Royden_Real_Analysis_Solutions
Ordyl SY 300商品说明书
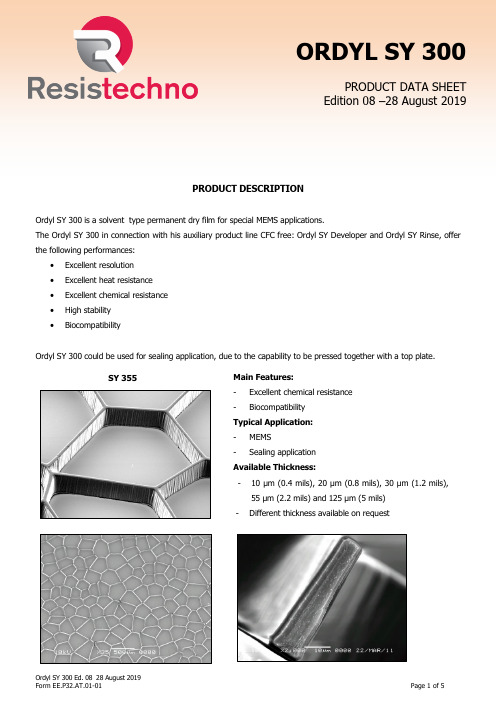
PRODUCT DESCRIPTIONOrdyl SY 300 is a solvent type permanent dry film for special MEMS applications.The Ordyl SY 300 in connection with his auxiliary product line CFC free: Ordyl SY Developer and Ordyl SY Rinse, offer the following performances:∙Excellent resolution ∙Excellent heat resistance ∙Excellent chemical resistance ∙High stability ∙BiocompatibilityOrdyl SY 300 could be used for sealing application, due to the capability to be pressed together with a top plate.SY 355Main Features: -Excellent chemical resistance -Biocompatibility Typical Application:-MEMS-Sealing applicationAvailable Thickness:-10 µm (0.4 mils), 20 µm (0.8 mils), 30 µm (1.2 mils), 55 µm (2.2 mils) and 125 µm (5 mils) -Different thickness available on requestORDYL SY 300PRODUCT DATA SHEETEdition 08 –28 August 2019PROCESS INFORMATIONOrdyl SY 300 guarantee good adhesion on the following surface:-Glass-Silicium-Kapton-MylarWe recommend good surface cleaning in order to obtain optimal performance.LaminationPanels must be thoroughly dry prior to lamination.MANUAL LAMINATOR AUTOMATIC LAMINATOR Pre-heat (OPTIONAL) (OPTIONAL) Hot roll temperature 105 – 125°C (221 – 257°F) 105 – 125°C (221 – 257°F)Lamination roll pressure 2.5 – 3.5 bar (36 – 50 Psi) 2.5 – 6.0 bar (36 – 87 Psi) Lamination speed 1 – 3m/min (3 – 10 feet/min) 1 – 3m/min (3 – 10 feet/min)Seal temperature --- 40 – 80°C (104 – 176°F)Seal pressure --- 3.0 – 6.0 bar (44 – 87 Psi)Seal time --- 1 – 4 sec.Post lamination Hold TimeWe recommend a hold time of at least 15 min, or in any case the minimum hold time necessary to allow panels to cool down to room temperature.Hold time should not be over 1 week.ExposureWe recommend using UV lamps or laser source with emission peak at 360 – 380 nm.Energy (mJ/cm2) 100 150 200 250 300 350 400 SST 21 5 6 7 8 9 9.5 10RST 25 4 7 10 13 16 17-18 19Hold Time after exposureWe recommend a minimum hold time after exposure of at least 15 minutes.DevelopingSY 300 could be develop with spray, paddle or dipping method.Using Ordyl SY Developer in dipping process at room temperature maintain the Break Point between 60% and 80% depend on application.Use Ordyl SY Rinse to remove scum and clean the surface.If final rinse with DI water is necessary an intermediate rinse with IPA is suggested.Post BakeAfter developing is necessary a post-baking at 150°C (302°F) for 30 – 60 min.StrippingOrdyl SY 300 could be stripped only before post bake using Ordyl SY Developer increasing dipping time indicated in “Ordyl SY Developer product data sheet” or using solvent like Acetone or MEK in dipping method.RESIST PROFILEFor the test we used a 55 µm thickness dry film, laminated on SiO2 wafer.EXPOSURE LINE SPACE100 mJ/cm260 µm (2.4 mils) 50 µm (2 mils)150 mJ/cm250 µm (2 mils) 60 µm (2.4 mils)200 mJ/cm240 µm (1.6 mils) 70 µm (2.8 mils)250 mJ/cm240 µm (1.6 mils) 80 µm (3.1 mils) Exposure Unit ORC HMW201B not collimated.REFERENCES:A Lab-On-A-Chip For Automated RNA Extraction From Bacteriahttp://www.freidok.uni-freiburg.de/volltexte/7020/Lab-on-a-Foil: microfluidics on thin and flexible filmsThis critical review is motivated by an increasing interest of the microfluidics community in developing complete Lab-on-a-Chip solutions based on thin and flexible films (Lab-on-a-Foil).Those implementations benefit from a broad range of fabrication methods that are partly adopted from well-established macroscale processes or are completely new and promising. In addition, thin and flexible foils enable various features like low thermal resistance for efficient thermocycling or integration of easily deformable chambers paving the way for new means of on-chip reagent storage or fluid transport. From an economical perspective, Lab-on-a-Foil systems are characterised by low material consumption and often low-cost materials which are attractive for cost-effective high-volume fabrication of self-contained disposable chips. The first part of this review focuses on available materials, fabrication processes and approaches for integration of microfluidic functions including liquid control and transport as well as storage and release of reagents. In the second part, an analysis of the state of Lab-on-a-Foil applications is provided with a special focus on nucleic acid analysis, immunoassays, cell-based assays and home care testing. We conclude that the Lab-on-a-Foil approach is very versatile and significantly expands the toolbox for the development of Lab-on-a-Chip solutions.http://www.loac-imtek.de/fileadmin/loac/Projekte_und_Publikationen/Ausgewaehlte_Publikationen/lab-on-a-foil_-_microfluidics_on_thin_and_flex.pdfDry Film Resist For Fast Fluidic PrototypingDry film photoresist is used for creating microfluidic structures by sandwiching the patterned resist in between of two substrates.The technique is applied for creating hybrid biochips for dielectrophoretic cell manipulation.Multiple level lithography is demonstrated and biocompatibility of the resist is proven.Due to simple fabrication procedures the resist can be processed in a low-tech environment.http://hal.inria.fr/docs/00/34/64/73/PDF/Dry_film_resist_for_fast_fluidic_prototyping.pdfFor any other technical information (storage conditions, packaging information, etc.) refer to the Ordyl Specification (Form EE.P11.CV.02-ww).The information stated in this Data Sheet regarding the use of materials is based upon experience under laboratory controls. Elga Europe makes no guaranty or warranty, express or implied, to such use, handling or possession of such materials, or of the application of any process described in our bulletins of the results sought to be obtained, whether in accordance with the directions or claimed so to be. Any informationor statements contained herein are expressly made subject to the foregoing provisions and the terms and conditions embodied in our invoice covering such materials with are to be deemed part herein. The publication hereof describing any process is not to be deemed not taken as license to operate under, nor recommendation to infringe, any patent.The seller binds itself only to deliver goods in accordance whit the general description upon which they are sold whether or not any special particular description shall have been given or implied by law.Any such special or particular description shall be taken only as the expression of seller’s opinion in that behalf. The seller does not give any warranty as to the quality (save that the goods are of merchantable quality), state condition fitness of the goods or use to which the goods may be put. Claims on account of weight, loss of or damage to the goods in transit ( so far as seller is liable) shall be made in writing to the seller within the period of 30 days of receipt thereof.No claim shall be entertained after the expiration of the appropriate period mentioned above and the seller’s liability by reason of any such claim shall not in any event the purchase price of the goods in respect of which a claim is made. Goods shall not be returned to the seller without the seller’s express written permission.Via Caldara 3 - 20020 Lainate (MI) ItalyE-mail: ********************。
演示文稿数学专业英语第八讲附数学课程英文表达
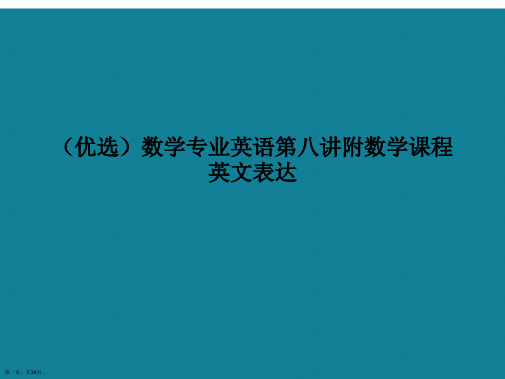
4、L. Hormander “Linear Partial Differential Operators, ” I&II:偏微分方程的经典参考书; 5、A Course in Abstract Harmonic Analysis by Folland:高级的研究生调和分析教材; 6、Abstract Harmonic Analysis by Ross Hewitt:抽象调和分析的经典参考书; 7、Harmonic Analysis by Elias M. Stein:标准的研究生调和分析教材; 8、Elliptic Partial Differential Equations of Second Order by David Gilbarg:偏微分 方程的经典参考书; 9、Partial Differential Equations ,by Jeffrey Rauch:标准的研究生偏微分方程教材。
覆盖范围较广;
9、Elements of Homotopy Theory by G.W. Whitehead:高级、经典的代数拓扑参考 书。
第六页,共34页。
• 实分析、泛函分析:
1、Royden, Real analysis:标准研究生分析教材; 2、Walter Rudin, Real and complex analysis:标准研究生分析教材;
;
4、Principles of Algebraic Geometry by giffiths/harris:全面、经典的代数几何参考 书,偏复代数几何; 5、Commutative Algebra with a view toward Algebraic Geometry by Eisenbud:高
调代数参考书;
基于粒子滤波算法的方位跟踪方法研究

基于粒子滤波算法的方位跟踪方法研究周彤;宋立臣【摘要】与传统的声压水听器相比,矢量水听器(AVS)可以同时、共点接收水下声场的声压和振速信息,利用单个水听器可以估计出目标的方位(DOA)信息.本文将MUISIC方位估计方法和粒子滤波(Particle Filtering,PF)跟踪方法结合,给出一种高效的水下运动目标的实时方位跟踪方法(PF-MUSIC),仿真对比分析了MUSIC方法和PF-MUISC方法的方位跟踪性能,并通过海上试验验证了跟踪方法的实用性.结果表明:PF-MUSIC法的计算量远小于MUSIC方法,PF-MUSIC方法的误差更小并且跟踪曲线更平滑,适用于对水下目标的实时方位跟踪.【期刊名称】《舰船科学技术》【年(卷),期】2018(040)011【总页数】5页(P134-138)【关键词】粒子滤波;单矢量水听器;方位跟踪【作者】周彤;宋立臣【作者单位】大连测控技术研究所,辽宁大连 116013;大连测控技术研究所,辽宁大连 116013【正文语种】中文【中图分类】TP3910 引言在舰船水下辐射噪声测量的过程中,安全、可靠地引导被测目标通过测量区域,并保证被测目标与测量系统之间的有效距离以满足测试需求是极其重要的,也是一直关心的问题。
现有的被测目标引导方法,往往依靠单一导航信标配合测距系统实现,通常仅提供被测目标与导航信标之间的单点距离信息,缺乏方位信息,使得目标实际航行轨迹与试验要求轨迹有一定的偏差,造成测量单程无效,从而降低了测试效率,延长了试验时间[1]。
目前方位估计算法的通常假设目标在观测时间内为静止状态,即静态方位算法,同时采用大计算量和多快拍数的批处理形式进行方位估计[2]。
然而在实际情况下,目标通常为运动状态,批处理方式的静态方位估计方法不适用于实时连续跟踪。
同时,静态方位估计方法是互不相关地估计2个连续时刻的方位信息,并没有考虑到2个连续时刻状态的关联性,进而造成动态方位估计偏差较大。
219515743_辐射源个体识别的一种可解释性测试架构
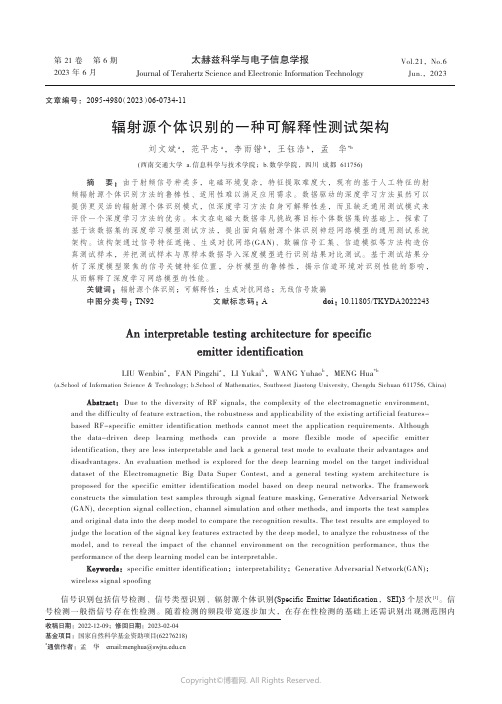
第 21 卷 第 6 期2023 年 6 月太赫兹科学与电子信息学报Journal of Terahertz Science and Electronic Information TechnologyVol.21,No.6Jun.,2023辐射源个体识别的一种可解释性测试架构刘文斌a,范平志a,李雨锴b,王钰浩b,孟华*b(西南交通大学 a.信息科学与技术学院;b.数学学院,四川成都611756)摘要:由于射频信号种类多,电磁环境复杂,特征提取难度大,现有的基于人工特征的射频辐射源个体识别方法的鲁棒性、适用性难以满足应用需求。
数据驱动的深度学习方法虽然可以提供更灵活的辐射源个体识别模式,但深度学习方法自身可解释性差,而且缺乏通用测试模式来评价一个深度学习方法的优劣。
本文在电磁大数据非凡挑战赛目标个体数据集的基础上,探索了基于该数据集的深度学习模型测试方法,提出面向辐射源个体识别神经网络模型的通用测试系统架构。
该构架通过信号特征遮掩、生成对抗网络(GAN)、欺骗信号汇集、信道模拟等方法构造仿真测试样本,并把测试样本与原样本数据导入深度模型进行识别结果对比测试。
基于测试结果分析了深度模型聚焦的信号关键特征位置,分析模型的鲁棒性,揭示信道环境对识别性能的影响,从而解释了深度学习网络模型的性能。
关键词:辐射源个体识别;可解释性;生成对抗网络;无线信号欺骗中图分类号:TN92 文献标志码:A doi:10.11805/TKYDA2022243An interpretable testing architecture for specificemitter identificationLIU Wenbin a,FAN Pingzhi a,LI Yukai b,WANG Yuhao b,MENG Hua*b(a.School of Information Science & Technology; b.School of Mathematics, Southwest Jiaotong University, Chengdu Sichuan 611756, China)AbstractAbstract::Due to the diversity of RF signals, the complexity of the electromagnetic environment, and the difficulty of feature extraction, the robustness and applicability of the existing artificial features-based RF-specific emitter identification methods cannot meet the application requirements. Althoughthe data-driven deep learning methods can provide a more flexible mode of specific emitteridentification, they are less interpretable and lack a general test mode to evaluate their advantages anddisadvantages. An evaluation method is explored for the deep learning model on the target individualdataset of the Electromagnetic Big Data Super Contest, and a general testing system architecture isproposed for the specific emitter identification model based on deep neural networks. The frameworkconstructs the simulation test samples through signal feature masking, Generative Adversarial Network(GAN), deception signal collection, channel simulation and other methods, and imports the test samplesand original data into the deep model to compare the recognition results. The test results are employed tojudge the location of the signal key features extracted by the deep model, to analyze the robustness of themodel, and to reveal the impact of the channel environment on the recognition performance, thus theperformance of the deep learning model can be interpretable.KeywordsKeywords::specific emitter identification;interpretability;Generative Adversarial Network(GAN);wireless signal spoofing信号识别包括信号检测、信号类型识别、辐射源个体识别(Specific Emitter Identification,SEI)3个层次[1]。
Rietveld方法原理.ppt
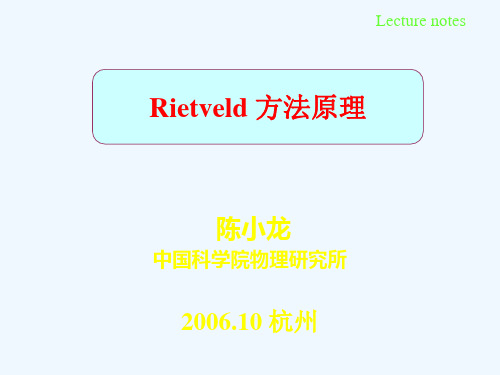
Structural model
Raw data
shift
Rietveld Refinement
Refined model
How RM works?
The RM refines a structure by minimizing a quantity through the Newton-Raphson algorithm
0
1
2
3
4
value of x
4000
3900
3800
3700
3600
3500
3400
0
1 value o2f x
3
4
Phase transition of BaTiO3 from
tetragonal to cubic at about 132˚C
YBa3B3O9: Phase transition and structure determination
Grain size
Atomic Occupancy Incommensurate Structure
Debye Temperatures Structure factors
Crystallinity
Phase transitions
Magnetic structures
……
History Review
• Rietveld originally introduced the Profile Refinement method (Using stepscanned data rather than integrated Powder peak intensity) (1966,1967)
StochasticProcessesRossSolutionsManual-…

Stochastic Processes Ross Solutions ManualIf looking for the book Stochastic processes ross solutions manual in pdf format, then you have come on to the rightsite. We present utter variation of this book in DjVu, txt, PDF, ePub, doc forms. You may reading Robinair model34134z repair manual online stochastic-processes-ross-solutions-manual.pdf or download. Additionally, on our websiteyou may read the guides and another art books online, or downloading their. We wish to invite attention that oursite does not store the eBook itself, but we give ref to site wherever you may download either read online. So ifyou have must to download pdf Stochastic processes ross solutions manual stochastic-processes-ross-solutions-manual.pdf,then you have come on to loyal website. We have Stochastic processes ross solutions manual doc, DjVu, ePub, txt,PDF formats. We will be glad if you go back us afresh.stochastic processes solutions manual to - Get this from a library! Stochastic processes Solutions manual to accompany stochastic processes. [Sheldon Mark Ross]ross stochastic processes solution manual - Description Date Speed Downloads; PROBABILITY AND STOCHASTIC PROCESSES solution manual pdf Download by raj0386sheldon ross stochastic processes solution manual - sheldon ross stochastic processes solution manual at - Download free pdf files,ebooks and documents of sheldon ross stochastic processes solution manualsolutions to sheldon ross stochastic processes | - Sheldon M. Ross, Stochastic Processes, John Wiley and Sons.3. S A First Course in Probability, 8th Edition, Ross, Solutions Manual. A First Course ininstructor solutions manual pdf download ~ - Stochastic processes ross solution manual, instructor's manual to accompany. introduction to. probability models. tenth edition. sheldon m. ross. university ofstochastic processes: an introduction, second - Stochastic Processes: An Introduction, Second Edition Solutions manual available for qualifying instructors . Stochastic Processes in Science,roy d. yates solutions manual probability and - Probability and Stochastic Processes Solutions Manual to accompany Corporate Finance By Stephen A. Ross 6 edition Solutions Manual to accompany Investmentessentials of stochastic processes solution manual - Essentials Of Stochastic Processes Solution Manual Pdf books, ebooks, manuals and documents at EDU Libs. Stochastic Processes Ross Solutions Manual.pdffundamentals of probability with stochastic - Fundamentals of Probability With stochastic processes 3/e (Solutions Manual ) By Saeed Ghahramani A First Course In Probability Solution Manual,Ross 6thsheldon ross, stochastic processes 2nd ed - Stochastic Processes (9780471120629): Sheldon M. Ross: the exercises should come with complete worked solutions. Read more Published on March 9,(2011) sheldon m ross stochastic process 2nd - (2011) Sheldon M Ross Stochastic Process 2nd Edition Solution Manual > /kmp7hp8introduction to stochastic processes - - Sheldon M. Ross. Stochastic processes. Very good book on stochastic processes, An Introduction to the Numerical Solution of Markov Chains.ross stochastic processes solution manual | - Download links for Ross Stochastic Process Solution .doc MSWord Document. Download links for Solution Manual Stochastic Processes Sheldon Ross .doc MSWord Document.ross solution manual stochastic processes - foursquare gospel bible lessons jeep grand cherokee wire context clues module gateway m360 manuals carrier how to get superheat pltw engineer crossword 1.1 amswersprobability - stochastic processes solution - Stochastic Processes Solution manuals. stash of solution manuals for stochastic processes Models by Sheldon M. Ross. It has the 10 edition solution manualstochastic processes ross solution manual - - Document/File: , filesize: n/a. Filetype: PDF. A Poisson process is a simple and widely used stochastic process for modelingstochastic processes solution manual - manuals - Stochastic processes solution manual. (Solution Manual) Sheldon Ross Probability and Stochastic Processes Solutions Manual 255 Problem 9.4.4 Thesesolutions manual _ probability, random variables and - Solutions Manual _ Probability, Random Variables and Stochastic Processes Solutions - Papoulis.2002 - Free ebook download as PDF File (.pdf), Text file solutions manual to accompany stochastic - Get this from a library! Solutions manual to accompany Stochastic processes. [Sheldon M Ross]probability and statistics ser.: stochastic - Probability and Statistics Ser.: Stochastic Processes : Solutions Manual by Sheldon M. Ross (1983, Paperback, Teacher's Edition of Textbook) (Paperback, 1983)probability stochastic processes solution manual | - Stochastic Process Ross Solution Manual Stochastic Processes Ross Solutions Manual | Probability And Stochastic Processes Solutions Manual rapidshare Related PDFs:principles of accounting 101 reeves solutions manual, 1995 polaris sportsman 400 service manual, peugeot 207 manual english, case ih rbx 562 operators manual, jsc lecture guide, carrier ac wall remote control manual model 68rv1112a, generac 15000 generator manual, cub cadet 1220 hydro manual, modern biology study guide answers chapter17, entrepreneurship hisrich 8th edition study guide, study guide for electrician apprentice eti, haas vf oe manual, 1996 yamaha 1300cc royal star manual, dibal l series service manual, 2015 vw jetta sportswagen tdi repair manual, mariner 15 hk 2015 manual, gilera runner 50 poggiali manual, pyxis guide console, fanuc powermate parameter manual, quiznos sandwich make guide, daisy 4500 manual, kenworth parts manual k100,p90x owners manual, yamaha it 200 service manual, quantitative chemical analysis 7th edition solution manual, kasea mighty mite 50 service manual, tennessee kindergarten pacing guide, ford ranger gearbox repair manual, 1987 1997 kawasaki zx600 750 service repair manual download, massey ferguson 699 service manual, wood badge training manual, troy bilt lawn mower repair manuals, kubota b7100 hst manual, maths golden guide of class 9 ncert, manual for 4360 ford diesel tractor, 1998 mercedes ml320 owners manual, nissan 15 electric forklift service manual, mechanical engineering design solutions manual 9th edition, carrier 58pav070 12 manual, weed eater ge21 repair manual。
Instructional_design
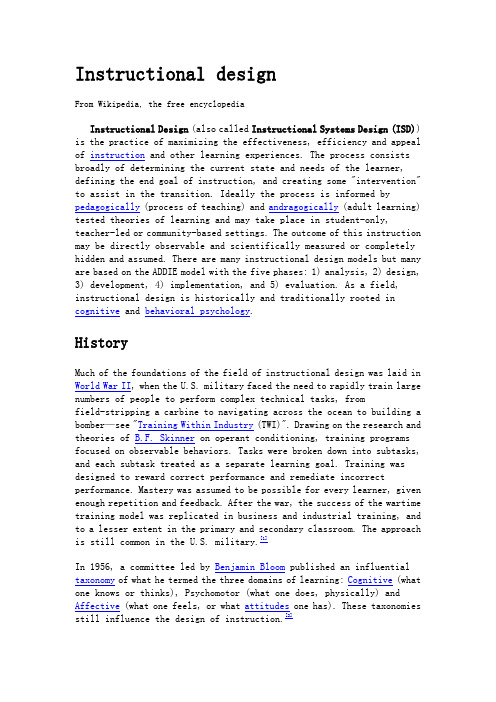
Instructional designFrom Wikipedia, the free encyclopediaInstructional Design(also called Instructional Systems Design (ISD)) is the practice of maximizing the effectiveness, efficiency and appeal of instruction and other learning experiences. The process consists broadly of determining the current state and needs of the learner, defining the end goal of instruction, and creating some "intervention" to assist in the transition. Ideally the process is informed by pedagogically(process of teaching) and andragogically(adult learning) tested theories of learning and may take place in student-only, teacher-led or community-based settings. The outcome of this instruction may be directly observable and scientifically measured or completely hidden and assumed. There are many instructional design models but many are based on the ADDIE model with the five phases: 1) analysis, 2) design, 3) development, 4) implementation, and 5) evaluation. As a field, instructional design is historically and traditionally rooted in cognitive and behavioral psychology.HistoryMuch of the foundations of the field of instructional design was laid in World War II, when the U.S. military faced the need to rapidly train large numbers of people to perform complex technical tasks, fromfield-stripping a carbine to navigating across the ocean to building a bomber—see "Training Within Industry(TWI)". Drawing on the research and theories of B.F. Skinner on operant conditioning, training programs focused on observable behaviors. Tasks were broken down into subtasks, and each subtask treated as a separate learning goal. Training was designed to reward correct performance and remediate incorrect performance. Mastery was assumed to be possible for every learner, given enough repetition and feedback. After the war, the success of the wartime training model was replicated in business and industrial training, and to a lesser extent in the primary and secondary classroom. The approach is still common in the U.S. military.[1]In 1956, a committee led by Benjamin Bloom published an influential taxonomy of what he termed the three domains of learning: Cognitive(what one knows or thinks), Psychomotor (what one does, physically) and Affective (what one feels, or what attitudes one has). These taxonomies still influence the design of instruction.[2]During the latter half of the 20th century, learning theories began to be influenced by the growth of digital computers.In the 1970s, many instructional design theorists began to adopt an information-processing-based approach to the design of instruction. David Merrill for instance developed Component Display Theory (CDT), which concentrates on the means of presenting instructional materials (presentation techniques).[3]Later in the 1980s and throughout the 1990s cognitive load theory began to find empirical support for a variety of presentation techniques.[4]Cognitive load theory and the design of instructionCognitive load theory developed out of several empirical studies of learners, as they interacted with instructional materials.[5]Sweller and his associates began to measure the effects of working memory load, and found that the format of instructional materials has a direct effect on the performance of the learners using those materials.[6][7][8]While the media debates of the 1990s focused on the influences of media on learning, cognitive load effects were being documented in several journals. Rather than attempting to substantiate the use of media, these cognitive load learning effects provided an empirical basis for the use of instructional strategies. Mayer asked the instructional design community to reassess the media debate, to refocus their attention on what was most important: learning.[9]By the mid- to late-1990s, Sweller and his associates had discovered several learning effects related to cognitive load and the design of instruction (e.g. the split attention effect, redundancy effect, and the worked-example effect). Later, other researchers like Richard Mayer began to attribute learning effects to cognitive load.[9] Mayer and his associates soon developed a Cognitive Theory of MultimediaLearning.[10][11][12]In the past decade, cognitive load theory has begun to be internationally accepted[13]and begun to revolutionize how practitioners of instructional design view instruction. Recently, human performance experts have even taken notice of cognitive load theory, and have begun to promote this theory base as the science of instruction, with instructional designers as the practitioners of this field.[14]Finally Clark, Nguyen and Sweller[15]published a textbook describing how Instructional Designers can promote efficient learning using evidence-based guidelines of cognitive load theory.Instructional Designers use various instructional strategies to reduce cognitive load. For example, they think that the onscreen text should not be more than 150 words or the text should be presented in small meaningful chunks.[citation needed] The designers also use auditory and visual methods to communicate information to the learner.Learning designThe concept of learning design arrived in the literature of technology for education in the late nineties and early 2000s [16] with the idea that "designers and instructors need to choose for themselves the best mixture of behaviourist and constructivist learning experiences for their online courses" [17]. But the concept of learning design is probably as old as the concept of teaching. Learning design might be defined as "the description of the teaching-learning process that takes place in a unit of learning (eg, a course, a lesson or any other designed learning event)" [18].As summarized by Britain[19], learning design may be associated with:∙The concept of learning design∙The implementation of the concept made by learning design specifications like PALO, IMS Learning Design[20], LDL, SLD 2.0, etc... ∙The technical realisations around the implementation of the concept like TELOS, RELOAD LD-Author, etc...Instructional design modelsADDIE processPerhaps the most common model used for creating instructional materials is the ADDIE Process. This acronym stands for the 5 phases contained in the model:∙Analyze– analyze learner characteristics, task to be learned, etc.Identify Instructional Goals, Conduct Instructional Analysis, Analyze Learners and Contexts∙Design– develop learning objectives, choose an instructional approachWrite Performance Objectives, Develop Assessment Instruments, Develop Instructional Strategy∙Develop– create instructional or training materialsDesign and selection of materials appropriate for learning activity, Design and Conduct Formative Evaluation∙Implement– deliver or distribute the instructional materials ∙Evaluate– make sure the materials achieved the desired goals Design and Conduct Summative EvaluationMost of the current instructional design models are variations of the ADDIE process.[21] Dick,W.O,.Carey, L.,&Carey, J.O.(2004)Systematic Design of Instruction. Boston,MA:Allyn&Bacon.Rapid prototypingA sometimes utilized adaptation to the ADDIE model is in a practice known as rapid prototyping.Proponents suggest that through an iterative process the verification of the design documents saves time and money by catching problems while they are still easy to fix. This approach is not novel to the design of instruction, but appears in many design-related domains including software design, architecture, transportation planning, product development, message design, user experience design, etc.[21][22][23]In fact, some proponents of design prototyping assert that a sophisticated understanding of a problem is incomplete without creating and evaluating some type of prototype, regardless of the analysis rigor that may have been applied up front.[24] In other words, up-front analysis is rarely sufficient to allow one to confidently select an instructional model. For this reason many traditional methods of instructional design are beginning to be seen as incomplete, naive, and even counter-productive.[25]However, some consider rapid prototyping to be a somewhat simplistic type of model. As this argument goes, at the heart of Instructional Design is the analysis phase. After you thoroughly conduct the analysis—you can then choose a model based on your findings. That is the area where mostpeople get snagged—they simply do not do a thorough-enough analysis. (Part of Article By Chris Bressi on LinkedIn)Dick and CareyAnother well-known instructional design model is The Dick and Carey Systems Approach Model.[26] The model was originally published in 1978 by Walter Dick and Lou Carey in their book entitled The Systematic Design of Instruction.Dick and Carey made a significant contribution to the instructional design field by championing a systems view of instruction as opposed to viewing instruction as a sum of isolated parts. The model addresses instruction as an entire system, focusing on the interrelationship between context, content, learning and instruction. According to Dick and Carey, "Components such as the instructor, learners, materials, instructional activities, delivery system, and learning and performance environments interact with each other and work together to bring about the desired student learning outcomes".[26] The components of the Systems Approach Model, also known as the Dick and Carey Model, are as follows:∙Identify Instructional Goal(s): goal statement describes a skill, knowledge or attitude(SKA) that a learner will be expected to acquire ∙Conduct Instructional Analysis: Identify what a learner must recall and identify what learner must be able to do to perform particular task ∙Analyze Learners and Contexts: General characteristic of the target audience, Characteristic directly related to the skill to be taught, Analysis of Performance Setting, Analysis of Learning Setting∙Write Performance Objectives: Objectives consists of a description of the behavior, the condition and criteria. The component of anobjective that describes the criteria that will be used to judge the learner's performance.∙Develop Assessment Instruments: Purpose of entry behavior testing, purpose of pretesting, purpose of posttesting, purpose of practive items/practive problems∙Develop Instructional Strategy: Pre-instructional activities, content presentation, Learner participation, assessment∙Develop and Select Instructional Materials∙Design and Conduct Formative Evaluation of Instruction: Designer try to identify areas of the instructional materials that are in need to improvement.∙Revise Instruction: To identify poor test items and to identify poor instruction∙Design and Conduct Summative EvaluationWith this model, components are executed iteratively and in parallel rather than linearly.[26]/akteacher/dick-cary-instructional-design-mo delInstructional Development Learning System (IDLS)Another instructional design model is the Instructional Development Learning System (IDLS).[27] The model was originally published in 1970 by Peter J. Esseff, PhD and Mary Sullivan Esseff, PhD in their book entitled IDLS—Pro Trainer 1: How to Design, Develop, and Validate Instructional Materials.[28]Peter (1968) & Mary (1972) Esseff both received their doctorates in Educational Technology from the Catholic University of America under the mentorship of Dr. Gabriel Ofiesh, a Founding Father of the Military Model mentioned above. Esseff and Esseff contributed synthesized existing theories to develop their approach to systematic design, "Instructional Development Learning System" (IDLS).The components of the IDLS Model are:∙Design a Task Analysis∙Develop Criterion Tests and Performance Measures∙Develop Interactive Instructional Materials∙Validate the Interactive Instructional MaterialsOther modelsSome other useful models of instructional design include: the Smith/Ragan Model, the Morrison/Ross/Kemp Model and the OAR model , as well as, Wiggins theory of backward design .Learning theories also play an important role in the design ofinstructional materials. Theories such as behaviorism , constructivism , social learning and cognitivism help shape and define the outcome of instructional materials.Influential researchers and theoristsThe lists in this article may contain items that are not notable , not encyclopedic , or not helpful . Please help out by removing such elements and incorporating appropriate items into the main body of the article. (December 2010)Alphabetic by last name∙ Bloom, Benjamin – Taxonomies of the cognitive, affective, and psychomotor domains – 1955 ∙Bonk, Curtis – Blended learning – 2000s ∙ Bransford, John D. – How People Learn: Bridging Research and Practice – 1999 ∙ Bruner, Jerome – Constructivism ∙Carr-Chellman, Alison – Instructional Design for Teachers ID4T -2010 ∙Carey, L. – "The Systematic Design of Instruction" ∙Clark, Richard – Clark-Kosma "Media vs Methods debate", "Guidance" debate . ∙Clark, Ruth – Efficiency in Learning: Evidence-Based Guidelines to Manage Cognitive Load / Guided Instruction / Cognitive Load Theory ∙Dick, W. – "The Systematic Design of Instruction" ∙ Gagné, Robert M. – Nine Events of Instruction (Gagné and Merrill Video Seminar) ∙Heinich, Robert – Instructional Media and the new technologies of instruction 3rd ed. – Educational Technology – 1989 ∙Jonassen, David – problem-solving strategies – 1990s ∙Langdon, Danny G - The Instructional Designs Library: 40 Instructional Designs, Educational Tech. Publications ∙Mager, Robert F. – ABCD model for instructional objectives – 1962 ∙Merrill, M. David - Component Display Theory / Knowledge Objects ∙ Papert, Seymour – Constructionism, LOGO – 1970s ∙ Piaget, Jean – Cognitive development – 1960s∙Piskurich, George – Rapid Instructional Design – 2006∙Simonson, Michael –Instructional Systems and Design via Distance Education – 1980s∙Schank, Roger– Constructivist simulations – 1990s∙Sweller, John - Cognitive load, Worked-example effect, Split-attention effect∙Roberts, Clifton Lee - From Analysis to Design, Practical Applications of ADDIE within the Enterprise - 2011∙Reigeluth, Charles –Elaboration Theory, "Green Books" I, II, and III - 1999-2010∙Skinner, B.F.– Radical Behaviorism, Programed Instruction∙Vygotsky, Lev– Learning as a social activity – 1930s∙Wiley, David– Learning Objects, Open Learning – 2000sSee alsoSince instructional design deals with creating useful instruction and instructional materials, there are many other areas that are related to the field of instructional design.∙educational assessment∙confidence-based learning∙educational animation∙educational psychology∙educational technology∙e-learning∙electronic portfolio∙evaluation∙human–computer interaction∙instructional design context∙instructional technology∙instructional theory∙interaction design∙learning object∙learning science∙m-learning∙multimedia learning∙online education∙instructional design coordinator∙storyboarding∙training∙interdisciplinary teaching∙rapid prototyping∙lesson study∙Understanding by DesignReferences1.^MIL-HDBK-29612/2A Instructional Systems Development/SystemsApproach to Training and Education2.^Bloom's Taxonomy3.^TIP: Theories4.^Lawrence Erlbaum Associates, Inc. - Educational Psychologist -38(1):1 - Citation5.^ Sweller, J. (1988). "Cognitive load during problem solving:Effects on learning". Cognitive Science12 (1): 257–285.doi:10.1016/0364-0213(88)90023-7.6.^ Chandler, P. & Sweller, J. (1991). "Cognitive Load Theory andthe Format of Instruction". Cognition and Instruction8 (4): 293–332.doi:10.1207/s1532690xci0804_2.7.^ Sweller, J., & Cooper, G.A. (1985). "The use of worked examplesas a substitute for problem solving in learning algebra". Cognition and Instruction2 (1): 59–89. doi:10.1207/s1532690xci0201_3.8.^Cooper, G., & Sweller, J. (1987). "Effects of schema acquisitionand rule automation on mathematical problem-solving transfer". Journal of Educational Psychology79 (4): 347–362.doi:10.1037/0022-0663.79.4.347.9.^ a b Mayer, R.E. (1997). "Multimedia Learning: Are We Asking theRight Questions?". Educational Psychologist32 (41): 1–19.doi:10.1207/s1*******ep3201_1.10.^ Mayer, R.E. (2001). Multimedia Learning. Cambridge: CambridgeUniversity Press. ISBN0-521-78239-2.11.^Mayer, R.E., Bove, W. Bryman, A. Mars, R. & Tapangco, L. (1996)."When Less Is More: Meaningful Learning From Visual and Verbal Summaries of Science Textbook Lessons". Journal of Educational Psychology88 (1): 64–73. doi:10.1037/0022-0663.88.1.64.12.^ Mayer, R.E., Steinhoff, K., Bower, G. and Mars, R. (1995). "Agenerative theory of textbook design: Using annotated illustrations to foster meaningful learning of science text". Educational TechnologyResearch and Development43 (1): 31–41. doi:10.1007/BF02300480.13.^Paas, F., Renkl, A. & Sweller, J. (2004). "Cognitive Load Theory:Instructional Implications of the Interaction between InformationStructures and Cognitive Architecture". Instructional Science32: 1–8.doi:10.1023/B:TRUC.0000021806.17516.d0.14.^ Clark, R.C., Mayer, R.E. (2002). e-Learning and the Science ofInstruction: Proven Guidelines for Consumers and Designers of Multimedia Learning. San Francisco: Pfeiffer. ISBN0-7879-6051-9.15.^ Clark, R.C., Nguyen, F., and Sweller, J. (2006). Efficiency inLearning: Evidence-Based Guidelines to Manage Cognitive Load. SanFrancisco: Pfeiffer. ISBN0-7879-7728-4.16.^Conole G., and Fill K., “A learning design toolkit to createpedagogically effective learning activities”. Journal of Interactive Media in Education, 2005 (08).17.^Carr-Chellman A. and Duchastel P., “The ideal online course,”British Journal of Educational Technology, 31(3), 229-241, July 2000.18.^Koper R., “Current Research in Learning Design,” EducationalTechnology & Society, 9 (1), 13-22, 2006.19.^Britain S., “A Review of Learning Design: Concept,Specifications and Tools” A report for the JISC E-learning Pedagogy Programme, May 2004.20.^IMS Learning Design webpage21.^ a b Piskurich, G.M. (2006). Rapid Instructional Design: LearningID fast and right.22.^ Saettler, P. (1990). The evolution of American educationaltechnology.23.^ Stolovitch, H.D., & Keeps, E. (1999). Handbook of humanperformance technology.24.^ Kelley, T., & Littman, J. (2005). The ten faces of innovation:IDEO's strategies for beating the devil's advocate & driving creativity throughout your organization. New York: Doubleday.25.^ Hokanson, B., & Miller, C. (2009). Role-based design: Acontemporary framework for innovation and creativity in instructional design. Educational Technology, 49(2), 21–28.26.^ a b c Dick, Walter, Lou Carey, and James O. Carey (2005) [1978].The Systematic Design of Instruction(6th ed.). Allyn & Bacon. pp. 1–12.ISBN020*******./?id=sYQCAAAACAAJ&dq=the+systematic+design+of+instruction.27.^ Esseff, Peter J. and Esseff, Mary Sullivan (1998) [1970].Instructional Development Learning System (IDLS) (8th ed.). ESF Press.pp. 1–12. ISBN1582830371. /Materials.html.28.^/Materials.htmlExternal links∙Instructional Design - An overview of Instructional Design∙ISD Handbook∙Edutech wiki: Instructional design model [1]∙Debby Kalk, Real World Instructional Design InterviewRetrieved from "/wiki/Instructional_design" Categories: Educational technology | Educational psychology | Learning | Pedagogy | Communication design | Curricula。
rdmulti 1.1 软件包用户指南:分析 RD 设计中的多个阈值或评分说明书
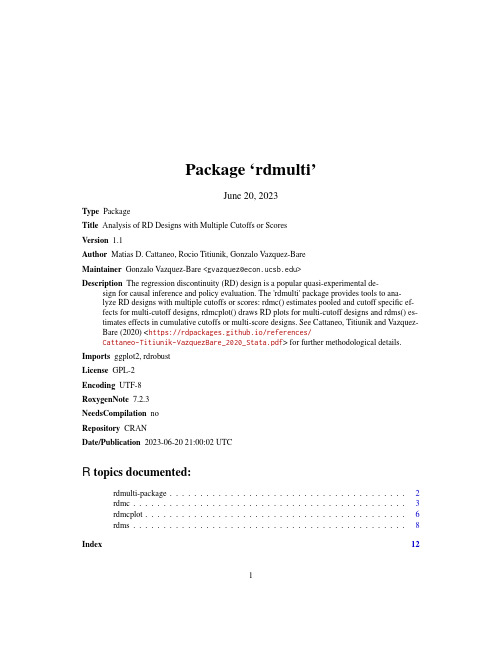
Package‘rdmulti’June20,2023Type PackageTitle Analysis of RD Designs with Multiple Cutoffs or ScoresVersion1.1Author Matias D.Cattaneo,Rocio Titiunik,Gonzalo Vazquez-BareMaintainer Gonzalo Vazquez-Bare<******************.edu>Description The regression discontinuity(RD)design is a popular quasi-experimental de-sign for causal inference and policy evaluation.The'rdmulti'package provides tools to ana-lyze RD designs with multiple cutoffs or scores:rdmc()estimates pooled and cutoff specific ef-fects for multi-cutoff designs,rdmcplot()draws RD plots for multi-cutoff designs and rdms()es-timates effects in cumulative cutoffs or multi-score designs.See Cattaneo,Titiunik and Vazquez-Bare(2020)<https://rdpackages.github.io/references/Cattaneo-Titiunik-VazquezBare_2020_Stata.pdf>for further methodological details. Imports ggplot2,rdrobustLicense GPL-2Encoding UTF-8RoxygenNote7.2.3NeedsCompilation noRepository CRANDate/Publication2023-06-2021:00:02UTCR topics documented:rdmulti-package (2)rdmc (3)rdmcplot (6)rdms (8)Index1212rdmulti-package rdmulti-package rdmulti:analysis of RD Designs with multiple cutoffs or scoresDescriptionThe regression discontinuity(RD)design is a popular quasi-experimental design for causal infer-ence and policy evaluation.The rdmulti package provides tools to analyze RD designs with multiple cutoffs or scores:rdmc()estimates pooled and cutoff-specific effects in multi-cutoff de-signs,rdmcplot()draws RD plots for multi-cutoff RD designs and rdms()estimates effects in cumulative cutoffs or multi-score designs.For more details,and related Stata and R packages useful for analysis of RD designs,visit https://rdpackages.github.io/.Author(s)Matias Cattaneo,Princeton University.<**********************>Rocio Titiunik,Princeton University.<**********************>Gonzalo Vazquez-Bare,UC Santa Barbara.<******************.edu>ReferencesCalonico,S.,M.D.Cattaneo,M.Farrell and R.Titiunik.(2017).rdrobust:Software for Regres-sion Discontinuity Designs.Stata Journal17(2):372-404.Calonico,S.,M.D.Cattaneo,and R.Titiunik.(2014).Robust Data-Driven Inference in the Regression-Discontinuity Design.Stata Journal14(4):909-946.Calonico,S.,M.D.Cattaneo,and R.Titiunik.(2015).rdrobust:An R Package for Robust Non-parametric Inference in Regression-Discontinuity Designs.R Journal7(1):38-51.Cattaneo,M.D.,L.Keele,R.Titiunik and G.Vazquez-Bare.(2016).Interpreting Regression Dis-continuity Designs with Multiple Cutoffs.Journal of Politics78(4):1229-1248.Cattaneo,M.D.,L.Keele,R.Titiunik and G.Vazquez-Bare.(2020).Extrapolating Treatment Effects in Multi-Cutoff Regression Discontinuity Designs.Journal of the American Statistical As-sociation116(536):1941,1952.Cattaneo,M.D.,R.Titiunik and G.Vazquez-Bare.(2020).Analysis of Regression Discontinuity Designs with Multiple Cutoffs or Multiple Scores.Stata Journal20(4):866-891.Keele,L.and R.Titiunik.(2015).Geographic Boundaries as Regression Discontinuities.Political Analysis23(1):127-155rdmc3 rdmc Analysis of RD designs with multiple cutoffsDescriptionrdmc()analyzes RD designs with multiple cutoffs.Usagerdmc(Y,X,C,fuzzy=NULL,derivvec=NULL,pooled_opt=NULL,verbose=FALSE,pvec=NULL,qvec=NULL,hmat=NULL,bmat=NULL,rhovec=NULL,covs_mat=NULL,covs_list=NULL,covs_dropvec=NULL,kernelvec=NULL,weightsvec=NULL,bwselectvec=NULL,scaleparvec=NULL,scaleregulvec=NULL,masspointsvec=NULL,bwcheckvec=NULL,bwrestrictvec=NULL,stdvarsvec=NULL,vcevec=NULL,nnmatchvec=NULL,cluster=NULL,level=95,plot=FALSE,conventional=FALSE)ArgumentsY outcome variable.X running variable.4rdmcC cutoff variable.fuzzy specifies a fuzzy design.See rdrobust()for details.derivvec vector of cutoff-specific order of derivatives.See rdrobust()for details.pooled_opt options to be passed to rdrobust()to calculate pooled estimand.verbose displays the output from rdrobust for estimating the pooled estimand.pvec vector of cutoff-specific polynomial orders.See rdrobust()for details.qvec vector of cutoff-specific polynomial orders for bias estimation.See rdrobust()for details.hmat matrix of cutoff-specific bandwidths.See rdrobust()for details.bmat matrix of cutoff-specific bandwidths for bias estimation.See rdrobust()fordetails.rhovec vector of cutoff-specific values of rho.See rdrobust()for details.covs_mat matrix of covariates.See rdrobust()for details.covs_list list of covariates to be used in each cutoff.covs_dropvec vector indicating whether collinear covariates should be dropped at each cutoff.See rdrobust()for details.kernelvec vector of cutoff-specific kernels.See rdrobust()for details.weightsvec vector of length equal to the number of cutoffs indicating the names of the vari-ables to be used as weights in each cutoff.See rdrobust()for details.bwselectvec vector of cutoff-specific bandwidth selection methods.See rdrobust()for de-tails.scaleparvec vector of cutoff-specific scale parameters.See rdrobust()for details.scaleregulvec vector of cutoff-specific scale regularization parameters.See rdrobust()fordetails.masspointsvec vector indicating how to handle repeated values at each cutoff.See rdrobust()for details.bwcheckvec vector indicating the value of bwcheck at each cutoff.See rdrobust()for de-tails.bwrestrictvec vector indicating whether computed bandwidths are restricted to the range orrunvar at each cutoff.See rdrobust()for details.stdvarsvec vector indicating whether variables are standardized at each cutoff.See rdrobust() for details.vcevec vector of cutoff-specific variance-covariance estimation methods.See rdrobust() for details.nnmatchvec vector of cutoff-specific nearest neighbors for variance estimation.See rdrobust() for details.cluster cluster ID variable.See rdrobust()for details.level confidence level for confidence intervals.See rdrobust()for details.plot plots cutoff-specific estimates and weights.conventional reports conventional,instead of robust-bias corrected,p-values and confidenceintervals.rdmc5Valuetau pooled estimatese.rb robust bias corrected standard error for pooled estimatepv.rb robust bias corrected p-value for pooled estimateci.rb.l left limit of robust bias corrected CI for pooled estimateci.rb.r right limit of robust bias corrected CI for pooled estimatehl bandwidth to the left of the cutoff for pooled estimatehr bandwidth to the right of the cutofffor pooled estimateNhl sample size within bandwidth to the left of the cutoff for pooled estimateNhr sample size within bandwidth to the right of the cutoff for pooled estimateB vector of bias-corrected estimatesV vector of robust variances of the estimatesCoefs vector of conventional estimatesW vector of weights for each cutoff-specific estimateNh vector of sample sizes within bandwidthCI robust bias-corrected confidence intervalsH matrix of bandwidthsPv vector of robust p-valuesrdrobust.resultsresults from rdrobust for pooled estimatecfail Cutoffs where rdrobust()encountered problemsAuthor(s)Matias Cattaneo,Princeton University.<**********************>Rocio Titiunik,Princeton University.<**********************>Gonzalo Vazquez-Bare,UC Santa Barbara.<******************.edu>ReferencesCattaneo,M.D.,R.Titiunik and G.Vazquez-Bare.(2020).Analysis of Regression Discontinuity Designs with Multiple Cutoffs or Multiple Scores.Stata Journal,forthcoming.Examples#Toy datasetX<-runif(1000,0,100)C<-c(rep(33,500),rep(66,500))Y<-(1+X+(X>=C))*(C==33)+(.5+.5*X+.8*(X>=C))*(C==66)+rnorm(1000)#rdmc with standard syntaxtmp<-rdmc(Y,X,C)rdmcplot RD plots with multiple cutoffs.Descriptionrdmcplot()RD plots with multiple cutoffs.Usagerdmcplot(Y,X,C,nbinsmat=NULL,binselectvec=NULL,scalevec=NULL,supportmat=NULL,pvec=NULL,hmat=NULL,kernelvec=NULL,weightsvec=NULL,covs_mat=NULL,covs_list=NULL,covs_evalvec=NULL,covs_dropvec=NULL,ci=NULL,col_bins=NULL,pch_bins=NULL,col_poly=NULL,lty_poly=NULL,col_xline=NULL,lty_xline=NULL,nobins=FALSE,nopoly=FALSE,noxline=FALSE,nodraw=FALSE)ArgumentsY outcome variable.X running variable.C cutoff variable.nbinsmat matrix of cutoff-specific number of bins.See rdplot()for details.binselectvec vector of cutoff-specific bins selection method.See rdplot()for details.scalevec vector of cutoff-specific scale factors.See rdplot()for details.supportmat matrix of cutoff-specific support conditions.See rdplot()for details..pvec vector of cutoff-specific polynomial orders.See rdplot()for details.hmat matrix of cutoff-specific bandwidths.See rdplot()for details.kernelvec vector of cutoff-specific kernels.See rdplot()for details.weightsvec vector of cutoff-specific weights.See rdplot()for details.covs_mat matrix of covariates.See rdplot()for details.covs_list list of of covariates to be used in each cutoff.covs_evalvec vector indicating the evaluation point for additional covariates.See rdrobust() for details.covs_dropvec vector indicating whether collinear covariates should be dropped at each cutoff.See rdrobust()for details.ci adds confidence intervals of the specified level to the plot.See rdrobust()for details.col_bins vector of colors for bins.pch_bins vector of characters(pch)type for bins.col_poly vector of colors for polynomial curves.lty_poly vector of lty for polynomial curves.col_xline vector of colors for vertical lines.lty_xline vector of lty for vertical lines.nobins omits bins plot.nopoly omits polynomial curve plot.noxline omits vertical lines indicating the cutoffs.nodraw omits plot.Valueclist list of cutoffscnum number of cutoffsX0matrix of X values for control unitsX1matrix of X values for treated unitsYhat0estimated polynomial for control unitsYhat1estimated polynomial for treated unitsXmean bin average of X valuesYmean bin average for Y valuesCI_l lower end of confidence intervalsCI_r upper end of confidence intervalscfail Cutoffs where rdrobust()encountered problems8rdmsAuthor(s)Matias Cattaneo,Princeton University.<**********************>Rocio Titiunik,Princeton University.<**********************>Gonzalo Vazquez-Bare,UC Santa Barbara.<******************.edu>ReferencesCattaneo,M.D.,R.Titiunik and G.Vazquez-Bare.(2020).Analysis of Regression Discontinuity Designs with Multiple Cutoffs or Multiple Scores.Stata Journal,forthcoming.Examples#Toy datasetX<-runif(1000,0,100)C<-c(rep(33,500),rep(66,500))Y<-(1+X+(X>=C))*(C==33)+(.5+.5*X+.8*(X>=C))*(C==66)+rnorm(1000)#rdmcplot with standard syntaxtmp<-rdmcplot(Y,X,C)rdms Analysis of RD designs with cumulative cutoffs or two running vari-ablesDescriptionrdms()analyzes RD designs with cumulative cutoffs or two running variables.Usagerdms(Y,X,C,X2=NULL,zvar=NULL,C2=NULL,rangemat=NULL,xnorm=NULL,fuzzy=NULL,derivvec=NULL,pooled_opt=NULL,pvec=NULL,qvec=NULL,hmat=NULL,bmat=NULL,rdms9 rhovec=NULL,covs_mat=NULL,covs_list=NULL,covs_dropvec=NULL,kernelvec=NULL,weightsvec=NULL,bwselectvec=NULL,scaleparvec=NULL,scaleregulvec=NULL,masspointsvec=NULL,bwcheckvec=NULL,bwrestrictvec=NULL,stdvarsvec=NULL,vcevec=NULL,nnmatchvec=NULL,cluster=NULL,level=95,plot=FALSE,conventional=FALSE)ArgumentsY outcome variable.X running variable.C vector of cutoffs.X2if specified,second running variable.zvar if X2is specified,treatment indicator.C2if specified,second vector of cutoffs.rangemat matrix of cutoff-specific ranges for the running variable.xnorm normalized running variable to estimate pooled effect.fuzzy specifies a fuzzy design.See rdrobust()for details.derivvec vector of cutoff-specific order of derivatives.See rdrobust()for details.pooled_opt options to be passed to rdrobust()to calculate pooled estimand.pvec vector of cutoff-specific polynomial orders.See rdrobust()for details.qvec vector of cutoff-specific polynomial orders for bias estimation.See rdrobust() for details.hmat matrix of cutoff-specific bandwidths.See rdrobust()for details.bmat matrix of cutoff-specific bandwidths for bias estimation.See rdrobust()for details.rhovec vector of cutoff-specific values of rho.See rdrobust()for details.covs_mat matrix of covariates.See rdplot()for details.covs_list list of of covariates to be used in each cutoff.10rdms covs_dropvec vector indicating whether collinear covariates should be dropped at each cutoff.See rdrobust()for details.kernelvec vector of cutoff-specific kernels.See rdrobust()for details.weightsvec vector of length equal to the number of cutoffs indicating the names of the vari-ables to be used as weights in each cutoff.See rdrobust()for details.bwselectvec vector of cutoff-specific bandwidth selection methods.See rdrobust()for de-tails.scaleparvec vector of cutoff-specific scale parameters.See rdrobust()for details.scaleregulvec vector of cutoff-specific scale regularization parameters.See rdrobust()fordetails.masspointsvec vector indicating how to handle repeated values at each cutoff.See rdrobust()for details.bwcheckvec vector indicating the value of bwcheck at each cutoff.See rdrobust()for de-tails.bwrestrictvec vector indicating whether computed bandwidths are restricted to the range orrunvar at each cutoff.See rdrobust()for details.stdvarsvec vector indicating whether variables are standardized at each cutoff.See rdrobust() for details.vcevec vector of cutoff-specific variance-covariance estimation methods.See rdrobust() for details.nnmatchvec vector of cutoff-specific nearest neighbors for variance estimation.See rdrobust() for details.cluster cluster ID variable.See rdrobust()for details.level confidence level for confidence intervals.See rdrobust()for details.plot plots cutoff-specific and pooled estimates.conventional reports conventional,instead of robust-bias corrected,p-values and confidenceintervals.ValueB vector of bias-corrected coefficientsV variance-covariance matrix of the estimatorsCoefs vector of conventional coefficientsNh vector of sample sizes within bandwidth at each cutoffCI bias corrected confidence intervalsH bandwidth used at each cutoffPv vector of robust p-valuesAuthor(s)Matias Cattaneo,Princeton University.<**********************>Rocio Titiunik,Princeton University.<**********************>Gonzalo Vazquez-Bare,UC Santa Barbara.<******************.edu>rdms11ReferencesCattaneo,M.D.,R.Titiunik and G.Vazquez-Bare.(2020).Analysis of Regression Discontinuity Designs with Multiple Cutoffs or Multiple Scores.Stata Journal,forthcoming.Examples#Toy dataset:cumulative cutoffsX<-runif(1000,0,100)C<-c(33,66)Y<-(1+X)*(X<C[1])+(0.8+0.8*X)*(X>=C[1]&X<C[2])+(1.2+1.2*X)*(X>=C[2])+rnorm(1000) #rmds:basic syntaxtmp<-rdms(Y,X,C)Index_PACKAGE(rdmulti-package),2rdmc,2,3rdmcplot,2,6rdms,2,8rdmulti-package,2rdmulti_package(rdmulti-package),212。
基于数字TDI技术的紫外成像系统的设计
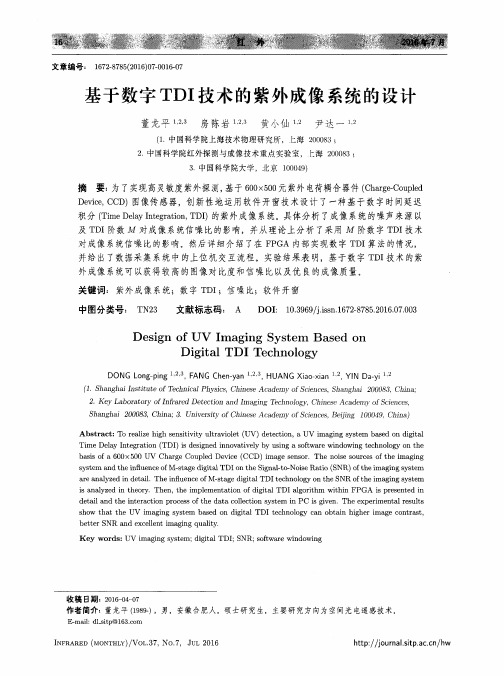
Abstract:To realize high sensitivity ultraviolet(UV)detection,a UV imaging system based on digital Time Delay Integration(TDI)is designed innovatively by using a sof tware windowing technology on the ba sis of a 6OO x 500 UV Charge Coupled Device(CCD)image sensor.The noise sources of the imaging system and the inf luence of M-stage digital TDI on the Signal-to-Noise Ratio(SNR)of the imaging system
3.中国科 学院 大学 ,北 京 100049)
摘 要:为 了实现高灵敏度紫外探测,基于 600 ̄500元紫外 电荷耦合器件 (Charge.Coupled Device,CCD)图像传感器,创新 性地 运用软件 开窗技 术设计 了一种基于数 字 时间延迟 积分 (Time Delay Integration,TDI)的紫外成像系统。具体分析 了成像 系统的噪声来源 以 及 TDI阶数 M 对成像 系统信噪 比的影响,并从理论上分析 了采用 M 阶数字 TDI技术 对成像 系统信 噪比的影响。然后详细介绍 了在 FPGA 内部实现数字 TDI算法 的情况, 并给 出了数据采集系 统中的上位机 交互流程。实验结果表 明,基于数字 TDI技术 的紫 外成像 系统可 以获得较高 的图像对 比度和信 噪 比以及优 良的成像质量。
水下光场的迭代求解
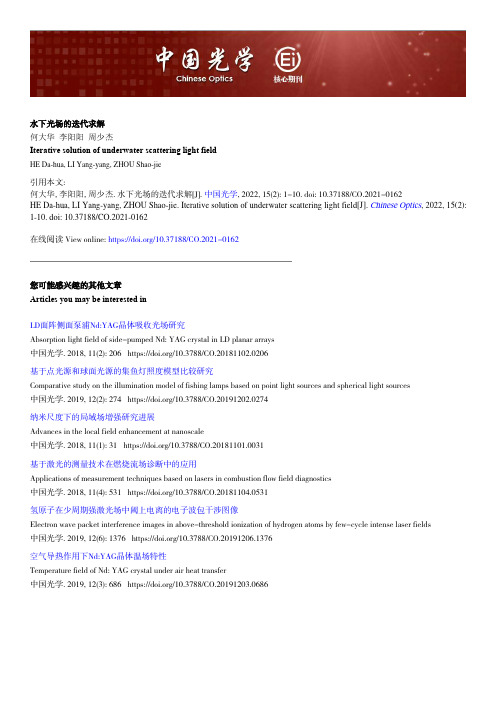
水下光场的迭代求解何大华 李阳阳 周少杰Iterative solution of underwater scattering light fieldHE Da-hua, LI Yang-yang, ZHOU Shao-jie引用本文:何大华,李阳阳,周少杰. 水下光场的迭代求解[J]. 中国光学, 2022, 15(2): 1-10. doi: 10.37188/CO.2021-0162HE Da-hua, LI Yang-yang, ZHOU Shao-jie. Iterative solution of underwater scattering light field[J]. Chinese Optics, 2022, 15(2): 1-10. doi: 10.37188/CO.2021-0162在线阅读 View online: https:///10.37188/CO.2021-0162您可能感兴趣的其他文章Articles you may be interested inLD面阵侧面泵浦Nd:YAG晶体吸收光场研究Absorption light field of side-pumped Nd: YAG crystal in LD planar arrays中国光学. 2018, 11(2): 206 https:///10.3788/CO.20181102.0206基于点光源和球面光源的集鱼灯照度模型比较研究Comparative study on the illumination model of fishing lamps based on point light sources and spherical light sources中国光学. 2019, 12(2): 274 https:///10.3788/CO.20191202.0274纳米尺度下的局域场增强研究进展Advances in the local field enhancement at nanoscale中国光学. 2018, 11(1): 31 https:///10.3788/CO.20181101.0031基于激光的测量技术在燃烧流场诊断中的应用Applications of measurement techniques based on lasers in combustion flow field diagnostics中国光学. 2018, 11(4): 531 https:///10.3788/CO.20181104.0531氢原子在少周期强激光场中阈上电离的电子波包干涉图像Electron wave packet interference images in above-threshold ionization of hydrogen atoms by few-cycle intense laser fields中国光学. 2019, 12(6): 1376 https:///10.3788/CO.20191206.1376空气导热作用下Nd:YAG晶体温场特性Temperature field of Nd: YAG crystal under air heat transfer中国光学. 2019, 12(3): 686 https:///10.3788/CO.20191203.0686文章编号 2095-1531(2022)02-0001-10水下光场的迭代求解何大华*,李阳阳,周少杰(华中光电技术研究所—武汉光电国家研究中心, 湖北 武汉 430223)摘要:水体对光线的散射是水下图像质量劣化的重要因素,为了定量分析在特定光源照射下水体散射的影响,建立了光线水下传输的散射模型,以此为基础推导出求解水下光场分布的Fredholm 积分方程。
一种基于深度学习的苦味肽精准预测方法
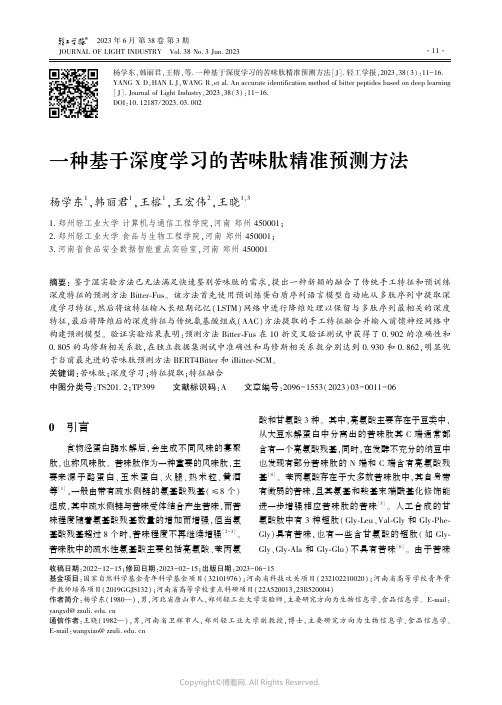
㊀㊀2023年6月第38卷第3期㊀㊀㊀㊀㊀㊀㊀㊀JOURNAL OF LIGHT INDUSTRY㊀Vol.38No.3Jun.2023㊀收稿日期:2022-12-15;修回日期:2023-02-15;出版日期:2023-06-15基金项目:国家自然科学基金青年科学基金项目(32101976);河南省科技攻关项目(232102210020);河南省高等学校青年骨干教师培养项目(2019GGJS132);河南省高等学校重点科研项目(22A520013,23B520004)作者简介:杨学东(1980 ),男,河北省唐山市人,郑州轻工业大学实验师,主要研究方向为生物信息学㊁食品信息学㊂E-mail :yangxd@通信作者:王晓(1982 ),男,河南省卫辉市人,郑州轻工业大学副教授,博士,主要研究方向为生物信息学㊁食品信息学㊂E-mail :wangxiao@.cn杨学东,韩丽君,王榕,等.一种基于深度学习的苦味肽精准预测方法[J].轻工学报,2023,38(3):11-16.YANG X D,HAN L J,WANG R,et al.An accurate identification method of bitter peptides based on deep learning [J].Journal of Light Industry,2023,38(3):11-16.DOI:10.12187/2023.03.002一种基于深度学习的苦味肽精准预测方法杨学东1,韩丽君1,王榕1,王宏伟2,王晓1,31.郑州轻工业大学计算机与通信工程学院,河南郑州450001;2.郑州轻工业大学食品与生物工程学院,河南郑州450001;3.河南省食品安全数据智能重点实验室,河南郑州450001摘要:鉴于湿实验方法已无法满足快速鉴别苦味肽的需求,提出一种新颖的融合了传统手工特征和预训练深度特征的预测方法Bitter-Fus ㊂该方法首先使用预训练蛋白质序列语言模型自动地从多肽序列中提取深度学习特征,然后将该特征输入长短期记忆(LSTM )网络中进行降维处理以保留与多肽序列最相关的深度特征,最后将降维后的深度特征与传统氨基酸组成(AAC )方法提取的手工特征融合并输入前馈神经网络中构建预测模型㊂验证实验结果表明:预测方法Bitter-Fus 在10折交叉验证测试中获得了0.902的准确性和0.805的马修斯相关系数,在独立数据集测试中准确性和马修斯相关系数分别达到0.930和0.862,明显优于当前最先进的苦味肽预测方法BERT4Bitter 和iBitter-SCM ㊂关键词:苦味肽;深度学习;特征提取;特征融合中图分类号:TS201.2;TP399㊀㊀文献标识码:A㊀㊀文章编号:2096-1553(2023)03-0011-060 引言食物经蛋白酶水解后,会生成不同风味的寡聚肽,也称风味肽㊂苦味肽作为一种重要的风味肽,主要来源于酪蛋白㊁玉米蛋白㊁火腿㊁热米粒㊁黄酒等[1],一般由带有疏水侧链的氨基酸残基(ɤ8个)组成,其中疏水侧链与苦味受体结合产生苦味,而苦味程度随着氨基酸残基数量的增加而增强,但当氨基酸残基超过8个时,苦味程度不再继续增强[2-3]㊂苦味肽中的疏水性氨基酸主要包括亮氨酸㊁苯丙氨酸和甘氨酸3种㊂其中,亮氨酸主要存在于豆类中,从大豆水解蛋白中分离出的苦味肽其C 端通常都含有一个亮氨酸残基,同时,在发酵不充分的纳豆中也发现有部分苦味肽的N 端和C 端含有亮氨酸残基[4]㊂苯丙氨酸存在于大多数苦味肽中,其自身带有微弱的苦味,且其氨基和羧基末端酰基化修饰能进一步增强相应苦味肽的苦味[5]㊂人工合成的甘氨酸肽中有3种短肽(Gly-Leu㊁Val-Gly 和Gly-Phe-Gly)具有苦味,也有一些含甘氨酸的短肽(如Gly-Gly㊁Gly-Ala 和Gly-Glu)不具有苦味[6]㊂由于苦味㊃11㊃㊀2023年6月第38卷第3期㊀肽会降低食物的味觉品质,因而人类和大部分动物会本能地厌恶苦味食物㊂为了改善人们对食物的味觉体验,苦味肽及苦味抑制技术得到了国内外学者的广泛关注㊂另外,苦味肽通常具有抗菌㊁抗血栓㊁抗氧化㊁血管紧张素转化酶抑制活性等生物活性[7],特别是其天然㊁安全㊁高效的广谱抗菌活性[8-9],可作为抗生素的替代药物㊂因此,对苦味肽的精准鉴别对于食品和药物研究开发具有重要意义㊂传统的湿实验方法鉴别苦味肽需要耗费大量的人力㊁物力㊁财力和时间成本㊂随着后基因组时代的来临,新产生的多肽序列呈指数级增长,湿实验方法已无法满足快速鉴别苦味肽的需求㊂因此,迫切需要开发基于计算技术的预测方法,以便快速精确地区别苦味肽和非苦味肽㊂手工设计的特征提取方法主要分为基于氨基酸组成(AAC)[10]的方法㊁基于氨基酸物理化学属性的方法和基于序列进化信息的方法㊂这些特征提取方法大都基于单一视角提取特征,并不能全面刻画多肽序列的整体特性,故影响了预测模型的鉴别能力㊂与手工设计的特征提取方法不同,深度学习方法可以直接从原始多肽序列数据中自动学习具有强鉴别能力的特征,已广泛应用于生物信息学和计算生物学领域[11-15]㊂目前,仅有iBitter-SCM[16]和BERT4Bitter[17]这两种预测方法可直接从多肽的氨基酸序列中鉴别苦味肽㊂其中, iBitter-SCM方法通过手工设计的特征提取方法从多肽序列中提取有鉴别能力的特征,进而结合传统机器学习方法构建预测模型;BERT4Bitter方法使用BERT[18]预训练模型自动地从多肽序列中提取特征,预测性能显著优于iBitter-SCM方法㊂基于此,本文拟提出一种新颖的融合传统手工特征和预训练深度特征的深度学习方法Bitter-Fus㊂该方法首先使用预训练蛋白质序列语言模型Prot-Bert[19]自动地从多肽序列中提取深度学习特征,然后将该深度学习特征输入长短期记忆网络(LSTM)[20]中进行降维处理,以保留与多肽序列最相关的深度特征,最后将降维处理后的深度特征与基于氨基酸组成的手工特征融合后,输入前馈神经网络中,进而构建苦味肽预测模型实现苦味肽鉴别,并结合相关验证方法验证该预测方法的准确性㊂1㊀相关方法与模型构建1.1㊀数据集为了与已有方法进行公平比较,本文使用已有研究中通用的数据集BTP640[16-17]进行预测模型的构建和评测,其中,BTP640数据集包含320个苦味肽和320个非苦味肽,已剔除重复的及包含X㊁B㊁U㊁Z等不确定氨基酸残基的多肽序列㊂为了训练预测模型和验证模型的泛化能力,BTP640数据集以8ʒ2的比例被随机分割为训练集BTP-CV和独立测试集BTP-TS㊂训练集BTP-CV包含256个苦味肽和256个非苦味肽,而独立测试集BTP-TS包含64个苦味肽和64个非苦味肽㊂BTP640数据集中的苦味肽均从文献中手工收集且经过实验验证,而非苦味肽数据集由于科学意义较小,其中只有很小一部分经过实验验证㊂因此,在生物活性肽序列数据库BIOPEP[21]中随机生成与苦味肽数目相同的多肽序列,将其作为非苦味肽数据集(前提是它们不存在于苦味肽数据集中)用于训练预测模型㊂这种将随机序列作为阴性数据集的方法使用了先前研究中构建阴性数据集的标准过程[22-23],该过程基于发现随机序列为正的概率非常低的假设㊂1.2㊀多肽序列特征提取1.2.1㊀传统手工特征提取㊀前期实验发现,与其他手工设计的特征提取方法相比,AAC方法的预测精度较好㊂因此,本文使用AAC方法提取多肽序列的手工特征㊂氨基酸是组成多肽序列的基本物质,自然界中共有20种基本氨基酸㊂多肽序列可看作是由不同数量基本氨基酸通过不同排列组合构成的㊂氨基酸组成则是指这些基本氨基酸在多肽序列中的出现频率㊂假设P是任意一个多肽序列,f(a i)表示氨基酸a i(i=1,2, ,20)在该多肽序列中出现的次数,则多肽序列P可表示为如下向量形式:P=(p1,p2, ,pi, ,p20)Tp i=f(a i)/ð20i=1f(a i)式中,i=1,2, ,20,p i表示氨基酸a i在多肽序列中的出现频率㊂1.2.2㊀预训练深度特征提取㊀BERT的模型架构㊃21㊃㊀杨学东,等:一种基于深度学习的苦味肽精准预测方法是一个多层双向变压器编码器,每层有12个或24个编码器块,分别用于BERT基带和BERT大型模型㊂其中,每个编码器块由一个多头自注意力子层和一个完全连接的前馈子层组成,在这两个子层周围部署剩余连接,进行层规范化㊂ProtBert是A.El-naggar等提出的预训练蛋白质序列语言模型,通过使用UniRef100和BFD这2个数据库的蛋白质序列微调原始BERT模型而获得,可直接从原始多肽序列数据中自动学习到具有强鉴别能力的特征㊂其中,UniRef100数据库是一个广泛使用的参考蛋白质序列数据库,BFD数据库合并了UniProt数据库中可使用的所有蛋白质序列及从多个元基因组测序项目翻译的蛋白质序列㊂本文使用预训练蛋白质序列语言模型ProtBert提取深度学习特征㊂1.3㊀融合传统手工特征和预训练深度特征的预测方法㊀㊀融合传统手工特征和预训练深度特征的苦味肽预测模型Bitter-Fus的整体架构如图1所示㊂首先,从输入多肽序列中提取潜在特征㊂根据BERT模型的要求,首先输入多肽序列标记化,即将字符 [CLS] 和 [SEP] 添加到整个多肽序列的开头和结尾,并添加位置编码㊂然后,将生成的向量输入预训练蛋白质序列语言模型ProtBert中以提取深度学习特征㊂经过ProtBert模型训练,每个输入的标记(即每个氨基酸)创建了上下文感知嵌入,也就是特征,输入的多肽序列被编码为1024维的高维特征向量㊂接着,将获得的1024维的高维深度学习特征向量输入长短期记忆(LSTM)网络中㊂LSTM网络基于多肽序列,对输入的特征信息进行提取,并对其中重要的特征进行选择及降维㊂本文使用网格搜索的方法确定LSTM网络的输出层参数,即对高维特征降维后的维度㊂在实验中,将输出层维度分别设置为128㊁256㊁512㊁640和768后进行训练,根据10折交叉验证的平均结果可知,输出层为512维时的模型预测效果最佳㊂故通过LSTM网络降维,最终获得512维的由ProtBerT模型提取的深度特征㊂随后,将该深度特征与传统AAC方法提取的20维手工特征进行特征融合,得到532维的融合特征㊂最后,将该融合特征输入前馈神经网络(使用Sigmoid图1㊀融合传统手工特征和预训练深度特征的苦味肽预测模型Bitter-Fus的整体架构Fig.1㊀The overall architecture of a bitter peptide prediction model Bitter-Fus by fusing handcrafted features and pretrained deep features分类),建立苦味肽分类预测模型㊂2㊀实验验证与分析2.1㊀评价指标在苦味肽预测领域,通常采用以下4个评价指标定量评估预测模型的预测性能:准确性(Acc)㊁马修斯相关系数(MCC)㊁敏感性(Sn)和特异性(Sp)㊂其中,Acc和MCC从总体分别反映预测模型的准确率和稳定性,而Sn和Sp则从局部分别反映预测模型对正类和负类样本的预测准确率㊂这4个评价指标的计算方法如下式所示:Sn=1-N+-N+㊀㊀0ɤSnɤ1Sp=1-N-+N-㊀㊀0ɤSpɤ1Acc=1-N+-+N-+N++N-㊀㊀0ɤAccɤ1M CC=1-N+-N++N-+N-()1+N-+-N+-N+()1+N+--N-+N-()㊀-1ɤM CCɤ1式中,N+表示苦味肽样本总数;N+-表示被错误预测为非苦味肽的苦味肽样本数量;N-表示非苦味肽样㊃31㊃㊀2023年6月第38卷第3期㊀本总数;N-+表示被错误预测为苦味肽的非苦味肽样本数量㊂当N+-=0,即没有苦味肽样本被错误预测为非苦味肽时,预测模型的Sn=1㊂当N+-=N+,即所有苦味肽样本被错误预测为非苦味肽时,预测模型的Sn=0㊂类似地,当N-+=0,即没有非苦味肽样本被错误预测为苦味肽时,预测模型的Sp=1㊂当N-+= N-,即所有非苦味肽样本被错误预测为苦味肽时,预测模型的Sp=0㊂当N+-=N-+=0,即所有苦味肽和非苦味肽样本都未被错误预测时,预测模型的Acc= 1,MCC=1㊂当N+-=N+且N-+=N-,即所有苦味肽和非苦味肽样本都被错误预测时,预测模型的Acc=0, MCC=-1㊂当N+-=N+/2且N-+=N-/2时,预测模型的Acc=0.5,MCC=0,意味着模型的预测结果与随机猜测相当㊂2.2㊀交叉验证交叉验证是一种广泛用于评估机器模型并测试其性能的方法㊂在预测分析中,10折交叉验证测试㊁独立数据集测试和jackknife测试是3种常用的检验模型预测性能的交叉验证方法㊂本文采用10折交叉验证测试和独立数据集测试对模型进行评估㊂在10折交叉验证测试中,首先将数据集拆分为10个相等的部分,依次选择其中9折作为训练集以训练模型,剩余1折作为测试集以验证模型,随后保存其验证结果㊂最后将获得的10次验证结果平均值作为对模型性能的估计值㊂泛化能力决定了预测模型对新输入的适应能力,而检验深度学习模型的泛化能力非常重要,故本文使用独立数据集BTP-TS 对模型进行独立测试,以评估模型的泛化能力和稳定性㊂2.3㊀本文预测方法与已有预测方法的比较为了公平比较,笔者在BTP-CV数据集上采用10折交叉验证方法对本文所提预测方法Bitter-Fus 与iBitter-SCM预测方法和BERT4Bitter预测方法的预测性能进行比较分析,3种预测方法的10折交叉验证结果见表1㊂由表1可知,与BERT4Bitter预测方法相比,Bitter-Fus预测方法的Acc和MCC分别提高了4.8%和10.9%,Sn和Sp分别提高了6.2%和3.4%㊂与iBitter-SCM预测方法相比,Bitter-Fus预测方法的Acc和MCC分别提高了3.6%和7.2%,Sn 和Sp分别提高了1.0%和6.6%㊂10折交叉验证结㊀㊀㊀表1㊀3种预测方法的10折交叉验证结果Table1㊀10-fold cross-validation resultsof3prediction methods预测方法Acc MCC Sn Sp iBitter-SCM0.8710.7510.9130.828 BERT4Bitter0.8610.7260.8680.854 Bitter-Fus0.9020.8050.9220.883果表明,本文提出的预测方法Bitter-Fus在4个性能指标上都表现优异㊂为了进一步评估本文所提预测方法Bitter-Fus 的泛化性能,在独立数据集BTP-TS上将其与iBit-ter-SCM预测方法和BERT4Bitter预测方法进行比较分析㊂3种预测方法的独立数据集测试结果见表2㊂由表2可知,Bitter-Fus预测方法在Acc㊁MCC和Sp这3项指标上均优于BERT4Bitter预测方法,其中,Sp提高最多,为7.0%,Acc和MCC分别提高了0.9%和2.1%㊂尽管Bitter-Fus预测方法的Sn略低于BERT4Bitter预测方法,但明显高于iBitter-SCM 预测方法㊂考虑到独立数据集测试是最严格的交叉验证方法,较高的Acc和MCC更可取,由此表明,本文所提预测方法更有效也更稳定㊂综上所述,本文所提预测方法Bitter-Fus的整体性能优于目前最先进的苦味肽预测方法BERT4Bitter和iBitter-SCM,且更精准和稳定㊂表2㊀3种预测方法的独立数据集测试结果Table2㊀The results of3prediction methods testedin independent data sets预测方法Acc MCC Sn Sp iBitter-SCM0.8440.6880.8440.844 BERT4Bitter0.9220.8440.9380.906 Bitter-Fus0.9300.8620.8910.9693㊀结论本文针对苦味肽的精准鉴别问题,提出了一种新颖的融合传统手工特征和预训练深度特征的预测方法㊂该方法首先使用预训练蛋白质语言模型ProtBert提取多肽序列的深度学习特征,并与传统手工特征相融合,然后使用LSTM网络进行特征降维处理,保留与多肽序列最相关的特征以使模型整体预测性能大幅度提高㊂10折交叉验证测试和独立数据集测试结果表明,该方法的Acc分别达到㊃41㊃㊀杨学东,等:一种基于深度学习的苦味肽精准预测方法0.902和0.930,MCC分别达到0.805和0.862,且与当前最先进的苦味肽鉴别方法BERT4Bitter和iBitter-SCM相比,Acc㊁MCC㊁Sn和Sp这4项性能指标均有明显提高㊂在苦味肽的鉴别预测领域,本文所提预测方法有望成为一种有潜力的预测工具㊂此外,将传统手工特征与ProtBert深度学习特征融合的特征工程模块也可应用于其他基于蛋白质序列的预测任务中,并可使下游预测模型获得更全面的序列信息,进而使预测模型得以更充分的训练从而获得更好的预测效果㊂参考文献:[1]㊀刘桃妹.风味增强肽及其应用研究[J].江苏调味副食品,2014(3):5-8.[2]㊀王知非,林璐,孙伟峰,等.苦味肽和苦味受体研究进展[J].中国调味品,2016,41(9):152-156.[3]㊀毕继才,崔震昆,张令文,等.苦味传递机制与苦味肽研究进展[J].食品工业科技,2018,39(11):333-338.[4]㊀司阔林,李志国,李玲玉,等.干酪苦味肽的形成及脱苦方法研究进展[J].食品工业,2021,42(3):267-271.[5]㊀郭兴峰,魏芳,周祥山,等.苦味肽的形成机理及脱苦技术研究进展[J].食品研究与开发,2017,38(21):207-211.[6]㊀应欣,张连慧,陈卫华.蛋白水解物苦味形成㊁评价及功能活性的研究进展[J].中国粮油学报,2017,32(12):141-146.[7]㊀邓尚贵,余妙灵,甄兴华,等.苦味肽抗氧化活性延长食品保鲜[J].食品安全质量检测学报,2020,11(2):375-380.[8]㊀杨保军,梁琪,宋雪梅.基于计算机虚拟技术研究牦牛乳硬质干酪苦味肽的抑菌活性差异[J].食品与生物技术学报,2021,40(12):75-87.[9]㊀杨保军,梁琪,宋雪梅.牦牛乳干酪苦味肽ACE抑制活性表征的分子机制[J].中国食品学报,2022,22(5):8-17.[10]CHOU K ing amphiphilic pseudo amino acid compo-sition to predict enzyme subfamily classes[J].Bioinfor-matics,2005,21(1):10-19.[11]BO W C,QIN D Y,ZHENG X,et al.Prediction of bitter-ant and sweetener using structure-taste relationship mod-els based on an artificial neural network[J].FoodResearch International,2022,153:110974. [12]HUANG W K,SHEN Q C,SU X B,et al.BitterX:A toolfor understanding bitter taste in humans[J].ScientificReports,2016,6(1):23450.[13]ZHANG Y Q,ZHU G C,LI K W,et al.HLAB:Learningthe BiLSTM features from the ProtBert-encoded proteinsfor the class I HLA-peptide binding prediction[J].Brief-ings in Bioinformatics,2022,23(5):bbac173. [14]WANG Z F,LEI X J.Prediction of RBP binding sites oncircRNAs using an LSTM-based deep sequence learningarchitecture[J].Briefings in Bioinformatics,2021,22(6):bbab342.[15]GUO Y C,YAN K,LYU H W,et al.PreTP-EL:Predictionof therapeutic peptides based on ensemble learning[J].Briefings in Bioinformatics,2021,22(6):bbab358. [16]CHAROENKWAN P,YANA J,SCHADUANGRAT N,et al.iBitter-SCM:Identification and characterization ofbitter peptides using a scoring card method with propensityscores of dipeptides[J].Genomics,2020,112(4):2813-2822.[17]CHAROENKWAN P,NANTASENAMAT C,HASAN M M,et al.BERT4Bitter:A bidirectional encoder representa-tions from transformers(BERT)-based model for impro-ving the prediction of bitter peptides[J].Bioinformatics,2021,37(17):2556-2562.[18]DEVLIN J,CHANG M W,LEE K,et al.BERT:Pre-train-ing of deep bidirectional transformers for language under-standing[C]ʊAssociation for Computational Linguistics.Proceedings of the2019Conference of the North Ameri-can Chapter of the Association for Computational Linguis-tics:Human Language Technologies.Minneapolis,Minne-sota:Association for Computational Linguistics,2019:4171-4186.[19]ELNAGGAR A,HEINZINGER M,DALLAGO C,et al.ProtTrans:Towards cracking the language of lifes codethrough self-supervised deep learning and high perform-ance computing[J].IEEE Transactions on Pattern Analy-sis and Machine Intelligence,2022,44(10):7112-7127.[20]HOCHREITER S,SCHMIDHUBER J.Long short-termmemory[J].Neural Computation,1997,9(8):1735-1780.[21]MINKIEWICZ P,DZIUBA J,IWANIAK A,et al.BIOPEPdatabase and other programs for processing bioactive pep-tide sequences[J].Journal of AOAC International,2008,91(4):965-980.[22]GAUTAM A,CHAUDHARY K,KUMAR R,et al.In silicoapproaches for designing highly effective cell penetratingpeptides[J].Journal of Translational Medicine,2013,11(1):74.[23]KUMAR R,CHAUDHARY K,CHAUHAN J S,et al.Anin silico platform for predicting,screening and designingof antihypertensive peptides[J].Scientific Reports,2015,5(1):12512.㊃51㊃㊀2023年6月第38卷第3期㊀An accurate identification method of bitter peptidesbased on deep learningYANG Xuedong 1,HAN Lijun 1,WANG Rong 1,WANG Hongwei 2,WANG Xiao 1,31.College of Computer and Communication Engineering ,Zhengzhou University of Light Industry ,Zhengzhou 450001,China ;2.College of Food and Bioengineering ,Zhengzhou University of Light Industry ,Zhengzhou 450001,China ;3.Henan Key Laboratory of Food Safety Data Intelligence ,Zhengzhou 450001,ChinaAbstract :Given that wet experimental methods were no longer adequate for the rapid identification of bitterpeptides,this paper presented Bitter-Fus,a novel predictive deep learning method incorporating traditional manualfeatures and pre-trained deep features.Firstly,the method automatically extracted deep learning features frompeptide sequences using a pre-trained protein sequence language model,then fed the deep learning features into a long short-term memory (LSTM)network for dimensionality reduction to retain the most relevant features.Finally,the reduced-dimensional deep features were fused with the manual features composed of traditional amino acidscomposition (AAC)method and passed into the feedforward neural network to construct a prediction model.Thevalidation experimental results showed that the prediction method Bitter-Fus obtained an accuracy precision value of0.902and a Mathews correlation coefficient value of 0.805in a 10-fold cross-validation,and an accuracy precision value of 0.930and a Mathews correlation coefficient value of 0.862in the independent dataset test,whichsignificantly outperformed the current state-of-the-art bitter peptide prediction methods BERT4Bitter and iBitter-SCM.Key words :bitter peptide;deep learning;feature extraction;feature fusion㊀(责任编辑:杨晓娟)本刊数字网络传播声明本刊已许可中国知网,万方数据资源系统,维普网,国家科技学术期刊开放平台,博看网,超星,中国科技论文在线,中教数据库,CA,EBSCO host ,Ulrichs,FSTA 等在其系列数据库产品中以数字化方式复制㊁汇编㊁发行㊁信息网络传播本刊全文㊂其相关著作权使用费与本刊稿酬一并支付㊂作者向本刊提交文章发表的行为即视为同意我刊上述声明㊂㊃61㊃。
OROS Products plus Solutions Introduction (2)
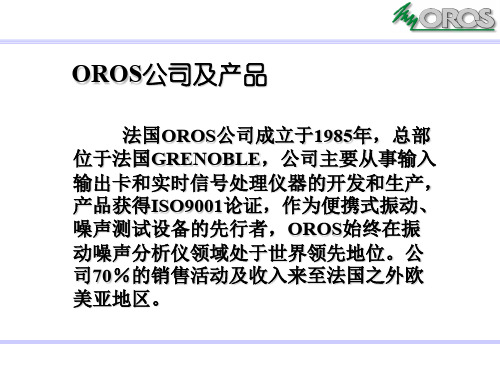
法国OROS公司成立于1985年,总部 位于法国GRENOBLE,公司主要从事输入 输出卡和实时信号处理仪器的开发和生产, 产品获得ISO9001论证,作为便携式振动、 噪声测试设备的先行者,OROS始终在振 动噪声分析仪领域处于世界领先地位。公 司70%的销售活动及收入来至法国之外欧 美亚地区。
• OR34/OR35是用户下一代试车台的选择
成功案例4
• 用户:Valeo Shanghai(上海法雷奥) • OROS OR36 8通道分析仪 • 汽车发电机、起动机的测试 • 原因
•生产线上OROS在线检测设备的成功应用 •OR36强大的分析能力和便携式设计 •FFT+Order+Recorder 并行工作能力 •Valeo对产品质量的严格保障,在给整车厂配套之 前,必须在用户方作试验。 • OR36帮助Valeo赢得了更多的用户:南汽、东风标致 、海南马自达、北京现代… • 其他汽车用户:一汽、Bosch 苏州、福亚、东风等
OR36:
• 概要: • 4,8,12 or 16个 输入通道 • 5.2 kg • 支持直流/交流供电 • 内置供电电池 • Lemo or BNC + LEDs • 2 个触发转速通道 • 2 个输出通道 • 支持 TEDS • 支持ICP • 内置40GB存储硬盘 • 独立工作能力 •
OR38:
• 模块化
• 通道数可扩展 • 输入类型可选择 • 实时分析能力可扩展
OR34:
• 概要: • 2 or 4模拟输入 通道 • 重量:1.4 kg (3 lbs) • 2 个触发/转速通道 • 1个 输出通道 • 支持 TEDS
• 支持ICP
• 内置供电电池 • OR3X 技术