Convergence rate of an iterative method for a nonlinear matrix equation
castep最全的资料refson1

Practical calculations usingfirst-principles QMConvergence,convergence,convergenceKeith RefsonSTFC Rutherford Appleton LaboratorySeptember18,2007Results of First-Principles Simulations (2)Synopsis (3)Convergence4 Approximations and Convergence (5)Convergence with basis set (6)Error Cancellation (7)Plane-wave cutoffconvergence/testing (8)Pseudopotentials and cutoffbehaviour (9)FFT Grid parameters (10)Force and Stress (11)Iterative Tolerances (12)K-point convergence (13)Golden rules of convergence testing (14)Structural Calculations15 Solids,molecules and surfaces (16)Convergence of Supercells for Molecules (17)Variable Volume Calculations (18)Variable Volume Calculations-II (19)Summary20 Summary (21)Results of First-Principles SimulationsFirst-principles methods may be used for subtle,elegant andaccurate computer experiments and insight into the structureand behaviour of matter.First principles results may be worthless nonsense2/21SynopsisThis aims of this lecture are1.To use the examples to demonstrate how to obtain converged results,ie correct predictions from the theory.2.How to avoid some of the common pitfalls and to avoid computing nonsense.Further reading:Designing meaningful density functional theory calculation in materials science-a primer Anne E Mattson et al.Model.Sim.Mater.Sci Eng.13R1-R31(2005).3/21Convergence4/21 Approximations and Convergences“Every ab-initio calculation is an approximate one”.s Distinguish physical approximationsx Born-Oppenheimerx Level of Theory and approximate XC functionaland convergable,numerical approximationsx basis-set size.x Integral evaluation cutoffsx numerical approximations-FFT gridx Iterative schemes:number of iterations and exit criteria(tolerances)x system sizes Scientific integrity and reproducibility:All methods should agree answer to(for example)“What is lattice constant of silicon in LDA approximation”if sufficiently well-converged.s No ab-initio calculation is ever fully-converged!5/21Convergence with basis setBasis set is fundamental approximation to shape of orbitals.How accurate need it be?s The variational principle states that E0≤<ψ|H|ψ>offprecision=COARSE/MEDIUM/FINE.s Though E is monotonic in E c it is not necessarily regular.6/21Error Cancellations Consider energetics of simple chemical reaction MgO (s)+H 2O (g)→Mg(OH)2,(s)s Reaction energy computed as ∆E =E product −P E reactants =E Mg (OH )2−(E MgO +E H 2O )sEnergy change on increasing E cut from 500→4000eVMgO -0.021eV H 2O -0.566eV Mg(OH)2-0.562eV Convergence error in ∆E -0.030eVs Errors associated with H atom convergence are similar on LHS and RHS and cancel in final result.s Energy differences converge much faster than ground-state energy.sAlways use same cutofffor all reactants and products .7/21FFT Grid parametersSome optimizations and tweaks of FFT grid dimensions...s FFT grid should be large enough to accommodate all G-vectors of density,n(r),within cutoff:G≤2G MAX.s Guaranteed to avoid”aliasing”errors in case of LDA and pseudopotentials without additional core-charge density.s In practice can often get away with1.5G MAX or1.75G MAX with little error penalty for LDA without core or augmentation charge.s GGA XC functionals give density with higher Fourier components,and need1.75G MAX-2G MAXs Finer grid may be needed to represent USP augmentation charges or core-charge densities.s CASTEP incorporates a second,finer grid for charge density to accommodate core/augmentation charges while usingG MAX for orbitals.s Set by either parameter fine scale(multiplier of G MAX)or finegmax is property of pseudopotential and transferable to other cells but fine scale is not.s(Rarely)may need to increase fine scale for DFPT phonon calculations using GGA functionals for acoustic sum rule to be accurately satisfied.10/21Iterative Tolerancess How to control the iterative solvers ?s Parameter elec tol specifies when SCF energy converged.s Optimizer also exits if max cycles reached –always check that it really did converge .sHow accurate does SCF convergence need to be?s Energetics:same accuracy of result.s Geometry/MD:much smaller energy tolerance needed to converge forces.s Cost of higher tolerance is only a few additional SCF iterations.sComing soon to a code near you –elec tol to exit SCF using force convergence criteriasInaccurate forces are common cause of geometry optimization failure.12/21Golden rules of convergence testing1.Test PW convergence on small model system(s)with same elements.2.Testing requires going to unreasonably high degree of convergence,so calculations could be expensive.Test oneparameter at a time.e knowledge of transferability of PW cutoffandfinegmax for USPs.e physical∆k spacing to scale k-point sampling from small system to large.e forces,stresses and other cheap properties as measure of convergence.7.May need small number of tests on full system andfinal property of interest(eg dielectric permittivities are verysensitive to k-point sampling).8.Write your convergence criteria in the paper,not just“250eV cutoffand10k-points in IBZ”.9.Convergence is achieved when value stops changing with parameter under test,NOT when the calculation exceedsyour computer resources and NOT when it agrees with experiment.14/21Structural Calculations15/21Solids,molecules and surfacesSometimes we need to compute a non-periodic system with a PBC code.s Surround molecule by vacuum space to model using periodic code.s Similar trick used to construct slab for surfaces.s Must include enough vacuum space that periodic images do not interact.s To model surface,slab should be thick enough that centre is“bulk-like”.s Beware of dipolar surfaces.Surface energy does not converge with slab thickness.s When calculating surface energy,try to use same cell for bulk and slab to maximise error cancellation of k-point set.s Sometimes need to compare dissimilar cells–must use absolutely converged k-point set as no error cancellation.16/21Variable Volume Calculationss Two ways to evaluate equilibrium lattice parameter-minimum of energy or zero of stress.s Stress converges less well than energy minimum with cutoff.Variable Volume Calculations-IIs Two possibilities for variable-cell MD or geometry optimization when using plane-wave basis set.s Infixed basis size calculation,plane-wave basis N PW is constant as cell changes.s Cell expansion lowers G max and K.E.of each plane wave,and therefore lowers effective E c.s Easier to implement but easy to get erroneous results.s Need very well-converged cutofffor success.sfixed cutoffcalculations reset basis for each volume,changing N PW but keeping G max and E cfixed.s This is almost always the correct method to use.18/21Summary19/21 Summarys Used with care,first principles simulations can give highly accurate predictions of materials properties.s Full plane-wave basis convergence is rarely if ever needed.Error cancellation ensure that energy differences,forces and stress converge at lower cutoff.s Convergence as a function of adjustable parameters must be understood and monitored for the property of interest to calculate accurate results.s Don’t forget to converge the statistical mechanics as well as the electronic structure!s A poorly converged calculation is of little scientific value.20/21。
幂法求矩阵最大特征值

幂法求矩阵最大特征值摘要在物理、力学和工程技术中的很多问题在数学上都归结为求矩阵特征值的问题,而在某些工程、物理问题中,通常只需要求出矩阵的最大的特征值(即主特征值)和相应的特征向量,对于解这种特征值问题,运用幂法则可以有效的解决这个问题。
幂法是一种计算实矩阵A的最大特征值的一种迭代法,它最大的优点是方法简单。
对于稀疏矩阵较合适,但有时收敛速度很慢。
用java来编写算法。
这个程序主要分成了三个大部分:第一部分为将矩阵转化为线性方程组;第二部分为求特征向量的极大值;第三部分为求幂法函数块。
其基本流程为幂法函数块通过调用将矩阵转化为线性方程组的方法,再经过一系列的验证和迭代得到结果。
关键词:幂法;矩阵最大特征值;j ava;迭代POWER METHOD TO CALCULATE THE MAXIMUMEIGENV ALUE MATRIXABSTRACTIn physics, mechanics and engineering technology of a lot of problems in math boil down to matrix eigenvalue problem, and in some engineering, physical problems, usually only the largest eigenvalue of the matrix (i.e., the main characteristics of the value) and the corresponding eigenvectors, the eigenvalue problem for solution, using the power law can effectively solve the problem.Power method is A kind of computing the largest eigenvalue of real matrix A of an iterative method, its biggest advantage is simple.For sparse matrix is right, but sometimes very slow convergence speed.Using Java to write algorithms.This program is mainly divided into three most: the first part for matrix can be converted to linear equations;The second part is the eigenvector of the maximum;The third part is the exponentiation method of function block.Its basic process as a power law function block by calling the method of matrix can be converted to linear equations, then after a series of validation and iteration to get the results.Key words: Power method; Matrix eigenvalue; Java; The iteration目录1幂法 (1)1.1 幂法基本思想 (1)1.2规范化 (2)2概要设计 (3)2.1 设计背景………………..…………………………………………………………. .32.2 运行流程 (3)2.3运行环境 (3)3 程序详细设计 (4)3.1 第一部分:矩阵转化为线性方程组……..………………………………………. .43.2 第二部分:特征向量的极大值 (4)3.3 第三部分:求幂法函数块 (5)4 运行过程及结果 (6)4.1 运行过程.........................................................………………………………………. .64.2 运行结果 (6)4.3 结果分析 (6)5 心得体会 (7)参考文献 (8)附录:源程序 (9)1 幂法设A n 有n 个线性相关的特征向量v 1,v 2,…,v n ,对应的特征值λ1,λ2,…,λn ,满足|λ1| > |λ2| ≥ …≥ |λn |1.1 基本思想因为{v 1,v 2,…,v n }为C n的一组基,所以任给x (0)≠ 0,∑==ni i i v a x 1)0( —— 线性表示所以有])([)(21111111)0(∑∑∑∑====+====ni i i ki kni k k i i ni ik i n i i i kkv a v a v a v A a v a A xA λλλλ若a 1 ≠ 0,则因11<λλi知,当k 充分大时 A (k )x (0) ≈ λ1k a 1v 1 = cv 1 属λ1的特征向量,另一方面,记max(x ) = x i ,其中|x i | = ||x ||∞,则当k 充分大时,111111*********)0(1)0()max()max()max()max()max()max(λλλλλ==≈---v a v a v a v a x A x A k kk k k k若a 1 = 0,则因舍入误差的影响,会有某次迭代向量在v 1方向上的分量不为0,迭代下去可求得λ1及对应特征向量的近似值。
Iterative methods for total variation denoising
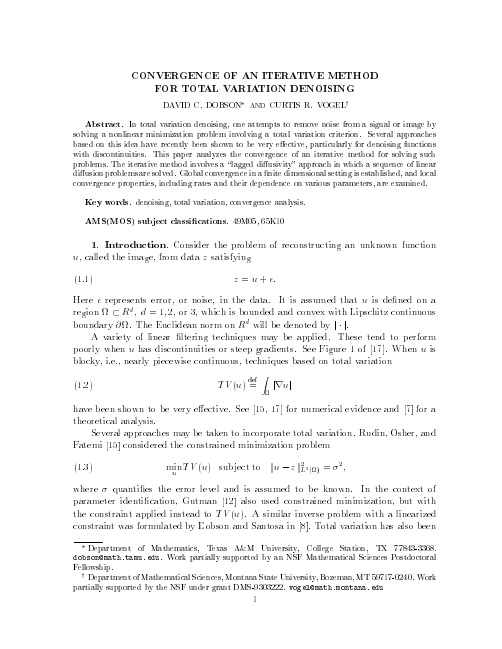
DAVID C. DOBSON AND CURTIS R. VOGELy
Abstract. In total variation denoising, one attempts to remove noise from a signal or image by
may be applied. To deal with the non-di erentiability of the TV functional, interior
point methods from linear programming (see Li and Santosa 14]; note that T V (u) has
In 17], Vogel and Oman introduced a xed point iteration to minimize the penalized least squares functional
(1.6)
1 2
ku
?
zk2L2(
)
+
J (u):
The corresponding Euler-Lagrange equations
solving a nonlinear minimization problem involving a total variation criterion. Several approaches based on this idea have recently been shown to be very e ective, particularly for denoising functions with discontinuities. This paper analyzes the convergence of an iterative method for solving such problems. The iterative method involves a \lagged di usivity" approach in which a sequence of linear di usion problems are solved. Global convergence in a nite dimensional setting is established, and local convergence properties, including rates and their dependence on various parameters, are examined.
边界层对三氯氢硅_氢气系统中多晶硅化学气相沉积的影响_英文_

FLUID FLOW AND TRANSPORT PHENOMENAChinese Journal of Chemical Engineering, 19(1) 1—9 (2011)Effect of Boundary Layers on Polycrystalline Silicon Chemical Vapor Deposition in a Trichlorosilane and Hydrogen System*ZHANG Pan (张攀)1, W ANG Weiwen (王伟文)2, CHENG Guanghui (陈光辉)2 and LI Jianlong (李建隆)2,**1 Institute of Electronmechanical Engineering, Qingdao University of Science and Technology, Qingdao 266061, China2 Institute of Chemical Engineering, Qingdao University of Science and Technology, Qingdao 266042, ChinaAbstract This paper presents the numerical investigation of the effects of momentum, thermal and species bound-ary layers on the characteristics of polycrystalline silicon deposition by comparing the deposition rates in three chemical vapor deposition (CVD) reactors. A two-dimensional model for the gas flow, heat transfer, and mass trans-fer was coupled to the gas-phase reaction and surface reaction mechanism for the deposition of polycrystalline sili-con from trichlorosilane (TCS)-hydrogen system. The model was verified by comparing the simulated growth rate with the experimental and numerical data in the open literature. Computed results in the reactors indicate that the deposition characteristics are closely related to the momentum, thermal and mass boundary layer thickness. To yield higher deposition rate, there should be higher concentration of TCS gas on the substrate, and there should also be thinner boundary layer of HCl gas so that HCl gas could be pushed away from the surface of the substrate immediately.Keywords boundary layer, polycrystalline silicon, numerical simulation, mass diffusion1 INTRODUCTIONChemical vapor deposition (CVD) is a synthesis process of the deposition of a solid on a heated surface from a chemical reaction in the vapor phase. With CVD, it is possible to produce most metals, many nonmetal-lic elements such as carbon and silicon as well as a large number of compounds including carbides, ni-trides, oxides and many others. The CVD technology integrates several scientific and engineering disci-plines including thermodynamics, plasma physics, kinetics, fluid dynamics, and of course chemistry. In order to understand the complex transport phenomena, various types of reactor configurations such as hori-zontal reactor [1], impinging jet and rotating disk re-actor [2], pancake reactor [3], and vortex enhanced reactor [4] have been investigated. The effects of many factors on the deposition characteristics of a CVD re-actor were also discussed, such as the choice of pre-cursor gases and carrier gas [5], their respective flow rates [6], the total pressure in the reactor [7], the sub-strate temperature [8, 9], the substrate position [10], the substrate geometry [11, 12], the substrate rotation rate [13], and the tilted substrate [14, 15].Many researchers paid more attention to the ef-fects of the macroscopical structure of the reactors, but the effects of the boundary layer characteristics at the substrate in the deposition process were less ad-dressed in the CVD reactor. The rate-limiting step of a CVD reactor is generally determined either by the surface reaction kinetics or by mass transport [16-18]. When the process is limited by mass-transport phe-nomena, the controlling factors are the diffusion rate of the reactant through the boundary layer and the diffusion out of the gaseous by-products through this layer. Thus the boundary layer characteristics have vital effect on the CVD of polycrystalline silicon.It has been recognized that numerical models based on computational fluid dynamics (CFD), accounting for the interaction between gas flow, heat and mass transfer and chemical reactions, can be of great help in the optimization of CVD equipment and processes. Besides, CVD numerical models may provide funda-mental insights into the underlying physico-chemical processes. In last few decades, many numerical stud-ies of CVD processes have been performed, which can be roughly divided into tow categories: (1) CVD re-actor studies (as those mentioned above), in combina-tion with rather simple descriptions of the CVD chem-istry; (2) CVD chemistry studies [19-22], in combina-tion with simple 0-dimensional (0-D) or 1 dimensional (1D) hydrodynamic models. Recent years, enabled by the ever increasing computer performance, it has be-come possible to combine detailed descriptions of transport phenomena and reaction chemistry into a single computational model [23]. But for the trichloro-silane (TCS)-hydrogen system, it is still a challenge.In this study, a two-dimensional model for the gas flow, heat transfer, and mass transfer was coupled to the detailed mechanism of gas-phase reaction and surface reaction for the deposition of polycrystalline silicon from TCS-hydrogen system. The model was used to study the boundary layer characteristics in three CVD reactors with a flat substrate, a tilted sub-strate and an additional rib placed on the upper wall, respectively, and investigate the effects of the bound-ary layer characteristics on the polycrystalline siliconReceived 2010-06-11, accepted 2010-11-16.* Supported by the Natural Science Foundation of Shandong Province of China (ZR2009BM011), and the Doctor Foundation of Shandong Province of China (BS2010NJ005).** To whom correspondence should be addressed. E-mail: jlong_li@Chin. J. Chem. Eng., Vol. 19, No. 1, February 20112 deposition rate, and the uniformity of the deposited polycrystalline silicon in the TCS-H 2 system. 2 PHYSICAL MODEL 2.1 Reactor geometryIn view of computational costs, a two-dimensional (2-D) model coupled with gas phase reaction and sur-face reaction is considered for three CVD reactors illustrated in Fig. 1. The overall geometry of the model in Fig. 1 (a) is identical to that employed by Habuka et al [24]. In Fig. 1 (b), the substrate is tilted with an angle of 7°. In the third reactor in Fig. 1 (c), an additional rib is placed on the center of the upper wall. The dimension of the rib is 6 mm (width) ×10 mm (height). The three reactors have same overall dimensions, and the same conditions otherwise areimposed on them.(a) A flat reactor(b) A tilted reactor(c) A rib reactorFigure 1 Geometry and internal structure of studied re-actorsA mixture of TCS and H 2 enters the reactors at the top left corner and exits through the lower right corner. The substrate surface is held at a fixed tem-perature. The gases react in the reactors depositing the desired solid silicon film on the surface of the sub-strate. Finally, the reactant and product gases leave the reactors through the outlet, which is fixed at atmos-pheric pressure. 2.2 Transport modelsAs the deposition rate are strongly influenced by the complex transport phenomena of reacting mixtures in the CVD reactor, detailed computational modeling of the gas flow, temperature distribution and species transport in the reactor is of vital importance. The model of the three CVD reactors involves four pri-mary governing equations: mass conservation (or con-tinuity), momentum conservation, energy conservation, and species conservation. It describes the turbulent flow of an incompressible gas with properties that depend on the local temperature. With the above as-sumption, the governing equations can be expressed as follows:Continuity equation:()0i iu t x ρρ∂∂+=∂∂ (1) Momentum equation:()()ij i i j i i j i jp u u u g F t x x x τρρρ∂∂∂∂+=−+++∂∂∂∂ (2) Energy equation:()()()eff effi i j j j ij h j ii E u E p t x T k h J u S x x ρρτ′′′∂∂⎡⎤++=⎣⎦∂∂∂∂⎛⎞−++⎜⎟∂∂⎝⎠∑ (3) where k eff is the effective thermal conductivity coeffi-cient (including the molecular and turbulent thermal conductivity). The effect of heat transfer by radiation on the substrate temperature is ignored. Since the concentrations of TCS and HCl are low, the effects of radiation heat on these gases are also assumed to be negligible. The last term on the right-hand side de-scribes the consumption and production of heat due to the chemical reactions. Although for most CVD sys-tems, especially when the reactants are highly diluted in an inert carrier gas, the heat of reactions has a neg-ligible influence on the gas temperature distribution, the source term is accounted for in the simulation. Mass equation:()()i i i i i Y Y R S tρρ∂+∇⋅=−∇++∂v J (4) where J i is the diffusion flux of species i . For thermal CVD, the relevant driving forces for diffusion are theChin. J. Chem. Eng., Vol. 19, No. 1, February 20113concentration and temperature gradients. Since the mass fractions of all species must sum up to unity, this equation is solved for all species except the carrier gas H2. At steady-state, the transport of the reagent species to the substrate surface is balanced by the surface re-action rate. The deposition reaction is considered as a boundary condition on the species transport equation.2.3 Chemical reactionThe knowledge of the detailed gas phase and surface chemistry involved in the deposition process is of fundamental importance. During the last years a great effort was devoted to the comprehension of the reactions involved in silicon deposition. We adopted TCS chemistry primarily because of the broad appli-cation of polycrystalline silicon [25-27]. TCS decom-position kinetics was compiled by Ho et al. [28], who pointed out that SiCl2 has a high desorption rate and thus assumed TCS as the most important precursor. Valente et al. [29] analyzed the kinetics of the HCl and SiCl2 adsorption and deposition, updating the original mechanism in Ref. [28]. The overall mechanism is taken from the literature and summarized in Table 1.Table 1 The mechanisms of the chemical reactions and surface reactions in trichlorosilane and hydrogen system (G: gas-phase reaction, F: surface reaction)No. Reaction lg AβE a Ref.G1 TCS SiCl2+HCl 14.690.0308582[28]G2 SiH2Cl2SiCl2+H2 13.920.0324074[28]G3 SiCl2H2HSiCl+HCl 14.940.066155[28]G4 Si2Cl5H SiCl4+HSiCl 13.70.0218980[28]G5 Si2Cl5H SiCl3H+SiCl2 13.90.0188415[28]G6 Si2Cl6SiCl4+SiCl2 14.20.0200976[28]F1 TCS+4σ→SiCl*+H*+2Cl* 8.05 0.5 −15911[30]F2 SiH2Cl2+4σ→SiCl*+2H*+Cl* 8.58 0.5 −15911[30]F3 SiCl4+4σ→SiCl*+3Cl* 9.840.5−2814[29]F4 3SiCl*→SiCl2+Cl*+2Si(s)+2σ 24.20 0.0 280529[29]F5 2Cl*+Si(s)→SiCl2+2σ 24.20.0280529[29]F6 H2+2σ→2H* 11.360.572226[29]The decomposition of TCS and SiH2Cl2 are con-sidered as the most important reactions in the gas-phase reaction mechanism. About the surface chemistry, three adsorbed species were considered: H*, Cl* and SiCl* [29]. All gaseous species adsorb dissociatively on the surface leading to the formation of adsorbed hydrogen and chlorine, and SiCl* through reactions.2.4 Turbulent model and numerical methodReynolds numbers in CVD reactors are usually quite low, and as a consequence the gas flow is as-sumed to be laminar [15, 31, 32]. But these low Rey-nolds numbers in combination with quite large tem-perature difference (up to 1000 K) can lead to signifi-cant interactions between forced and free convection in the CVD equipment. The mixed convection can be an important factor influencing heat, mass transfer and chemistry. van Santen et al. [33] found that turbulence can increases the heat flux, which offer the possibility for a high deposition rate. Thus, the low-Reynolds- number k-ε turbulent model was used to simulate the turbulent transport [22].The governing equations coupled with the bound-ary conditions have been solved using a commercially available software package FLUENT, which is based on the finite volume method (FVM) to discretize the non-linear partial differential equations of the model, and the segregated solution algorithm has been se-lected. For the pressure-velocity coupling, the SIM-PLE (Semi-Implicit Method for Pressure-Linked Equa-tions) method was used.The geometry and the mesh are created using GAMBIT 2.2 starting from its primitives (shown in Fig. 1). Numerical tests are carried out using 2300, 4800 and 6600 cells to evaluate the effect of mesh size on the calculated results. Comparison of 4800 cells velocities estimates with 6600 cells estimates showed a mean relative difference of 0.87% and a root mean square difference of 2.8%. The computational grid is defined by hexahedral cells, non-uniformly distributed, with a total of 4800 cells.Compared to the solution of the hydrodynamics problem, the solution of the accompanying chemical reactions is not a trivial task because it gives the im-portant chemical source terms in the aforementioned governing equations. Especially for the surface reac-tion in the study, the transport process (diffusion) that carries species to and from the surface may be com-parable in rate to that of reaction at the surface. Transport and reaction have to be solved simultane-ously as a set of nonlinear algebraic equations at each node on the reacting surface. The numerical stiffness of the multi-dimensional multi-species transport cou-pled detailed CVD chemistry models leads to poor convergence, excessive computation time, and unreli-able predictions.Thus, computationally efficient methods are needed for simulating CVD reacting flows. Different numerical methods have been developed to solve these nonlinear equations introduced by the chemistry [34, 35]. Available approaches include direct integra-tion (DI) in the FLUENT package, and DI is computa-tionally expensive in CVD simulation.The in-situ-adaptive tabulation (ISA T) method [36], which is an effective preconditioning technique, was used to implement detailed chemical kinetics. Since the chemical source term is computationally costly, a pre-computed chemical look-up table is calculated and stored for a set of representative initial conditions in the composition space. Then, numerical interpolation is used to find the values based on the neighboring points, replacing DI operation in the simulation.Chin. J. Chem. Eng., Vol. 19, No. 1, February 20114 The turbulence-chemistry interactions were com-puted by Eddy-Dissipation- Concept (EDC) model [37]. The results presented in this paper were obtained using the second order scheme for spatial discretization of the momentum equations and species transports equations. For pressure, linear discretization was used. A con-vergence criterion of 1×10−6 was used for continuity, momentum, energy and species transport equations. 2.5 Boundary condition and gas propertiesAt the inlet, the velocity distribution is consid-ered uniform; the gas feed is assumed to be uniform at 300 K. The mass fraction of the species in the inlet mixture is specified. A no-slip and an adiabatic or a temperature condition is imposed on the side walls, respectively as shown in Fig. 1 (a), and the substrate boundary is set to a constant temperature. A thermal conductivity of 204 W·m −1·K −1 was applied for the susceptors.Some reasonable simplifying assumptions have been made to reduce the complexity of the numerical problem: the gases, being highly diluted in hydrogen, obey the ideal gas law and Newton’s law of viscosity; the gaseous mixture behaves as continuum under steady state conditions; pressure variations in the en-ergy equation are neglected as the Mach number is very small.The viscosity and thermal conductivity of the gas species may be calculated from the kinetic theory. The coefficients of the specific heat capacity polynomial and the Lennard-Jones parameters (the characteristic energy and the collision diameter) were taken from Ref. [38].Mixture gas density is estimated based on the ideal gas law [39, 40]:iiiY p RT m ρ=∑(5) The full multi-component diffusion model is used to calculate the ordinary diffusion, because the mass fraction of the hydrogen reagent species is too large for it to be considered dilute. 3 RESULTS AND DISCUSSION 3.1 Model validationIn order to ascertain proper functioning of the model, the operation conditions and boundary condi-tions applied by Habuka et al . [24] were used, so that the computed results can be compared with those ob-tained previously for the flat reactor [Fig. 1 (a)] [41].The comparison between the average growth rates obtained from various models at the flat substrate is presented in Fig. 2. It can be derived that the model predicts the growth rate. More detailed can be seen in Ref. [42].Figure 2 Growth rates obtained from the model presented and those presented in the literature (T s =1389 K, p =0.1 MPa) △ the present model; × Habuk calculated in Ref. [24]; ○ Habuk measured in Ref. [24]; Coso calculated in Ref. [41]3.2 Velocity boundary layerSome work [12, 32, 43, 44] revealed that the tilted susceptor can produce a greater deposition rate and a more uniform distribution of material than a non-tilted susceptor. Cheng and Hsiao [12] also concluded that the deposition rate can be improved if a rib was placed at the center of the susceptor. So we have selected the three reactors (shown in Fig. 1) to detect the effects of the various boundary layers on polycrystalline silicon CVD in the TCS-H 2 system. The follow results are obtained with the same boundary conditions at the three reactors, which were employed that an inlet ve-locity of 0.67 m·s −1, an inlet TCS mole concentration of 0.05, inlet temperature of 300 K, surface tempera-ture of the substrate of 1398 K and the atmospheric pressure (as applied in [24]).The deposition rates along the three substrates are shown in Fig. 3. The average deposition rates at tilted substrate and at the substrate in the rib reactor reach 8.0 and 10.4 µm·min −1, which are 1.5 and 1.9 times that at the flat substrate, respectively. The polysilicon deposition rate along the flat substrate is large firstly and then becomes smaller. For the tilted substrate and the substrate in the rib reactor, the varia-tion trend of deposition rate is similar to that at the flat substrate. And there is a hump at the center at the sub-strate in the rib reactor. The growth rates at the tail section of the substrates are all decreasing, and that at tilted substrate sharply decrease. Generally, this dif-ference in deposition rate is mostly considered due to a change in the velocity boundary layer thickness along the substrate [16, 45, 46]. The nominal boundary layer is thin at the beginning and increases along the substrate (qualitatively shown as Fig. 4), and it is the most thinnest at the center of the substrate with addi-tional rib, that leads to a difference in the deposition rate as shown in Fig. 3.And the velocity boundary layer characteristics can not explain the change of the deposition rate at the beginning and at the tail section of the substrates, but also not explain that the deposition rates at the tilted substrate and at the substrate with additional rib areChin. J. Chem. Eng., Vol. 19, No. 1, February 2011 5higher than that at the flat substrate. 3.3 Profiles of Nu numberIt has been shown that the distribution of the lo-cal heat flux on the susceptor can be closely related tothe growth rate and the uniformity of the deposition in the CVD reactor [12, 44]. Therefore, the local heat flux, in terms of Nusselt number (Nu ), on the heated sub-strate is calculated to identify the effects of the sub-strate tilting and the additional rib on the heat flux and the uniformity of deposition. Fig. 5 shows the com-parison of the isotherm at the three CVD reactors. Itcan be seen that the tilted substrate and the additional rib result in thinner boundary layer and higher tem-perature gradient. The retardation of the growth ther-mal boundary layer and the increase of temperature gradient could yield an increased average heat flux. The computed Nusselt number distributions along the three substrates are shown in Fig. 6. It can be seen that the Nusselt numbers are large at the frontier and small at the tail section. This non-uniformity is due to the heat dissipation through the substrate, and the tem-perature decrease sharply along the substrates. This phenomenon can explain that the deposition rates are low at the frontier and the tail section.We conclude that there is a similar trend between the deposition rate and the local heat flux along the substrate [Figs. 3 and 6 (b)]. The Nusselt numbers at the tail section of the substrate in the rib reactor are less than those at the same section of the tilted sub-strate. The deposition rate also has the same trend.Comparisons of the computed results for the tilted substrate reveal that the converging channels is in favor of an increase of heat transfer as compared to a flat substrate. Moreover, this geometry can effec-tively improve the uniformity of heat flux distribution and deposition rate on the substrate. The uniformity of heat flux distribution at the substrate in the rib reactor is worse. But the addition of the rib enhances the heat flux on the substrate. The mean Nesselt numbers at the tilted substrate and at the substrate with rib are 90.2, 102.1, which are 1.99 and 2.25 times that at the flat substrate, respectively. The local heat transfer on the substrate is taken as a qualitative measure of the depo-sition rate, and the enhancement of heat transfer rate could lead to the improvement of deposition rate [12]. The results are consistent with the theory. 3.4 Mass transferHowever, this kind of understanding is not com-prehensive enough. For these CVD systems, the diffu-sion rates of the reactant through the boundary layer and the diffusion out through this layer of the gaseous by-products have primary effect on the deposition progress. Habuka et al . [47] considered that transport of TCS molecules to the substrate surface plays a dominant role in the silicon epitaxial growth in the TCS-H 2 system and concluded that the dominant chlorosilane species in the system is TCS gas, whereas HCl gas is a major product in a TCS-H 2 system.In order to investigate the mass boundary layers of the above main species, we defined the seven equi-distant lines (named as a, b, c, d, e, f and g in Fig. 1) in the three reactors, respectively, which are from sub-strate to the upper wall, and perpendicular to the sub-strates. The molar concentration distributions of the two substances in the three reactors are shown in Figs. 7 and 8, respectively.Figure 7 plots the molar concentration of TCSagainst the vertical position above the substrates. ItFigure 3 Comparison of polysilicon deposition rates along the substrate ☆ flat; ■ rib; △tilted(a) Flat reactor(b) Tilted reactor(c) Rib reactorFigure 4 Velocity distributions in the three CVD reactorsChin. J. Chem. Eng., Vol. 19, No. 1, February 20116 can be seen that the thickness of the species boundary layer extends from about 10 mm to the entire channel in the so-called horizontal reactor. Furthermore, the molar fraction of TCS at the flat substrate is almost equal to zero especially at the b, c, d, e, f lines, and the value near the up wall reaches to 0.088, which is 1.6 times that at the inlet. This means that much of TCS gas was pushed away from the substrate and was con-densed near the upper left wall. The results show that the deposition rate at these conditions is severely re-stricted by the diffusion rate of TCS.But there is no such phenomenon in the other two reactors. The TCS molar faction in the two reactors is still less than 0.05, even though it begins to decrease until d line in the rib reactor. The boundary layer thickness of TCS on the substrate in the rib reactor is less than 10 mm until line e. The thickness at tilted substrate slowly increases from 7 mm to 12 mm along the substrate. Especially, the molar factions of TCS at these two substrates maintain a relatively higher value, which approach four times higher than that at the flat substrate. It is beneficial to TCS gas getting chemi-sorbed on the silicon substrate surface. The compari-son between the TCS boundary layer thickness on thethree substrates is shown in Fig. 9. The concentration gradient of TCS decreases due to the convergence of the tilted substrate at the tail section in the tilted reac-tor, rather than increases in the flat and rib reactors. The TCS boundary layer thickness has reverse varia-tion trend with the deposition rate on the substrates.Fig. 8 shows the molar concentration of HCl pro-files on those seven lines (seen in Fig. 1) above the flat substrate, the tilted substrate and the substrate in the rib reactor. The behavior of HCl gas produced and the concentration of this gas are very important for dis-cussing the details of the CVD processes [47]. It can be seen that the average HCl molar faction at the tilted substrate and at the substrate in the rib reactor are ap-proximately 2.07 and 2.06 times that at the flat substrate. There is some discrepancy with Habuka’s conclusion [47] that the concentration of HCl gas produced in the reactor increases in proportion to silicon growth rate. 3.5 Adverse role of HClThe negative effect of HCl gas inducing the sili-con etching must be considered. The etching rate at(a) Flat reactor(b) Tilted reactor(c) Rib reactorFigure 5 Computed isotherms in the three CVD reactors(unit of K)(a) Nu distribution along the substrates (b) The local enlargement of the shaded part in Fig. 6 (a)Figure 6 Comparisons of the Nusselt numbers along the substratesflat substrate; tilted substrate; addition ribChin. J. Chem. Eng., Vol. 19, No. 1, February 2011 7each temperature increasing with the hydrogen chlo-ride concentration was observed [48]. So the immedi-ate diffusion out of HCl gas produced from the species boundary layer is favorable to the deposition progress. It can be observed that the concentration of HCl gas at the substrate with additional rib is almost equal to that at the tilted substrate, even a little higher. But the re-verse trend was observed between the deposition rateand the concentration of HCl gas at the two last lines (i .e . f, g lines seen in Fig. 8). Though the molar con-centration of HCl gas at the substrate in the rib reactor increases slightly, the differential concentration of HCl gas decreases rapidly after d line as shown in Fig. 8. The diffusing rate of HCl gas would reduce, and the silicon etching rate increases, which reduce the depo-sition rate at the tail section of the substrate, especially on the substrate in the rib reactor.Now, it can be concluded that the great difference between the deposition rates in the three reactors re-sulted from the combined effect of the momentum, thermal and mass transfer. The deposition rate de-creases with increasing the momentum, thermal and mass boundary layer thickness. The mean concentra-tions of TCS at the substrate tilting and at the substrate with rib are 3.82, 4.18 times that at the flat substrate, respectively, which also reflect qualitatively the depo-sition rate. At the middle of the substrates, though the difference between the concentration of TCS at the substrate tilting and at the substrate with rib is only less than 20.2%, the boundary layer thickness of TCS at the substrate with rib is less 50% than that at thesubstrate tilting, which may be explained that there isFigure 7 Profiles of concentration of TCS above the sub-strate for different linesflat substrate; tilted substrate; addition ribFigure 8 Profiles of concentration of HCl above the sub-strate for different linesflat substrate; tilted substrate; addition ribFigure 9 Variation of SiHCl 3 boundary layer thickness on the three substrates□ flat reactor; △ tilted reactor; ☆ rib reactorChin. J. Chem. Eng., Vol. 19, No. 1, February 20118 a hump of the deposition rate at the substrate with rib. The boundary layer thickness of TCS at tail section in rib reactor is thicker (up to 22.2%) than that in tilted reactor, which may cause that the deposition rates at tail section in rib reactor is less (up to 10.8%) than corresponding values in the tilted reactor. 4 CONCLUSIONSA two-dimensional model with gas phase and surface reactions is used to study the effects of mo-mentum, thermal and species boundary layer on the polycrystalline silicon deposition characteristics in the TCS-H 2 system in three reactors of different geometry. The model validation based on the experimental and numerical data in the open literature was satisfactory. Our numerical simulations show that the momentum boundary layer has significant impact on the thermal boundary layer characteristics. Thermal boundary layer thickness can reflect qualitatively the deposition rate. The concentration of TCS gas at the substrate should maintain a relatively higher value in a good CVD reactor. After the formation of polycrystalline silicon, the HCl gas should be removed from the sur-face of the substrate immediately; otherwise, the deposition rate would be reduced. NOMENCLATUREA pre-exponential factor E energy per mass gas, J·kg −1 E a activation energy, J·mol −1 F i mass force, N g gravity acceleration, m·s −2 H distance from the substrate, cm h rib height, m j J ′ diffusive flux of the species j ′, mol·m −2·s −1 k eff effective thermal conductivity coefficient l distance along the substrate, m p static pressure, Pa R gas constant, 8.314 J·mol −1·K −1 R i net rate of production of the species i r deposition rate, µm·min −1 S h the heat of chemical reaction, JS ithe rate of creation by addition from the dispersed phase T gas temperature, K T s substrate temperature, K u gas velocity, m·s −1 w rib width, mx coordinate, m 2H x mole fraction of H 2 gas x HCl mole fraction of HCl gas 3SiHCl x mole fraction of TCS gas Y i the mass concentration of the species i *i Y fine-scale species mass fraction α tilted angle β temperature exponent ρ density, kg·m −3 σ free surface site τij stresses tensor, PaREFERENCES1 Moffat, H., Jensen, K., “Three-dimensional flow effects in silicon CVD in horizontal reactors”, J . Electrochem . Soc ., 135, 459-466 (1988).2 Vanka, S., Luo, G ., Glumac, N., “Parametric effects on thin film growth and uniformity in an atmospheric pressure impinging jet CVD reactor”, J . Cryst . Growth , 267, 22-34 (2004).3 Oh, I., Takoudis, C., Neudeck, G ., “Mathematical modeling of epi-taxial silicon growth in pancake Chemical Vapor Deposition reac-tors”, J . Electrochem . Soc ., 138, 554-562 (1991).4Kuwana, K., Andrews, R., Grulke, E.A., Saito, K., “CFD analysis on a vortex enhanced CVD reactor design”, in Symposium on Making Functional Materials with Nanotubes held at the 2001 MRS Fall Meeting, P. Bernier, P. Ajayan, Y . Iwasa, P. Nikolaev, eds, Materials Research Society, Boston, MA, 61-66 (2001).5 Wang, A.Y ., Lee, K., Sun, C., Wen, L.S., “Simulations of the de-pendence of gas physical parameters on deposition variables during HFCVD diamond films”, J . Mater . Sci . Technol ., 22, 599-604 (2006). 6Luo, G ., Vanka, S.P., Glumac, N., “Fluid flow and transport proc-esses in a large area atmospheric pressure stagnation flow CVD re-actor for deposition of thin films”, Int . J . Heat Mass Transfer , 47, 4979-4994 (2004).7 Oda, A., Suda, Y ., Okita, A., “Numerical analysis of pressure depend-ence on carbon nanotube growth in CH 4/H 2 plasmas”, Thin Solid Films , 516, 6570-6574 (2007).8 Leakeas, C.L., Sharif, M.A.R., “Effects of thermal diffusion and substrate temperature on silicon deposition in an impinging-jet CVD reactor”, Numer . Heat Tranfer A Appl ., 44, 127-147 (2003).9 Zhuang, Q., Guo, H., Heberlein, J., Pfender, E., “Effect of substrate temperature distribution on thermal plasma jet CVD of diamond”, Diamond Relat . Mater ., 3, 319-324 (1994).10Xu, Q., Baciou, L., Sebban, P., Gunner, M., “Exploring the energy landscape for Q(A)(-) to Q(B) electron transfer in bacterial photo-synthetic reaction centers: Effect of substrate position and tail length on the conformational gating step”, Biochemistry , 41, 10021-10025 (2002).11 Sharifi, Y ., Achenie, L.E.K., “Effect of substrate geometry on the deposition rate in chemical vapor deposition”, J . Cryst . Growth , 304, 520-525 (2007).12 Cheng, T.S., Hsiao, M.C., “Numerical investigations of geometric effects on flow and thermal fields in a horizontal CVD reactor”, J . Cryst . Growth , 310, 3097-3106 (2008).13 Asmann, M., Borges, C., Heberlein, J., Pfender, E., “The effects of substrate rotation on thermal plasma chemical vapor deposition of diamond”, Surf . Coat . Technol ., 142, 724-732 (2000).14Salinger, A.G., Pawlowski, R.P., Shadid, J.N., van Bloemen Waand-ers, B.G ., “Computational analysis and optimization of a chemical vapor deposition reactor with large-scale computing”, Ind . Eng . Chem . Res ., 43, 4612-4623 (2004).15Kunz, T., Burkert, I., Auer, R., Lovtsus, A.A., Talalaev, R.A., Makarov, Y .N., “Convection-assisted chemical vapor deposition (CoCVD) of silicon on large-area substrates”, J . Cryst . Growth , 310, 1112-1117 (2008).16 Kleijn, C., Dorsman, R., Kuijlaars, K., Okkerse, M., van Santen, H., “Multi-scale modeling of chemical vapor deposition processes for thin film technology”, J . Cryst. Growth , 303, 362-380 (2006).17 Pierson, H., Handbook of Chemical Vapor Deposition: Principles, Technology, and Applications, Noyes Publications, NY , USA, 32-43 (1999).18 Yang, Y ., Zhang, W., “Kinetic and microstructure of SiC deposited from SiCl 4-CH 4-H 2”, Chin . J . Chem . Eng ., 17, 419-426 (2009).19 Coltrin, M., Kee, R., Evans, G ., “A mathematical model of the fluid mechanics and gas-phase chemistry in a rotating disk chemical vapor deposition reactor”, J . Electrochem . Soc ., 136, 819-829 (1989).20Arora, R., Pollard, R., “A mathematical model for chemical vapor deposition processes influenced by surface reaction kinetics: Appli-cation to low-pressure deposition of tungsten”, J . Electrochem . Soc ., 138, 1523-1537 (1991).21Tanimoto, S., Matsui, M., Kamisako, K., Kuroiwa, K., Tarui, Y ., “Investigation on leakage current reduction of photo-CVD tantalum oxide films accomplished by active oxygen annealing”, J . Electrochem . Soc ., 139, 320-328 (1992).22Kommu, S., Khomami, B., “High-volume single-wafer reactors for。
Empirical processes of dependent random variables
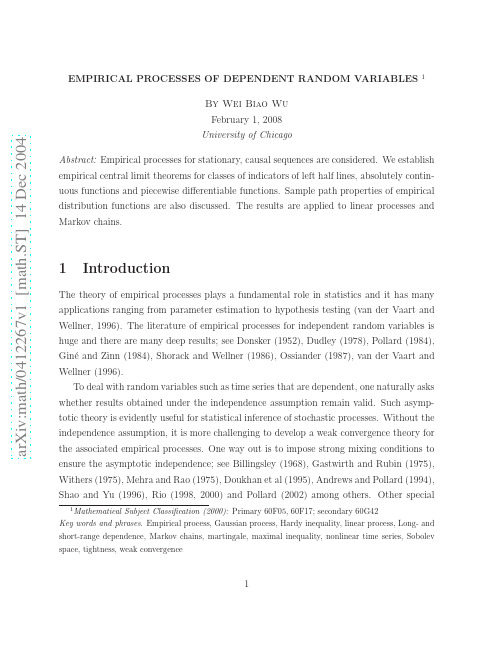
2
Preliminaries
n i=1
from R to R. The centered G -indexed empirical process is given by (P n − P )g = 1 n
n
the marginal and empirical distribution functions. Let G be a class of measurabrocesses that have been discussed include linear processes and Gaussian processes; see Dehling and Taqqu (1989) and Cs¨ org˝ o and Mielniczuk (1996) for long and short-range dependent subordinated Gaussian processes and Ho and Hsing (1996) and Wu (2003a) for long-range dependent linear processes. A collection of recent results is presented in Dehling, Mikosch and Sorensen (2002). In that collection Dedecker and Louhichi (2002) made an important generalization of Ossiander’s (1987) result. Here we investigate the empirical central limit problem for dependent random variables from another angle that avoids strong mixing conditions. In particular, we apply a martingale method and establish a weak convergence theory for stationary, causal processes. Our results are comparable with the theory for independent random variables in that the imposed moment conditions are optimal or almost optimal. We show that, if the process is short-range dependent in a certain sense, then the limiting behavior is similar to that of iid random variables in that the limiting distribution is a Gaussian process and the norming √ sequence is n. For long-range dependent linear processes, one needs to apply asymptotic √ expansions to obtain n-norming limit theorems (Section 6.2.2). The paper is structured as follows. In Section 2 we introduce some mathematical preliminaries necessary for the weak convergence theory and illustrate the essence of our approach. Two types of empirical central limit theorems are established. Empirical processes indexed by indicators of left half lines, absolutely continuous functions, and piecewise differentiable functions are discussed in Sections 3, 4 and 5 respectively. Applications to linear processes and iterated random functions are made in Section 6. Section 7 presents some integral and maximal inequalities that may be of independent interest. Some proofs are given in Sections 8 and 9.
数值迭代法 简单易懂

数值迭代法简单易懂When it comes to numerical iteration methods, one of the most commonly used techniques is the Newton-Raphson method. This method is widely used in various fields such as physics, engineering, and mathematics to find the roots of a given equation. Essentially, the Newton-Raphson method is an iterative process that uses linear approximation to approximate the roots of a function. It is a powerful tool for solving nonlinear equations and is popular due to its rapid convergence rate.谈到数值迭代法,最常用的技术之一是牛顿-拉夫逊法。
这种方法被广泛应用于物理学、工程学和数学等领域,用来寻找给定方程的根。
本质上,牛顿-拉夫逊方法是一种迭代过程,利用线性逼近来近似函数的根。
它是求解非线性方程的强大工具,由于其快速的收敛速度而备受青睐。
The Newton-Raphson method works by starting with an initial guess for the root of the function and then iteratively updating this guess until a satisfactory approximation is obtained. The key idea behind this method is to use the tangent line of the function at a given point to approximate where the root is located. By iteratively updating theguess using this tangent line, the method converges towards the actual root of the function.牛顿-拉夫逊方法的工作原理是从函数的根的初始猜测开始,然后迭代更新这一猜测,直到得到令人满意的近似值。
【2010-2018丘成桐大学生数学竞赛笔试真题】applied2017-individual
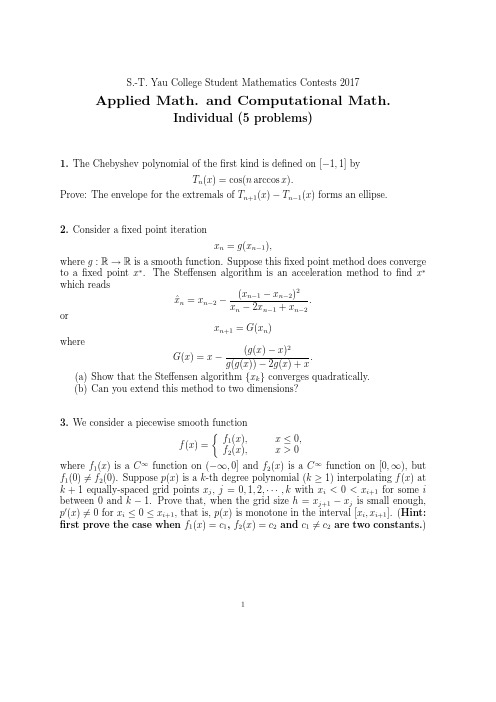
where M is symmetric and positive definite, ω1 > 1, ω2, ..., ωk > 0 and c1, ..., ck−1 ∈ Rn. Deduce from (a) that the iterative scheme converges if and only if all eigenvalues of M (denote it as λ(M )) satisfies:
3. We consider a piecewise smooth function
f (x) =
f1(x), f2(x),
x ≤ 0, x>0
where f1(x) is a C∞ function on (−∞, 0] and f2(x) is a C∞ function on [0, ∞), but f1(0) = f2(0). Suppose p(x) is a k-th degree polynomial (k ≥ 1) interpolating f (x) at k + 1 equally-spaced grid points xj, j = 0, 1, 2, · · · , k with xi < 0 < xi+1 for some i between 0 and k − 1. Prove that, when the grid size h = xj+1 − xj is small enough, p (x) = 0 for xi ≤ 0 ≤ xi+1, that is, p(x) is monotone in the interval [xi, xi+1]. (Hint: first prove the case when f1(x) = c1, f2(x) = c2 and c1 = c2 are two constants.)
线性方程组的迭代解法及收敛分析
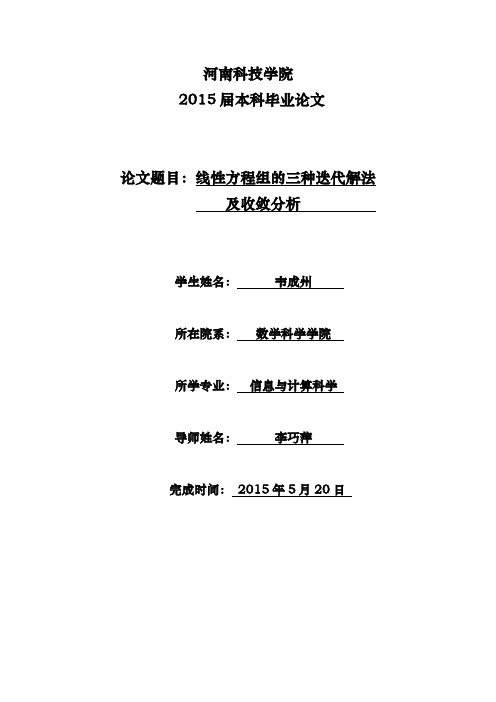
1.9583
0.8468
0.2974
9
1.0975
2.0954
2.8217
1.9788
0.8847
0.2533
10
1.0850
2.0738
2.8671
1.9735
0.8969
0.2041
11
1.0673
2.0645
2.8802
1.9843
0.9200
0.1723
12
1.0577
2.0509
2.9077
1.9828
0.9303
0.1400
13
1.0463
2.0437
2.9191
1.9887
0.9448
0.1174
14
1.0392
2.0350
2.9363
1.9886
0.9527
0.0959
15
1.0318
2.0297
2.9451
1.9920
0.9620
0.0801
16
1.0267
2.0241
Keywords:MATLAB,Mathematical model,Iterative method,ConvergenceSystem of linear equations
1
在实际生活中,存在着大量求解线性方程组的问题。这些方程组具有数据量大,系数矩阵稀疏,在一定精度保证下,只需要求解近似解等特点。线性方程组的迭代解法特别适合于这类方程组的求解,它具有程序设计简单,需要计算机的贮存单元少等特点,但也有收敛性与收敛速度问题。因此,研究线性方程组的迭代解法及收敛分析对于解决实际问题具有非常重要的作用。
krylov子空间方法的分类

krylov子空间方法的分类The Krylov subspace method is a powerful numerical technique used to solve large, sparse linear systems of equations. It is widely used in various engineering and scientific applications, including computational fluid dynamics, structural engineering, and quantum physics. Krylov subspace methods can be categorized into several different types based on their underlying principles and algorithms.Krylov子空间方法是一种强大的数值技术,用于解决大型稀疏线性方程组。
它被广泛应用于各种工程和科学应用中,包括计算流体力学,结构工程和量子物理学。
Krylov子空间方法可以根据其基本原理和算法进行几种不同类型的分类。
One way to classify Krylov subspace methods is based on the type of matrix-vector multiplication used. For example, the conjugate gradient method is a type of Krylov subspace method that uses symmetric positive definite matrices, while the generalized minimal residual method is used for non-symmetric matrices. These methods are designed to efficiently compute approximate solutions to largelinear systems by iteratively building a subspace of the Krylov space generated by the matrix.一种分类Krylov子空间方法的方法是基于使用的矩阵-向量乘法的类型。
梯度下降法更新参数

梯度下降法更新参数英文回答:Gradient descent is an optimization algorithm commonly used in machine learning and deep learning to update the parameters of a model. It is an iterative method that aims to find the minimum of a cost function by adjusting the parameters in the direction of steepest descent.The basic idea behind gradient descent is to compute the gradient of the cost function with respect to each parameter and update the parameters in the opposite direction of the gradient. This process is repeated until the algorithm converges to a minimum or until a predefined number of iterations is reached.The update rule for gradient descent can be expressed as follows:θ = θ α ∇J(θ)。
where θ represents the parameters of the model, α is the learning rate, J(θ) is the cost function, and ∇J(θ) is the gradient of the cost function with respect to θ.The learning rate α determines the step size of each parameter update. A larger learning rate can lead to faster convergence, but it may also cause the algorithm to overshoot the minimum. On the other hand, a smaller learning rate may result in slower convergence, but it can help the algorithm to find a more precise minimum.To illustrate how gradient descent works, let's consider a simple example of linear regression. In linear regression, we aim to find the best-fit line that minimizes the sum of squared differences between the predicted and actual values.Suppose we have a dataset with two features (x1 and x2) and a target variable (y). We initialize the parameters (θ0 and θ1) randomly and compute the cost function J(θ) using the current parameters. Then, we compute thegradients ∇J(θ) with respect to each parameter and update the parameters using the update rule.For each iteration, we calculate the gradients and update the parameters until the algorithm converges or reaches the maximum number of iterations. The algorithm continues to adjust the parameters in the direction of steepest descent, gradually reducing the cost function and improving the model's performance.中文回答:梯度下降法是一种常用的优化算法,用于更新模型的参数。
Accelerated iterative hard thresholding
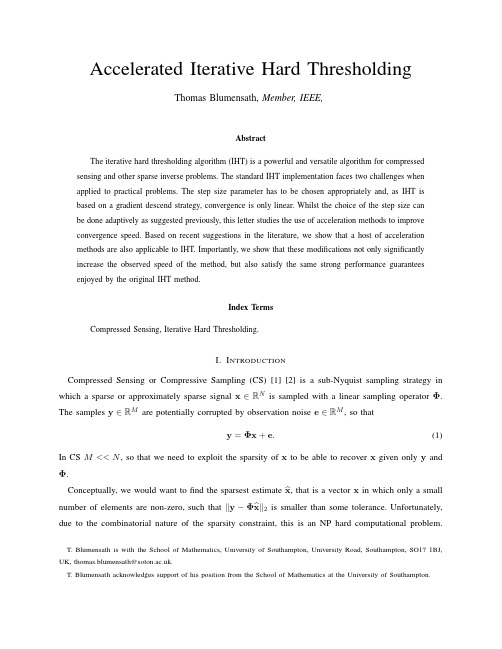
Accelerated Iterative Hard ThresholdingThomas Blumensath,Member,IEEE,AbstractThe iterative hard thresholding algorithm(IHT)is a powerful and versatile algorithm for compressed sensing and other sparse inverse problems.The standard IHT implementation faces two challenges when applied to practical problems.The step size parameter has to be chosen appropriately and,as IHT is based on a gradient descend strategy,convergence is only linear.Whilst the choice of the step size can be done adaptively as suggested previously,this letter studies the use of acceleration methods to improve convergence speed.Based on recent suggestions in the literature,we show that a host of acceleration methods are also applicable to IHT.Importantly,we show that these modifications not only significantly increase the observed speed of the method,but also satisfy the same strong performance guarantees enjoyed by the original IHT method.Index TermsCompressed Sensing,Iterative Hard Thresholding.I.I NTRODUCTIONCompressed Sensing or Compressive Sampling(CS)[1][2]is a sub-Nyquist sampling strategy in which a sparse or approximately sparse signal x∈R N is sampled with a linear sampling operatorΦ. The samples y∈R M are potentially corrupted by observation noise e∈R M,so thaty=Φx+e.(1) In CS M<<N,so that we need to exploit the sparsity of x to be able to recover x given only y and Φ.Conceptually,we would want tofind the sparsest estimate x,that is a vector x in which only a small number of elements are non-zero,such that y−Φ x 2is smaller than some tolerance.Unfortunately, due to the combinatorial nature of the sparsity constraint,this is an NP hard computational problem.T.Blumensath is with the School of Mathematics,University of Southampton,University Road,Southampton,SO171BJ, UK,thomas.blumensath@.T.Blumensath acknowledges support of his position from the School of Mathematics at the University of Southampton.Instead,CS reconstruction is typically solved using either a convex relaxation of the recovery problem [1]such asminxx 1: y−Φ x 2≤ ,(2) or a greedy algorithm such as the Compressive Sampling Matching Pursuit(CoSaMP)[3]or the Iterative Hard Thresholding(IHT)algorithm[4],[9].The IHT algorithm is an iterative methodx n+1=H k(x n+µΦT(y−Φx n)),(3) where H k is the hard thresholding operator that sets all but the k largest(in magnitude)elements1in a vector to zero.IHT is a very simple algorithm and yet,it can be shown that,under certain conditions,IHT can recover sparse and approximately sparse vectors with near optimal accuracy[4].However,in practice,there are two issues with this simple scheme.1)The step sizeµhas to be chosen appropriately to avoid instability of the method and2)IHT has only a linear rate of convergence.Recently,several approaches have been proposed to address these issues([5],[6],[7][8]).In[10],a normalised IHT(NIHT)algorithm was suggested that choosesµadaptively in each iteration.This was shown to guarantees the stability of NIHT.In[10],the step size is set toµ=ΦTΓn(y−Φx n) 22ΦΓnΦT(y−Φx n) 22(4)in each iteration,whereΓn is the support set of x n.Whilst this is sufficient to guarantee convergence under certain RIP conditions[10],if these conditions fail,then an additional line search was proposed in[10] to guarantee stability.A similar approach was suggested in[8],where againµis calculated as in(4),but this time,the setΓis the union of the support of x n and the support of H k(ΦT(y−Φx n)),which again guarantees stability and performance under RIP conditions.Qiu and Dogandzic[5]proposed another approach and analysed the Expectation-Conditional Maximisation Either(ECME)algorithm which is similar to,though not quite identical with,the‘iterative thresholding with inversion’algorithm studied by Maleki[6]x n+1=H k(x n+ΦT(ΦΦT)−1(y−Φx n)),(5) which is guaranteed to converge,as the use of the inverse matrix(ΦΦT)−1guarantees stability,thus circumventing the need to tuneµ.Importantly,as pointed out in[5],if(ΦΦT)is the identity matrix(that1In case the k largest elements are not defined uniquely,Hkis allowed to choose from the offending elements in an arbitrary way.For example,it can use a respecified ordering or random selection.is,if the rows ofΦare orthonormal),then the ECME algorithm is identical to the IHT algorithm with µ=1.Thus,ifΦhas orthonormal rows,then the IHT algorithm withµ=1is guaranteed to be stable (that is,the automatic step-size selection step in IHT is not required in this case).However,if(ΦΦT)is not diagonal,then the ECME algorithm requires the pre-computation and storage of the inverse matrix (ΦΦT)−1,which might not be feasible for certain large scale problems.For these problems,the NIHT algorithm remains an important alternative.Qui and Dogandzic further suggested a double over-relaxation scheme[5]to address the convergence speed issue.After calculating an update x as in(5), x is combined with the two previous estimates x n and x n−1to reduce a specific cost function.The new estimate is then again thresholded.If this newly thresholded estimate has a lower cost than x n+1itself,then this new estimate is accepted,whilst x n+1 is used otherwise.This double relaxation approach(abbreviated DORE)led to a significant improvement in the convergence speed of the method as compared to the IHT algorithm.Furthermore,Qui and Dogandzic[5]provided a performance bound for sparse recovery under a‘2k-sparse subspace quotient condition’2ρ2k=minx: x 0≤2k ΦT(ΦΦT)−1Φx 22x 22>0.5.(6)Inspired by these results and related work in[7]and[8],this letter studies the use of similar acceleration schemes in IHT.As it is not clear when the subspace quotient condition of Qui and Dogandzic holds and how to construct matrices with this property,our main contribution is to analyse the accelerated IHT algorithms based on the Restricted Isometry Property commonly used in CS theory.Importantly,we can show that the accelerated IHT algorithms have exactly the same strong,near optimal recovery results enjoyed by standard IHT.This result is a direct generalisation of a similar result by Foucart derived in [7].II.A CCELERATION OF IHTAs in IHT,we define an accelerated IHT algorithm(AIHT)as any method that calculates an initial updatex n+1=H k(x n+µΦT(y−Φx n)).(7) However,instead of continuing the iterative process with x n+1,following the same reasoning as in[5], we suggest the use of a strategy that tries tofind an estimate x n+1,that satisfies two conditions:2Here and throughout, x 0denotes the number of non-zero elements in the vector x.1)x n+1is k-sparse,2)x n+1satisfies y−Φx n+1 2≤ y−Φ x n+1 2.Any algorithm that calculates such an estimate will be called an accelerated IHT algorithm.One can envisage a range of different approaches to update x n+1.These can be roughly split into two categories,methods that only update the non-zero elements in x n+1and methods that are allowed to update all elements of x n+1but which use a second thresholding step to guarantee the new estimate is k-sparse.Thefirst type of approach is conceptually the simplest.For example,assume the set of non-zero elements in x n+1is Γ.IfΦ Γis the matrix with the columns not in the set Γremoved and if x n+1Γisdefined similarly,then all we need to do is to optimise the cost function y−ΦΓ x 22.This approach hasfirst been proposed and analysed by Foucart in[7].This optimisation can be done for example witha gradient(as in[7])or conjugate gradient algorithm,which when initialised with x n+1Γ,will always produce estimates that satisfy the condition2)above.Importantly,in practice,it is advisable to only run a small number of gradient or conjugate gradient steps in each IHT iteration so not to spend too much time in optimising the cost function in the inner loop(see below).The double-overrelaxation approach of[5]falls into the second category of approaches.The double-over-relaxation approach of[5]uses two relaxation stepsx n+11= x n+1+a1( x n+1−x n),(8) andx n+12= x n+11+a2( x n+11−x n−1),(9) where,for the AIHT algorithm,the line search parameters a1and a2can be calculated in closed form tominimise the quadratic cost function y−Φ x n+11 22and y−Φ x n+1222respectively.With this approach,x n+12is no longer guaranteed to be k-sparse,so that the optimisation step needs to be followed by an additional thresholding step,which in turn is likely to increase the quadratic cost.It can thus happen that y−ΦH k( x n+12) 22> y−Φ x n+1 22,which would violate our second condition.Thus,if y−ΦH k( x n+12) 22> y−Φ x n+1 22,we set x n+1= x n+1whilst we use x n+1=H k( x n+12)otherwise.III.RIP ANALYSIS OF AIHTThe advantage of AIHT methods is that,as long as each estimate x n+1satisfies the two conditions given above,then AIHT has the same performance guarantees as IHT itself.In CS,these guarantees aretypically stated in terms of the Restricted Isometry Constant (RIP).For a given matrix Φ,the Restricted Isometry Constants of order 2k are the largest α2k and smallest β2k ,such thatα2k x 1+x 2 22≤ Φ(x 1+x 2) 22≤β2k x 1+x 2 22(10)holds for all k sparse vectors x 1and x 2.AIHT satisfies the following performance bound that states that,as long as Φhas RIP constants that are not too different,then AIHT can recover any signal x to near optimal accuracy.Theorem 1:For arbitrary x ,given y =Φx +e where Φsatisfies the RIP with β2k ≤µ−1<1.5α2k ,after n = 2log( e 2/ x k 2)log(2/(µα2k )−2)(11)iterations,the AIHT algorithm calculates a solution x n satisfyingx −x n2≤(1+c β2k ) x −x k 2+c β2k x −x k 1√k +c e 2.(12)where c ≤ 43α2k −2µ+1, e =Φ(x −x k )+e and x k is the best k -term approximation to x .Proof:The proof is an extension of the proof in [11]and establishes an upper bound on x −x n +1 2.We here only summarise the main steps,concentrating on those areas that differ from [11].As in [11],we havex −x n +1 2≤ x k −x n +1 2+2α2k ( y −Φx n +1 22+ e 22),(13)where e =Φ(x −x k )+e .The proof of [11]is modified by realising that,by the second condition of the acceleration scheme,any AIHT algorithm satisfiesy −Φx n +1 22≤ y −Φ x n +1 22.(14)It is thus sufficient to bound y −Φ x n +1 22,which can be done as follows (where g =2Φ∗(y −Φx n )).y −Φ x n +1 22− y −Φx n 22=− ( x n +1−x n ),g + Φ( x n +1−x n ) 22≤−2µ ( x n +1−x n ),µ2g +1µ ( x n +1−x n ) 22=1µ x n +1−x n −µ2g 22−µ2 g 22 =1µ inf x : x 0≤0 x −x n −µ2g 22+−µ2g 22=inf x : x 0≤0− (x −x n ),g +1µ (x −x n ) 22 ≤− (x k −x n ),g +1µ(x k −x n ) 22=−2 (x k −x n ),Φ∗(y −Φx n ) +α x k −x n 22+(1µ−α) x k −x n 22≤−2 (x k −x n ),Φ∗(y −Φx n ) + Φ(x k −x n ) 22+(1µ−α) x k −x n 22= y −Φx k 22− y −Φx n 22+(1µ−α) x k −x n 22= e 22− y −Φx n 22+(1µ−α) (x k −x n ) 22.The inequalities are due to (from top to bottom)1)the RIP condition and the choice of β≤1µ,2)the fact that x k is k -sparse and 3)the RIP condition again.The third equality is due to the definition of x n +1=H k (x n +µ2g ).Thus,wrapping up as in [11],we get the bound x −x n +1 22≤ 2µα2k −2 (x k −x n ) 22+4α2ke 22+ x k −x 2(15)Therefore,the condition 2(1µα2k−1)<1implies that x −x n 2≤ 2µα2k −2 n/2 x k 2+√c e 2+ x k −x 2so that the theorem follows using Lemma 6.1in [3].IV.N UMERICAL S IMULATIONSTwo experiments were conducted.In the firs,random matrices Φ∈R 256×512were created with i.i.d.normal entries followed by normalisation of the columns of Φ.For each sparsity k in the interval from 1to 128,1000matrices were generated and k -sparse vectors x were drawn with the k non-zero entries also drawn from the unit variance normal distribution.No noise was added.Two accelerated IHT approach were compared.In the first approach three conjugate gradient steps were used per outer iteration (AIHT CG ),whilst the other approach used the double-over-relaxation method of [5](AIHT DORE ).TheIHT algorithm(NIHT)and the ECME algorithm with the double-over-relaxation(DORE)as proposed in[5]were also used.Both the AIHT as well as the IHT methods used the automatic step-size selection approach which we slightly modified here to reduce the number of line searches.In each iteration,the current proposed step-size was compared to the previously used step-size and the smaller of the two was used.We also relaxed the line search criterion in[10]so that a line search was only initialised when the proposed step sizeµ>1.5( x n+1−x n 22)/( Φ(x n+1−x n) 22).These two modification reduced the number of line searches.For the ECME algorithm,the matrix inverse was precomputed,the cost of which was counted toward the computation time shown.All algorithms were stopped once x n+1−x n 22/N<10−9.The code for the simulations is available on the authors webpage.Figure1shows the average Signal to Noise Ratio(SNR)(top panel)and the average computation time in seconds(lower panel)for each sparsity level k/M.The simulations were run in Matlab on a Intel Core2Duo CPU E85003.16GHz PC.It is clear that both acceleration methods work well with IHT.Both significantly improve the convergence speed of the method,however,ECME is still somewhat faster in this example and also works somewhat better in terms of signal recovery when k/M≈0.35.Though this needs to be contrasted with the fact that the AIHT implementation used here did not require the computation and storage of an inverse matrix,which in some applications can be a significant advantage. Figure2,which shows the average computation time for the above experiment(run on a8GB2.8 GHz Intel Core i7Macbook Pro computer)when AIHT uses1,3,5and10conjugate gradient steps, demonstrate that it is advisable to only use a small number of such steps.The second example used the Shepp-Logan image of size512×512(seefigure3),where between50 to70radial slices were sampled from the2D-Fourier transform of the image which were then used as the measurements y.The image was assumed to be k sparse in the Haar Wavelet domain with k=3769. The algorithms were run with the same parameters as before but stopped once x n+1−x n 22/N<10−16. Figure3,which also gives the results obtained by back-projection,shows the Peak Signal to Noise Ratio(PSNR)for each estimate as well as the computation time(run on a8GB2.8GHz Intel Core i7 Macbook Pro computer).NIHT is seen to be significantly slower than the other approaches.In contrast, using three iterations of a conjugate gradient solver per iteration to accelerate the NIHT algorithm not only significantly reduces the computation time but also lead to significantly better PSNR values.The DORE algorithm,which in this example does not have to use matrix inversion due to the orthogonality of the observation matrix(and is thus identical to our AIHT DORE method),shows comparable performance.Fig.1.Average SNR(20log10 x 2/ x−ˆx 2)and computation time(in seconds)for the four algorithms usingΦ∈R256×512 with i.i.d.normal entries and normalised rows with x only k non-zero entries drawn form a unit variance normal distribution.parison of average computation time for AIHT with1,3,6and10conjugate gradient steps.V.D ISCUSSION AND CONCLUSIONThe Iterative Hard Thresholding algorithms is a simple yet powerful tool to reconstruct sparse signals. Not only does it give near optimal recovery guarantees under the RIP,it is also very versatile and can be easily adapted to a range of constraint sets[11]as well as to non-linear measurement systems[12].Fig.3.Reconstruction accuracy and computation time for the512×512Shepp-Logan phantom image which was sampled taking between50to70equally spaced radial slices from the2D Fourier transform of the image and reconstructed assuming sparsity in the Haar wavelet domain.Shown are the PSNR(20log10 x ∞/ x−ˆx 2)and the computation time in seconds for different ratios of sparsity(k)to number of observations(M).Inspired by the recently developed ECME algorithm,we have here introduced and analysed an accelerated IHT framework.We have in particular looked at two acceleration strategies,the use of a conjugate gradient method and the use of the double-over-relaxation approach of[5],though other approaches can equally well be slotted into the AIHT algorithm.Our main contribution here was to show that, if done correctly,then any accelerated IHT algorithm inherits the strong performance guarantees from the IHT algorithm.Furthermore,combining these acceleration methods with NIHT significant increased the algorithm’s convergence speed,making the accelerated NIHT algorithm a strong competitor to the ECME method.Importantly,the accelerated NIHT method is extremely simple to implement and does not require the computation,storage and repeated use of matrix inverses.This is an advantage in many compressed sensing applications where the measurement matrix is often based on fast transforms such as the wavelet and Fourier transform.R EFERENCES[1] E.Cand`e s and J.Romberg,“Practical signal recovery from random projections,”in Proc.SPIE Conf.,Wavelet Applicationsin Signal and Image Processing XI,Jan.2005.[2] D.Donoho,“Compressed sensing,”IEEE Trans.on Information Theory,vol.52,no.4,pp.1289–1306,2006.[3]Needell D,Tropp JA.CoSaMP:Iterative signal recovery from incomplete and inaccurate samples.Applied andComputational Harmonic Analysis.2008May;26(3):301–321.[4]T.Blumensath and M.Davies,“Iterative hard thresholding for compressed sensing,”Applied and Computational HarmonicAnalysis,vol.27,no.3,.pp.265–2742009.[5]K.Qiu and A.Dogandzic,“ECME Thresholding Methods for Sparse Signal Reconstruction,”arXiv,no.1004.4880v3.[6] A.Maleki“Coherence Analysis of Iterative Thresholding Algorithms,”sin Proc.of the47th Annual Allerton Conferenceon Communication,Control,and Computing,pp.236–241,2009[7]S.Foucart“Hard thresholding pursuit:an algorithm for compressive sensing,”submitted[8]V.Cevher“On Accelerated Hard Thresholding Methods for Sparse Approximation,”EPFL Tec Rep.,2011.[9]T.Blumensath and M.E.Davies,“Iterative thresholding for sparse approximations,”Journal of Fourier Analysis andApplications,vol.14,no.5,pp.629–654,2008.[10]T.Blumensath and M.E.Davies,“Normalised Iterative Hard Thresholding;guaranteed stability and performance,”IEEEJournal of Selected Topics in Signal Processing,vol.4,no.2,pp.298–309,2010.[11]T.Blumensath,“Sampling and reconstructing signals from a union of linear subspaces,”to appear in IEEE Transactionson Information Theory,2011.[12]T.Blumensath,“Compressed Sensing with Nonlinear Observations,”submitted,2011.。
计量经济学中英文词汇对照

Controlled experiments Conventional depth Convolution Corrected factor Corrected mean Correction coefficient Correctness Correlation coefficient Correlation index Correspondence Counting Counts Covaห้องสมุดไป่ตู้iance Covariant Cox Regression Criteria for fitting Criteria of least squares Critical ratio Critical region Critical value
Asymmetric distribution Asymptotic bias Asymptotic efficiency Asymptotic variance Attributable risk Attribute data Attribution Autocorrelation Autocorrelation of residuals Average Average confidence interval length Average growth rate BBB Bar chart Bar graph Base period Bayes' theorem Bell-shaped curve Bernoulli distribution Best-trim estimator Bias Binary logistic regression Binomial distribution Bisquare Bivariate Correlate Bivariate normal distribution Bivariate normal population Biweight interval Biweight M-estimator Block BMDP(Biomedical computer programs) Boxplots Breakdown bound CCC Canonical correlation Caption Case-control study Categorical variable Catenary Cauchy distribution Cause-and-effect relationship Cell Censoring
迭代法的收敛定理
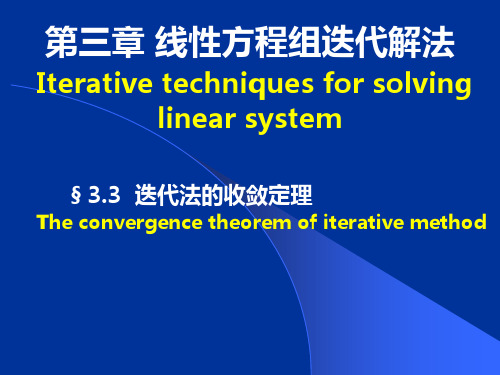
一、基本收敛定理
The Fundamental convergence theorem
Theorem :for any initial value x (0) R n, the fundamental iterative method defined
by x(k+1)=Bx(k)+f (k=0,1,2,…) converges to the unique solution of x=Bx+f if only if
1.25 x1 3.69 x2 12.37 x3 0.58 10.01x1 9.05 x2 0.12 x3 1.43 1.22 x 4.33x 2.67 x 3.22 1 2 3
无法直接判断Jacobi 迭代法和G-S迭代法的收敛性,但如果 将方程组的次序修改为
对角占优矩阵
diagonally dominant matrix
如果线性方程组AX=b的系数矩阵A具有某种特殊性质 (如对称正定、对角占优等),则可从A本身直接得出某些 迭代法收敛性结论。 定义3.1 如果矩阵A满足条件
aii aij
j i
(i 1,2,
, n)
(2)
则称A是严格对角占优阵(strictly diagonally dominant matrix); 如果矩阵A满足条件 aii aij (i 1,2, , n) (3)
在偏微分方程数值解中,有限差分往往导出对角占优的 线性代数方程组,有限元法中的刚性矩阵往往是对称正定阵, 因此这两个判断定理是很实用的。 对于给定的线性方程组,借助于定理3.3和定理3.4可 以直接判断Jacobi 迭代法和G-S迭代法的收敛性。 但同时应当注意,迭代法收敛与否与方程组中方程排列 顺序有关,如线性方程组
离散周期lyapunov方程和离散周期riccati方程的迭代算法

摘要作为线性时变系统的最简单形式,线性周期系统由于其广泛的应用,一直是学者们研究的热点。
线性周期系统,是一类系数矩阵带有周期性的线性系统,在各个领域中都有着广泛的应用。
为了研究离散周期系统的稳定性问题,离散周期Lyapunov方程的求解就显得至关重要。
同样,在进行离散周期系统的线性二次最优状态反馈控制器的设计时,需要用到离散周期Riccati方程的解。
基于这样的研究背景,本文针对离散周期系统下的Lyapunov方程和Riccati方程,给出了其求解的迭代算法。
针对离散周期Lyapunov方程,推导出了相应的迭代算法,分别对零初始条件和任意初始条件的情况给出了严谨的收敛性证明,并通过数值仿真验证了算法的有效性。
并且将最新估计信息的思想引入了迭代算法,得到了新的基于最新估计信息的迭代算法,同样对给出了算法在零初始条件下和非零初始条件下,迭代算法的严谨的收敛性证明,利用数值仿真例子证明了算法是有效并且收敛的。
并且通过对两种算法的数值仿真对比发现,基于最新估计信息的迭代算法的收敛速度要快于原始的迭代算法,从而验证了加入最新估计信息的迭代算法的优越性。
针对推导出的离散周期Riccati方程的迭代算法,给出了其在零初始条件下的收敛性证明,并通过数值仿真验证了算法的有效性,同样,为了改进算法,加入了最新估计信息,得到了新的基于最新估计信息的迭代算法。
同样对该算法的收敛性进行了严谨的证明与数值仿真验证,说明了该算法是有效可用的。
针对两种方程的迭代算法,为了研究最新估计信息对迭代算法的影响程度,引入了加权的思想,得到了带权重因子的新的迭代算法,并进行了收敛性证明。
通过数值仿真,给出了不同权重因子下的收敛性曲线,通过对比可以看出当全部使用最新估计信息时,算法的收敛速度最快,由此可见,加入最新估计信息能有效提高迭代算法的收敛速度。
关键词:离散周期系统;Lyapunov方程;Riccati方程;迭代算法AbstractAs the simplest form of time-varying linear systems, periodic linear systems have been attracting much attention during the past several decades. This is partially because this type of systems has very wide application. To investigate the stabilization problem of the periodic linear systems, it is important to achieve the solution of the periodic Lyapunov matrix equation. Similarly, the design of linear quadratic optimal state feedback controller based on the robust control is related to the stabilizing positive definite solution of Riccati equation. Based on this research background, we propose iterative algorithms for solving discrete-time periodic Lyapunov matrix equation and discrete-time periodic Riccati matrix equation.Iterative algorithms for discrete periodic Lyapunov equations are derived, respectively to the zero initial conditions and arbitrary initial conditions. And the proof of convergence is given. The effectiveness of the algorithm is verified by numerical simulation. And the latest information estimation theory is into the iterative algorithm, the proof of the convergence is also given. The validity of the algorithm is verified by numerical simulations. Finally, the simulation analysis of the two algorithms find that the convergence rate of the iterative algorithm based on the estimation of the latest information is faster than the original algorithm. It proves the superiority of the iterative algorithm adding the latest information of the estimation.Iterative algorithm for discrete periodic Riccati equations is derived, given the zero initial condition of convergence, and the effectiveness of the algorithm is verified through numerical simulation. In order to improve the algorithm with the latest estimate information, a new iterative algorithm based on the information of the latest estimation is given. The convergence of the new algorithm is proved and the validity of the algorithm is verified by numerical simulation. Through numerical simulation, the convergence curves of different weighting factors are given. It found that using the latest estimate information, the convergence speed is the fastest. Therefore, adding the latest estimation information can effectively improve the convergence speed of iterative algorithm.Key words:discrete-time linear periodic system,periodic Lyapunov equations,periodic Riccati equations,iterative algorithms目录摘要 (I)ABSTRACT ..................................................................................................................... I I 第1章绪论 . (1)1.1课题的来源及研究的背景意义 (1)1.2国内外在该方向上的研究现状及分析 (2)1.3本文的主要研究内容 (6)第2章离散周期系统Lyapunov方程快速迭代算法 (8)2.1相关的概念与性质 (8)2.2原始迭代算法 (9)2.2.1显式迭代算法 (9)2.2.2数值仿真 (12)2.3基于最新估计信息的迭代算法 (16)2.3.1显示迭代算法 (16)2.3.2数值仿真 (19)2.4本章小结 (24)第3章离散周期Riccati方程的迭代算法 (25)3.1相关的概念与性质 (25)3.2问题的描述 (25)3.3原始迭代算法 (25)3.3.1显示迭代算法 (26)3.3.2数值仿真 (28)3.4基于最新估计信息的迭代算法 (29)3.4.1显示迭代算法 (30)3.4.2数值仿真 (32)3.5本章小结 (34)第4章离散周期Riccati方程的加权最新估计迭代算法 (35)4.1 带加权因子的快速迭代算法 (35)4.2数值仿真 (37)4.3本章小结 (39)结论 (40)参考文献 (41) (45)致谢 (46)第1章绪论1.1课题的来源及研究的背景意义随着对控制系统的研究越来越深入,人们发现,许多生活中的系统是线性周期系统。
分裂可行问题自适应步长惯性球松弛CQ算法
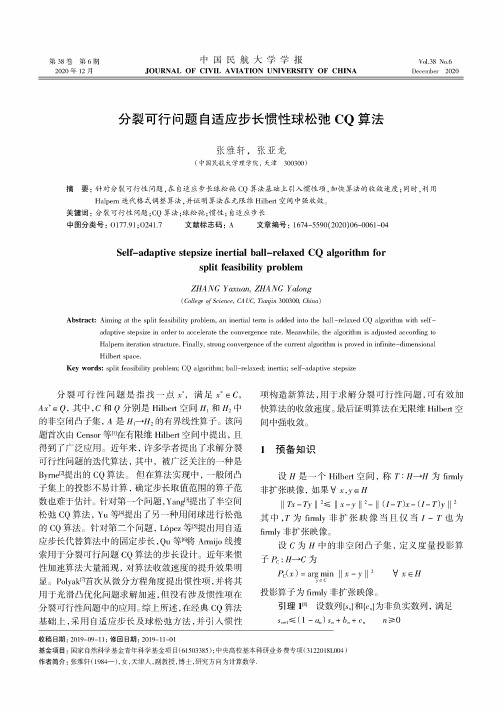
第38卷第6期2020年12月Vol.38No.6December2020中国民航大学学报JOURNAL OF CIVIL AVIATION UNIVERSITY OF CHINA分裂可行问题自适应步长惯性球松弛CQ算法张雅轩,张亚龙(中国民航大学理学院,天津300300)摘要:针对分裂可行性问题,在自适应步长球松弛CQ算法基础上引入惯性项,加快算法的收敛速度;同时,利用Halpern迭代格式调整算法,并证明算法在无限维Hilbert空间中强收敛遥关键词:分裂可行性问题;CQ算法;球松弛;惯性;自适应步长中图分类号:O177.91;O241.7文献标志码:A文章编号:1674-5590(2020)06-0061-04Self-adaptive stepsize inertial ball-relaxed CQ algorithm forsplit feasibility problemZHANG Yaxuan,ZHANG Yalo昭(College of Science,CA UC,Tian^'in300300,China)Abstract:Aiming at the split feasibility problem,an inertial term is added into the ball-relaxed CQ algorithm with self-adaptive stepsize in order to accelerate the convergence rate.Meanwhile,the algorithm is adjusted according to Halpern iteration structure.Finally,strong convergence of the current algorithm is proved in infinite-dimensional Hilbert space.Key words:split feasibility problem;CQ algorithm;ball-relaxed;inertia;self-adaptive stepsize分裂可行性问题是指找一点X*,满足X*沂C, A x*沂Q,其中,C和Q分别是Hilbert空间H和H中的非空闭凸子集,A是H1寅H2的有界线性算子。
有效衰变常数法 氡析出率
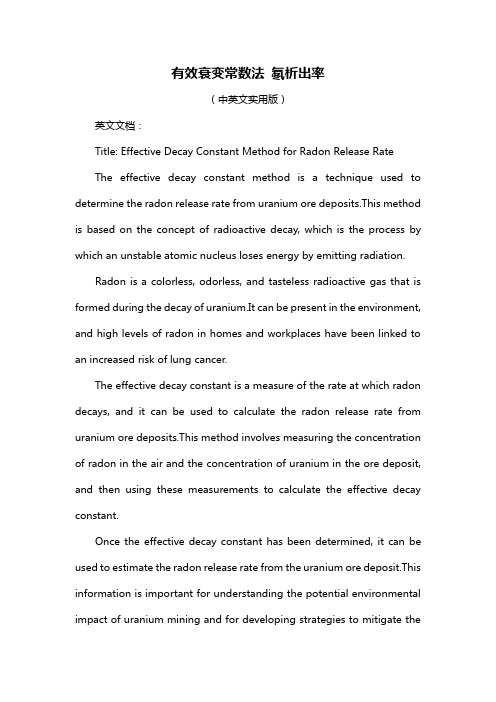
有效衰变常数法氡析出率(中英文实用版)英文文档:Title: Effective Decay Constant Method for Radon Release RateThe effective decay constant method is a technique used to determine the radon release rate from uranium ore deposits.This method is based on the concept of radioactive decay, which is the process by which an unstable atomic nucleus loses energy by emitting radiation.Radon is a colorless, odorless, and tasteless radioactive gas that is formed during the decay of uranium.It can be present in the environment, and high levels of radon in homes and workplaces have been linked to an increased risk of lung cancer.The effective decay constant is a measure of the rate at which radon decays, and it can be used to calculate the radon release rate from uranium ore deposits.This method involves measuring the concentration of radon in the air and the concentration of uranium in the ore deposit, and then using these measurements to calculate the effective decay constant.Once the effective decay constant has been determined, it can be used to estimate the radon release rate from the uranium ore deposit.This information is important for understanding the potential environmental impact of uranium mining and for developing strategies to mitigate therisks associated with radon exposure.中文文档:标题:有效衰变常数法测定氡析出率有效衰变常数法是一种用于确定铀矿床中氡析出率的技术。
鞍点问题的等价模型及其求解

鞍点问题的等价模型及其求解张秀梅;王川龙【摘要】In this paper, we equivalently transform the saddle point problem into a new model whose coefficient matrix is a symmetric positive definite matrix. Under similar settings, we compare the proposed SOR method for the equivalent model with the traditional SOR-like method, which are used to solve the saddle point problem, and find that the equivalent model hasa better performance. In addition, we propose a modified Chebyshev accelerative iterative method, whose parameter is obtained from an optimization model while not the conventional Chebyshev polynomial. The convergence of the modified Chebyshev accelerative iterative method is also studied. Finally, a numerical example is given to compare the convergence rate and the iteration number of all kinds of algorithms. The results have showed that the modified Chebyshev accelerative iterative method has a better convergence rate than other accelerative iterative methods.%本文将鞍点问题转化为一个具有对称正定系数矩阵的等价模型。
重根情形的Newton迭代法收敛性加速

重根情形的 Newton 迭代法收敛性加速张保祥(淮阴师范学院数学系 ,江苏淮安 223000)[ 摘 要 ]基于 New ton 迭代法对于求重根具有线性收敛性 ,给出了加速其收敛的方法以及迭代公式 ,收敛速度得到了有效的提高 。
最后从数值实验加以比较 ,此算法是可行的 。
[ 关键词 ]重根 ;New ton 迭代法 ;收敛性 [ 文章编号 ]1008 - 178X (2006) 05 - 0010203[ 中图分类号 ]O242[ 文献标识码 ]A已知与 同时对于重数未知情形下继续使用 Newton 迭代法作用于 g ( x )f ( x )= 最后进行数值实验 ,均得到较好的结果 。
f ′( x ) 1 相关知识 33定义 :的根 x ,记 e n = x n - x ( n = 1 ,2 , ) 为迭代误差 。
若存在常 数 p ≥1 和 是 p 阶收敛的 。
其中 p 的大小刻画了迭代序列 的收敛x n x n 速度 , p 越大 ,收敛的速度越快 。
若 p = 1 ,0 < C < 1 时 ,称序列 是线性收敛的 ;若 1 < p < 2 时称序列 是 x n x n 超线性收敛的 ;若 p = 2 时称序列 引理[ 1 ] : (收敛阶判别方法) x n 是平方收敛的 。
若迭代函数 g ( x ) 在根 x 3 附近有连续的 p ( p> 1) 阶导 数 , 且 g ′( x 3 ) =g ″( x 3 ) = = g( p - 1)( x 3 ) = 0 , g ( p )( x 3 ) ≠0 。
则迭代公式 x = g ( x ) 产生的序列 在 x 3的领域是 p 阶收x n n + 1 n ( p ) 3g ( x ) 敛的 ,且 = ≠0 。
p !n ′设 x n 是非线性方程 f ( x ) = 0 的一个近似根 ,把 f ( x ) 在 x n 处作 1 阶 T ayl or 展式 ,即 f ( x ) ≈ f ( x n ) + f ( x n )′( x - x n ) 。
leapp包的中文名称:Latent Effect Adjustment After Primary

Package‘leapp’October13,2022Version1.3Date2022-06-19Title Latent Effect Adjustment After Primary ProjectionAuthor Yunting Sun<*********************>,Nancy R.Zhang<*******************>,Art B.Owen<*****************>Maintainer Yunting Sun<*********************>Description These functions take a gene expression value matrix,aprimary covariate vector,an additional known covariatesmatrix.A two stage analysis is applied to counter the effectsof latent variables on the rankings of hypotheses.Theestimation and adjustment of latent effects are proposed bySun,Zhang and Owen(2011).``leapp''is developed in thecontext of microarray experiments,but may be used as a generaltool for high throughput data sets where dependence may beinvolved.Depends R(>=3.1.1),sva,MASS,corpcorLicense GPL(>=2)Repository CRANDate/Publication2022-06-1921:10:02UTCNeedsCompilation noR topics documented:leapp-package (2)AlternateSVD (3)FindAUC (4)FindFpr (4)FindPrec (5)FindRec (6)FindTpr (6)IPOD (7)IPODFUN (8)12leapp-package leapp (9)Pvalue (10)ridge (11)ROCplot (12)simdat (13)Index15 leapp-package latent effect adjustment after primary projectionDescriptionThese functions take a gene expression value matrix,a primary covariate vector,an additional known covariates matrix.A two stage analysis is applied to counter the effects of latent variables on the rankings of hypotheses.The estimation and adjustment of latent effects are proposed by Sun, Zhang and Owen(2011)."leapp"is developed in the context of microarray experiments,but may be used as a general tool for high throughput data sets where dependence may be involved. DetailsPackage:leappType:PackageVersion: 1.1Date:2013-01-05License:What license is it under?LazyLoad:yesAuthor(s)Maintainer:Yunting Sun<*********************>See AlsoSun,Zhang and Owen(2011),"Multiple hypothesis testing,adjusting for latent variables"Examples##Not run:library(sva)library(MASS)library(leapp)data(simdat)model<-cbind(rep(1,60),simdat$g)model0<-cbind(rep(1,60))AlternateSVD3 p.raw<-f.pvalue(simdat$data,model,model0)p.oracle<-f.pvalue(simdat$data-simdat$up.leapp<-leapp(simdat$data,pred.prim=simdat$g)$pp=cbind(p.raw,p.oracle,p.leapp)topk=seq(0,0.5,length.out=50)*1000null.set=which(simdat$gamma!=0)fpr=apply(p,2,FindFpr,null.set,topk)tpr=apply(p,2,FindTpr,null.set,topk)ROCplot(fpr,tpr,main="ROC Comparison",name.method=c("raw","oracle","leapp"),save=FALSE)##End(Not run)AlternateSVD Alternating singular value decompositionDescriptionThe algorithm alternates between1)computing latent loadings u and latent variable v and2)esti-mating noise standard deviation for each of the N genes.UsageAlternateSVD(x,r,pred=NULL,max.iter=10,TOL=1e-04)Argumentsx an N by n data matrixr a numeric,number of latent factors to estimatepred an n by s matrix,each column is a vector of known covariates for n samples,s covariates are considered,default to NULLmax.iter a numeric,maximum number of iteration allowed,default to10TOL a numeric,tolerance level for the algorithm to converge,default to1e-04 Valuesigma a vector of length N,noise standard deviations for N genescoef an N by s matrix,estimated coefficients for known covariatesuest an N by r matrix,estimated latent loadingsvest an n by r matrix,estiamted latent factorsAuthor(s)Yunting Sun<*********************>,Nancy R.Zhang<*******************>,Art B.Owen <*****************>4FindFpr FindAUC Compute the area under the ROC curve(AUC)DescriptionGiven a vector of p values for m genes and a set of null genes,compute the area under ROC curve using the Wilcoxin statisticsUsageFindAUC(pvalue,ind)Argumentspvalue A vector of p values,one for each gene,with length mind A vector of indices that the corresponding gene are true positiveValueauc A numeric,area under the ROC curve for the given gene listAuthor(s)Yunting Sun<*********************>,Nancy R.Zhang<*******************>,Art B.Owen <*****************>FindFpr Compute the false positive rate at given sizes of retrieved genesDescriptionGiven a vector of sizes of retrieved genes,for each size k,select the top k genes with smallest p values and compute the false positive rate from the retrieved genes and the true positive genes. UsageFindFpr(pvalue,ind,topk)Argumentspvalue A vector of p values,one for each gene,with length mind A vector of indices that the corresponding gene are true positivetopk A vector of integers ranging from1to m,length of retrieved gene listFindPrec5 Valuefpr A vector of false positive rates at given sizes of retrieval.Author(s)Yunting Sun<*********************>,Nancy R.Zhang<*******************>,Art B.Owen <*****************>FindPrec compute the precision at given sizes of retrieved genesDescriptionGiven a vector of sizes of retrieved genes,for each size k,select the top k genes with smallest p values and compute the precision from the retrieved genes and the true positive genes.UsageFindPrec(pvalue,ind,topk)Argumentspvalue A vector of p values,one for each gene,with length mind A vector of indices that the corresponding gene are true positivetopk A vector of integers ranging from1to m,length of retrieved gene listValueprec A vector of precisions at given sizes of retrieval.Author(s)Yunting Sun<*********************>,Nancy R.Zhang<*******************>,Art B.Owen <*****************>6FindTpr FindRec compute the recall at given sizes of retrieved genesDescriptionGiven a vector of sizes of retrieved genes,for each size k,select the top k genes with smallest p values and compute the recall from the retrieved genes and the true positive genes.UsageFindRec(pvalue,ind,topk)Argumentspvalue A vector of p values,one for each gene,with length mind A vector of indices that the corresponding gene are true positivetopk A vector of integers ranging from1to m,length of retrieved gene listValuerec A vector of precisions at given sizes of retrieval.Author(s)Yunting Sun<*********************>,Nancy R.Zhang<*******************>,Art B.Owen <*****************>FindTpr compute the true positive rate at given sizes of retrieved genesDescriptionGiven a vector of sizes of retrieved genes,for each size k,select the top k genes with smallest p values and compute the true positive rate from the retrieved genes and the true positive genes. UsageFindTpr(pvalue,ind,topk)Argumentspvalue A vector of p values,one for each gene,with length mind A vector of indices that the corresponding gene are true positivetopk A vector of integers ranging from1to m,length of retrieved gene listIPOD7Valuetpr A vector of True positive rates at given sizes of retrieval.Author(s)Yunting Sun<*********************>,Nancy R.Zhang<*******************>,Art B.Owen <*****************>IPOD Iterative penalized outlier detection algorithmDescriptionOutlier detection and robust regression through an iterative penalized regression with tuning param-eter chosen by modified BICUsageIPOD(X,Y,H,method="hard",TOL=1e-04,length.out=50)ArgumentsX an N by k design matrixY an N by1responseH an N by N projection matrix X(X X)^{-1}Xmethod a string,if method="hard",hard thresholding is applied;if method="soft", soft thresholding is appliedTOL relative iterative converence tolerance,default to1e-04length.out A numeric,number of candidate tuning parameter lambda under consideration for further modified BIC model selection,default to50.DetailsIf there is no predictors,set X=NULL.Y=X beta+gamma+sigma epsilonY is N by1reponse vector,X is N by k design matrix,beta is k by1coefficients,gamma is N by 1outlier indicator,sigma is a scalar and the noise standard deviation and epsilon is N by1vector with components independently distributed as standard normal N(0,1).Valuegamma a vector of length N,estimated outlier indicator gammaresOpt.scale a vector of length N,test statistics for each of the N genesp a vector of length N,p-values for each of the N genes8IPODFUNAuthor(s)Yunting Sun<*********************>,Nancy R.Zhang<*******************>,Art B.Owen <*****************>IPODFUN compute the iterative penalized outlier detection given the noise stan-dard deviation sigmaDescriptionY=X beta+gamma+sigma epsilon estimate k by1coefficients vector beta and N by1outlier indicator vector gamma from(Y,X).UsageIPODFUN(X,Y,H,sigma,betaInit,method="hard",TOL=1e-04)ArgumentsX an N by k design matrixY an N by1response vectorH an N by N projection matrix X(X’X)^-1X’sigma a numeric,noise standard deviationbetaInit a k by1initial value for coeffient betamethod a string,if"hard",conduct hard thresholding,if"soft",conduct soft threshold-ing,default to"hard"TOL a numeric,tolerance of convergence,default to1e-04DetailsThe initial estimator for the coefficient beta can be chosen to be the estimator from a robust linear regressionValuegamma an N by1vector of estimated outlier indicatorress an N by1vector of residual Y-X beta-gammaAuthor(s)Yunting Sun<*********************>,Nancy R.Zhang<*******************>,Art B.Owen <*****************>ReferencesShe,Y.and Owen,A.B."Outlier detection using nonconvex penalized regression"2010leapp9 leapp latent effect adjustment after primary projectionDescriptionAdjust for latent factors and conduct multiple hypotheses testing from gene expression data using the algorithm of Sun,Zhang and Owen(2011).Number of latent factors can be chosen by Buja and Eyuboglu(1992).Usageleapp(data,pred.prim,pred.covar,O=NULL,num.fac="buja",method="hard",sparse=TRUE,centered=FALSE,verbose=FALSE,perm.num=50,TOL=1e-4,length.out=50)Argumentsdata An N genes by n arrays matrix of expression datapred.prim An n by1primary predictorpred.covar An n by s known covariate matrix not of primary interestO An n by n rotation matrix such that O pred.prim=(1,0, 0num.fac A numeric or string,number of latent factors chosen.it has default value"buja"which uses Buja and Eyuboglu(1992)to pick the number of factors method A string which takes values in("hard","soft")."hard":hard thresholding in the IPOD algorithm;"soft":soft thresholding in the IPOD algorithm sparse A logical value,if TRUE,the signal is sparse and the proportion of non-null genes is small,use IPOD algorithm in Owen and She(2010)to enforce sparsity.IfFALSE,the signal is not sparse,use ridge type penalty to carry out the inferenceas in Sun,Zhang,Owen(2011).Default to TRUEcentered A logical value,indicates whether the data has been centered at zero,default to FALSEverbose A logical value,if TRUE,will print much information as algorithm proceeds, default to FALSEperm.num A numeric,number of permutation performed using algorithm of Buja and Eyuboglu(1992),default to50TOL A numeric,convergence tolerance level,default to1e-4length.out A numeric,number of candidate tuning parameter lambda under consideration for further modified BIC model selection,default to50.DetailsThe data for test i should be in the ith row of data.If the rotation matrix O is set to NULL,the function will compute one rotation from primary predictor pred.prim.10PvalueValuep A vector of p-values one for each row of data.vest An n by num.fac matrix,estimated latent factorsuest An N by num.fac matrix,estimated latent loadingsgamma An N by1vector,estimated primary effectsigma An N by1vector,estimated noise standard deviation one for each row of data Author(s)Yunting Sun<*********************>,Nancy R.Zhang<*******************>,Art B.Owen <*****************>Examples##Not run:##Load datadata(simdat)#Calculate the p-valuesp<-leapp(simdat$data,pred.prim=simdat$g,method="hard")$pauc<-FindAUC(p,which(simdat$gamma!=0))##End(Not run)Pvalue Calculate statistics and p-valuesDescriptionCalculate F-statistics,t-statistics and corresponding p-values given multiple regression models un-der the null and alternative hypotheses.UsagePvalue(dat,mod,mod0)Argumentsdat An N genes by n arrays matrix of expression datamod An n by(s+1)design matrix under the full model(alternative),thefirst column is the primary predictor,s>=0and the rest of the columns are additional covariates mod0An n by s design matrix under the null hypothesis,s>=0,should be the same as the2:(s+1)columns of mod.If s=0,please set mod0=NULLridge11Valuep An N by1vector of p-values one for each row of data.tstat An N by1vector of t-statistics for primary parameters.fstat An N by1vector of F-statistics for primary parameters.coef An N by(s+1)matrix of coefficients with respect to design matrix mod under the full model.Author(s)Yunting Sun<*********************>,Nancy R.Zhang<*******************>,Art B.Owen <*****************>Examples##Not run:data(simdat)n=ncol(simdat$data)mod=cbind(simdat$g,rep(1,n))mod0=cbind(rep(1,n))result=Pvalue(dimdat$data,mod=mod,mod0=mod0)##End(Not run)ridge Outlier detection with a ridge penaltyDescriptionOutlier detection and robust regression with a ridge type penalty on the outlier indicator gamma.Allow non sparse outliers and require known noise standard deviation.Usageridge(X,Y,H,sigma)ArgumentsX an N by k design matrixY an N by1response vectorH an N by N projection matrix X(X X)^{-1}Xsigma a numeric,noise standard deviationValuep an N by1vector of p-values for each of the N genesgamma an N by1vector of estimated primary variable gamma12ROCplot Author(s)Yunting Sun<*********************>,Nancy R.Zhang<*******************>,Art B.Owen <*****************>ROCplot plot ROC curveDescriptionInput an p by d matrix,each column of which contains false positive rates(FPR)computed from each of the d methods and p significance levels and a matrix of corresponding true positive rates(TPR)at the same significance levels.Plot ROC curve for each of the d methods.UsageROCplot(fpr,tpr,main,name.method,xlim=c(0,0.2),ylim=c(0.4,1),save=TRUE,name.file=NULL) Argumentsfpr A matrix of false positive rates for increasing sizes of retrieved significant genes tpr A vector of corresponding true positive rates at the same significance levels main a string,title of thefigurename.method a string vector of length d containing names of the d methodsxlim the range of the x axis(FPR),default to c(0,0.2)ylim the range of the y axis(TPR),default to c(0.4,1)save a logical value,if TRUE,will save the plot to an pngfile,default to TRUEname.file a string giving the name of the pngfile to save the plotDetailsThe order of the name.method should be the same as that in the fpr and tpr.Author(s)Yunting Sun<*********************>,Nancy R.Zhang<*******************>,Art B.Owen <*****************>Examples##Not run:library(sva)library(MASS)library(leapp)data(simdat)model<-cbind(rep(1,60),simdat$g)model0<-cbind(rep(1,60))p.raw<-f.pvalue(simdat$data,model,model0)p.oracle<-f.pvalue(simdat$data-simdat$up.leapp<-leapp(simdat$data,pred.prim=simdat$g,method="hard")$pp=cbind(p.raw,p.oracle,p.leapp)topk=seq(0,0.5,length.out=50)*1000null.set=which(simdat$gamma!=0)fpr=apply(p,2,FindFpr,null.set,topk)tpr=apply(p,2,FindTpr,null.set,topk)ROCplot(fpr,tpr,main="ROC Comparison",name.method=c("raw","oracle","leapp"),save=FALSE)##End(Not run)simdat Simulated gene expression data affected by a group variable and anunobserved factorDescriptionThis data set is a simulated gene expression matrix with N(0,1)background noise and affected by two variables.gene expression values of1000genes from60samples are simulated.First30 samples are from case group and last30samples are from control group.The primary treatment variable g affects ten percent of the genes and the latent variable affects uniformly on the genes.The correlation between primary treatment variable g and the latent variable is0.5and the SNR= 1,SLR=0.5.The variances of noise across genes are distributed as inverse gamma.Also included in the data are a vector of normalized primary variable g,a vector of primary parameter gamma,a vector of latent factor v,a vector of latent loadings u and a vector of noise standard deviation for all genes sigma.Usagedata(simdat)FormatA list of6componentsValuedata A1000x60gene expression value matrix with genes in rows and arrays in columnsg A vector of length60,primary variablegamma A vector of length1000,primary parameterv A vector of length60,latent variableu A vector of length1000,latent loadingssigma A vector of length1000,noise standard deviation for each of the1000genes Author(s)Yunting Sun<*********************>,Nancy R.Zhang<*******************>,Art B.Owen <*****************>Index∗datasetssimdat,13∗ipodIPOD,7IPODFUN,8∗miscAlternateSVD,3FindAUC,4FindFpr,4FindPrec,5FindRec,6FindTpr,6leapp,9Pvalue,10ridge,11ROCplot,12∗packageleapp-package,2AlternateSVD,3FindAUC,4FindFpr,4FindPrec,5FindRec,6FindTpr,6IPOD,7IPODFUN,8leapp,9leapp-package,2Pvalue,10ridge,11ROCplot,12simdat,1315。
- 1、下载文档前请自行甄别文档内容的完整性,平台不提供额外的编辑、内容补充、找答案等附加服务。
- 2、"仅部分预览"的文档,不可在线预览部分如存在完整性等问题,可反馈申请退款(可完整预览的文档不适用该条件!)。
- 3、如文档侵犯您的权益,请联系客服反馈,我们会尽快为您处理(人工客服工作时间:9:00-18:30)。
CHUN-HUA GUO† Abstract. We prove a convergence result for an iterative method, proposed recently by B. Meini, for finding the maximal Hermitian positive definite solution of the matrix equation X +A∗ X −1 A = Q, where Q is Hermitian positive definite. Key words. matrix equation, maximal Hermitian solution, cyclic reduction, iterative methods, convergence rate AMS subject classifications. 15A24, 65F10, 65H10
1
2
CHUN-HUA GUO
For algebraic Riccati equations, it is well known that the desirable solution is the maximal solution. For (1.2), the maximal solution is also the right choice in view of the presence of X −1 in the equation. The main purpose of the paper is to prove a convergence result for an iterative method proposed by Meini [14] for finding the maximal solution of (1.2). Roughly speaking, our new result together with the results obtained in [14] shows that the convergence of Meini’s method is no slower than Newton’s method. Meini’s method is thus preferable when we try to find the maximal solution of (1.2), since the computational work per iteration for Newton’s method is 5 ∼ 10 times that for Meini’s method. To put our result in a proper setting, we review in Section 2 some theoretical results for the solution of (1.2) and present in Section 3 three iterative methods, with emphasis on Meini’s method. Our convergence result for Meini’s method is then presented in Section 4. The paper ends with some discussions in Section 5. 2. Theoretical background. Necessary and sufficient conditions for the existence of a positive definite solution of (1.2) have been given in [5]. Theorem 2.1. Equation (1.2) has a positive definite solution if and only if the rational matrix function ψ (λ) = λA + Q + λ−1 A∗ is regular (i.e., the determinant of ψ (λ) is not identically zero) and ψ (λ) ≥ 0 for all λ on the unit circle. The existence of the maximal solution of (1.2) has also been established in [5], along with a characterization of the maximal solution. Theorem 2.2. If equation (1.2) has a positive definite solution, then it has a maximal solution X+ . Moreover, X+ is the unique positive definite solution such that X + λA is nonsingular for all λ with |λ| < 1. This result has the following immediate corollary, where ρ(·) is the spectral radius. −1 Corollary 2.3. For the maximal solution X+ of (1.2), ρ(X+ A) ≤ 1; for any −1 other positive definite solution X , ρ(X A) > 1. We also have the following characterization for the eigenvalues of the matrix −1 X+ A (see [8]). −1 Theorem 2.4. For equation (1.2), the eigenvalues of X+ A are precisely the eigenvalues of the matrix pencil I 0 0 0 0 −I λ 0 0 0 − Q −I A ∗ 0 −I 0 −A 0 0 inside or on the unit circle, with half of the partial multiplicities for each eigenvalue on the unit circle. 3. Iterative methods. The maximal solution X+ of (1.2) can be found by the following basic fixed point iteration: Algorithm 3.1. X0 = Q, −1 Xn+1 = Q − A∗ Xn A,
∗ This work was supported in part by a grant from the Natural Sciences and Engineering Research Council of Canada. † Department of Mathematics and Statistics, University of Regina, Regina, SK S4S 0A2, Canada (chguo@math.uregina.ca).
is X itself (see [1]). There is some connection between (1.2) and (1.1). For example, if X is a solution of (1.2), then X −1 A is a solution of A∗ Y 2 − QY + A = 0. Equation (1.2) is also a special case of the discrete algebraic Riccati equation (1.3) −X + C ∗ XC + Q − (A + B ∗ XC )∗ (R + B ∗ XB )−1 (A + B ∗ XC ) = 0,
where A, Q ∈ Cm×m with Q Hermitian positive definite and a Hermitian positive definite solution is required. This equation has been studied recently by several authors (see [1], [4], [5], [8], [14], [16], [17]). For the application areas in which the equation arises, see the references given in [1]. Note also that a solution X of (1.2) is such that the Schur complement of X in the matrix X A∗ A Q
n = 0, 1, . . . .
For Algorithm 3.1, we have X0 ≥ X1 ≥ · · · , and limn→∞ Xn = X+ (see, e.g., [5]). Moreover, the following result is proved in [8]. Theorem 3.2. Let {Xn } be given by Algorithm 3.1. Then lim sup
where A, B, C are given coefficient matrices. This equation has also been the topic of many papers, including two recent papers by Higham and Kim (see [9] and [10]). In this paper, our interest is in the matrix equation (1.2) X + A∗ X −1 A = Q,