Towards Stochastic Conjugate Gradient Methods
英语数学词汇E

数学专业词汇对照以字母 E 开头eccentric angle 离心角eccentric angle of an ellipse 椭圆的离心角eccentric anomaly 离心角eccentricity 离心率eccentricity of a hyperbola 双曲线的离心率echelon matrix 梯阵econometrics 计量经济学eddy 涡流旋涡edge 边edge connectivity 边连通度edge homomorphism 边缘同态edge of a solid 立体棱edge of regression 回归边缘edge of the wedge theorem 楔的边定理editcyclic markov chain 循环马尔可夫链effective area 有效面积effective convergence 有效收敛effective cross section 有效截面effective differential cross section 有效微分截面effective divisor 非负除数effective interest rate 有效利率effective number of replications 有效重复数effective variance of error 有效误差方差effectively computable function 能行可计算函数efficiency 效率efficiency factor 效率因子efficient estimator 有效估计量efficient point 有效点egyptian numerals 埃及数字eigenelement 特摘素eigenfunction 特寨数eigenspace 本照间eigenvalue 矩阵的特盏eigenvalue problem 特盏问题eigenvector 特镇量eigenvector of linear operator 线性算子的特镇量einstein equation 爱因斯坦方程einstein metric 爱因斯坦度量elastic coefficient 弹性常数elastic constant 弹性常数elastic deformation 弹性变形elastic limit 弹性限度elastic modulus 弹性模数elastic scattering 弹性散射elasticity 弹性elastodynamics 弹性动力学electrodynamics 电动力学electromagnetism 电磁electronic computer 电子计算机electronic data processing machine 电子数据处理机electronics 电子学electrostatics 静电学element 元件element of area 面积元素element of best approximation 最佳逼近元素element of finite order 有限阶元素element of surface 面元素elementary 基本的elementary chain 初等链elementary circuit 基本回路elementary conjunction 基本合取式elementary divisor 初等因子elementary divisor theorem 初等因子定理elementary event 简单事件elementary formula 原始公式elementary function 初等函数elementary geometry 初等几何elementary matrix 初等阵elementary number theory 初等数论elementary operation 初等运算elementary path 有向通路elementary set 初等集elementary subdivision 初等重分elementary symmetric function 初等对称函数elementary theory of numbers 初等数沦elevation 正视图eliminant 结式eliminate 消去elimination 消去elimination by substitution 代入消元法elimination method 消元法elimination of unknowns 未知数消去elimination theorem 消去定理ellipse 椭圆ellipse of deformation 变形椭圆ellipsograph 椭圆规ellipsoid 椭面ellipsoid of inertia 惯性椭球ellipsoid of revolution 回转椭面ellipsoid of rotation 回转椭面ellipsoidal 椭面的ellipsoidal coordinates 椭球面]坐标ellipsoidal harmonics 椭球低函数elliptic catenary 椭圆悬链线elliptic coordinates 椭圆坐标elliptic curve 椭圆曲线elliptic cylinder 椭圆柱elliptic cylinder function 椭圆柱函数elliptic differential operator 椭圆型微分算子elliptic equation 椭圆型微分方程elliptic function 椭圆函数elliptic function of the second kind 第二类椭圆函数elliptic function of the third kind 第三类椭圆函数elliptic geometry 椭圆几何elliptic integral 椭圆积分elliptic irrational function 椭圆无理函数elliptic modular function 椭圆模函数elliptic modular group 椭圆模群elliptic motion 椭圆运动elliptic orbit 椭圆轨道elliptic paraboloid 椭圆抛物面elliptic point 椭圆点elliptic quartic curve 椭圆四次曲线elliptic space 椭圆空间elliptic surface 椭圆曲面elliptic system 椭圆型方程组elliptic type 椭圆型ellipticity 椭圆率elongation 伸长embedding 嵌入embedding operator 嵌入算子embedding theorem 嵌入定理empirical curve 经验曲线empirical distribution curve 经验分布曲线empirical distribution function 经验分布函数empirical formula 经验公式empty mapping 空映射empty relation 零关系empty set 空集end 端end around carry 循环进位end device 输出设备endless 无穷的endomorphism 自同态endomorphism group 自同态群endomorphism ring 自同态环endpoint 端点energetic inequality 能量不等式energy 能量energy barrier 能量障碍energy distribution 能量分布energy integral 能量积分energy level 能级energy method 能量法energy momentum tensor 能量动量张量energy norm 能量范数energy operator 能量算子energy principle 能量原理energy space 能量空间energy surface 能面enlarge 扩大enneagon 九边形enriques surface 讹凯斯面ensemble 总体entire 整个的entire function 整函数entire modular form 整模形式entire rational function 整有理函数entire series 整级数entire transcendental function 整超越函数entrance angle 入射角entropy 熵enumerability 可数性enumerable 可数的enumerable set 可数集enumerate 列举enumeration 列举enumeration data 计数数据enumeration problem 列举问题enumerative geometry 枚举几何envelope 包络线envelope of holomorphy 正则包enveloping algebra 包络代数enveloping ring 包络环enveloping surface 包络面epicycle 周转圆epicycloid 外摆线epicycloidal 圆外旋轮线的epimorphic 满射的epimorphic image 满射像epimorphism 满射epitrochoid 长短辐圆外旋轮线epitrochoidal curve 圆外旋轮曲线epsilon chain 链epsilon function 函数epsilon map 映射epsilon neighborhood 邻域epsilon net 网epsilonnumber 数equal 相等的equal set 相等集equal sign 等号equality 等式equality constraint 等式约束equalization 平衡化;同等化equally possible event 相等可能事件equate 使...相等equation 方程equation of a circle 圆方程equation of a curve 曲线方程equation of continuity 连续方程equation of heat conduction 热传导方程equation of higher order 高阶方程式equation of jacobi 雅可比方程equation of mixed type 混合型方程equation of motion 运动方程equation of state 状态方程equation of the straight line 直线方程equation root 方程的根equation with integral coefficients 整系数方程equatorial coordinates 赤道座标equatorial radius 赤道半径equi asymptotic stability 等度渐近稳定性equi luminosity curve 均匀光度曲线equiangular 等角的equiangular spiral 对数螺线equiareal 保积的equiconjugate diameter 等共轭直径equicontinuity 同等连续性equicontinuous 等度连续的equicontinuous functions 等度连续函数equicontinuous set 等度连续集equiconvergence 同等收敛性equidimensional ideal 纯理想equidistant 等距的equidistant curve 等距曲线equilateral 等边的equilateral cone 等边锥面equilateral hyperbola 等轴双曲线equilateral triangle 等边三角形equilibrium 平衡equilibrium concentration 平衡浓度equilibrium conditions 平衡条件equilibrium constant 平衡常数equilibrium diagram 平衡图equilibrium point 平衡点equilibrium principle 平衡原理equilibrium state 平衡状态equipartition 匀分equipotent 等势的;对等的equipotent set 等势集equipotential 等势的equipotential line 等位线equipotential surface 等位面equivalence 等价equivalence class 等价类equivalence problem 等价问题equivalence relation 等价关系equivalent 等价的equivalent equation 等价方程equivalent fiber bundle in g g 等价纤维丛equivalent form 等价形式equivalent functions 等价函数equivalent knot 等价纽结equivalent mapping 保面积映射equivalent matrix 等价阵equivalent metric 等价度量equivalent neighborhood system 等价邻域系equivalent norm 等价范数equivalent point 等价点equivalent proposition 等值命题equivalent states 等价状态equivalent stochastic process 等价随机过程equivalent terms 等价项equivalent transformation 初等运算equivariant map 等变化映射erasing 擦除ergodic chain 遍历马尔可夫链ergodic hypothesis 遍历假说ergodic markov chain 遍历马尔可夫链ergodic property 遍历性ergodic state 遍历态ergodic theorem 遍历定理ergodic theorem in the mean 平均遍历定理ergodic theory 遍历理论ergodic transformation 遍历变换ergodicity 遍历性error 误差error analysis 误差分析error band 误差范围error coefficient 误差系数error component 误差分量error curve 误差曲线error equation 误差方程error estimation 误差估计error function 误差函数error in the input data 输入数据误差error law 误差律error limit 误差界限error mean square 误差方差error model 误差模型error of estimation 估计误差error of first kind 第一类误差error of measurement 测量误差error of observation 观测误差error of reading 读数误差error of second kind 第二类误差error of the third kind 第三类误差error of truncation 舍位误差error originated from input 输入误差error probability 误差概率error sum of squares 误差平方和error variance 误差方差escribe 旁切escribed 旁切的escribed circle 旁切圆essential 本性的essential boundary condition 本质边界条件essential convergence 本质收敛essential epimorphism 本质满射essential extension 本质开拓essential homomorphism 本质同态essential inferior limit 本质下极限essential infimum 本性下确界essential parameter 本质参数essential point 本质点essential singular kernel 本性奇核essential singularity 本性奇点essential spectrum 本质谱essential strategy 本质策略essential superior limit 本质上极限essential supremum 本性上确界essential undecidability 本质不可判定性essentially bounded 本质有界的essentially convergent sequence 本质收敛序列essentially self adjoint operator 本质自伴算子estimable function 可估计函数estimable hypothesis 可估计假设estimate 估计estimation 估计estimation of error 误差估计estimation of parameter 参数的估计estimation region 估计区域estimation theory 估计论estimator 估计量etale neighborhood 层邻域etale space 层空间etale topology 层拓扑etalon 标准euclid factorization theorem for rational integers 因子分解定理euclid lemma 欧几里得引理euclid parallel postulate 欧几里得平行公设euclidean algorithm 欧几里得算法euclidean domain 欧几里得整环euclidean geometry 欧几里得几何euclidean metric 欧几里得度量euclidean norm 欧几里得范数euclidean plane 欧几里得平面euclidean ring 欧几里得整环euclidean space 欧几里得空间euclidean vector space 欧几里得向量空间euler characteristic 欧拉示性数euler class 欧拉类euler constant 欧拉常数euler criterion 欧拉判别准则euler differential equation 欧拉微分方程euler formula 欧拉公式euler identity 欧拉恒等式euler number 欧拉数euler poincare formula 欧拉庞加莱公式euler poincare relation 欧拉庞加莱公式euler polyhedron theorem 欧拉多面体定理euler polynomial 欧拉多项式euler summation formula 欧拉总和公式eulerian angle 欧拉角evaluate 求...的值evaluation 计算evaluation of functions 函数值计算evaluation of integrals 积分计算even 偶数的even function 偶函数even number 偶数even parity 偶数同位even permutation 偶置换evenness 偶数性event 事件everywhere convergent sequence 处处收敛序列evidence 迷evident 迷的evolute 缩闭线evolute surface 渐屈面evolution 开方evolution equation 发展方程evolvent 渐伸线exact cohomology sequence 正合上同凋列exact differential equation 全微分方程exact division 正合除法exact homotopy sequence 正合同伦序列exact solution 精确解exact square 正合平方exactitude 精确度exactness axiom 正合性公理example 例exceed 超过excenter 外心exceptional curve 例外曲线exceptional jordan algebra 例外约当代数exceptional point 例外点exceptional value 例外值excess 超过excess function 超过函数excess of nine 舍九法exchange 交换exchange integral 交换积分exchange lattice 交换格exchange theorem 交换定理excircle 旁切圆excision 切除excision isomorphism 切除同构exclude 排除exclusion 排除exclusive disjunction 不可兼析取exclusive events 互斥事件exclusive or 不可兼的或executive program 执行程序exist 存在existence 存在existence conditions 存在条件existence of extremum 极值的存在existence theorem 存在定理existence theorem for roots 根的存在性定理existence theorem of implicit function 隐函数的存在性定理existential quantifier 存在量词exogenous variable 局外变量exotic space 异种空间expactation vector 期望值向量expand 展开expansion 展开expansion coefficient 展开系数expansion in series 级数展开expansion in terms of eigenfunction 本寨数展开expansion of a determinant 行列式的展开expansion theorem 展开定理expectation 期望值expected gain 期望增益expected payoff 期望增益expected value 期望值expected value vector 期望值向量experiment 实验experimental 实验的experimental error 实验误差explicit difference scheme 显式差分格式explicit differential equation 显式微分方程explicit function 显函数exponent 指数exponent notation 指数记法exponent of convergence 收敛指数exponential 指数函数exponential curve 指数曲线exponential distribution 指数分布exponential equation 指数方程exponential family 指数族exponential form of complex number 复数的指数形式exponential fourier transformation 指数型傅里叶变换exponential function 指数函数exponential integral 积分指数exponential law 指数定律exponential map 指数映射exponential p adic valuation 指数p 进赋值exponential process 指数过程exponential series 指数级数exponential sum 指数和exponential type 指数型exponential valuation 指数赋值exponentially asymptotic stability 指数式渐近稳定exportation 输出express 表示expression 式exradius 外圆半径extend 扩大extended commutator 广义换位子extended complex plane 扩张平面extended ideal 广义理想extended mean value theorem 广义均值定理extended plane 扩张平面extended point transformation 开拓的点变换extended predicate calculus 广义谓词演算extended riemann hypothesis 广义黎曼假设extended unitary group 广义酉群extension 扩张extension module 扩张模extension of a field 域的扩张extension of the residue field 剩余域的扩张extension principle of propositional logic 命题逻辑的外延性原理extension theorem 扩张定理extensionality 外延extensive quantity 外延量extent 范围exterior 外exterior algebra 外代数exterior angle 外角exterior approximation 外逼近exterior boundary problem 外边界问题exterior derivative 外导数exterior differential 外微分exterior differential form 外微分形式exterior differentiation 外微分法exterior domain 外域exterior interior angles 同位角exterior multiplication 外乘exterior normal 外法线exterior point 外点exterior power 外幂exterior problem 外边界问题exterior product 外积exterior product of tensors 张量的外积external 外部的external composition 外部合成external composition law 外部合成律external direct sum 外直和external division 外分external law of composition 外部合成律external memory 外存储器external program 外部程序external ratio 外分比external store 外存储器externally stable set 控制集externally tangent 外切的extract 开方extraction of a root 开方extraneous root 额外根extrapolate 外推extrapolation 外插extremal 极值曲线;极值的extremal element 极值元素extremal function 极值函数extremal length 极值长度extremal point 极值点extremal property 极值性质extremal surface 极值曲面extreme 外项extreme form 极型extreme point 极值点extreme term 外项extreme value 极值extreme value distribution 极值分布extreme value problem 极值问题extremity 端extremum 极值extremum conditions 极值条件extremum problem with subsidiary condition 附加条件极值问题extremum with a condition 条件极值extremum with a constraint 条件极值。
自动控制理论词汇表

Glossary for Feedback Control of Dynamic Systems自动控制理论词汇表Chapter 1thermostat n.恒温器predictive control 预测控制power generation plant 发电厂micron n.微米cell phone 移动电话jumbo jet 巨型喷气式客机block diagram 方框图actuator 执行机构process n.过程feedback n.反馈plant n.被控对象mph=mile per houropen-loop 开环closed-loop 闭环throttle n.油门gain n.增益orifice n.孔、小孔controlled variable 被控变量error n.误差incubator n.孵化器flue n.烟道chronicle n.编年史、年代记录conical a.圆锥体的mill wright 技工、造水车工匠inertia n.惯性、惯量oscillate about…在….周围振荡reference input 参考输入prescribed direction 预定的方向actual direction 实际方向flyball n.飞球governor n.控制器、调节器、总督、省长equilibrium n平衡点differencial equation 微分方程characteristicequation 特征方程third-order 三阶polynomial n.多项式state variable 状态变量distortion n.畸变complex variable 复变量methdology n.方法论proportional a. 比例的、成比例的integral a.积分的derivative a.微分的stochastic a.随机的servomechanism n.伺服机构calculus n.微积分ubiquitous a.到处存在的、普遍存在的radar-tracking 雷达跟踪SISO systems 单输入单输出系统Laplace transform 拉普拉斯变换pole n.极点zero n.零点transfer function传递函数trajectory optimization 轨迹优化root locus 根轨迹specifications n.指标、规格、规范discrete-data 离散数据sampled-data 采样数据performance n.性能Chapter 2desired reference variable 期望参考变量prototype n.原型system identification 系统辨识time response 时域响应step input 阶跃输入defer n.推迟、延期vector n.向量、矢量slug n.斯(勒格),质量单位(32.2磅)impart n.赋予、传授、告知heavy line 粗实线dashed line 虚线coordinate n.坐标numerator n.分子denominator n.分母suspension n.悬架、悬挂deflection n.偏移、偏转、偏差displacement n.位移shock absorber 减震器、缓冲器dashpot n.缓冲器bump n. vt.颠簸bounce n. vt.反弹、跳跃moment of inertia 转动惯量、惯性矩attitude n.姿态、姿势antenna n.天线perpendicular n.垂直线 a.垂直的asymmetry a.不对称的torque n.转矩resonant n.谐振、共振damper n.阻尼器prudent a.谨慎的,有远见的,精打细算的anti-alias 抗混频operational amplifer 运算放大器passive circuit 无源电路Kirchhoff’s current law 基尔霍夫电流定律algebraic sum 代数和summer n.加法器integrator n.积分器tesla n.特斯拉(磁通量单位)louderspeaker n.扬声器bobbin线轴,线筒stator n.定子rotor n.转子back emf 反电势maze n.曲径,迷宫specific heat 比热spatially ad.空间地hydraulic a.液压的、水力学的gimbal n.平衡环,万向接头nozzle n.喷嘴grooming n.修饰,美容piston n.活塞porous a.可渗透的,多孔的laminar a.多层的、层流的n.层流turbulent a.湍流的Chapter 3linear time-invariant systems 线性时不变(定常)系统signal flow graph 信号流图simulation n.仿真frequency-response 频率响应superposition n.叠加convolution n.卷积inpulse-response 脉冲响应unit step function 单位阶跃函数root-locus 根轨迹stability properties 稳定性特性principles linear algebra 线性代数原理state variable methods 状态变量法matrix n..矩阵nonlinear n.非线性mathematical mode 数学模型trivial a.琐碎的、不重要的linearize vt.线性化operating point 工作点state-space 状态空间partial differential equations 偏微分方程equilibrium n.平衡点complex frequency variables 复频率变量zero initial conditions 零初始条件steady-state 稳态ramp input 斜坡输入dc gain 直流增益inverse Laplace transform 逆拉氏变换partial fraction expansion 部分分式展开rantional a.有理的residue n.余式unilateral a.单边的convergence n.收敛final-value theorem 终值定理homogeneous differential equation 齐次微分方程ordinary differential equation 常微分方程overall transfer function 总的传递函数“loading” effect 负载效应cascade blocks 方框串联(级联)to reduce 化简eliminating 消去equivalent a.等效的simplification n.化简、简化integrodifferential a.积分-微分的time constant 时间常数imaginary axis 虚轴damping ratio n.阻尼比natural undamped frequency 自然无阻尼频率overdamped a.过阻尼critically damped n.临界阻尼rectangular coordinate 直角坐标oscillatory a.振荡的transient response 瞬态响应overshoot n.超调量delay time 延迟时间peak time 峰值时间rise time 上升时间settling time 调节时间steady state 稳态characteristic equation 特征方程RHP(Right Half-Plane) 右半平面elevator n.飞机升降舵,飞机升降仪,电梯nonminimum-phase 非最小相位diverge v.发散、分歧asymptotically stable 渐进稳定stability n.稳定性absolute stability 绝对稳定性relative stability 相对稳定性stability criterion 稳定性判据equilibrium state 平衡状态product n.乘积coefficient n..系数nagtive feedback 负反馈positive feedback 正反馈unity feedback system 单位负反馈系统reduction n.化简simultaneous a.联立的common factor 公因子expedient a.权宜的,有用的attenuate v.变弱,衰减,变细,变薄,稀释cofactor n.公因子Routh stability criterion 劳斯稳定性判据determinant n. 行列式tune v.调节retune v.再调节pseudorandom-noise 伪随机噪声signal-to-noise ratio 信-噪比Mason Gain Formula 梅森(增益)公式term n.术语signal flow graph 信号流图nodepathenvelope n.包络线dominant root 主导极点Chapter 4steady-state 稳态with respect to 关于….deviation n.偏离steady-state error 稳态误差load torque 负载转矩viscous friction 粘性摩擦repeater n.中继器drift v.漂移fidelity n.准确性,忠实,忠诚parabolic antenna 抛物线天线position error constant. 位置误差常数velocity error constant 速度误差常数robust property 鲁棒性shaft n.轴tachometer n.转速计inductance n.电感sampled v.采样quantized v.量化extrapolate v.预测,推测trapezoid n.梯形,不等边四边形vertices n.顶点order n.阶次,数量级proportional control 比例控制derivative control 微分控制sinusoidal a.正弦parameter n.参数Chapter 5root-locus method 根轨迹法monic a.首一的feedforward a.前向的denominator n.分母numerator n.分子quadratic n.二次项branch n.分支factored a.分解的asymptote vt.渐进n.渐进线division n.除法vantage point 有利地位,观点imaginary part 虚部breakaway point 分离点common denominator 公分母conjugate pairs 共扼对multiplicity n.多重,多数trial and error 凑试(法)spirule n.螺旋尺intersection n.交汇symmetrical a.对称的magnitude condition 幅值条件angle condition 相角条件phase condition 相角条件origin n.起始点terminus n.终点angle of departure 分离角、出发角angle of arrival汇合角、到达角cubic a.三阶的、立方的quartic a.四次的remainder n.留数、余数remainder theorem 留数定理taking the limit 取…极限synthetic division 综合除法dominant root 主导根compensator n.补偿器azimuth n.地位角、地平经度inertial guidance 惯性导航constant term 常数项symmetrical with respect to 关于…对称trial point 试验点terminate vt.终止于first differentiate 一阶微分real parts实部imaginary part 虚部lag compensator 滞后补偿器lead compensator 超前补偿器spill over 溢出,无法容纳autopilot n.自动导航trim v.n.使整齐,微调trim tab 平衡调整片margin n.裕量iteration n.重复、循环、迭代intact a.完好无缺的,原封不动的Chapter 6frequency response 频率响应rendered v.使成为,提供,报答,着色; 执行ratio of the magnitudes 幅值比bandwidth n.带宽resonant peak 谐振峰值low-pass filter低通滤波器sanity n.神智健全,头脑清楚,健全tangent n.正切、切线reciprocal a.互补的,相互的,互惠的phase difference 相角差transport lag 传输延迟irrational factor 非有理因子phase shift 相位移动moduli n.模(复数)poke vt.戳、刺、捅drudgery n.苦工、单调乏味的工作logarithmic coordinate 对数坐标semilog n.半对数decibel n.分贝decade n.十倍量程octave n.八倍频程、八度、八阶asymptotic behavior 渐进行为dotted n.虚线break frequency 转折频率corner frequency 转折频率slope n.斜率20dB/decade 20分贝/十倍频程superimpose vt.迭加polar plot 极坐标图pass function 旁路函数servomotor-amplifier 伺服电机-放大器angular velocity 角速度minimum phase 最小相位tilt angle 倾斜角lateral force 侧面力、横向力perceived velocity 可察觉的速度croseover frequency 穿越频率appendage n.附件、备件Nyquist criterion 奈奎斯特判据Semi-graphical 半图形Nyquist plot 奈奎斯特图Bode diagram 伯德图positive real part 正实部necessary and sufficient condition 充分必要条件left half of the s-plane s平面左半平面formidable a. 可怕的、令人生畏的determinant 行列式pole-zero cancellation 零极点相消rational functions 有理函数quotient n. 商、份额multi-loop control system 多环控制系统encircled vt. 环绕enclosed vt. 包围closed path 闭合路径counterclockwise a.逆时针的clockwise a.顺时针的encirclement n. 环绕enclosure n. 包围contour n.围线,轮廓线argument principle 幅角原理complex variable 复变量single-valued rational function 单值有理函数analytic a.解析的Nyquist path 奈奎斯特路径singularity n.奇异(点、值)semicircle n.半圆artifice n.技巧、技能gain margin 增益裕量phase margin 相角裕量vicinity n.邻近compromise n.折中,妥协trapezoidal a.梯形的iterate v.重复、循环、迭代bracket v.放在括号内,归入一类,包含octave n.八个一组的事物,八度enumerate v.数,点detrimental a.有害的,不利的threshold n.阈值Chapter 8sampling n.采样sample period 采样周期aliasing n.混频,别名inherent a.内在的z transform Z变换radar tracking system 雷达跟踪系统discrete period 离散周期discrete equivalent 离散等效digitization n.数字化recursive a.递归的,循环的difference equation 差分方程sample rate 采样速率sampler 采样器zero-order holder 零阶保持器inverse Z transform 反Z变换、逆Z变换long division 长除法unit circle 单位圆overlap n.重叠rephrase v.重新措辞,改述extrapolate v.预测,推测alleviate v.减轻,使 ... 缓和judicious a.明智的,贤明的,审慎的fictitious a.假想的,虚伪的impulse transfer function 脉冲传递函数piecewise-continuous 分段连续的pseudo-continuous-time 准连续时间Pade approximation Pade 近似Fourier analysis 傅立叶分析modulation n.调制Fourier transform 傅立叶变换spurious a.寄生的、伪的、假的ideal sampler 理想采样器impulse train 脉冲列、脉冲串transcendental a.超自然的、超常的rational function 有理函数closed form 封闭形式degree n.阶denominator n 分母numerator n 分子initial value 初始值identical a.相等的starred a.打星号的impulse response transfer function 脉冲响应传递函数uniformly spaced 均匀分布map into 影射、映射circles of radius 圆弧multiple-sheeted surfaceRiemann surface 黎曼曲面radial ray 射线by virtue of 借助、凭借、依靠….(的力量)logarithmic spiral 对数螺旋intersection n.相交power series 幂级数sampling instant 采样时刻natural logarithm 自然对数rationalizing 有理化cascading property 串联(级联)特性attenuation factor 衰减因子warp vt.使弯曲、使变形tune vt.调节、调整cross-hatched vt.用交叉线画出(图画上)阴影performance specification 性能指标trial-and-error approach 试凑法bilinear n.双线性Chapter 9equilibrium point 平衡点neighborhood n.邻域saturate n.饱和robotic n.机器人学heuristic a.启发式的,搜索式的sinusoidal a.正弦的sinusoid n.正弦harmonic a.谐波的describing-function 描述函数static nonlinearity 静态非线性dynamic nonlinearity. 动态非线性periodic response 周期响应phase-plane 相平面catastrophe n.灾难、浩劫shaky a.不稳定的,不可靠的scalar function 标量函数Liapunov function 李亚普诺夫函数linearization n.线性化inverse nonlinearity 可逆非线性perturbation n.摄动operating point 工作点eigenvalue n.特征值bearing n.轴承levitate v.浮动,使漂浮,使悬浮turbo n.汽轮机deviation n.偏差rigid link 刚性连接regime n.情形,体制dead-zone 死区viscous friction 粘性摩擦coulomb friction 库仑摩擦relay n.继电(特性)limit cycle 极限环deflect v.使偏,使歪windup n.终结,结束akin a.同类的,相似的odd function 奇函数backlash n.齿轮间隙magnetic hysteresis 磁滞coincident a.重合的,一致的on/off system 通断(控制)系统superposition n. 迭加sub-harmonic a.谐波的magnetic flux 磁通iron-cored coil 铁芯线圈stiction n. 静摩擦力autonomous a.自治的hypersphere n. 超球stability in the sense of Liapunov 李亚普诺夫意义下的稳定性asymptotically stable 渐进稳定monotonically stable 单调稳定origin n. 原点globally stable 全局稳定locally stable 局部稳定electronic oscillator 电子谐振器Van der Pol’s differential equation 范德波尔微分方程nonsinusoidal waveform 非正弦波形rated voltage 额定电压phase variable 相变量phase portrait 相图perpendicular a. 垂直的、正交的、成直角的Taylor series 泰勒级数increment n.增量Euler method 欧拉法singular point 奇异点。
参数更新公式
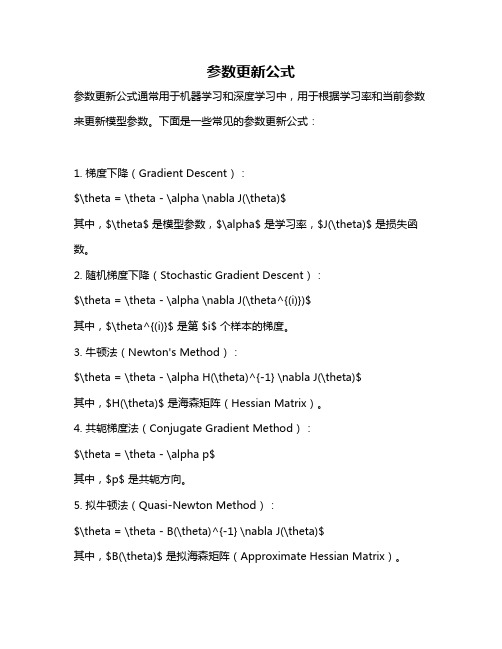
参数更新公式参数更新公式通常用于机器学习和深度学习中,用于根据学习率和当前参数来更新模型参数。
下面是一些常见的参数更新公式:1. 梯度下降(Gradient Descent):$\theta = \theta - \alpha \nabla J(\theta)$其中,$\theta$ 是模型参数,$\alpha$ 是学习率,$J(\theta)$ 是损失函数。
2. 随机梯度下降(Stochastic Gradient Descent):$\theta = \theta - \alpha \nabla J(\theta^{(i)})$其中,$\theta^{(i)}$ 是第 $i$ 个样本的梯度。
3. 牛顿法(Newton's Method):$\theta = \theta - \alpha H(\theta)^{-1} \nabla J(\theta)$其中,$H(\theta)$ 是海森矩阵(Hessian Matrix)。
4. 共轭梯度法(Conjugate Gradient Method):$\theta = \theta - \alpha p$其中,$p$ 是共轭方向。
5. 拟牛顿法(Quasi-Newton Method):$\theta = \theta - B(\theta)^{-1} \nabla J(\theta)$其中,$B(\theta)$ 是拟海森矩阵(Approximate Hessian Matrix)。
6. 坐标梯度下降(Coordinate Gradient Descent):$\theta_j = \theta_j - \alpha \frac{\partial J(\theta)}{\partial \theta_j}$ 其中,$\theta_j$ 是第 $j$ 个参数。
7. 带动量的梯度下降(Momentum Gradient Descent):$v = \beta v - \alpha \nabla J(\theta)$$\theta = \theta + v$其中,$v$ 是动量,$\beta$ 是动量系数。
英语翻译
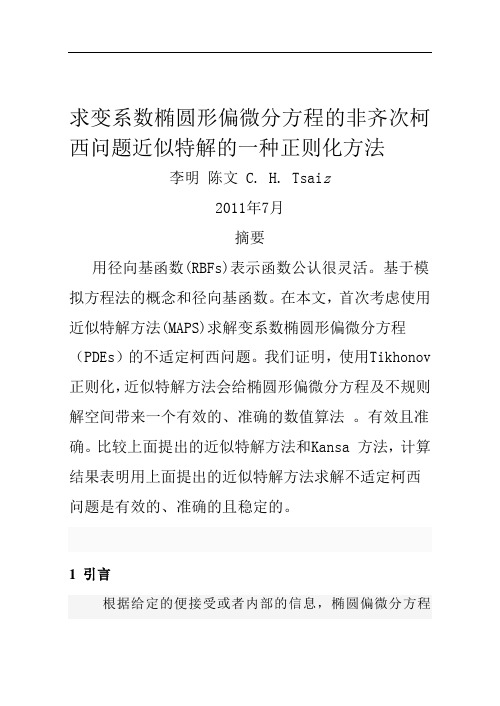
求变系数椭圆形偏微分方程的非齐次柯西问题近似特解的一种正则化方法李明陈文 C. H. Tsai z2011年7月摘要用径向基函数(RBFs)表示函数公认很灵活。
基于模拟方程法的概念和径向基函数。
在本文,首次考虑使用近似特解方法(MAPS)求解变系数椭圆形偏微分方程(PDEs)的不适定柯西问题。
我们证明,使用Tikhonov 正则化,近似特解方法会给椭圆形偏微分方程及不规则解空间带来一个有效的、准确的数值算法。
有效且准确。
比较上面提出的近似特解方法和Kansa 方法,计算结果表明用上面提出的近似特解方法求解不适定柯西问题是有效的、准确的且稳定的。
1 引言根据给定的便接受或者内部的信息,椭圆偏微分方程(PDEs) 大致可以分为以下五个主要问题:1.边界值问题[2,6]。
这些问题已经发展和应用很长一段时间。
例如:Dirichlet, Neumann 或者 Robin 混合问题。
2.Cauchy问题[23]。
这些问题也被称为边值的确定问题。
对于椭圆形Cauchy问题,Dirichlet和Neumann边界条件只在解空间边界的一部分给定。
3.边界的确定问题[11]。
这些是工程上典型的非破坏性测试问题。
这些问题确定解空间的未知边界。
4.系数的重建问题[26]。
5.潜在源的确定问题。
上述问题中。
只有第一个问题是适定的。
适定问题的定义是由Hadamard【7】给出的。
他认为,为某物理现象建立的合适的数学模型应具有以下三个属性:存在,唯一且稳定。
如若其中之一不满足,则建立在物理现象之上的这个数学模型就是不适定的。
然而,越来越多的不适定问题正出现于科学cs,中国香港特别行政区,香港城市大学相关作者,中国南京河海大学工程力学系型的逆问题,从Hadamard角度上来说,他们是不适定的,因为测量数据任何小误差都可能导致解的大差距。
偏微分方程的系数通常对应于问题的材料参数。
在非均匀介质中,材料参数可能会随位置而变化。
因此在非均匀介质的物理问题的控制方程中很可能会涉及到变系数。
计算机术语表
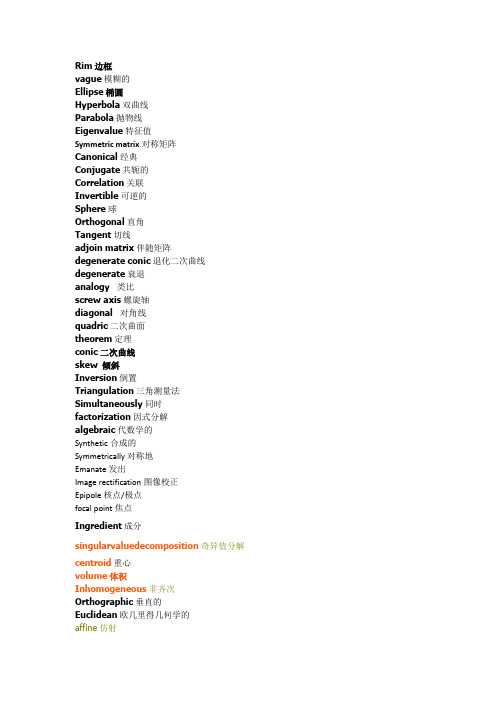
Rim边框vague模糊的Ellipse椭圆Hyperbola双曲线Parabola抛物线Eigenvalue特征值Symmetric matrix对称矩阵Canonical经典Conjugate共轭的Correlation关联Invertible可逆的Sphere球Orthogonal直角Tangent切线adjoin matrix伴随矩阵degenerate conic退化二次曲线degenerate衰退analogy类比screw axis螺旋轴diagonal 对角线quadric二次曲面theorem定理conic二次曲线skew 倾斜Inversion倒置Triangulation三角测量法Simultaneously同时factorization因式分解algebraic代数学的Synthetic合成的Symmetrically对称地Emanate发出Image rectification图像校正Epipole核点/极点focal point焦点Ingredient成分singularvaluedecomposition奇异值分解centroid重心volume体积Inhomogeneous非齐次Orthographic垂直的Euclidean欧几里得几何学的affine仿射Simultaneous同步的Clutter凌乱的Stereo立体的perspective透视图Binoculars双眼的envision想象monocular单眼的Intensity强度Synset同义词集cardinality基数Reification具体化pencil光束Subject主语Predicate谓语Object宾语idiomatic成语习惯的Contrast对比、清晰度stride跨度spotlights聚光灯tailored剪裁的retrospective回溯的pseudo假的benchmark基准点alphabet字母表empirical经验主义的exponential指数的semidefinite半负定的asymptotically渐进denote表示Spanned跨越的Portion部分residual剩余的subject to以……为条件orthogonal直角的vector magnitude向量大小foregoing前述的ensemble全体admissible可容许的delinate描绘lemma补充定理coherence 连贯性Synthetic虚构的Perpendicular垂直的Unfeasible不可行的anthropomorphical拟人的Pupil瞳孔Bivariate双变量auxiliary辅助的Fiducial基准的Drastic激烈的Intersection交界交点discreate摧毁discrete离散的Encyclopedia百科全书biometrics生物统计学eigenface特征脸Intuitively 直观地alleviate 减轻holistic 整体的orthogonal 正交Predominant卓越的exclusive排外的Approximation filter 估计滤波器Approximation pyramid 估计金字塔Binary image 二值图像Block neighborhood averaging 块邻域平均Blur 模糊block diagram 流程图Boundary pixel 边界像素Boundary tracking 边界跟踪Closed curve 封闭曲线color model 彩色模型complex conjugate复共轭Connected 连通的Convolution 卷积Curve 曲线contour plot 等值线图4-neighbors 4邻域8—neighbors 8邻域4-adjacency 4邻接8-adjacency 8邻接m—adjacency m邻接Path 路径Dilation 膨胀Downsampler 下采样Erosion 腐蚀Opening 开运算(先腐蚀,后膨胀)Closing 闭运算(先膨胀,后腐蚀)Structuring element 结构元素DFT 离散的傅立叶变换Inverse DFT 逆离散的傅立叶变换Digital image 数字图像Digital image processing 数字图像处理Digitization 数字化Edge 边缘Edge detection 边缘检测Edge enhancement 边缘增强Edge image 边缘图像Edge linking 边缘连接Edge operator 边缘算子Edge pixel 边缘像素Enhance 增强fits snugly 宽松适应Fourier transform 傅立叶变换Gray level 灰度级别Gray scale 灰度尺度gridline 网格线Horizontal edge 水平边缘Highpass filtering 高通滤波histogram 直方图Hough transform 哈夫变换Lowpass filtering 低通滤波Image sampling 图像采样Image restoration 图像复原Image segmentation 图像分割Image sharpening 图像锐化image subsets 图像子集Image quantization 图像量化Image pyramid 图像金字塔Interpolation filter 插值滤波器Inverse transformation 逆变换Line detection 线检测Line pixel 直线像素Linear filter线性滤波Median filter中值滤波Mask 掩模Measurement space 度量空间morphological transform 形态学变换morphological operations 形态学操作Neighborhood 邻域Neighborhood operation 邻域运算Noise 噪音Noise reduction 噪音抑制Pixel 像素Point operation 点运算pseudo-code 伪代码prediction residual pyramid 预测残差金字塔Quantitative image analysis 图像定量分析Quantization 量化rectangle 矩形Region 区域Region averaging 区域平均Region growing 区域增长Resolution 分辨率salt and pepper noise 盐白噪声Sampling 采样Sharpening 锐化Shape number 形状数Smoothing mask平滑掩模square boxes 正方形surface plot 曲面图structuring element 结构化元素Threshold 阈值Thresholding 二值化Transfer function 传递函数Upsampler 上采样Weighted region averaging加权区域平均Vertical edge 垂直边缘Horizontal edge 水平边缘RGB color cube RGB色彩立方体HSI color model HSI 色彩模型Circular color plane 圆形彩色平面Triangular color plane 三角形彩色平面Magnitude大小discrete离散convolution卷积z(t)=x(t)*y(t)= ∫x(m)y(t-m)dm第一次扇你鼓起来的包还没消肿,第二个巴掌就来了,你脸上的包就可能鼓起来两倍高,老板不断扇你,脉冲不断作用在你脸上,效果不断叠加了,这样这些效果就可以求和了,结果就是你脸上的包的高度岁时间变化的一个函数了(注意理解);如果老板再狠一点,频率越来越高,以至于你都辨别不清时间间隔了,那么,求和就变成积分了.可以这样理解,在这个过程中的某一固定的时刻,你的脸上的包的鼓起程度和什么有关呢?和之前每次打你都有关!但是各次的贡献是不一样的,越早打的巴掌,贡献越小,所以这就是说,某一时刻的输出是之前很多次输入乘以各自的衰减系数之后的叠加而形成某一点的输出,然后再把不同时刻的输出点放在一起,形成一个函数,这就是卷积,卷积之后的函数就是你脸上的包的大小随时间变化的函数。
几种常见的优化算法
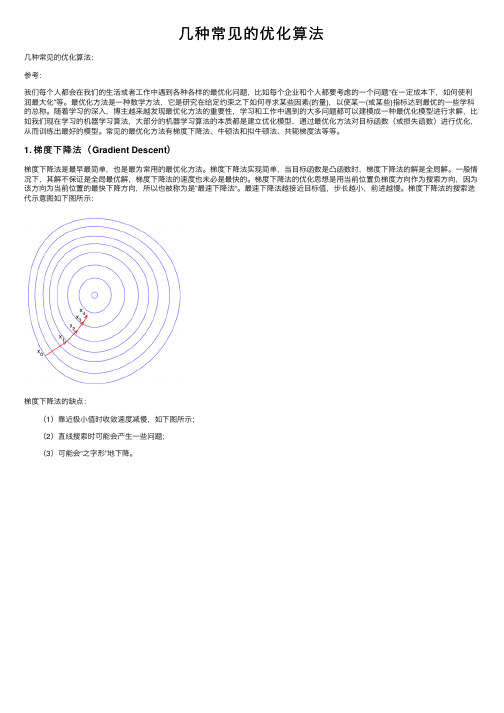
⼏种常见的优化算法⼏种常见的优化算法:参考:我们每个⼈都会在我们的⽣活或者⼯作中遇到各种各样的最优化问题,⽐如每个企业和个⼈都要考虑的⼀个问题“在⼀定成本下,如何使利润最⼤化”等。
最优化⽅法是⼀种数学⽅法,它是研究在给定约束之下如何寻求某些因素(的量),以使某⼀(或某些)指标达到最优的⼀些学科的总称。
随着学习的深⼊,博主越来越发现最优化⽅法的重要性,学习和⼯作中遇到的⼤多问题都可以建模成⼀种最优化模型进⾏求解,⽐如我们现在学习的机器学习算法,⼤部分的机器学习算法的本质都是建⽴优化模型,通过最优化⽅法对⽬标函数(或损失函数)进⾏优化,从⽽训练出最好的模型。
常见的最优化⽅法有梯度下降法、⽜顿法和拟⽜顿法、共轭梯度法等等。
1. 梯度下降法(Gradient Descent)梯度下降法是最早最简单,也是最为常⽤的最优化⽅法。
梯度下降法实现简单,当⽬标函数是凸函数时,梯度下降法的解是全局解。
⼀般情况下,其解不保证是全局最优解,梯度下降法的速度也未必是最快的。
梯度下降法的优化思想是⽤当前位置负梯度⽅向作为搜索⽅向,因为该⽅向为当前位置的最快下降⽅向,所以也被称为是”最速下降法“。
最速下降法越接近⽬标值,步长越⼩,前进越慢。
梯度下降法的搜索迭代⽰意图如下图所⽰:梯度下降法的缺点: (1)靠近极⼩值时收敛速度减慢,如下图所⽰; (2)直线搜索时可能会产⽣⼀些问题; (3)可能会“之字形”地下降。
从上图可以看出,梯度下降法在接近最优解的区域收敛速度明显变慢,利⽤梯度下降法求解需要很多次的迭代。
在机器学习中,基于基本的梯度下降法发展了两种梯度下降⽅法,分别为随机梯度下降法和批量梯度下降法。
⽐如对⼀个线性回归(Linear Logistics)模型,假设下⾯的h(x)是要拟合的函数,J(theta)为损失函数,theta是参数,要迭代求解的值,theta求解出来了那最终要拟合的函数h(theta)就出来了。
其中m是训练集的样本个数,n是特征的个数。
土木专业术语英文翻译

自由振动 free vibration
固有振动 natural vibration
暂态 transient state
环境振动 ambient vibration
反共振 anti-resonance
衰减 attenuation
优势频率 dominant frequency
模态分析 modal analysis
固有模态 natural mode of vibration
同步 synchronization
超谐波 ultraharmonic
范德波尔方程 van der pol equation
等倾线法 isocline method
跳跃现象 jump phenomenon
负阻尼 negative damping
达芬方程 Duffing equation
希尔方程 Hill equation
KBM方法 KBM method, Krylov-Bogoliu-
自动定心 self-alignment
亚临界转速 subcritical speed
科研中国
涡动 whirl
固体力学类:
弹性力学 elasticity
弹性理论 theory of elasticity
均匀应力状态 homogeneous state of stress
扭[转]应力函数 Stress function of torsion
翘曲函数 Warping function
半逆解法 semi-inverse method
瑞利--里茨法 Rayleigh-Ritz method
迭代优化
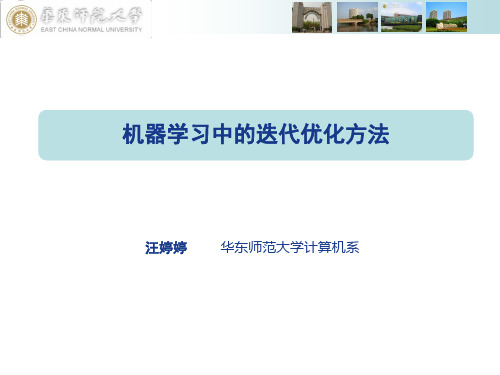
4
ECNU, 2014
梯度下降法
我们随机在超平面上取一个点,对应我们θ的初始值,然后每次改变一点Δθ ,使J(θ)也改变ΔJ(θ),只要能保证ΔJ<0就一直更新θ直到J(θ)不再减少为止 。具体如下:
1. 随机初始化θ;
2. 对于每一个θi选择合适的Δθi,使得J(θ+Δθ)−J(θ)<0,如果找不到这样的Δθ ,则结束算法;
17
ECNU, 2014
6
ECNU, 2014
梯度下降法
举一个非常简单的例子,如求函数 方法解题步骤如下:
1、求梯度, 2、向梯度相反的方向移动x,如下, 其中, 为步长。如果步长足够小,则可以保证每一次迭代都在减小,但可 能导致收敛太慢,如果步长太大,则不能保证每一次迭代都减少,也不能保 证收敛。 3、循环迭代步骤2,直到x的值变化到使得 在两次迭代之间的差值足够 小,比如0.00000001,也就是说,直到两次迭代计算出来的基本没有变化 ,则说明此时 已经达到局部最小值了。
的最小值。利用梯度下降的
4、此时,输出x,这个x就是使得函数最小时的的取值 。
7
ECNU, 2014
共轭梯度法
最速下降法(Steepest Descent)
8
ECNU, 2014
共轭梯度法
我们可以发现,每一次走的步伐和上一次都是垂直的(事实上是可以证明的 ,在前面我推荐的文中有详细的证明:-)),这样必然有很多步伐是平行的, 造成同一 个方向要走好几次。既然同一个方向要走好几次,能不能有什么 办法,使得同一个方向只走一次就可以了呢?
10
ECNU, 2014
共轭梯度法
共轭梯度法求解线性方程组
假如我们已经找到n个两两共轭的方向{d(i)},如果将这些方向作为基,也就 可以将Ax=b的解表示为d(i)的线性组合:
几种常见的优化方法ppt课件
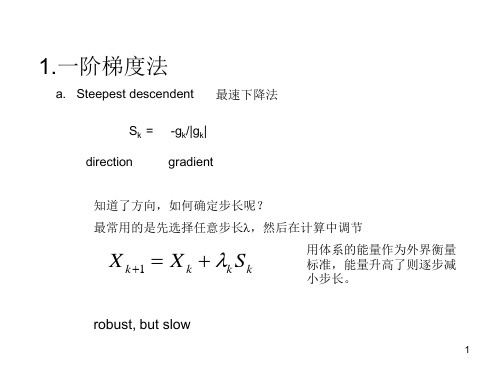
required for integration is usually trivial in comparison to
the time required for the force calculations.
Example of integrator for MD simulation
• One of the most popular and widely used integrators is
the Verlet leapfrog method: positions and velocities of
7
Continuous time molecular dynamics
1. By calculating the derivative of a macromolecular force
field, we can find the forces on each atom
as a function of its position.
11
Choosing the correct time step…
1. The choice of time step is crucial: too short and
phase space is sampled inefficiently, too long and the
energy will fluctuate wildly and the simulation may
– Rigid body dynamics
– Multiple time step algorithms
conjugate gradient 例子-概述说明以及解释
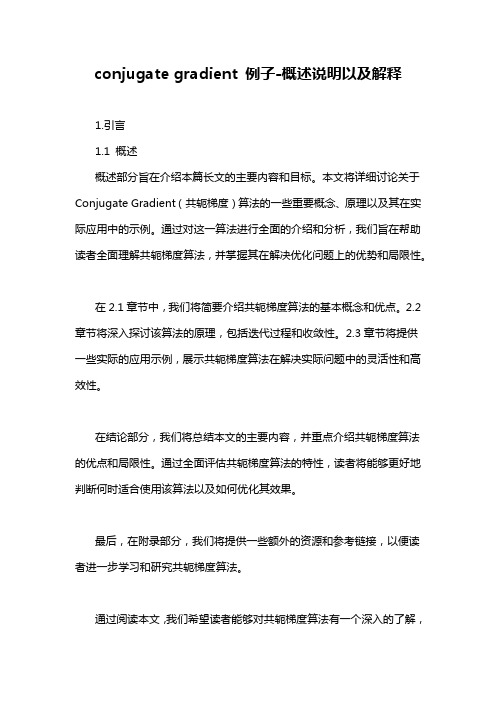
conjugate gradient 例子-概述说明以及解释1.引言1.1 概述概述部分旨在介绍本篇长文的主要内容和目标。
本文将详细讨论关于Conjugate Gradient(共轭梯度)算法的一些重要概念、原理以及其在实际应用中的示例。
通过对这一算法进行全面的介绍和分析,我们旨在帮助读者全面理解共轭梯度算法,并掌握其在解决优化问题上的优势和局限性。
在2.1章节中,我们将简要介绍共轭梯度算法的基本概念和优点。
2.2章节将深入探讨该算法的原理,包括迭代过程和收敛性。
2.3章节将提供一些实际的应用示例,展示共轭梯度算法在解决实际问题中的灵活性和高效性。
在结论部分,我们将总结本文的主要内容,并重点介绍共轭梯度算法的优点和局限性。
通过全面评估共轭梯度算法的特性,读者将能够更好地判断何时适合使用该算法以及如何优化其效果。
最后,在附录部分,我们将提供一些额外的资源和参考链接,以便读者进一步学习和研究共轭梯度算法。
通过阅读本文,我们希望读者能够对共轭梯度算法有一个深入的了解,并能够将其应用于实际问题中,进一步提升优化问题的求解能力。
文章结构部分的内容如下:1.2 文章结构本文按照如下结构进行组织:引言部分:首先对文章进行概述,介绍Conjugate Gradient算法的背景和意义,并提出本文的目的。
正文部分:分为三个主要部分,分别介绍Conjugate Gradient算法的简介、原理和应用示例。
- 2.1 Conjugate Gradient算法简介:在这一部分中,我们将详细介绍Conjugate Gradient算法的基本概念和一般步骤。
我们将解释算法的目标、求解的问题类型以及算法的基本思想。
- 2.2 Conjugate Gradient算法的原理:在这一部分中,我们将深入探讨Conjugate Gradient算法的原理。
我们将详细介绍算法的数学基础和推导过程,包括如何选择迭代方向、计算步长等关键步骤。
人工智能岗位招聘笔试题与参考答案(某大型集团公司)
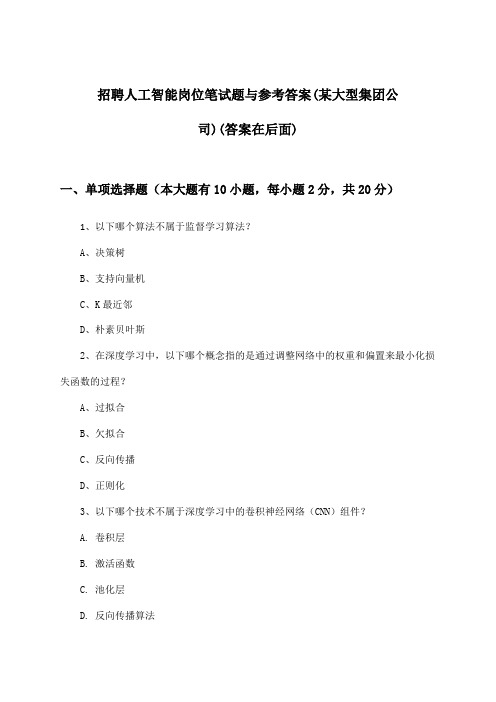
招聘人工智能岗位笔试题与参考答案(某大型集团公司)(答案在后面)一、单项选择题(本大题有10小题,每小题2分,共20分)1、以下哪个算法不属于监督学习算法?A、决策树B、支持向量机C、K最近邻D、朴素贝叶斯2、在深度学习中,以下哪个概念指的是通过调整网络中的权重和偏置来最小化损失函数的过程?A、过拟合B、欠拟合C、反向传播D、正则化3、以下哪个技术不属于深度学习中的卷积神经网络(CNN)组件?A. 卷积层B. 激活函数C. 池化层D. 反向传播算法4、在自然语言处理(NLP)中,以下哪种模型通常用于文本分类任务?A. 决策树B. 朴素贝叶斯C. 支持向量机D. 长短期记忆网络(LSTM)5、题干:以下哪项不属于人工智能的核心技术?A. 机器学习B. 深度学习C. 数据挖掘D. 计算机视觉6、题干:以下哪个算法在处理大规模数据集时,通常比其他算法更具有效率?A. K最近邻(K-Nearest Neighbors, KNN)B. 支持向量机(Support Vector Machines, SVM)C. 决策树(Decision Tree)D. 随机森林(Random Forest)7、以下哪个技术不属于深度学习领域?A. 卷积神经网络(CNN)B. 支持向量机(SVM)C. 递归神经网络(RNN)D. 随机梯度下降(SGD)8、以下哪个算法不是用于无监督学习的?A. K-均值聚类(K-means)B. 决策树(Decision Tree)C. 主成分分析(PCA)D. 聚类层次法(Hierarchical Clustering)9、以下哪个技术不属于深度学习中的神经网络层?A. 卷积层(Convolutional Layer)B. 循环层(Recurrent Layer)C. 线性层(Linear Layer)D. 随机梯度下降法(Stochastic Gradient Descent)二、多项选择题(本大题有10小题,每小题4分,共40分)1、以下哪些技术或方法通常用于提升机器学习模型的性能?()A、特征工程B、数据增强C、集成学习D、正则化E、迁移学习2、以下关于深度学习的描述,哪些是正确的?()A、深度学习是一种特殊的机器学习方法,它通过多层神经网络来提取特征。
训练算法和训练函数的关系-概述说明以及解释

训练算法和训练函数的关系-概述说明以及解释1. 引言1.1 概述概述部分应该阐述整篇文章要探讨的问题和主题。
在这篇文章中,就是"训练算法和训练函数的关系"。
本文主要讨论的是机器学习和深度学习中的训练算法和训练函数之间的关系。
通过理解和研究这两者之间的关联,我们将能够更好地理解机器学习和深度学习的基本原理,并能更有效地应用于实际问题。
训练算法是指用于估计和优化模型参数的方法,它们通过与真实数据进行迭代计算来逐步提高模型的准确性和泛化能力。
而训练函数则是用于定义和计算这些训练算法的函数,它们提供了一种框架和接口,使得我们可以轻松地使用各种训练算法进行模型训练。
本文将首先介绍训练算法的定义和原理,包括梯度下降法、随机梯度下降法、牛顿法等常见的训练算法。
然后,我们将详细讨论训练函数的定义和作用,以及训练函数的种类,包括损失函数、正则化函数等。
最后,通过总结算法和函数之间的关系,我们将得出结论,说明训练算法和训练函数是紧密相连的,两者是相辅相成的。
同时,我们还将思考训练算法和训练函数的重要性,以及它们在机器学习和深度学习领域的应用前景。
通过本文的阐述,读者将能够深入了解训练算法和训练函数之间的关系,并能够更加灵活地选择和应用适合的训练算法和训练函数来解决实际问题。
1.2 文章结构本文将探讨训练算法和训练函数之间的关系。
为了更好地理解这一关系,文章将按照以下结构进行讲解。
首先,引言部分将对本文的主题进行概述,介绍训练算法和训练函数的基本概念和作用。
同时,我们将介绍文章的结构,明确将涵盖的内容。
接下来,正文部分将重点介绍训练算法和训练函数的定义、原理和常见种类。
首先,我们将深入探讨训练算法的定义和原理,介绍其在机器学习中的重要性和应用。
然后,我们将介绍一些常见的训练算法,例如梯度下降算法、随机梯度下降算法和牛顿法等,并对它们的特点和适用范围进行分析。
在接下来的部分,我们将着重讨论训练函数的定义、作用和种类。
前馈神经网络中的模型优化方法(七)

在机器学习和深度学习领域,神经网络是一个非常重要的模型。
其中,前馈神经网络(feedforward neural network)是一种常见的神经网络模型,它通过多层神经元的连接和权重调节,实现对输入数据的复杂非线性映射。
然而,前馈神经网络在实际应用中存在一些问题,比如训练过程中的收敛速度、泛化能力和局部极小点等。
为了解决这些问题,研究者们提出了许多不同的模型优化方法。
首先,最基本的神经网络模型优化方法之一是梯度下降(gradient descent),它是一种迭代优化算法,通过不断地调整模型参数,使得损失函数(loss function)的值逐渐减小。
梯度下降有多种变种,比如随机梯度下降(stochastic gradient descent)和小批量梯度下降(mini-batch gradient descent),它们在计算效率和收敛速度上有不同的优势。
除了传统的梯度下降方法,还有一些新型的模型优化方法被提出,比如动量法(momentum)和自适应学习率方法(adaptive learning rate methods)。
动量法通过引入动量项来加速收敛过程,减少震荡,并且可以跳过局部极小点,从而更快地找到全局最优解。
而自适应学习率方法则根据每个参数的历史梯度信息来动态地调整学习率,从而提高收敛速度和泛化能力。
另外,近年来,深度学习领域出现了许多基于二阶导数信息的优化方法,比如牛顿法(Newton's method)和共轭梯度法(conjugate gradient method)。
这些方法利用参数的二阶导数信息来调整学习率和更新方向,通常可以更快地收敛到全局最优解。
然而,由于计算复杂度较高,这些方法在大规模神经网络上的应用还存在一定的挑战。
除了优化算法之外,正则化(regularization)和批归一化(batch normalization)也是提高神经网络泛化能力和训练速度的重要手段。
迭代优化
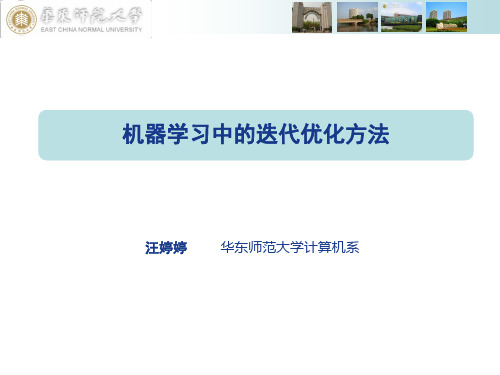
的最小值。利用梯度下降的
4、此时,输出x,这个x就是使得函数最小时的的取值 。
7
ECNU, 2014
共轭梯度法
最速下降法(Steepest Descent)
8
ECNU, 2014
共轭梯度法
我们可以发现,每一次走的步伐和上一次都是垂直的(事实上是可以证明的 ,在前面我推荐的文中有详细的证明:-)),这样必然有很多步伐是平行的, 造成同一 个方向要走好几次。既然同一个方向要走好几次,能不能有什么 办法,使得同一个方向只走一次就可以了呢?
5 ECNU, 2014
梯度下降法
梯度下降法(gradient descent),完整算法如下:
由于每次计算梯度都需要用到所有N条训练数据,所以这种算法也叫批量 梯度下降法(Batch gradient descent)。在实际情况中,有时候我们的训练 数据数以亿计,那么这样的批量计算消耗太大了,所以我们可以近似计算梯 度,也就是只取M(M<<N)条 数据来计算梯度,这种做法是现在最流行的训 练神经网络算法,叫mini-batch gradient descent。 最极端的,我们只用一条训练数据来计算梯度,此时这样的算法叫做随机 梯度下降法(stochastic gradient descent),适合数据是流式数据,一次只 给一条训练数据。
4
ECNU, 2014
梯度下降法
我们随机在超平面上取一个点,对应我们θ的初始值,然后每次改变一点Δθ ,使J(θ)也改变ΔJ(θ),只要能保证ΔJ<0就一直更新θ直到J(θ)不再减少为止 。具体如下:
1. 随机初始化θ;
2. 对于每一个θi选择合适的Δθi,使得J(θ+Δθ)−J(θ)<0,如果找不到这样的Δθ ,则结束算法;
力学专业英语词汇翻译
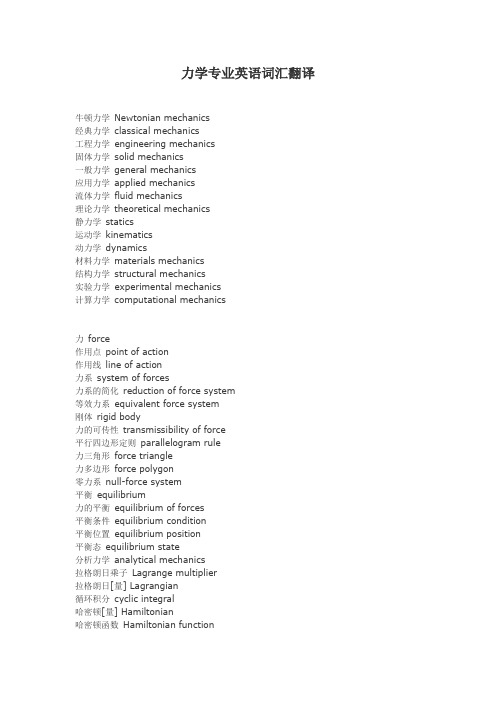
力学专业英语词汇翻译牛顿力学Newtonian mechanics经典力学classical mechanics工程力学engineering mechanics固体力学solid mechanics一般力学general mechanics应用力学applied mechanics流体力学fluid mechanics理论力学theoretical mechanics静力学statics运动学kinematics动力学dynamics材料力学materials mechanics结构力学structural mechanics实验力学experimental mechanics计算力学computational mechanics力force作用点point of action作用线line of action力系system of forces力系的简化reduction of force system等效力系equivalent force system刚体rigid body力的可传性transmissibility of force平行四边形定则parallelogram rule力三角形force triangle力多边形force polygon零力系null-force system平衡equilibrium力的平衡equilibrium of forces平衡条件equilibrium condition平衡位置equilibrium position平衡态equilibrium state分析力学analytical mechanics拉格朗日乘子Lagrange multiplier拉格朗日[量] Lagrangian循环积分cyclic integral哈密顿[量] Hamiltonian哈密顿函数Hamiltonian function正则方程canonical equation正则摄动canonical perturbation正则变换canonical transformation正则变量canonical variable哈密顿原理Hamilton principle作用量积分action integral哈密顿--雅可比方程Hamilton-Jacobi equation作用--角度变量action-angle variables泊松括号poisson bracket边界积分法boundary integral method运动稳定性stability of motion轨道稳定性orbital stability渐近稳定性asymptotic stability结构稳定性structural stability倾覆力矩capsizing moment拉力tensile force正应力normal stress切应力shear stress静水压力hydrostatic pressure集中力concentrated force分布力distributed force线性应力应变关系linear relationship between stress and strain 弹性模量modulus of elasticity横向力lateral force transverse force轴向力axial force拉应力tensile stress压应力compressive stress平衡方程equilibrium equation静力学方程equations of static比例极限proportional limit应力应变曲线stress-strain curve拉伸实验tensile test‘屈服应力yield stress极限应力ultimate stress轴shaft梁beam纯剪切pure shear横截面积cross-sectional area挠度曲线deflection curve曲率半径radius of curvature曲率半径的倒数reciprocal of radius of curvature纵轴longitudinal axis悬臂梁cantilever beam简支梁simply supported beam微分方程differential equation惯性矩moment of inertia静矩static moment扭矩torque moment弯矩bending moment弯矩对x的导数derivative of bending moment with respect to x弯矩对x的二阶导数the second derivative of bending moment with respect to x 静定梁statically determinate beam静不定梁statically indeterminate beam相容方程compatibility equation补充方程complementary equation中性轴neutral axis圆截面circular cross section两端作用扭矩twisted by couples at two ends刚体rigid body扭转角twist angle静力等效statically equivalent相互垂直平面mutually perpendicular planes通过截面形心through the centroid of the cross section一端铰支pin support at one end一端固定fixed at one end弯矩图bending moment diagram剪力图shear force diagram剪力突变abrupt change in shear force、旋转和平移rotation and translation虎克定律hook’s law边界条件boundary condition初始位置initial position、力矩面积法moment-area method绕纵轴转动rotate about a longitudinal axis横坐标abscissa扭转刚度torsional rigidity拉伸刚度tensile rigidity剪应力的合力resultant of shear stress正应力的大小magnitude of normal stress脆性破坏brittle fail对称平面symmetry plane刚体的平衡equilibrium of rigid body约束力constraint force重力gravitational force实际作用力actual force三维力系three-dimentional force system合力矩resultant moment标量方程scalar equation、矢量方程vector equation张量方程tensor equation汇交力系cocurrent system of forces任意一点an arbitrary point合矢量resultant vector反作用力reaction force反作用力偶reaction couple转动约束restriction against rotation平动约束restriction against translation运动的趋势tendency of motion绕给定轴转动rotate about a specific axis沿一个方向运动move in a direction控制方程control equation共线力collinear forces平面力系planar force system一束光 a beam of light未知反力unknown reaction forces参考框架frame of reference大小和方向magnitude and direction几何约束geometric restriction刚性连接rigidly connected运动学关系kinematical relations运动的合成superposition of movement固定点fixed point平动的叠加superposition of translation刚体的角速度angular speed of a rigid body质点动力学particle dynamics运动微分方程differential equation of motion 工程实际问题practical engineering problems变化率rate of change动量守恒conservation of linear momentum 定性的描述qualitative description点线dotted line划线dashed line实线solid line矢量积vector product点积dot product极惯性矩polar moment of inertia角速度angular velocity角加速度angular accelerationinfinitesimal amount 无穷小量definite integral 定积分a certain interval of time 某一时间段kinetic energy 动能conservative force 保守力damping force 阻尼力coefficient of damping 阻尼系数free vibration 自由振动periodic disturbance 周期性扰动viscous force 粘性力forced vibration 强迫震动general solution 通解particular solution 特解transient solution 瞬态解steady state solution 稳态解second order partial differential equation 二阶偏微分方程external force 外力internal force 内力stress component 应力分量state of stress 应力状态coordinate axes 坐标系conditions of equilibrium 平衡条件body force 体力continuum mechanics 连续介质力学displacement component 位移分量additional restrictions 附加约束compatibility conditions 相容条件mathematical formulations 数学公式isotropic material 各向同性材料sufficient small 充分小state of strain 应变状态unit matrix 单位矩阵dilatation strain 膨胀应变the first strain invariant 第一应变不变量deviator stress components 应力偏量分量the first invariant of stress tensor 应力张量的第一不变量bulk modulus 体积模量constitutive relations 本构关系linear elastic material 线弹性材料mathematical derivation 数学推导a state of static equilibrium 静力平衡状态Newton‘s first law of motion 牛顿第一运动定律directly proportional to 与……成正比stress concentration factor 应力集中系数state of loading 载荷状态st venant’ principle 圣维南原理uniaxial tension 单轴拉伸cylindrical coordinates 柱坐标buckling of columns 柱的屈曲critical value 临界值stable equilibrium 稳态平衡unstable equilibrium condition 不稳定平衡条件critical load 临界载荷a slender column 细长杆fixed at the lower end 下端固定free at the upper end 上端自由critical buckling load 临界屈曲载荷potential energy 势能fixed at both ends 两端固定hinged at both ends 两端铰支tubular member 管型杆件transverse dimention 横向尺寸stability of column 柱的稳定axial force 轴向力elliptical hole 椭圆孔plane stress 平面应力nominal stress 名义应为principal stress directions 主应力方向axial compression 轴向压缩dynamic loading 动载荷dynamic problem 动力学问题inertia force 惯性力resonance vibration 谐振static states of stress 静态应力dynamic response 动力响应time of contact 接触时间length of wave 波长resonance frequency 谐振频率自由振动free vibration固有振动natural vibration暂态transient state环境振动ambient vibration反共振anti-resonance衰减attenuation库仑阻尼Coulomb damping参量[激励]振动parametric vibration模糊振动fuzzy vibration临界转速critical speed of rotation阻尼器damper半峰宽度half-peak width相平面法phase plane method相轨迹phase trajectory解谐detuning耗散函数dissipative function硬激励hard excitation硬弹簧hard spring, hardening spring谐波平衡法harmonic balance method久期项secular term自激振动self-excited vibration软弹簧soft spring ,softening spring软激励soft excitation模态分析modal analysis固有模态natural mode of vibration同步synchronization频谱frequency spectrum基频fundamental frequency缓冲器buffer风激振动aeolian vibration嗡鸣buzz倒谱cepstrum颤动chatter蛇行hunting阻抗匹配impedance matching机械导纳mechanical admittance机械效率mechanical efficiency机械阻抗mechanical impedance随机振动stochastic vibration, random vibration 隔振vibration isolation减振vibration reduction方位角azimuthal angle多体系统multibody system静平衡static balancing动平衡dynamic balancing静不平衡static unbalance动不平衡dynamic unbalance现场平衡field balancing不平衡unbalance不平衡量unbalance质量守恒conservation of mass动量守恒conservation of momentum能量守恒conservation of energy动量方程momentum equation能量方程energy equation结构分析structural analysis结构动力学structural dynamics拱Arch三铰拱three-hinged arch抛物线拱parabolic arch圆拱circular arch穹顶Dome空间结构space structure空间桁架space truss雪载[荷] snow load风载[荷] wind load土压力earth pressure地震载荷earthquake loading弹簧支座spring support支座位移support displacement支座沉降support settlement超静定次数degree of indeterminacy机动分析kinematic analysis结点法method of joints截面法method of sections结点力joint forces共轭位移conjugate displacement影响线influence line三弯矩方程three-moment equation单位虚力unit virtual force刚度系数stiffness coefficient柔度系数flexibility coefficient力矩分配moment distribution力矩分配法moment distribution method力矩再分配moment redistribution分配系数distribution factor矩阵位移法matri displacement method单元刚度矩阵element stiffness matrix单元应变矩阵element strain matrix总体坐标global coordinates高斯--若尔当消去法Gauss-Jordan elimination Method屈曲模态buckling mode线弹性断裂力学linear elastic fracture mechanics, LEFM 弹塑性断裂力学elastic-plastic fracture mechanics, EPFM 断裂Fracture脆性断裂brittle fracture解理断裂cleavage fracture蠕变断裂creep fracture裂纹Crack裂缝Flaw缺陷Defect割缝Slit微裂纹Microcrack折裂Kink椭圆裂纹elliptical crack深埋裂纹embedded crack损伤力学damage mechanics损伤Damage连续介质损伤力学continuum damage mechanics细观损伤力学microscopic damage mechanics损伤区damage zone 疲劳Fatigue低周疲劳low cycle fatigue应力疲劳stress fatigue随机疲劳random fatigue蠕变疲劳creep fatigue腐蚀疲劳corrosion fatigue疲劳损伤fatigue damage疲劳失效fatigue failure疲劳断裂fatigue fracture疲劳裂纹fatigue crack疲劳寿命fatigue life疲劳破坏fatigue rupture疲劳强度fatigue strength交变载荷alternating load交变应力alternating stress应力幅值stress amplitude应变疲劳strain fatigue应力循环stress cycle应力比stress ratio安全寿命safe life过载效应overloading effect循环硬化cyclic hardening循环软化cyclic softening环境效应environmental effect裂纹片crack gage裂纹扩展crack growth, crack Propagation裂纹萌生crack initiation循环比cycle ratio实验应力分析experimental stress Analysis工作[应变]片active[strain] gage基底材料backing material应力计stress gage零[点]飘移zero shift, zero drift应变测量strain measurement应变计strain gage应变指示器strain indicator应变花strain rosette应变灵敏度strain sensitivity机械式应变仪mechanical strain gage直角应变花rectangular rosette引伸仪Extensometer应变遥测telemetering of strain横向灵敏系数transverse gage factor横向灵敏度transverse sensitivity焊接式应变计weldable strain gage平衡电桥balanced bridge粘贴式应变计bonded strain gage粘贴箔式应变计bonded foiled gage粘贴丝式应变计bonded wire gage桥路平衡bridge balancing电容应变计capacitance strain gage补偿片compensation technique补偿技术compensation technique基准电桥reference bridge电阻应变计resistance strain gage温度自补偿应变计self-temperature compensating gage 半导体应变计semiconductor strain Gage计算结构力学computational structural mechanics加权残量法weighted residual method有限差分法finite difference method有限[单]元法finite element method配点法point collocation里茨法Ritz method广义变分原理generalized variational Principle最小二乘法least square method胡[海昌]一鹫津原理Hu-Washizu principle赫林格-赖斯纳原理Hellinger-Reissner Principle修正变分原理modified variational Principle约束变分原理constrained variational Principle混合法mixed method杂交法hybrid method边界解法boundary solution method有限条法finite strip method半解析法semi-analytical method协调元conforming element非协调元non-conforming element混合元mixed element杂交元hybrid element边界元boundary element强迫边界条件forced boundary condition 自然边界条件natural boundary condition 离散化Discretization离散系统discrete system连续问题continuous problem坐标变换transformation of Coordinates 广义位移generalized displacement广义载荷generalized load广义应变generalized strain广义应力generalized stress界面变量interface variable节点node, nodal point[单]元Element角节点corner node边节点mid-side node内节点internal node无节点变量nodeless variable杆元bar element桁架杆元truss element梁元beam element二维元two-dimensional element一维元one-dimensional element三维元three-dimensional element轴对称元axisymmetric element板元plate element壳元shell element厚板元thick plate element三角形元triangular element四边形元quadrilateral element四面体元tetrahedral element曲线元curved element二次元quadratic element线性元linear element三次元cubic element四次元quartic element等参[数]元isoparametric element单元分析element analysis单元特性element characteristics刚度矩阵stiffness matrix几何矩阵geometric matrix等效节点力equivalent nodal force节点位移nodal displacement节点载荷nodal load位移矢量displacement vector载荷矢量load vector质量矩阵mass matrix集总质量矩阵lumped mass matrix相容质量矩阵consistent mass matrix阻尼矩阵damping matrix瑞利阻尼Rayleigh damping刚度矩阵的组集assembly of stiffness Matrices 载荷矢量的组集consistent mass matrix质量矩阵的组集assembly of mass matrices单元的组集assembly of elements局部坐标系local coordinate system局部坐标local coordinate面积坐标area coordinates体积坐标volume coordinates曲线坐标curvilinear coordinates静凝聚static condensation形状函数shape function试探函数trial function检验函数test function权函数weight function样条函数spline function节点号node number单元号element number带宽band width带状矩阵banded matrix变带状矩阵profile matrix带宽最小化minimization of band width波前法frontal method子空间迭代法subspace iteration method行列式搜索法determinant search method逐步法step-by-step method增量法incremental method初应变initial strain初应力initial stress切线刚度矩阵tangent stiffness matrix割线刚度矩阵secant stiffness matrix模态叠加法mode superposition method 平衡迭代equilibrium iteration子结构Substructure子结构法substructure technique网格生成mesh generation结构分析程序structural analysis program 前处理pre-processing后处理post-processing网格细化mesh refinement应力光顺stress smoothing组合结构composite structure。
梯度类算法
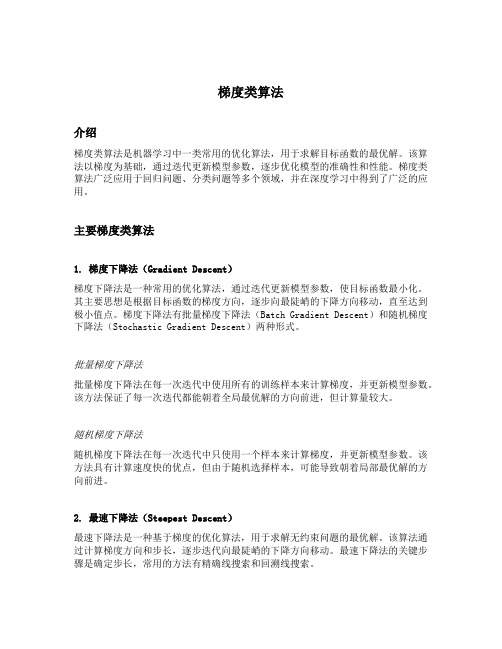
梯度类算法介绍梯度类算法是机器学习中一类常用的优化算法,用于求解目标函数的最优解。
该算法以梯度为基础,通过迭代更新模型参数,逐步优化模型的准确性和性能。
梯度类算法广泛应用于回归问题、分类问题等多个领域,并在深度学习中得到了广泛的应用。
主要梯度类算法1. 梯度下降法(Gradient Descent)梯度下降法是一种常用的优化算法,通过迭代更新模型参数,使目标函数最小化。
其主要思想是根据目标函数的梯度方向,逐步向最陡峭的下降方向移动,直至达到极小值点。
梯度下降法有批量梯度下降法(Batch Gradient Descent)和随机梯度下降法(Stochastic Gradient Descent)两种形式。
批量梯度下降法批量梯度下降法在每一次迭代中使用所有的训练样本来计算梯度,并更新模型参数。
该方法保证了每一次迭代都能朝着全局最优解的方向前进,但计算量较大。
随机梯度下降法随机梯度下降法在每一次迭代中只使用一个样本来计算梯度,并更新模型参数。
该方法具有计算速度快的优点,但由于随机选择样本,可能导致朝着局部最优解的方向前进。
2. 最速下降法(Steepest Descent)最速下降法是一种基于梯度的优化算法,用于求解无约束问题的最优解。
该算法通过计算梯度方向和步长,逐步迭代向最陡峭的下降方向移动。
最速下降法的关键步骤是确定步长,常用的方法有精确线搜索和回溯线搜索。
3. 共轭梯度法(Conjugate Gradient)共轭梯度法是一种迭代的最优化算法,用于求解对称正定系统的线性方程组。
该算法通过构造一组共轭的搜索方向,逐步迭代更新模型参数,直至达到最优解。
共轭梯度法的收敛速度较快,尤其在求解大规模线性方程组时具有优势。
4. 牛顿法(Newton’s Method)牛顿法是一种基于二阶导数的优化算法,用于求解非线性方程和最优化问题。
该算法通过构造二阶导数矩阵的逆,优化目标函数的二次近似。
牛顿法的收敛速度较快,但对于高维大规模问题,计算复杂度较高。
物理海洋大气科学常用专业词汇中英文对照

1 advection n. 平流2 Aleutian Low 阿留申低压3 angular momentum 角动量conservation of angular momentum4 antiphase n. 反位相 propagate in antiphase5 arbitrary adj. 任意的 arbitrary constant (任意常数)6 attenuate v. 削弱7 available potential energy 有效位能8 background wind field 背景风场9 baroclinic a. 斜压的 baroclinic instability10 barotropic a. 正压的 barotropic instability11 be proportional to 与……成比例12 benthic adj. 深海底的13 boundary condition 边值条件14 bounded domain 有界域→反:unbounded domain15 carrier wave 载波16 Cartesian coordinates 笛卡尔坐标系17 circumglobal a. 环绕地球旋转的,环地球的18 compensate v. 偿还,补偿19 conjugate adj. 共轭的 complex conjugate (共轭复数)20 convection n. 对流21 convergence n. 辐合→反:divergence22 convert v. 转换,相变 convert water into ice23 coordinate n. 坐标 Cartesian coordinates;spherical coordinates24 cumulative adj. 累积的 cumulative impact on25 damping n. 阻尼;减辐,衰减26 derivative n. 导数 the second order derivative27 diffusion n. 扩散28 diffusivity n. 扩散率29 discrepancy n. 差异,矛盾30 discrete adj. 离散的,不连续的31 displacement n. 位移 angular displacement32 dissipative a. 耗散的 dissipative effect33 divergence n. 辐散→反:convergence34 Drake Passage 德雷克海峡35 empirical adj. 经验的 model and empirical studies36 entropy n. 熵 specific entropy (比熵)37 equilibrium n. 平衡,均衡38 equivalent n. & adj. 相似的(物),等价的(物)39 essence n. 基本,本质40 exponential n. & adj. 指数(的)41 geostrophic adj. 地转的42 Icelandic Low 冰岛低压43 Indonesian Throughflow 印尼贯穿流44 initial condition 初值条件45 interfere v. (波的)干涉46 inviscid adj. 无粘性的47 isopycn n. 等体积线,等容线48 manifestation n. 显示, 表现49 meridional adj. 经向的50 mesoscale adj. 中尺度的 mesoscale eddy51 meteorological parameters 气象参数52 monotonically adv. 单调地 increase monotonically53 nontrivial adj. 重要的54 optimal adj. 最优的 optimal solution55 pendulum n. 钟摆, 摇锤56 penetrate v. 穿透,渗透57 potential vorticity 位势涡度 conservation of potential vorticity58 propagation n. (信号、声波)的传播59 quantify v. 量化60 radiative equilibrium 辐射平衡61 rectangle n. 长方形, 矩形62 respectively adv. 分别地,各个地63 Rossby deformation radius 罗斯贝变形半径64 schematic adj. 示意性的 summarize schematically in the figure65 sinusoidal adj. 正弦曲线的66 solar insolation 太阳照辐射量67 specular reflection 镜面反射(equal angles of incidence and reflection)68 spherical coordinates 球面坐标系69 standing wave 驻波70 stochastic adj. 随机的71 stochastic adj. 随机的72 stratification n. 层化73 stratosphere n. 平流层74 superposition n. 重叠, 重合75 superposition n. 重叠, 重合76 surface tension 表面张力77 symmetric adj. 对称性的78 synchronicity n. 同步性79 temporal average 时间平均→反:spatial average80 the product of A and B A与B的乘积81 thermocline slope 温跃层倾斜82 Tibetan Plateau 青藏高原83 trajectory n. 轨迹84 transient adj. 瞬时的 transient eddies85 tropic of cancer 北回归线86 tropic of carpricorn 南回归线87 troposphere n. 对流层88 variability n. 可变性89 variable n. 变量90 variance n. 方差91 variation n. 变更, 变化;变数,变分92 vector notation 向量符号表示, 向量记法93 velocity n. 速度94 ventilated thermocline 通风温跃层95 verify the hypothesis 证实了猜想96 vice versa 反之亦然97 viscosity n. 粘性,粘质98 vorticity n. 涡度99 westerlies n. 西风带100 Western Intensification 西向强化101 MOC 经向翻转环流 Meridianal Overturning Circulation。
自动化专业专业外语单词

abound v. 大量存在accelerate v. 加速active network 有源网络ad hoc 尤其,特定地admissible adj. 允许的advent n. 出现aforementioned adj. 上述的airgap = air gap 气隙algebraic equation 代数方程alignment n. 组合alleviate v. 减轻,缓和altitude n. 海拔amortisseur n. 阻尼器amplifier n. 放大器amplify v. 放大amplitude n. 振幅apparatus n. 装置approach n. 途径方法研究aptness n. 恰当arbitrary adj. 任意的argument n. 辐角,相位armature n. 电枢,衔铁,加固arrival angle 入射角arrival point 汇合点assembly n. 装置,构件assumption n. 假设asymmetric adj. 不对称的asymptote n. 渐进线asymptotically stable渐近稳定at rest 处于平衡状态attain v. 达到,实现autonomous adj.自激的bandwidth n. 带宽become adept in 熟练binary adj. 二进制的bipolar adj. 双向的Boolean algebra 布尔代数bound v. 限制break frequency 转折频率breakdown n. 击穿,雪崩breakover n. 导通brush n. 电刷building blocks 积木cage n. 笼子,笼形capacitor n. 电容器carrier n. 载波,载体cascade n., v. 串联characteristic adj. 特性(的)n. 特性曲线characteristicequation特方程circuitry n. 电路closed-loop n. 闭环coefficient n. 系数coil n. 绕组,线圈;v. 盘绕coincide v. 一致common logarithm 常对数complex adj. 复数的n.复数comprise v. 包含conduction n. 导电,传导configuration n. 构造,结构confine v. 限制…范围内conjugate adj. 共轭的constant matrix 常数矩阵constitute v. 构造,组织constraint n. 强迫,约束constraint n. 约束条件continuum n. 连续controllabillity n. 能控性converge v. 集中,汇聚,收敛core n. 铁心corresponding adj. 相应的criteria n. 判据critically damped 临界阻尼crystal n. 晶体cumulative adj. 累积的damp v. 阻尼,减幅,衰减damping n. 阻尼;adj. 阻尼的decay v. 衰减decibel n. 分贝decouple v. 解耦,退耦deduce v. 演绎demagnetization 去磁,退磁denominator n. 分母dependent variable 应变量depict v. 描述derivation n. 导数deteriorate v. 恶化,变坏deterministic adj. 确定的develop v. 导出,引入difference equation 差分方程differential 差别的,微分的differentiate v. 微分diode n. 二极管discrete adj. 离散的distributed 分散的分布的disturbance n. 扰动,干扰domain n. 域,领域dominate v. 支配,使服从dominating pole 主极点dual adj. 双的,对偶的dutyratio 占空比,功率比dynamic response 动态响应eigenvalue n. 特征根elastic adj. 有弹性的electric charge 电荷eliminate v. 消除,对消elongate v. 延长,拉长emf(electromotive force )电动势emitter n. 发射极encircle v. 环绕enclose v. 围绕encompass v. 包含entail v., n. 负担,需要equation 方程even adj. 偶数的excitation n. 激励exponential adj. 指数的extreme adj. 极端的facilitatev. 使容易,促进factor n. 因子;v. 分解因式factored adj. 可分解的feedback n. 反馈fictitious adj. 假想的field winding n. 励磁绕组field-weakening n. 弱磁filter n. 滤波器,滤波final value 终值firing angle 触发角flip-flop n. 触发器force commutated 强制换向forcing frequency 强制频率formulation n. 公式化forward biased 正向偏置fractional adj. 分数的fractional adj. 小数的fundamental n. 基本原理gate n. 门,门电路general form 一般形式generalize v. 一般化,普及generator n. 发生器,发电机geometry 几何学几何形状globally stable 全局稳定gouge v. 挖gross national product 国民生产总值GTO 门极可关断晶闸管guarantee v., n. 保证,担保hardware n. 硬件harmonics n. 谐波holding current 保持电流homogeneous solution 通解horizontally adv. 水平地horsepower n. 功率horsepower n. 马力,功率Hurwitz criterion 赫尔维茨据hybrid n. 混合identify v. 确认,识别,辨识identity n. 一致性,等式igit n. 位数imaginary axis 虚轴implement v. 实现implementation 实现履行impulse v. 冲激in series 串联incidentally adv. 偶然地increment n. 增量indentation n. 缺口independent variable 自变量inductor n. 电感器infinitesimal adj. 无限小的inhibit v. 抑制initial condition 初始条件initial value 初值insofar as 到这样的程度在……范围内integer n. 整数integral / integrate 积分integrated circuit 集成电路intersect v. 相交interval n. 间隔intrinsic adj. 固有的,本征intrinsic adj. 内在的intuition n. 直觉intuitively adv. 直观地inverse transform 反(逆)变换Kirchhoff’s first law 基尔霍夫第一定律lag v., n. 延迟lagging n. 滞后Laplace transformation拉变换lead n. 导线leading adj. 超前的leakage current 漏电流linearazation n. 线性化locally stable 局域稳定loop current 回路电流lumped adj. 集中的magnitude n. 幅值manipulate v. 处理matrix n. 模型,矩阵matrix algebra 矩阵代数mechanize v. 使机械化merit n. 优点;指标,准则mesh n. 网孔minimize v. (使)最小化minimum phase 最小相位misinterpretation 曲解误译model n. 模型v. 建模modification n. 修正,修改multiplication n. 复合性multiply v. 加倍,倍增multivariable adj. 多变量的multivariable n. 多变量n-dimensional n维的net n. 净值;adj. 净值的network n. 网络,电路neutral adj. 中性的;n. 中性线nonlinear adj. 非线性的notch n. 换相点,换级点numerator n. 分子numerical adj. 数字的observability n. 能观性observable adj. 可观测的odd multiple 奇数倍omit v. 省略on the order of 约为open-loop n. 开环optimal control 最优控制order n. 阶origin n. 原点oscillation n. 振荡outline n. 轮廓;v. 提出……的要点overdamped adj. 过阻尼的overshoot n. 超调量,超调package n. 包parallel n. 类似parameter n. 参数partial fraction expansion 部分分式展开式particular solution 特解passive adj. 被动的,无源的passive network 无源网络peak time 峰值时间performance criteria 性能指标performance index 性能指标periodic adj. 周期性的phase n. 状态,相位phase sequence 相序phase-lag n. 相位滞后phase-plane equation相平面方程pilot n. 飞行员plant n. 机器,设备被控对象plot v. 绘图n. 曲线图polar plot 极坐标图polarity n. 极性polynomial n. 多项式portrait n. 描述positive definite 正定potential n. (电)势power factor 功率因数predictable adj. 可断定的predominance n. 优势prevalent adj. 流行的prevent…from doing 使……不……principal adj. 主要的probability theory 概率论procedure n. 程序,过程product n. 乘积property n. 性质proportional to 与…成正比Pulsate v. 脉动,跳动,振动quadrant n. 象限quadratic adj. 二次的;n. 二次方程qualitatively adv. 定性地quasi adj. 近似的radically adv. 完全地radius n. 半径radix n. 权random adj. 随机的rated adj. 额定的,设计的,适用的rationale n. 理论real axis 实轴recovery n. 恢复rectification n. 整流rectifier n. 整流器regulate v. 调整relay n. 继电器relevance n. 关联remainder n. 余数represent v. 代表,表示resistance n. 阻抗resistor n. 电阻器reveal v. 显现,揭示reverse 反转变换极性的reverse biased 反向偏置rheostat n. 变阻器ripple n. 波纹,波动rise time 上升时间rms = root-mean-square 有效值,方均根rotor n. 转子Routh criterion 劳斯判据rugged adj. 结实的,耐用的sampled-data n. 采样数据saturation n. 饱和scalar adj. 数量的,标量的;n.数量,标量scheme n. 方法,形式,示意图schottky diode 肖基特二极管semicircle n. 半圆形semiconductor n. 半导体sensor n. 传感器settling time 调节时间shaft n. 转轴shifting theorem 平移定理shutdown v. 关闭sign n. 符号significance n. 意义simplicity n. 简单simultaneously adv. 同时地sketch v., n. (绘)草图,素描slip n. 转差(率)slop n. 斜率slot n. 槽SMPS 开关电源snubber n. 缓冲器,减震器spatial adj. 空间的spring n. 弹簧square-wave n. 方波stability n. 稳定性startup n. 启动state variable 状态变量state-controllable 状态可控的stationary adj. 静态的stator n. 定子steady-state n. 稳态step n. 阶跃(信号)stepper motor 步进电动机stimulus n. 刺激,鼓励stochastic adj. 随机的straight-forward 直截了当的,简单的strategy n. 方法superposition n. 叠加supersede v. 取代suppress v. 抑制symbolic 符号的,记号的symmetrical adj. 对称的terminology n. 术语threshold n. 门限,阈限,极限thyratron n. 闸流管thyristor n. 晶闸管time-invariant adj. 时不变的tip n. 顶端tolerant adj. 容许的,容忍的trade deficit 贸易赤字trade off 换取trail-and-error n. 试凑法trajectories n. 轨迹,弹道transient adj. 暂态的,瞬态的,过渡的transient response 暂态响应transistor n. 晶体管triangular adj. 三角的turn n. 匝数tutorial adj. 指导性的underdampted 欠阻尼的undergo v. 经历uniform adj. 一致的variable n. 变量vector n. 矢量vertically adv. 垂直地violently adv. 激烈地voltage drop 电压降winding 缠绕的,线圈,绕组with respect to 相对于workhorse n. 重载,重负荷wound-rotor n. 绕线转子yield v. 推导出,得出。
scaled conjugate gradient算法 -回复
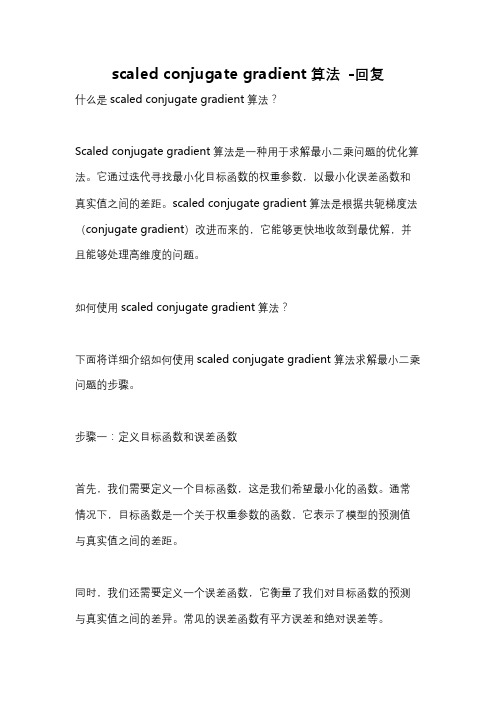
scaled conjugate gradient算法-回复什么是scaled conjugate gradient算法?Scaled conjugate gradient算法是一种用于求解最小二乘问题的优化算法。
它通过迭代寻找最小化目标函数的权重参数,以最小化误差函数和真实值之间的差距。
scaled conjugate gradient算法是根据共轭梯度法(conjugate gradient)改进而来的,它能够更快地收敛到最优解,并且能够处理高维度的问题。
如何使用scaled conjugate gradient算法?下面将详细介绍如何使用scaled conjugate gradient算法求解最小二乘问题的步骤。
步骤一:定义目标函数和误差函数首先,我们需要定义一个目标函数,这是我们希望最小化的函数。
通常情况下,目标函数是一个关于权重参数的函数,它表示了模型的预测值与真实值之间的差距。
同时,我们还需要定义一个误差函数,它衡量了我们对目标函数的预测与真实值之间的差异。
常见的误差函数有平方误差和绝对误差等。
步骤二:初始化权重参数在使用scaled conjugate gradient算法之前,我们需要对权重参数进行初始化。
通常情况下,我们可以将权重参数初始化为一个较小的随机数,或者根据某种经验法则进行初始化。
步骤三:计算梯度接下来,我们需要计算目标函数关于权重参数的梯度。
梯度表示了函数在某一点上的变化率,它可以指导我们朝着函数值减小的方向进行更新。
步骤四:更新权重参数使用scaled conjugate gradient算法,我们可以根据梯度的信息来更新权重参数。
算法会自动调整步长,并且会尝试不同的学习率来加速收敛速度。
当误差函数的值收敛到一个较小的阈值时,我们可以认为算法已经找到了最优解。
步骤五:检查收敛性在每一次更新权重参数之后,我们需要检查误差函数的值是否收敛。
如果误差函数的值已经收敛到一个较小的阈值,并且在之后的迭代过程中不再发生明显的变化,那么我们可以认为算法已经收敛,并且找到了最优解。
Stochastic Gradient Boosted Distributed Decision Trees
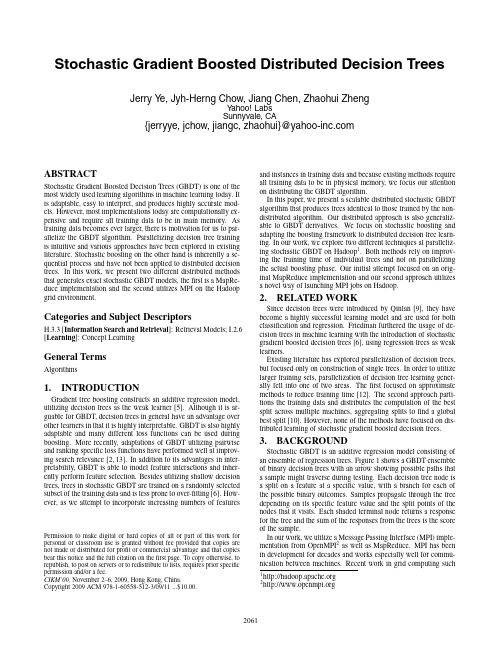
Stochastic Gradient Boosted Distributed Decision TreesJerry Y e,Jyh-Herng Chow,Jiang Chen,Zhaohui ZhengY ahoo!LabsSunnyvale,CA{jerryye,jchow,jiangc,zhaohui}@ABSTRACTStochastic Gradient Boosted Decision Trees(GBDT)is one of the most widely used learning algorithms in machine learning today.It is adaptable,easy to interpret,and produces highly accurate mod-els.However,most implementations today are computationally ex-pensive and require all training data to be in main memory.As training data becomes ever larger,there is motivation for us to par-allelize the GBDT algorithm.Parallelizing decision tree training is intuitive and various approaches have been explored in existing literature.Stochastic boosting on the other hand is inherently a se-quential process and have not been applied to distributed decision trees.In this work,we present two different distributed methods that generates exact stochastic GBDT models,thefirst is a MapRe-duce implementation and the second utilizes MPI on the Hadoop grid environment.Categories and Subject DescriptorsH.3.3[Information Search and Retrieval]:Retrieval Models;I.2.6 [Learning]:Concept LearningGeneral TermsAlgorithms1.INTRODUCTIONGradient tree boosting constructs an additive regression model, utilizing decision trees as the weak learner[5].Although it is ar-guable for GBDT,decision trees in general have an advantage over other learners in that it is highly interpretable.GBDT is also highly adaptable and many different loss functions can be used during boosting.More recently,adaptations of GBDT utilizing pairwise and ranking specific loss functions have performed well at improv-ing search relevance[2,13].In addition to its advantages in inter-pretability,GBDT is able to model feature interactions and inher-ently perform feature selection.Besides utilizing shallow decision trees,trees in stochastic GBDT are trained on a randomly selected subset of the training data and is less prone to over-fitting[6].How-ever,as we attempt to incorporate increasing numbers of features Permission to make digital or hard copies of all or part of this work for personal or classroom use is granted without fee provided that copies are not made or distributed for profit or commercial advantage and that copies bear this notice and the full citation on thefirst page.To copy otherwise,to republish,to post on servers or to redistribute to lists,requires prior specific permission and/or a fee.CIKM’09,November2–6,2009,Hong Kong,China.Copyright2009ACM978-1-60558-512-3/09/11...$10.00.and instances in training data and because existing methods require all training data to be in physical memory,we focus our attention on distributing the GBDT algorithm.In this paper,we present a scalable distributed stochastic GBDT algorithm that produces trees identical to those trained by the non-distributed algorithm.Our distributed approach is also generaliz-able to GBDT derivatives.We focus on stochastic boosting and adapting the boosting framework to distributed decision tree learn-ing.In our work,we explore two different techniques at paralleliz-ing stochastic GBDT on Hadoop1.Both methods rely on improv-ing the training time of individual trees and not on parallelizing the actual boosting phase.Our initial attempt focused on an orig-inal MapReduce implementation and our second approach utilizesa novel way of launching MPI jobs on Hadoop.2.RELATED WORKSince decision trees were introduced by Qinlan[9],they have become a highly successful learning model and are used for both classification and regression.Friedman furthered the usage of de-cision trees in machine learning with the introduction of stochastic gradient boosted decision trees[6],using regression trees as weak learners.Existing literature has explored parallelization of decision trees, but focused only on construction of single trees.In order to utilize larger training sets,parallelization of decision tree learning gener-ally fell into one of two areas.Thefirst focused on approximate methods to reduce training time[12].The second approach parti-tions the training data and distributes the computation of the best split across multiple machines,aggregating splits tofind a global best split[10].However,none of the methods have focused on dis-tributed learning of stochastic gradient boosted decision trees. 3.BACKGROUNDStochastic GBDT is an additive regression model consisting of an ensemble of regression trees.Figure1shows a GBDT ensemble of binary decision trees with an arrow showing possible paths that a sample might traverse during testing.Each decision tree node is a split on a feature at a specific value,with a branch for each of the possible binary outcomes.Samples propagate through the tree depending on its specific feature value and the split points of the nodes that it visits.Each shaded terminal node returns a response for the tree and the sum of the responses from the trees is the score of the sample.In our work,we utilize a Message Passing Interface(MPI)imple-mentation from OpenMPI2as well as MapReduce.MPI has been in development for decades and works especially well for commu-nication between machines.Recent work in grid computing such 12as the Apache Hadoop project have allowed for large scale deploy-ments of cheap,interchangeable compute nodes [4].Hadoop is an open source implementation of MapReduce [3]and it allows for streaming jobs where users can specify tasks for each compute node independent of MapReduce.In our work,we used MPI on Hadoop directly by writing a customized launcher.The next section describes how we distributed the training pro-cess for stochasticGBDT.Figure 1:A gradient boosted decision tree ensemble.4.METHODIn this section,we describe how to parallelize the stochastic GBDT learning process.Chen et.al.[2]presented a good overview of regression trees and gradient boosting in Section 3of TRADA.We describe various ways of partitioning the training data,our MapReduce implementation,and finally our MPI implementation on Hadoop.Before we proceed further,it is worth reiterating that we are only interested in learning exact models.The models are identical to those trained with the non-distributed version of the al-gorithm.This guided us as we made important design decisions.Alternatives to boosting and other tree ensemble methods such as random forests [11]were not attempted for this reason.4.1Distributed Training DataIn order to parallelize our decision tree training process,we must distribute the training data among machines.We are only interested in methods that would partition the data onto different machines rather than simply replicating it to reduce memory usage.There are already several existing approaches to distributed tree construction that aimed to improve scalability in terms of memory usage and improving training performance [8].Caregea et.al.outlined algo-rithms for distributed tree training and presented different methods for partitioning training data,either horizontally or vertically [1].In our work,we distributed our data using both vertically and hori-zontally partitioned methods.4.2MapReduce ImplementationOur initial implementation of distributed decision trees tried to frame the problem in the MapReduce paradigm and used a hori-zontal partitioning approach.Gehrke et.al.presented the concept of aggregating attribute,value,class label pairs and we utilized this in our MapReduce implementation [7].Mappers would collect suf-ficient statistics [1]for tree construction,where each computes the candidate cutpoints by aggregating the unique attribute-value pairs.Algorithm 1details the mapper and reducer code for finding candi-date split points.During the map phase,the sufficient statistics con-sist of a key (f,v ),containing the feature f and the feature value v ,and the corresponding value (r i ,w i )consisting of the current residual and the weight of sample i .The reduce phase aggregates the residual and weight sums for each key.Given the output file,we then perform a single pass over the sorted cutpoints and the global best cut can be found.This MapReduce method reduces the complexity of finding the optimal cutpoint for each feature from the dimension of the number of samples to the number of unique sample values.This method scales particularily well on datasets with categorical or Boolean features (e.g.click data).The entire process requires another mapAlgorithm 1Aggregating candidate splits map (key,value ):F ⇐set of featuressample ⇐split(value,delim)for f in F dokey =(f,sample[f])value =(sample[residual],sample[weight])emit(key,value)end for reduce (key,values ):residual_sum ⇐0weight_sum ⇐0for v in values doresidual_sum ⇐residual_sum +v.residual weight_sum ⇐weight_sum +v.weight end foremit(key,(residual_sum,weight_sum))Algorithm 2Partitioning a Node n map (key,value ):sample ⇐split(value,delim)if sample[n.feature]<n.splitpoint thenresidual =sample[residual]+n.left_response elseresidual =sample[residual]+n.right_response end ifemit(key,value)task to partition the data for each node and a final one to apply the current ensemble after training an entire tree.Algorithm 2shows pseudo code for updating the residuals for each sample.The par-titioner writes samples out to different output files depending on which side of the split the sample ends up.The applier code is trivial to write in MapReduce and is omitted.The MapReduce implementation is relatively straight forward to implement and requires few lines of code.However,because we essentially use HDFS for communication by writing out multiple files when splitting a node,we suffer from high system overhead.Hadoop is currently just not a good fit for this class of algorithms.Due to high communication overhead,we shift the focus of the remaining parts of this section to our MPI approach.4.3Learning a Distributed Regression Tree withMPI on HadoopOur second approach tries to optimize communication by using MPI on Hadoop streaming rather than MapReduce.For this im-plementation,we chose vertical partitioning since it minimizes the communication overhead of computing a tree node.For the rest of the paper,we will be working with vertically partitioned data,unless otherwise noted.Load balancing was performed to reduce time spent waiting for stragglers.For our implementation,we used our own MPI launcher for Hadoop.4.3.1MPI on HadoopIn order to utilize existing Hadoop clusters,we modified Open-MPI to launch using Hadoop streaming.The main advantage of our approach is that we can use existing clusters without having to build out a dedicated MPI cluster.Technical challenges such as determining and communicating the master node,SSH-less job launching,and fault tolerance had to be solved in the process.4.3.2Finding the Best Split for a NodeThe best split for a node is the split c i,j that maximizes gain(c) across all unique cut points j and feature i∈F,where F is the set of all features.For vertical partitioning,each machine works on a subset of the feature space F L and have only enough information to compute the best local split S i,j for a unique cut point j and feature i∈F L.S i,j=argmax i,j{gain(c i,j)}Each machine computes the best gain among its subset of features and sends this information to all of the other machines using an MPI broadcast.Each machine then determines the global best split S∗i,j by aggregating the local splits from each machine and deter-mining which split S i,j corresponds to the cut point c i,j that maxi-mizes gain.S∗i,j=argmax i,j{gain(c i,j)}Every machine now knows the global best cut and waits for the machine with the split feature in memory to partition its samples and then transmit the updated post-split indices.4.3.3Partitioning the DataDuring traditional decision tree construction,samples are parti-tioned into two subsets after a split point is learned.In distributed partitioning,only one machine has the feature in memory to parti-tion the dataset.Therefore,only the machine with the best split can partition the data,updating other machines after it isfinished.In stochastic GBDT,we start off with a random subset of the training data for each tree.Since the targets for each sample in the next tree is the gradient of the loss function,we need the current score for every sample in the training set during boosting.This poses a unique problem in the distributed case where we have to apply the current ensemble on samples with features distributed on multiple machines.To remedy this,we modify our partition process to operate over all samples in the training data and maintain an incremental score indexscore m(x)=score m−1(x)+J mXj=1γjm I(x∈R jm)where the current score at tree m depends on the score of the sample from training the previous m−1trees,the indicator func-tion,I and the responseγjm of the corresponding region if x is in region R jm.Since the response is the residual mean of samples at the inner nodes of the tree,we can incrementally update the score index as we train new nodes.The additional overhead at each tree is inversely linear with the sampling rate.4.3.4Finding the Best Node for SplittingWe employ the same greedy tree growing procedure that splits the node with the highest gain among current leaf nodes.Although this does not guarantee the optimal tree,it is an efficient linear pro-cess and follows the method used in our non-distributed version. Other tree growing methods can be implemented with additional parallelism,such as growing by level,but in the interest of attaining identical trees,we opted to use the greedy process.4.4Stochastic Gradient Boosting withDistributed Decision TreesGradient Boosted Decision Trees is an additive regression model consisting of numerous weak decision tree learners h i(x).H k(x)=kXi=1γi h i(x)whereγi denotes the learning rate.In Stochastic Gradient Boost-ing,each weak learner h i(x)is trained on a subsample of the train-ing data.Normally,during sequential training,the target t i of sample x i for the k-th tree is the gradient of the loss function L(y i,s i),usu-ally with parameters y i being the true label of the sample and the score s i=H k−1(x i).The loss function could be as generic as least squares or as specialized as GBRank[13].A unique challenge occurs when training distributed decision trees where data is partitioned across multiple nodes.Since the loss function depends on the score of every sample in the training data for the ensemble thus far,we need to retrieve non-local fea-ture values from other machines or keep track of the sample score throughout training.Our method implements the latter to minimize communication between machines.Therefore,during training,we modify our applier function to beH k(x)=s k(x)where s k(x)is the index described in Section4.3.3,with s0(x)= 0.Boosting then follows as shown below:1.Randomly sample the training data with replacement to getsubset S k.2.Set the target r i of examples in S k to be r i=L(y i,s k(x i))where y i is the true label of the sample3.Train the k-th tree h k(x)with the samples(x i,ri),wherex i∈S k.5.EXPERIMENTSThis section overviews the results of our experiments.Since our distributed GBDT construction process is designed to produce ex-actly the same results as the non-distributed version,we do not need to evaluate for differences in accuracy between learned models.We focus solely on evaluating performance and scalability of our sys-tems.Wefirst describe our dataset and experimental setup,then the results of our MapReduce implementation.Finally,we discuss the results of our implementation utilizing MPI on Hadoop.5.1Experimental SetupOur experiments focused on evaluating the scalability of our sys-tems when more machines are added to the process or when the feature or sample size is varied.All runs were made on a Hadoop cluster with only the number of machines allocated as needed. The dataset D used in our experiments consisted of1.2million (query,uri,grade)samples with520numeric features.To stress the system,we randomly generated,while respecting the existing feature distributions,additional datasets that were multiples of the dimension of D.5.2Experimental Results5.2.1MapReduce ImplementationFigure2is a log-log plot of training times for training a decision tree node.For each of the datasets,we trained a tree and aver-aged the training time for splitting a node,partitioning the samples, and updating the residuals for its children.As expected,the larger1000500100510050 105T r a i n i n g T i m e (s e c o n d s )Number of Machines1.2 Million150KFigure 2:Training time per node using the MapReduce implementation shown in log scale.dataset saw the largest improvement in training time as more map-pers were added.Due to communication overhead,there were no further performance gains on the smaller dataset after 50machines were used.Communication costs were the main concern with this imple-mentation.We discovered that this process was restricted by high cost of communication across HDFS (Hadoop currently does not have support for inter-node communication).Scheduling of multi-ple MapReduce jobs also proved to be a costly proposition.Given these factors,we were looking at minutes per node.This implemen-tation was actually slower than the non-distributed version.From our results,we believe that MapReduce is simply not a good fit for communications heavy algorithms such as GBDT and highly iterative machine learning algorithms in general.Although our MapReduce implementation scales well with even larger datasets,we were primarily interested in improving over-all training time and changed our focus to using MPI on paratively,the MPI implementation trains an entire 20termi-nal node tree in only 9seconds with 20machines on the 1.2M sam-ple dataset while the MapReduce implementation took 273seconds to train a single node with 100machines.5.2.2MPI Implementation.To evaluate the scalability of our MPI system,we used three dif-ferent datasets of varying sizes consisting of 1.2million samples,500K samples,and 100K samples each.There were 520features across all three datasets.For each dataset,we learned 10trees with each tree trained until there were 20leaf nodes.We repeated this experiment multiple times as we varied the number of machines during our distributed training process.Figure 3shows a log-log plot of the average training time as a function of the number of machines.The graph shows that the speedups depend on the size of the training data and that improve-ments start to taper off as more machines are added.In our dataset with 100K samples,the overhead from distributed tree training was too high for our implementation to be useful.For the 500K dataset,training time was reduced in half after using 2machines but contin-ued to improve sub-linearly until 5machines were used and having no further improvements from additional machines.The biggest advantage to using our implementation came with the larger 1.2million sample dataset.We see that training time is halved by adding an additional machine and that improvements continue up to 20machines with the final improvement from 70seconds per tree to 9seconds per tree.Figure 3appears to indicate that we should be able to obtain even better scaling as our dataset grows larger.We gained a lot in terms of performance using the MPI approach,but we lost some of the scalability afforded to us in the MapReduceimplementation.Because we focused on minimizing inter-machine communication,we decided to go with a vertical partitioning of our dataset.Although most datasets have many features and will benefit from the scalability of this implementation,we do reach an upper limit when the size of one feature cannot fit in main memory on one machine.1102010 5 4 3 2 1T r a i n i n g T i m e (s e c o n d s )Number of Machines1.2 Million500K 100KFigure 3:Average training time per tree over 10trees shown in log scale.6.CONCLUSIONSWe have shown two different methods of parallelizing stochastic gradient boosted decision trees.Our first implementation follows the MapReduce paradigm and ran on Hadoop.This approach re-quired a very limited amount of code and scaled well.However,communication costs of reading from HDFS was too expensive for this method to be useful.In fact,it was slower than the sequential version.We believe that the main factor behind the results was that our communication intensive implementation is not well suited for the MapReduce paradigm.Our second method utilizes MPI and Hadoop streaming to run on the grid.This approach proved to be successful,obtained near ideal speedups,and scales well with large datasets.7.REFERENCES [1]C ARAGEA ,D.,S ILVESCU ,A.,AND H ONAVAR ,V.A framework for learningfrom distributed data using sufficient statistics and its application to learning decision trees.International Journal of Hybrid Intelligent Systems 1,2(2004).[2]C HEN ,K.,L U ,R.,W ONG ,C.K.,S UN ,G.,H ECK ,L.,AND T SENG ,B.L.Trada:tree based ranking function adaptation.In CIKM (2008),pp.1143–1152.[3]D EAN ,J.,AND G HEMAWAT ,S.Mapreduce:simplified data processing on large mun.ACM 51,1(2008),107–113.[4]F OUNDATION ,A.Apache hadoop /hadoop .[5]F RIEDMAN ,J.H.Greedy function approximation:A gradient boosting machine.Annals of Statistics 29(2001),1189–1232.[6]F RIEDMAN ,J.H.Stochastic gradient put.Stat.Data Anal.38,4(February 2002),367–378.[7]G EHRKE ,J.,R AMAKRISHNAN ,R.,AND G ANTI ,V.Rainforest -a framework for fast decision tree construction of large datasets.In VLDB’98,Proceedings of 24rd International Conference on Very Large Data Bases,August 24-27,1998,New York City,New York,USA (1998),A.Gupta,O.Shmueli,and J.Widom,Eds.,Morgan Kaufmann,pp.416–427.[8]P ROVOST ,F.,K OLLURI ,V.,AND F AYYAD ,U.A survey of methods for scaling up inductive algorithms.Data Mining and Knowledge Discovery 3(1999),131–169.[9]Q UINLAN ,J.R.Induction of decision trees.In Machine Learning (1986),pp.81–106.[10]S HAFER ,J.C.,A GRAWAL ,R.,AND 0002,M.M.Sprint:A scalable parallel classifier for data mining.In VLDB’96,Proceedings of 22th International Conference on Very Large Data Bases,September 3-6,1996,Mumbai(Bombay),India (1996),T.M.Vijayaraman,A.P.Buchmann,C.Mohan,and N.L.Sarda,Eds.,Morgan Kaufmann,pp.544–555.[11]S TATISTICS ,L.B.,AND B REIMAN ,L.Random forests.In Machine Learning (2001),pp.5–32.[12]S U ,J.,AND Z HANG ,H.A fast decision tree learning algorithm.In AAAI (2006).[13]Z HENG ,Z.,C HEN ,K.,S UN ,G.,AND Z HA ,H.A regression framework for learning ranking functions using relative relevance judgments.Proceedings of the 30th annual international ACM SIGIR conference on Research and development in information retrieval (2007),287–294.。
- 1、下载文档前请自行甄别文档内容的完整性,平台不提供额外的编辑、内容补充、找答案等附加服务。
- 2、"仅部分预览"的文档,不可在线预览部分如存在完整性等问题,可反馈申请退款(可完整预览的文档不适用该条件!)。
- 3、如文档侵犯您的权益,请联系客服反馈,我们会尽快为您处理(人工客服工作时间:9:00-18:30)。
TOWARDS STOCHASTIC CONJUGATE GRADIENT METHODSNicol N.Schraudolph Thore Graepelschraudo@inf.ethz.ch graepel@inf.ethz.chInstitute of Computational ScienceETH Z¨u rich,Switzerlandhttp://www.icos.ethz.ch/To appear in Proc.9th Intl.Conf.Neural Information Processing,Singapore2002ABSTRACTThe method of conjugate gradients provides a very effectiveway to optimize large,deterministic systems by gradient de-scent.In its standard form,however,it is not amenable tostochastic approximation of the gradient.Here we explorea number of ways to adopt ideas from conjugate gradient in the stochastic setting,using fast Hessian-vector products toobtain curvature information cheaply.In our benchmark ex-periments the resulting highly scalable algorithms converge about an order of magnitude faster than ordinary stochasticgradient descent.1.INTRODUCTIONFor the optimization of large,differentiable systems,meth-ods that require the inversion of a curvature matrix(Leven-berg-Marquardt[1,2]),or the storage of an iterative ap-proximation of that inverse(quasi-Newton methods such as BFGS),are prohibitively expensive.Conjugate gradienttechniques[3],which are capable of exactly minimizing a -dimensional unconstrained quadratic problem in itera-tions without requiring explicit knowledge of the curvaturematrix,have become the method of choice for such cases. Their rapid convergence,however,breaks down when the function to be optimized is noisy,as occurs in online(stoch-astic)gradient descent problems.Here the state of the art is simple gradient descent,coupled with adaptation of local step size parameters.The most advanced of these meth-ods,SMD[4,5],uses curvature matrix-vector products that can be obtained efficiently and automatically[5,6].Here we use the same curvature matrix-vector products to adopt some ideas from conjugate gradient in the stochastic setting.2.CONJUGATE GRADIENTBasic concepts.The fundamental idea of conjugate gradi-ent methods is to successively minimize the parameters of a differentiable function along a set of non-interfering search directions.Two directions and are non-interfering if they are conjugate,that is,(1) where is the Hessian of the system.A set of mutually conjugate directions is produced by the iteration(2) where denotes the gradient at the-th itera-tion,and(5)Nonlinear problems.For conjugate gradient on nonlinear optimization problems,an explicit line minimization is usu-ally employed to set the step size.However,it has been shown that a local quadratic approximation(i.e.,the given in(5)above)can be very effective here,provided that suit-able trust-region modifications are made(scaled conjugate gradient[7]).Of course a nonlinear problem will not be minimized exactly after iterations,but the method can be restarted at that point by resetting to the current gradient. Fast curvature matrix-vector products.It should be noted that the calculation of in(5)does not require explicit stor-age of the Hessian,which would be.There are waysto calculate the product of the Hessian with an arbitrary vector at a cost comparable to that of obtaining the gradi-ent,which typically is[6].The same goes for other measures of curvature,such as the Gauss-Newton approx-imation of the Hessian,and the Fisher information matrix [5].Algorithmic differentiation software1provides generic implementations of the building blocks from which these algorithms are constructed.3.STOCHASTIC GRADIENT DESCENTEmpirical loss functions are often minimized using noisy measurements of gradient(and,if applicable,curvature)ob-tained on small,random(hence“stochastic”)subsamples (“batches”)of data,or even individual data points(batch size=1).This is done for reasons of computational effi-ciency on large,redundant data sets,and out of necessity when adapting online to a continual stream of noisy,poten-tially non-stationary data.Simple stochastic gradient.Unfortunately conjugate gra-dient techniques are not designed to cope with noisy gra-dient and curvature information,and hence tend to diverge in stochastic settings.Occasional reports to the contrary in-variably concern regimes that we would term near-determi-nistic(i.e.,large batch sizes).For relatively small systems, the extended Kalmanfilter is a robust alternative,albeit at a cost of per rge stochastic systems are therefore still often optimized using simple(first-order)gra-dient descent.Local step size adaptation.The convergence of simple stochastic gradient descent can be improved by adjusting a local step size for each system parameter.The most ad-vanced of these algorithms,stochastic meta-descent(SMD), adapts local step sizes by a dual gradient descent procedure that uses fast curvature matrix-vector products[4,5]. Adaptive momentum.This method adapts the system pa-rameters by an iterative stochastic approximation of sec-ond-order gradient steps.As formulated originally[8]it was unfortunately stable only for linear systems.We have re-cently succeeded in making it robust for nonlinear optimiza-tion problems by incorporating a trust-region modification [9].This algorithm also uses fast curvature matrix-vector products.4.TOW ARDS STOCHASTIC CONJUGATEGRADIENT:THREE ALGORITHMSWe now seek to improve upon the above stochastic gradi-ent methods by adopting certain ideas from conjugate gradi-ent.The resulting three new algorithms all use fast Hessian-gradient products.(7) This choice of achieves pairwise conjugation of gradients (i.e.,)in a deterministic quadratic bowl,as can easily be verified using the identity. Diagonal Conditioner.In systems where the Hessian has some sparsity,it is advantageous to be able to decouple the step sizes for individual subspaces.To this end we construct a diagonal conditioner from(7)by replacing the inner prod-ucts by Hadamard(component-wise)products and division. In order to remove the poles resulting from zero compo-nents in the denominator,we then average both numerator and denominator over the trajectory,obtaining(10) Note that an accurate line minimization along ensures that,so in that case the above reduces to stan-dard conjugate gradient.The correction term comes into play only to the extent that the line minimization fails and the gradient grows in magnitude,reducing the impact on of this pathological situation.5.QUADRATIC BOWL EXPERIMENTS Deterministic bowl.The-dimensional quadratic bowl pro-vides us with a simplified test setting in which every aspect of the optimization can be controlled.It is defined by the unconstrained problem of minimizing with respect to pa-rameters the function(13) where is a matrix collecting a batch of random input vectors to the system,each drawn i.i.d.from a normal distribution:.This means that,so that in expectation this is identical to the deterministic formulation:if mod modotherwise(17)We call the optimization problem resulting from settingto this matrix the modified Hilbert bowl. Experiments.We tested the three proposed new algorithmsin deterministic,intermediate(batch size5),and fully stoch-astic(batch size1)settings on the modified Hilbert bowls ofdimension5and20,whose Hessians have condition num-bers of and,respectively.For compari-son,we also tested standard conjugate gradient—equations(2),(3),(4),and(5)—and simple gradient descent with theglobal step size set to the inverse of the largest eigenvalue of the Hessian.We repeated each experiment for100differentrandom starting points on the unit hypersphere,and reportthe geometric mean loss over those repetitions.Results(Fig.1).Unsurprisingly,both conjugate gradientalgorithms show identical,strong performance in the deter-ministic setting but diverge in the fully stochastic one.In theintermediate regime,however,standard conjugate gradientgets stuck early on(flat curves),whereas our stabilized vari-ant continues to perform,converging an order of magnitudefaster than simple gradient descent.The pairwise conjugation method is the best algorithmin the fully stochastic setting,where it converges an order of magnitude faster than simple gradient descent(),andgives reliable performance where the latter diverges( ).We note that its performance in fact improves with increasing stochasticity(from to)—a remark-able feat.Finally,our diagonally conditioned method(with )also converges reliably,if somewhat more slowly than pairwise conjugation.We expect that the decoupling will make diagonal conditioning advantageous on sparser problems.6.SUMMARY AND CONCLUSIONS Adopting ideas from conjugate gradient methods,we have proposed three new stochastic gradient descent techniques, and tested them in very ill-conditioned quadratic bowls.The results are quite promising in that one of our techniques outperforms simple stochastic gradient descent by an order of magnitude in all settings.We are now investigating the adaptation of our algorithms to nonlinear optimization prob-lems,such as online learning in multi-layer perceptrons.We also hope to further improve upon these techniques through a systematic rederivation of the conjugate gradient method in the linear stochastic setting[10].7.REFERENCES[1]K.Levenberg.A method for the solution of certainnon-linear problems in least squares.Quarterly Jour-nal of Applied Mathematics,II(2):164–168,1944.01101001000100001e-001e-021e-041e-061e-081e-101e-12deterministic110100100010000intermediate (b = 5)110100100010000stochastic (b = 1)d = 501101001000100001e-061e-051e-041e-031e-021e-011e-00110100100010000110100100010000d = 20Figure 1:Log-log plots of average loss (geometric mean over 100repetitions)vs.iterations of training in modified Hilbert bowls of dimension (top)and (bottom),for conjugate gradient (dot-dashed),stabilized conjugate gradient(dot-dot-dashed),pairwise conjugation (solid),diagonal conditioner (,dotted),and simple gradient descent (dashed).[2]D.Marquardt.An algorithm for least-squares estima-tion of non-linear parameters.Journal of the Society ofIndustrial and Applied Mathematics ,11(2):431–441,1963.[3]M.R.Hestenes and E.Stiefel.Methods of conjugategradients for solving linear systems.Journal of Re-search of the National Bureau of Standards ,49:409–436,1952.[4]N.N.Schraudolph.Local gain adaptation in stochas-tic gradient descent.In Proceedings of the 9th In-ternational Conference on Artificial Neural Networks ,pages 569–574,Edinburgh,Scotland,1999.IEE,London.http://www.inf.ethz.ch/˜schraudo/pubs/smd.ps.gz .[5]N.N.Schraudolph.Fast curvature matrix-vector prod-ucts for second-order gradient descent.Neural Com-putation ,14(7):1723–1738,2002.[6]B.A.Pearlmutter.Fast exact multiplication by theHessian.Neural Computation ,6(1):147–160,1994.[7]M.F.Møller.A scaled conjugate gradient algorithmfor fast supervised learning.Neural Networks ,6(4):525–533,1993.[8]G.B.Orr.Dynamics and Algorithms for StochasticLearning .PhD thesis,Department of Computer Science and Engineering,Oregon Graduate Institute,Beaverton,OR 97006,1995.ftp://neural.cse./pub/neural/papers/orrPhDch1-5.ps.Z ,orrPhDch6-9.ps.Z .[9]T.Graepel and N.N.Schraudolph.Stable adaptivemomentum for rapid online learning in nonlinear sys-tems.In Dorronsoro [11].http://www.inf.ethz.ch/˜schraudo/pubs/sam.ps.gz .[10]N.N.Schraudolph and T.Graepel.Conjugate direc-tions for stochastic gradient descent.In Dorronsoro [11].http://www.inf.ethz.ch/˜schraudo/pubs/hg.ps.gz .[11]J.R.Dorronsoro,editor.Proceedings of the Inter-national Conference on Artificial Neural Networks ,volume 2415of Lecture Notes in Computer Science ,Madrid,Spain,2002.Springer Verlag,Berlin.。