the environment.Barlow has argued that such redundancy provides knowledge[5]and that the role of the sensory system is to develop factorial representations in which these dependencies are separated into independent componentsManuscript received May21,2001;revised May8,2002.This work was supported by University of California Digital Media Innovation Program D00-10084,the National Science Foundation under Grants0086107and IIT-0223052,the National Research Service Award MH-12417-02,the Lawrence Livermore National Laboratories ISCR agreement B291528,and the Howard Hughes Medical Institute.An abbreviated version of this paper appears in Proceedings of the SPIE Symposium on Electronic Imaging:Science and Technology;Human Vision and Electronic Imaging III,Vol.3299,B.Rogowitz and T.Pappas,Eds.,1998.Portions of this paper use the FERET database of facial images,collected under the FERET program of the Army Research Laboratory.The authors are with the University of California-San Diego,La Jolla, CA92093-0523USA(e-mail:marni@;javier@; terry@).T.J.Sejnowski is also with the Howard Hughes Medical Institute at the Salk Institute,La Jolla,CA92037USA.Digital Object Identifier10.1109/TNN.2002.804287(ICs).Barlow also argued that such representations are advan-tageous for encoding complex objects that are characterized by high-order dependencies.Atick and Redlich have also argued for such representations as a general coding strategy for the vi-sual system[3].Principal component analysis(PCA)is a popular unsuper-vised statistical method to find useful image representations. Consider a setofpixels as random variables and the images as outcomes.1Matlab code for the ICA representations is available at /~marni.Face recognition performance was tested using the FERET database [52].Face recognition performances using the ICA representations were benchmarked by comparing them to per-formances using PCA,which is equivalent to the “eigenfaces”representation [51],[57].The two ICA representations were then combined in a single classifier.II.ICAThere are a number of algorithms for performing ICA [11],[13],[14],[25].We chose the infomax algorithm proposed by Bell and Sejnowski [11],which was derived from the principle of optimal information transfer in neurons with sigmoidal transfer functions [27].The algorithm is motivated as follows:Letbeaninvertiblematrix,and-neurons.Each componentof is an invertible squashing function,mapping real numbers intothe(1)The-neurons.The.The goal in Belland Sejnowski’s algorithm is to maximize the mutual informa-tion between theenvironment.The gradient update rule for the weightmatrix,,the ratio between the second andfirst partial derivatives of the activationfunction,,andof this matrixis the derivativeofwith respectto,resulting in the following learning rule[12]:encourages the individual out-puts to move toward statistical independence.When the form1Preliminaryversions of this work appear in [7]and [9].A longer discussionof unsupervised learning for face recognition appears in [6].of the nonlinear transferfunction[12],[42].In practice,thelogistic transfer function has been found sufficient to separate mixtures of natural signals with sparse distributions including sound sources [11].The algorithm is speeded up by including a “sphering”step prior to learning [12].The row meansof(4)This removes the first and the second-order statistics of the data;both the mean and covariances are set to zero and the variances are equalized.When the inputs to ICA are the “sphered”data,the full transformmatrixfor the following generative model of thedata:,the inverse of the weight matrix in Bell and Sejnowski’s algo-rithm,can be interpreted as the source mixing matrix andthevariables can be interpreted as the maximum-likeli-hood (ML)estimates of the sources that generated the data.A.ICA and Other Statistical TechniquesICA and PCA:PCA can be derived as a special case of ICA which uses Gaussian source models.In such case the mixingmatrixis unidentifiable in the sense that there is an infinite number of equally good ML solutions.Among all possible ML solutions,PCA chooses an orthogonal matrix which is optimal in the following sense:1)Regardless of the distributionofwhich areuncorrelatedwith.If the sources are Gaussian,the likelihood of the data depends only on first-and second-order statistics (the covariance matrix).In PCA,the rowsofof natural images,we can scramble their phase spectrum while maintaining their power spectrum.This will dramatically alter the appearance of the images but will not change their second-order statistics.The phase spectrum,not the power spectrum,contains the structural information in images that drives human perception.For example,as illustrated in Fig.1,a face image synthesized from the amplitude spectrum of face A and the phase spectrum of face B will be perceived as an image of face B [45],[53].The fact that PCA is only sensitive to the power spectrum of images suggests that it might not be particularly well suited for representing natural images.The assumption of Gaussian sources implicit in PCA makes it inadequate when the true sources are non-Gaussian.In par-ticular,it has been empirically observed that many natural signals,including speech,natural images,and EEG are better described as linear combinations of sources with long tailed distributions [11],[19].These sources are called “high-kur-tosis,”“sparse,”or “super-Gaussian”sources.Logistic random variables are a special case of sparse source models.When sparse source models are appropriate,ICA has the following potential advantages over PCA:1)It provides a better proba-bilistic model of the data,which better identifies where the data concentratein.3)It finds a not-necessarily orthogonalbasis which may reconstruct the data better than PCA in the presence of noise.4)It is sensitive to high-order statistics in the data,not just the covariance matrix.Fig.2illustrates these points with an example.The figure shows samples from a three-dimensional (3-D)distribution constructed by linearly mixing two high-kurtosis sources.The figure shows the basis vectors found by PCA and by ICA on this problem.Since the three ICA basis vectors are nonorthogonal,they change the relative distance between data points.This change in metric may be potentially useful for classification algorithms,like nearest neighbor,that make decisions based on relative distances between points.The ICA basis also alters the angles between data points,which affects similarity measures such as cosines.Moreover,if an undercomplete basis set is chosen,PCA and ICA may span different subspaces.For example,in Fig.2,when only two dimensions are selected,PCA and ICA choose different subspaces.The metric induced by ICA is superior to PCA in the sense that it may provide a representation more robust to the effect of noise [42].It is,therefore,possible for ICA to be better than PCA for reconstruction in noisy or limited precision environ-ments.For example,in the problem presented in Fig.2,we found that if only 12bits are allowed to represent the PCA and ICA coefficients,linear reconstructions based on ICA are 3dB better than reconstructions based on PCA (the noise power is re-duced by more than half).A similar result was obtained for PCA and ICA subspaces.If only four bits are allowed to represent the first 2PCA and ICA coefficients,ICA reconstructions are 3dB better than PCA reconstructions.In some problems,one can think of the actual inputs as noisy versions of some canon-ical inputs.For example,variations in lighting and expressions can be seen as noisy versions of the canonical image of a person.Having input representations which are robust to noise may po-tentially give us representations that better reflect thedata.Fig.1.(left)Two face images.(Center)The two faces with scrambled phase.(right)Reconstructions with the amplitude of the original face and the phase of the other face.Faces images are from the FERET face database,reprinted with permission from J.Phillips.When the sources models are sparse,ICA is closely related to the so called nonorthogonal “rotation”methods in PCA and factor analysis.The goal of these rotation methods is to find di-rections with high concentrations of data,something very sim-ilar to what ICA does when the sources are sparse.In such cases,ICA can be seen as a theoretically sound probabilistic method to find interesting nonorthogonal “rotations.”ICA and Cluster Analysis:Cluster analysis is a technique for finding regionsinbe a data matrixwithas outcomes (independent trials)of a random experiment.We think of theas the specific value taken by a randomvariableFig.2.(top)Example3-D data distribution and corresponding PC and IC axes.Each axis is a column of the mixing matrix W found by PCA or ICA.Note the PC axes are orthogonal while the IC axes are not.If only two components are allowed,ICA chooses a different subspace than PCA.(bottom left)Distribution of the first PCA coordinates of the data.(bottom right)Distribution of the first ICA coordinates of the data.Note that since the ICA axes are nonorthogonal,relative distances between points are different in PCA than in ICA,as are the angles between points.a distribution.For example,we say that rows ofOur goal in this paper is to find a good set of basis imagesto represent a database of faces.We organize each image in thedatabase as a long vector with as many dimensions as numberof pixels in the image.There are at least two ways in which ICAcan be applied to this problem.1)We can organize our database into a matrixare independent if whenmoving across pixels,it is not possible to predict the valuetaken by the pixel on imageare in the columns ofsponding value taken by pixelFig.4.Image synthesis model for Architecture I.To find a set of IC images,the images in X are considered to be a linear combination of statistically independent basis images,S ,where A is an unknown mixing matrix.The basis images were estimated as the learned ICA output U.Fig.5.Image synthesis model for Architecture II,based on [43]and [44].Each image in the dataset was considered to be a linear combination of underlying basis images in the matrix A .The basis images were each associated with a set of independent “causes,”given by a vector of coefficients in S .The basisimages were estimated by A =Wis the learned ICA weight matrix.individual.The training set was comprised of 50%neutral ex-pression images and 50%change of expression images.The al-gorithms were tested for recognition under three different con-ditions:same session,different expression;different day,same expression;and different day,different expression (see Table I).Coordinates for eye and mouth locations were provided with the FERET database.These coordinates were used to center the face images,and then crop and scale them to60so that the images are in rows and the pixels arein columns,i.e.,such that the rowsof,as shown in Fig.7.These coordinatesare contained in the mixingmatrixlinear combinations of those images,where.Recall that the image synthesis model assumes that the imagesintions (pixels).The use of PCA vectors in the input did not throw away the high-order relationships.These relationships still ex-isted in the data but were not separated.Letindependent source images in the rowsofthat comprised the face imagesinis obtainedbythat comprised,wherewas a minimum squared error approximationofwas,therefore,given by the rows of thematrix3000eigenvec-torsin3000inputmatrixwere updated according to (3)for 1900iterations.The learning rate was initialized at 0.0005and annealed down3B.However,if ICA did not removeall of the second-order dependencies then U will not be precisely orthonormal.4In pilot work,we found that face recognition performance improved with the number of components separated.We chose 200components as the largest number to separate within our processing limitations.5Although PCA already removed the covariances in the data,the variances were not equalized.We,therefore,retained the spheringstep.Fig.8.Twenty-five ICs of the image set obtained by Architecture I,which provide a set of statistically independent basis images (rows of U in Fig.4).ICs are ordered by the class discriminability ratio,r (4).to 0.0001.Training took 90minutes on a Dec Alpha 2100a.Fol-lowing training,a set of statistically independent source images were contained in the rows of the outputmatrixfound by ICA represents a cluster of pixelsthat have similar behavior across images.Each row oftheby the nearest neighbor algorithm,using cosinesas the similarity measure.Coefficient vectors in each test set were assigned the class label of the coefficient vector in the training set that was most similar as evaluated by the cosine of the angle betweenthem.Fig.9.First25PC axes of the image set(columns of P),ordered left to right, top to bottom,by the magnitude of the corresponding eigenvalue.In experiments to date,ICA performs significantly better using cosines rather than Euclidean distance as the similarity measure,whereas PCA performs the same for both.A cosine similarity measure is equivalent to length-normalizing the vectors prior to measuring Euclidean distance when doing nearestneighbor,be the overall meanof acoefficient.For both the PCA and ICA representations,we calculated theratio of between-class to within-classvariabilityclassmeans,andwere calculated separately for each test set,ex-cluding the test images from the analysis.Both the PCA and ICAcoefficients were then ordered by the magnitudeofFig.11.Selection of components by class discriminability,Architecture II. Top:Discriminability of the ICA coefficients(solid lines)and discriminability of the PCA components(dotted lines)for the three test ponents were sorted by the magnitude of r.Bottom:Improvement in face recognition performance for the ICA and PCA representations using subsets of components selected by the class discriminability r.The improvement is indicated by the gray segments at the top of the bars.Face classification performance was compared usingthe,so thatrows represent different pixels and columns represent differentimages.[See(Fig.3right)].This corresponds to treating thecolumnsof.Eachcolumnof(Fig.12).ICA attempts tomake theoutputs,comprised the columns ofthe input data matrix,where each coefficient had zero mean.The Architecture II representation for the training images wastherefore contained in the columnsofwas200for each face image,consisting of the outputsof each of the ICA filters.7The architecture II representation fortest images was obtained in the columnsof.A sample of the basis images is shown6Here,each pixel has zero mean.7An image filter f(x)is defined as f(x)=w1x.Fig.13.Basis images for the ICA-factorial representation(columns of AAA(a)(b)Fig.16.Pairwise mutual information.(a)Mean mutual information between basis images.Mutual information was measured between pairs of gray-level images,PC images,and independent basis images obtained by Architecture I.(b)Mean mutual information between coding variables.Mutual information was measured between pairs of image pixels in gray-level images,PCA coefficients, and ICA coefficients obtained by Architecture II.obtained85%,56%,and44%correct,respectively.Again,as found for200separated components,selection of subsets of components by class discriminability improved the performance of ICA1to86%,78%,and65%,respectively,and had little ef-fect on the performances with the PCA and ICA2representa-tions.This suggests that the results were not simply an artifact due to small sample size.VI.E XAMINATION OF THE ICA R EPRESENTATIONSA.Mutual InformationA measure of the statistical dependencies of the face repre-sentations was obtained by calculating the mean mutual infor-mation between pairs of50basis images.Mutual information was calculatedas(18)where.Again,therewere considerable high-order dependencies remaining in thePCA representation that were reduced by more than50%by theinformation maximization algorithm.The ICA representationsobtained in these simulations are most accurately described notas“independent,”but as“redundancy reduced,”where the re-dundancy is less than half that in the PC representation.B.SparsenessField[19]has argued that sparse distributed representationsare advantageous for coding visual stimuli.Sparse representa-tions are characterized by highly kurtotic response distributions,in which a large concentration of values are near zero,with rareoccurrences of large positive or negative values in the tails.Insuch a code,the redundancy of the input is transformed intothe redundancy of the response patterns of the the individualoutputs.Maximizing sparseness without loss of information isequivalent to the minimum entropy codes discussed by Barlow[5].8Given the relationship between sparse codes and minimumentropy,the advantages for sparse codes as outlined by Field[19]mirror the arguments for independence presented byBarlow[5].Codes that minimize the number of active neuronscan be useful in the detection of suspicious coincidences.Because a nonzero response of each unit is relatively rare,high-order relations become increasingly rare,and therefore,more informative when they are present in the stimulus.Field8Information maximization is consistent with minimum entropy coding.Bymaximizing the joint entropy of the output,the entropies of the individual out-puts tend to be minimized.Fig.18.Recognition successes and failures.{left)Two face image pairs which both ICA algorithms correctly recognized.(right)Two face image pairs that were misidentified by both ICA algorithms.Images from the FERET face database were reprinted with permission from J.Phillips.contrasts this with a compact code such as PCs,in which a few units have a relatively high probability of response,and there-fore,high-order combinations among this group are relatively common.In a sparse distributed code,different objects are rep-resented by which units are active,rather than by how much they are active.These representations have an added advantage in signal-to-noise,since one need only determine which units are active without regard to the precise level of activity.An ad-ditional advantage of sparse coding for face representations is storage in associative memory works with sparse inputs can store more memories and provide more effective re-trieval with partial information[10],[47].The probability densities for the values of the coefficients of the two ICA representations and the PCA representation are shown in Fig.17.The sparseness of the face representations were examined by measuring the kurtosis of the distributions. Kurtosis is defined as the ratio of the fourth moment of the dis-tribution to the square of the second moment,normalized to zero for the Gaussian distribution by subtracting3kurtosis,,,correspond to the similaritymeasure.Performance of the combined classifier is shown in Fig.19.Thecombined classifier improved performance to91.0%,88.9%,and81.0%for the three test cases,respectively.The difference inperformance between the combined ICA classifier and PCA wassignificant for all three test sets(,,;auditory signals into independent sound sources.Under this ar-chitecture,ICA found a basis set of statistically independent im-ages.The images in this basis set were sparse and localized in space,resembling facial features.Architecture II treated pixels as random variables and images as random trials.Under this ar-chitecture,the image coefficients were approximately indepen-dent,resulting in a factorial face code.Both ICA representations outperformed the“eigenface”rep-resentation[57],which was based on PC analysis,for recog-nizing images of faces sampled on a different day from the training images.A classifier that combined the two ICA rep-resentations outperformed eigenfaces on all test sets.Since ICA allows the basis images to be nonorthogonal,the angles and dis-tances between images differ between ICA and PCA.Moreover, when subsets of axes are selected,ICA defines a different sub-space than PCA.We found that when selecting axes according to the criterion of class discriminability,ICA-defined subspaces encoded more information about facial identity than PCA-de-fined subspaces.ICA representations are designed to maximize information transmission in the presence of noise and,thus,they may be more robust to variations such as lighting conditions,changes in hair,make-up,and facial expression,which can be considered forms of noise with respect to the main source of information in our face database:the person’s identity.The robust recogni-tion across different days is particularly encouraging,since most applications of automated face recognition contain the noise in-herent to identifying images collected on a different day from the sample images.The purpose of the comparison in this paper was to examine ICA and PCA-based representations under identical conditions.A number of methods have been presented for enhancing recognition performance with eigenfaces(e.g.,[41]and[51]). ICA representations can be used in place of eigenfaces in these techniques.It is an open question as to whether these techniques would enhance performance with PCA and ICA equally,or whether there would be interactions between the type of enhancement and the representation.A number of research groups have independently tested the ICA representations presented here and in[9].Liu and Wech-sler[35],and Yuen and Lai[61]both supported our findings that ICA outperformed PCA.Moghaddam[41]employed Euclidean distance as the similarity measure instead of cosines.Consistent with our findings,there was no significant difference between PCA and ICA using Euclidean distance as the similarity mea-sure.Cosines were not tested in that paper.A thorough compar-ison of ICA and PCA using a large set of similarity measures was recently conducted in[17],and supported the advantage of ICA for face recognition.In Section V,ICA provided a set of statistically independent coefficients for coding the images.It has been argued that such a factorial code is advantageous for encoding complex objects that are characterized by high-order combinations of features, since the prior probability of any combination of features can be obtained from their individual probabilities[2],[5].According to the arguments of both Field[19]and Barlow[5],the ICA-fac-torial representation(Architecture II)is a more optimal object representation than the Architecture I representation given its sparse,factorial properties.Due to the difference in architec-ture,the ICA-factorial representation always had fewer training samples to estimate the same number of free parameters as the Architecture I representation.Fig.16shows that the residual de-pendencies in the ICA-factorial representation were higher than in the Architecture I representation.The ICA-factorial repre-sentation may prove to have a greater advantage given a much larger training set of images.Indeed,this prediction has born out in recent experiments with a larger set of FERET face im-ages[17].It also is possible that the factorial code representa-tion may prove advantageous with more powerful recognition engines than nearest neighbor on cosines,such as a Bayesian classifier.An image set containing many more frontal view im-ages of each subject collected on different days will be needed to test that hypothesis.In this paper,the number of sources was controlled by re-ducing the dimensionality of the data through PCA prior to per-forming ICA.There are two limitations to this approach[55]. The first is the reverse dimensionality problem.It may not be possible to linearly separate the independent sources in smaller subspaces.Since we retained200dimensions,this may not have been a serious limitation of this implementation.Second,it may not be desirable to throw away subspaces of the data with low power such as the higher PCs.Although low in power,these sub-spaces may contain ICs,and the property of the data we seek is independence,not amplitude.Techniques have been proposed for separating sources on projection planes without discarding any ICs of the data[55].Techniques for estimating the number of ICs in a dataset have also recently been proposed[26],[40]. The information maximization algorithm employed to per-form ICA in this paper assumed that the underlying“causes”of the pixel gray-levels in face images had a super-Gaussian (peaky)response distribution.Many natural signals,such as sound sources,have been shown to have a super-Gaussian distribution[11].We employed a logistic source model which has shown in practice to be sufficient to separate natural signals with super-Gaussian distributions[11].The under-lying“causes”of the pixel gray-levels in the face images are unknown,and it is possible that better results could have been obtained with other source models.In particular,any sub-Gaussian sources would have remained mixed.Methods for separating sub-Gaussian sources through information maximization have been developed[30].A future direction of this research is to examine sub-Gaussian components of face images.The information maximization algorithm employed in this work also assumed that the pixel values in face images were generated from a linear mixing process.This linear approxima-tion has been shown to hold true for the effect of lighting on face images[21].Other influences,such as changes in pose and ex-pression may be linearly approximated only to a limited extent. Nonlinear ICA in the absence of prior constraints is an ill-condi-tioned problem,but some progress has been made by assuming a linear mixing process followed by parametric nonlinear func-tions[31],[59].An algorithm for nonlinear ICA based on kernel methods has also recently been presented[4].Kernel methods have already shown to improve face recognition performance。
基于人脸识别的学生听课状态监测技术
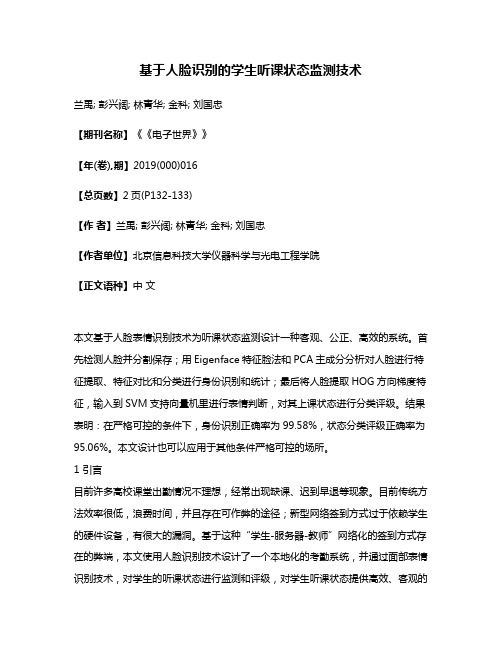
基于人脸识别的学生听课状态监测技术兰禹; 彭兴阔; 林青华; 金科; 刘国忠【期刊名称】《《电子世界》》【年(卷),期】2019(000)016【总页数】2页(P132-133)【作者】兰禹; 彭兴阔; 林青华; 金科; 刘国忠【作者单位】北京信息科技大学仪器科学与光电工程学院【正文语种】中文本文基于人脸表情识别技术为听课状态监测设计一种客观、公正、高效的系统。
首先检测人脸并分割保存;用Eigenface特征脸法和PCA主成分分析对人脸进行特征提取、特征对比和分类进行身份识别和统计;最后将人脸提取HOG方向梯度特征,输入到SVM支持向量机里进行表情判断,对其上课状态进行分类评级。
结果表明:在严格可控的条件下,身份识别正确率为99.58%,状态分类评级正确率为95.06%。
本文设计也可以应用于其他条件严格可控的场所。
1 引言目前许多高校课堂出勤情况不理想,经常出现缺课、迟到早退等现象。
目前传统方法效率很低,浪费时间,并且存在可作弊的途径;新型网络签到方式过于依赖学生的硬件设备,有很大的漏洞。
基于这种“学生-服务器-教师”网络化的签到方式存在的弊端,本文使用人脸识别技术设计了一个本地化的考勤系统,并通过面部表情识别技术,对学生的听课状态进行监测和评级,对学生听课状态提供高效、客观的评判依据,在保持高正确率的情况下,克服了一般方式过于依赖硬件设备的缺点。
这个系统同样可以应用在类似的场景,例如中小学授课、公司考勤和工作状态评定等场景。
2 系统实现方案本项目用于学生听课状态监测。
系统流程图如图1所示。
首先在全局光照正常的条件下采取每个学生的两张人脸图像作为人脸库。
在课堂上对摄像机拍摄的实时视频进行处理,每隔一段时间进行一次截帧,得到的图像通过Matlab的Face Parts Detection工具包捕捉人脸并分割,操作结果作为测试图像。
身份识别采用Eigenface特征脸法,利用主成分分析进行特征计算,将测试图像和训练集人脸库中的图像进行匹配,进行身份识别。
eigenfaces for recognition 一文中,核心步骤
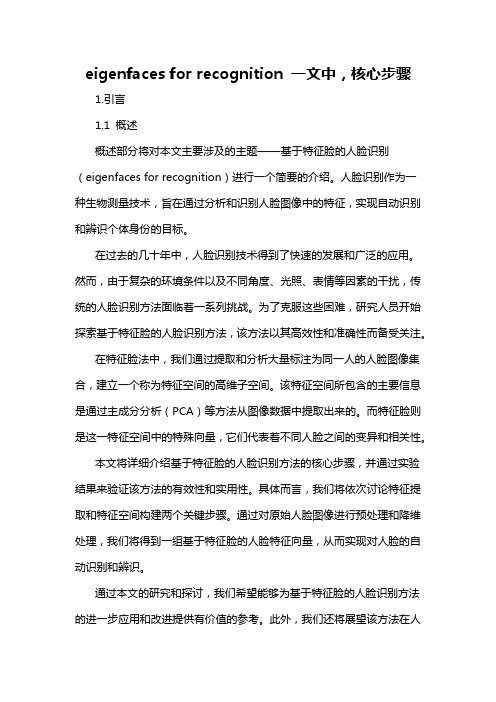
eigenfaces for recognition 一文中,核心步骤1.引言1.1 概述概述部分将对本文主要涉及的主题——基于特征脸的人脸识别(eigenfaces for recognition)进行一个简要的介绍。
人脸识别作为一种生物测量技术,旨在通过分析和识别人脸图像中的特征,实现自动识别和辨识个体身份的目标。
在过去的几十年中,人脸识别技术得到了快速的发展和广泛的应用。
然而,由于复杂的环境条件以及不同角度、光照、表情等因素的干扰,传统的人脸识别方法面临着一系列挑战。
为了克服这些困难,研究人员开始探索基于特征脸的人脸识别方法,该方法以其高效性和准确性而备受关注。
在特征脸法中,我们通过提取和分析大量标注为同一人的人脸图像集合,建立一个称为特征空间的高维子空间。
该特征空间所包含的主要信息是通过主成分分析(PCA)等方法从图像数据中提取出来的。
而特征脸则是这一特征空间中的特殊向量,它们代表着不同人脸之间的变异和相关性。
本文将详细介绍基于特征脸的人脸识别方法的核心步骤,并通过实验结果来验证该方法的有效性和实用性。
具体而言,我们将依次讨论特征提取和特征空间构建两个关键步骤。
通过对原始人脸图像进行预处理和降维处理,我们将得到一组基于特征脸的人脸特征向量,从而实现对人脸的自动识别和辨识。
通过本文的研究和探讨,我们希望能够为基于特征脸的人脸识别方法的进一步应用和改进提供有价值的参考。
此外,我们还将展望该方法在人脸识别领域未来的发展和应用前景,为相关研究和实践提供新的思路和方向。
1.2 文章结构文章结构部分的内容可以按照以下方式编写:文章结构本文主要分为引言、正文和结论三个部分。
其中,引言部分包括概述、文章结构和目的三个小节;正文部分包括特征提取和特征空间构建两个小节;结论部分包括总结和展望两个小节。
引言部分在引言部分,我们将首先对本文的研究课题进行一个概述,引入主题。
随后,我们将介绍文章的结构,明确各个部分的内容和组织顺序。
翻译2

Bayesian face *, Tony Jebara , Alex Pentland
Mitsubishi Electric Research Laboratory, 201 Broadway, 8th yoor, Cambridge, MA 02139, USA Massachusettes Institute of Technology, Cambridge, MA 02139, USA Received 15 January 1999; received in revised form 28 July 1999; accepted 28 July 1999
1772
B. Moghaddam et al. / Pattern Recognition 33 (2000) 1771 } 1782
discriminant analysis (LDA) as used by Etemad and Chellappa [16], the `Fisherfacea technique of Belhumeur et al. [17], hierarchical discriminants used by Swets and Weng [18] and `evolutionary pursuita of optimal subspaces by Liu and Wechsler [19] * all of which have proved equally (if not more) powerful than standard `eigenfacesa. Eigenspace techniques have also been applied to modeling the shape (as opposed to texture) of the face. Eigenspace coding of shape-normalized or `shape-freea faces, as suggested by Craw and Cameron [20], is now a standard pre-processing technique which can enhance performance when used in conjunction with shape information [21]. Lanitis et al. [22] have developed an automatic face-processing system with subspace models of both the shape and texture components, which can be used for recognition as well as expression, gender and pose classi"cation. Additionally, subspace analysis has also been used for robust face detection [12,14,23], nonlinear facial interpolation [24], as well as visual learning for general object recognition [13,25,26].