大数据挖掘-advertising-19
2020年高考英语外刊时事命题阅读理解:在“大数据”的“加持”下,户外广告牌焕发新生 Sign of the Times

Sign of the Times在“大数据”的“加持”下,户外广告牌焕发新生。
PEDESTRIANS walking down 8th Avenue in Manhattan’s Hell’s Kitchen neighborhood will be struck by the face-side(正面) of the Hearst Magazine Building. The 3,716-square-metre building is decorated with columns and statues, and topped by a 183-meter glass and steel skyscraper.{1} Another distinctive feature is a vast digital screen that transmits advertisements. Despite being one of the world’s oldest forms of marketing, the outdoor-advertising business is embracing digital technologies.Most forms of traditional advertising — print, radio and broadcast television — have been losing ground to online ads for years. Only billboards, dating back to the 1800s, and TV ads are holding their own (see chart below). Out-of-home (OOH) advertising is estimated to have grown by 3.4 percent in 2018; digital out-of-home (DOOH) advertising, which includes the LCD screens (液晶屏) found in airports and shopping malls, by 16 percent. Such ads draw viewers’ attentionfrom phones and cannot be skipped or blocked, unlike ads online.Billboard owners are also making hay from the location data that are pouring off people’s smartphones.{2} Information about their owners’ whereabouts and online browsing gets collected and anonymized (匿名处理) by carriers (运营商) and data firms, and sold to media owners. They then use these data to work out when different groups — say, business travelers — walk by their ads. That knowledge is added to insights into traffic, weather and other data to produce highly relevant ads. For example, DOOH providers can deliver ads for coffee when it is cold.Billboards can also be programmed to show ads for allergy (过敏症) medication when the air is full of pollen(花粉).Such targeting works particularly well when it is accompanied by “programmatic” advertising methods. Supporters say outdoor ads will increasingly be bought like online ones, based on audience and views as well as location.That is possible because billboard owners claim to be able to measure how well their ads are working, even though no “click-through” rates are involved. Data firms can tell advertisers how many people walk past individual advertisements at particular times of the day. Advertisers can estimate how many individuals exposed to an ad go on to visit a nearby shop (or website) and buy the product. Such metrics (指标) make outdoor ads more data-driven, automated and measurable.Tech giants are among those to see more value in outdoor advertising. Netflix acquired a series of billboards along Hollywood’s Sunset Strip, where it advertises its films and TV shows. Tech firms, including Apple and Google, are heavy buyers of OOH ads, accounting for 25 of the top 100 OOH ad spenders in America.The outdoor-ad revolution is not problem-free, however. The collection of mobile phone data raises privacy concerns. And criticisms of the online-ad business may also turn to the OOH business as it becomes bigger and more complex. One of the benefits of following the online-ad leaders is that the OOH advertisers can learn from their mistakes.(选自Economist)1. The underlined words in Paragraph 3 probably mean “______.”A. restricting access toB. earning a profit fromC. taking advantage ofD. finding fault with2. According to the article, which of the following statements is true?A. The billboard has a history of more than 300 years.B. The billboard is the only form of conventional advertising that winsagainst online ads.C. DOOH refers to any type of digital billboards used for advertising outdoors.D. It is estimated that OOH advertising grew slower than DOOH advertising in 2018.3. What can we infer from the article?A. Billboard advertising is more likely to be targeted toward business travelers.B. “Click-through” rates are the only reliable metrics in advertising.C. Tech giants invest more money in OOH ads than in online ones.D. The outdoor-ad industry is likely to address privacy concerns.译文:沿着曼哈顿地狱厨房街区的第八大街行走的PEDESTRIANS将被赫斯特杂志大楼正面遮住。
25个大数据专业术语入门大数据必备知识

25个大数据专业术语入门大数据必备知识大数据是指跨越传统数据处理能力范围,无法使用常规数据库工具进行处理和管理的大量、高速度、多样性的结构化和非结构化数据的集合。
随着信息技术的高速发展,大数据已经成为当今社会的热门话题之一。
掌握大数据的相关专业术语对于大数据领域的从业者和对大数据感兴趣的人来说至关重要。
本文将介绍25个常见的大数据专业术语,帮助读者快速入门大数据领域。
1. 数据挖掘(Data Mining)数据挖掘是指通过分析大量数据来发现隐藏在其中的模式和关联性的过程。
通过数据挖掘技术,可以从海量数据中提取有价值的知识和信息,支持决策和业务发展。
2. 机器学习(Machine Learning)机器学习是一种人工智能的技术,通过让计算机系统从数据中学习和改进,实现自主学习和自主决策的能力。
机器学习在大数据处理中起到了重要作用,可以从大量数据中挖掘出模式和规律。
3. 云计算(Cloud Computing)云计算是一种基于互联网的计算方式,可以通过网络提供各种计算资源和服务。
云计算通过将计算任务分配给大量的计算机集群来处理大数据,提高计算效率和资源利用率。
4. 流式处理(Stream Processing)流式处理是指对实时产生的数据流进行实时分析和处理的技术。
在大数据领域,流式处理可以对海量的实时数据进行连续的计算和分析,实现实时决策和实时应用。
5. 数据湖(Data Lake)数据湖是指一个存储了各种结构化和非结构化数据的集合,可以容纳大量的原始数据。
数据湖不要求进行数据的预处理或格式转换,使得数据的获取和利用更加灵活和高效。
6. 数据仓库(Data Warehouse)数据仓库是指一个用于存储和管理各种企业数据的集中化数据存储系统。
数据仓库通过将来自不同数据源的数据进行整合和清洗,为企业决策提供可靠的数据支持。
7. 数据可视化(Data Visualization)数据可视化是指使用图表、图像和其他可视化方式将数据表达出来的过程。
科技英语词汇大全
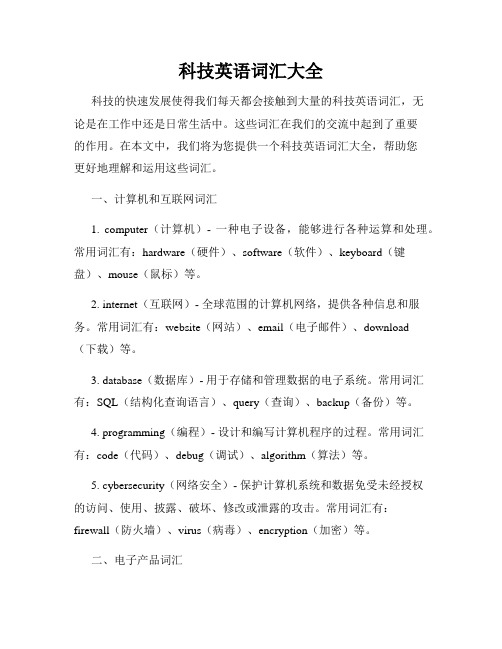
科技英语词汇大全科技的快速发展使得我们每天都会接触到大量的科技英语词汇,无论是在工作中还是日常生活中。
这些词汇在我们的交流中起到了重要的作用。
在本文中,我们将为您提供一个科技英语词汇大全,帮助您更好地理解和运用这些词汇。
一、计算机和互联网词汇1. computer(计算机)- 一种电子设备,能够进行各种运算和处理。
常用词汇有:hardware(硬件)、software(软件)、keyboard(键盘)、mouse(鼠标)等。
2. internet(互联网)- 全球范围的计算机网络,提供各种信息和服务。
常用词汇有:website(网站)、email(电子邮件)、download(下载)等。
3. database(数据库)- 用于存储和管理数据的电子系统。
常用词汇有:SQL(结构化查询语言)、query(查询)、backup(备份)等。
4. programming(编程)- 设计和编写计算机程序的过程。
常用词汇有:code(代码)、debug(调试)、algorithm(算法)等。
5. cybersecurity(网络安全)- 保护计算机系统和数据免受未经授权的访问、使用、披露、破坏、修改或泄露的攻击。
常用词汇有:firewall(防火墙)、virus(病毒)、encryption(加密)等。
二、电子产品词汇1. smartphone(智能手机)- 具有智能功能的移动电话。
常用词汇有:android(安卓)、iOS(苹果操作系统)、app(应用程序)等。
2. tablet(平板电脑)- 一种便携式的计算机设备,通常具有触摸屏和无键盘设计。
常用词汇有:iPad、Android平板电脑等。
3. laptop(笔记本电脑)- 一种便携式的个人计算机。
常用词汇有:Windows笔记本电脑、MacBook等。
4. camera(相机)- 用于拍摄照片和录制视频的设备。
常用词汇有:digital(数码)camera、DSLR(数码单反相机)等。
数据挖掘简介

数据挖掘简介数据挖掘简介2010-04-28 20:47数据挖掘数据挖掘(Data Mining)是采用数学、统计、人工智能和神经网络等领域的科学方法,从大量数据中挖掘出隐含的、先前未知的、对决策有潜在价值的关系、模式和趋势,并用这些知识和规则建立用于决策支持的模型,为商业智能系统服务的各业务领域提供预测性决策支持的方法、工具和过程。
数据挖掘前身是知识发现(KDD),属于机器学习的范畴,所用技术和工具主要有统计分析(或数据分析)和知识发现。
知识发现与数据挖掘是人工智能、机器学习与数据库技术相结合的产物,是从数据中发现有用知识的整个过程。
机器学习(Machine Learning)是用计算机模拟人类学习的一门科学,由于在专家系统开发中存在知识获取的瓶颈现象,所以采用机器学习来完成知识的自动获取。
数据挖掘是KDD过程中的一个特定步骤,它用专门算法从数据中抽取模式(Patterns)。
1996年,Fayyad、Piatetsky-Shapiror和Smyth将KDD过程定义为:从数据中鉴别出有效模式的非平凡过程,该模式是新的、可能有用的和最终可理解的;KDD是从大量数据中提取出可信的、新颖的、有效的,并能被人理解的模式的处理过程,这种处理过程是一种高级的处理过程。
数据挖掘则是按照既定的业务目标,对大量的企业数据进行探索,揭示隐藏其中的规律性,并进一步将其设计为先进的模型和有效的操作。
在日常的数据库操作中,经常使用的是从数据库中抽取数据以生成一定格式的报表。
KDD与数据库报表工具的区别是:数据库报表制作工具是将数据库中的某些数据抽取出来,经过一些数学运算,最终以特定的格式呈现给用户;而KDD则是对数据背后隐藏的特征和趋势进行分析,最终给出关于数据的总体特征和发展趋势。
报表工具能制作出形如"上学期考试未通过及成绩优秀的学生的有关情况"的表格;但它不能回答"考试未通过及成绩优秀的学生在某些方面有些什么不同的特征"的问题,而KDD就可以回答。
大数据挖掘——数据挖掘的方法
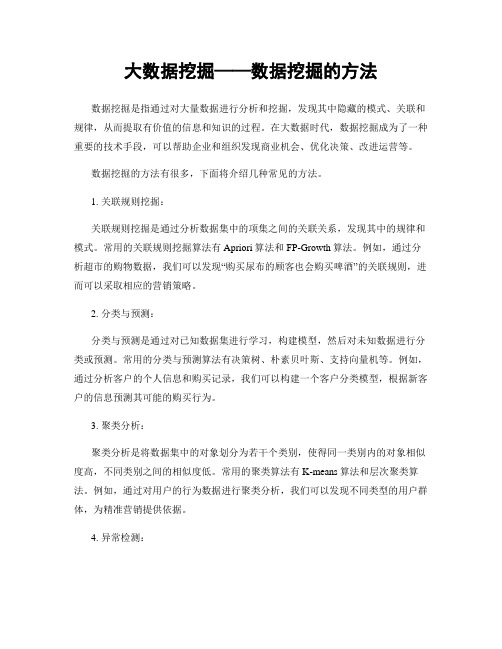
大数据挖掘——数据挖掘的方法数据挖掘是指通过对大量数据进行分析和挖掘,发现其中隐藏的模式、关联和规律,从而提取有价值的信息和知识的过程。
在大数据时代,数据挖掘成为了一种重要的技术手段,可以帮助企业和组织发现商业机会、优化决策、改进运营等。
数据挖掘的方法有很多,下面将介绍几种常见的方法。
1. 关联规则挖掘:关联规则挖掘是通过分析数据集中的项集之间的关联关系,发现其中的规律和模式。
常用的关联规则挖掘算法有Apriori算法和FP-Growth算法。
例如,通过分析超市的购物数据,我们可以发现“购买尿布的顾客也会购买啤酒”的关联规则,进而可以采取相应的营销策略。
2. 分类与预测:分类与预测是通过对已知数据集进行学习,构建模型,然后对未知数据进行分类或预测。
常用的分类与预测算法有决策树、朴素贝叶斯、支持向量机等。
例如,通过分析客户的个人信息和购买记录,我们可以构建一个客户分类模型,根据新客户的信息预测其可能的购买行为。
3. 聚类分析:聚类分析是将数据集中的对象划分为若干个类别,使得同一类别内的对象相似度高,不同类别之间的相似度低。
常用的聚类算法有K-means算法和层次聚类算法。
例如,通过对用户的行为数据进行聚类分析,我们可以发现不同类型的用户群体,为精准营销提供依据。
4. 异常检测:异常检测是通过分析数据集中的异常值,发现其中的异常模式和趋势。
常用的异常检测算法有基于统计的方法和基于机器学习的方法。
例如,在金融领域,通过对交易数据进行异常检测,可以及时发现欺诈行为。
5. 文本挖掘:文本挖掘是通过对大量的文本数据进行分析和挖掘,提取其中的主题、情感、关键词等信息。
常用的文本挖掘算法有词频统计、主题模型、情感分析等。
例如,通过对社交媒体上的用户评论进行情感分析,可以了解用户对产品或服务的满意度。
6. 时间序列分析:时间序列分析是对按时间顺序排列的数据进行建模和预测。
常用的时间序列分析方法有ARIMA模型、指数平滑法等。
数据挖掘与机器学习在在线广告推荐系统中的应用

数据挖掘与机器学习在在线广告推荐系统中的应用随着互联网的快速发展,在线广告推荐系统成为了数字广告行业的重要组成部分。
数据挖掘与机器学习作为现代计算机科学领域的前沿技术,为在线广告推荐系统的发展和优化提供了强大的支持。
本文将探讨数据挖掘与机器学习在在线广告推荐系统中的应用,并介绍其在提升广告效果和用户体验方面的优势。
一、用户画像与兴趣模型在线广告推荐系统的核心目标是根据用户的兴趣和需求,为其推荐最相关和个性化的广告内容。
数据挖掘与机器学习技术可以通过分析用户的历史行为数据,构建用户画像和兴趣模型,从而实现精准推荐。
例如,可以使用聚类算法将用户划分为不同的兴趣群体,进而根据用户所在群体的特征推荐相关广告。
此外,通过机器学习的方法,可以预测用户的兴趣变化和行为趋势,及时调整广告推荐策略,提高广告点击率和转化率。
二、广告内容优化与个性化推荐传统的广告投放往往是采用广播式的方式,将相同的广告内容推送给所有的用户。
而数据挖掘与机器学习技术可以根据用户的兴趣和偏好,实现广告内容的优化和个性化推荐。
通过分析用户的历史点击行为、浏览记录等数据,可以了解用户的兴趣爱好和消费需求,并根据这些信息进行广告内容的定制。
例如,当用户在浏览某个电商网站时,系统可以根据用户的购买记录和浏览行为,推荐关联性高的商品广告,提高广告的点击率和购买转化率。
三、CTR预估与智能投放CTR(Click-Through Rate)预估是在线广告推荐系统中的重要任务,目的是预测用户对广告的点击概率,从而帮助广告主和平台选择最合适的广告位。
数据挖掘与机器学习技术可以通过分析用户的历史点击数据和广告的特征,构建CTR预估模型,实现对广告效果的预测。
通过CTR预估,广告主可以根据不同广告位的CTR指标,选择最优的广告位置进行投放;广告平台可以根据CTR预估模型,自动选择合适的广告进行投放,提高广告整体效果。
四、反作弊与安全保障在线广告推荐系统面临着各种类型的恶意行为和作弊行为,如刷点击、恶意竞价等,这些行为严重影响了广告主和广告平台的权益。
关于大数据你应该知道的50个专业术语
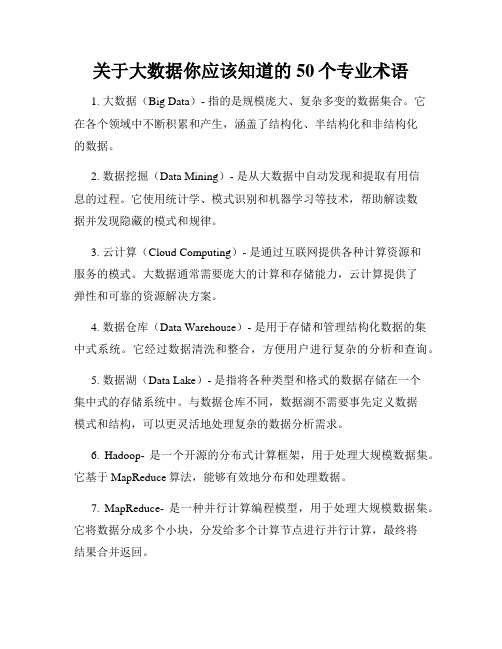
关于大数据你应该知道的50个专业术语1. 大数据(Big Data)- 指的是规模庞大、复杂多变的数据集合。
它在各个领域中不断积累和产生,涵盖了结构化、半结构化和非结构化的数据。
2. 数据挖掘(Data Mining)- 是从大数据中自动发现和提取有用信息的过程。
它使用统计学、模式识别和机器学习等技术,帮助解读数据并发现隐藏的模式和规律。
3. 云计算(Cloud Computing)- 是通过互联网提供各种计算资源和服务的模式。
大数据通常需要庞大的计算和存储能力,云计算提供了弹性和可靠的资源解决方案。
4. 数据仓库(Data Warehouse)- 是用于存储和管理结构化数据的集中式系统。
它经过数据清洗和整合,方便用户进行复杂的分析和查询。
5. 数据湖(Data Lake)- 是指将各种类型和格式的数据存储在一个集中式的存储系统中。
与数据仓库不同,数据湖不需要事先定义数据模式和结构,可以更灵活地处理复杂的数据分析需求。
6. Hadoop- 是一个开源的分布式计算框架,用于处理大规模数据集。
它基于MapReduce算法,能够有效地分布和处理数据。
7. MapReduce- 是一种并行计算编程模型,用于处理大规模数据集。
它将数据分成多个小块,分发给多个计算节点进行并行计算,最终将结果合并返回。
8. Spark- 是一个快速、通用、高级的大数据处理引擎。
它支持内存计算,能够在大数据集上进行复杂的数据处理和分析。
9. 数据可视化(Data Visualization)- 是将数据以图表、图形和其他可视化形式展示的过程。
它能够帮助用户更好地理解和分析数据,发现潜在的信息和见解。
10. 数据清洗(Data Cleaning)- 是处理和修正数据中的错误、缺失和不一致之前的过程。
清洗后的数据更加准确可靠,有助于后续的分析和应用。
11. 数据集成(Data Integration)- 是将来自不同数据源的数据合并成一个统一的数据集的过程。
数据挖掘在日常生活中的应用
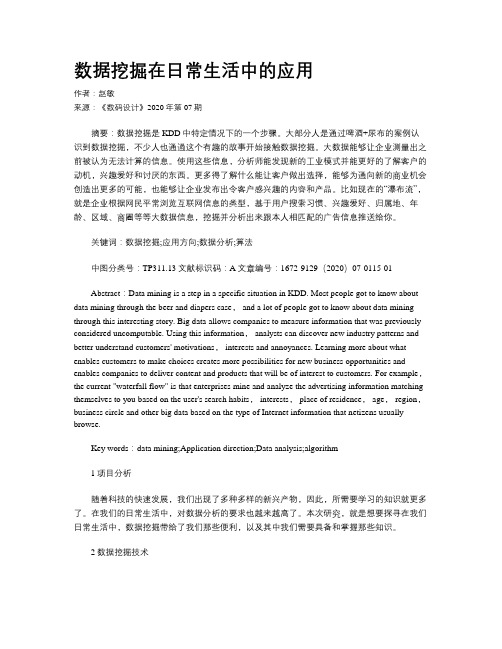
数据挖掘在日常生活中的应用作者:赵敏来源:《数码设计》2020年第07期摘要:数据挖掘是KDD中特定情况下的一个步骤。
大部分人是通过啤酒+尿布的案例认识到数据挖掘,不少人也通過这个有趣的故事开始接触数据挖掘。
大数据能够让企业测量出之前被认为无法计算的信息。
使用这些信息,分析师能发现新的工业模式并能更好的了解客户的动机,兴趣爱好和讨厌的东西。
更多得了解什么能让客户做出选择,能够为通向新的商业机会创造出更多的可能,也能够让企业发布出令客户感兴趣的内容和产品。
比如现在的“瀑布流”,就是企业根据网民平常浏览互联网信息的类型,基于用户搜索习惯、兴趣爱好、归属地、年龄、区域、商圈等等大数据信息,挖掘并分析出来跟本人相匹配的广告信息推送给你。
关键词:数据挖掘;应用方向;数据分析;算法中图分类号:TP311.13文献标识码:A文章编号:1672-9129(2020)07-0115-01Abstract:Data mining is a step in a specific situation in KDD. Most people got to know about data mining through the beer and diapers case, and a lot of people got to know about data mining through this interesting story. Big data allows companies to measure information that was previously considered uncomputable. Using this information, analysts can discover new industry patterns and better understand customers' motivations, interests and annoyances. Learning more about what enables customers to make choices creates more possibilities for new business opportunities and enables companies to deliver content and products that will be of interest to customers. For example,the current "waterfall flow" is that enterprises mine and analyze the advertising information matching themselves to you based on the user's search habits, interests, place of residence, age, region,business circle and other big data based on the type of Internet information that netizens usually browse.Key words:data mining;Application direction;Data analysis;algorithm1项目分析随着科技的快速发展,我们出现了多种多样的新兴产物,因此,所需要学习的知识就更多了。
大数据的经典的四种算法
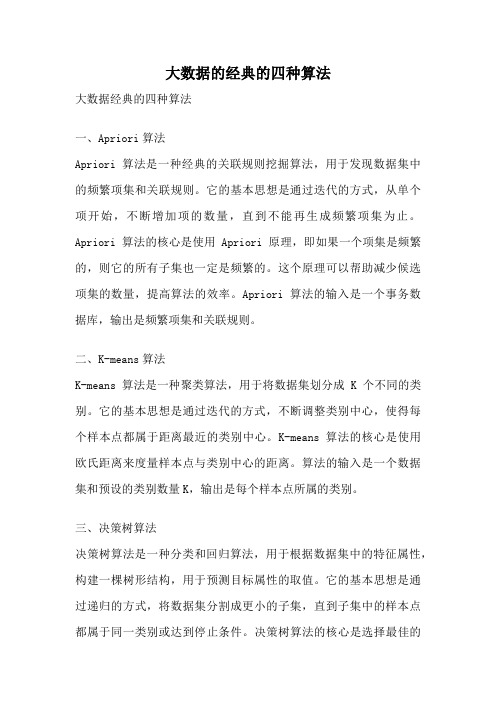
大数据的经典的四种算法大数据经典的四种算法一、Apriori算法Apriori算法是一种经典的关联规则挖掘算法,用于发现数据集中的频繁项集和关联规则。
它的基本思想是通过迭代的方式,从单个项开始,不断增加项的数量,直到不能再生成频繁项集为止。
Apriori算法的核心是使用Apriori原理,即如果一个项集是频繁的,则它的所有子集也一定是频繁的。
这个原理可以帮助减少候选项集的数量,提高算法的效率。
Apriori算法的输入是一个事务数据库,输出是频繁项集和关联规则。
二、K-means算法K-means算法是一种聚类算法,用于将数据集划分成K个不同的类别。
它的基本思想是通过迭代的方式,不断调整类别中心,使得每个样本点都属于距离最近的类别中心。
K-means算法的核心是使用欧氏距离来度量样本点与类别中心的距离。
算法的输入是一个数据集和预设的类别数量K,输出是每个样本点所属的类别。
三、决策树算法决策树算法是一种分类和回归算法,用于根据数据集中的特征属性,构建一棵树形结构,用于预测目标属性的取值。
它的基本思想是通过递归的方式,将数据集分割成更小的子集,直到子集中的样本点都属于同一类别或达到停止条件。
决策树算法的核心是选择最佳的划分属性和划分点。
算法的输入是一个数据集,输出是一个决策树模型。
四、朴素贝叶斯算法朴素贝叶斯算法是一种基于贝叶斯定理的分类算法,用于根据数据集中的特征属性,预测目标属性的取值。
它的基本思想是假设特征属性之间相互独立,通过计算后验概率来进行分类。
朴素贝叶斯算法的核心是使用贝叶斯定理和条件独立性假设。
算法的输入是一个数据集,输出是一个分类模型。
五、支持向量机算法支持向量机算法是一种用于分类和回归的机器学习算法,用于找到一个超平面,将不同类别的样本点分开。
它的基本思想是找到一个最优的超平面,使得离它最近的样本点到超平面的距离最大化。
支持向量机算法的核心是通过求解凸二次规划问题来确定超平面。
算法的输入是一个数据集,输出是一个分类或回归模型。
大数据英文版
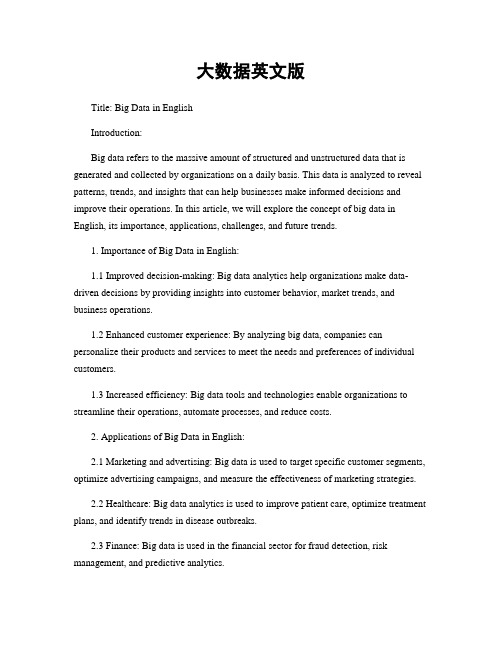
大数据英文版Title: Big Data in EnglishIntroduction:Big data refers to the massive amount of structured and unstructured data that is generated and collected by organizations on a daily basis. This data is analyzed to reveal patterns, trends, and insights that can help businesses make informed decisions and improve their operations. In this article, we will explore the concept of big data in English, its importance, applications, challenges, and future trends.1. Importance of Big Data in English:1.1 Improved decision-making: Big data analytics help organizations make data-driven decisions by providing insights into customer behavior, market trends, and business operations.1.2 Enhanced customer experience: By analyzing big data, companies can personalize their products and services to meet the needs and preferences of individual customers.1.3 Increased efficiency: Big data tools and technologies enable organizations to streamline their operations, automate processes, and reduce costs.2. Applications of Big Data in English:2.1 Marketing and advertising: Big data is used to target specific customer segments, optimize advertising campaigns, and measure the effectiveness of marketing strategies.2.2 Healthcare: Big data analytics is used to improve patient care, optimize treatment plans, and identify trends in disease outbreaks.2.3 Finance: Big data is used in the financial sector for fraud detection, risk management, and predictive analytics.3. Challenges of Big Data in English:3.1 Data privacy and security: With the increasing amount of data being collected, organizations face challenges in protecting sensitive information from cyber threats and data breaches.3.2 Data quality and governance: Ensuring the accuracy, consistency, and reliability of data is crucial for successful big data analytics.3.3 Scalability and infrastructure: Organizations need to invest in scalable infrastructure and technology to handle the volume, velocity, and variety of big data.4. Future Trends in Big Data in English:4.1 Artificial intelligence and machine learning: AI and ML technologies are being integrated into big data analytics to automate processes, uncover insights, and improve decision-making.4.2 Edge computing: With the rise of IoT devices, edge computing is becoming more important for processing and analyzing data closer to the source.4.3 Data ethics and regulation: As big data continues to grow, there is a need for ethical guidelines and regulations to ensure the responsible use of data.5. Conclusion:In conclusion, big data plays a crucial role in helping organizations gain insights, improve decision-making, and enhance their operations. By understanding the importance, applications, challenges, and future trends of big data in English, businesses can harness the power of data to drive innovation and success.。
大数据分析中的数据挖掘算法

大数据分析中的数据挖掘算法大数据时代的到来已经让数据分析成为了人们必须面对的重要工作。
作为数据分析的重要工具之一,数据挖掘算法显得尤为重要。
在众多的数据挖掘算法中,哪些算法比较适用于大数据分析呢?下面将分析几种常用的数据挖掘算法。
1. Apriori算法Apriori算法是一种用于挖掘关联规则的算法,它可以发现数据中已知的任何类型之间的相互关系。
在大数据分析中,Apriori算法可以用于挖掘出数据的规律和模式,通过对这些规律和模式的分析,可以帮助企业在市场中更好地应对竞争。
Apriori算法是一种基于频繁项集的挖掘算法,它的核心思想是首先找到数据中出现频率最高的模式,然后再继续挖掘该模式的子集。
2. K-Means算法K-Means算法是一种聚类算法,它可以将数据划分为多个不同的类别。
在大数据分析中,K-Means算法可以用于对数据进行分类和预测。
通过将大量的数据分为多个类别,并对每个类别进行独立的分析,可以更加快速和准确地分析大数据。
K-Means算法的主要思想是通过对数据进行聚类,使得同一类别的数据相似度更高。
该算法一般通过距离的计算来确定数据之间的相似度。
3. 决策树算法决策树算法是一种通过分类和回归来进行数据挖掘的算法。
在大数据分析中,决策树算法可以用于对数据进行预测和分类。
通过对决策树的构建和优化,可以更加精确地分析大数据中的潜在规律。
决策树算法的主要思想是通过不断的迭代和优化,将数据分为高度相关的几个类别,并在每个类别中进行进一步的分析。
在将数据分为不同的类别时,决策树算法主要通过信息增益的计算来确定每个类别之间的差异。
4. 随机森林算法随机森林算法是一种基于分类和回归的算法,它可以对数据进行预测和分类。
在大数据分析中,随机森林算法可以用于分析数据中的潜在规律和模式,从而帮助企业更好地应对市场竞争。
随机森林算法的主要思想是通过将多颗决策树结合起来,形成一个“森林”。
在每个决策树的构建过程中,都会随机选取一定数量的数据和特征,从而使得每个决策树都具有独立的分析和预测能力。
最新-数据挖掘论文(精选10篇)范文
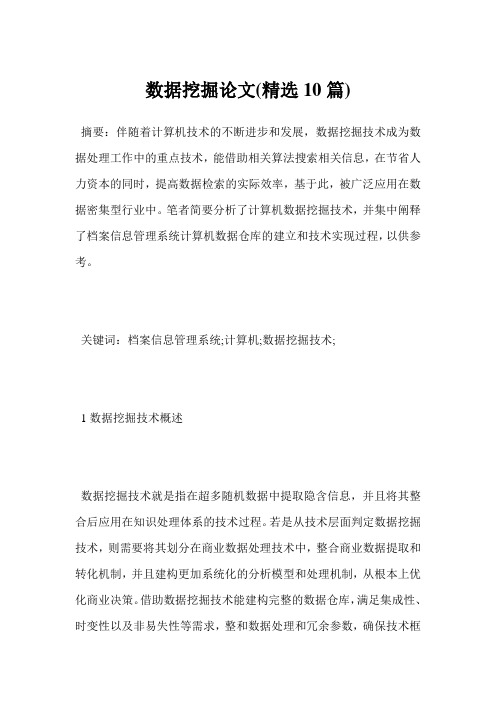
数据挖掘论文(精选10篇)摘要:伴随着计算机技术的不断进步和发展,数据挖掘技术成为数据处理工作中的重点技术,能借助相关算法搜索相关信息,在节省人力资本的同时,提高数据检索的实际效率,基于此,被广泛应用在数据密集型行业中。
笔者简要分析了计算机数据挖掘技术,并集中阐释了档案信息管理系统计算机数据仓库的建立和技术实现过程,以供参考。
关键词:档案信息管理系统;计算机;数据挖掘技术;1数据挖掘技术概述数据挖掘技术就是指在超多随机数据中提取隐含信息,并且将其整合后应用在知识处理体系的技术过程。
若是从技术层面判定数据挖掘技术,则需要将其划分在商业数据处理技术中,整合商业数据提取和转化机制,并且建构更加系统化的分析模型和处理机制,从根本上优化商业决策。
借助数据挖掘技术能建构完整的数据仓库,满足集成性、时变性以及非易失性等需求,整和数据处理和冗余参数,确保技术框架结构的完整性。
目前,数据挖掘技术常用的工具,如SAS企业的EnterpriseMiner、IBM企业的IntellientMiner以及SPSS企业的Clementine等应用都十分广泛。
企业在实际工作过程中,往往会利用数据源和数据预处理工具进行数据定型和更新管理,并且应用聚类分析模块、决策树分析模块以及关联分析算法等,借助数据挖掘技术对相关数据进行处理。
2档案信息管理系统计算机数据仓库的建立2.1客户需求单元为了充分发挥档案信息管理系统的优势,要结合客户的实际需求建立完整的处理框架体系。
在数据库体系建立中,要适应迭代式处理特征,并且从用户需求出发整合数据模型,保证其建立过程能按照整体规划有序进行,且能按照目标和分析框架参数完成操作。
首先,要确立基础性的数据仓库对象,由于是档案信息管理,因此,要集中划分档案数据分析的主题,并且有效录入档案信息,确保满足档案的数据分析需求。
其次,要对日常工作中的用户数据进行集中的挖掘处理,从根本上提高数据仓库分析的完整性。
大数据常用的算法
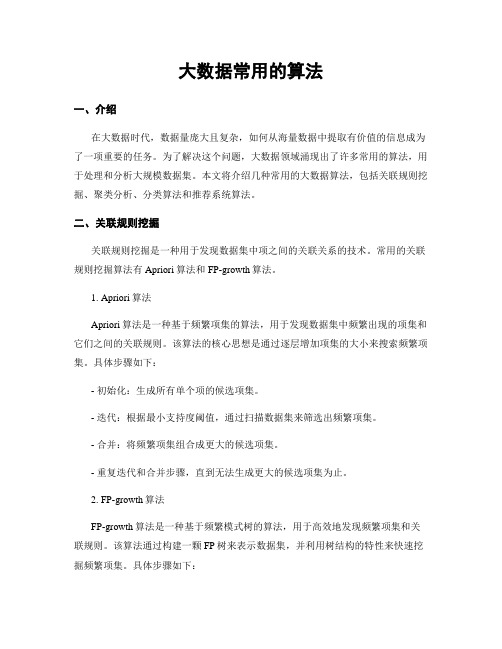
大数据常用的算法一、介绍在大数据时代,数据量庞大且复杂,如何从海量数据中提取有价值的信息成为了一项重要的任务。
为了解决这个问题,大数据领域涌现出了许多常用的算法,用于处理和分析大规模数据集。
本文将介绍几种常用的大数据算法,包括关联规则挖掘、聚类分析、分类算法和推荐系统算法。
二、关联规则挖掘关联规则挖掘是一种用于发现数据集中项之间的关联关系的技术。
常用的关联规则挖掘算法有Apriori算法和FP-growth算法。
1. Apriori算法Apriori算法是一种基于频繁项集的算法,用于发现数据集中频繁出现的项集和它们之间的关联规则。
该算法的核心思想是通过逐层增加项集的大小来搜索频繁项集。
具体步骤如下:- 初始化:生成所有单个项的候选项集。
- 迭代:根据最小支持度阈值,通过扫描数据集来筛选出频繁项集。
- 合并:将频繁项集组合成更大的候选项集。
- 重复迭代和合并步骤,直到无法生成更大的候选项集为止。
2. FP-growth算法FP-growth算法是一种基于频繁模式树的算法,用于高效地发现频繁项集和关联规则。
该算法通过构建一颗FP树来表示数据集,并利用树结构的特性来快速挖掘频繁项集。
具体步骤如下:- 构建FP树:遍历数据集,统计每个项的频次,并构建FP树。
- 构建条件模式基:根据FP树,生成每个项的条件模式基。
- 递归挖掘频繁项集:对每个项的条件模式基,递归地构建子FP树,并挖掘频繁项集。
三、聚类分析聚类分析是一种将数据集中的对象划分为不同组或簇的技术。
常用的聚类算法有K-means算法和DBSCAN算法。
1. K-means算法K-means算法是一种基于距离的聚类算法,用于将数据集划分为K个簇。
该算法的核心思想是通过迭代优化簇的中心点,使得每个样本点与其所属的簇中心点的距离最小化。
具体步骤如下:- 初始化:随机选择K个样本作为初始的簇中心点。
- 分配:对于每个样本点,计算其与各个簇中心点的距离,并将其分配到距离最近的簇中。
大数据挖掘主要算法
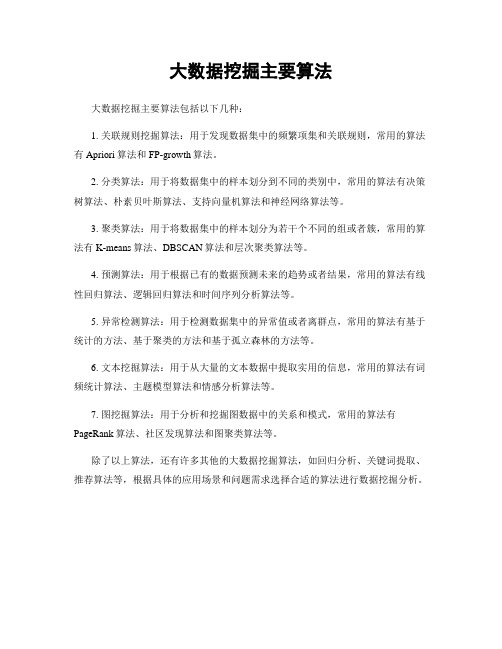
大数据挖掘主要算法
大数据挖掘主要算法包括以下几种:
1. 关联规则挖掘算法:用于发现数据集中的频繁项集和关联规则,常用的算法有Apriori算法和FP-growth算法。
2. 分类算法:用于将数据集中的样本划分到不同的类别中,常用的算法有决策树算法、朴素贝叶斯算法、支持向量机算法和神经网络算法等。
3. 聚类算法:用于将数据集中的样本划分为若干个不同的组或者簇,常用的算法有K-means算法、DBSCAN算法和层次聚类算法等。
4. 预测算法:用于根据已有的数据预测未来的趋势或者结果,常用的算法有线性回归算法、逻辑回归算法和时间序列分析算法等。
5. 异常检测算法:用于检测数据集中的异常值或者离群点,常用的算法有基于统计的方法、基于聚类的方法和基于孤立森林的方法等。
6. 文本挖掘算法:用于从大量的文本数据中提取实用的信息,常用的算法有词频统计算法、主题模型算法和情感分析算法等。
7. 图挖掘算法:用于分析和挖掘图数据中的关系和模式,常用的算法有PageRank算法、社区发现算法和图聚类算法等。
除了以上算法,还有许多其他的大数据挖掘算法,如回归分析、关键词提取、推荐算法等,根据具体的应用场景和问题需求选择合适的算法进行数据挖掘分析。
大数据算法简介-课件

分类又称为有监督的学习
2 分类算法
2.2 贝叶斯决策与分类器
算法思想
贝叶斯学派思想可概括为:先验概率+数据=后验概率
我们在实际问题中需要得到的后验概率,可以通过先验概率和数据一起综合得到。
贝叶斯学派需要假设先验分布的模型,如正态分布,beta分布等。
C5.0
C4.5算法的修订版,适用于处理大数据集,采用Boosting方式提高模型准确率,又称为 BoostingTrees,在软件上计算速度比较快,占用的内存资源较少
2 分类算法
2.3.1 决策树
决策树(Decision Tree)模型
通过对训练样本学习,建立分类规则 依据分类规则,实现对新样本的分类 属于有监督式学习方法,有两类变量
2 分类算法
2.3.3 C5.0 (3/3) --算法实例
2 分类算法
2.4 SVM (1/3) --算法思想
支持向量机(Support Vector Machine)建立在统计学习理论VC 维理论和结构风险最小 原理基础上,根据有限样本信息在模型复杂性(对特定训练样本的学习精度,Accuracy) 和学习能力(无错误地识别任意样本的能力)之间寻求最佳折中,以期获得最好泛化能力。
这个假设一般没有特定依据,因此一直被频率学派认为很荒谬。虽然难以从严密的数学逻 辑里推出贝叶斯学派的逻辑,但是在很多实际应用中,贝叶斯理论很好用,比如: 垃圾邮件分类,文本分类。
2 分类算法
2.2 贝叶斯决策与分类器 算法流程
2 分类算法
2.2.1 相关基础数学知识
条件概率
事件A 在另外一个事件B 已经发生条件下的发生概率,称为在B 条件下A 的概率。表示 P A | B
第三方数据平台精准营销收费模式研究
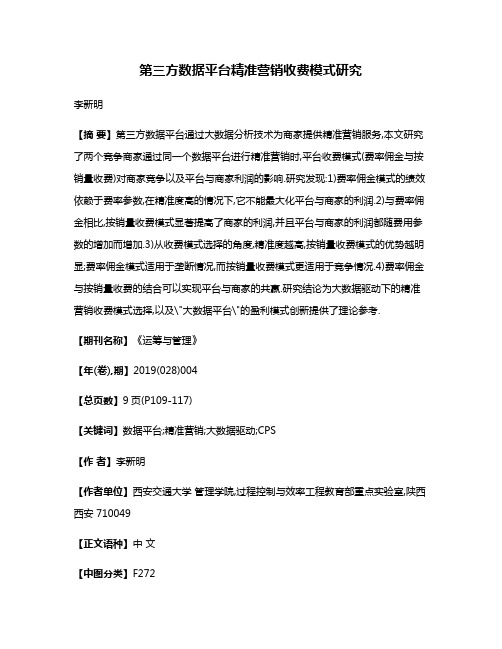
第三方数据平台精准营销收费模式研究李新明【摘要】第三方数据平台通过大数据分析技术为商家提供精准营销服务,本文研究了两个竞争商家通过同一个数据平台进行精准营销时,平台收费模式(费率佣金与按销量收费)对商家竞争以及平台与商家利润的影响.研究发现:1)费率佣金模式的绩效依赖于费率参数,在精准度高的情况下,它不能最大化平台与商家的利润.2)与费率佣金相比,按销量收费模式显著提高了商家的利润,并且平台与商家的利润都随费用参数的增加而增加.3)从收费模式选择的角度,精准度越高,按销量收费模式的优势越明显;费率佣金模式适用于垄断情况,而按销量收费模式更适用于竞争情况.4)费率佣金与按销量收费的结合可以实现平台与商家的共赢.研究结论为大数据驱动下的精准营销收费模式选择,以及\"大数据平台\"的盈利模式创新提供了理论参考.【期刊名称】《运筹与管理》【年(卷),期】2019(028)004【总页数】9页(P109-117)【关键词】数据平台;精准营销;大数据驱动;CPS【作者】李新明【作者单位】西安交通大学管理学院,过程控制与效率工程教育部重点实验室,陕西西安 710049【正文语种】中文【中图分类】F2720 引言近年来,随着云计算和互联网等信息技术的发展,“数据”作为一种新的生产要素已经渗透个各个行业和领域。
大数据正以各种方式和路径影响着企业的商业生态,它已经成为企业商业模式创新的基本时代背景[1]。
无论是百分点、盖乐传媒、亿玛在线等第三方大数据平台,还是天猫、淘宝、京东等电商平台,都积累了越来越多的用户数据,这些数据平台依靠大数据分析技术得到用户画像,从而可以向商家提供精准营销服务。
不同于传统的大众营销,精准营销是根据用户特征设定目标受众,并根据营销效果进行收费。
例如获得“最佳精准营销平台”的百分点科技推出的“营销管家”向商家提供精准的受众人群,然后帮商家向潜在用户群投放精准广告,最后根据广告效果进行收费。
大数据的英语作文

大数据的英语作文Title: The Impact and Implications of Big Data。
In the digital era, the emergence of big data has revolutionized the way we perceive and utilize information. With the exponential growth of data generated from various sources such as social media, sensors, and transactions, big data analytics has become a powerful tool forextracting valuable insights, driving innovation, and shaping decision-making processes across industries. This essay explores the impact and implications of big data on society, economy, and technology.Firstly, big data has significantly transformed business operations and strategies. By harnessing large volumes of data, organizations can gain a deeper understanding of consumer behavior, market trends, and competitive landscapes. This enables them to tailor products and services to meet customer demands more effectively, enhance marketing strategies, and optimizesupply chain management. Moreover, big data analytics facilitates predictive modeling and forecasting, empowering businesses to anticipate market shifts and mitigate risks proactively.Secondly, big data plays a pivotal role in driving scientific research and technological advancements. In fields such as healthcare, genomics, and environmental science, the analysis of massive datasets enables researchers to identify patterns, correlations, and anomalies that were previously undetectable. This has led to breakthroughs in disease diagnosis and treatment, personalized medicine, and environmental monitoring. Furthermore, big data fuels the development of artificial intelligence (AI) and machine learning algorithms, empowering machines to learn from data, make autonomous decisions, and perform complex tasks with human-like intelligence.However, the proliferation of big data also raises significant ethical and privacy concerns. The extensive collection and analysis of personal data raise questionsabout individual privacy, consent, and data ownership. Moreover, the use of big data analytics for profiling and targeted advertising has sparked debates about the manipulation of consumer behavior and the erosion of autonomy. Additionally, there are concerns about data security and the risk of data breaches, which can lead to identity theft, financial fraud, and reputational damage.Furthermore, the unequal distribution of data access and analytical capabilities exacerbates existing socioeconomic disparities. While large corporations and tech giants possess the resources to harness big data for competitive advantage, smaller businesses and underserved communities may lack the expertise and infrastructure to leverage data effectively. This digital divide widens the gap between the data-rich and the data-poor, perpetuating inequalities in access to opportunities, resources, and decision-making power.In conclusion, big data holds immense potential to drive innovation, enhance decision-making, and improve societal well-being. However, its widespread adoption alsoraises complex challenges related to privacy, ethics, and equity. As we navigate the era of big data, it is imperative to strike a balance between innovation and responsibility, ensuring that the benefits of data-driven insights are equitably distributed and ethically governed.。
- 1、下载文档前请自行甄别文档内容的完整性,平台不提供额外的编辑、内容补充、找答案等附加服务。
- 2、"仅部分预览"的文档,不可在线预览部分如存在完整性等问题,可反馈申请退款(可完整预览的文档不适用该条件!)。
- 3、如文档侵犯您的权益,请联系客服反馈,我们会尽快为您处理(人工客服工作时间:9:00-18:30)。
Online algorithms Search advertising
Online algorithms
Classic model of algorithms
You get to see the entire input, then compute some function of it In this context, “offline algorithm”
Arbitrarily pick an eligible advertiser for each keyword
Bad scenario for greedy
Two advertisers A and B A bids on query x, B bids on x and y Both have budgets of $4 Query stream: xxxxyyyy
Example of application: assigning tasks to servers
Online problem
1 2 3 4
a
b c d
(1,a) (2,b) (3,d)
Greedy algorithm
Pair the new girl with any eligible boy
[Mehta, Saberi, Vazirani, and Vazirani] For each query, pick the advertiser with the largest unspent budget
Break ties arbitrarily
Example: BALANCE
Search engine guarantees that the advertiser will not be charged more than their daily budget
Simplified model (for now)
Assume all bids are 0 or 1 Each advertiser has the same budget B One advertiser per query Let’s try the greedy algorithm
Multi-billion-dollar industry
Interesting problems
What ads to show for a search? If I’m an advertiser, which search terms should I bid on and how much to bid?
Two advertisers A and B A bids on query x, B bids on x and y Both have budgets of $4 Query stream: xxxxyyyy BALANCE choice: ABABBB__
Optimal: AAAABBBB
Instead of raw bids, use the “expected revenue per click”
The Adwords Innovation
Advertiser A B C Bid $1.00 $0.75 $0.50 CTR 1% 2% 2.5% Bid * CTR 1 cent 1.5 cents 1.125 cents
Adwords problem
A stream of queries arrives at the search engine
q1, q2,…
Several advertisers bid on each query When query qi arrives, search engine must pick a subset of advertisers whose ads are shown Goal: maximize search engine’s revenues Clearly we need an online algorithm!
Worst case greedy choice: BBBB____ Optimal: AAAABBBB Competitive ratio = ½
Simple analysis shows this is the worst case
BALANCE algorithm [MSVV]
Advertiser 1 bids $2, click probability = 0.1 Advertiser 2 bids $1, click probability = 0.5 Clickthrough rate measured historically
Simple solution
Greedy algorithm
Simplest algorithm is greedy It’s easy to see that the greedy algorithm is actually optimal!
Complications (1)
Each ad has a different likelihood of being clicked
Online problem
Initially, we are given the set Boys In each round, one girl’s choices are revealed At that time, we have to decide to either:
Pair the girl with a boy Don’t pair the girl with any boy
The Adwords Innovation
Advertiser B C A Bid $0.75 $0.50 $1.00 CTR 2% 2.5% 1% Bid * CTR 1.5 cents 1.125 cents 1 cent
Complications (2)
Each advertiser has a limited budget
If not, we can allocate more queries Assume BALANCE exhausts A2’s budget
Analyzing Balance
B Queries allocated to A1 in optimal solution Queries allocated to A2 in optimal solution A1 A2
1 2 3 4
a
b c d
(1,a) (2,b)
History of web advertising
Banner ads (1995-2001)
Initial form of web advertising Popular websites charged X$ for every 1000 “impressions” of ad
Otherwise the optimal algorithm could not have matched all the G girls
Therefore |Mgreedy| ¸|G| = |Mopt - Mgreedy| |Mgreedy|/|Mopt| ¸1/2
Worst-case scenario
A perfect one if it exists
There is a polynomial-time offline algorithm (Hopcroft and Karp 1973) But what if we don’t have the entire graph upfront?
Similar model adopted by Google with some changes around 2002
Called “Adwords”
Ads vs. search results
Web 2.0
Performance-based advertising works!
Online algorithm
You get to see the input one piece at a time, and need to make irrevocable decisions along the way
Similar to data stream models
Example: Bipartite matching
1 2 3 Girls 4
a
b c d Boys
M = {(1,c),(2,b),(3,d),(4,a)} is a perfect matching
Matching Algorithm
Problem: Find a maximum-cardinality matching for a given bipartite graph
If there is none, don’t pair girl
How good is the algorithm?
Competitiv, suppose greedy produces matching Mgreedy while an optimal matching is Mopt
Introduced by Overture around 2000
Advertisers “bid” on search keywords When someone searches for that keyword, the highest bidder’s ad is shown Advertiser is charged only if the ad is clicked on