Keith Bennett,
计算机领域著名人物
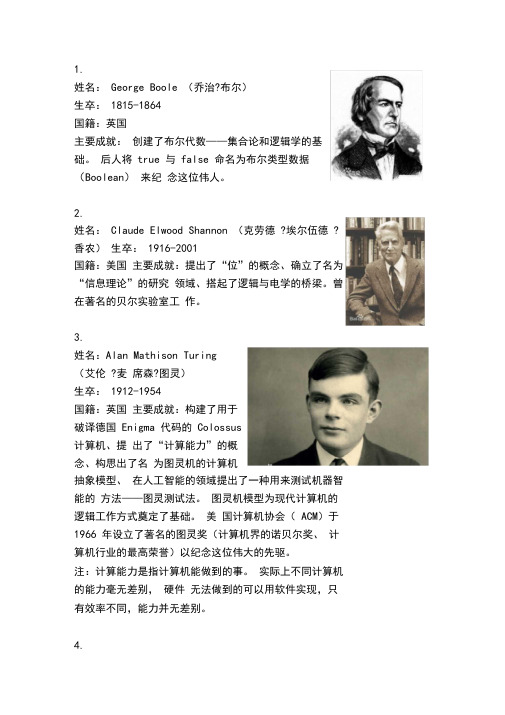
1.姓名:George Boole (乔治?布尔)生卒:1815-1864国籍:英国主要成就:创建了布尔代数——集合论和逻辑学的基础。
后人将true 与false 命名为布尔类型数据(Boolean)来纪念这位伟人。
2.姓名:Claude Elwood Shannon (克劳德?埃尔伍德?香农)生卒:1916-2001国籍:美国主要成就:提出了“位”的概念、确立了名为“信息理论”的研究领域、搭起了逻辑与电学的桥梁。
曾在著名的贝尔实验室工作。
3.姓名:Alan Mathison Turing(艾伦?麦席森?图灵)生卒:1912-1954国籍:英国主要成就:构建了用于破译德国Enigma 代码的Colossus计算机、提出了“计算能力”的概念、构思出了名为图灵机的计算机抽象模型、在人工智能的领域提出了一种用来测试机器智能的方法——图灵测试法。
图灵机模型为现代计算机的逻辑工作方式奠定了基础。
美国计算机协会(ACM)于1966 年设立了著名的图灵奖(计算机界的诺贝尔奖、计算机行业的最高荣誉)以纪念这位伟大的先驱。
注:计算能力是指计算机能做到的事。
实际上不同计算机的能力毫无差别,硬件无法做到的可以用软件实现,只有效率不同,能力并无差别。
4.姓名:Charles Babbage(查尔斯?巴贝奇)生卒:1792-1871国籍:英国主要成就:被誉为计算机的先驱、计算机革命先驱,构想了差分机、解析机。
解析机用卡片编程,设计理念同现代计算机极为相似,可以说在当时又着超越时代的认知,虽然从未完成过,但是其理念对后世影响深远。
5.姓名:Joseph Marie Jacquard(约瑟夫?玛丽?雅卡尔)生卒:1752-1834 国籍:法国主要成就:发明了自动织布机,奠定了后来卡片编程的基础6.姓名:Blaise Pasca(l 布莱斯?帕斯卡)生卒:1623-1662国籍:法国主要成就:1642 年他设计并制作了一台能自动进位的加减法计算装置,被称为是世界上第一台数字计算器,为以后的计算机设计提供了基本原理。
英语发展史_教学课件 Part 1Early_Modern_English_2
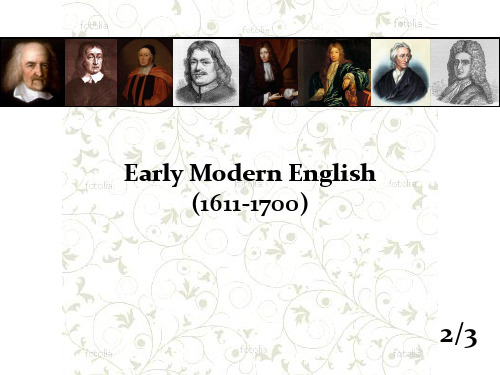
1633 King James English trading posts
established in Bengal.
1637 English traders established in
Canton.
1639 English established at Madras.
1640 Twenty-four pamphlets were
Pilgrim’s Progress. / Dryden published his greatest play: All for Love.
1679 Parliament dismissed; Charles II
rejected petitions calling for a new Parliament; petitioners became known as Whigs; their opponents (royalists) known as Tories.
Bengal
Ben Jonson
(1572-1637)
The Civil War
1646 English occupied the Bahamas.
1648 Second Civil War.
1649 Charles I beheaded.
Commonwealth established.
1651 Thomas Hobbes published his
outbreak of bubonic plague in England.
The Royal Society
1666 The great fire of London.
New Amsterdam
1667 John Milton, the greatest of English
公共政策终结理论研究综述
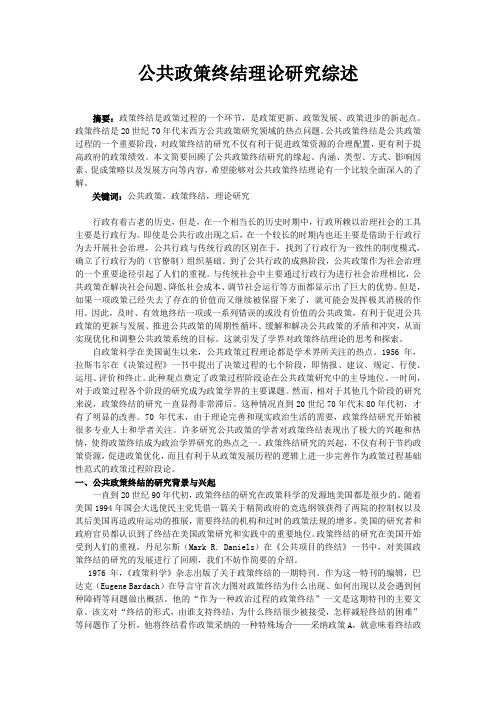
公共政策终结理论研究综述摘要:政策终结是政策过程的一个环节,是政策更新、政策发展、政策进步的新起点。
政策终结是20世纪70年代末西方公共政策研究领域的热点问题。
公共政策终结是公共政策过程的一个重要阶段,对政策终结的研究不仅有利于促进政策资源的合理配置,更有利于提高政府的政策绩效。
本文简要回顾了公共政策终结研究的缘起、内涵、类型、方式、影响因素、促成策略以及发展方向等内容,希望能够对公共政策终结理论有一个比较全面深入的了解。
关键词:公共政策,政策终结,理论研究行政有着古老的历史,但是,在一个相当长的历史时期中,行政所赖以治理社会的工具主要是行政行为。
即使是公共行政出现之后,在一个较长的时期内也还主要是借助于行政行为去开展社会治理,公共行政与传统行政的区别在于,找到了行政行为一致性的制度模式,确立了行政行为的(官僚制)组织基础。
到了公共行政的成熟阶段,公共政策作为社会治理的一个重要途径引起了人们的重视。
与传统社会中主要通过行政行为进行社会治理相比,公共政策在解决社会问题、降低社会成本、调节社会运行等方面都显示出了巨大的优势。
但是,如果一项政策已经失去了存在的价值而又继续被保留下来了,就可能会发挥极其消极的作用。
因此,及时、有效地终结一项或一系列错误的或没有价值的公共政策,有利于促进公共政策的更新与发展、推进公共政策的周期性循环、缓解和解决公共政策的矛盾和冲突,从而实现优化和调整公共政策系统的目标。
这就引发了学界对政策终结理论的思考和探索。
自政策科学在美国诞生以来,公共政策过程理论都是学术界所关注的热点。
1956年,拉斯韦尔在《决策过程》一书中提出了决策过程的七个阶段,即情报、建议、规定、行使、运用、评价和终止。
此种观点奠定了政策过程阶段论在公共政策研究中的主导地位。
一时间,对于政策过程各个阶段的研究成为政策学界的主要课题。
然而,相对于其他几个阶段的研究来说,政策终结的研究一直显得非常滞后。
这种情况直到20世纪70年代末80年代初,才有了明显的改善。
美英报刊Lessons 10 Reining In the Test of Tests
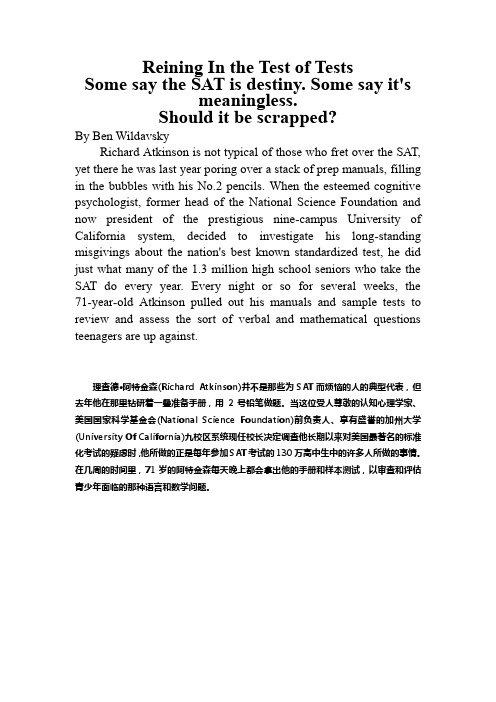
Reining In the Test of TestsSome say the SAT is destiny. Some say it'smeaningless.Should it be scrapped?By Ben WildavskyRichard Atkinson is not typical of those who fret over the SAT, yet there he was last year poring over a stack of prep manuals, filling in the bubbles with his No.2 pencils. When the esteemed cognitive psychologist, former head of the National Science Foundation and now president of the prestigious nine-campus University of California system, decided to investigate his long-standing misgivings about the nation's best known standardized test, he did just what many of the 1.3 million high school seniors who take the SAT do every year. Every night or so for several weeks, the 71-year-old Atkinson pulled out his manuals and sample tests to review and assess the sort of verbal and mathematical questions teenagers are up against.理查德·阿特金森(Richard Atkinson)并不是那些为SAT而烦恼的人的典型代表,但去年他在那里钻研着一叠准备手册,用2号铅笔做题。
1_男生英文名大全
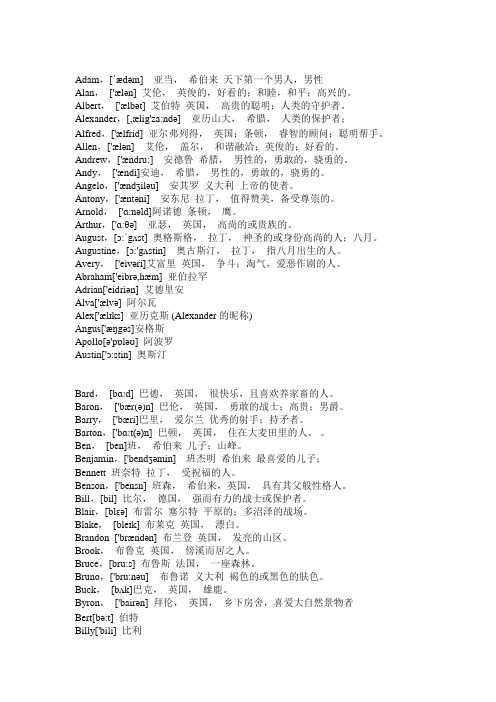
Adam,[ˈædəm] 亚当,希伯来天下第一个男人,男性Alan,['ælən] 艾伦,英俊的,好看的;和睦,和平;高兴的。
Albert,['ælbət] 艾伯特英国,高贵的聪明;人类的守护者。
Alexander,[,ælig'za:ndə] 亚历山大,希腊,人类的保护者;Alfred,['ælfrid] 亚尔弗列得,英国;条顿,睿智的顾问;聪明帮手。
Allen,['ælən] 艾伦,盖尔,和谐融洽;英俊的;好看的。
Andrew,['ændru:] 安德鲁希腊,男性的,勇敢的,骁勇的。
Andy,['ændi]安迪,希腊,男性的,勇敢的,骁勇的。
Angelo,['ændʒiləu] 安其罗义大利上帝的使者。
Antony,['æntəni] 安东尼拉丁,值得赞美,备受尊崇的。
Arnold,['ɑ:nəld]阿诺德条顿,鹰。
Arthur,['ɑ:θə] 亚瑟,英国,高尚的或贵族的。
August,[ɔ:ˈgʌst] 奥格斯格,拉丁,神圣的或身份高尚的人;八月。
Augustine,[ɔ:'gʌstin] 奥古斯汀,拉丁,指八月出生的人。
Avery,['eivəri]艾富里英国,争斗;淘气,爱恶作剧的人。
Abraham['eibrə,hæm] 亚伯拉罕Adrian['eidriən] 艾德里安Alva['ælvə] 阿尔瓦Alex['ælɪks] 亚历克斯 (Alexander的昵称)Angus['æŋgəs]安格斯Apollo[ə'pɒləʊ] 阿波罗Austin['ɔ:stin] 奥斯汀Bard,[bɑːd] 巴德,英国,很快乐,且喜欢养家畜的人。
外国人名大全男名
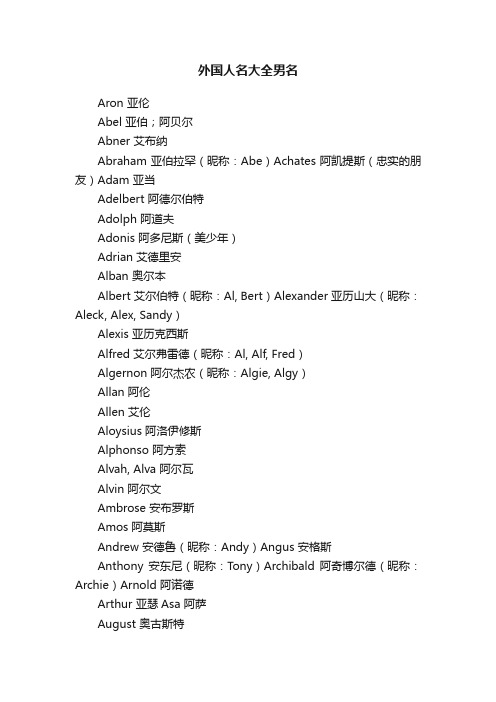
外国人名大全男名Aron 亚伦Abel 亚伯;阿贝尔Abner 艾布纳Abraham 亚伯拉罕(昵称:Abe)Achates 阿凯提斯(忠实的朋友)Adam 亚当Adelbert 阿德尔伯特Adolph 阿道夫Adonis 阿多尼斯(美少年)Adrian 艾德里安Alban 奥尔本Albert 艾尔伯特(昵称:Al, Bert)Alexander 亚历山大(昵称:Aleck, Alex, Sandy)Alexis 亚历克西斯Alfred 艾尔弗雷德(昵称:Al, Alf, Fred)Algernon 阿尔杰农(昵称:Algie, Algy)Allan 阿伦Allen 艾伦Aloysius 阿洛伊修斯Alphonso 阿方索Alvah, Alva 阿尔瓦Alvin 阿尔文Ambrose 安布罗斯Amos 阿莫斯Andrew 安德鲁(昵称:Andy)Angus 安格斯Anthony 安东尼(昵称:T ony)Archibald 阿奇博尔德(昵称:Archie)Arnold 阿诺德Arthur 亚瑟Asa 阿萨August 奥古斯特Augustine 奥古斯丁Augustus 奥古斯都;奥古斯塔斯Austin 奥斯汀Baldwin 鲍德温Barnaby 巴纳比Barnard 巴纳德Bartholomew 巴托洛缪(昵称:Bart)Benedict 本尼迪克特Benjamin 本杰明(昵称:Ben, Benny)Bennett 贝内特Bernard 伯纳德(昵称:Bernie)Bertram 伯特伦(昵称:Bertie)Bertrand 伯特兰Bill 比尔(昵称:Billy)Boris 鲍里斯Brian 布赖恩Bruce 布鲁斯Bruno 布鲁诺Bryan 布莱恩Byron 拜伦Caesar 凯撒Caleb 凯莱布Calvin 卡尔文Cecil 塞西尔Christophe 克里斯托弗Clare 克莱尔Clarence 克拉伦斯Claude 克劳德Clement 克莱门特(昵称:Clem)Clifford 克利福德(昵称:Cliff)Clifton 克利夫顿Clinton 克林顿Clive 克莱夫Clyde 克莱德Colin 科林Conan 科南Conrad 康拉德Cornelius 科尼利厄斯Crispin 克利斯平Curtis 柯蒂斯Cuthbert 卡斯伯特Cyril 西里尔Cyrus 赛勒斯(昵称:Cy)David 大卫;戴维(昵称:Dave, Davy)Denis, Dennis 丹尼斯Dominic 多米尼克Donald 唐纳德(昵称:Don)Douglas 道格拉斯(昵称:Doug)Dudley 达德利Duncan 邓肯Dwight 德怀特Earl, Earle 厄尔Earnest 欧内斯特Ebenezer 欧贝尼泽Edgar 埃德加(昵称:Ed, Ned)Edward 爱德华(昵称:Ed, Ned)Edwin 埃德温(昵称:Ed)Egbert 艾格伯特Elbert 埃尔博特Eli 伊莱Elias 伊莱亚斯Elihu 伊莱休Elijah 伊莱贾(昵称:Lige)Eliot 埃利奥特Elisha 伊莱沙(昵称:Lish)Elliot, Elliott 埃利奥特Ellis 埃利斯Elmer 艾尔默Emery, Emory 艾默里Emil 埃米尔Emmanuel 伊曼纽尔Enoch 伊诺克Enos 伊诺思Ephraim 伊弗雷姆Erasmus 伊拉兹马斯Erastus 伊拉斯塔斯Eric 埃里克Ernest 欧内斯特(昵称:Errie)Erwin 欧文Essex 埃塞克斯(埃塞克斯伯爵,英国军人和廷臣,因叛国罪被处死)Ethan 伊桑Ethelbert 埃塞尔伯特Eugene 尤金(昵称:Gene)Eurus 欧罗斯(东风神或东南风神)Eustace 尤斯塔斯Evan 埃文,伊万Evelyn 伊夫林Everett 埃弗雷特Ezakiel 伊齐基尔(昵称:Zeke)Ezra 埃兹拉Felix 菲利克斯Ferdinand 费迪南德Finlay, Finley 芬利Floyd 弗洛伊德Francis 弗朗西斯(昵称:Frank)Frank 弗兰克Franklin 富兰克林Frederick 弗雷德里克(昵称:Fred)Gabriel 加布里埃尔(昵称:Gabe)Gail 盖尔Gamaliel 甘梅利尔Gary 加里George 乔治Gerald 杰拉尔德Gerard 杰勒德Gideon 吉迪恩Gifford 吉福德Gilbert 吉尔伯特Giles 加尔斯Glenn, Glen 格伦Godfrey 戈弗雷Gordon 戈登Gregory 格雷戈里(昵称:Greg)Griffith 格里菲斯Gustavus 古斯塔夫斯Guthrie 格思里Guy 盖伊Hans 汉斯Harlan 哈伦Harold 哈罗德(昵称:Hal)Harry 哈里Harvey 哈维Hector 赫克托Henry 亨利(昵称:Hal, Hank, Henny)Herbert 赫伯特(昵称:Herb, Bert)Herman 赫尔曼Hilary 希拉里Hiram 海勒姆(昵称:Hi)Homer 霍默Horace 霍勒斯Horatio 霍雷肖Hosea 霍奇亚Howard 霍华德Hubert 休伯特Hugh 休Hugo 雨果Humphrey 汉弗莱Ichabod 伊卡伯德Ignatius 伊格内修斯Immanuel 伊曼纽尔Ira 艾拉Irene 艾林Irving, Irwin 欧文Isaac 艾萨克(昵称:Ike)Isador, Isadore 伊萨多Isaiah 埃塞亚Isidore, Isidor 伊西多(昵称:Izzy)Islington 伊斯林顿Israel 伊斯雷尔(昵称:Izzy)Ivan 伊凡Jack 杰克Jackson 杰克逊Jacob 雅各布(昵称:Jack, Jake)James 詹姆斯(昵称:Jamie, Jim, Jimmy)Jared 贾雷德Jarvis 贾维斯Jason 贾森Jasper 加斯珀Jean 琼Jeffrey 杰弗里(昵称:Jeff)Jeremiah 杰里迈亚(昵称:Jerry)Jeremy 杰里米Jerome 杰罗姆(昵称:Jerry)Jervis 杰维斯Jesse 杰西(昵称:Jess)Jesus 杰苏斯Jim 吉姆Joab 乔巴Job 乔布Joe 乔Joel 乔尔John 约翰(昵称:Jack, Johnnie, Johnny)Jonah 乔纳Jonathan 乔纳森(昵称:Jon)Joseph 约瑟夫(昵称:Joe, Jo)Joshua 乔舒亚(昵称:Josh)Josephus 约瑟夫斯Josiah 乔塞亚Judah 朱达(昵称:Jude)Jude 朱达Jules 朱尔斯Julian 朱利安(昵称:Jule)Julius 朱利叶斯(昵称:Jule, Julie)Junius 朱尼厄斯Justin 贾斯丁Justus 贾斯特斯Keith 基斯Kelvin 凯尔文Kenneth 肯尼斯(昵称:Ken)Kevin 凯文Kit 基特Larry 拉里Laurence, Lawrence 劳伦斯(昵称:Larry)Lazarus 拉扎勒斯Leander 利安德Lee 李Leif 利夫Leigh 李,利Lemuel 莱缪艾尔(昵称:Lem)Leo 利奥Leon 利昂Leonard 列奥纳多(昵称:Len, Lenny)Leopold 利奥波德Leroy 勒鲁瓦Leslie 莱斯利Lester 莱斯特(昵称:Les)Levi 利瓦伊(昵称:Lev)Lewis 刘易斯(昵称:Lew, Lewie)Lionel 莱昂内尔Lewellyn 卢埃林Loyd 劳埃德Lorenzo 洛伦佐Louis 路易斯(昵称:Lou, Louie)Lucian 卢西恩Lucius 卢修斯Luke 卢克Luther 卢瑟Lyman 莱曼Lynn 林恩Manuel 曼纽尔Marcel 马赛尔Marcellus 马塞勒斯Marcus 马库斯Marion 马里恩Mark 马克Marshal 马歇尔Martin 马丁Matthew 马修(昵称:Mat, Matt)Maurice 莫里斯Max 马克斯Maximilian 马克西米利安(昵称:Max)Maynard 梅纳德Melville 梅尔维尔Melvin 梅尔文Merle 莫尔Merlin 莫林Mervin 莫文Micah 迈卡Michael 迈克尔(昵称:Mike, Mickey)Miles 迈尔斯Milton 米尔顿(昵称:Milt, Miltie)Morgan 摩根Morton 莫顿(昵称:Mort, Morty)Moses 摩西(昵称:Mo, Mose)Murray 默里Myron 米隆Nathan 内森(昵称:Nat, Nate)Nathanael 纳撒尼尔Neal,Neil 尼尔Ned 内德Nelson 纳尼森Nestor 涅斯托尔Nicholas, Nicolas 尼古拉斯Nick 尼克Noah 诺亚Noel 诺埃尔Norman 诺曼Octavius 屋大维Oliver 奥利弗Orlando 奥兰多Orson 奥森Orville 奥维尔Oscar 奥斯卡Oswald, Oswold 奥斯瓦尔德Otis 奥蒂斯Otto 奥拓Owen 欧英Patrick 帕特里克(昵称:Paddy, Pat)Paul 保罗Perceval, Percival 帕西瓦尔(昵称:Percy)Percy 珀西Perry 佩里Peter 彼得(昵称:Pete)Philip, Phillip 菲利普(昵称:Phil)Phineas 菲尼亚斯Pierre 皮埃尔Quentin, Quintin 昆廷Ralph 拉尔夫Randal, Randall 兰德尔Randolph 兰道夫Raphael 拉斐尔Raymond 雷蒙德(昵称:Ray)Reginald 雷金纳德Reubin 鲁本Rex 雷克斯Richard 理查德Robert 罗伯特Rodney 罗德尼Roger 罗杰Roland, Rowland 罗兰Rolf 罗尔夫Ronald 罗纳德Roscoe 罗斯科Rudolph 鲁道夫Rufus 鲁弗斯Rupert 鲁珀特Russell, Russel 罗素Sammy 萨米Sam 萨姆Samson 萨姆森Samuel 塞缪尔Saul 索尔Seth 赛思Seymour 西摩尔Sidney 西德尼Sigmund 西格蒙德Silas 塞拉斯Silvester 西尔维斯特Simeon 西米恩Simon 西蒙Solomon 所罗门(昵称:Sol)Stanley 斯坦利Stephen 斯蒂芬Steven 史蒂文Stewart, Stuart 斯图尔特Sydney 西德尼Sylvanus 西尔韦纳斯Sylvester 西尔威斯特Terence 特伦斯(昵称:Terry)Thaddeus, Thadeus 萨迪厄斯Theobald 西奥波尔德Theodore 西奥多Theodosius 西奥多西Thomas 托马斯(昵称:Tom, Tommy)Timothy 蒂莫西(昵称:Tim)Titus 泰特斯Tobiah 托比厄(昵称:Toby)Tony 托尼Tristram 特里斯托拉姆(昵称:Tris)Uriah 尤赖亚Valentine 瓦伦丁Vernon 弗农Victor 维克托(昵称:Vic)Vincent 文森特Virgil 弗吉尔Waldo 沃尔多Wallace 华莱士(昵称:Wally)Walter 沃尔特(昵称:Walt, Wat)Warren 沃伦Wayne 韦恩Wesley 韦斯利Wilbert 威尔伯特Wilbur 威尔伯Wilfred, Wilfrid 威尔弗雷德Willard 威拉德William 威廉(昵称:Will, Willy)Willis 威利斯Winfred 温弗雷德Woodrow 伍德罗(昵称:Woody)Zachariah 扎卡赖亚(昵称:Zach)Zacharias 扎卡赖亚斯Zachary 扎卡里Zebulun 泽布伦。
《神经网络与深度学习综述DeepLearning15May2014
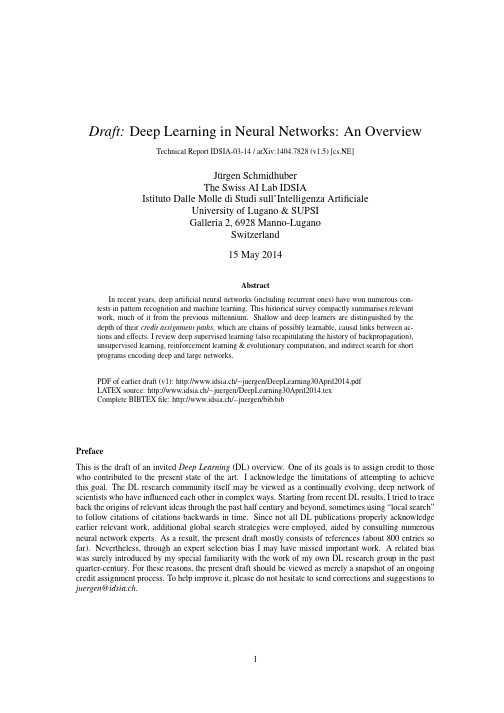
Draft:Deep Learning in Neural Networks:An OverviewTechnical Report IDSIA-03-14/arXiv:1404.7828(v1.5)[cs.NE]J¨u rgen SchmidhuberThe Swiss AI Lab IDSIAIstituto Dalle Molle di Studi sull’Intelligenza ArtificialeUniversity of Lugano&SUPSIGalleria2,6928Manno-LuganoSwitzerland15May2014AbstractIn recent years,deep artificial neural networks(including recurrent ones)have won numerous con-tests in pattern recognition and machine learning.This historical survey compactly summarises relevantwork,much of it from the previous millennium.Shallow and deep learners are distinguished by thedepth of their credit assignment paths,which are chains of possibly learnable,causal links between ac-tions and effects.I review deep supervised learning(also recapitulating the history of backpropagation),unsupervised learning,reinforcement learning&evolutionary computation,and indirect search for shortprograms encoding deep and large networks.PDF of earlier draft(v1):http://www.idsia.ch/∼juergen/DeepLearning30April2014.pdfLATEX source:http://www.idsia.ch/∼juergen/DeepLearning30April2014.texComplete BIBTEXfile:http://www.idsia.ch/∼juergen/bib.bibPrefaceThis is the draft of an invited Deep Learning(DL)overview.One of its goals is to assign credit to those who contributed to the present state of the art.I acknowledge the limitations of attempting to achieve this goal.The DL research community itself may be viewed as a continually evolving,deep network of scientists who have influenced each other in complex ways.Starting from recent DL results,I tried to trace back the origins of relevant ideas through the past half century and beyond,sometimes using“local search”to follow citations of citations backwards in time.Since not all DL publications properly acknowledge earlier relevant work,additional global search strategies were employed,aided by consulting numerous neural network experts.As a result,the present draft mostly consists of references(about800entries so far).Nevertheless,through an expert selection bias I may have missed important work.A related bias was surely introduced by my special familiarity with the work of my own DL research group in the past quarter-century.For these reasons,the present draft should be viewed as merely a snapshot of an ongoing credit assignment process.To help improve it,please do not hesitate to send corrections and suggestions to juergen@idsia.ch.Contents1Introduction to Deep Learning(DL)in Neural Networks(NNs)3 2Event-Oriented Notation for Activation Spreading in FNNs/RNNs3 3Depth of Credit Assignment Paths(CAPs)and of Problems4 4Recurring Themes of Deep Learning54.1Dynamic Programming(DP)for DL (5)4.2Unsupervised Learning(UL)Facilitating Supervised Learning(SL)and RL (6)4.3Occam’s Razor:Compression and Minimum Description Length(MDL) (6)4.4Learning Hierarchical Representations Through Deep SL,UL,RL (6)4.5Fast Graphics Processing Units(GPUs)for DL in NNs (6)5Supervised NNs,Some Helped by Unsupervised NNs75.11940s and Earlier (7)5.2Around1960:More Neurobiological Inspiration for DL (7)5.31965:Deep Networks Based on the Group Method of Data Handling(GMDH) (8)5.41979:Convolution+Weight Replication+Winner-Take-All(WTA) (8)5.51960-1981and Beyond:Development of Backpropagation(BP)for NNs (8)5.5.1BP for Weight-Sharing Feedforward NNs(FNNs)and Recurrent NNs(RNNs)..95.6Late1980s-2000:Numerous Improvements of NNs (9)5.6.1Ideas for Dealing with Long Time Lags and Deep CAPs (10)5.6.2Better BP Through Advanced Gradient Descent (10)5.6.3Discovering Low-Complexity,Problem-Solving NNs (11)5.6.4Potential Benefits of UL for SL (11)5.71987:UL Through Autoencoder(AE)Hierarchies (12)5.81989:BP for Convolutional NNs(CNNs) (13)5.91991:Fundamental Deep Learning Problem of Gradient Descent (13)5.101991:UL-Based History Compression Through a Deep Hierarchy of RNNs (14)5.111992:Max-Pooling(MP):Towards MPCNNs (14)5.121994:Contest-Winning Not So Deep NNs (15)5.131995:Supervised Recurrent Very Deep Learner(LSTM RNN) (15)5.142003:More Contest-Winning/Record-Setting,Often Not So Deep NNs (16)5.152006/7:Deep Belief Networks(DBNs)&AE Stacks Fine-Tuned by BP (17)5.162006/7:Improved CNNs/GPU-CNNs/BP-Trained MPCNNs (17)5.172009:First Official Competitions Won by RNNs,and with MPCNNs (18)5.182010:Plain Backprop(+Distortions)on GPU Yields Excellent Results (18)5.192011:MPCNNs on GPU Achieve Superhuman Vision Performance (18)5.202011:Hessian-Free Optimization for RNNs (19)5.212012:First Contests Won on ImageNet&Object Detection&Segmentation (19)5.222013-:More Contests and Benchmark Records (20)5.22.1Currently Successful Supervised Techniques:LSTM RNNs/GPU-MPCNNs (21)5.23Recent Tricks for Improving SL Deep NNs(Compare Sec.5.6.2,5.6.3) (21)5.24Consequences for Neuroscience (22)5.25DL with Spiking Neurons? (22)6DL in FNNs and RNNs for Reinforcement Learning(RL)236.1RL Through NN World Models Yields RNNs With Deep CAPs (23)6.2Deep FNNs for Traditional RL and Markov Decision Processes(MDPs) (24)6.3Deep RL RNNs for Partially Observable MDPs(POMDPs) (24)6.4RL Facilitated by Deep UL in FNNs and RNNs (25)6.5Deep Hierarchical RL(HRL)and Subgoal Learning with FNNs and RNNs (25)6.6Deep RL by Direct NN Search/Policy Gradients/Evolution (25)6.7Deep RL by Indirect Policy Search/Compressed NN Search (26)6.8Universal RL (27)7Conclusion271Introduction to Deep Learning(DL)in Neural Networks(NNs) Which modifiable components of a learning system are responsible for its success or failure?What changes to them improve performance?This has been called the fundamental credit assignment problem(Minsky, 1963).There are general credit assignment methods for universal problem solvers that are time-optimal in various theoretical senses(Sec.6.8).The present survey,however,will focus on the narrower,but now commercially important,subfield of Deep Learning(DL)in Artificial Neural Networks(NNs).We are interested in accurate credit assignment across possibly many,often nonlinear,computational stages of NNs.Shallow NN-like models have been around for many decades if not centuries(Sec.5.1).Models with several successive nonlinear layers of neurons date back at least to the1960s(Sec.5.3)and1970s(Sec.5.5). An efficient gradient descent method for teacher-based Supervised Learning(SL)in discrete,differentiable networks of arbitrary depth called backpropagation(BP)was developed in the1960s and1970s,and ap-plied to NNs in1981(Sec.5.5).BP-based training of deep NNs with many layers,however,had been found to be difficult in practice by the late1980s(Sec.5.6),and had become an explicit research subject by the early1990s(Sec.5.9).DL became practically feasible to some extent through the help of Unsupervised Learning(UL)(e.g.,Sec.5.10,5.15).The1990s and2000s also saw many improvements of purely super-vised DL(Sec.5).In the new millennium,deep NNs havefinally attracted wide-spread attention,mainly by outperforming alternative machine learning methods such as kernel machines(Vapnik,1995;Sch¨o lkopf et al.,1998)in numerous important applications.In fact,supervised deep NNs have won numerous of-ficial international pattern recognition competitions(e.g.,Sec.5.17,5.19,5.21,5.22),achieving thefirst superhuman visual pattern recognition results in limited domains(Sec.5.19).Deep NNs also have become relevant for the more generalfield of Reinforcement Learning(RL)where there is no supervising teacher (Sec.6).Both feedforward(acyclic)NNs(FNNs)and recurrent(cyclic)NNs(RNNs)have won contests(Sec.5.12,5.14,5.17,5.19,5.21,5.22).In a sense,RNNs are the deepest of all NNs(Sec.3)—they are general computers more powerful than FNNs,and can in principle create and process memories of ar-bitrary sequences of input patterns(e.g.,Siegelmann and Sontag,1991;Schmidhuber,1990a).Unlike traditional methods for automatic sequential program synthesis(e.g.,Waldinger and Lee,1969;Balzer, 1985;Soloway,1986;Deville and Lau,1994),RNNs can learn programs that mix sequential and parallel information processing in a natural and efficient way,exploiting the massive parallelism viewed as crucial for sustaining the rapid decline of computation cost observed over the past75years.The rest of this paper is structured as follows.Sec.2introduces a compact,event-oriented notation that is simple yet general enough to accommodate both FNNs and RNNs.Sec.3introduces the concept of Credit Assignment Paths(CAPs)to measure whether learning in a given NN application is of the deep or shallow type.Sec.4lists recurring themes of DL in SL,UL,and RL.Sec.5focuses on SL and UL,and on how UL can facilitate SL,although pure SL has become dominant in recent competitions(Sec.5.17-5.22). Sec.5is arranged in a historical timeline format with subsections on important inspirations and technical contributions.Sec.6on deep RL discusses traditional Dynamic Programming(DP)-based RL combined with gradient-based search techniques for SL or UL in deep NNs,as well as general methods for direct and indirect search in the weight space of deep FNNs and RNNs,including successful policy gradient and evolutionary methods.2Event-Oriented Notation for Activation Spreading in FNNs/RNNs Throughout this paper,let i,j,k,t,p,q,r denote positive integer variables assuming ranges implicit in the given contexts.Let n,m,T denote positive integer constants.An NN’s topology may change over time(e.g.,Fahlman,1991;Ring,1991;Weng et al.,1992;Fritzke, 1994).At any given moment,it can be described as afinite subset of units(or nodes or neurons)N= {u1,u2,...,}and afinite set H⊆N×N of directed edges or connections between nodes.FNNs are acyclic graphs,RNNs cyclic.Thefirst(input)layer is the set of input units,a subset of N.In FNNs,the k-th layer(k>1)is the set of all nodes u∈N such that there is an edge path of length k−1(but no longer path)between some input unit and u.There may be shortcut connections between distant layers.The NN’s behavior or program is determined by a set of real-valued,possibly modifiable,parameters or weights w i(i=1,...,n).We now focus on a singlefinite episode or epoch of information processing and activation spreading,without learning through weight changes.The following slightly unconventional notation is designed to compactly describe what is happening during the runtime of the system.During an episode,there is a partially causal sequence x t(t=1,...,T)of real values that I call events.Each x t is either an input set by the environment,or the activation of a unit that may directly depend on other x k(k<t)through a current NN topology-dependent set in t of indices k representing incoming causal connections or links.Let the function v encode topology information and map such event index pairs(k,t)to weight indices.For example,in the non-input case we may have x t=f t(net t)with real-valued net t= k∈in t x k w v(k,t)(additive case)or net t= k∈in t x k w v(k,t)(multiplicative case), where f t is a typically nonlinear real-valued activation function such as tanh.In many recent competition-winning NNs(Sec.5.19,5.21,5.22)there also are events of the type x t=max k∈int (x k);some networktypes may also use complex polynomial activation functions(Sec.5.3).x t may directly affect certain x k(k>t)through outgoing connections or links represented through a current set out t of indices k with t∈in k.Some non-input events are called output events.Note that many of the x t may refer to different,time-varying activations of the same unit in sequence-processing RNNs(e.g.,Williams,1989,“unfolding in time”),or also in FNNs sequentially exposed to time-varying input patterns of a large training set encoded as input events.During an episode,the same weight may get reused over and over again in topology-dependent ways,e.g.,in RNNs,or in convolutional NNs(Sec.5.4,5.8).I call this weight sharing across space and/or time.Weight sharing may greatly reduce the NN’s descriptive complexity,which is the number of bits of information required to describe the NN (Sec.4.3).In Supervised Learning(SL),certain NN output events x t may be associated with teacher-given,real-valued labels or targets d t yielding errors e t,e.g.,e t=1/2(x t−d t)2.A typical goal of supervised NN training is tofind weights that yield episodes with small total error E,the sum of all such e t.The hope is that the NN will generalize well in later episodes,causing only small errors on previously unseen sequences of input events.Many alternative error functions for SL and UL are possible.SL assumes that input events are independent of earlier output events(which may affect the environ-ment through actions causing subsequent perceptions).This assumption does not hold in the broaderfields of Sequential Decision Making and Reinforcement Learning(RL)(Kaelbling et al.,1996;Sutton and Barto, 1998;Hutter,2005)(Sec.6).In RL,some of the input events may encode real-valued reward signals given by the environment,and a typical goal is tofind weights that yield episodes with a high sum of reward signals,through sequences of appropriate output actions.Sec.5.5will use the notation above to compactly describe a central algorithm of DL,namely,back-propagation(BP)for supervised weight-sharing FNNs and RNNs.(FNNs may be viewed as RNNs with certainfixed zero weights.)Sec.6will address the more general RL case.3Depth of Credit Assignment Paths(CAPs)and of ProblemsTo measure whether credit assignment in a given NN application is of the deep or shallow type,I introduce the concept of Credit Assignment Paths or CAPs,which are chains of possibly causal links between events.Let usfirst focus on SL.Consider two events x p and x q(1≤p<q≤T).Depending on the appli-cation,they may have a Potential Direct Causal Connection(PDCC)expressed by the Boolean predicate pdcc(p,q),which is true if and only if p∈in q.Then the2-element list(p,q)is defined to be a CAP from p to q(a minimal one).A learning algorithm may be allowed to change w v(p,q)to improve performance in future episodes.More general,possibly indirect,Potential Causal Connections(PCC)are expressed by the recursively defined Boolean predicate pcc(p,q),which in the SL case is true only if pdcc(p,q),or if pcc(p,k)for some k and pdcc(k,q).In the latter case,appending q to any CAP from p to k yields a CAP from p to q(this is a recursive definition,too).The set of such CAPs may be large but isfinite.Note that the same weight may affect many different PDCCs between successive events listed by a given CAP,e.g.,in the case of RNNs, or weight-sharing FNNs.Suppose a CAP has the form(...,k,t,...,q),where k and t(possibly t=q)are thefirst successive elements with modifiable w v(k,t).Then the length of the suffix list(t,...,q)is called the CAP’s depth (which is0if there are no modifiable links at all).This depth limits how far backwards credit assignment can move down the causal chain tofind a modifiable weight.1Suppose an episode and its event sequence x1,...,x T satisfy a computable criterion used to decide whether a given problem has been solved(e.g.,total error E below some threshold).Then the set of used weights is called a solution to the problem,and the depth of the deepest CAP within the sequence is called the solution’s depth.There may be other solutions(yielding different event sequences)with different depths.Given somefixed NN topology,the smallest depth of any solution is called the problem’s depth.Sometimes we also speak of the depth of an architecture:SL FNNs withfixed topology imply a problem-independent maximal problem depth bounded by the number of non-input layers.Certain SL RNNs withfixed weights for all connections except those to output units(Jaeger,2001;Maass et al.,2002; Jaeger,2004;Schrauwen et al.,2007)have a maximal problem depth of1,because only thefinal links in the corresponding CAPs are modifiable.In general,however,RNNs may learn to solve problems of potentially unlimited depth.Note that the definitions above are solely based on the depths of causal chains,and agnostic of the temporal distance between events.For example,shallow FNNs perceiving large“time windows”of in-put events may correctly classify long input sequences through appropriate output events,and thus solve shallow problems involving long time lags between relevant events.At which problem depth does Shallow Learning end,and Deep Learning begin?Discussions with DL experts have not yet yielded a conclusive response to this question.Instead of committing myself to a precise answer,let me just define for the purposes of this overview:problems of depth>10require Very Deep Learning.The difficulty of a problem may have little to do with its depth.Some NNs can quickly learn to solve certain deep problems,e.g.,through random weight guessing(Sec.5.9)or other types of direct search (Sec.6.6)or indirect search(Sec.6.7)in weight space,or through training an NNfirst on shallow problems whose solutions may then generalize to deep problems,or through collapsing sequences of(non)linear operations into a single(non)linear operation—but see an analysis of non-trivial aspects of deep linear networks(Baldi and Hornik,1994,Section B).In general,however,finding an NN that precisely models a given training set is an NP-complete problem(Judd,1990;Blum and Rivest,1992),also in the case of deep NNs(S´ıma,1994;de Souto et al.,1999;Windisch,2005);compare a survey of negative results(S´ıma, 2002,Section1).Above we have focused on SL.In the more general case of RL in unknown environments,pcc(p,q) is also true if x p is an output event and x q any later input event—any action may affect the environment and thus any later perception.(In the real world,the environment may even influence non-input events computed on a physical hardware entangled with the entire universe,but this is ignored here.)It is possible to model and replace such unmodifiable environmental PCCs through a part of the NN that has already learned to predict(through some of its units)input events(including reward signals)from former input events and actions(Sec.6.1).Its weights are frozen,but can help to assign credit to other,still modifiable weights used to compute actions(Sec.6.1).This approach may lead to very deep CAPs though.Some DL research is about automatically rephrasing problems such that their depth is reduced(Sec.4). In particular,sometimes UL is used to make SL problems less deep,e.g.,Sec.5.10.Often Dynamic Programming(Sec.4.1)is used to facilitate certain traditional RL problems,e.g.,Sec.6.2.Sec.5focuses on CAPs for SL,Sec.6on the more complex case of RL.4Recurring Themes of Deep Learning4.1Dynamic Programming(DP)for DLOne recurring theme of DL is Dynamic Programming(DP)(Bellman,1957),which can help to facili-tate credit assignment under certain assumptions.For example,in SL NNs,backpropagation itself can 1An alternative would be to count only modifiable links when measuring depth.In many typical NN applications this would not make a difference,but in some it would,e.g.,Sec.6.1.be viewed as a DP-derived method(Sec.5.5).In traditional RL based on strong Markovian assumptions, DP-derived methods can help to greatly reduce problem depth(Sec.6.2).DP algorithms are also essen-tial for systems that combine concepts of NNs and graphical models,such as Hidden Markov Models (HMMs)(Stratonovich,1960;Baum and Petrie,1966)and Expectation Maximization(EM)(Dempster et al.,1977),e.g.,(Bottou,1991;Bengio,1991;Bourlard and Morgan,1994;Baldi and Chauvin,1996; Jordan and Sejnowski,2001;Bishop,2006;Poon and Domingos,2011;Dahl et al.,2012;Hinton et al., 2012a).4.2Unsupervised Learning(UL)Facilitating Supervised Learning(SL)and RL Another recurring theme is how UL can facilitate both SL(Sec.5)and RL(Sec.6).UL(Sec.5.6.4) is normally used to encode raw incoming data such as video or speech streams in a form that is more convenient for subsequent goal-directed learning.In particular,codes that describe the original data in a less redundant or more compact way can be fed into SL(Sec.5.10,5.15)or RL machines(Sec.6.4),whose search spaces may thus become smaller(and whose CAPs shallower)than those necessary for dealing with the raw data.UL is closely connected to the topics of regularization and compression(Sec.4.3,5.6.3). 4.3Occam’s Razor:Compression and Minimum Description Length(MDL) Occam’s razor favors simple solutions over complex ones.Given some programming language,the prin-ciple of Minimum Description Length(MDL)can be used to measure the complexity of a solution candi-date by the length of the shortest program that computes it(e.g.,Solomonoff,1964;Kolmogorov,1965b; Chaitin,1966;Wallace and Boulton,1968;Levin,1973a;Rissanen,1986;Blumer et al.,1987;Li and Vit´a nyi,1997;Gr¨u nwald et al.,2005).Some methods explicitly take into account program runtime(Al-lender,1992;Watanabe,1992;Schmidhuber,2002,1995);many consider only programs with constant runtime,written in non-universal programming languages(e.g.,Rissanen,1986;Hinton and van Camp, 1993).In the NN case,the MDL principle suggests that low NN weight complexity corresponds to high NN probability in the Bayesian view(e.g.,MacKay,1992;Buntine and Weigend,1991;De Freitas,2003), and to high generalization performance(e.g.,Baum and Haussler,1989),without overfitting the training data.Many methods have been proposed for regularizing NNs,that is,searching for solution-computing, low-complexity SL NNs(Sec.5.6.3)and RL NNs(Sec.6.7).This is closely related to certain UL methods (Sec.4.2,5.6.4).4.4Learning Hierarchical Representations Through Deep SL,UL,RLMany methods of Good Old-Fashioned Artificial Intelligence(GOFAI)(Nilsson,1980)as well as more recent approaches to AI(Russell et al.,1995)and Machine Learning(Mitchell,1997)learn hierarchies of more and more abstract data representations.For example,certain methods of syntactic pattern recog-nition(Fu,1977)such as grammar induction discover hierarchies of formal rules to model observations. The partially(un)supervised Automated Mathematician/EURISKO(Lenat,1983;Lenat and Brown,1984) continually learns concepts by combining previously learnt concepts.Such hierarchical representation learning(Ring,1994;Bengio et al.,2013;Deng and Yu,2014)is also a recurring theme of DL NNs for SL (Sec.5),UL-aided SL(Sec.5.7,5.10,5.15),and hierarchical RL(Sec.6.5).Often,abstract hierarchical representations are natural by-products of data compression(Sec.4.3),e.g.,Sec.5.10.4.5Fast Graphics Processing Units(GPUs)for DL in NNsWhile the previous millennium saw several attempts at creating fast NN-specific hardware(e.g.,Jackel et al.,1990;Faggin,1992;Ramacher et al.,1993;Widrow et al.,1994;Heemskerk,1995;Korkin et al., 1997;Urlbe,1999),and at exploiting standard hardware(e.g.,Anguita et al.,1994;Muller et al.,1995; Anguita and Gomes,1996),the new millennium brought a DL breakthrough in form of cheap,multi-processor graphics cards or GPUs.GPUs are widely used for video games,a huge and competitive market that has driven down hardware prices.GPUs excel at fast matrix and vector multiplications required not only for convincing virtual realities but also for NN training,where they can speed up learning by a factorof50and more.Some of the GPU-based FNN implementations(Sec.5.16-5.19)have greatly contributed to recent successes in contests for pattern recognition(Sec.5.19-5.22),image segmentation(Sec.5.21), and object detection(Sec.5.21-5.22).5Supervised NNs,Some Helped by Unsupervised NNsThe main focus of current practical applications is on Supervised Learning(SL),which has dominated re-cent pattern recognition contests(Sec.5.17-5.22).Several methods,however,use additional Unsupervised Learning(UL)to facilitate SL(Sec.5.7,5.10,5.15).It does make sense to treat SL and UL in the same section:often gradient-based methods,such as BP(Sec.5.5.1),are used to optimize objective functions of both UL and SL,and the boundary between SL and UL may blur,for example,when it comes to time series prediction and sequence classification,e.g.,Sec.5.10,5.12.A historical timeline format will help to arrange subsections on important inspirations and techni-cal contributions(although such a subsection may span a time interval of many years).Sec.5.1briefly mentions early,shallow NN models since the1940s,Sec.5.2additional early neurobiological inspiration relevant for modern Deep Learning(DL).Sec.5.3is about GMDH networks(since1965),perhaps thefirst (feedforward)DL systems.Sec.5.4is about the relatively deep Neocognitron NN(1979)which is similar to certain modern deep FNN architectures,as it combines convolutional NNs(CNNs),weight pattern repli-cation,and winner-take-all(WTA)mechanisms.Sec.5.5uses the notation of Sec.2to compactly describe a central algorithm of DL,namely,backpropagation(BP)for supervised weight-sharing FNNs and RNNs. It also summarizes the history of BP1960-1981and beyond.Sec.5.6describes problems encountered in the late1980s with BP for deep NNs,and mentions several ideas from the previous millennium to overcome them.Sec.5.7discusses afirst hierarchical stack of coupled UL-based Autoencoders(AEs)—this concept resurfaced in the new millennium(Sec.5.15).Sec.5.8is about applying BP to CNNs,which is important for today’s DL applications.Sec.5.9explains BP’s Fundamental DL Problem(of vanishing/exploding gradients)discovered in1991.Sec.5.10explains how a deep RNN stack of1991(the History Compressor) pre-trained by UL helped to solve previously unlearnable DL benchmarks requiring Credit Assignment Paths(CAPs,Sec.3)of depth1000and more.Sec.5.11discusses a particular WTA method called Max-Pooling(MP)important in today’s DL FNNs.Sec.5.12mentions afirst important contest won by SL NNs in1994.Sec.5.13describes a purely supervised DL RNN(Long Short-Term Memory,LSTM)for problems of depth1000and more.Sec.5.14mentions an early contest of2003won by an ensemble of shallow NNs, as well as good pattern recognition results with CNNs and LSTM RNNs(2003).Sec.5.15is mostly about Deep Belief Networks(DBNs,2006)and related stacks of Autoencoders(AEs,Sec.5.7)pre-trained by UL to facilitate BP-based SL.Sec.5.16mentions thefirst BP-trained MPCNNs(2007)and GPU-CNNs(2006). Sec.5.17-5.22focus on official competitions with secret test sets won by(mostly purely supervised)DL NNs since2009,in sequence recognition,image classification,image segmentation,and object detection. Many RNN results depended on LSTM(Sec.5.13);many FNN results depended on GPU-based FNN code developed since2004(Sec.5.16,5.17,5.18,5.19),in particular,GPU-MPCNNs(Sec.5.19).5.11940s and EarlierNN research started in the1940s(e.g.,McCulloch and Pitts,1943;Hebb,1949);compare also later work on learning NNs(Rosenblatt,1958,1962;Widrow and Hoff,1962;Grossberg,1969;Kohonen,1972; von der Malsburg,1973;Narendra and Thathatchar,1974;Willshaw and von der Malsburg,1976;Palm, 1980;Hopfield,1982).In a sense NNs have been around even longer,since early supervised NNs were essentially variants of linear regression methods going back at least to the early1800s(e.g.,Legendre, 1805;Gauss,1809,1821).Early NNs had a maximal CAP depth of1(Sec.3).5.2Around1960:More Neurobiological Inspiration for DLSimple cells and complex cells were found in the cat’s visual cortex(e.g.,Hubel and Wiesel,1962;Wiesel and Hubel,1959).These cellsfire in response to certain properties of visual sensory inputs,such as theorientation of plex cells exhibit more spatial invariance than simple cells.This inspired later deep NN architectures(Sec.5.4)used in certain modern award-winning Deep Learners(Sec.5.19-5.22).5.31965:Deep Networks Based on the Group Method of Data Handling(GMDH) Networks trained by the Group Method of Data Handling(GMDH)(Ivakhnenko and Lapa,1965; Ivakhnenko et al.,1967;Ivakhnenko,1968,1971)were perhaps thefirst DL systems of the Feedforward Multilayer Perceptron type.The units of GMDH nets may have polynomial activation functions imple-menting Kolmogorov-Gabor polynomials(more general than traditional NN activation functions).Given a training set,layers are incrementally grown and trained by regression analysis,then pruned with the help of a separate validation set(using today’s terminology),where Decision Regularisation is used to weed out superfluous units.The numbers of layers and units per layer can be learned in problem-dependent fashion. This is a good example of hierarchical representation learning(Sec.4.4).There have been numerous ap-plications of GMDH-style networks,e.g.(Ikeda et al.,1976;Farlow,1984;Madala and Ivakhnenko,1994; Ivakhnenko,1995;Kondo,1998;Kord´ık et al.,2003;Witczak et al.,2006;Kondo and Ueno,2008).5.41979:Convolution+Weight Replication+Winner-Take-All(WTA)Apart from deep GMDH networks(Sec.5.3),the Neocognitron(Fukushima,1979,1980,2013a)was per-haps thefirst artificial NN that deserved the attribute deep,and thefirst to incorporate the neurophysiolog-ical insights of Sec.5.2.It introduced convolutional NNs(today often called CNNs or convnets),where the(typically rectangular)receptivefield of a convolutional unit with given weight vector is shifted step by step across a2-dimensional array of input values,such as the pixels of an image.The resulting2D array of subsequent activation events of this unit can then provide inputs to higher-level units,and so on.Due to massive weight replication(Sec.2),relatively few parameters may be necessary to describe the behavior of such a convolutional layer.Competition layers have WTA subsets whose maximally active units are the only ones to adopt non-zero activation values.They essentially“down-sample”the competition layer’s input.This helps to create units whose responses are insensitive to small image shifts(compare Sec.5.2).The Neocognitron is very similar to the architecture of modern,contest-winning,purely super-vised,feedforward,gradient-based Deep Learners with alternating convolutional and competition lay-ers(e.g.,Sec.5.19-5.22).Fukushima,however,did not set the weights by supervised backpropagation (Sec.5.5,5.8),but by local un supervised learning rules(e.g.,Fukushima,2013b),or by pre-wiring.In that sense he did not care for the DL problem(Sec.5.9),although his architecture was comparatively deep indeed.He also used Spatial Averaging(Fukushima,1980,2011)instead of Max-Pooling(MP,Sec.5.11), currently a particularly convenient and popular WTA mechanism.Today’s CNN-based DL machines profita lot from later CNN work(e.g.,LeCun et al.,1989;Ranzato et al.,2007)(Sec.5.8,5.16,5.19).5.51960-1981and Beyond:Development of Backpropagation(BP)for NNsThe minimisation of errors through gradient descent(Hadamard,1908)in the parameter space of com-plex,nonlinear,differentiable,multi-stage,NN-related systems has been discussed at least since the early 1960s(e.g.,Kelley,1960;Bryson,1961;Bryson and Denham,1961;Pontryagin et al.,1961;Dreyfus,1962; Wilkinson,1965;Amari,1967;Bryson and Ho,1969;Director and Rohrer,1969;Griewank,2012),ini-tially within the framework of Euler-LaGrange equations in the Calculus of Variations(e.g.,Euler,1744). Steepest descent in such systems can be performed(Bryson,1961;Kelley,1960;Bryson and Ho,1969)by iterating the ancient chain rule(Leibniz,1676;L’Hˆo pital,1696)in Dynamic Programming(DP)style(Bell-man,1957).A simplified derivation of the method uses the chain rule only(Dreyfus,1962).The methods of the1960s were already efficient in the DP sense.However,they backpropagated derivative information through standard Jacobian matrix calculations from one“layer”to the previous one, explicitly addressing neither direct links across several layers nor potential additional efficiency gains due to network sparsity(but perhaps such enhancements seemed obvious to the authors).。
The_Bullwhip_Effect_in_Supply_Chains

The Bullwhip Effect In Supply Chains1Hau L Lee, V Padmanabhan, and Seungjin Whang;Sloan Management Review, Spring 1997, Volume 38, Issue 3, pp. 93-102 Abstract:The bullwhip effect occurs when the demand order variabilities in the supply chain are amplified as they moved up the supply chain. Distorted information from one end of a supply chain to the other can lead to tremendous inefficiencies. Companies can effectively counteract the bullwhip effect by thoroughly understanding its underlying causes. Industry leaders are implementing innovative strategies that pose new challenges: 1. integrating new information systems, 2. defining new organizational relationships, and 3. implementing new incentive and measurement systems.Distorted information from one end of a supply chain to the other can lead to tremendousinefficiencies: excessive inventory investment, poor customer service, lost revenues, misguided capacity plans, inactive transportation, and missed production schedules. How do exaggeratedorder swings occur? What can companies do to mitigate them?Not long ago, logistics executives at Procter & Gamble (P&G) examined the order patterns for one of their best-selling products, Pampers. Its sales at retail stores were fluctuating, but the variabilities were certainly not excessive. However, as they examined the distributors' orders, the executives were surprised by the degree of variability. When they looked at P&G's orders of materials to their suppliers, such as 3M, they discovered that the swings were even greater. At first glance, the variabilities did not make sense. While the consumers, in this case, the babies, consumed diapers at a steady rate, the demand order variabilities in the supply chain were amplified as they moved up the supply chain. P&G called this phenomenon the "bullwhip" effect. (In some industries, it is known as the "whiplash" or the "whipsaw" effect.)When Hewlett-Packard (HP) executives examined the sales of one of its printers at a major reseller, they found that there were, as expected, some fluctuations over time. However, when they examined the orders from the reseller, they observed much bigger swings. Also, to their surprise, they discovered that the orders from the printer division to the company's integrated circuit division had even greater fluctuations.What happens when a supply chain is plagued with a bullwhip effect that distorts its demand information as it is transmitted up the chain? In the past, without being able to see the sales of its products at the distribution channel stage, HP had to rely on the sales orders from the resellers to make product forecasts, plan capacity, control inventory, and schedule production. Big variations in demand were a major problem for HP's management. The common symptoms of such variations could be excessive inventory, poor product forecasts, insufficient or excessive capacities, poor customer service due to unavailable products or long backlogs, uncertain production planning (i.e., excessive revisions), and high costs for corrections, such as for expedited shipments and overtime. HP's product division was a victim of order swings that were exaggerated by the resellers relative to their sales; it, in turn, created additional exaggerations of order swings to suppliers.In the past few years, the Efficient Consumer Response (ECR) initiative has tried to redefine how the grocery supply chain should work.[1] One motivation for the initiative was the excessive amount of inventory in the supply chain. Various industry studies found that the total supply chain, from when1 Copyright Sloan Management Review Association, Alfred P. Sloan School of Management Spring 1997products leave the manufacturers' production lines to when they arrive on the retailers' shelves, has more than 100 days of inventory supply. Distorted information has led every entity in the supply chain - the plant warehouse, a manufacturer's shuttle warehouse, a manufacturer's market warehouse, a distributor's central warehouse, the distributor's regional warehouses, and the retail store's storage space - to stockpile because of the high degree of demand uncertainties and variabilities. It's no wonder that the ECR reports estimated a potential $30 billion opportunity from streamlining the inefficiencies of the grocery supply chain.[2]Figure 1 Increasing Variability of Orders up the Supply ChainOther industries are in a similar position. Computer factories and manufacturers' distribution centers, the distributors' warehouses, and store warehouses along the distribution channel have inventory stockpiles. And in the pharmaceutical industry, there are duplicated inventories in a supply chain of manufacturers such as Eli Lilly or Bristol-Myers Squibb, distributors such as McKesson, and retailers such as Longs Drug Stores. Again, information distortion can cause the total inventory in this supply chain to exceed 100 days of supply. With inventories of raw materials, such as integrated circuits and printed circuit boards in the computer industry and antibodies and vial manufacturing in the pharmaceutical industry, the total chain may contain more than one year's supply.In a supply chain for a typical consumer product, even when consumer sales do not seem to vary much, there is pronounced variability in the retailers' orders to the wholesalers (see Figure 1). Orders to the manufacturer and to the manufacturers' supplier spike even more. To solve the problem of distorted information, companies need to first understand what creates the bullwhip effect so they can counteract it. Innovative companies in different industries have found that they can control the bullwhip effect and improve their supply chain performance by coordinating information and planning along the supply chain.Causes of the Bullwhip EffectPerhaps the best illustration of the bullwhip effect is the well-known "beer game."[3] In the game, participants (students, managers, analysts, and so on) play the roles of customers, retailers, wholesalers, and suppliers of a popular brand of beer. The participants cannot communicate with each other and must make order decisions based only on orders from the next downstream player. The ordering patterns share a common, recurring theme: the variabilities of an upstream site are always greater than those of the downstream site, a simple, yet powerful illustration of the bullwhip effect. This amplified order variability may be attributed to the players' irrational decision making. Indeed, Sterman's experiments showed that human behavior, such as misconceptions about inventory and demand information, may cause the bullwhip effect.[4]In contrast, we show that the bullwhip effect is a consequence of the players' rational behavior within the supply chain's infrastructure. This important distinction implies that companies wanting to control the bullwhip effect have to focus on modifying the chain's infrastructure and related processes rather than the decision makers' behavior.We have identified four major causes of the bullwhip effect:1. Demand forecast updating2. Order batching3. Price fluctuation4. Rationing and shortage gamingEach of the four forces in concert with the chain's infrastructure and the order managers' rational decision making create the bullwhip effect. Understanding the causes helps managers design and develop strategies to counter it.[5]Demand Forecast UpdatingEvery company in a supply chain usually does product forecasting for its production scheduling, capacity planning, inventory control, and material requirements planning. Forecasting is often based on the order history from the company's immediate customers. The outcomes of the beer game are the consequence of many behavioral factors, such as the players' perceptions and mistrust. An important factor is each player's thought process in projecting the demand pattern based on what he or she observes. When a downstream operation places an order, the upstream manager processes that piece of information as a signal about future product demand. Based on this signal, the upstream manager readjusts his or her demand forecasts and, in turn, the orders placed with the suppliers of the upstream operation. We contend that demand signal processing is a major contributor to the bullwhip effect.For example, if you are a manager who has to determine how much to order from a supplier, you use a simple method to do demand forecasting, such as exponential smoothing. With exponential smoothing, future demands are continuously updated as the new daily demand data become available. The order you send to the supplier reflects the amount you need to replenish the stocks to meet the requirements of future demands, as well as the necessary safety stocks. The future demands and the associated safety stocks are updated using the smoothing technique. With long lead times, it is not uncommon to have weeks of safety stocks. The result is that the fluctuations in the order quantities over time can be much greater than those in the demand data.Now, one site up the supply chain, if you are the manager of the supplier, the daily orders from the manager of the previous site constitute your demand. If you are also using exponential smoothing to update your forecasts and safety stocks, the orders that you place with your supplier will have even bigger swings. For an example of such fluctuations in demand, see Figure 2. As we can see from the figure, the orders placed by the dealer to the manufacturer have much greater variability than theconsumer demands. Because the amount of safety stock contributes to the bullwhip effect, it is intuitive that, when the lead times between the resupply of the items along the supply chain are longer, the fluctuation is even more significant.Order BatchingIn a supply chain, each company places orders with an upstream organization using some inventory monitoring or control. Demands come in, depleting inventory, but the company may not immediately place an order with its supplier. It often batches or accumulates demands before issuing an order. There are two forms of order batching: periodic ordering and push ordering.Figure 2 Higher Variability in Orders from Dealer to Manufacturer than Actual SalesInstead of ordering frequently, companies may order weekly, biweekly, or even monthly. There are many common reasons for an inventory system based on order cycles. Often the supplier cannot handle frequent order processing because the time and cost of processing an order can be substantial. P&G estimated that, because of the many manual interventions needed in its order, billing, and shipment systems, each invoice to its customers cost between $35 and $75 to process.' Many manufacturers place purchase orders with suppliers when they run their material requirements planning (MRP) systems. MRP systems are often run monthly, resulting in monthly ordering with suppliers. A company with slow-moving items may prefer to order on a regular cyclical basis because there may not be enough items consumed to warrant resupply if it orders more frequently.Consider a company that orders once a month from its supplier. The supplier faces a highly erratic stream of orders. There is a spike in demand at one time during the month, followed by no demands for the rest of the month. Of course, this variability is higher than the demands the company itself faces. Periodic ordering amplifies variability and contributes to the bullwhip effect.One common obstacle for a company that wants to order frequently is the economics of transportation. There are substantial differences between full truckload (FTL) and less-than-truckload rates, so companies have a strong incentive to fill a truckload when they order materials from a supplier. Sometimes, suppliers give their best pricing for FTL orders. For most items, a full truckload could be a supply of a month or more. Full or close to full truckload ordering would thus lead to moderate to excessively long order cycles.In push ordering, a company experiences regular surges in demand. The company has orders "pushed" on it from customers periodically because salespeople are regularly measured, sometimes quarterly or annually, which causes end-of-quarter or end-of-year order surges. Salespersons who need to fill sales quotas may "borrow" ahead and sign orders prematurely. The U.S. Navy's study of recruiter productivity found surges in the number of recruits by the recruiters on a periodic cycle that coincided with their evaluation cycle.[7] For companies, the ordering pattern from their customers is more erratic than the consumption patterns that their customers experience. The "hockey stick" phenomenon is quite prevalent. When a company faces periodic ordering by its customers, the bullwhip effect results. If all customers' order cycles were spread out evenly throughout the week, the bullwhip effect would be minimal. The periodic surges in demand by some customers would be insignificant because not all would be orderingat the same time. Unfortunately, such an ideal situation rarely exists. Orders are more likely to be randomly spread out or, worse, to overlap. When order cycles overlap, most customers that order periodically do so at the same time. As a result, the surge in demand is even more pronounced, and the variability from the bullwhip effect is at its highest.If the majority of companies that do MRP or distribution requirement planning (DRP) to generate purchase orders do so at the beginning of the month (or end of the month), order cycles overlap. Periodic execution of MRPs contributes to the bullwhip effect, or "MRP jitters" or "DRP jitters."Price FluctuationEstimates indicate that 80 percent of the transactions between manufacturers and distributors in the grocery industry were made in a "forward buy" arrangement in which items were bought in advance of requirements, usually because of a manufacturer's attractive price offer.[8] Forward buying constitutes $75 billion to $100 billion of inventory in the grocery industry.Forward buying results from price fluctuations in the marketplace. Manufacturers and distributors periodically have special promotions like price discounts, quantity discounts, coupons, rebates, and so on. All these promotions result in price fluctuations. Additionally, manufacturers offer trade deals (e.g., special discounts, price terms, and payment terms) to the distributors and wholesalers, which are an indirect form of price discounts. For example, Kotler reports that trade deals and consumer promotion constitute 47 percent and 28 percent, respectively, of their total promotion budgets.[10] The result is that customers buy in quantities that do not reflect their immediate needs; they buy in bigger quantities and stock up for the future.Such promotions can be costly to the supply chain.[11] What happens if forward buying becomes the norm? When a product's price is low (through direct discount or promotional schemes), a customer buys in bigger quantities than needed. When the product's price returns to normal, the customer stops buying until it has depleted its inventory As a result, the customer's buying pattern does not reflect its consumption pattern, and the variation of the buying quantities is much bigger than the variation of the consumption rate - the bullwhip effect.When high-low pricing occurs, forward buying may well be a rational decision. If the cost of holding inventory is less than the price differential, buying in advance makes sense. In fact, the high-low pricing phenomenon has induced a stream of research on how companies should order optimally to take advantage of the low price opportunities.Although some companies claim to thrive on high-low buying practices, most suffer. For example, a soup manufacturer's leading brand has seasonal sales, with higher sales in the winter (see Figure 3). However, the shipment quantities from the manufacturer to the distributors, reflecting orders from the distributors to the manufacturer, varied more widely. When faced with such wide swings, companies often have to run their factories overtime at certain times and be idle at others. Alternatively, companies may have to build huge piles of inventory to anticipate big swings in demand. With a surge in shipments, they may also have to pay premium freight rates to transport products. Damage also increases from handling larger than normal volumes and stocking inventories for long periods. The irony is that these variations are induced by price fluctuations that the manufacturers and the distributors set up themselves. It's no wonder that such a practice was called "the dumbest marketing ploy ever."[12]Figure 3 Bullwhip Effect due to Seasonal Sales of SoupUsing trade promotions can backfire because of the impact on the manufacturers' stock performance. A group of shareholders sued Bristol-Myers Squibb when its stock plummeted from $74 to $67 as a result of a disappointing quarterly sales performance; its actual sales increase was only 5 percent instead of the anticipated 13 percent. The sluggish sales increase was reportedly due to the company's trade deals in a previous quarter that flooded the distribution channel with forward-buy inventories of its product.[13]Rationing and Shortage GamingWhen product demand exceeds supply, a manufacturer often rations its product to customers. In one scheme, the manufacturer allocates the amount in proportion to the amount ordered. For example, if the total supply is only 50 percent of the total demand, all customers receive 50 percent of what they order. Knowing that the manufacturer will ration when the product is in short supply, customers exaggerate their real needs when they order. Later, when demand cools, orders will suddenly disappear and cancellations pour in. This seeming overreaction by customers anticipating shortages results when organizations and individuals make sound, rational economic decisions and "game" the potential rationing.[14] The effect of"gaming" is that customers' orders give the supplier little information on the product's real demand, a particularly vexing problem for manufacturers in a products early stages. The gaming practice is very common. In the 1980s, on several occasions, the computer industry perceived a shortage of DRAM chips. Orders shot up, not because of an increase in consumption, but because of anticipation. Customers place duplicate orders with multiple suppliers and buy from the first one that can deliver, then cancel all other duplicate orders.[15]More recently, Hewlett-Packard could not meet the demand for its LaserJet III printer and rationed the product. Orders surged, but HP managers could not discern whether the orders genuinely reflected real market demands or were simply phantom orders from resellers trying to get better allocation of the product. When HP lifted its constraints on resupply of the LaserJets, many resellers canceled their orders. HP's costs in excess inventory after the allocation period and in unnecessary capacity increases were in the millions of dollars.[16]During the Christmas shopping seasons in 1992 and 1993, Motorola could not meet consumer demand for handsets and cellular phones, forcing many distributors to turn away business. Distributors like AirTouch Communications and the Baby Bells, anticipating the possibility of shortages and acting defensively, drastically over ordered toward the end of 1994.[17] Because of such overzealous ordering by retail distributors, Motorola reported record fourth-quarter earnings in January 1995. Once Wall Street realized that the dealers were swamped with inventory and new orders for phones were not as healthy before, Motorola's stock tumbled almost 10 percent.In October 1994, IBM's new Aptiva personal computer was selling extremely well, leading resellers to speculate that IBM might run out of the product before the Christmas season. According to some analysts, IBM, hampered by an overstock problem the previous year, planned production too conservatively. Other analysts referred to the possibility of rationing: "Retailers - apparently convinced Aptiva will sell well and afraid of being left with insufficient stock to meet holiday season demand -- increased their orders with IBM, believing they wouldn't get all they asked for."" It was unclear to IBM how much of the increase in orders was genuine market demand and how much was due to resellers placing phantom orders when IBM had to ration the product.How to Counteract the Bullwhip EffectUnderstanding the causes of the bullwhip effect can help managers find strategies to mitigate it. Indeed, many companies have begun to implement innovative programs that partially address the effect. Next we examine how companies tackle each of the four causes. We categorize the various initiatives and other possible remedies based on the underlying coordination mechanism, namely, information sharing, channel alignment, and operational efficiency. With information sharing, demand information at a downstream site is transmitted upstream in a timely fashion. Channel alignment is the coordination of pricing, transportation, inventory planning, and ownership between the upstream and downstream sites in a supply chain. Operational efficiency refers to activities that improve performance, such as reduced costs and lead-time. We use this topology to discuss ways to control the bullwhip effect (see Table 1). Avoid Multiple Demand Forecast UpdatesOrdinarily, every member of a supply chain conducts some sort of forecasting in connection with its planning (e.g., the manufacturer does the production planning, the wholesaler, the logistics planning, and so on). Bullwhip effects are created when supply chain members process the demand input from their immediate downstream member in producing their own forecasts. Demand input from the immediate downstream member, of course, results from that member's forecasting, with input from its own downstream member.One remedy to the repetitive processing of consumption data in a supply chain is to make demand data at a downstream site available to the upstream site. Hence, both sites can update their forecasts with thesame raw data In the computer industry, manufacturers request sell-through data on withdrawn stocks from their resellers' central warehouse. Although the data are not as complete as point-of-sale (POS) data from the resellers' stores, they offer significantly more information than was available when manufacturers didn't know what happened after they shipped their products. IBM, HP, and Apple all require sell-through data as part of their contract with resellers.Supply chain partners can use electronic data interchange (EDI) to share data. In the consumer products industry, 20 percent of orders by retailers of consumer products was transmitted via EDI in 1990.[1] In 1992, that figure was close to 40 percent and, in 1995, nearly 60 percent. The increasing use of EDI will undoubtedly facilitate information transmission and sharing among chain members. Even if the multiple organizations in a supply chain use the same source demand data to perform forecast updates, the differences in forecasting methods and buying practices can still lead to unnecessary fluctuations in the order data placed with the upstream site. In a more radical approach, the upstream site could control resupply from upstream to downstream. The upstream site would have access to the demand and inventory information at the downstream site and update the necessary forecasts and resupply for the downstream site. The downstream site, in turn, would become a passive partner in the supply chain. For example, in the consumer products industry, this practice is known as vendor-managed inventory (VMI) or a continuous replenishment program (CRP). Many companies such as Campbell Soup, M&M/Mars, Nestle, Quaker Oats, Nabisco, P&G, and Scott Paper use CRP with some or most of their customers. Inventory reductions of up to 25 percent are common in these alliances. P&G uses VMI in its diaper supply chain, starting with its supplier, 3M, and its customer, Wal-Mart. Even in the high-technology sector, companies such as Texas Instruments, HP Motorola, and Apple use VMI with some of their suppliers and, in some cases, with their customers.Inventory researchers have long recognized that multi-echelon inventory systems can operate better when inventory and demand information from downstream sites is available upstream. Echelon inventory - the total inventory at its upstream and downstream sites - is key to optimal inventory control."Another approach is to try to get demand information about the downstream site by bypassing it. Apple Computer has a "consumer direct" program, i.e., it sells directly to consumers without going through the reseller and distribution channel. A benefit of the program is that it allows Apple to see the demand patterns for its products. Dell Computers also sells its products directly to consumers without going through the distribution channel.Finally, as we noted before, long resupply lead times can aggravate the bullwhip effect. Improvements in operational efficiency can help reduce the highly variable demand due to multiple forecast updates. Hence, just-in-time replenishment is an effective way to mitigate the effect.Break Order BatchesSince order batching contributes to the bullwhip effect, companies need to devise strategies that lead to smaller batches or more frequent resupply. In addition, the counterstrategies we described earlier are useful. When an upstream company receives consumption data on a fixed, periodic schedule from its downstream customers, it will not be surprised by an unusually large batched order when there is a demand surge.One reason that order batches are large or order frequencies low is the relatively high cost of placing an order and replenishing it. EDI can reduce the cost of the paperwork in generating an order. Using EDI, companies such as Nabisco perform paperless, computer-assisted ordering (CAO), and, consequently, customers order more frequently. McKesson's Economost ordering system uses EDI to lower the transaction costs from orders by drugstores and other retailers." P&G has introduced standardized ordering terms across all business units to simplify the process and dramatically cut the number of invoices.[22] And General Electric is electronically matching buyers and suppliers throughout the company.It expects to purchase at least $1 billion in materials through its internally developed Trading Process Network. A paper purchase order that typically cost $50 to process is now $5.23Table 1 A Framework for Supply Chain Coordination InitiativesAnother reason for large order batches is the cost of transportation. The differences in the costs of full truckloads and less-than-truckloads are so great that companies find it economical to order full truckloads, even though this leads to infrequent replenishments from the supplier. In fact, even if orders are made with little effort and low cost through EDI, the improvements in order efficiency are wasted due to the full truckload constraint. Now some manufacturers induce their distributors to order assortments of different products. Hence a truckload may contain different products from the same manufacturer (either a plant warehouse site or a manufacturer's market warehouse) instead of a full load of the same product.。
费尔贝恩的人格客体关系理论研究
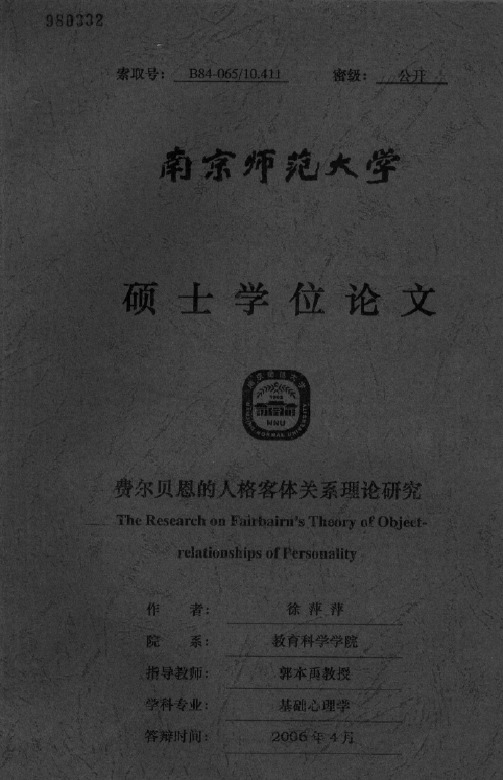
39.郭本禹当代心理学新进展 2003
40.叶浩生西方心理学的历史与体系 1998
41.弗洛伊德.严志军.张沫文明及其不满 2003
42.L·A·柏文.周榕人格科学 2001
43.B·R·赫根汉.郭本禹心理学史导论 2004
44.李小龙回忆费尔贝恩 2004
45.缪绍疆客体关系基本文献序言 2005
States:Introduction to a Newer Psychoanalytic Metapsychology 1980
16.Grotstein J S Newer Perspectives in Object Relations Theory 1982
17.Grotstein J S A Reappraisal ofw.R D.Fairbairn's 1993(04)
33.Sutherland J D Fairbairn's Journey into the Interior 1989
34.Michael St Clair.贾晓明.苏晓波现代精神分析"圣经
36.蔡飞精神的新范式--自身心理学 2001
37.车文博弗洛伊德主义论评 1992
1.期刊论文徐萍萍.XU Ping-ping自我·客体关系·人格——费尔贝恩的纯粹心理的人格发展观-南京师大学报
(社会科学版)2006(5)
精神分析的客体关系理论家费尔贝恩在修正与发展弗洛伊德和克莱因思想的基础上,结合自己的临床经验,从客体关系角度出发,阐述了一种纯粹心理的人格发展理论.该理论指出,人格发展的实质是自我客体关系的成熟;母婴关系是影响人格发展的首要因素.费尔贝恩还综合了弗洛伊德的阶段理论与克莱因的心态概念,构想了一个全新的人格发展图式.费尔贝恩的人格发展理论以其革命性和独创性在精神分析学、人格心理学、发展心理学领域都占据了一席之地.
初二英语阅读理解文学常识题单选题40题

初二英语阅读理解文学常识题单选题40题1. Which of the following is a novel written by Charles Dickens?A. Pride and PrejudiceB. Oliver TwistC. Wuthering HeightsD. Jane Eyre答案:B。
解析:Charles Dickens 是英国著名作家,其代表作品有《Oliver Twist》。
选项A《Pride and Prejudice》的作者是Jane Austen;选项C Wuthering Heights》的作者是Emily Bronte;选项D Jane Eyre》的作者是Charlotte Bronte。
2. Who wrote Romeo and Juliet?A. William ShakespeareB. Geoffrey ChaucerC. Thomas HardyD. George Eliot答案:A。
解析:Romeo and Juliet》是William Shakespeare 的作品。
Geoffrey Chaucer 的代表作是《The Canterbury Tales》;Thomas Hardy 的作品有《Tess of the d'Urbervilles》等;George Eliot 的作品有Middlemarch》。
3. The famous novel David Copperfield was written by _____.A. Mark TwainB. Leo TolstoyC. Charles DickensD. Herman Melville答案:C。
解析:Charles Dickens 创作了 David Copperfield》。
Mark Twain 是美国作家;Leo Tolstoy 是俄国作家;Herman Melville 也是美国作家。
自由民主与选举民主
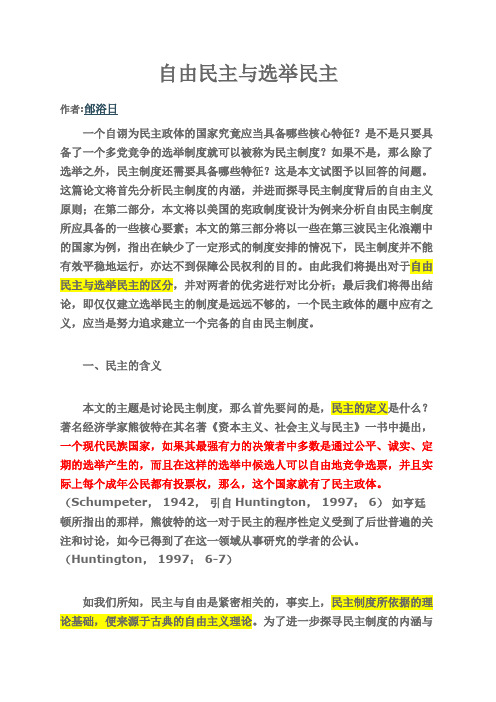
自由民主与选举民主作者:邰浴日一个自诩为民主政体的国家究竟应当具备哪些核心特征?是不是只要具备了一个多党竞争的选举制度就可以被称为民主制度?如果不是,那么除了选举之外,民主制度还需要具备哪些特征?这是本文试图予以回答的问题。
这篇论文将首先分析民主制度的内涵,并进而探寻民主制度背后的自由主义原则;在第二部分,本文将以美国的宪政制度设计为例来分析自由民主制度所应具备的一些核心要素;本文的第三部分将以一些在第三波民主化浪潮中的国家为例,指出在缺少了一定形式的制度安排的情况下,民主制度并不能有效平稳地运行,亦达不到保障公民权利的目的。
由此我们将提出对于自由民主与选举民主的区分,并对两者的优劣进行对比分析;最后我们将得出结论,即仅仅建立选举民主的制度是远远不够的,一个民主政体的题中应有之义,应当是努力追求建立一个完备的自由民主制度。
一、民主的含义本文的主题是讨论民主制度,那么首先要问的是,民主的定义是什么?著名经济学家熊彼特在其名著《资本主义、社会主义与民主》一书中提出,一个现代民族国家,如果其最强有力的决策者中多数是通过公平、诚实、定期的选举产生的,而且在这样的选举中候选人可以自由地竞争选票,并且实际上每个成年公民都有投票权,那么,这个国家就有了民主政体。
(Schumpeter, 1942,引自Huntington, 1997: 6)如亨廷顿所指出的那样,熊彼特的这一对于民主的程序性定义受到了后世普遍的关注和讨论,如今已得到了在这一领域从事研究的学者的公认。
(Huntington, 1997: 6-7)如我们所知,民主与自由是紧密相关的,事实上,民主制度所依据的理论基础,便来源于古典的自由主义理论。
为了进一步探寻民主制度的内涵与基础,我们不得不对古典自由主义理论的基本主张予以适当的关注。
美国著名政治学者高于斯教授在他的著作中指出了古典自由主义的四个主要特征:首先,古典自由主义将宽容视作人类社会的首要美德,赋予其极高的正面价值;其次,古典自由主义将某种特定的人类自由赋予了特殊的重要性;第三,(古典)自由主义者们都普遍信奉个人主义;最后,古典自由主义以一种对于无限制的集中、专断的权力的担忧与警惕为特征,因此,对于这种权力的限制便一直成为自由主义政治的一个主要目标(Geuss, 2005:14)。
岛屿生态地理学理论【岛屿生物地理学理论与生物多样性保护】
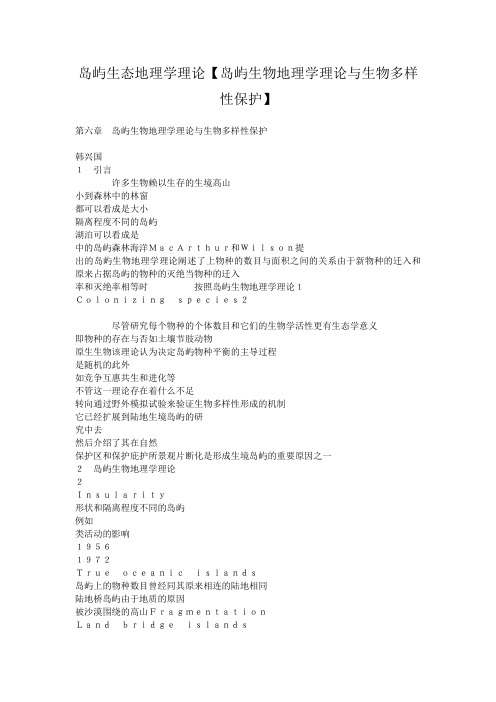
岛屿的面积和
隔离程度都影响鸟类物种的数目
2
当一个物种占据某个
岛屿后
就会在一定程度上由该物种的选择方向时期
建立种效应
Founder principle
简单他说是指一个传播体
建立种的等位基因数量相对较少
遗传变异将逐渐得到恢复环境条件下岛屿同种种群和纯合水平的提高
研究种群大小对后代种群遗传变异的影响
所谓最小动态面积是指能够包含较复杂的生境类型
1975
MarquesasMarianas IslandsPelew Palau Islands
Somoa
Fiji
16
Renell Ialands
D'Entrecasteaux Islands
我们就可得到生态学中的所谓
1913
Arrhenius
1925
Diamond和
Mayr
1962aMcQuinness
将这一关系用
它已经扩展到陆地生境岛屿的研
究中去
然后介绍了其在自然
保护区和保护庇护所景观片断化是形成生境岛屿的重要原因之一
2 岛屿生物地理学理论
2
Insularity
形状和隔离程度不同的岛屿
例如
类活动的影响
1956
1972
True oceanic islands
岛屿上的物种数目曾经同其原来相连的陆地相同
陆地桥岛屿由于地质的原因
那么z值可能增加经过对数转换后IsolateSample而样本则是群落中的部分个体样本的z值要比隔离种群小12171835之间MacArthur和Wilson
面积曲线以及z值的狭窄范围主要是由于下列两方面引起的
其二是个体总数和物种数目之间的关系非常接近对数正态分布Distribution of species abundance
福建省三明市第一中学2023-2024学年高二下学期期中考试英语试题
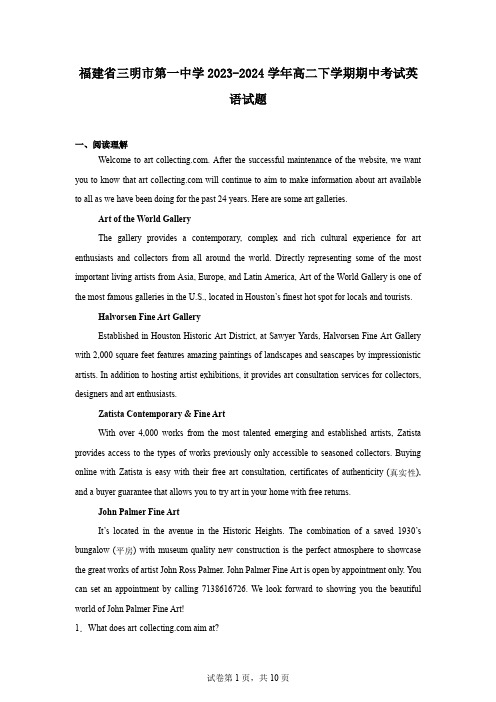
福建省三明市第一中学2023-2024学年高二下学期期中考试英语试题一、阅读理解Welcome to . After the successful maintenance of the website, we want you to know that will continue to aim to make information about art available to all as we have been doing for the past 24 years. Here are some art galleries.Art of the World GalleryThe gallery provides a contemporary, complex and rich cultural experience for art enthusiasts and collectors from all around the world. Directly representing some of the most important living artists from Asia, Europe, and Latin America, Art of the World Gallery is one of the most famous galleries in the U.S., located in Houston’s finest hot spot for locals and tourists.Halvorsen Fine Art GalleryEstablished in Houston Historic Art District, at Sawyer Yards, Halvorsen Fine Art Gallery with 2,000 square feet features amazing paintings of landscapes and seascapes by impressionistic artists. In addition to hosting artist exhibitions, it provides art consultation services for collectors, designers and art enthusiasts.Zatista Contemporary & Fine ArtWith over 4,000 works from the most talented emerging and established artists, Zatista provides access to the types of works previously only accessible to seasoned collectors. Buying online with Zatista is easy with their free art consultation, certificates of authenticity (真实性), and a buyer guarantee that allows you to try art in your home with free returns.John Palmer Fine ArtIt’s located in the avenue in the Historic Heights. The combination of a saved 1930’s bungalow (平房) with museum-quality new construction is the perfect atmosphere to showcase the great works of artist John Ross Palmer. John Palmer Fine Art is open by appointment only. You can set an appointment by calling 7138616726. We look forward to showing you the beautiful world of John Palmer Fine Art!1.What does aim at?A.Collecting artworks.B.Helping talented artists.C.Offering art information.D.Founding art organizations.2.What can visitors do in Halvorsen Fine Art Gallery?A.Hold personal exhibitions.B.Experience diverse cultures.C.Obtain authentic certificates.D.Admire impressionist paintings.3.What makes John Palmer Fine Art different from the other three?A.It is in Houston’s best spot.B.It offers art consultation services.C.It displays only one artist’s works.D.It can be visited without an appointment.Throughout all the events in my life, one in particular sticks out more than the others. As I reflect on this significant event, a smile spreads across my face. As I think of Shanda, I feel loved and grateful.It was my twelfth year of dancing, and I thought it would end up like any other year: stuck in emptiness, forgotten and without the belief of any teacher or friend that I really had the potential to achieve greatness.However, I met Shanda, a talented chorcographer (编舞者). She influenced me to work to the best of my ability, pushed me to keep going when I wanted to quit, encouraged me and showed me the importance of courage. Throughout our hard work, not only did my ability to dance grow, but my friendship with Shanda grew as well.With the end of the year came our show time. As I walked backstage and saw many other dancers, I hoped for a good performance that would prove my improvement. I waited anxiously for my turn. Finally, after what seemed like days, the loudspeaker announced my name. Butterflies filling my stomach, I took trembling steps onto the big lighted stage. But, with the determination to succeed and eagerness to live up to Shanda’s expectations for me, I began to dance. All my troubles and nerves went away as I danced my whole heart out.As I walked up to the judge to receive my first place shining, gold trophy (奖杯), I realized that dance is not about becoming the best. It was about loving dance for dance itself, a getaway from all my problems in the world. Shanda showed me that you could let everything go and just do what you feel at that moment. After all the doubts that people had in me, I believed in myself and did not care what others thought. Thanks to Shanda, dance became more than a love of mine,but a passion.4.What did the author think her dancing would be for the twelfth year?A.A change for the better.B.A disappointment as before.C.A proof of her potential.D.The pride of her teachers and friends. 5.How did Shanda help the author?A.By offering her financial help.B.By entering her in a competition.C.By coaching her for longer hours.D.By awakening her passion for dancing. 6.What do the underlined words in paragraph 4 probably mean?A.Nervous.B.Dynamic.C.Courageous.D.Enthusiastic. 7.What can we learn from the author’s story?A.Success lies in courage.B.Adversity helps one grow up.C.A good teacher matters.D.Reputation comes from hard work.Part of the reason American shoppers are so attracted to wholesale shopping is their belief that it not only prevents waste but can save time and money, providing more value for the dollar. However, recent research suggests that the opposite may be true.Victoria Ligon, an expert on consumer sciences, studied food purchasing habits of consumers and found that people tended to buy too much food and waste more of it than they realized. “The problem is that people are not shopping frequently enough,” Ligon said, “People are very price sensitive at the grocery store, but tend to fail to notice the cost of unused and wasted food at home.”A common practice is to visit different stores for different items on a grocery list, “But people tend to overbuy at each of the places,” Ligon said. “People are not planning for the next day, but planning for the next week or two.”“In theory, planning a week or more in advance sounds ideal. But given the reality of many people’s lives, this is challenging to do well,” Ligon said. “All of our food promotions are designed to get people to buy more. We believe it’s cheaper if we buy more now, but we rarely take into account how much we throw out in the end.”Ligon noted shifts in the grocery industry that appear promising to help customers reduce food waste. Examples include cost-effective delivery services such as Amazon Fresh and GoogleExpress, which allow consumers to purchase food items when they want to consume them, also reducing their need to frequent so many different stores. However, the study resulted in another troubling finding: The majority of people involved in the study had no idea that they were buying too much and wasting so much.“When you read advice about reducing waste, it usually centers on what people do after the food is purchased,” Ligon said. “But more importantly, shop on a more frequent basis, so that you are only buying what you are going to consume in the short term.”8.What do people often ignore when buying food in large quantities?A.How good the food is.B.How much will be wasted.C.How much the food costs.D.How often they should shop.9.What is the author’s attitude towards meal planning for the next two weeks?A.It is worth trying.B.It is not practical.C.It takes great effort.D.It is not good for health.10.What is the advantage of Amazon Fresh and Google Express?A.Food prices are lowered.B.Food waste is prevented.C.Food consumption is reduced.D.Food purchasing can be done at home. 11.What can be the best title for the passage?A.Shop More, Buy Less B.Shop Wisely, Eat WiselyC.Consume More, Waste Less D.The More You Shop, the More You WasteWriters of science fiction often feel more prescient (预知的) than others. Whether it’s the architectural and social dystopias of J.G. Ballard’s novels, or the world of E.M. Forster’s The Machine Stops, the genre is full of prescient writers dealing with ever more familiar issues.Out of all such writers, few seem more likely to predict our times than author Philip K. Dick, who died 42 years ago. In a remarkably 30-year period of work, Dick authored 44 novels and countless short stories, adaptations of which redefined science fiction on screen — in particular Ridley Scott’s Blade Runner, based on Dick’s story Do Androids Dream of Electric Sheep? and Paul Verhoeven’s Total Recall, which took his 1966 short story We Can Remember It for You Wholesale as its source material.Dick had a astonishing ability to predict what would happen in modern world. Celebratedscience-fiction and fantasy author Stan Nicholls suggested Dick’s work was prescient because it explored the future through the then-present. “His stories foresaw the availability of the Internet, virtual reality, facial recognition software, driverless cars and 3D printing,” Nicholls said — while also pointing out that “it’s a misinterpretation that prediction is the primary purpose of science fiction.” The genre’s hit rate is actually not very good in that respect. Like all the best science fiction, his stories weren’t really about the future; they were about the here and now.”Putting aside Dick’s ability to foresee the future we now take for granted, his most disturbing vision was of the world itself ultimately being a simulation (模拟). Dick’s reality was already a delicate and complex one. In many of his later books, the idea of reality being a façade (假象) grew as a dominant theme. “Dick argued we were existing in a simulation,” Nicholls suggested.Whether his visions were true, as he believed, a product of small problems in the simulation or his fading mental health, one thing is for certain: the world in which the work of Philip K. Dick is celebrated today feels ever closer to the ones imagined by this most unique and exceptional of writer.12.How does the author explain the topic in Paragraph 1?A.By listing examples.B.By using metaphors.C.By making a comparison.D.By introducing an concept.13.What could be inferred from Paragraph 2?A.Dick can predict the future precisely.B.Some directors like to adapt Dick’s novels into movies.C.Dick’s novels redefined what science fiction was about.D.No one wrote more science fiction novels of our times than Dick.14.A universal feature of all the best science fiction stories is that they _______.A.have a high hit rate B.are good at predictingC.focus on the present D.explore the distant future15.What does the author want to convey in the last paragraph?A.Philip K. Dick had a great impact on science.B.Philip K. Dick had traveled into the future then.C.People don’t agree to Philip K. Dick’s prediction.D.The world in Philip K. Dick’s works is similar to today’s world.Imagine that as you are boarding an airplane, half the engineers who built the plane tell you there is a 10 percent chance the plane will crash, killing you and everyone else on board. Would you still board?In 2022, over 700 top academics and researchers behind the leading artificial intelligence companies were asked in a survey about future AI risk. Half of those surveyed stated that there was a 10 percent or greater chance of human extinction from future AI systems. 16 The fear of AI has haunted humanity since the mid-20th century, yet until recently it has remained a distant prospect, something that belongs in sci-fi more than in serious scientific and political debates. 17 It is even harder to grasp the speed at which these tools are developing even more advanced and powerful capabilities. But most of the key skills boil down to one thing: the ability to manipulate (操纵) and generate language, whether with words, sounds or images.In the beginning was the word. 18 From language emerges myth and law, goods and money, art and science, friendships and nations—even computer code. AI’s new mastery of language means it can now hack and manipulate the operating system of civilization. What would it mean for humans to live in a world where a large percentage of stories, melodies, images, laws, policies and tools are shaped by non-human intelligence? 19 What happens when the same thing occurs in art, politics, and even religion?20 We are surrounded by culture, experiencing reality through a cultural prism (棱镜). Our views are shaped by the reports of journalists and the accounts of friends. What will it be like to experience reality through a prism produced by non-human intelligence? The time to reckon with AI is before our politics, our economy and our daily life become dependent on it. A.Humans often don’t have direct access to reality.B.Language is the operating system of human culture.C.In games like chess, no human can hope to beat a computer.D.By gaining mastery of language, AI is seizing the master key to civilization. E.Technology companies are caught in a race to put all of humanity on that plane.F.For thousands of years we humans have lived inside the dreams of other humans.G.It’s difficult for human minds to grasp the capabilities of GPT-4 and similar tools.二、完形填空At school, art class is fun. We can 21 with different techniques and generally get creative. However, a field trip to an art gallery is often 22 . Last year my art teacher organized a trip to an art exhibition. The gallery was full of older people, who obviously didn’t want to be with 23 students. We all got quite 24 and couldn’t stop chatting. Our teacher was getting 25 and kept telling us to be quiet.The next day we complained to our teacher about the 26 of activities for teens at art galleries. She 27 that a visit should be both educational and fun. That was when I decided to go online and look for art galleries that have special 28 for teens. Eventually, I29 to find a huge range of activities and proposed some to my teacher.I also used the 30 to learn about more artists. Recently, I found a contemporary artist called Martin Bailey. I’ve 31 seen artists who combine different techniques, but Bailey is totally different. He does unique illustrations with 32 household objects such as umbrellas, headphones and even cookies. His art is simple, but it enables you to see things 33 . For example, he notices that a flower is similar to a mop (拖把) and puts this 34 into life by drawing a little man with a real flower mop. It’s really 35 ! I hope I’ll be able to go to an exhibition of his work in the future.21.A.live B.start C.struggle D.experiment 22.A.exciting B.disturbing C.rewarding D.disappointing 23.A.noisy B.humble C.creative D.innocent 24.A.bored B.annoyed C.concerned D.enthusiastic 25.A.cruel B.sensitive C.worn out D.stressed out 26.A.lack B.abuse C.theme D.schedule 27.A.agreed B.demanded C.criticised D.announced 28.A.prices B.events C.entries D.paintings 29.A.expected B.managed C.resolved D.happened 30.A.trip B.activity C.Internet D.exhibition31.A.barely B.merely C.already D.apparently 32.A.delicate B.ordinary C.suitable D.sustainable 33.A.clearly B.equally C.differently D.precisely 34.A.tool B.idea C.design D.blossom 35.A.abstract B.realistic C.amusing D.practical三、语法填空阅读下面短文,在空白处填入1个适当单词或括号内单词的正确形式。
河南省南阳市2023-2024学年高二上学期11月期中英语试题
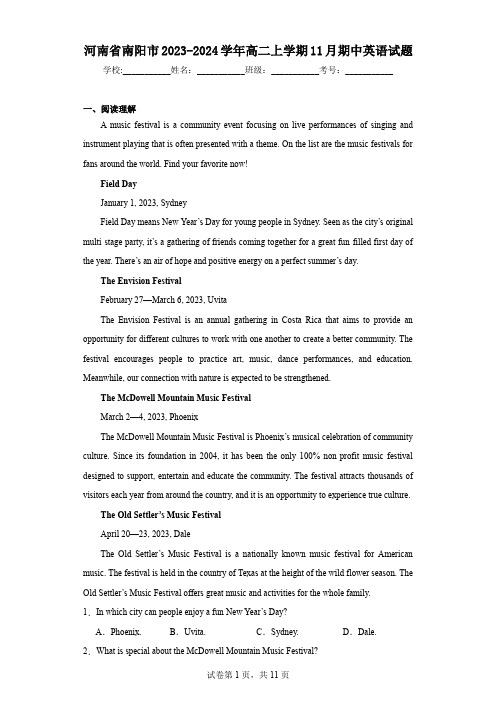
河南省南阳市2023-2024学年高二上学期11月期中英语试题学校:___________姓名:___________班级:___________考号:___________一、阅读理解A music festival is a community event focusing on live performances of singing and instrument playing that is often presented with a theme. On the list are the music festivals for fans around the world. Find your favorite now!Field DayJanuary 1, 2023, SydneyField Day means New Year’s Day for young people in Sydney. Seen as the city’s original multi-stage party, it’s a gathering of friends coming together for a great fun-filled first day of the year. There’s an air of hope and positive energy on a perfect summer’s day.The Envision FestivalFebruary 27—March 6, 2023, UvitaThe Envision Festival is an annual gathering in Costa Rica that aims to provide an opportunity for different cultures to work with one another to create a better community. The festival encourages people to practice art, music, dance performances, and education. Meanwhile, our connection with nature is expected to be strengthened.The McDowell Mountain Music FestivalMarch 2—4, 2023, PhoenixThe McDowell Mountain Music Festival is Phoenix’s musical celebration of community culture. Since its foundation in 2004, it has been the only 100% non-profit music festival designed to support, entertain and educate the community. The festival attracts thousands of visitors each year from around the country, and it is an opportunity to experience true culture.The Old Settler’s Music FestivalApril 20—23, 2023, DaleThe Old Settler’s Music Festival is a nationally known music festival for American music. The festival is held in the country of Texas at the height of the wild flower season. The Old Settler’s Music Festival offers great music and activities for the whole family.1.In which city can people enjoy a fun New Year’s Day?A.Phoenix.B.Uvita.C.Sydney.D.Dale. 2.What is special about the McDowell Mountain Music Festival?A.It encourages people to receive education.B.It is not aimed at making money.C.It provides an opportunity for friend gathering.D.It focuses on cultural exchanges. 3.Which festivals are connected with nature?A.Field Day and the Envision Festival.B.The Envision Festival and the McDowell Mountain Music Festival.C.The Old Settler’s Music Festival and the McDowell Mountain Music Festival.D.The Envision Festival and the Old Settler’s Music Festival.In the year 2000, as usual, my family were coming back from a T-ball game at the weekend. However, little did we know that a surprise was waiting for us in our driveway —panic, with their baby left behind.Hours passed before night eventually fell. It was clear that the small goose needed protection, warmth and food to make it to the morning. We brought him to our backyard. Each morning, we would try to drive the small goose away to his parents, who kept coming back to our yard. He wouldn’t go to them, though, and neither would the adult geese come close enough to take him back. Realizing the young goose had apparently decided we were his family then, we gave him a name, calling the little guy Peeper.Days turned into weeks and weeks into months. The little creature had grown into a big bird with two powerful wings before we knew it. One day, when my dad threw Peeper in the air, he just flew away and didn’t come back. With night falling, all of us became increasingly worried. We looked for him, called his name and anxiously expected his return. But he never appeared again. It took a long time before we accepted the fact that he was missing. We could only pray he found his parents and went off on his natural way.So I was thrilled to see when, in 2019, an adult goose made his way back to my family home. He did all of the same things Peeper used to do! Much to my amazement, he even responded to the name Peeper. It became clear to me that my old best friend had returned many years later.This experience has been as meaningful to me as anything in my life. Looking beyond our reach high in the sky, birds have feelings like human beings, so do many other living things. We human beings should learn to get along with them. We need each other’s care and protection for a better world.4.Which of the following can best replace “startled” in paragraph 1?A.Astonished.B.Confused.C.Terrified.D.Embarrassed. 5.Why did the family give the goose a name Peeper?A.Because the small goose regarded himself as one of the family members.B.Because the small goose was abandoned by its parents.C.Because the small goose didn’t respond to the family when spoken to.D.Because the small goose finally came back many years later.6.How did the family feel when they found the goose was missing?A.Thrilled and Relieved.B.Puzzled and Desperate.C.Frightened and Upset.D.Concerned and Sorrowful.7.What does the author intend to convey in the last paragraph?A.To raise people’s awareness of environmental protection.B.To share a story about a goose and his family.C.To remind readers to live in harmony with wild animals.D.To call for people’s love and care for geese.David Bennett Sr. , who was seriously ill and seeking a miracle, took the last bet on Jan. 7, when he became the first human to be successfully transplanted with the heart of a pig. “It creates the beat; it creates the pressure; it is his heart,” declared Bartley Griffith, director of the surgical team that performed the operation at the University of Maryland Medical Center.Bennett, aged 57, held on through 60 tomorrows, far longer than any previous patient who’d received a heart from another species. His remarkable run offered new hope that such procedures, known as xenotransplantation (异种移植) could help relieve the shortage of replacement organs, saving thousands of lives each year.The earliest attempts at xenotransplantation of organs, involving kidneys from rabbits, goats, and other animals, occurred in the early 20th century, decades before the first successful human-to-human transplants. Rejection, which occurs when the recipient’s body system recognizes the donor organ as a foreign object and attacks it, followed within hours or days. Results improved after some special drugs arrived in the 1960s, but most recipients still died after a few weeks. The record for a heart xenotransplantation was set in 1983, when an infant named Baby Fae survived for 20 days with an organ from a baboon (狒狒).However, in recent years, advances in gene editing have opened a new possibility:re-edit some genes in animals to provide user-friendly spare parts. Pigs could be ideal for this purpose, because they’re easy to raise and reach adult human size in months. Some biotech companies, including Revivicor, are investing heavily in the field. The donor pig was offered by Revivicor from a line of animals in which 10 genes had been re-edited to improve the heart’s condition Besides, the pig was raised in isolation and tested regularly for viruses that could infect humans or damage the organ itself.This medical breakthrough provided an alternative for the 20% of patients on the heart transplant waiting list who die while waiting or become too sick to be a good candidate. 8.What can we learn from the passage?A.Bennett survived the surgery and has lived healthily since then.B.The operation on Bennett was successfully carried out.C.Xenotransplantation completely solve the problem of the shortage of replacement organs.D.Many patients have received the heart from a pig but failed to live as long as Bennett. 9.What does the author intend to show in paragraph 3?A.The process of an xenotransplantation operation.B.The consequence of the xenotransplantation operation.C.The significance of the xenotransplantation operation.D.The past exploration of the xenotransplantation operation.10.What makes pigs ideal for providing spare parts in xenotransplantation?A.Their growth speed and health condition.B.Their life pattern and resistance to viruses.C.Their easiness of keeping and rapid growth.D.Their investment value and natural qualities.11.Why was Bennett’s operation considered as a breakthrough?A.It offered a possibility of replacement organs through gene editing.B.It proved the potential for using organs from various animals.C.It guaranteed a sufficient supply of donor pigs for transplants.D.It introduced new medications to prevent organ rejection.Ask the new artificial intelligence (AI) tool ChatGPT to write an essay about the cause of the American Civil War and you can watch it produce a persuasive term paper in a matterof seconds that has even be enable to pass school exams. That’s one reason why New York City school officials this week started blocking the impressive but controversial writing tool that can generate paragraphs of human-like text. The free tool has been around for just five weeks but is already raising tough questions about the future of AI in education, the tech industry and a host of professions.ChatGPT was launched on Nov. 30 and is part of a new generation of AI systems that can chat, generate readable text on demand and even produce novel images and video based on what they’ve learned from a vast database of digital books, online writings and other media. But unlike previous models of so-called “large language models”, such as Open AI’s GPT-3, launched in 2020, the ChatGPT tool is available to anyone with an Internet connection for free and designed to be more user-friendly. It works like a written dialogue between the AI system and the person asking it questions.Millions of people have played with it over the past month, using it to write silly poems or songs, trying to trick it into making mistakes, or for more practical purposes such as helping compose an email.As with similar systems, ChatGPT can generate convincing prose, but that doesn't mean what it says is factual or logical. Its launch came with little guidance on how to use it, other than a promise that ChatGPT will admit when it's wrong.Many school districts are still struggling to figure out how to set policies on whether and how it can be used. “While the tool may be able to provide quick and easy answers to questions, it does not build critical-thinking and problem-solving skills, which are essential for academic and lifelong success,” said a school’s spokesperson Jenna Lyle from NYC.But there's no stopping a student from accessing ChatGPT from a personal phone or computer at home.12.What can we learn about the term paper from paragraph 1?A.It is a result of the improvement of education.B.It can be rated as passing byschoolteachers.C.It has caught the attention of the public.D.It acts as a model for students to follow. 13.What makes Chat GPT different from GPT-3?A.ChatGPT can create text.B.ChatGPT can edit digital books.C.ChatGPT is free of charge to all.D.ChatGPT can ask its users questions. 14.What is Jenna's attitude towards students’ use of Chat GPT?A.Favourable.B.Tolerant.C.Uncaring.D.Disapproving. 15.What is the best title for the text?A.How Are Schools Handling Chat GPT?B.You Can Check When ChatGPT’s Telling the TruthC.What Is ChatGPT and Why Are Schools Blocking It?D.Students Are Using ChatGPT to Do Their Homework二、七选五The human brain is the command center for the nervous system and enables thoughts, memory, movement, and emotions. As the population ages, the challenges for thehow to protect it.Exercise regularly. Regular physical exercise tends to help fight against the natural reduction in brain connections that occur during aging. Multiple studies show that physically active people are less likely to experience a decline in their mental function and have a lower risk of developing brain disease. 17Have a “right” nap. Having a nap after lunch can be good for your brain and many seniors have developed such a good habit for long. 18 While a 30-min to 90-min nap has brain benefits, anything longer than an hour and a half may create problems with cognition, the ability to think and form memories.19 Your brain is similar to a muscle -you need to use it or lose it. There are many things that you can do to keep your brain in shape, such as doing crossword puzzles, reading, or playing cards. You can also learn something new such as musical instruments, drawing, or even digital devices. Combine different types of activities to increase the effectiveness.Eat a Mediterranean diet. Studies show people who closely follow a Mediterranean diet are less likely to have brain disease than people who don’t follow the diet. 20 However, we at least know that omega fatty acids found in olive oil and other healthy fats are vital for your cells to function correctly.A.Stay mentally active.B.Explore new interests if possible.C.But keep in mind that the length matters.D.These benefits result from regular exercise.E.Your diet plays a large role in your brain health.F.Therefore a healthy brain is the primary goal in pursuing health for seniors.G.Further research is needed to decide which parts of the diet help brain the most三、完形填空Since finishing my studies at Harvard and Oxford, I’ve watched one friend after another land high-ranking, high-paying Wall Street jobs. As executives with banks, consulting firms,most.21.A.much B.never C.very D.well 22.A.least B.last C.first D.best 23.A.shared B.paid C.equaled D.spent 24.A.committed B.witnessed C.admitted D.classified 25.A.complain B.dream C.hear D.approve 26.A.curious B.guilty C.envious D.empty 27.A.accustomed B.appointed C.accessible D.available 28.A.also B.but C.instead D.rather 29.A.let out B.give away C.give up D.believe in 30.A.fundamental B.practical C.impossible D.unforgettable 31.A.take off B.drop off C.put off D.pay off 32.A.missing B.inspiring C.sinking D.shining 33.A.measure B.suffer C.digest D.deliver 34.A.catastrophes B.motivations C.campaigns D.decisions 35.A.assessed B.involved C.covered D.estimated四、用单词的适当形式完成短文阅读下面短文,在空白处填入1个适当的单词或括号内单词的正确形式。
managers' Green Investment Disclosures and Investors' reaction
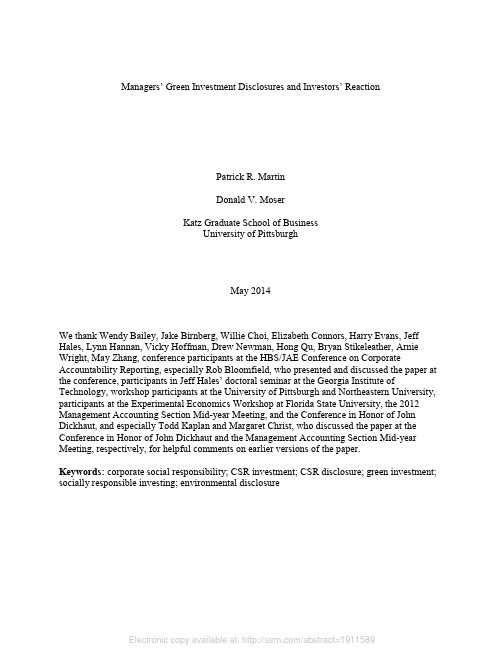
Managers’ Green Investment Disclosures and Investors’ ReactionPatrick R. MartinDonald V. MoserKatz Graduate School of BusinessUniversity of PittsburghMay 2014We thank Wendy Bailey, Jake Birnberg, Willie Choi, Elizabeth Connors, Harry Evans, Jeff Hales, Lynn Hannan, Vicky Hoffman, Drew Newman, Hong Qu, Bryan Stikeleather, Arnie Wright, May Zhang, conference participants at the HBS/JAE Conference on Corporate Accountability Reporting, especially Rob Bloomfield, who presented and discussed the paper at the conference, participants in Jeff Hales’ doctoral seminar at the Georgia Institute of Technology, workshop participants at the University of Pittsburgh and Northeastern University, participants at the Experimental Economics Workshop at Florida State University, the 2012 Management Accounting Section Mid-year Meeting, and the Conference in Honor of John Dickhaut, and especially Todd Kaplan and Margaret Christ, who discussed the paper at the Conference in Honor of John Dickhaut and the Management Accounting Section Mid-year Meeting, respectively, for helpful comments on earlier versions of the paper.Keywords: corporate social responsibility; CSR investment; CSR disclosure; green investment; socially responsible investing; environmental disclosureManagers’ Green Investment Disclosures and Investor s’ ReactionAbstractMost large companies voluntarily disclose information about their corporate social responsibility (CSR) activities. We use experimental markets to examine how managers’ disclosures of a particular type of CSR, green investment, affect investors’ bidding behavior. We find that, although in our setting such investments have no impact on future cash flows, investors value knowing that a green investment was made and also respond more favorably to disclosures that focus on the societal benefits of the investment versus on the cost to the company. Managers appear to anticipate investors’ positive reaction, overwhelmingly disclosing when they made a green investment and more often focusing their disclosures on the societal benefits rather than on the cost to the company. Although managers and other current shareholders benefit when managers disclose their green investment, the benefit is always lower than the cost of the investment, and thus both the manager and other current shareholder always bear a cost when the manager makes a green investment. This suggests that many managers in our study make green investments because they value the associated societal benefits. Collectively, our results show that both investors and managers trade off personal wealth for societal benefits associated with CSR activities and help explain why voluntary CSR disclosures often focus on the benefits to society rather than on the cost to the company. Our study also demonstrates how experiments can effectively study important CSR issues that are difficult to address using archival data.1. IntroductionAlthough not required, most large companies now issue reports on CSR performance.1 This voluntary disclosure of CSR activities is likely driven at least in part by a desire to communicate CSR information to investors. If CSR activities affect the firm’s future earnings and cash flows, investors will find related disclosures useful for their valuation decisions.However, it is possible that investors react to CSR disclosures for another reason as well. If investors value the societal benefits associated with CSR activities, they may respond positively to disclosures that the firm has engaged in such activities independent of how they expect the activities to affect future earnings and cash flows. We conduct an experiment to test whether investors respond t o managers’ disclosure of their CSR investment independent of the effect on the firm’s future cash flows. In addition, we examine whether managers anticipate investors’ response when maki ng their disclosure decisions. Finally, we examine whether managers’ CSR investment decisions are driven only by investors’ expected response or also by their preferences for the societal benefits associated with their CSR investments.Understanding how investors’ respond to CSR disclosure is important because this can help explain managers’ voluntary CSR disclosure practices. For example, knowing that investors value the societal benefits of CSR activities would help explain why manager s’ CSR disclosures tend to focus on such benefits. Also, knowing whether investors’ reaction to CSR disclosures goes beyond the expected effect of CSR activities on the firm’s future cash flows could help explain the rapid growth in Socially Responsible Investment (SRI) funds (Social Investment Forum Foundation 2012). More broadly, a more complete understanding of investors’ reaction to1 Although most CSR disclosures are voluntary, domestic U.S. public companies are required to disclose any material risks resulting from the legislative, regulatory, business, market, or physical impact of climate change (SEC, 2010. Commission Guidance Regarding Disclosure Related to Climate Change).1CSR disclosure can inform standard setters who are considering whether CSR disclosures should be required, what information should be disclosed, and whether such disclosures should be audited.2 Finally, understanding why managers invest in CRS activities helps inform the ongoing debate regarding whether all CRS activities must be shareholder value maximizing (Friedman 1970, Karnani 2010) or whether some such activities sacrifice profits in the social interest (Benabou and Tirole 2010, Reinhardt et al. 2008, Kolstad 2007).We examine a particular type of CSR activity, green investing, in an experimental market setting. There are several critical features of our setting that allow us to isolate the effects necessary to answer our research questions. First, in our experimental setting, the impact of manager’s green investment is fully reflected in the firm’s current earnings, and as such there can be no further impact on the firm’s future cash flows. This ensures that any observed investor reaction to disclosure of the green investment is not based on investors’ expectations regarding how the investment will affect future earnings. Second, we ensure that both investors and managers in our experiment know that the financial cost to the company of a green investment always exceeds the financial benefit, i.e., the investment is always unprofitable. Thus, any green investment a manager makes always lowers shareholder value and therefore any positive investors’ response to the disclosure of a green investment must reflect the investors desire to reward the manager for engaging in an activity that the investors value.We find that potential investors’ standardized bids for the company are higher when managers disclose their green investments than when they do not, providing evidence that2 In addition to the traditional standard setters such as the SEC, FASB, and IASB, a number of other organizations have established or are working on establishing guidelines regarding sustainability reporting. Some notable examples include the Global Reporting Initiative (GRI), the International Integrated Reporting Council (IIRC) and the Sustainability Accounting Standards Board (SASB).2investors value the societal benefits associated with the investment. We also provide some evidence that investors respond more positively when managers’ disclosures focus on the societal benefits of their investment rather than on the cost to the company. In addition, it appears that managers’ anticipate investors’ reaction in that managers overwhelmingly disclose their investment and tend to focus their disclosure on the societal benefits of the investment rather than on the cost to the firm. Finally, despite the positive investor response to disclosure of the green investment, both managers and current investors nevertheless always bear a cost when the manager makes a green investment. Thus, managers’ investment decisions cannot be fully explained by the expected investor reaction, but must also reflect the value they place on the societal benefits associated with their investment.In addition to the findings reported above, one unexpected finding is that, while managers who make very high amounts of unprofitable green investment often disclose that they have made such investments, they typically do not disclose the amount. This result is consistent with managers’ responses to a post experiment question indicating that, although they expected potential investors to react favorably to increases in the amount of investment in the lower range of investment amounts, they also expected that potential investors might react unfavorably to very high amounts of investment. Consistent with this expectation, we document a positive correlation between potential investor s’ standardized bids and the disclosed amount of investment. However, because managers rarely disclosed very high green investment amounts, we do not have sufficient data to test whether managers were right to be concerned that investors’ might react unfav orably to such high investment amounts.Our findings contribute to the CSR literature in several ways. First, our finding that investors’ positive response to disclosures of a green investment are based at least in part on the3societal benefits associated with the investments helps us better understand the rapid increase in SRI funds (Social Investment Forum Foundation 2012). Second, our results offer insights into how and why managers disclose their CSR activities to investors. Third, our study helps inform standard setters who are considering possible CSR disclosure requirements. Finally, our study demonstrates the advantages of using experiments to examine important CSR issues that are difficult to study effectively using archival data.In Sections 2 and 3 we provide background information and present our hypotheses. We describe our experiment in Section 4 and report our results in Section 5. The paper concludes with a discussion of our results and their implications in Section 6.2. BackgroundThe KPMG International Survey of CSR (2013) reports that 93 percent of the 250 largest global companies and 86 percent of the 100 largest US companies now engage in some type of voluntary CSR disclosure. If CSR activities affect the future earnings and cash flows of the company, disclosing such activities will be useful for investors’ valuation decisions. There are several ways that CSR performance could af fect a firm’s future earnings. For example, being more socially responsible could add customers, increase sales, or increase pricing power (Lev et al. 2010), attract or motivate employees (Balakrisnan et al. 2011, Bhattacharya et al. 2008), lower the cost of equity capital (Dhaliwal et al. 2011) or reduce the risk of governmental regulation (Paine 2000). Based on such arguments, researchers have often focused on establishing a positive association between CSR and measures of financial performance. Margolis et al. (2009), a recent meta-analysis of 251 such studies over the last 40 years, conclude that “the overall effect is positive but small…and the results for the 106 studies for the past decade are even smaller.” Of the 251 studies, 59% reported a non-significant result, 28% found a4positive result, 2% a negative result, and the remaining 10% did not report sample size or significance.Although this prior research does not completely resolve whether firms’ CSR performance is associated with their future financial performance, investors’ reaction s to CSR disclosures are likely to be at least partially due to their expectations regarding the effect on financial performance. However, investors could also react to CSR disclosures because they value the societal benefits associated with CSR activities, and thus they could respond positively to knowing that the firm has engaged in such activities independent of how they expect CSR activities to affec t the firms’ future cash flows. Such a reaction would be very difficult to isolate using archival data because it is not possible to separate it from a reaction based on investor expectations regarding the effect of CSR activities on future earnings. We overcome this difficulty by using an experimental setting in which the impact of the CSR activity is fully incorporated into the current earnings, and as such there can be no further impact on the firm’s future cash flows. This ensures that any investor reaction is not due to their expectations regarding the impact of CSR activities on future earnings.3. Development of Research Question and HypothesesA positive response by investors to disclosed CSR activities could reflect their desire to reward company managers for taking an action they value. The rapid growth in SRI funds in recent years is consistent with a growing group of investors rewarding companies for being socially responsible. Social Investment Forum Foundation (2012) estimates that $3.74 trillion of the $33.3 trillion being professionally managed in the US in 2012 was invested using criteria based on social responsibility, and the amounts invested using such criteria grew 22% from 2009 to 2012 such that by 2012 over 720 investment funds incorporating socially responsible criteria5were available. It appears that some investors value the societal benefits associated with CSR activities and consequently want to reward managers for engaging in such activities by investing in their companies.The behavior described above is consistent with a line of experimental research documenting reciprocal behavior, in which a kind act is reciprocated with a similarly kind act in return (Rabin 1993). Such positive reciprocal behavior has been documented in a number of settings including labor markets and other experimental games. In labor market settings, research shows that in incomplete contract environments, employers often offer a “gift” of a wage greater than the market-clearing level and workers reciprocate with a “gift” of greater effort than the minimum enforceable amount (Akerlof 1982; Fehr et al. 1993, 1997; Hannan et al. 2002; Hannan 2005; Kuang and Moser 2009, 2011).3Reciprocal behavior has also been documented in a widely examined experimental game known as the “trust game.” In this game, a “first mover” is given a sum of money and must decide how much of it, if any, to give to an anonymous counterparty. Any money given to the counterparty is increased by a multiplier (often tripled), and then the counterparty decides how much of the new amount to keep for him/herself and how much to give back to the first mover. Berg et al. (1995) introduced this game and found strong reciprocal behavior by the counterparty. On average, the first mover transferred 52% of their money to their counterparty, apparently anticipating that the counterpart would reciprocate, and the counterparty did, in fact, reciprocate by returning on average 30% of the new tripled amount.Our experimental setting shares some similarities with the standard gift-exchange or trust game settings described above in that our managers are similar to the employers in the gift-3 See Fehr et al. (2009) for a review of this literature.6exchange studies and to the first movers in the trust game studies, while our investors are similar to the employees in the gift-exchange studies and the counterparty in the trust game studies.However, our setting differs from the previous settings in important ways. First, in our setting, the counterpart to the employer’s gift and first mover’s transfer in the previous studies is the manager’s“green investment”, and importantly, unlike in the previous studies, the manager’s green investment does not financially benefit the investors directly. Therefore, in our setting any reciprocal motivation on the part of investors must operate through the value they place on the societal benefits of the green investment rather than on any potential personal financial gain. Second, managers who make a green investment in our study affect not only their own wealth, but also the wealth of another current shareholder. In prior studies, when employers (first movers) offer a gift (transfer), they only reduce their own wealth. Third, in prior studies, the employee (second mover) could directly reciprocate the employer’s (first mover’s) behavior, but in our market setting, it is less clear that any individual investors can directly reciprocate the managers’ behavior because only the winning bidder can ultimately do so.The differences described above make positive reciprocation by investors less likely in our setting than in previous studies documenting reciprocity. Nevertheless, there are still reasons to believe that investors in our setting will react positively when managers disclose their green investment. For example, Martin (2009) found that investors in a market setting similar to ours were willing to bear part, but not all, of the cost of a green investment made by the sole owner of a company. In addition, Elfenbein et al. (2010) used data from eBay auctions to show that customers were more likely to buy, and pay higher prices for, items for which the seller had committed to donate a portion of the sales proceeds to charity than identical items for which the seller had not made such a commitment. Again, the higher prices paid by customers reduced, but7did not fully offset, the cost to the firm of the charitable contribution. Finally, Balakrishnan et al. (2011) provide evidence that employer charitable giving can help motivate employee effort that benefits the employer, but, again, many employers only recovered part of the cost of their charitable donations. Based on the discussion above, we test the following hypothesis: Hypothesis 1(H1): Holding the distribution of possible cash flows constant, investors will respond more positively to disclosure of a green investment than to no report about green investing.As hypothesized above, we expect that investors who value the societal benefits associated with green investments will reciprocate the manager’s behavior by paying higher prices when managers disclose that they made a green investment. However, investor reaction is expected to vary depending on how managers frame their green investment disclosure. Different framing of equivalent information has been shown to influence individuals’ judgments in many different settings (see Levin et al. 1998 for a review of the framing literature). If investors’ reciprocal response reflects the value they place on the societal benefits of a green investment, they are likely to respond more favorably to reports that focus on the societal benefits of the investment rather than on the cost of the investment to the firm. Thus, our second hypothesis is: Hypothesis 2 (H2): Investors will react more positively to disclosures that focus on the societal benefits of green investments than on disclosures that focus on the costs to the company of such investments.Since disclosures of CSR activities are largely voluntary, managers have a great deal of leeway in deciding what, if anything, they wish to disclose about such activities. It is reasonable to expect managers to make disclosure decisions regarding their CSR activities based on their expectations regar ding investors’ reaction. However, recall that in our experimental setting the impact of the green investment is fully incorporated into the firm’s current earnings, and as such there can be no effect on the future cash flows of the firm. Thus, manager s’ disclosure decisions8in our setting must be based on expectations of investor responses to non-economic information in the disclosure. If investors respond to disclosures in the manner hypothesized in H1 and H2, managers would be expected to either anticipate or learn which forms of disclosure yield the most positive investor response. Thus, we investigate the two hypotheses below:Hypothesis 3 (H3): Managers who make a green investment will more often disclose to investors that they have done so rather than make no report.Hypothesis 4 (H4): Managers’ disclosures of their green investment will more often focus on the societal benefits of the investment than on the cost to the company.Recall that in our setting any green investment the manager makes is always unprofitable for the company. Therefore, managers and the other current investors will always bear a cost when managers make a green investment unless investors reward managers sufficiently to overcome the cost of the unprofitable investment. If managers in our study are repeatedly willing to bear such a personal cost and also repeatedly willing to impose such a cost on the other current investors, this suggests that they are not making green investments because they naively expect investors to fully compensate them for the cost of the investment. Rather, because we can rule out this explanation and all other potential standard economic explanations for managers’ green investments, we can attribute managers’ green investments to the value they place on the societal benefits associated with such investments.4. Experiment4.1 Overview of ExperimentWe conducted our experimental markets using z-tree software in a networked computer lab (Fischbacher 2007). We recruited 90 volunteer participants from a lab participant pool of approximately 1,300 individuals. Our participants were 55% male and averaged 21 years of age. Three experimental sessions with 30 participants each were conducted. Each experimental9session consisted of 20 independent periods and lasted approximately 90 minutes. At the conclusion of each session, one of the 20 periods was randomly selected and participants were paid their $5 participation fee plus their earnings for the randomly selected payment period. Participants’ earnings depended on the decisions that they and other participants made during the experiment (details provided below).In each of the three sessions, participants were randomly assigned to one of three roles: 1) a manager who was a shareholder in the company, 2) another current shareholder in the company, or 3) one of three potential investors in the company. We distinguish between “potential investors” and “current shareholder s” in our setting and measure investor reaction based on the price set by the potential investors. That is, our other current shareholders do not play a role in setting the market price. However, it was important to include other current shareholders in our design to reflect the fact that, like our managers, the other current shareholders also bear a direct financial cost when managers make an unprofitable green investment. Participants’ randomly assigned roles as a manager, a current shareholder, or a potential investor were constant throughout the experiment.Each period, one manager was randomly matched with one current shareholder and three potential investors, creating a group of 5 participants. There were 6 such groups of 5 in each of our 3 experimental sessions, resulting in a total of 18 groups. Thus, our 90 participants consisted of 18 managers (one per group), 18 current investors (one per group) and 54 potential investors (three per group). With 20 periods in each session, this resulted in 360 observations (investment and reporting decisions) from managers (18 managers x 20 periods) and 360 observations (winning bids) from the potential investors (one winning bid for each of the 18 groups of three investors x 20 periods). Because managers, current shareholders, and potential investors were10randomly re-matched into new 5-member groups each period, they never knew with whom they were matched at any point in their experimental session. Therefore, participants knew that all their decisions were anonymous and neither managers nor investors could form reputations in our experiment.4At the start of each period, the manager and the other current shareholder each owned one-half of the company. This ownership structure captures forces that are important in actual corporate settings. Specifically, this structure provides managers with 1) a personal financial deterrent against investing in the unprofitable green project, 2) a deterrent against investing in the unprofitable green project because of a fiduciary responsibility to the other current shareholder, and 3) an incentive to invest in the unprofitable green project because half of the cost of the investment can be shifted to the other current shareholder.Managers decided whether to make a green investment and what to disclose about their investment choice to potential investors. Potential investors then placed bids to purchase the company. Both managers and potential investors knew that any amount of green investment that was made had a real societal benefit in reducing carbon emissions because they knew that the full amount of any green investment would be donated by the researchers to , a real non-profit environmental organization that invests contributions in renewable energy and reforestation projects that reduce the amount of greenhouse gases in the environment.5 After the experiment was completed, the actual dollar amount of the green investment made by managers for the randomly selected payment period was contributed to .4.2 Detailed Experimental Procedures4 In addition to being anonymous between each other, participants’ knew that their decisions were also anonymous to the experimenters because their decisions were only tracked by participant number.5 See for additional information11A time-line of the steps in each period of each experimental session is provided in Figure1. As shown in Step 1, each period managers learned the amount of earnings for the company before they made any green investment (hereafter referred to as the “before-investment earnings”) and then decided whether to invest a portion of those earni ngs to reduce carbon emissions. Possible green investment amounts ranged from $0 to $20 in $1 increments.(Figure 1)The company’s before-investment earnings for each period were drawn from a uniformly distributed distribution ranging from $25-$35 in two stages. In the first stage, a distribution with a smaller $5 range was randomly drawn from the uniformly distributed larger $10 range ($25-$35). We refer to t his smaller $5 range as the “be fore-investment earnings range.” In the second stage, the before-investment earnings amount (i.e., a single specific earnings amount) was randomly drawn from the uniformly distributed smaller $5 before-investment earnings range. This specific amount is the before-investment earnings amount that managers saw before making their green investment decision. As described in m ore detail later, the company’s before-investment earnings were selected using this two-stage process to limit the inferences potential investors could make regarding whether a green investment had been made.Because any amount of green investment reduced the company’s energy costs, the net cost of the green investment to the company was always less than the amount contributed to . (hereafter referred to as the societal benefit associated with the investment)6. In6 We recognize that not all individuals view the reduction in carbon emissions caused by the contribution to when managers invest in the green project as resulting in the same amount of societal benefit, or even any societ al benefit at all. However, describing the amount of the green investment as the “societal benefit” is at least partially justified because unless participants valued the effect of the contribution on society there would be no reason to ever make a green i nvestment in the experiment. That is, managers’ expected payoffs in the experiment were always higher when they did not make a green investment. For this reason and to facilitate exposition, we refer to the amount of a manager’s green investment as the soc ietal benefit.12。
ON THE “HOT SPOTS ” CONJECTURE OF J. RAUCH
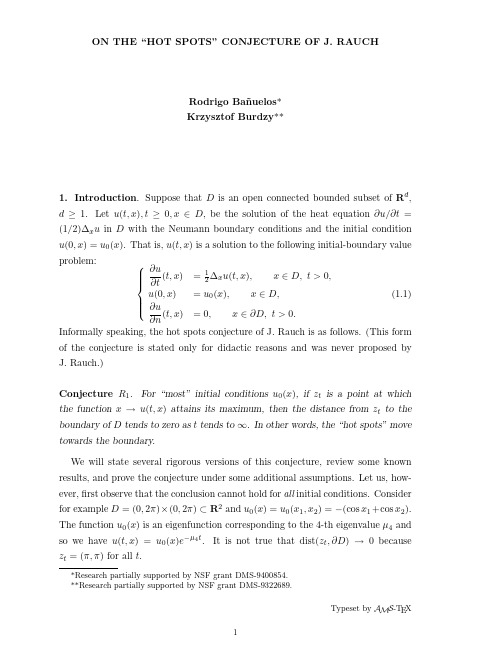
where c1 and c2 = 0 are constants depending on the initial condition, µ2 is the second eigenvalue for the Neumann problem in D, ϕ2 (x) is a corresponding eigenfunction, and R(t, x) goes to 0 faster than e−µ2 t , as t → ∞. We will make this precise below in Proposition 2.1. The eigenfunction expansion (1.2) leads to a version of the “hot spots” conjecture which involves the second eigenfunction. We will state several versions of the conjecture, with varying strength of the analytic condition and for various classes of domains. Consider the following statements for a domain D. (HS1) For every eigenfunction ϕ2 (x) corresponding to µ2 which is not identically 0, and all y ∈ D, we have inf x∈∂D ϕ2 (x) < ϕ2 (y ) < supx∈∂D ϕ2 (x). (HS2) For every eigenfunction ϕ2 (x) corresponding to µ2 and all y ∈ D, we have inf x∈∂D ϕ2 (x) ≤ ϕ2 (y ) ≤ supx∈∂D ϕ2 (x). (HS3) There exists an eigenfunction ϕ2 (x) corresponding to µ2 which is not identically 0, and such that for all y ∈ D, we have inf x∈∂D ϕ2 (x) ≤ ϕ2 (y ) ≤ supx∈∂D ϕ2 (x). The strongest statement (HS1) asserts that the inequalities are strict, while the other two statements involve weaker assertions. Note that all statements (HS1)(HS3) make assertions about both “hot spots” and “cold spots” of eigenfunctions. This is because if ϕ is an eigenfunction, so is −ϕ and so maxima and minima are indistinguishable in the context of this problem. Conjecture R2 . (Rauch) The statement (HS1) is true for every domain D ⊂ Rd . The “hot spots” conjecture was made, as we recently learned from Rauch, in 1974 in a lecture he gave at a Tulane University PDE conference. Despite the
NONLINEAR TIME SERIES ANALYSIS

More informationNONLINEAR TIME SERIES ANALYSISThis book represents a modern approach to time series analysis which is based onthe theory of dynamical systems.It starts from a sound outline of the underlyingtheory to arrive at very practical issues,which are illustrated using a large number ofempirical data sets taken from variousfields.This book will hence be highly usefulfor scientists and engineers from all disciplines who study time variable signals,including the earth,life and social sciences.The paradigm of deterministic chaos has influenced thinking in manyfields ofscience.Chaotic systems show rich and surprising mathematical structures.In theapplied sciences,deterministic chaos provides a striking explanation for irregulartemporal behaviour and anomalies in systems which do not seem to be inherentlystochastic.The most direct link between chaos theory and the real world is the anal-ysis of time series from real systems in terms of nonlinear dynamics.Experimentaltechnique and data analysis have seen such dramatic progress that,by now,mostfundamental properties of nonlinear dynamical systems have been observed in thelaboratory.Great efforts are being made to exploit ideas from chaos theory where-ver the data display more structure than can be captured by traditional methods.Problems of this kind are typical in biology and physiology but also in geophysics,economics and many other sciences.This revised edition has been significantly rewritten an expanded,includingseveral new chapters.In view of applications,the most relevant novelties will be thetreatment of non-stationary data sets and of nonlinear stochastic processes insidethe framework of a state space reconstruction by the method of delays.Hence,non-linear time series analysis has left the rather narrow niche of strictly deterministicsystems.Moreover,the analysis of multivariate data sets has gained more atten-tion.For a direct application of the methods of this book to the reader’s own datasets,this book closely refers to the publicly available software package TISEAN.The availability of this software will facilitate the solution of the exercises,so thatreaders now can easily gain their own experience with the analysis of data sets.Holger Kantz,born in November1960,received his diploma in physics fromthe University of Wuppertal in January1986with a thesis on transient chaos.InJanuary1989he obtained his Ph.D.in theoretical physics from the same place,having worked under the supervision of Peter Grassberger on Hamiltonian many-particle dynamics.During his postdoctoral time,he spent one year on a Marie Curiefellowship of the European Union at the physics department of the University ofMore informationFlorence in Italy.In January1995he took up an appointment at the newly foundedMax Planck Institute for the Physics of Complex Systems in Dresden,where heestablished the research group‘Nonlinear Dynamics and Time Series Analysis’.In1996he received his venia legendi and in2002he became adjunct professorin theoretical physics at Wuppertal University.In addition to time series analysis,he works on low-and high-dimensional nonlinear dynamics and its applications.More recently,he has been trying to bridge the gap between dynamics and statis-tical physics.He has(co-)authored more than75peer-reviewed articles in scien-tific journals and holds two international patents.For up-to-date information seehttp://www.mpipks-dresden.mpg.de/mpi-doc/kantzgruppe.html.Thomas Schreiber,born1963,did his diploma work with Peter Grassberger atWuppertal University on phase transitions and information transport in spatio-temporal chaos.He joined the chaos group of Predrag Cvitanovi´c at the Niels BohrInstitute in Copenhagen to study periodic orbit theory of diffusion and anomaloustransport.There he also developed a strong interest in real-world applications ofchaos theory,leading to his Ph.D.thesis on nonlinear time series analysis(Univer-sity of Wuppertal,1994).As a research assistant at Wuppertal University and duringseveral extended appointments at the Max Planck Institute for the Physics of Com-plex Systems in Dresden he published numerous research articles on time seriesmethods and applications ranging from physiology to the stock market.His habil-itation thesis(University of Wuppertal)appeared as a review in Physics Reportsin1999.Thomas Schreiber has extensive experience teaching nonlinear dynamicsto students and experts from variousfields and at all levels.Recently,he has leftacademia to undertake industrial research.NONLINEAR TIME SERIES ANALYSIS HOLGER KANTZ AND THOMAS SCHREIBERMax Planck Institute for the Physics of Complex Systems,DresdenMore informationMore informationpublished by the press syndicate of the university of cambridgeThe Pitt Building,Trumpington Street,Cambridge,United Kingdomcambridge university pressThe Edinburgh Building,Cambridge CB22RU,UK40West20th Street,New York,NY10011–4211,USA477Williamstown Road,Port Melbourne,VIC3207,AustraliaRuiz de Alarc´o n13,28014Madrid,SpainDock House,The Waterfront,Cape Town8001,South AfricaC Holger Kantz and Thomas Schreiber,2000,2003This book is in copyright.Subject to statutory exceptionand to the provisions of relevant collective licensing agreements,no reproduction of any part may take place withoutthe written permission of Cambridge University Press.First published2000Second edition published2003Printed in the United Kingdom at the University Press,CambridgeTypeface Times11/14pt.System L A T E X2ε[tb]A catalogue record for this book is available from the British LibraryLibrary of Congress Cataloguing in Publication dataKantz,Holger,1960–Nonlinear time series analysis/Holger Kantz and Thomas Schreiber.–[2nd ed.].p.cm.Includes bibliographical references and index.ISBN0521821509–ISBN0521529026(paperback)1.Time-series analysis.2.Nonlinear theories.I.Schreiber,Thomas,1963–II.TitleQA280.K3552003519.5 5–dc212003044031ISBN0521821509hardbackISBN0521529026paperbackThe publisher has used its best endeavours to ensure that the URLs for external websites referred to in this bookare correct and active at the time of going to press.However,the publisher has no responsibility for the websites and can make no guarantee that a site will remain live or that the content is or will remain appropriate.More informationContentsPreface to thefirst edition page xiPreface to the second edition xiiiAcknowledgements xvI Basic topics11Introduction:why nonlinear methods?32Linear tools and general considerations132.1Stationarity and sampling132.2Testing for stationarity152.3Linear correlations and the power spectrum182.3.1Stationarity and the low-frequency component in thepower spectrum232.4Linearfilters242.5Linear predictions273Phase space methods303.1Determinism:uniqueness in phase space303.2Delay reconstruction353.3Finding a good embedding363.3.1False neighbours373.3.2The time lag393.4Visual inspection of data393.5Poincar´e surface of section413.6Recurrence plots434Determinism and predictability484.1Sources of predictability484.2Simple nonlinear prediction algorithm504.3Verification of successful prediction534.4Cross-prediction errors:probing stationarity564.5Simple nonlinear noise reduction58vMore informationvi Contents5Instability:Lyapunov exponents655.1Sensitive dependence on initial conditions655.2Exponential divergence665.3Measuring the maximal exponent from data696Self-similarity:dimensions756.1Attractor geometry and fractals756.2Correlation dimension776.3Correlation sum from a time series786.4Interpretation and pitfalls826.5Temporal correlations,non-stationarity,and space timeseparation plots876.6Practical considerations916.7A useful application:determination of the noise level using thecorrelation integral926.8Multi-scale or self-similar signals956.8.1Scaling laws966.8.2Detrendedfluctuation analysis1007Using nonlinear methods when determinism is weak1057.1Testing for nonlinearity with surrogate data1077.1.1The null hypothesis1097.1.2How to make surrogate data sets1107.1.3Which statistics to use1137.1.4What can go wrong1157.1.5What we have learned1177.2Nonlinear statistics for system discrimination1187.3Extracting qualitative information from a time series1218Selected nonlinear phenomena1268.1Robustness and limit cycles1268.2Coexistence of attractors1288.3Transients1288.4Intermittency1298.5Structural stability1338.6Bifurcations1358.7Quasi-periodicity139II Advanced topics1419Advanced embedding methods1439.1Embedding theorems1439.1.1Whitney’s embedding theorem1449.1.2Takens’s delay embedding theorem1469.2The time lag148More informationContents vii9.3Filtered delay embeddings1529.3.1Derivative coordinates1529.3.2Principal component analysis1549.4Fluctuating time intervals1589.5Multichannel measurements1599.5.1Equivalent variables at different positions1609.5.2Variables with different physical meanings1619.5.3Distributed systems1619.6Embedding of interspike intervals1629.7High dimensional chaos and the limitations of the time delayembedding1659.8Embedding for systems with time delayed feedback17110Chaotic data and noise17410.1Measurement noise and dynamical noise17410.2Effects of noise17510.3Nonlinear noise reduction17810.3.1Noise reduction by gradient descent17910.3.2Local projective noise reduction18010.3.3Implementation of locally projective noise reduction18310.3.4How much noise is taken out?18610.3.5Consistency tests19110.4An application:foetal ECG extraction19311More about invariant quantities19711.1Ergodicity and strange attractors19711.2Lyapunov exponents II19911.2.1The spectrum of Lyapunov exponents and invariantmanifolds20011.2.2Flows versus maps20211.2.3Tangent space method20311.2.4Spurious exponents20511.2.5Almost two dimensionalflows21111.3Dimensions II21211.3.1Generalised dimensions,multi-fractals21311.3.2Information dimension from a time series21511.4Entropies21711.4.1Chaos and theflow of information21711.4.2Entropies of a static distribution21811.4.3The Kolmogorov–Sinai entropy22011.4.4The -entropy per unit time22211.4.5Entropies from time series data226More informationviii Contents11.5How things are related22911.5.1Pesin’s identity22911.5.2Kaplan–Yorke conjecture23112Modelling and forecasting23412.1Linear stochastic models andfilters23612.1.1Linearfilters23712.1.2Nonlinearfilters23912.2Deterministic dynamics24012.3Local methods in phase space24112.3.1Almost model free methods24112.3.2Local linearfits24212.4Global nonlinear models24412.4.1Polynomials24412.4.2Radial basis functions24512.4.3Neural networks24612.4.4What to do in practice24812.5Improved cost functions24912.5.1Overfitting and model costs24912.5.2The errors-in-variables problem25112.5.3Modelling versus prediction25312.6Model verification25312.7Nonlinear stochastic processes from data25612.7.1Fokker–Planck equations from data25712.7.2Markov chains in embedding space25912.7.3No embedding theorem for Markov chains26012.7.4Predictions for Markov chain data26112.7.5Modelling Markov chain data26212.7.6Choosing embedding parameters for Markov chains26312.7.7Application:prediction of surface wind velocities26412.8Predicting prediction errors26712.8.1Predictability map26712.8.2Individual error prediction26812.9Multi-step predictions versus iterated one-step predictions27113Non-stationary signals27513.1Detecting non-stationarity27613.1.1Making non-stationary data stationary27913.2Over-embedding28013.2.1Deterministic systems with parameter drift28013.2.2Markov chain with parameter drift28113.2.3Data analysis in over-embedding spaces283More informationContents ix13.2.4Application:noise reduction for human voice28613.3Parameter spaces from data28814Coupling and synchronisation of nonlinear systems29214.1Measures for interdependence29214.2Transfer entropy29714.3Synchronisation29915Chaos control30415.1Unstable periodic orbits and their invariant manifolds30615.1.1Locating periodic orbits30615.1.2Stable/unstable manifolds from data31215.2OGY-control and derivates31315.3Variants of OGY-control31615.4Delayed feedback31715.5Tracking31815.6Related aspects319A Using the TISEAN programs321A.1Information relevant to most of the routines322A.1.1Efficient neighbour searching322A.1.2Re-occurring command options325A.2Second-order statistics and linear models326A.3Phase space tools327A.4Prediction and modelling329A.4.1Locally constant predictor329A.4.2Locally linear prediction329A.4.3Global nonlinear models330A.5Lyapunov exponents331A.6Dimensions and entropies331A.6.1The correlation sum331A.6.2Information dimension,fixed mass algorithm332A.6.3Entropies333A.7Surrogate data and test statistics334A.8Noise reduction335A.9Finding unstable periodic orbits336A.10Multivariate data336B Description of the experimental data sets338B.1Lorenz-like chaos in an NH3laser338B.2Chaos in a periodically modulated NMR laser340B.3Vibrating string342B.4Taylor–Couetteflow342B.5Multichannel physiological data343More informationx ContentsB.6Heart rate during atrialfibrillation343B.7Human electrocardiogram(ECG)344B.8Phonation data345B.9Postural control data345B.10Autonomous CO2laser with feedback345B.11Nonlinear electric resonance circuit346B.12Frequency doubling solid state laser348B.13Surface wind velocities349References350Index365More informationPreface to thefirst editionThe paradigm of deterministic chaos has influenced thinking in manyfields of sci-ence.As mathematical objects,chaotic systems show rich and surprising structures.Most appealing for researchers in the applied sciences is the fact that determinis-tic chaos provides a striking explanation for irregular behaviour and anomalies insystems which do not seem to be inherently stochastic.The most direct link between chaos theory and the real world is the analysis oftime series from real systems in terms of nonlinear dynamics.On the one hand,experimental technique and data analysis have seen such dramatic progress that,by now,most fundamental properties of nonlinear dynamical systems have beenobserved in the laboratory.On the other hand,great efforts are being made to exploitideas from chaos theory in cases where the system is not necessarily deterministicbut the data displays more structure than can be captured by traditional methods.Problems of this kind are typical in biology and physiology but also in geophysics,economics,and many other sciences.In all thesefields,even simple models,be they microscopic or phenomenological,can create extremely complicated dynamics.How can one verify that one’s model isa good counterpart to the equally complicated signal that one receives from nature?Very often,good models are lacking and one has to study the system just from theobservations made in a single time series,which is the case for most non-laboratorysystems in particular.The theory of nonlinear dynamical systems provides new toolsand quantities for the characterisation of irregular time series data.The scope ofthese methods ranges from invariants such as Lyapunov exponents and dimensionswhich yield an accurate description of the structure of a system(provided thedata are of high quality)to statistical techniques which allow for classification anddiagnosis even in situations where determinism is almost lacking.This book provides the experimental researcher in nonlinear dynamics with meth-ods for processing,enhancing,and analysing the measured signals.The theorist willbe offered discussions about the practical applicability of mathematical results.ThexiMore informationxii Preface to thefirst editiontime series analyst in economics,meteorology,and otherfields willfind inspira-tion for the development of new prediction algorithms.Some of the techniquespresented here have also been considered as possible diagnostic tools in clinical re-search.We will adopt a critical but constructive point of view,pointing out ways ofobtaining more meaningful results with limited data.We hope that everybody whohas a time series problem which cannot be solved by traditional,linear methodswillfind inspiring material in this book.Dresden and WuppertalNovember1996More informationPreface to the second editionIn afield as dynamic as nonlinear science,new ideas,methods and experimentsemerge constantly and the focus of interest shifts accordingly.There is a continuousstream of new results,and existing knowledge is seen from a different angle aftervery few years.Five years after thefirst edition of“Nonlinear Time Series Analysis”we feel that thefield has matured in a way that deserves being reflected in a secondedition.The modification that is most immediately visible is that the program listingshave been be replaced by a thorough discussion of the publicly available softwareTISEAN.Already a few months after thefirst edition appeared,it became clearthat most users would need something more convenient to use than the bare libraryroutines printed in the book.Thus,together with Rainer Hegger we prepared stand-alone routines based on the book but with input/output functionality and advancedfeatures.Thefirst public release was made available in1998and subsequent releasesare in widespread use now.Today,TISEAN is a mature piece of software thatcovers much more than the programs we gave in thefirst edition.Now,readerscan immediately apply most methods studied in the book on their own data usingTISEAN programs.By replacing the somewhat terse program listings by minuteinstructions of the proper use of the TISEAN routines,the link between book andsoftware is strengthened,supposedly to the benefit of the readers and users.Hencewe recommend a download and installation of the package,such that the exercisescan be readily done by help of these ready-to-use routines.The current edition has be extended in view of enlarging the class of data sets to betreated.The core idea of phase space reconstruction was inspired by the analysis ofdeterministic chaotic data.In contrast to many expectations,purely deterministicand low-dimensional data are rare,and most data fromfield measurements areevidently of different nature.Hence,it was an effort of our scientific work over thepast years,and it was a guiding concept for the revision of this book,to explore thepossibilities to treat other than purely deterministic data sets.xiiiMore informationxiv Preface to the second editionThere is a whole new chapter on non-stationary time series.While detectingnon-stationarity is still briefly discussed early on in the book,methods to deal withmanifestly non-stationary sequences are described in some detail in the secondpart.As an illustration,a data source of lasting interest,human speech,is used.Also,a new chapter deals with concepts of synchrony between systems,linear andnonlinear correlations,information transfer,and phase synchronisation.Recent attempts on modelling nonlinear stochastic processes are discussed inChapter12.The theoretical framework forfitting Fokker–Planck equations to datawill be reviewed and evaluated.While Chapter9presents some progress that hasbeen made in modelling input–output systems with stochastic but observed inputand on the embedding of time delayed feedback systems,the chapter on mod-elling considers a data driven phase space approach towards Markov chains.Windspeed measurements are used as data which are best considered to be of nonlinearstochastic nature despite the fact that a physically adequate mathematical model isthe deterministic Navier–Stokes equation.In the chapter on invariant quantities,new material on entropy has been included,mainly on the -and continuous entropies.Estimation problems for stochastic ver-sus deterministic data and data with multiple length and time scales are discussed.Since more and more experiments now yield good multivariate data,alternativesto time delay embedding using multiple probe measurements are considered at var-ious places in the text.This new development is also reflected in the functionalityof the TISEAN programs.A new multivariate data set from a nonlinear semicon-ductor electronic circuit is introduced and used in several places.In particular,adifferential equation has been successfully established for this system by analysingthe data set.Among other smaller rearrangements,the material from the former chapter“Other selected topics”,has been relocated to places in the text where a connectioncan be made more naturally.High dimensional and spatio-temporal data is now dis-cussed in the context of embedding.We discuss multi-scale and self-similar signalsnow in a more appropriate way right after fractal sets,and include recent techniquesto analyse power law correlations,for example detrendedfluctuation analysis.Of course,many new publications have appeared since1997which are potentiallyrelevant to the scope of this book.At least two new monographs are concerned withthe same topic and a number of review articles.The bibliography has been updatedbut remains a selection not unaffected by personal preferences.We hope that the extended book will prove its usefulness in many applicationsof the methods and further stimulate thefield of time series analysis.DresdenDecember2002More informationAcknowledgementsIf there is any feature of this book that we are proud of,it is the fact that almost allthe methods are illustrated with real,experimental data.However,this is anythingbut our own achievement–we exploited other people’s work.Thus we are deeplyindebted to the experimental groups who supplied data sets and granted permissionto use them in this book.The production of every one of these data sets requiredskills,experience,and equipment that we ourselves do not have,not forgetting thehours and hours of work spent in the laboratory.We appreciate the generosity ofthe following experimental groups:NMR laser.Our contact persons at the Institute for Physics at Z¨u rich University were Leci Flepp and Joe Simonet;the head of the experimental group is E.Brun.(See AppendixB.2.)Vibrating string.Data were provided by Tim Molteno and Nick Tufillaro,Otago University, Dunedin,New Zealand.(See Appendix B.3.)Taylor–Couetteflow.The experiment was carried out at the Institute for Applied Physics at Kiel University by Thorsten Buzug and Gerd Pfister.(See Appendix B.4.) Atrialfibrillation.This data set is taken from the MIT-BIH Arrhythmia Database,collected by G.B.Moody and R.Mark at Beth Israel Hospital in Boston.(See Appendix B.6.) Human ECG.The ECG recordings we used were taken by Petr Saparin at Saratov State University.(See Appendix B.7.)Foetal ECG.We used noninvasively recorded(human)foetal ECGs taken by John F.Hofmeister as the Department of Obstetrics and Gynecology,University of Colorado,Denver CO.(See Appendix B.7.)Phonation data.This data set was made available by Hanspeter Herzel at the Technical University in Berlin.(See Appendix B.8.)Human posture data.The time series was provided by Steven Boker and Bennett Bertenthal at the Department of Psychology,University of Virginia,Charlottesville V A.(SeeAppendix B.9.)xvMore informationxvi AcknowledgementsAutonomous CO2laser with feedback.The data were taken by Riccardo Meucci and Marco Ciofini at the INO in Firenze,Italy.(See Appendix B.10.)Nonlinear electric resonance circuit.The experiment was designed and operated by M.Diestelhorst at the University of Halle,Germany.(See Appendix B.11.)Nd:YAG laser.The data we use were recorded in the University of Oldenburg,where we wish to thank Achim Kittel,Falk Lange,Tobias Letz,and J¨u rgen Parisi.(See AppendixB.12.)We used the following data sets published for the Santa Fe Institute Time SeriesContest,which was organised by Neil Gershenfeld and Andreas Weigend in1991:NH3laser.We used data set A and its continuation,which was published after the contest was closed.The data was supplied by U.H¨u bner,N.B.Abraham,and C.O.Weiss.(SeeAppendix B.1.)Human breath rate.The data we used is part of data set B of the contest.It was submitted by Ari Goldberger and coworkers.(See Appendix B.5.)During the composition of the text we asked various people to read all or part of themanuscript.The responses ranged from general encouragement to detailed technicalcomments.In particular we thank Peter Grassberger,James Theiler,Daniel Kaplan,Ulrich Parlitz,and Martin Wiesenfeld for their helpful remarks.Members of ourresearch groups who either contributed by joint work to our experience and knowl-edge or who volunteered to check the correctness of the text are Rainer Hegger,Andreas Schmitz,Marcus Richter,Mario Ragwitz,Frank Schm¨u ser,RathinaswamyBhavanan Govindan,and Sharon Sessions.We have also considerably profited fromcomments and remarks of the readers of thefirst edition of the book.Their effortin writing to us is gratefully appreciated.Last but not least we acknowledge the encouragement and support by SimonCapelin from Cambridge University Press and the excellent help in questions ofstyle and English grammar by Sheila Shepherd.。
简单不俗气好听英文名最新版的

简单不俗气好听英文名最新版的英文已经成为了一种潮流,越来越多人喜欢给自己取英文名,那么好听不俗气的英文名都有哪些呢?下面店铺为大带来好听的英文名不俗气简单,供你收藏。
这么多的英文名之中,有哪些是不俗气,又简单易记还好听的?以下是店铺给大家带来简洁简单不俗气最新英文名,以供参阅。
简单的英文名1. Bernie,伯尼,条顿,像熊一般勇敢。
2. Bert,伯特,英国,光辉灿烂;全身散发出荣耀和光辉的人。
3. Berton,伯顿,英国,勤俭治产之人。
4. Chad,查德,英国,有经验的战士。
5. Channing,强尼,法国,牧师。
6. Chapman 契布曼英国,商人;小贩。
7. Charles 查理斯拉丁-条顿,强壮的,男性的,高贵心灵,强健的。
8. Chasel,夏佐,古法国猎人。
9. Chester 贾斯特罗马,小镇10. Christ,克莱斯特,希伯来基督。
11. Christian,克里斯汀,希腊,基督的追随者,信徒。
12. Christopher,克里斯多夫,希腊,基督的信差或仆人,表之意。
13. Dean,迪恩,英国,山谷;学校的领导者;教堂的领导者。
14. Don,唐,塞尔特世界领袖。
15. Donahue 唐纳修爱尔兰红褐色的战士。
16. Donald,唐纳德塞尔特世界领袖;酋长。
17. Douglas 道格拉斯,盖尔,来自黑海的人;深灰色。
18. Reuben,鲁宾,希腊,一个儿子!新生者。
19. Rex,雷克斯拉丁,国王。
20. Richard 理查,德国,勇猛的,大胆的。
21. Robert,罗伯特条顿,辉煌的名声。
22. Robin,罗宾,条顿,辉煌的名声,知更鸟。
23. Rock,洛克,英国,岩石,非常强壮之人。
24. Rod,罗德,英国,公路服务者;有名气的。
25. Roderick,罗得里克,英国,很有名气;很出名的领导者。
26. Eric,艾利克斯堪的那维亚,领导者。
【能力】企业能力静态能力与动态能力理论界定及关系辨析

【关键字】能力企业能力:静态能力与动态能力理论界定及关系辨析黄培伦尚航标中国广州华南理工大学工商管理学院摘要:企业能力是企业的组织能力,按静态的观点称之为静态能力,按动态的观点称之为动态能力。
企业能力是静态能力与动态能力的统一,以静态能力为基础、动态能力为主导。
企业的静态能力集中表现为企业实力,企业的动态能力集中表现为企业活力。
静态能力和动态能力是企业能力的不同组成部分。
根据现有的文献分析,阐明企业能力、静态能力和动态能力的概念。
并且对静态能力与动态能力的关系进行辨析,认为动态能力是对静态能力的一种扬弃。
根据文中的研究,提出动态能力的研究模型。
此外,也探讨对未来研究的建议和在管理方面的含义。
关键词:企业能力;静态能力;动态能力引言学者对于“企业如何获取并保持其比赛优势”有许多不同的探讨,但大体上可以分为三类:比赛力量理论、战略冲突理论和资源基础理论。
这三个经典理论在基于静态的假设上解释企业是如何获取并保持比赛优势的(Teece, Pisano, and Shuen 1997)。
但,自上世纪九十年代以来,比赛的白炽化和环境的急剧动荡对这些忽视市场动态的理论提出挑战,使企业不断的否定其现有的资源和能力,所以要以动态的观点来审视企业的资源和能力,这促使动态能力理论的产生。
虽然对于动态能力的研究被视作最具发展前景的战略流派之一,但是由于动态能力的研究基础比较薄弱,在这个研究领域出现一些争论,甚至有学者提出“动态能力的概念和理论有价值么?”(鄢德春,2007)。
为什么会出现这种现象?显然这些学者把动态能力和企业静态能力对立起来。
本文的意图:(1)通过简要的描述关于企业能力的演化轨迹,明确企业静态能力与动态能力的理论界定;(2)明确企业静态能力、动态能力是企业能力的两个不同的层面,对于成功企业来说,二者缺一不可;(3)比较动态能力与静态能力的关系,并在此基础上提出一个包含动态能力逻辑推力的研究框架;(4)拟为下一步的研究提供理论支持和理论引导。
- 1、下载文档前请自行甄别文档内容的完整性,平台不提供额外的编辑、内容补充、找答案等附加服务。
- 2、"仅部分预览"的文档,不可在线预览部分如存在完整性等问题,可反馈申请退款(可完整预览的文档不适用该条件!)。
- 3、如文档侵犯您的权益,请联系客服反馈,我们会尽快为您处理(人工客服工作时间:9:00-18:30)。
Prototype Implementations of an Architectural Model for Service-Based Flexible SoftwareKeith Bennett, Malcolm Munro,Jie Xu Dept.of Computer Science University of Durham,UK keith.bennett@ Nicolas Gold,Paul LayzellNikolay MehandjievDepartment of ComputationUMIST,UKn.mehandjiev@David BudgenPearl BreretonDept.of Computer ScienceKeele University,UKdb@AbstractThe need to change software easily to meet evolving business requirements is urgent,and a radical shift is required in the development of software,with a more demand-centric view leading to software which will be delivered as a service,within the framework of an open marketplace.We describe a service architecture and its rationale,in which components may be bound instantly,just at the time they are needed and then the binding may be disengaged. This allows highly flexible software services to be evolved in“Internet time”.The paper focuses on early results: some of the aims have been demonstrated and amplified through two experimental implementations,enabling us to assess the strengths and weakness of the approach.It is concluded that some of the key underpinning concepts–discovery and late binding–are viable and demonstrate the basic feasibility of the architecture.1.ObjectivesContemporary organisations must be in a constant state of evolution if they are to compete and survive in an increasingly global and rapidly changing marketplace. They operate in a time-critical environment,rather than a safety critical application domain.If a change or enhancement to software is not brought to market sufficiently quickly,thus retaining competitive advantage, the organisation may collapse.This poses significantly new problems for software development,characterised by a shift in emphasis from producing‘a system’to the need to produce‘a family of systems’,with each system being an evolution from a previous version,developed and deployed in ever shorter business cycles.It may be that the released new version is not complete,and still has errors.If the product succeeds,it can be put on an “emergency life support”to resolve these.If it misses the market time slot,it probably will not succeed at all.It is possible to inspect each activity of the software evolution process and determine how it may be speeded up.Certainly,new technology to automate some parts (e.g.program comprehension,testing)may be expected. However,it is very difficult to see that such improvements will lead to a radical reduction in the time to evolve a large software system.This prompted us to believe that a new and different way is needed to achieve ultra rapid evolution;we term this“evolution in Internet time”.It is important to stress that such ultra rapid evolution does not imply poor quality,or software which is simply hacked together without thought.The real challenge is to achieve very fast change yet provide very high quality software. Strategically,we plan to achieve this by bringing the evolution process much closer to the business process.In1995,British Telecommunications plc(BT) recognised the need to undertake long-term research leading to different,and possibly radical,ways in which to develop software for the future.Senior academics from UMIST,Keele University and the University of Durham came together with staff at BT to form DiCE(The Distributed Centre of Excellence in Software Engineering).This work established the foundations for the research described here,and its main outcomes are summarised in Section2of the paper.From1998,the core group of researchers switched to developing a new overall paradigm for software engineering:a service-based approach to structuring, developing and deploying software.This new approach is described in the second half of this paper.In Section3,we express the objectives of the current phase of research in terms of the vision for software-how it will behave,be structured and developed in the future. In Section4,we describe two prototype implementationsof the service architecture,demonstrating its feasibility and enabling us to elucidate research priorities.In addition,we are exploring technologies in order to createa distributed laboratory for software service experiments.2.Developing a future visionThe method by which the DiCE group undertook its research is described in[2].Basically,the group formulated three questions about the future of software: How will software be used?How will software behave? How will software be developed?In answering these questions,a number of key issues emerged.K1.Software will need to be developed to meet necessary and sufficient requirements,i.e.for the majority of users whilst there will be a minimum set of requirements software must meet,over-engineered systems with redundant functionality are not required.K2.Software will be personalised.Software will be capable of personalisation,providing users with their own tailored,unique working environment which is best suited to their personal needs and working styles,thus meeting the goal of software which will meet necessary and sufficient requirements.K3.Software will be self-adapting.Software will contain reflective processes which monitor and understand how it is being used and will identify and implement ways in which it can change in order to better meet user requirements,interface styles and patterns of working.K4.Software will be fine-grained.Future software will be structured in small simple units which co-operate through rich communication structures and information gathering.This will provide a high degree of resilience against failure in part of the software network and allow software to re-negotiate use of alternatives in order to facilitate self-adaptation and personalisation.K5.Software will operate in a transparent manner. Software may continue to be seen as a single abstract object even when distributed across different platforms and geographical locations.This is an essential property if software is to be able to reconfigure itself and substitute one component or network of components for another without user or professional intervention.Although rapid evolution is just one of these five needs,it clearly interacts strongly with the other demands, and hence a solution which had the potential to address all the above factors was sought.3.Service-based software3.1.The problemMost software engineering techniques,including those of software maintenance,are conventional supply-side methods,driven by technological advance.This works well for systems with rigid boundaries of concern such as embedded systems.It breaks down for applications where system boundaries are not fixed and are subject to constant urgent change.These applications are typically found in emergent organisations-“organisations in a state of continual process change,never arriving,always in transition”[4].Examples are e-businesses or more traditional companies which continually need to reinvent themselves to gain competitive advantage[5].These applications are,in Lehman’s terms,“E-type”[7];the introduction of software into an organisation changes the work practices of that organisation,so the original requirements of the software change.It is not viable to identify a closed set of requirements;these will be forever changing and many will be tacit.We concluded that a“silver bullet”,which would somehow transform software into something which could be changed far more quickly than at present,was not viable.Instead,we took the view that software is actually hard to change,and this takes time to accomplish.We needed to look for other solutions.Subsequent research by DiCE has taken a demand-led approach to the provision of software services,addressing delivery mechanisms and processes which,when embedded in emergent organisations,give a software solution in emergent terms-one with continual change. The solution never ends and neither does the provision of software.This is most accurately termed engineering for emergent solutions.3.2.Service-based approach to software evolutionCurrently,almost all commercial software is sold on the basis of ownership(we exclude free software and open source software).Thus an organisation buys the object code,with some form of license to use it.Any updates, however important to the purchaser,are the responsibility of the vendor.Any attempt by the user to modify the software is likely to invalidate warranties as well as ongoing support.In effect,the software is a“black box”that cannot be altered in any way,apart from built-in parameterization.This form of marketing(known as supply-led)applies whether the software is run on the client machine or on a remote server.A similar situation can arise whether the user takes on responsibility for in-house support or uses an applications service provider.Inthe latter case there is still a“black box”software,which is developed and maintained in the traditional manner,it is just owned by the applications service provider rather than by the business user.Let us now consider a very different scenario.We see the support provided by our software system as structured into a large number of small functional units,each supporting a purposeful human activity or a business transaction(see K1,K4,K5above).There are no unnecessary units,and each unit provides exactly the necessary support and no more.Suppose now that an activity or a transaction changes,or a new one is introduced.We will now require a new or improved functional unit for this activity.The traditional approach would be to raise a change request with the vendor of the software system,and wait for several months for this to be (possibly)implemented,and the modified unit integrated.In our solution,the new functional unit is procured by the use of an open market mechanism at the moment we specify the change in our needs.At this moment the obsolete unit is disengaged and the new unit is integrated with the rest of the system automatically.In such a solution,we no longer have an ownership of the software product which provides all the required units of support functionality.The software is now owned by the producer of each functional unit.Instead of product owners,we are now consumers of a service,which consists of us being provided with the functionality of each unit when we need it.We can thus refer to each functional unit as a software service.Of course,this vision assumes that the marketplace can provide the desired software services at the point of demand.However,it is a well-established property of marketplaces that they can spot trends,and make new products available when they are needed.The rewards for doing so are very strong and the penalties for not doing so are severe.Note that any particular software supplier of software services can either assemble their services out of existing ones,or develop and evolve atomic services using traditional software development techniques.The new dimension is that these services are sold and assembled within a demand-led marketplace.Therefore,if we can find ways to disengage an existing service and bind in a new one(with enhanced functionality and other attributes) dynamically at the point of request for execution,we have the potential to achieve ultra-rapid evolution in the target system.These ideas led us to conclude that the fundamental problem with slow evolution was a result of software that is marketed as a product in a supply-led marketplace.By removing the concept of ownership,we have instead a service i.e.something that is used,not owned.Thus we widened the traditional component-based solution to the much more generic service-based software in a demand-led marketplace.This service-based model of software is one in which services are configured to meet a specific set of requirements at a point in time,executed and then disengaged-the vision of instant service,conforming to the widely accepted definition of a service:“an act or performance offered by one party to another. Although the process may be tied to a physical product, the performance is essentially intangible and does not normally result in ownership of any of the factors of production”[6].Services are composed out of smaller ones(and so on recursively),procured and paid for on demand.An analogy is the service of organising weddings or business travel:in both cases customers configure their service for each individual occasion from a number of sub-services, where each sub-service can be further customised or decomposed recursively.This strategy enables users to create,compose and assemble a service by bringing together a number of suppliers to meet needs at a specific point in time.parison with existing approaches tobuilding flexible softwareSoftware vendors attempt to offer a similar level of flexibility by offering products such as SAP,which is composed out of a number of configurable modules and options.This,however,offers extremely limited flexibility,where consumers are not free to substitute functions and modules with those from another supplier, because the software is subject to vendor-specific binding which configures and links the component parts,making it very difficult to perform substitution.Component-based software development[11]aims to create platform-independent component integration frameworks,which provide standard interfaces and thus enable flexible binding of encapsulated software ponent reuse and alignment between components and business concepts are often seen as major enablers of agile support for e-business[14].Component marketplaces are now appearing,bringing our vision of marketplace-enabled software procurement closer to reality.They,however,tend to be organised along the lines of supply push rather than demand pull.Even more significant difference from our approach is that the assembly and integration(binding)of marketplace-procured components are still very much part of the human-performed activity of developing a software product,rather than a part of the automatic process of fulfilling user needs as soon as they are specified.Current work in Web services does bring binding closer to execution,allowing an application or user to find andexecute business and information services,such as airline reservations and credit card validations.Web services platforms such as HP’s e-Speak[8]and IBM Web Services Toolkit[12]provide some basic mechanisms and standards such as the Universal Description,Discovery, and Integration(UDDI)that can be used for describing, publishing,discovering and invoking business-oriented Web services in a dynamic distributed environment.We have found e-Speak to be a useful platform for building our second prototype as discussed further down. However,work on Web services is technology-focused and fails to consider the interdisciplinary aspects of service provision such as marketplace organization and trading contracts.3.4.Novel aspects of our approachThe aim of our research is to develop the technology which will enable ultra-late binding,that is the delay of binding until the execution of a system.This will enable consumers to obtain the most appropriate and up-to-date combination of services required at any point in time.Our approach to service-based software provision seeks to change the nature of software from product to service provision.To meet users’needs of evolution, flexibility and personalisation,an open market-place framework is necessary in which the most appropriate versions of software products come together,are bound and executed as and when needed.At the extreme,the binding that takes place just prior to execution is disengaged immediately after execution in order to permit the‘system’to evolve for the next point of execution. Flexibility and personalisation are achieved through a variety of service providers offering functionality through a competitive market-place,with each software provision being accompanied by explicit properties of concern for binding(e.g.dependability,performance,quality,license details etc).Such ultra-late binding,however,comes at a price,and for many consumers,issues of reliability,security,cost and convenience may mean that they prefer to enter into contractual agreements where they have some early binding for critical or stable parts of a system,leaving more volatile functions to late binding and thereby maximising competitive advantage.The consequence is that any such approach to software development must be interdisciplinary so that non-technical issues,such as supply contracts,terms and conditions,and error recovery are addressed and built in to the new technology.We have established the Interdisciplinary Software Engineering Network(ISEN),which will seek the input of a experts from law,marketing,engineering and other cognate disciplines.The network is funded by the UK’s Engineering and Physical Sciences Research Council (EPSRC)and further details can be found at our WWW site These non-technical issues will have a central role in the specification of a service,unlike a software component,which is simply a reusable software executable.Our serviceware clearly includes the software itself,but in addition has many non-functional attributes, such as cost and payment,trust,brand allegiance,legal status and redress,security and so on.The idea of ultra-late binding requires us to negotiate across all such attributes(as far as possibly electronically)to establish optimal combinations of service components just before execution.This is seen as imperative in a business to business e-commerce environment.3.5.Architecture for service-based softwaredeliveryThe theoretical model behind our architecture is shown on Figure1.In this architecture we have three major groups of Service Providers:Information service providers(ISPs):those that provide information to other services e.g.catalogue and ontology services.Contractor service providers(CSPs):those that have the ability to negotiate and assemble the necessary components/services to deliver a service to the end-user.Software service providers(SSPs):those software vendors that provide either the operational software components/services themselves,or descriptions of the components required and how they should be assembled.SSPs register services in an electronic service marketplace,using ISPs.A service is a named entity providing either(a)operational functionality,in which case its vendor is a Component Provider,or(b)a composition template,in which case its vendor is a Solution Provider(see detailed explanation further down).A service consumer,which may be the end user,will specify a desired service functionality.A Contractor Service Provider(CSP),which acts as a broker to represent the user interests at the marketplace,will then search the marketplace for a suitable service through a discovery process involving ISPs.Assuming such a service exists(i.e.a match can be made),the service interface is passed to the CSP,which is responsible(again on the fly)for satisfying the user needs with the service found.This will either involve interpreting this service’s composition template and recursively searching for the sub-services specified there,or using the atomic service that actually delivers a result.The CSP/broker will discover and use the most appropriate sub-services that meet the composition criteria at the time of need.This may involve negotiation of non-service composition(the design activity)is not undertaken by the client or user,but the templates are supplied by SSPs in the marketplace.It can be seen that this architectural model offers a dynamic composition of services at the instant of need.Of course this raises the question of a service request for which there is no offering in the marketplace.Although in the long term there may be technological help for automatic composition(ing reflection),currently we see this as a market failure;where the market has been unable to provide the needs of a purchaser.It is important to distinguish binding and service composition.The design of a composition is a highly skilled task which is not yet automatable,and there is no attempt at“on the fly”production of designs.However, we can foresee the use of variants or design patterns in the future.We call this design a composition template.Once it exists,we can populate the composition template with services from the marketplace which will fulfill the composition.Our architecture offers the possibility of locating and binding such sub-services just before the super-service is executed.The application code is replaced by recursive sub-service invocation.4.Service Implementation–Prototypes andResults4.1.Aims of the prototype implementationThis section describes the objectives of the two experimental systems(referred to as prototypes1and2), the rationale for using the platforms,the results obtained from the implementations,and the conclusions drawn by bringing together the results of both experiments.The general aim of the prototypes was to test ideas about the following:• dynamically bound services at run-time within the flexible software service architecture;• service binding with limited negotiation;• service discovery.To guide the development of our prototype series,we have mapped some of the problems of service-based software delivery into an established transaction model [10].This model characterises a transaction between buyer and seller and provides the four process phases shown in Table1.The activities identified within the phases are drawn both from the model and our own work.Phase Activities Prototypeno. Information Service DescriptionService DiscoveryRequest Construction2Negotiation NegotiateEvaluate1Settlement Service InvocationMonitoringClaim&Redress1,2After-sales Evaluate for futureTable1:Transaction model for software services Our first experimental system had the aim of demonstrating the capability of service binding and limited service negotiation[9].The objectives of the second prototype were to investigate two aspects of the above theoretical model:service discovery,and service binding(see Table2).Prototype Aim Infrastructure1.Calculation Servicebinding&negotiation PHP,MySQL and HTML2.Print service Discovery&bindinge-SpeakTable2:Prototype Aims and Infrastructures 4.2.Prototype applications4.2.1.A calculation service.The first prototype was designed to supply a basic calculation service to an end-user.The particular service selected was the problem of cubing a number.Note that due to the service nature of the architecture,we aim to supply the service of cubing, rather than the product of a calculator with that function in it.This apparently simple application was chosen as it highlights many pertinent issues yet the domain is understood by all.4.2.2.A printing service.The second prototype was a simple client application implemented on the e-Speak platform.The application requests a high-speed printing service with a specified speed requirement.The e-Speak approach allows a single registration and discovery mechanism for both composite and atomic services.This supports our recursive model(Section3.2) for service composition.The key to the implementation is a class,written outside e-Speak,called DGS(Dynamically Generated Service).When a service composition is returned from the discovery process,the DGS interprets it to invoke sub-services.4.3.Experimental infrastructures4.3.1.Prototype1:Calculation.This prototype is implemented using an HTML interface in a Web browser.PHP scripts are used to perform negotiation and servicecomposition by opening URLs to subsidiary scripts.Eachscript contains generic functionality,loading its“personality”from a MySQL database as it starts.Thisallows a single script to be used to represent many serviceproviders.End-user and service provider profiles arestored on the database,which also simulates a simpleservice discovery environment.4.3.2.Prototype2:Printing.We used e-Speak[8]for building this prototype.It offers a comprehensiveinfrastructure for distributed service discovery,mediationand binding for Internet based applications.e-Speak hasthe following advantages as an experimental framework:• A basic name-matching service discovery environment,with an exception mechanism if noservice can be found.• Issues of distribution and location are handled through virtualisation.• It is based on widely used systems such as Java and XML.It also has the following drawbacks:• The dynamic interpretation of composition templates and subsequent binding in our theoreticalmodel need to be implemented outside the core e-Speak system.• The discovery mechanism does not support a more flexible scheme than name matching.• It intercepts all invocations of services and clients, potentially resulting in supplier lock-in fororganizations using the system.4.4.The prototype implementations4.4.1.Calculator prototype.Three main types of entities are involved in service delivery in the prototype:the end-user,an interface,and service providers.The arrows on Figure1show the interactions and relationships between them.The interface(in this case,a Web browser)allows the end-user to(a)specify their needs as shown on Figure 2,and then(b)to interact with the delivered software.It is expected that the interface will be light-weight and perhaps supplied free in a similar manner to today’s Web browsers.Service from the end-user’s point of view is provided using the following basic model:1)The end-user requests a software service.2)The end-user selects a service domain(e.g.calculation).3)The end-user selects a service within the domain(e.g.cube).4)The end-user enters the number they want tocube.5)The end-user receives the result.Apart from the notion of requesting the service of cube rather than the product of calculator,it can be seen that the process of cubing is similar to selecting the function from a menu in a software product.However,the hidden activity for service provision is considerable.Each provision of service is governed by a simple contract.This contains the terms agreed by the service provider and service consumer for the supply of the service.The specific elements of a contract are not prescribed in terms of the general architecture;providers and consumers may add any term they wish to the negotiation.However,for the prototype,three terms are required:1)The law under which the contract is made.2)Minimum performance(represented in theprototype by a single integer).3)Cost(represented by a single integer).In order to negotiate a contract,both end-users and service providers must define profiles that contain acceptable values for contract terms.The profiles also contain policies to govern how these values may be negotiated.The profiles used in the first demonstrator are extremely simple.End-user profiles contain acceptable legal systems for contracts,the minimum service performance required,the maximum acceptable cost,and the percentage of average market cost within which negotiation is possible.Service provider profiles contain acceptable legal systems for contracts,guaranteed performance levels,and the cost of providing the service. Negotiation in the prototype thus becomes a process of ensuring that both parties can agree a legal system and that the service performance meets the minimum required by the end-user.If successful,service providers are picked on the basis of lowest cost.Acceptable costs are determined by taking the mean of all service costs on the network for the service in question and ensuring that the cost of the service offered is less than the mean plus the percentage specified in the end-user profile.It must also be less than the absolute maximum cost.To avoid the overhead of negotiation for basic, common services such as catalogues,it is assumed that external bodies will provide“certificates”which represent fixed cost,fixed performance contracts that do not require negotiation.Both end-user and service provider profiles contain acceptable certificates.Negotiations take place within the following simplified procedure for service provision:• A contractor(assembly)service is selected using negotiation,and the requirements passed to theservice.• The selected contractor service obtains available solution domains from the catalogue services,andthe end-user selects the calculation service domain.• The contractor then retrieves services available within the calculation domain.Again,a list ispresented and the end-user selects cube.• Now the contractor negotiates the supply of a cube solution description from a software serviceprovider.The solution tells the contractor servicewhich other services are required to performcubing and how to compose them.• The contractor then finds potential sub-services, negotiates contracts with them,and composes themfollowing the template.• The user uses the cube service.• Having completed the service provision,the contractor disengages all the sub-services.4.4.2.Printing service prototype.For the client application that requests a printing service,the e-Speak engine first attempts to locate a single printing service onFigure2:Specifying requirements for the calculator prototype service。