Adaptive collocation with application in height system transformation
Autodesk Nastran 2023 参考手册说明书
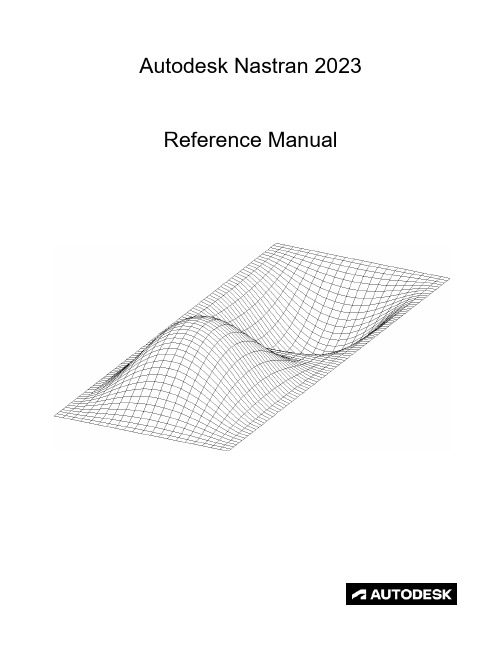
FILESPEC ............................................................................................................................................................ 13
DISPFILE ............................................................................................................................................................. 11
File Management Directives – Output File Specifications: .............................................................................. 5
BULKDATAFILE .................................................................................................................................................... 7
USB Type-C 规范1.2(中文版)
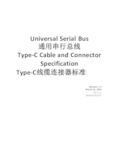
知识产权声明
THIS SPECIFICATION IS PROVIDED TO YOU “AS IS” WITH NO WARRANTIES WHATSOEVER, INCLUDING ANY WARRANTY OF MERCHANTABILITY, NON-INFRINGEMENT, OR FITNESS FOR ANY PARTICULAR PURPOSE. THE AUTHORS OF THIS SPECIFICATION DISCLAIM ALL LIABILITY, INCLUDING LIABILITY FOR INFRINGEMENT OF ANY PROPRIETARY RIGHTS, RELATING TO USE OR IMPLEMENTATION OF INFORMATION IN THIS SPECIFICATION. THE PROVISION OF THIS SPECIFICATION TO YOU DOES NOT PROVIDE YOU WITH ANY LICENSE, EXPRESS OR IMPLIED, BY ESTOPPEL OR OTHERWISE, TO ANY INTELLECTUAL PROPERTY RIGHTS.
预发行行业审查公司提供反馈
Revision History.......................................................................................................................14
LIMITED COPYRIGHT LICENSE: The USB 3.0 Promoters grant a conditional copyright license under the copyrights embodied in the USB Type-C Cable and Connector Specification to use and reproduce the Specification for the sole purpose of, and solely to the extent necessary for, evaluating whether to implement the Specification in products that would comply with the specification.
三星HM1700蓝牙耳机使用说明书
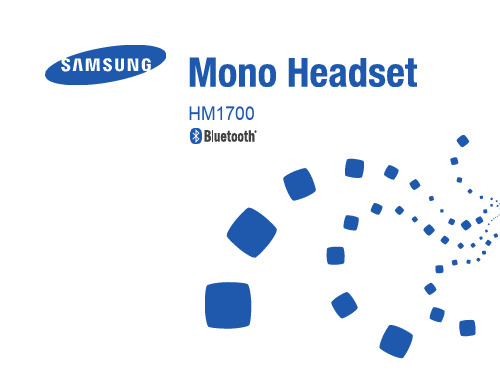
或“2 Devices
或“二设备已连接”
Connected”
“Device Disconnected”
“设备已断开”
“Call Terminated” “通话挂断”
11
配对和连接耳机
配对表示当两个蓝牙设备同意相互通信时,它们之间的唯一且加密的无线连接。 在配对模式中,两台设备应互相靠得足够近。
将耳机与手机配对并连接 1 进入配对模式。
简体中文
• 可用功能和特性可能因手机类型而异。 • 某些设备,尤其是未经 Bluetooth SIG 测试或认可的设备,可能会与您的
耳机不兼容。
若要确保获得最佳的耳机性能 • 保持耳机与设备之间的距离尽可能近,避免您的身体或其他物体挡住信号
通路。 • 若经常使用右手操作手机,请将耳机戴于右耳。 • 遮挡耳机或设备可能影响其性能,因此请尽量减少遮挡。
坏,制造商将不予保修。 • 请避免靠近儿童或动物的眼睛使用设备指示灯。 • 请勿在雷暴天气下使用该设备。雷暴可导致设备工作不正常,并增加触电的危险。
如果长时间以高音量使用耳机进行收听,可能会损害您的听力。建议只使用收听通 话或音乐所需的最低音量。
3
入门指南
耳机概述
话筒 音量按钮
指示灯 通话按钮
听筒
电源开关
耳挂 充电插座
• 确认您的耳机配有下列物品:旅行充电器、耳机、听筒垫、耳挂和用户 手册。
• 随附的物品可能因所在的区域而有所不同。
4
简体中文
按钮功能
按钮
电源 开关
通话
音量键
功能
• 向上或向下滑动可开启或关闭耳机。
• 按住 3 秒可进入配对模式。 • 按下以拨打最近的号码。 • 按住以语音拨号。 • 按下可接听或结束通话。 • 有来电时,按住可拒接来电。 • 按住可在通话中保持通话。 • 按下可调节音量。 • 按住可开启或关闭话筒。
自动化英语专业英语词汇表

自动化英语专业英语词汇表文章摘要:本文介绍了自动化英语专业的一些常用的英语词汇,包括自动化技术、控制理论、系统工程、人工智能、模糊逻辑等方面的专业术语。
本文按照字母顺序,将这些词汇分为26个表格,每个表格包含了以相应字母开头的词汇及其中文释义。
本文旨在帮助自动化专业的学习者和从业者掌握和使用这些专业英语词汇,提高他们的英语水平和专业素养。
A英文中文acceleration transducer加速度传感器acceptance testing验收测试accessibility可及性accumulated error累积误差AC-DC-AC frequency converter交-直-交变频器AC (alternating current) electric drive交流电子传动active attitude stabilization主动姿态稳定actuator驱动器,执行机构adaline线性适应元adaptation layer适应层adaptive telemeter system适应遥测系统adjoint operator伴随算子admissible error容许误差aggregation matrix集结矩阵AHP (analytic hierarchy process)层次分析法amplifying element放大环节analog-digital conversion模数转换annunciator信号器antenna pointing control天线指向控制anti-integral windup抗积分饱卷aperiodic decomposition非周期分解a posteriori estimate后验估计approximate reasoning近似推理a priori estimate先验估计articulated robot关节型机器人assignment problem配置问题,分配问题associative memory model联想记忆模型associatron联想机asymptotic stability渐进稳定性attained pose drift实际位姿漂移B英文中文attitude acquisition姿态捕获AOCS (attritude and orbit control system)姿态轨道控制系统attitude angular velocity姿态角速度attitude disturbance姿态扰动attitude maneuver姿态机动attractor吸引子augment ability可扩充性augmented system增广系统automatic manual station自动-手动操作器automaton自动机autonomous system自治系统backlash characteristics间隙特性base coordinate system基座坐标系Bayes classifier贝叶斯分类器bearing alignment方位对准bellows pressure gauge波纹管压力表benefit-cost analysis收益成本分析bilinear system双线性系统biocybernetics生物控制论biological feedback system生物反馈系统C英文中文calibration校准,定标canonical form标准形式canonical realization标准实现capacity coefficient容量系数cascade control级联控制causal system因果系统cell单元,元胞cellular automaton元胞自动机central processing unit (CPU)中央处理器certainty factor确信因子characteristic equation特征方程characteristic function特征函数characteristic polynomial特征多项式characteristic root特征根英文中文charge-coupled device (CCD)电荷耦合器件chaotic system混沌系统check valve单向阀,止回阀chattering phenomenon颤振现象closed-loop control system闭环控制系统closed-loop gain闭环增益cluster analysis聚类分析coefficient of variation变异系数cogging torque齿槽转矩,卡齿转矩cognitive map认知图,认知地图coherency matrix相干矩阵collocation method配点法,配置法combinatorial optimization problem组合优化问题common mode rejection ratio (CMRR)共模抑制比,共模抑制率commutation circuit换相电路,换向电路commutator motor换向电动机D英文中文damping coefficient阻尼系数damping ratio阻尼比data acquisition system (DAS)数据采集系统data fusion数据融合dead zone死区decision analysis决策分析decision feedback equalizer (DFE)决策反馈均衡器decision making决策,决策制定decision support system (DSS)决策支持系统decision table决策表decision tree决策树decentralized control system分散控制系统decoupling control解耦控制defuzzification去模糊化,反模糊化delay element延时环节,滞后环节delta robot德尔塔机器人demodulation解调,检波density function密度函数,概率密度函数derivative action微分作用,微分动作design matrix设计矩阵E英文中文eigenvalue特征值,本征值eigenvector特征向量,本征向量elastic element弹性环节electric drive电子传动electric potential电势electro-hydraulic servo system电液伺服系统electro-mechanical coupling system电机耦合系统electro-pneumatic servo system电气伺服系统electronic governor电子调速器encoder编码器,编码装置end effector末端执行器,末端效应器entropy熵equivalent circuit等效电路error analysis误差分析error bound误差界,误差限error signal误差信号estimation theory估计理论Euclidean distance欧几里得距离,欧氏距离Euler angle欧拉角Euler equation欧拉方程F英文中文factor analysis因子分析factorization method因子法,因式分解法feedback反馈,反馈作用feedback control反馈控制feedback linearization反馈线性化feedforward前馈,前馈作用feedforward control前馈控制field effect transistor (FET)场效应晶体管filter滤波器,滤波环节finite automaton有限自动机finite difference method有限差分法finite element method (FEM)有限元法finite impulse response (FIR) filter有限冲激响应滤波器first-order system一阶系统fixed-point iteration method不动点迭代法flag register标志寄存器flip-flop circuit触发器电路floating-point number浮点数flow chart流程图,流程表fluid power system流体动力系统G英文中文gain增益gain margin增益裕度Galerkin method伽辽金法game theory博弈论Gauss elimination method高斯消元法Gauss-Jordan method高斯-约当法Gauss-Markov process高斯-马尔可夫过程Gauss-Seidel iteration method高斯-赛德尔迭代法genetic algorithm (GA)遗传算法gradient method梯度法,梯度下降法graph theory图论gravity gradient stabilization重力梯度稳定gray code格雷码,反向码gray level灰度,灰阶grid search method网格搜索法ground station地面站,地面控制站guidance system制导系统,导航系统gyroscope陀螺仪,陀螺仪器H英文中文H∞ control H无穷控制Hamiltonian function哈密顿函数harmonic analysis谐波分析harmonic oscillator谐振子,谐振环节Hartley transform哈特利变换Hebb learning rule赫布学习规则Heisenberg uncertainty principle海森堡不确定性原理hidden layer隐层,隐含层hidden Markov model (HMM)隐马尔可夫模型hierarchical control system分层控制系统high-pass filter高通滤波器Hilbert transform希尔伯特变换Hopfield network霍普菲尔德网络hysteresis滞后,迟滞,磁滞I英文中文identification识别,辨识identity matrix单位矩阵,恒等矩阵image processing图像处理impulse response冲激响应impulse response function冲激响应函数inadmissible control不可接受控制incremental encoder增量式编码器indefinite integral不定积分index of controllability可控性指标index of observability可观测性指标induction motor感应电动机inertial navigation system (INS)惯性导航系统inference engine推理引擎,推理机inference rule推理规则infinite impulse response (IIR) filter无限冲激响应滤波器information entropy信息熵information theory信息论input-output linearization输入输出线性化input-output model输入输出模型input-output stability输入输出稳定性J英文中文Jacobian matrix雅可比矩阵jerk加加速度,冲击joint coordinate system关节坐标系joint space关节空间Joule's law焦耳定律jump resonance跳跃共振K英文中文Kalman filter卡尔曼滤波器Karhunen-Loeve transform卡尔胡南-洛维变换kernel function核函数,核心函数kinematic chain运动链,运动链条kinematic equation运动方程,运动学方程kinematic pair运动副,运动对kinematics运动学kinetic energy动能L英文中文Lagrange equation拉格朗日方程Lagrange multiplier拉格朗日乘子Laplace transform拉普拉斯变换Laplacian operator拉普拉斯算子laser激光,激光器latent root潜根,隐根latent vector潜向量,隐向量learning rate学习率,学习速度least squares method最小二乘法Lebesgue integral勒贝格积分Legendre polynomial勒让德多项式Lennard-Jones potential莱纳德-琼斯势level set method水平集方法Liapunov equation李雅普诺夫方程Liapunov function李雅普诺夫函数Liapunov stability李雅普诺夫稳定性limit cycle极限环,极限圈linear programming线性规划linear quadratic regulator (LQR)线性二次型调节器linear system线性系统M英文中文machine learning机器学习machine vision机器视觉magnetic circuit磁路,磁电路英文中文magnetic flux磁通量magnetic levitation磁悬浮magnetization curve磁化曲线magnetoresistance磁阻,磁阻效应manipulability可操作性,可操纵性manipulator操纵器,机械手Markov chain马尔可夫链Markov decision process (MDP)马尔可夫决策过程Markov property马尔可夫性质mass matrix质量矩阵master-slave control system主从控制系统matrix inversion lemma矩阵求逆引理maximum likelihood estimation (MLE)最大似然估计mean square error (MSE)均方误差measurement noise测量噪声,观测噪声mechanical impedance机械阻抗membership function隶属函数N英文中文natural frequency固有频率,自然频率natural language processing (NLP)自然语言处理navigation导航,航行negative feedback负反馈,负反馈作用neural network神经网络neuron神经元,神经细胞Newton method牛顿法,牛顿迭代法Newton-Raphson method牛顿-拉夫逊法noise噪声,噪音nonlinear programming非线性规划nonlinear system非线性系统norm范数,模,标准normal distribution正态分布,高斯分布notch filter凹槽滤波器,陷波滤波器null space零空间,核空间O英文中文observability可观测性英文中文observer观测器,观察器optimal control最优控制optimal estimation最优估计optimal filter最优滤波器optimization优化,最优化orthogonal matrix正交矩阵oscillation振荡,振动output feedback输出反馈output regulation输出调节P英文中文parallel connection并联,并联连接parameter estimation参数估计parity bit奇偶校验位partial differential equation (PDE)偏微分方程passive attitude stabilization被动姿态稳定pattern recognition模式识别PD (proportional-derivative) control比例-微分控制peak value峰值,峰值幅度perceptron感知器,感知机performance index性能指标,性能函数period周期,周期时间periodic signal周期信号phase angle相角,相位角phase margin相位裕度phase plane analysis相平面分析phase portrait相轨迹,相图像PID (proportional-integral-derivative) control比例-积分-微分控制piezoelectric effect压电效应pitch angle俯仰角pixel像素,像元Q英文中文quadratic programming二次规划quantization量化,量子化quantum computer量子计算机quantum control量子控制英文中文queueing theory排队论quiescent point静态工作点,静止点R英文中文radial basis function (RBF) network径向基函数网络radiation pressure辐射压random variable随机变量random walk随机游走range范围,区间,距离rank秩,等级rate of change变化率,变化速率rational function有理函数Rayleigh quotient瑞利商real-time control system实时控制系统recursive algorithm递归算法recursive estimation递归估计reference input参考输入,期望输入reference model参考模型,期望模型reinforcement learning强化学习relay control system继电器控制系统reliability可靠性,可信度remote control system遥控系统,远程控制系统residual error残差误差,残余误差resonance frequency共振频率S英文中文sampling采样,取样sampling frequency采样频率sampling theorem采样定理saturation饱和,饱和度scalar product标量积,点积scaling factor缩放因子,比例系数Schmitt trigger施密特触发器Schur complement舒尔补second-order system二阶系统self-learning自学习,自我学习self-organizing map (SOM)自组织映射sensitivity灵敏度,敏感性sensitivity analysis灵敏度分析,敏感性分析sensor传感器,感应器sensor fusion传感器融合servo amplifier伺服放大器servo motor伺服电机,伺服马达servo valve伺服阀,伺服阀门set point设定值,给定值settling time定常时间,稳定时间T英文中文tabu search禁忌搜索,禁忌表搜索Taylor series泰勒级数,泰勒展开式teleoperation遥操作,远程操作temperature sensor温度传感器terminal终端,端子testability可测试性,可检测性thermal noise热噪声,热噪音thermocouple热电偶,热偶threshold阈值,门槛time constant时间常数time delay时延,延时time domain时域time-invariant system时不变系统time-optimal control时间最优控制time series analysis时间序列分析toggle switch拨动开关,切换开关tolerance analysis公差分析torque sensor扭矩传感器transfer function传递函数,迁移函数transient response瞬态响应U英文中文uncertainty不确定性,不确定度underdamped system欠阻尼系统undershoot低于量,低于值unit impulse function单位冲激函数unit step function单位阶跃函数unstable equilibrium point不稳定平衡点unsupervised learning无监督学习upper bound上界,上限utility function效用函数,效益函数V英文中文variable structure control变结构控制variance方差,变异vector product向量积,叉积velocity sensor速度传感器verification验证,校验virtual reality虚拟现实viscosity粘度,黏度vision sensor视觉传感器voltage电压,电位差voltage-controlled oscillator (VCO)电压控制振荡器W英文中文wavelet transform小波变换weighting function加权函数Wiener filter维纳滤波器Wiener process维纳过程work envelope工作空间,工作范围worst-case analysis最坏情况分析X英文中文XOR (exclusive OR) gate异或门,异或逻辑门Y英文中文yaw angle偏航角Z英文中文Z transform Z变换zero-order hold (ZOH)零阶保持器zero-order system零阶系统zero-pole cancellation零极点抵消。
halcon畸变校正adaptive算法原理 -回复

halcon畸变校正adaptive算法原理-回复Halcon (海康) 是一种用于机器视觉应用的强大的软件库。
它提供了广泛的图像处理和分析功能,包括畸变校正(Distortion Correction) 算法。
畸变校正在机器视觉中非常重要,因为它可以去除图像中由镜头畸变引起的形变,从而提高图像的准确性和可靠性。
在Halcon中,adaptive算法是一种常用的畸变校正方法。
本文将逐步介绍adaptive算法的原理及其应用。
第一节:畸变校正基础畸变校正是通过转换像素坐标来纠正图像中的形状失真。
镜头畸变主要包括径向畸变和切向畸变。
径向畸变是由于镜头形状引起的,会使得图像中的直线弯曲或弯曲。
切向畸变是由于镜头放置角度引起的,会导致图像中的直线扭曲或倾斜。
畸变校正的目标是将图像重新映射到一个平面上,使得图像中的直线变为直的。
畸变校正方法通常包括建立畸变模型和对图像进行数学变换两个步骤。
第二节:adaptive算法原理adaptive算法是一种自适应的畸变校正方法,它可以根据已知的畸变模型参数自动调整校正结果,以减小残余畸变。
adaptive算法的原理基于对畸变模型的灵活性进行优化。
adaptive算法包括以下主要步骤:1. 提供已知的畸变模型参数,包括径向畸变系数和切向畸变系数。
这些参数可以通过镜头制造商提供的校准数据或通过标定板的图像处理得到。
2. 将待校正的图像分割成小的校正区域。
这可以通过图像中的特定特征或用户定义的区域来完成。
3. 对于每个校正区域,计算出原始图像中的坐标和校正后图像中的坐标之间的映射关系。
这通常使用畸变模型参数来进行计算。
具体算法包括对原始图像的每个像素进行反向畸变计算,并查找最近邻像素来获得校正后图像中的坐标。
4. 根据映射关系对图像进行数学变换。
这通常包括对校正后图像进行插值来生成新的校正图像。
插值方法可以根据应用需求进行选择,常见的插值方法有双线性插值和双三次插值等。
5. 对校正图像进行残余畸变分析。
AMC+Edge英文基础知识 26页PPT文档
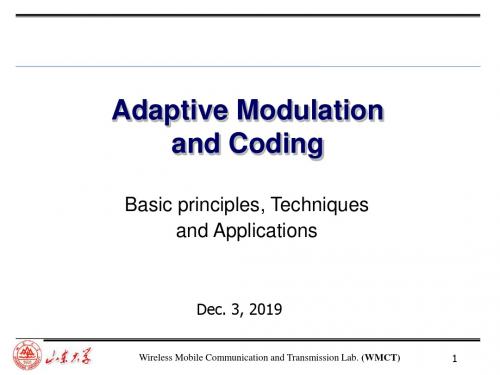
Wireless Mobile Communication and Transmission Lab. (WMCT)
5
Outline
Introduction of Adaptive Modulation and Coding Adaptive Transmission System Adaptive Techniques Application of Adaptive Modulation and Coding
Wireless Mobile Communication and Transmission Lab. (WMCT)
16
Outline
Introduction of Adaptive modulation and coding Adaptive Transmission System Adaptive Techniques
The higher data rates are achieved with large constellations – such as 64QAM – and less robust error correcting codes, for example rate 3/4 convolutional, turbo, or LDPC codes.
Wireless Mobile Communication and Transmission Lab. (WMCT)
15
ADC for multi-user
AMC vs Shannon Limit
Optionally turbo-codes or LDPC codes can be used instead of simple block/convolutional codes in these schemes
PTX10000模块路由器产品说明说明书

Data SheetFigure 1: PTX10000 router deployment•Security : Distributed Denial of Service (DDoS) attacks are a significant part of today’s threat landscape, and it is no longer feasible for providers to address this problem with traditional out-of-band scrubbing centers and manual intervention.Instead, they need a new real-time defense against volumetric DDoS attacks that delivers line-rate detection and mitigation while reducing costs. Juniper Connected Security is key to a reliable, predictable network.In order to address these challenges, network operators need a router that delivers scale, operational efficiency, and SDNprogrammability. Juniper Networks ® PTX Series Routers offer the industry’s only routing solution that exceeds these requirements while easily fitting into both cloud- and service-provider networks,providing full-feature support for core, peering, data center spine,data center edge, and infrastructure edge routing (see Figure 1).PTX10000 LineThe Juniper Networks PTX10000 line of Packet T ransport Routers brings physical and virtual innovations to the cloud and service provider networks. These next-generation modular routers help network operators achieve their business goals while effectively handling current and future traffic demands through automation,optimization, and programmability.PTX10004, PTX10008, and PTX10016 Modular RoutersThe modular PTX10000 line features three models: the PTX10004,the PTX10008, and the PTX10016.The PTX10004 Modular Router is the ultra-compact, ultra-high-density router for today’s space- and power-constrained facilities,supporting 400GbE architectures with inline Media Access Control Security (MACsec) on all ports for uncompromised security. The PTX10004 scales from 19.2 Tbps to 57.6 Tbps in a compact 7 U platform, design-optimized for data center and colocation racks.Powered by Juniper’s custom T riton silicon, the PTX10004 delivers predictable IP/MPLS packet performance and functionality for multirate 100GbE/400GbE QSFP-DD. The PTX10004 is equipped with four slots, supporting from 4.8 Tbps to 14.4 Tbps per slot. A fully equipped PTX10004 with 4.8 Tbps line cards can support 192100GbE and 16 400GbE ports. A fully equipped PTX10004 with 14.4 Tbps line cards can support 576 10GbE, 576 25GbE, 14440GbE, 576 100GbE, or 144 400GbE ports.The PTX10008 Modular Router is the perfect router for today’s space-constrained facilities, supporting 400GbE architectures with inline MACsec on all ports for uncompromised security. The PTX10008 offers unprecedented system capacity in a 13 Uplatform, scaling from 28.8 Tbps to 115.2 Tbps per standard 19-inch telecommunications rack—all at wire speed.Powered by Juniper’s custom T riton silicon, the PTX10008 delivers predictable IP/MPLS packet performance and functionality,eliminating the complex packet profiles found in elaborate, over-engineered network processing units (NPUs) deployed in other core routers. The PTX10008 has eight slots, each supporting 4.8 Tbps to 14.4 Tbps. A fully equipped PTX10008 with 4.8 Tbps line cards can support 256 100GbE and 32 400GbE ports. A fully equipped PTX10008 with 14.4 Tbps line cards can support 1152 10GbE,1152 25GbE, 288 40GbE, 1152 100GbE, or 288 400GbE interfaces in a single chassis.The PTX10016 Modular Router offers ultra-high system capacity in a 21 U platform that supports 48 Tbps (96 Tbps half-duplex) per chassis, or 96 Tbps (192 Tbps half-duplex) per standard 19-inch telecommunications rack. The PTX10016 has 16 slots, eachsupporting 3 Tbps (6 Tbps half-duplex). A fully equipped PTX10016can support 2304 10GbE, 576 40GbE, or 480 100GbE interfaces in a single chassis.These modular routers enable network operators to build core architectures that optimize label-switching router (LSR), Internet backbone, peering, and optical convergence applications.As a result, operators can—for the first time—match traffic demands with enhanced core router performance and flexible deployments. With its ultra-optimized and compact form factor, the PTX10000 line is ideal for peering, collocation, and central office locations where space and power are at a premium.Innovations in SiliconInnovations in silicon enable the PTX10000 line to accommodate scale-up and scale-out architectures with smooth migration paths as traffic patterns change. Juniper silicon allows adaptive load balancing, data structure sharing, and better resource utilization, all well as supporting value-added resources for additional filtering flexibility—all while lowering cost per bit.The PTX10004 and PTX10008 are powered by the highly scalable custom Juniper T riton silicon, the industry’s first inline MACsec for 400GbE chip to support universal multirate QSFP56-DD. The Juniper T riton silicon delivers consistently low latency, 8 m counters, 256 Advanced Encryption Standard (AES) MACsec encryption supported on all ports, and wire-rate packet performance for IP traffic without sacrificing the optimized system power profile. Preserving the spirit of the Junos® Express silicon family, Juniper T riton silicon is the first purpose-built telecommunications silicon to incorporate a 3D memory architecture into the base design, offering the industry’s highest packet performance per gigabit in the fewest rack units. It also provides dynamic table memory allocation for massive IP routing scale while delivering tremendous power efficiency gains at 0.14 watts/gigabit.The PTX10016 is powered by the highly functional Juniper Express silicon. Offering native 100GbE MACsec and 2 million+ forwarding information base, also known as forwarding table (FIB), the Express silicon family offers deep telemetry and flex packet processing, optimized for density, power, and scale with 0.4 watts/gigabit power efficiency. Express-based line cards are not compatible with T riton-based line cards.The ability to address a provider’s core networking requirements—scale, operational flexibility, and SDN control— begins with the silicon. With the PTX10000 line, operators can now deploy a core architecture with SDN control. Combining Juniper Networks NorthStar Controller with a robust full-featured Internet backbone router, and a regional IP/MPLS core router with integrated 100GbE coherent transport for superior performance, operators can tune their network infrastructure through proactive monitoring and what-if planning capabilities. The NorthStar Controller dynamically creates explicit routing paths using a global view based on user-defined constraints to create a fully autonomous operation.Scale is one of the guiding design principles for the PTX10000 line, allowing network operators to smoothly handle increased traffic demands. The PTX10000 line simplifies network engineering challenges with predictable system latency, improving the overall service experience by delivering best-in-class resiliency to help providers meet strict customer service-level agreements (SLAs).SDN programmability brings virtual innovations to the service provider core, while the NorthStar Controller offers an open, standards-based solution that optimizes both the IP layer and the transport layer with precise SDN control, allowing network operators to fully automate and scale their operations with ease.Architecture and Key ComponentsThe PTX10000 line of Packet T ransport Routers features a number of key architectural elements. Dual redundant routing engines (REs) on the PTX10004, PTX10008, and PTX10016 run the Juniper Networks Junos operating system, where they manage all routing protocol processes, router interface control, and control plane functions such as chassis component, system management, and user access to the router. These processes interact with the Packet Forwarding Engine (PFE) on the line cards via dedicated high-bandwidth management channels, providing a clean separation of the control and forwarding planes.The PTX10000 T riton-based line cards currently support 10GbE, 25GbE, 40GbE, 100GbE, and 400GbE interfaces. The horizontal line cards in the front of the chassis connect directly to the vertical switch fabric cards in the rear of the chassis via orthogonal interconnects without requiring a midplane. This provides unparalleled investment protection by ensuring a smooth upgrade path to higher speed switch fabric cards as they become available. The midplane-less design improves airflow with a front-to-back design and enables limitless scale.T o maintain uninterrupted operation, the PTX10000 line modular chassis fan trays cool the line cards and REs with redundant, variable-speed fans. In addition, the PTX10000 line power supplies convert building power to the internal voltage required by the system. All PTX10000 line components are hot-swappable, and all central functions are available in redundant configurations, providing high operational availability by allowing continuous system operation during maintenance or repairs.PTX10000 Line Hardware ComponentsKey hardware components of the PTX10000 line of PacketT ransport Routers include the line cards, switch fabrics, and Routing Engines (REs).Switch FabricsTwo generations of switch fabric exist: SF and SF3. The SF fabric offers 3 Tbps capacity per slot and supports Express silicon-based line cards. The SF3 fabric enables 14.4 Tbps capacity per slot and supports T riton silicon-based line cards only.Juniper SF and SF3 fabrics utilize purpose-built silicon to enable nonblocking communication between line cards, offering lower latency and lower delay variation to reduce power requirements and overall system costs for 100GbE deployments.Triton Line CardsThe PTX10004 and PTX10008 routers' second-generation line card is based on the highly scalable custom Juniper T riton silicon, making it one of the industry’s leading core routers for MPLS, Internet backbone, peering, and transport integration applications. Each slot on the PTX10004 and PTX10008 can support 4.8 Tbps (9.6 Tbps half-duplex) or 14.4 Tbps (28.8 Tbps half-duplex), while the line cards support multirate 10GbE, 25GbE, 40GbE, 100GbE, and400GbE interfaces. The line card also supports native MACsec without compromising throughput on any supported interface rate up to 400GbE, providing point-to-point security on Ethernet links. MACsec blocks security threats such as denial of service (DoS), intrusion, man-in-the-middle, masquerading, passive wiretapping, and playback attacks while securing links for most traffic frames from the Link Layer Discovery Protocol (LLDP), Link Aggregation Control Protocol (LACP), Dynamic Host Configuration Protocol (DHCP), Address Resolution Protocol (ARP), and others. All ports can support 400GbE ZR and ZR+ optics, making it ready for full packet/optical convergence without compromising density.•PTX10K-LC1201-36CD (JNP10K-LC1201): 14.4 Tbpscapacity per slot, supporting 144 10GbE, 144 25GbE, 3640GbE, 144 100GbE, or 36 400GbE ports with QSFP56-DD/ QSFP56/QSFP28-DD/QSFP28/QSFP+.•PTX10K-LC1202-36MR (JNP10K-LC1202): 4.8 Tbps capacity per slot, supporting 32 100GbE and four 400GbE portssimultaneously with QSFP56-DD and QSFP28. Additionally,144 10GbE, 144 25GbE, 36 40GbE are supported; 10GbEports are also supported via breakout cables.Express Line CardsThe PTX10016 is based on the highly capable custom Juniper Express silicon, making it one of the industry’s leading core routers for MPLS, Internet backbone, peering, and transport integration applications. Each slot on the PTX10016 supports 3 Tbps forwarding bandwidth, while the line cards support 10GbE, 40GbE, and 100GbE interfaces. Multiple Express-based interface cards are available under the PTX10016:•PTX10K-LC1101 (JNP10K-LC1101): 3 Tbps capacity per slot supporting 96 10GbE, 30 40GbE, or 30 100GbE ports withQSFP28/QSFP+. Additionally, 10GbE ports are supported via breakout cables.•PTX10K-LC1102 (JNP10K-LC1102): 1.4 Tbps capacity perslot supporting 144 10GbE, 36 40GbE, or 12 100GbE portswith QSFP28/QSFP+. Additionally, 10GbE ports are supported via breakout cables.•PTX10K-LC1104 (JNP10K-LC1104): 1.2 Tbps packet optical dense wavelength-division multiplexing (DWDM) line card with MACsec supporting six 100GbE, six 150GbE, and six 200GbE ports with integrated coherent optics.•PTX10K-LC1105 (JNP10K-LC1105): 3 Tbps capacity per slot with inline MACsec, supporting 96 10GbE, 30 40GbE, or 30100GbE ports with QSFP28/QSFP+.•PTX10K-LC1103 (QFX10000-60S-6Q support in PTX10K):Native 10GbE line card with 840 Gbps capacity supporting 60 1GbE, 60 10GbE, 6 40GbE, and 2 100GbE ports withQSFP28/QSFP+/SFP+.Routing EngineRouting Engines in the PTX10000 line perform system management and routing control functions. REs are based on theX86 architecture and can be installed in a PTX10000 line chassis to provide 1+1 redundancy. Two generations of Routing Engines exist:•JNP10K-RE0: The first-generation RE0 Routing Enginefeatures a quad-core 2.5 GHz Intel processor with 32 GBmemory and 2x50 GB solid-state drive (SSD) storage.•JNP10K-RE1: The second-generation RE1 Routing Enginefeatures a core 2.2 GHz Intel processor with memory options of 64 GB or 128 GB and 2x200 GB solid-state drive (SSD)storage.PowerThe PTX10004 has three power supply slots, the PTX10008 offers six power supply slots, and the PTX10016 has 10 power supply slots, providing complete flexibility for provisioning and redundancy. Each power supply has its own internal fan for cooling. ThePTX10000 line supports both AC and DC power supplies; however, AC and DC supplies cannot be mixed in the same chassis. Two generations of power supplies exist: the first generation is designed to support Express line cards, while the second generation is designed to support both Express and T riton line cards.The first generation of AC power supplies on the PTX10000 line routers accept 200 to 240 volts alternating current (VAC) input, delivering 2700 watts of power to the chassis. The first generation of DC power supplies accept -40 to -72 volts direct current (VDC) input, delivering 2500 watts of power to the chassis. Each AC and DC power supply has two inputs for feed redundancy.Second-generation AC power supplies (AC2) on the PTX10000 line routers are high-capacity, high-line models designed to support either AC or DC systems in either a low-power or high-power mode. The power supply takes AC input and provides DC output of 12.3 VDC, delivering 5000 watts with a single feed and 5500 watts with a dual feed. For AC systems, the operating input is 180 to 305 VAC; for DC systems, the operating input is190 to 410 VDC. Second-generation DC power supplies (DC2) provide two power supplies in a single housing that accepts either 60 A or 80 A using four redundant input power feeds.CoolingThe PTX10000 line supports front-to-back cooling with air drawn in through the perforations on the REs and the line cards in the front of the platform. The fan trays are in front of the fabric cards and are accessible from the rear of the chassis. Hot air exhausts through the rear of the chassis.Chassis ManagementThe PTX10000 line delivers powerful Junos OS chassis management that allows environmental monitoring and field-replaceable unit (FRU) control. Chassis management provides a faster primary switchover, enhanced power budgeting with a modular power management, reduced power consumption for partially populated systems, granular control over FRU power-on, adaptive cooling, and CPU leveling during monitoring intervals.Simplified ManagementThe PTX10000 line routers simplify management based on the elegance and simplicity of the Junos OS. Management applications can receive streaming telemetry data to provide robust protocol analytics for an SDN environment. Junos OS enables resilience by design, operational consistency, and the versatility needed to evolve your network.SONiC Support on the PTX10008The PTX10008 supports Juniper’s SONiC implementation, delivering best-of-breed hardware for cloud operators while taking advantage of the flexibility of SONiC’s open and disaggregated architecture. The SONiC-enabled PTX10008 plugs seamlessly into a unified SONiC network infrastructure, leveraging the existing PTX10008 hardware. The Juniper-provided SONiC image, installed on the hardware at the factory, includes the platform device drivers and Juniper's Hardware Abstraction Layer (HAL), including Juniper's implementation of the Switch Abstraction Interface (SAI) for theT riton ASIC and the line card PFE software.As a modular and dense multi-PFE 400GbE/100GbE platform, the PTX10008 is perfectly suited for large spine layer applications in data center IP fabrics. Juniper complements the SONiC OS with the containerized Routing Protocol Daemon (cRPD), a full-function routing and management stack packaged as a container. This ensures a consistent end-to-end routing experience across different tiers in the data center. In addition, the cRPD enables high-performance telemetry, automation, and programmability in a lightweight deployment. For features available with SONiC, please refer to the SONiC deployment guide.Features and BenefitsTable 1 summarizes the features available on the PTX10004, PTX10008, and PTX10016 routers. Table 1. PTX10000 Line Features and BenefitsSpecificationsTable 2. PTX10000 Line SpecificationsJuniper Networks Services and SupportJuniper Networks is the leader in performance-enabling services that are designed to accelerate, extend, and optimize your high-performance network. Our services allow you to maximize operational efficiency while reducing costs and minimizing risk, achieving a faster time to value for your network. Juniper Networks ensures operational excellence by optimizing the network to maintain required levels of performance, reliability, and availability. For more details, please visit /uk/en/products-services.PTX10000 Line Ordering InformationFor more information, please contact your Juniper Networks representative.About Juniper NetworksJuniper Networks brings simplicity to networking with products,solutions and services that connect the world. Through engineering innovation, we remove the constraints and complexities of networking in the cloud era to solve the toughest challenges our customers and partners face daily. At Juniper Networks, we believe that the network is a resource for sharing knowledge and human advancement that changes the world. We are committed toimagining groundbreaking ways to deliver automated, scalable and secure networks to move at the speed of business.Corporate and Sales HeadquartersJuniper Networks, Inc.1133 Innovation Way Sunnyvale, CA 94089 USAPhone: 888.JUNIPER (888.586.4737)or +APAC and EMEA HeadquartersJuniper Networks International B.V. Boeing Avenue 240 1119 PZ Schiphol-Rijk Amsterdam, The Netherlands Phone: +31.0.207.125.700Copyright 2021 Juniper Networks, Inc. All rights reserved. Juniper Networks, the Juniper Networks logo, Juniper, and Junos are registered trademarks of Juniper Networks, Inc. in the United States and other countries. All other trademarks, service marks, registered marks, or registered service marks are the property of their respective owners. Juniper Networks assumes no。
罗克韦尔自动化 运动控制 快速入门 无需调谐功能配置 基尼克斯 5300、基尼克斯 5500 和基尼
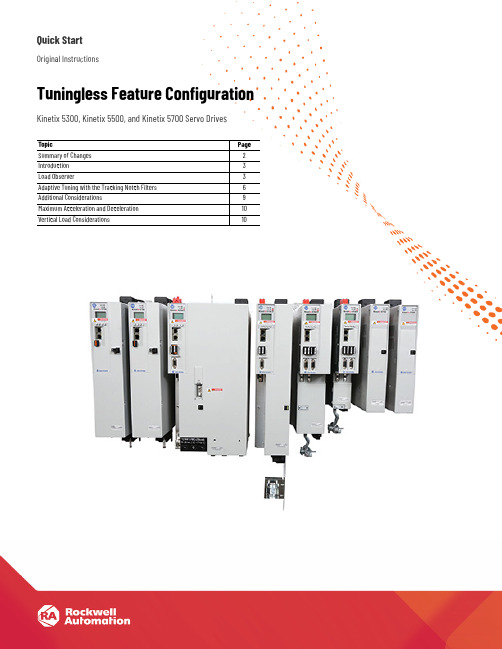
Quick StartOriginal InstructionsTuningless Feature Configuration Kinetix 5300, Kinetix 5500, and Kinetix 5700 Servo DrivesTopic Page Summary of Changes2 Introduction3 Load Observer3 Adaptive Tuning with the Tracking Notch Filters6 Additional Considerations9 Maximum Acceleration and Deceleration10 Vertical Load Considerations10Tuningless Feature Configuration Quick StartSummary of ChangesThis publication contains the following new or updated information. This list includes substantive updates only and is not intended to reflect all changes.Topic PageAdded references to Kinetix® 5300 servo drives throughoutUpdated screen captures to reflect latest version of the Studio 5000 Logix Designer® application throughout2Rockwell Automation Publication MOTION-QS001D-EN-P - November 2020Rockwell Automation Publication MOTION-QS001D-EN-P - November 20203Tuningless Feature Configuration Quick StartIntroductionClosed-loop servo systems require settings for the control loop gains and filter values to make sure that the load accurately follows the desired input-command signal. The process of adjusting and refining the gain and filter configuration is called tuning.Appropriate tuning settings depend heavily upon the system characteristics. Each machine behaves differently due to variables such as compliance, backlash, changing inertias, manufacturing tolerances, and machine degradation, so the tuning configuration can vary greatly from one machine to the next.With the tuning features of the Kinetix® 5300, Kinetix 5500, and Kinetix 5700 servo drives, tuningless operation can now be achieved without compromising on performance. By using both the load observer and the tracking notch filters in the servo drives, most applications no longer require tuning procedures and tests during the commissioning process to achieve an effective level of machine performance.Load ObserverThe load observer feature operates in real time while the machine is running. During machine operation, the load observer estimates the mechanical load inertia on the motor and compensates for it. The result is that the drive controls the motor as if it is unloaded, which provides a relatively high level of drive performance. In addition, the drive automatically compensates for mechanical variations in the system such as changing loads, compliance, and machine wear over time.ConfigurationThe following steps describe how to configure an axis with the recommended load observer settings for most applications. With the Logix Designer application version 33 and later, the load observer feature is enabled by default for the following drives, so these configuration steps are not required for tuningless operation:•Kinetix 5300 drives •Kinetix 5700 ERS3 Series B drives, with firmware revision 13 and later •Kinetix 5700 ERS4 drives, with firmware revision 13 and later1.In the Controller Organizer, right-click an axis and choose Properties. IMPORTANT Use the load observer with the tracking notch filters to achieve effective tuningless operation. IMPORTANTTo ensure stable operation, it is recommended that you do not perform the autotune test when applying the load observer feature.Tuningless Feature Configuration Quick Start2.Select the Autotune category.3.From the Application Type pull-down menu, choose Custom.4Rockwell Automation Publication MOTION-QS001D-EN-P - November 2020Rockwell Automation Publication MOTION-QS001D-EN-P - November 20205Tuningless Feature Configuration Quick Start 4.Clear the Torque Low Pass Filter checkbox.5.Select the Load category and verify that the Load Ratio is zero; otherwise, set it to zero.6.Under the Load category, select Observer.6Rockwell Automation Publication MOTION-QS001D-EN-P - November 2020Tuningless Feature Configuration Quick Start7.From the Configuration pull-down menu, choose ‘Load Observer with Velocity Estimate’ if the axis is configured for Position Loop control or ‘Load Observer Only’ if the axis is configured for Velocity Loop control. Load Observer is not available for Torque Loop control.8.Click Apply.Adaptive Tuning with the Tracking Notch FiltersThe tracking notch filters operate in real time while the machine runs. During machine operation, the drive measures the mechanical resonances in the system and dynamically sets the frequencies of the various notch filters to attenuate the effect of the resonances. ConfigurationThe following steps describe how to configure an axis with the recommended adaptive tuning settings for most applications. With the Logix Designer application version 33 and later, the tracking notch filters are enabled by default for the following drives, so these configuration steps are not required for tuningless operation•Kinetix 5300 drives •Kinetix 5700 ERS3 Series B drives, with firmware revision 13 and later •Kinetix 5700 ERS4 drives, with firmware revision 13 and laterIt is recommended that Position Loop control is used for both positioning and velocity applications. In select cases, application requirements may dictate Velocity Loop control.IMPORTANTUse the load observer with the tracking notch filters to achieve effective tuningless operation.Rockwell Automation Publication MOTION-QS001D-EN-P - November 20207Tuningless Feature Configuration Quick Start1.In the Controller Organizer, right-click an axis and then choose Properties.2.Under the Load category, select Compliance.3.From the Adaptive Tuning Configuration pull-down menu, choose Tracking Notch.With the Logix Designer application version 33 and later, the Compliance category parameters for the following drives will differ since they have four notch filters:•Kinetix 5300 drives•Kinetix 5700 ERS3 Series B drives, with firmware revision 13 and later•Kinetix 5700 ERS4 drives, with firmware revision 13 and laterTuningless Feature Configuration Quick Start4.Select the Cyclic Parameters Category.5.Scroll down and check TorqueNotchFilterFrequencyEstimate and TorqueNotchFilterMagnitudeEstimate.6.Click Apply.Selecting these parameters is optional. They are available to assist withcommissioning and provide diagnostic information.8Rockwell Automation Publication MOTION-QS001D-EN-P - November 2020Rockwell Automation Publication MOTION-QS001D-EN-P - November 20209Tuningless Feature Configuration Quick StartAdditional ConsiderationsFor more detailed technical information on the operation of the load observer or adaptive tuning features, see Chapter 1 of Motion System Tuning Application Techniques, publication MOTION-AT005.Notch Filter InitializationWith the Logix Designer application version 33 and later, the drive will persist adaptive tuning values through a drive power cycle for the following drives:•Kinetix 5300 drives •Kinetix 5700 ERS3 Series B drives, with firmware revision 13 and later •Kinetix 5700 ERS4 drives, with firmware revision 13 and laterFor details on how to retain the notch filter settings when power is removed and reapplied to the system, see Knowledgebase Technote Retaining Notch Filter Frequency through System Power Cycle .Increased PerformanceManual tuning may be used if higher performance is required after applying the default load observer gain values. It is recommended that you incrementally increase the bandwidth values while maintaining the following relationships:For Position Loop Control•Load Observer Bandwidth = 4 x Velocity Bandwidth = 16 x Position BandwidthFor Velocity Loop Control•Load Observer Bandwidth = Velocity BandwidthThe bandwidth values can be increased until the desired system performance is achieved.To reduce following error, it is recommended that Integrator Bandwidth be applied according to the following relationship:For Position Loop Control•Position Integrator Bandwidth = Position Bandwidth/100For Velocity Loop Control•Velocity Integrator Bandwidth = Velocity Bandwidth/10The integrator bandwidth value can be adjusted until the desired system performance is achieved. It is not recommended to use the position and velocity integrators simultaneously.For more detailed technical information on manual tuning, see chapter 4 of Motion System Tuning Application Techniques, publication MOTION-AT005.It is recommended that Position Loop control is used for both positioning and velocity applications. In select cases, application requirements may dictate Velocity Loop control.10Rockwell Automation Publication MOTION-QS001D-EN-P - November 2020Tuningless Feature Configuration Quick StartMaximum Acceleration and DecelerationWhen using the load observer feature, it is recommended that the load ratio is set to zero. For applications that use ‘percent of maximum’ as acceleration units for motion instructions, the Maximum Acceleration and Maximum Deceleration values for the application should bedecreased (as appropriate for the load) to stay within drive and motor limits during operation. The Maximum Acceleration and Deceleration attribute values can be found in the Planner category of the Axis Properties dialog box.Vertical Load ConsiderationsThe gain stabilization feature is not recommended for vertical loads, as detuning of the control loop gains produced by this feature may cause load drops. For more detailed information on techniques for managing vertical loads, see the Vertical Load and Holding Brake Management Application Technique, publication MOTION-AT003.Rockwell Automation Publication MOTION-QS001D-EN-P - November 202011Tuningless Feature Configuration Quick StartAdditional ResourcesThese documents contain additional information concerning related products from Rockwell Automation.You can view or download publications at rok.auto/literature .Resource DescriptionIndustrial Automation Wiring and Grounding Guidelines, publication 1770-4.1Provides general guidelines for installing a Rockwell Automation® industrial system.Product Certifications website, /global/certification/overview.pageProvides declarations of conformity, certificates, and other certification details.Servo Drive Installation Best Practices, publication MOTION-AT004Provides a quick reference of installation best practices for Rockwell Automation single-axis and multi-axis servo drive systems.Motion System Tuning Application Techniques, publication MOTION-AT005Provides information and tips for motion system tuning. Kinetix 5300 User Manual, publication MOTION-UM005Provides information on installing, configuring, starting, and troubleshooting your Kinetix 5300 servo drive system.Kinetix 5500 Servo Drives User Manual, publication 2198-UM001Provides information on installing, configuring, starting, and troubleshooting your Kinetix 5500 servo drive system.Kinetix 5700 Servo Drives User Manual, publication 2198-UM002Provides information on installing, configuring, starting, and troubleshooting your Kinetix 5700 servo drive system.Integrated Motion on the EtherNet/IP Network: Configuration and Startup User Manual, publication MOTION-UM003Information on how to configure and troubleshoot your ControlLogix® and CompactLogix™ EtherNet/IP™ network modules.Industrial Automation Wiring and Grounding Guidelines, publication 1770-4.1Provides general guidelines for installing a Rockwell Automation® industrial system.Product Certifications website, /global/certification/overview.pageProvides declarations of conformity, certificates, and other certification details.Servo Drive Installation Best Practices, publication MOTION-AT004Provides a quick reference of installation best practices for Rockwell Automation single-axis and multi-axis servo drive systems.Motion System Tuning Application Techniques, publication MOTION-AT005Provides information and tips for motion system tuning.Kinetix 5300 User Manual, publication MOTION-UM005Provides information on installing, configuring, starting, and troubleshooting your Kinetix 5300 servo drive system.Publication MOTION-QS001D-EN-P - November 2020Supersedes Publication MOTION-QS001C-EN-P - November 2018Copyright © 2020 Rockwell Automation, Inc. All rights reserved. Printed in the U.S.A.Rockwell Automation SupportUse these resources to access support information.Documentation FeedbackYour comments help us serve your documentation needs better. If you have any suggestions on how to improve our content, complete the form at rok.auto/docfeedback .Technical Support CenterFind help with how-to videos, FAQs, chat, user forums, and product notification updates.rok.auto/support KnowledgebaseAccess Knowledgebase articles.rok.auto/knowledgebase Local Technical Support Phone NumbersLocate the telephone number for your country.rok.auto/phonesupport Literature LibraryFind installation instructions, manuals, brochures, and technical data publications.rok.auto/literature Product Compatibility and Download Center (PCDC)Download firmware, associated files (such as AOP, EDS, and DTM), and access product release notes.rok.auto/pcdc Rockwell Automation maintains current product environmental compliance information on its website at rok.auto/pec .Allen-Bradley, ControlLogix, CompactLogix, expanding human possibility, Kinetix, Rockwell Automation, and Studio 5000 Logix Designer are trademarks of Rockwell Automation, Inc.EtherNet/IP is a trademark of ODVA, Inc.Trademarks not belonging to Rockwell Automation are property of their respective companies.Rockwell Otomasyon Ticaret A.Ş. Kar Plaza İş Merkezi E Blok Kat:6 34752, İçerenköy, İstanbul, Tel: +90 (216) 5698400 EEE Yönetmeliğine Uygundur。
新视野大学英语4 UNIT10课文翻译及答案
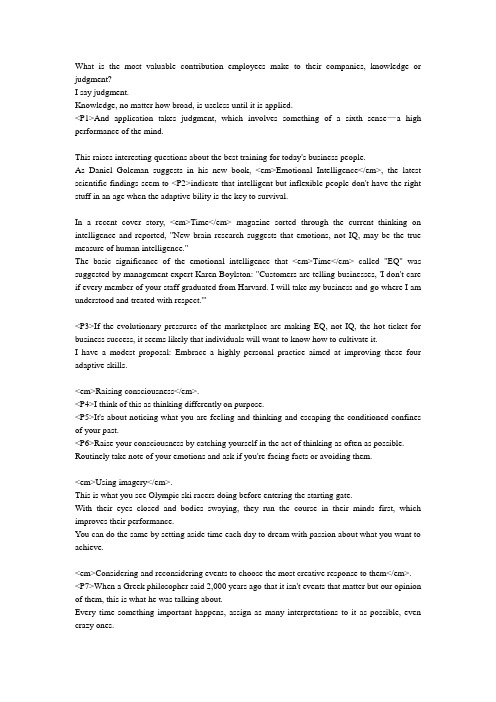
What is the most valuable contribution employees make to their companies, knowledge or judgment?I say judgment.Knowledge, no matter how broad, is useless until it is applied.<P1>And application takes judgment, which involves something of a sixth sense—a high performance of the mind.This raises interesting questions about the best training for today's business people.As Daniel Goleman suggests in his new book, <em>Emotional Intelligence</em>, the latest scientific findings seem to <P2>indicate that intelligent but inflexible people don't have the right stuff in an age when the adaptive bility is the key to survival.In a recent cover story, <em>Time</em> magazine sorted through the current thinking on intelligence and reported, "New brain research suggests that emotions, not IQ, may be the true measure of human intelligence."The basic significance of the emotional intelligence that <em>Time</em> called "EQ" was suggested by management expert Karen Boylston: "Customers are telling businesses, 'I don't care if every member of your staff graduated from Harvard. I will take my business and go where I am understood and treated with respect.'"<P3>If the evolutionary pressures of the marketplace are making EQ, not IQ, the hot ticket for business success, it seems likely that individuals will want to know how to cultivate it.I have a modest proposal: Embrace a highly personal practice aimed at improving these four adaptive skills.<em>Raising consciousness</em>.<P4>I think of this as thinking differently on purpose.<P5>It's about noticing what you are feeling and thinking and escaping the conditioned confines of your past.<P6>Raise your consciousness by catching yourself in the act of thinking as often as possible. Routinely take note of your emotions and ask if you're facing facts or avoiding them.<em>Using imagery</em>.This is what you see Olympic ski racers doing before entering the starting gate.With their eyes closed and bodies swaying, they run the course in their minds first, which improves their performance.You can do the same by setting aside time each day to dream with passion about what you want to achieve.<em>Considering and reconsidering events to choose the most creative response to them</em>. <P7>When a Greek philosopher said 2,000 years ago that it isn't events that matter but our opinion of them, this is what he was talking about.Every time something important happens, assign as many interpretations to it as possible, even crazy ones.Then go with the interpretation most supportive of your dreams.<em>Integrating the perspectives of others</em>.Brain research shows that our view of the world is limited by our genes and the experiences we've had.<P8>Learning to <1>incorporate</1> the useful perspectives of others is nothing less than a form of enlarging your senses.The next time someone interprets something differently from you—say, a controversial political event—pause to reflect on the role of life experience and consider it a gift of perception.<P9>The force of habit—literally the established wiring of your brain—will pull you away from practicing these skills.Keep at it, however, because they are based on what we're learning about the mechanism of the mind.Within the first six months of life the human brain doubles in capacity.It doubles again by age four and then grows rapidly until we reach sexual maturity.The body has about a hundred billion nerve cells, and every experience <2>triggers</2> a brain response that literally shapes our senses.The mind, we now know, is not confined to the brain but is distributed throughout the body's universe of cells.Yes, we do think with our hearts, brains, muscles, blood and bones.During a single crucial three-week period during our teenage years, chemical activity in the brain is cut in half.<P10>That done, we are "biologically wired" with what one of the nation's leading brain researchers calls our own "world view".He says it is impossible for any two people to see the world exactly alike.So unique is the personal experience that people would understand the world differently.However, it is not only possible to change your world view, he says, it's actually easier than overcoming a drug habit.<P11>But you need a discipline for doing it.Hence, the method recommended here.<P12>No, it's not a curriculum in the sense that an MBA is.But the latest research seems to imply that without the software of emotional maturity and self-knowledge, the hardware of academic training alone is worth less and less.员工对公司最有价值的贡献是什么,是知识还是判断力?我说是判断力。
考研英语词汇,词根
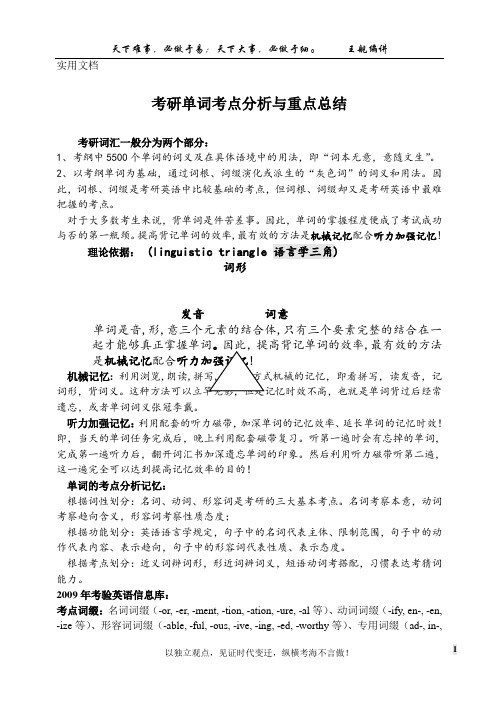
考研单词考点分析与重点总结考研词汇一般分为两个部分:1、考纲中5500个单词的词义及在具体语境中的用法,即“词本无意,意随文生”。
2、以考纲单词为基础,通过词根、词缀演化或派生的“灰色词”的词义和用法。
因此,词根、词缀是考研英语中比较基础的考点,但词根、词缀却又是考研英语中最难把握的考点。
对于大多数考生来说,背单词是件苦差事。
因此,单词的掌握程度便成了考试成功与否的第一瓶颈。
提高背记单词的效率,最有效的方法是机械记忆配合听力加强记忆!理论依据: (linguistic triangle 语言学三角)词形发音词意单词是音,形,意三个元素的结合体,只有三个要素完整的结合在一,最有效的方法是机械记忆配合机械记忆: 利用浏览,朗读,遗忘,或者单词词义张冠李戴。
听力加强记忆:利用配套的听力磁带,加深单词的记忆效率、延长单词的记忆时效!即,当天的单词任务完成后,晚上利用配套磁带复习。
听第一遍时会有忘掉的单词,完成第一遍听力后,翻开词汇书加深遗忘单词的印象。
然后利用听力磁带听第二遍,这一遍完全可以达到提高记忆效率的目的!单词的考点分析记忆:根据词性划分:名词、动词、形容词是考研的三大基本考点。
名词考察本意,动词考察趋向含义,形容词考察性质态度;根据功能划分:英语语言学规定,句子中的名词代表主体、限制范围,句子中的动作代表内容、表示趋向,句子中的形容词代表性质、表示态度。
根据考点划分:近义词辩词形,形近词辨词义,短语动词考搭配,习惯表达考猜词能力。
2009年考验英语信息库:考点词缀:名词词缀(-or, -er, -ment, -tion, -ation, -ure, -al等)、动词词缀(-ify, en-, -en, -ize等)、形容词词缀(-able, -ful, -ous, -ive, -ing, -ed, -worthy等)、专用词缀(ad-, in-,dis-, mis-, under-, multi-, over-, trans-, -ism等)。
可穿戴计算音频信息压缩的上下文相关通知模型_张超钦
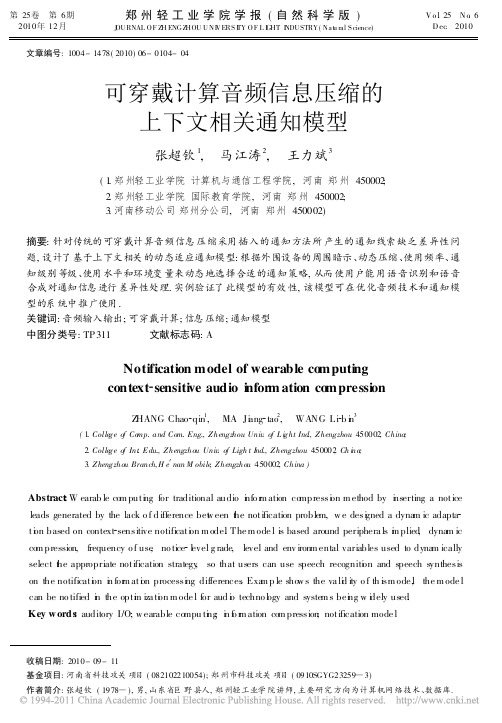
第25卷 第6期2010年12月郑州轻工业学院学报(自然科学版)J OURNAL OF Z H ENGZ HOU UNI VERS I TY OF L I G HT I NDUSTRY(Nat u ral S cience)V o.l 25 N o .6D ec .2010收稿日期:2010-09-11基金项目:河南省科技攻关项目(082102210054);郑州市科技攻关项目(0910SGYG23259 3)作者简介:张超钦(1978 ),男,山东省巨野县人,郑州轻工业学院讲师,主要研究方向为计算机网络技术、数据库.文章编号:1004-1478(2010)06-0104-04可穿戴计算音频信息压缩的上下文相关通知模型张超钦1, 马江涛2, 王力斌3(1.郑州轻工业学院计算机与通信工程学院,河南郑州450002;2.郑州轻工业学院国际教育学院,河南郑州450002;3.河南移动公司郑州分公司,河南郑州450002)摘要:针对传统的可穿戴计算音频信息压缩采用插入的通知方法所产生的通知线索缺乏差异性问题,设计了基于上下文相关的动态适应通知模型:根据外围设备的周围暗示、动态压缩、使用频率、通知级别等级、使用水平和环境变量来动态地选择合适的通知策略,从而使用户能用语音识别和语音合成对通知信息进行差异性处理.实例验证了此模型的有效性,该模型可在优化音频技术和通知模型的系统中推广使用.关键词:音频输入输出;可穿戴计算;信息压缩;通知模型中图分类号:TP311 文献标志码:ANotification model of wearable co mputi ng context sensiti ve audio i nfor m ation co mpressi onZ HANG Chao qin 1, MA Ji a ng tao 2, WANG Li b i n3(1.Colle ge of Co mp.and Com.Eng.,Zhengzhou Univ .of L i ght Ind .,Zhengzhou 450002,China ;2.Colle ge of Int .Edu.,Zhengzhou Univ .of Ligh t Ind.,Zhengzhou 450002,Ch i na ;3.Zhengzhou Branch,H e nan M obile ,Zhengzhou 450002,China )Abstract :W earab le co m puti n g for traditional audio i n fo r m ation co mpressi o n m ethod by i n serting a notice leads generated by the lack o f d ifference bet w een t h e notification proble m,w e desi g ned a dyna m ic adapta ti o n based on context sensitive notificati o n m ode.l The m ode l is based around periphera ls i m plied ,dyna m ic co m pression,frequency o f use ,no tice l e vel g rade ,level and env ironm ental variables used to dyna m ically select t h e appropriate notification strategy ,so that users can use speech recogniti o n and speech synthesis on the notificati o n i n for m ati o n processi n g differences .Exa m p le sho w s the va li d ity o f th ism ode,l the m ode l can be no tified i n the opti m iza ti o n m ode l for aud i o techno l o gy and syste m s bei n g w i d ely used .Key w ords :auditory I/O;w earable co mpu ti n g ;i n f o r m ation co m pression ;notification mode l张超钦,等:可穿戴计算音频信息压缩的上下文相关通知模型0 引言在传统的可穿戴计算音频信息压缩中,信息线索通知模型对信息处理缺乏差异性,并且对用户环境的敏感度较差,缺乏协调通知的一致性.针对这些不足之处,本文拟在分析通知模型中断特点的基础上提出上下文相关的通知模型,并在开发的系统中进行验证,以期克服传统通知模型的不足.1 传统通知模型的特点和不足移动设备中断是指不是由用户发起的异步的不按时间的交互导致当前工作的停止[1].总体上,每h中断4次,每次持续时间2m i n.因此每h处理中断的时间将近10m i n,尽管大部分的中断是现场处理,但是还有20%是电话中断.在64%的中断中,用户受到有用的交互,这表明全部的屏蔽中断(如1d中关掉电话一段时间)会阻止有益的中断响应.用户认为41%的中断响应没有当前工作的优先级别高,使用新的通信工具会使用户更容易被不期望的中断打断.每一台设备都有一些独特的通知形式,其在许多情况下是不同的听觉线索.大部分线索具有二元性质,即它们只转达发生的通知,而没有紧迫性或动态特征,这样用户就无法辨别出哪些通知是最重要的.1.1 对用户和环境的意识性弱这类通知在发生时不考虑用户的当前状态或其关注的焦点,因此中断会话会干扰用户的工作思路.为了避免不必要的尴尬,用户通常会在会议或讲座中关掉手机,而这样会阻止用户及时获得信息.1.2 缺乏从先前的用户互动中学习这种系统通常没有依据用户的积极的或者消极的行动来调整的机制.比如,用户在谈话时,无视它有一段时间了,而手机却一直在振铃.1.3 通知缺乏协调一致性所有的设备在没有任何协调一致通知的情况下竞争用户的唯一响应.如果同一个小的时间段内有2个或以上通知同时响应,用户就会混淆从而使通知落空.用户会因为不停地关注这些移动设备,经常被打断而影响了日常工作.以上原因导致设备无法达到通知的目的,在它的周期中也不能有效地运行,新的用户看到这些明显的问题就不会选择这些设备.另外,在很多情况下,用户会关掉这些设备从而影响设备的正常工作.2 基于上下文相关的动态适应通知模型针对传统通知模型的不足,本文提出基于上下文相关的动态适应通知模型.此模型能根据信息优先级、使用水平和环境变量来动态地选择合适的通知策略.此系统必须通过观察用户的当前活动(如与设备进行交互或在办公室谈话等)来推断用户的目的.用户的优先响应还必须考虑适合通知时间的长短.此模型使用音频I/O来存储可穿戴设备的信息.在一个导游扩音器原型[2]中根据浏览者在博物馆的位置来播放录音,扩音设备[3]使用户能用语音识别和语音合成来操作或检索录音数据.预测音频开发了根据背景音频信息来推测人在工作场所中的位置.在本模型中,用户推断文本的目的不是定位而是在什么时候或者以什么方式传送通知音频信息.本文采用可变的音频展示和面向上下文相关的参数化方法,动态适应模型包括以下几个部分. 2.1 压缩音频技术压缩音频必须有足够的信息并且最少地打断接听者.本系统的信息通知可分为7个等级,即静音、周围暗示、听觉暗示、信息摘要、预览、全文和突出.2.2 外围设备的周围暗示技术在本系统中外围音频暗示一直在记录系统的状态和信息被下载的状态.外围的流水声表明系统是激活状态(静音是睡眠模式),此声音过一段后会渐变为背景音,因此它不会分散接听者的注意力.在下载过程中前台设置为外围声音,一个短的电子邮件信息声音像泼水声,而一个2m i n的新闻摘要在下载过程中像快速流水声.这些外围设备减少相关的通知信息,将其变为明显的音频暗示,无疑会增加接听者的负担.2.3 动态压缩技术在本模型中,动态地压缩文本信息使用的主要的上下文线索包括:从电子邮件过滤得到的信息优105第6期郑州轻工业学院学报(自然科学版)先级;基于上次用户动作后的使用频率;由实时分析音频场景而得出的对话的可能性.在实验中这些参数可提供足够的文本来压缩通知,从运动和位置传感器得到的数据同样可以集成到此模型中.根据中断的估计代价和传送给用户的信息可以使用一个线性的压缩音频通知模型,此方法类似于基于关注焦点的压缩图像模型.2.4 信息优先级信息优先级采用基于内容的电子邮件过滤线索系统[4],是一个采用过滤方法的优先级系统.此系统被集成在模型中,根据用户的日历、日程表以及发送信息记录和电话来决定信息的属性.这些集成的静态规则由用户的优先级和信息主题决定,当新的电子邮件到来时,从发送者的关键字和主题头信息来确定过滤规则并赋于信息优先级.如果用户旅行或者在同一地区开会(可以通过电话号码区号),其电子邮件信息同样会被优先级化.当前优先级为:组、个人、非常重要、一般重要和及时.参数化的优先级用0到1之间的对数表示,对数压缩能保证高优先级的信息用较高的权值表示.2.5 使用频率用户上次与设备的交互决定了设备的使用频率,如果对系统下达声音命令或者浏览系统上的信息,用户就很可能听到新的通知或者语音反馈.每个用户行为都带有时间戳,并且计时器能比较自从上次行为后的值.它将到达时间与上次行为时间比较,并且压缩睡眠时间(默认为15m i n),高使用率的信息赋值接近1,将睡眠之后到达的信息使用率赋值为0.对数压缩比例方法能保证任何持续时间内接近睡眠时间的行为有更一致的使用率.因此,对于10~60s的行为比10~15m in延迟的行为有更少的通知影响.使用上次行为设置使用率的问题是,如果用户休眠一个信息,此信息获得时间戳,因此负面行为(如停止音频播放或者休眠会话)会在计算使用率时被排除在外[5].2.6 通知级别等级3个上下文线索的加权平均值提供所有的通知级别,会话级别有一个可以反转的通知关系,比如在高级别的会话中,一个低级别的通知必须被提供.此通知级别必须压缩成离散量来播放信息.目前有7个通知级别:最重要、全文信息、预览、摘要、音频线索、周围环境和静音.通知级别必须按照7个阈值来适当地播放信息,以通知级别的最大值的1/2来确定不同中断的通知级别值的阈值范围.3 上下文相关的动态适应通知模型的验证为了验证此模型的有效性,本模型应用于一个可穿戴的计算平台,此平台提供一个统一的、只有音频的接口,此接口提供远程服务及电子邮件、声音邮件、每小时广播和个人日历备忘信息.这些信息自动下载到设备上,用户可以使用语音命令或者触摸输入来浏览这些信息.此平台使用基于Java的客户端和用C或Perl语言开发的远程服务器来连接无线网,并在语音接口组中使用电话结构.使用基于HRTF的Java API来接收音频信息,语音I/O 使用AT&T W atson Speech API.为了使用户解放双手并使接口友好,平台只采用音频设备.在用户的肩膀上安放1个喇叭,胸前1个麦克风.信息和反馈通过音频信息、空间音频信息和合成语音的组合提供给用户.在可穿戴计算中集成各种音频技术无需用手获取信息以及根据信息通知进行导航.本系统中集成一个只面向音频的接口,并且此系统中还有为可穿戴接入设计的统一信息的网络结构.此系统控制着穿在用户身上的100个微型移动PC,关键是减少对接听者的干扰,同时根据相关的文本信息及时提供通知.本系统采用基于上下文相关的动态适应通知模型来实现通知模块.用户可以把通知模型的权重初始化为高级、中级或者低级(中断),这些权重根据实验通知交互视频化的参数来确定.通过观察模型、修改权重从而根据不同的权重策略来推断对通知的影响.预先确定的权重为新启动系统的新用户提供适当的模型行为,系统也允许用户根据播放或忽略动作行为来矫正权重(改变中断和通知级别).同时允许根据通知期间的用户行为来加减权重,如果计算的通知级别高于必要的音频线索阈值,当信息到来时系统播放音频线索,使用计算的延迟间隔来等待何时播放摘要或预览信息.在此期间,用户1062010年张超钦,等:可穿戴计算音频信息压缩的上下文相关通知模型可以通过语音或按钮命令请求更早播放信息或者放弃通知,如果放弃,所有的权重会减少一定的比例(默认为5%).如果用户在得到通知60s之内激活信息,播放比例会增加所有的权重;如果信息被忽略,权重没有变化,此信息会在60s内处于激活状态,用户的行为同样可以影响权重.3.1 通知模型的有效性验证可穿戴计算的外围设备通知模型必须根据长期的有效性来评价,但是,预测有效性通知模型必须定量计算,因此需要对所有的信息和通知参数进行分析.不断地增强性能使系统达到稳定和健壮的状态来满足用户的通知需求.当前的用户行为主要为后来的信息矫正权重,但是有效的强化学习需要一个通知策略来处理长期的增强方法.3.2 测试评估模型测试结果如图1所示.由图1可以看出:上下文通知模型的反馈时间最低20m s,最高50m s,而传统通知模型的反馈时间1~10m s.上下文通知模型与传统通知模型相比,前者在得到信息后所拥有的反馈时间大大提高,与单纯的语音反馈相比,用户发现外围音频线索可使用户有足够的时间来关注到来的信息.熟悉音频线索非常必要,与不同的音频音调相比,用户更喜欢长的、渐变的通知.信息的优先级没有把信息以合理的方式处理好,用户建议把信息优先级加入到外围音频中,建议用一个包括外围设备、声音线索和谈话文本的简单通知来代替.用户强调外围音频效果最好而需要的最小的开销,希望一直听到外围音频来确保系统良好.启动暂停键时,挂起正在参与会话的所有信息,周期等图1 模型测试结果待队列中微弱的没有阅读信息的音频报警.4 结语本系统采用外围设备的周围暗示技术、动态压缩信息技术,根据信息的优先级、信息的通知级别设计了基于上下文相关的动态适应通知模型.通过系统测试,此模型较好地解决了可穿戴计算的音频信息压缩及信息通知问题,可以应用到优化音频技术和通知模型的系统中.参考文献:[1] 罗森林,潘丽敏.情感计算理论与技术[J].系统工程与电子技术,2003,25(7):905.[2] 盛卫华,祝纯.人机交互中基于可穿戴式计算的手势和活动辨识[J].山东大学学报:工学版,2010,40(3):37.[3] 夏侯士戟,陈东义.基于可穿戴计算的现场作业辅助模式[J].计算机集成制造系统,2007(11):2270. [4] 张展,慈轶为.基于A d H oc的可穿戴计算机信息采集软件设计[J].计算机工程,2005(11):173.[5] 刘宏伟,杨孝宗,曲峰,等.可穿戴计算机多媒体任务处理技术研究[J].计算机工程与应用,2003(2):35.107第6期。
资料_基于分裂Bregman算法的玉米种子品种识别(英文版)

第28卷增刊2农业工程学报V ol.28Supp.2 2482012年10月Transactions of the Chinese Society of Agricultural Engineering Oct.2012Variety identification of corn seed based onBregman Split methodJiang Jingtao1,Wang Yanyao1,Yang Ranbing1,Mei Shuli2(1.College of Mechanical and Electrical Engineering,Qingdao Agricultural University,Qingdao266109,China;2.College of Information and Electrical Engineering,China Agricultural University,Beijing100083,China)Abstract:Corn seed purity is closely related to corn yield,so seed selection plays an important role in improving grain yield product.The automatic seed selection procedure based on the machine vision is usually divided into three steps: image segmentation,feature extraction and classification.Variational model for image segmentation and corresponding numerical technique of Split Bregman method were introduced into the identification procedure,which had advantages of feature extraction such as high accuracy and closed continuous border.In addition,the adaptive wavelet collocation method was employed to solve the optimality conditions in Bregman split method.Based on the improved method,the corn geometric features can be extracted more precisely.Nongda108and Ludan981were taken as examples to test the new method.Based on a classifier designed with SVM,results showed the identification accuracy of Nongda108and Ludan981were97.3%and98%,respectively,better than95%in previous research.Key words:image recognition,feature extraction,models,Bregman split method,multi-levels wavelet interpolation operator doi:10.3969/j.issn.1002-6819.2012.z2.043CLC number:TN911173;O55113Document code:A Article ID:1002-6819(2012)-Supp.2-0248-05 Jiang Jingtao,Wang Yanyao,Yang Ranbing,et al.Variety identification of corn seed based on Bregman Split method[J].Transactions of the Chinese Society of Agricultural Engineering(Transactions of the CSAE),2012,28(Supp.2):248-252.(in English with Chinese abstract)江景涛,王延耀,杨然兵,等.基于分裂Bregman算法的玉米种子品种识别[J].农业工程学报,2012,28(增刊2):248-252.0IntroductionIt is well known that grain seed purity is close related to the grain output[1-2].Seed identification can be both a science and an art.Some seed scientists use “seed keys”to identify seeds[3-4],others visualization, and most use both depending upon what experience they have in the field and what they are trying to identify.Unfortunately,only the most common agricultural and weed seeds have been described, drawn,or photographed.And so it is hard to identify the less common seeds by this method.For any seeds, there are some important characteristics need to be identified,such as size,shape,texture,color[5-7].When it comes to size,both the overall size of the seed and the size of each of the seed's individual parts[8]should Received date:2012-05-09Revised date:2012-08-20Foundation item:Special Fund for Agro-scientific Research in the Public Interest,China(No.201203028);The“Twelfth Five-Year”National Science and technology support program,China(No.2012BAD35B02). Biography:Jiang Jingtao(1963-),Female,Qingdao,Professor,Major in College of Mechanical and Electrical Engineering,Qingdao Agricultural University,Qingdao266109.Email:******************.※Corresponding author:Mei Shuli(1968-),Male,Beijing,Major in Computer Science,College of Information and Electrical Engineering, China Agricultural University,Beijing100083.Email:****************.be considered.Corn identification needs such a large amount of time and effort that it’s necessary to develop the automatic identification of corn seed based on machine vision.In general,the automatic identification procedure includes image acquisition and segmentation[9],seed geometric and color features extraction,seeds classification.Obviously,the corn seed identification precision is up to the image segmentation precision.In fact,segmentation and object extraction is one of most important tasks in image processing and computer vision[10].Many of the most general and effective segmentation methods can be written as variational based models such as fuzzy connectedness, watershed algorithm[11],Bayesian methods[12],Otsu’s method[13].This category of variational models has been proved to be very effective in many applications, especially in the processing and analysis of medical images[14].While there are many disparate approaches to image segmentation,this paper will focus on recently proposed methods which can be cast in the form of totally convex optimization problems and the corresponding numerical method-split Bregman method[15].Combined with the classifier based on support vector machine(SVM)[16],a novel corn seed增刊2江景涛等:基于分裂Bregman 算法的玉米种子品种识别249varieties intelligent identification system will be constructed.1The split Bregman method on the globally convex segmentation1.1Convex methods for image segmentationThe gloval convex segmentation (GCS)method ,first proposed by Chan et al.[17],eliminate difficulties associated with those non-convex models by proposing an approach to segmentation based on convex energies.The GCS formulation based on the gradient flow can be described as follows:2212[()()]||u u c f c f t u μ∂∇=∇----∂∇(1)Where u is the level set function,μis a constant variable,t is the time parameter,f is the image intensity,c 1and c 2represent the mean intensity inside and outside of the segmented region,respectively [18].The strength of the regularization can be controlled by the parameter.This simplified flow represents the gradient descent for minimizing the energy1()||,E u u u r μ=∇+<>(2)where 2212()()r c f c f =---.To make the global minima well defined,we must constrain thesolution to lie in the interval [0,1].This results in the optimization problem:011min ||,u u u r ∇+<>≤≤(3)Once this optimization problem is solved,thesegmented region can be found by thresholding the level set function to get{;()}x u x αΩ=>(4)for some α∈(0,1).1.2Split Bregman method on GCSIn fact,it’s difficult to get the minimize of the model (2).Goldstein and Osher [15]proposed to enforce the inequality constraint using an exact penalty function.Then,the convexified segmentation can be reduced to a sequence of problems of the form01min ||,u g u u r μ∇+<>≤≤(5)where r =(c 1−f )2−(c 2−f )2.In order to apply the Split Bregman method,the auxiliary variable d wasintroduced,that is,dcan be employed to take theplace of u ∇.To weakly enforce the resulting equality constraint,a quadratic penalty function was added,the following unconstrained problem can be got:01,**(,)arg min ||,2u dg u d d u r d u λμ=+<>+-∇ ≤≤(6)In order to strictly enforce the constraint d u -∇,Bregman iteration can be applied to the problem.Theresulting sequence of the optimization problme is01,112(,)arg min ||,2u dk k g k u d d u r d u b μλ++=+<>+-∇-≤≤(7)1k k kkb b u d +=+∇- (8)To the Optimization problem in Eq.(7),the optimization condition can be described as()u r d b μλ∆=+∇⋅- If the solution to this equation lies in the interval [0,1]then this global minimizer coincides with the minimizer of the constrained problem.If the solution lies outside of this interval,then the energy is strictly monotonic inside [0,1],and the minimizer lies at the endpoint closest to the unconstrained minimizer.We have the following element-wise minimization formula:,1,,1,,,1,,1,,1,1,1,1,1,,,1()4max{min{,1},0}x x x x y y y yi j i j i j i j i j i j i j i j i ji j i j i j i j i j i j i j i j d d b b d d b b u u u u u αμβαλβ-----+-+=--++--+=+++-=Minimization with respect to dis performed using the following formula:11(,)k k k g d shrink b u λ++=+∇1.3Maize image segmentation experimentIn order to examine the effectiveness of the split Bregman method on the globally convex segmentation,it was applied to segment the maize image,as shown in Fig.1.The purpose of method is to find the maize shape,the exact edge and the color information.The segmentation results were shown inFig.2~3.Fig.1Original maizeimageFig.2Segmentation with the Split Bregman method农业工程学报2012年250Fig.3Contour of the maize image with split Bregman method Compared with the watershed method shown in Fig.4,the segmentation result with split Bregman method was more accurate and had closed continuous border,which would be helpful in measuring the geometric feature of the maize images.But we can’t get the different regions with different color.If we can get them,the more features of the maize image can be obtained,which are helpful in identification of the maizeseeds.Fig.4Watershed method2Modified split Bregman method based on the morphological reconstructionMorphological reconstruction is a useful but little-known method for extracting meaningful information about shapes in an image.The shapes could be just about anything:letters in a scanned text document,fluorescently stained cell nuclei,or galaxies in a far-infrared telescope image.We can use morphological reconstruction to extract marked objects,find bright regions surrounded by dark pixels, detect or remove objects touching the image border, detect or fill in object holes,filter out spurious high or low points,and perform many other operations.Essentially a generalization of flood-filling, morphological reconstruction processes to an image, which can be called the marker,based on the characteristics of another image,called the mask.The high points,or peaks,in the marker image specify where processing begins.The peaks spread out,or dilate,while being forced to fit within the mask image. The spreading processing continues until the image values stop changing.If G is the mask and F is the marker,the reconstruction of G from F,denoted by R G(F),is defined by the following iterative procedure:1)Initialize h1to be the marker image,F.2)Create the structuring element:B=ones(3).3)Repeat:1()k kh h B+=⊕∩Guntil h k+1=h k.4)R G(F)=h k+1Fig.5~6illustrate the preceding iterative procedure.Although this iterative formulation is useful conceptually,much faster computational algorithmsexist.Fig.5Modified regionalmaximaFig.6Opening-closing by reconstructionAfter the morphological reconstruction,we can segment the maize images with the split Bregman method,the result was shown in Fig.7.It’s easy to observe that the modified method can identify the different color regionsexactly.Fig.7Modified split Bregman method combing withmorphological reconstruction增刊2江景涛等:基于分裂Bregman 算法的玉米种子品种识别2513Multi-Object feature extractionIn order to quantitatively describe the color information of maize seeds,six color features were defined as the mean values of Red,Green,Blue color,the mean value of the Hue,Saturation,bining with the geometric features measured with the segmentation results.Table 1shows parts of the geometric feature parameters of two varieties of maize seeds,Nongda 108and Ludan 981.Table 2shows the color features.Table 1Geometric feature parameter of maize seedsGeometric feature Nongda 108Ludan 981Contour points amount834756Circumference952878Area 6003448061Length of long-axis 336287length of minor-axis 256241Maximum inscribed circle radius 162134Minimum inscribed circle radius121112Largest span 321243Equivalent diameter276241Table 2Mean value of color feature parameter for maizeseedsColor featureNongda 108mean valueLudan 981mean valueR227218G 195181B 127132H0.880.66S 0.150.036I1821764Corn seeds identification with SVMSupport Vector Machine (SVM)is a classification and regression prediction tool that uses machine learning theory to maximize predictive accuracy while automatically avoiding over-fit to the data.SVM can be defined as systems which use hypothesis space of a linear functions in a high dimensional feature space,trained with a learning algorithm from optimization theory that implements a learning bias derived from statistical learning theory.Here we present the QP formulation for SVM classification.This is a simple representation only.SV classification:i2i Kf,1min fC li ξξ=+∑y i f (x i )≥1−ξi ,for all iξi ≥0SVM classification,dual formulation:1111min (,)2i ll li i j i j i j i i j y y K x x αααα===-∑∑∑0≤αi ≤C ,for all i:1li ii yα==∑Variables ξi are called slack variables and they measure the error made at point (x i ,y i ).Training SVM becomes quite challenging when the number of training points is large.A number of methods for fast SVM training have been proposed.Applying the feature parameters extracted in section 3,we can construct a classfier of corn seeds identification based on the SVM ing the varieties identification classifier,the test of single variety identification and mixed varieties identification are done to 2varieties maize seeds such as Nongda108and Ludan981.The identification accuracy of Nongda108and Ludan981by the method were 97.3and 98%,respectively,showing that the identification accuracy was improved than that by Shi [19],in which the identification accuracy of Nongda108and Ludan981were about 95%,respectively.5ConclusionsThe variational models for image segmentation and the corresponding split Bregman method were first employed to identify the corn seed variety in this paper,and the result showed the methods had advantages of high accuracy and closed continuous border .In fact,the reason that the method can improve the seeds identification precision is that image segementation results are more precise.Future research will focus on accelerating the Split Bregman scheme in the case of fidelity parameters,allowing for faster coarse segmentation of large images,and faster evolution of the GAC contour.[References][1]Yan Xiaomei,Liu Shuangxi,Zhang Chunqing,et al.Purity identification of maize seed based on color characteristics[J].Transactions of the Chinese Society of Agricultural Engineering (Transactions of the CSAE),2010,26(Supp.1):46-50.[2]Huang Yanyan,Zhu Liwei,Li Junhui,et al.Rapid and nondestructive discrimination of hybrid maize seed purity using near infrared spectroscopy[J].Spectroscopy and Spectral Analysis,2011,31(3):661-664.[3]Bedane G M,Gupta M,George D,et al.Optimum harvest maturity for guayule seed[J].Industrial Crops and Products,2006,24(1):26-33.[4]Bedane G M,Gupta M L,George D L,et al.Effect of plant population on seed yield,mass and size of guayule[J].Industrial Crops and Products,2009,29(1):139-144.农业工程学报2012年252[5]Granitto P M,Verdes P,Ceccatto H rge-scaleinvestigation of weed seed identification by machinevision[J].Computers and Electronics in Agriculture,2005, 47(1):15-24.[6]Kovinich N,Saleem A,John A,et al.Identification oftwo anthocyanidin reductase genes and three red-brownsoybean accessions with reduced anthocyanidin reductase1mRNA,activity,and seed coat proanthocyanidinamounts[J].Journal of Agricultural and Food Chemistry,2012,60(2):574-584.[7]Liu Zhaoyan,Cheng Fang,Ying Yibin,et al.Identification of rice seed varieties using neuralnetwork[J].Journal of Zhejiang University:Science,2005,6(11):1095-1100.[8]Yi S,Davis B J,Robb R A.A method for size estimationfor small objects and its application in brachytherapy seedidentification[J].Proceedings of SPIE-The InternationalSociety for Optical Engineering,2004,5370(3):1679-1684.[9]Zhang Junxiong,Wu Kebin,Song Peng,et al.Imagesegmentation of maize haploid seeds based on BP neuralnetwork[J].Journal of Jiangsu University(NaturalScience Edition),2011,32(6):621-625.[10]Lin Haibo,Dong Shuliang,Qiu Yan,et al.Research ofwheat precision seeding test system based on imageprocessing[J].Advanced Materials Research,2011,311-313:1559-1563.[11]Kuang Fangjun,Xu Weihong,Wang Yanhua.Novelwatershed algorithm for touching rice image segmentation[J].Advanced Materials Research,2011,271-273:1-6. [12]Ruben A,Inaki I,Pedro L.Detecting reliable geneinteractions by a hierarchy of Bayesian networkclassifiers[J].Computer Methods and Programs inBiomedicine,2008,91(2):110-121.[13]Long Mansheng,He Dongjian.Weed identification fromcorn seedling based on computer vision[J].Transactionsof the Chinese Society of Agricultural Engineering,2007,23(7):139-144.[14]Jonasson L,Bresson X,Hagmann P,et al.White matterfiber tract segmentation in dt-mri using gemetric flows[J].Med.Image Anal.,2005,9(9):223-236.[15]Goldstein T,Bresson X and Osher S.Geometricapplications of the split Bregman method:Setmentationand surface reconstruction[J].J Sci Comput,2010,45:272-293.[16]Wu Di,Feng Lei,He Yong,et al.Variety identificationof Chinese cabbage seeds using visible and near-infraredspectroscopy[J].Transactions of the ASABE,2008,51(6): 2193-2199.[17]Chan T F,Esedoglu S,Nikolova M.Algorithms forfinding global minimizers of image segmentation anddenoising models.SIAM J[J].Appl.Math.,2006,66:1932-1948.[18]Chan T F,Vese L.Active contours witout edges[J].IEEEtrans.Image Process.,2001,10:266-277.[19]Shi Zhonghui.Research on corn seed varieties intelligentidentification system[D].Tai’an:Shandong AgriculturalUniversity,2011.基于分裂Bregman算法的玉米种子品种识别江景涛1,王延耀1,杨然兵1,梅树立2(1.青岛农业大学机电工程学院,青岛266109;2.中国农业大学信息与电气工程学院,北京100083)摘要:玉米品种的纯度和玉米产量密切相关,因此玉米品种的筛选对提高粮食产量具有非常重要的作用。
新版新视野大学英语读写教程第四册unit8~10答案
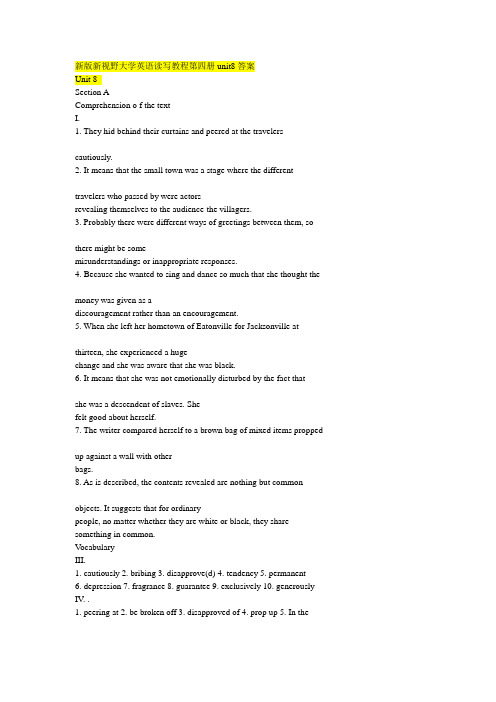
新版新视野大学英语读写教程第四册unit8答案Unit 8Section AComprehension o f the textI.1. They hid behind their curtains and peered at the travelerscautiously.2. It means that the small town was a stage where the differenttravelers who passed by were actorsrevealing themselves to the audience-the villagers.3. Probably there were different ways of greetings between them, sothere might be somemisunderstandings or inappropriate responses.4. Because she wanted to sing and dance so much that she thought themoney was given as adiscouragement rather than an encouragement.5. When she left her hometown of Eatonville for Jacksonville atthirteen, she experienced a hugechange and she was aware that she was black.6. It means that she was not emotionally disturbed by the fact thatshe was a descendent of slaves. Shefelt good about herself.7. The writer compared herself to a brown bag of mixed items proppedup against a wall with otherbags.8. As is described, the contents revealed are nothing but commonobjects. It suggests that for ordinarypeople, no matter whether they are white or black, they share something in common.V ocabularyIII.1. cautiously2. bribing3. disapprove(d)4. tendency5. permanent6. depression7. fragrance8. guarantee9. exclusively 10. generously IV. .1. peering at2. be broken off3. disapproved of4. prop up5. In themain6. in company with7. in that8. pay the price9. belong to 10. moreor lessV1. L2.O3. H4. D5.16. E7. N8. K9. G 10. BCollocationVI.1 .plans 2. lifestyle 3. fact 4. policies 5. rules6. concentration7. world8. voice9. inheritance 10. contentWord BuildingVII.1. devalued2. demobilized3. deregulated4. decentralized5. destabilizing6. depersonalized7. de-emphasize8. decodedVIII.1. retirees2. interviewees3. trainee4. employees5. escapees6. addressee7. devotee8. divorceesentence structureIX1. I know she isn't really interested in window-shopping, but shedoesn't mind waiting while I have alook.2. If people don't mind my not having a degree, I could give a fewmusic lessons!3. People don't mind paying a lot more for good software because theycan see the benefits of using it.4.1 don't mind if people perceive me as arrogant. You need certain degree of it to succeed.5. We don't mind if it takes time for people to express their hopes,goals, fears, and needs in theirlives.X.1. No friends came to see her; she shut herself off, in the oldfamiliar world of bedroom and drawingroom.2. No cigarette end could give us any clue to or sign of anyone havingever been there.3. No gas, no water and no electricity in the hospital! How could youexpect the children to survivethis?4. No other information is given about the book itself, although thereis a brief biographical, note aboutthe writer.5. No effort has been made to investigate the "disappearances" thathave occurred over the past threeyears.TranslationXI.1. 1 don't mind his staying out so late as long as he behaves himself.2. 1 prefer his plan to others in that I think no plan is morepractical than his.3. They broke off business relations with that company as it sufferedhuge losses in the last fiscal yearand went bankrupt.4. Now that you don't like him, why did you invite him to yourbirthday party in the first place?5. Though we knew our chances to win were slim, we were more or lessdepressed when we lost in thegame.6. Perhaps this was the price that has to be paid for progress-whoknows?XII.1.我不在乎他们否定我的计划,但在内心深处我仍渴望得到他们的支持。
adaptivethreshold 偏移量 -回复
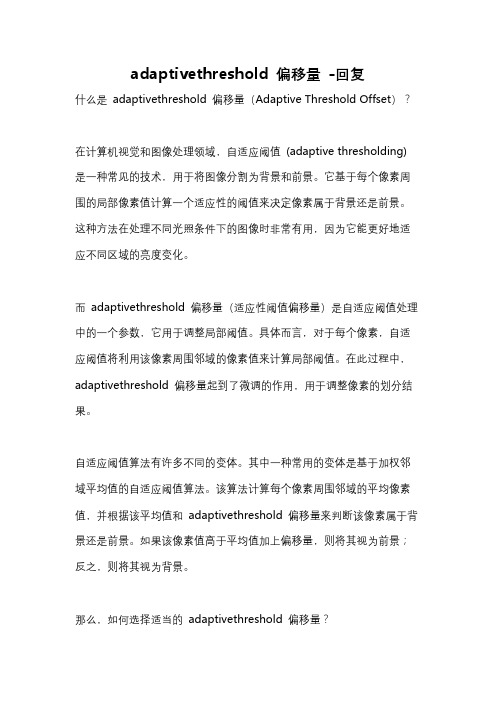
adaptivethreshold 偏移量-回复什么是adaptivethreshold 偏移量(Adaptive Threshold Offset)?在计算机视觉和图像处理领域,自适应阈值(adaptive thresholding) 是一种常见的技术,用于将图像分割为背景和前景。
它基于每个像素周围的局部像素值计算一个适应性的阈值来决定像素属于背景还是前景。
这种方法在处理不同光照条件下的图像时非常有用,因为它能更好地适应不同区域的亮度变化。
而adaptivethreshold 偏移量(适应性阈值偏移量)是自适应阈值处理中的一个参数,它用于调整局部阈值。
具体而言,对于每个像素,自适应阈值将利用该像素周围邻域的像素值来计算局部阈值。
在此过程中,adaptivethreshold 偏移量起到了微调的作用,用于调整像素的划分结果。
自适应阈值算法有许多不同的变体。
其中一种常用的变体是基于加权邻域平均值的自适应阈值算法。
该算法计算每个像素周围邻域的平均像素值,并根据该平均值和adaptivethreshold 偏移量来判断该像素属于背景还是前景。
如果该像素值高于平均值加上偏移量,则将其视为前景;反之,则将其视为背景。
那么,如何选择适当的adaptivethreshold 偏移量?选择适当的adaptivethreshold 偏移量是一个关键的步骤,它直接影响到自适应阈值处理的结果。
一个合理的偏移量应该能够在准确分割图像的同时,保留足够的图像细节。
在实际应用中,选择适当的偏移量通常需要进行实验和调试。
一种常见的方法是基于视觉效果进行手动调整,观察不同偏移量下的分割结果,并选择最佳的效果。
这种方法虽然简单直观,但需要大量的人工实验和主观判断,不够自动化和准确。
另一种更为智能的方法是基于图像统计特征进行自动优化。
通过分析图像的统计信息,可以确定某个范围内的像素值分布情况,并根据这些信息选择适当的偏移量。
这种方法通常结合了数学模型和启发式算法,通过学习和迭代优化来确定最佳偏移量。
PCV-2V压力释放阀,直接操作手动可调说明书

w e e n g i n e e r y o u r p r o g r e s sTable of Contents1 Product Details .....................................................................................................................................................................................2 1.1 Application ............................................................................................................................................................................................. 2 1.2 Recommended Installation .................................................................................................................................................................... 2 2 Function ................................................................................................................................................................................................ 2 2.1 Features ................................................................................................................................................................................................. 23 Technical Data ...................................................................................................................................................................................... 34 Ordering Information ........................................................................................................................................................................... 3 4.1 Type Code ............................................................................................................................................................................................. 3 4.2Versions currently available (3)5 Description of Characterisics in Accordance with Type Code ........................................................................................................ 4 5.1 Characteristic 1: Variant DSU ................................................................................................................................................................ 4 5.2 Characteristic 2: Port / Case: Variant CA - Cartridge ............................................................................................................................. 4 5.3 Characteristic 3: input flow rate .............................................................................................................................................................. 4 5.4 Characteristic 4: Max.permissible pressure ........................................................................................................................................... 4 5.5 Characteristic 5: Activation / Setting ...................................................................................................................................................... 4 5.6 Characteristic 6: Stepped cavity 8.00239 (corresponds to Bucher UVP- 4) ......................................................................................... 4 Das vorgesteuerte Druckbegrenzungsventil ist ein Cartridgebauteil und wird in eine Stufenbohrung entsprechend nebenstehender Zeichnung eingeschraubt. ..................................................................................................................................................................................................... 4 5.7 Characteristic 7: Seal ............................................................................................................................................................................. 4 6 Installation ............................................................................................................................................................................................ 5 6.1 General information ............................................................................................................................................................................... 5 6.2 Connection Recommendations .............................................................................................................................................................. 5 6.3 Installation - installation space ............................................................................................................................................................... 5 7 Notes, Standards and Safety Instructions ......................................................................................................................................... 5 7.1 General Instructions ............................................................................................................................................................................... 5 7.2 Standards ............................................................................................................................................................................................... 58 Zubehör .................................................................................................................................................................................................5w e e n g i n e e r y o u r p r o g r e s s1The pressure valve is designed as cartridge valve. It is a direct operated valve for flow rates up to 10 l / min, which can be adjusted manually. The adjustment can be protected by a cap. The components are designed robust. The valve can be charged up to 500 bar and is delivered at a certain pressure.1.1 ApplicationThe pressure valve is used to protect high volume lift cylinders in truck cranes. It should avoid excessive pressure increase in unmoving cylin-ders due to warming (“sushine valve”).1.2 Recommended Installation2 FunctionThe pressure valve operates as a direct acting seat valve. The pressure can be set using an adjusting screw. The screw is locked after adjustment with a backup sealing nut and can be protected by a cap.2.1 Features▪ Cartridge type▪ Small installation space ▪ Robust construction▪Stepped cavity (corresponds to Bucher UVP-4) ▪Seat valve, leakage freeP – protected port T - tankw e e n g i n e e r y o u r p r o g r e s s3 Technical Data4 4.1 Type CodeXXX – fest vorgegebene Merkmale XXX – vom Kunden wählbare Merkmale4.2 Versions currently availableThe versions listed below are available as standard. Further versions as part of the options given on the type code can be configured upon request.designationtype codepart nr.PRV –DSU –CA -10LPM -500BAR –MAN230BAR –239 -NBR PRV –DSU –CA -10 -500 –MAN230 –239 -N 412.072.451.9 PRV –DSU –CA -10LPM -500BAR –MAN235BAR –239 -NBR PRV –DSU –CA -10 -500 –MAN235 –239 -N 412.072.430.9 PRV –DSU –CA -10LPM -500BAR –MAN290BAR –239 -NBR PRV –DSU –CA -10 -500 –MAN290 –239 -N 412.072.433.9 PRV –DSU –CA -10LPM -500BAR –MAN340BAR –239 -NBR PRV –DSU –CA -10 -500 –MAN340 –239 -N 412.072.431.9 PRV –DSU –CA -10LPM -500BAR –MAN420BAR –239 -NBR PRV –DSU –CA -10 -500 –MAN420 –239 -N 412.072.432.9CriteriaUnit Value Installation position any Weightkg 0,1Surface protectiveZinc coated Maximum input pressure (P) bar 550Adjustable pressurebar 100 - 500 Maximum Tankpressure (T) bar 8 Maximum input flow rate (P) l/min 10Hydraulic fluidMineral oil (HL, HLP) conforming with DIN 51524, other fluids upon re-Hydraulic fluid pressure range °C -25 bis +80 Ambient temperature °C < +50 Viscosity rangemm2/s 2,8 - 500Contamination gradeFiltering conforming with NAS 1638, class 9, with minimum retentionPRVDSUCA10500239N000102030405060700 Product group Pressure relief valves PRV 01 Variant manual adjustable DSU 02 Port / Case Cartridgeventil CA 03 Input flow rate Qmax.10 l/min 1004 Max.permissible pressure Pmax.. 500bar50005 Activation Man ually adjustable 100-500barMAN100 06 Stepped cavity WESSEL-Patrone 8.00239 (stepped cavity) 239 07 Seal NBR, temperatur range -25°C bis +80°CNw e e n g i n e e r y o u r p r o g r e s s5 5.1 Characteristic 1: Variant DSUAdjustable pressure relief valve5.2 Characteristic 2: Port / Case: Variant CA - CartridgeAs variant CA, the valve is delivered as a cartridge valve. The Cavity has to be designed according to characteristic 6 (stepped cavity)5.3 Characteristic 3: input flow rateRecommended maximum flow rate of 10 l/min.5.4 Characteristic 4: Max.permissible pressureMaximum permissible pressure is 500bar (adjustable range100 - 500bar)5.5 Characteristic 5: Activation / SettingThe valve can be adjusted with a set screw. For this purpose, the protective cap must be removed and the counter nut undone.5.6 Characteristic 6: Stepped cavity 8.00239 (corresponds to Bucher UVP- 4)Das vorgesteuerte Druckbegrenzungsventil ist ein Cartridgebauteilund wird in eine Stufenbohrung entsprechend nebenstehender Zeichnung eingeschraubt.5.7 Characteristic 7: SealNBR, temperature range -25°C bis +80°Cw e e n g i n e e r y o u r p r o g r e s s6 Installation6.1 General information▪ Observe all installation and safety information of the construction machine / attachment tools manufacturer. ▪ Only technically permitted changes are to be made on the construction machine. ▪ The user has to ensure that the device is suitable for the respective application. ▪ Application exclusively for the range of application specified by the manufacturer. ▪ Before installation or de-installation, the hydraulic system is to be depressurized. ▪ Settings are to be made by qualified personnel only.▪ Opening is only to be performed with the approval of the manufacturer, otherwise the warranty is invalidated.6.2 Connection RecommendationsNOTE : Enclosed proposed resolution is not always guaranteed. The functionality and the technical details of the construction ma-chine must be checked.5.3 Montage – BauraumObserve connection names.Do not damage seals and flange surface. Its hydraulic system must be ventedEnsure sufficient free space for setting and installation work6.3 Installation - installation space▪ Observe connection names.▪ Do not damage seals and flange surface. ▪ Its hydraulic system must be vented▪ Ensure sufficient free space for setting and installation workCAUTION: Hydraulic hoses must not touch the pressure relief valve, otherwise they are subject to thermal damaging. Tightening torques must be observed. Torque wrench needed.77.1 General Instructions▪The views in drawings are shown in accordance with the European normal projection variant▪ A comma ( , ) is used as a decimal point in drawings ▪All dimensions are given in mm7.2 StandardsThe following standards must be observed when installing and operating the valve:▪ DIN EN ISO 13732-1:2008-12, Temperatures on accessible surfaces8 ZubehörSafety cap: 275.066.000.6。
UCD3138中文版

3.6 上电复位 (POR) / 掉电复位 (BOR)
3.7 典型的门控时钟省电
3.8 典型温度特性
4 功能概览
4.1 ARM 处理器
4.2 存储器
4.2.1
CPU 存储器映射和中断
4.2.1.1 存储器映射 (在复位操作之后)
4.2.1.2 存储器映射 (常规操作)
4.2.1.3 存储器映射 (系统和外设模块)
– 脉宽分辨率为 250ps – 频率分辨率为 4ns – 相位分辨率为 4ns – 输出间的可调相移 – 配对间的可调死区 – 逐周期占空比匹配 – 高达 2MHz 开关频率 • 可配置的 PWM 边沿运动 – 后缘调制 – 前缘调制 – 三角调制 • 可配置的反馈控制 – 电压模式 – 平均电流模式 – 峰值电流模式控制 – 恒定电流 – 恒定功率 • 可配置调制方法 – 频率调制 – 相移调制 – 脉宽调制 • 快速、自动和平滑模式开关 – 频率调制和 PWM – 相移调制和 PWM • 高效和轻负载管理 – 突发模式 – 理想的二极管仿真
4.15.1 JTAG 用于 I/O 和 JTAG 安全
4.22 均流控制
4.23 温度参考
5 IC 接地和布局建议
6 工具和文件
7 参考文献
修正历史
UCD3138
ZHCS429B –MARCH 2012 –REVISED JULY 2012
39 41 41 41 42 44 45 46 48 49 51 51 51 52 53 53 54 54 54 54 54 55 56 56 57 57 58 59 60 62 63
UCD3138
用于隔离式电源的高度集成数字控制器
数据手册
产品数据信息是当前的出版日期。产品符合规 格是德州仪器标准保修的每个条款。产品加工 不需要包括所有参数的测试。
ADAPTIVE CONTROLLER
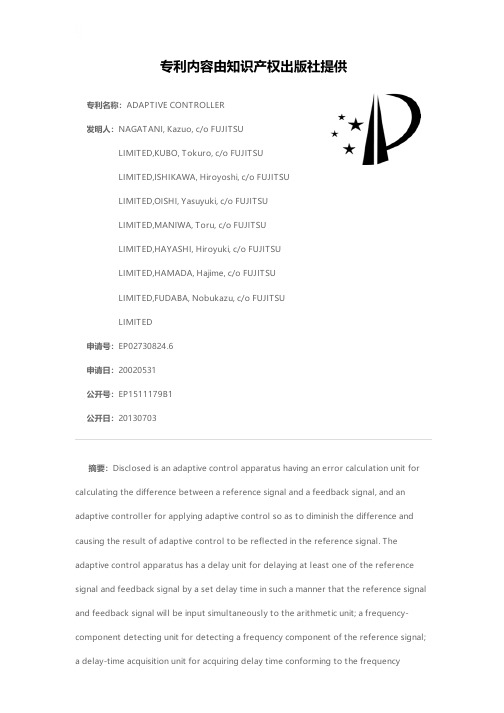
专利名称:ADAPTIVE CONTROLLER发明人:NAGATANI, Kazuo, c/o FUJITSULIMITED,KUBO, Tokuro, c/o FUJITSULIMITED,ISHIKAWA, Hiroyoshi, c/o FUJITSULIMITED,OISHI, Yasuyuki, c/o FUJITSULIMITED,MANIWA, Toru, c/o FUJITSULIMITED,HAYASHI, Hiroyuki, c/o FUJITSULIMITED,HAMADA, Hajime, c/o FUJITSULIMITED,FUDABA, Nobukazu, c/o FUJITSULIMITED申请号:EP02730824.6申请日:20020531公开号:EP1511179B1公开日:20130703专利内容由知识产权出版社提供摘要:Disclosed is an adaptive control apparatus having an error calculation unit for calculating the difference between a reference signal and a feedback signal, and an adaptive controller for applying adaptive control so as to diminish the difference and causing the result of adaptive control to be reflected in the reference signal. The adaptive control apparatus has a delay unit for delaying at least one of the reference signal and feedback signal by a set delay time in such a manner that the reference signal and feedback signal will be input simultaneously to the arithmetic unit; a frequency-component detecting unit for detecting a frequency component of the reference signal;a delay-time acquisition unit for acquiring delay time conforming to the frequencycomponent; and a delay-time setting unit for setting the acquired delay time in the delay unit.申请人:FUJITSU LTD地址:JP国籍:JP代理机构:Sunderland, James Harry更多信息请下载全文后查看。
探月返回飞行器跳跃式再入轨迹优化

第42卷第2期2021年2月㊀宇㊀航㊀学㊀报Journal of Astronautics Vol.42February ㊀No.22021探月返回飞行器跳跃式再入轨迹优化赵吉松1,王江华2,王泊乔1,张金明1,朱航标1(1.南京航空航天大学航天学院,南京210016;2.北京机电工程研究所,北京100074)㊀㊀摘㊀要:基于节点自适应稀疏配点法,提出一种高精度求解探月返回飞行器跳跃式再入轨迹优化问题的方法㊂该方法的基本策略是:首先,应用节点自适应稀疏配点法对完整的跳跃式再入轨迹进行优化;然后,根据优化得到的控制变量对再入动力学方程进行数值积分;当积分至跳跃轨迹的最高点时,以积分得到的状态变量值作为新的初始条件,对二次再入轨迹重新优化㊂仿真结果表明:1)对二次再入轨迹重新优化能够显著提高跳跃式再入轨迹的优化精度,否则轨迹优化精度低,终端误差较大;2)在跳跃轨迹的最高点进行的二次优化是一种准实时优化,在跳跃式再入轨迹的制导领域具有潜在应用价值㊂关键词:跳跃式再入;轨迹优化;二次优化;配点法中图分类号:V412㊀㊀㊀文献标识码:A㊀㊀㊀文章编号:1000-1328(2021)02-0211-09DOI :10.3873/j.issn.1000-1328.2021.02.009Skip Entry Trajectory Optimization of Lunar Return VehiclesZHAO Ji-song 1,WANG Jiang-hua 2,WANG Bo-qiao 1,ZHANG Jin-ming 1,ZHU Hang-biao 1(1.College of Astronautics,Nanjing University of Aeronautics and Astronautics,Nanjing 210016,China;2.Beijing Electro-Mechanical Engineering Institute,Beijing 100074,China)Abstract :A high-precision approach for the skip entry trajectory optimization of lunar return vehicles based on anadaptive sparse collocation method is presented.Firstly,the whole skip entry trajectory is optimized by using an adaptive sparse collocation method;then,the numerical integration of the state dynamic equations is carried out according to the obtained optimal control;when the integration reaches the highest point of the skip trajectory,the re-optimization of the secondary entry trajectory is carried out with the state values that are obtained by the integration as new initial conditions.The numerical simulation results show that:1)the optimization accuracy is significantly improved when the secondary optimization is used and otherwise the accuracy is poor and the terminal errors are large;2)the secondary optimization that is performed at the highest point of the skip trajectory is a quasi-real-time optimization which has potential applications inthe field of skip reentry trajectory guidance.Key words :Skip entry;Trajectory optimization;Secondary optimization;Collocation method收稿日期:2019-12-23;修回日期:2020-05-27基金项目:国家自然科学基金(11602107);南京航空航天大学实验技术研究与开发项目(2019051500055180)0㊀引㊀言再入返回技术是载人航天飞行的关键和难点之一㊂与从近地轨道返回不同,月球探测器的返回速度高达11km /s,火星探测器的返回速度高达14.5km /s㊂对于如此高的返回速度,飞行器如果采用直接再入的方式返回地球,最大过载高达17以上,远远超出了人的承受能力㊂跳跃式再入是减小最大过载的一种有效途径㊂如图1所示,飞行器以较小的再入角进入大气层,减速的同时利用大气提供的升力,跳出大气层,在大气层外作一段椭圆轨道飞行,然后重新再入大气层[1]㊂这种跳跃式再入方式通过两次再入减速,延长了减速时间,从而能够减小飞行器再入过程的最大过载和最大热流等[2]㊂再入轨迹优化对于探月返回飞行器总体设计和制导控制系统设计具有重要意义[3-4]㊂与常规轨迹优化问题相比,跳跃式再入轨迹优化问题的特殊之处是首次再入段的微小轨迹误差会在跳出大气层的惯性飞行段被放大,进而给二次再入段轨迹带来比较大的偏差㊂这种二次再入段对首次再入段误差的高度敏感特性给轨迹优化带来了挑战㊂目前的方法为了简化,对首次再入段和二次再入段分开优化[5-6],但是这种解耦处理方法难以保证轨迹的整体最优性㊂文献[7]采用高斯伪谱法对探月飞船跳跃式再入轨迹的可达域进行了分析,但是没有研究轨迹优化结果与数值积分结果的误差㊂此外,还有一些文献从设计的角度对跳跃式再入轨迹的相关参数影响规律[8]和能量管理方法[9]等进行了研究,但是这些方法不能充分挖掘跳跃式再入轨迹的性能㊂图1㊀跳跃式再入示意图Fig.1㊀Sketch of skip entry跳跃式再入轨迹优化问题本质上属于最优控制问题,其求解方法主要分为间接法和直接法[10]㊂间接法借助变分法或者最大值原理,把泛函优化问题转化为两点边值问题求解;直接法通过对控制变量和/或状态变量进行离散把泛函优化转化为非线性规划(Nonlinear programming,NLP)问题,然后采用各种非线性规划算法求解,比如基于序列二次规划(Sequential quadratic programming,SQP)算法的SNOPT[11]和基于内点法的IPOPT[12]㊂直接法中的配点法[10]由于不需要推导最优性必要条件,并且对初值的敏感性较低,容易收敛,近年来得到广泛研究和应用㊂此外,研究还表明,将配点法与网格细化技术相结合,能够进一步提高配点法对复杂轨迹优化问题的适应能力[13-17]㊂这种方法的原理是应用网格细化技术在优化过程中根据轨迹的特点动态调整离散节点分布,从而采用较少的离散节点达到较高的优化精度,降低了配点法的计算量㊂以文献[17]为例,该研究基于稀疏配点法和网格细化技术对近地轨道返回的高超声速滑翔再入轨迹进行了优化,研究结果表明所述方法能够快速优化出一条严格满足路径约束和端点约束的再入轨迹㊂与高超声速滑翔再入轨迹不同,探月返回飞行器的再入返回轨迹具有跳跃特性并且由于二次再入轨迹对首次再入段的误差非常敏感,给轨迹优化带来挑战㊂本文在节点自适应稀疏配点法的基础上,给出跳跃式再入轨迹的一种高精度优化方法,以探月飞行器跳跃式再入返回轨迹为例,通过数值仿真检验了所建立的轨迹优化方法的有效性㊂1㊀跳跃式再入轨迹优化问题1.1㊀再入轨迹运动模型将飞行器简化为质点,那么描述飞行器质心运动的微分方程组为(忽略地球自转影响)[10]̇r=v sinγθ㊃=v cosγcosψr cosϕϕ㊃=v cosγsinψṙv=as-g sinγψ㊃=a wv cosγ-vr cosγcosψtanϕγ㊃=a n-g cosγv+v cosγrìîíïïïïïïïïïïïïï(1)式中:r,θ,ϕ分别为飞行器在地心赤道坐标系的矢径㊁经度㊁纬度;v,ψ,γ分别为飞行器的速度㊁航向角和航迹角;g为重力加速度,g=μ/r2,μ为地球引力常数,μ=3.98603195ˑ1014m3/s2㊂空气动力产生的加速度的沿飞行轨迹切向㊁法向和侧向的三个分量a s,a n,a w分别为a s=-D m,a n=L cosσm,a w=L sinσm式中:σ为速度倾侧角,m为飞行器的质量㊂升力和阻力的表达式分别为L=12ρv2A㊃C L,D=12ρv2A㊃C D212㊀㊀㊀㊀㊀㊀㊀㊀㊀㊀㊀㊀㊀㊀㊀㊀宇航学报第42卷式中:ρ为大气密度,A 为气动参考面积,C L 和C D 分别为飞行器的升力系数和阻力系数㊂1.2㊀再入初始条件再入轨迹优化问题的初始条件如下h (t 0)=h 0,θ(t 0)=θ0,ϕ(t 0)=ϕ0v (t 0)=v 0,ψ(t 0)=ψ0,γ(t 0)=γ0{(2)式中:t 0为初始时刻;h 为飞行高度,h =r -R e ,R e 为地球半径,R e =6371.20km;r 0,θ0,ϕ0,v 0,ψ0和γ0分别为相应的状态变量的初值㊂1.3㊀路径约束再入轨迹优化问题的控制变量为速度倾侧角,即u =σ(t ),其变化范围受到如下限制σmin ɤσ(t )ɤσmax(3)式中:σmin 和σmax 为速度倾侧角的边界㊂飞行器再入返回过程的过载直接关系到宇航员的生命安全和舒适度,需要对过载进行限制n =D 2+L 2mgɤn max(4)式中:n max 为再入过程允许的最大过载㊂为了使得飞行器安全返回,需要对高超声速再入过程的对流气动加热进行限制,即Q ㊃=k qρr n()NvMɤQ ㊃max(5)式中:N =0.5,M =3,k q =1.9027ˑ10-4,r n 为飞行器头部驻点半径,Q ㊃max 为允许的热流上限㊂此外,考虑到飞行器结构的承受能力,还需要对再入过程的动压进行限制,即q =12ρv 2ɤq max(6)式中:q max 为再入过程允许的动压上限㊂1.4㊀末端约束当飞行器的速度降至预定速度时,阻力降落伞打开,飞行器进一步减速下降㊂为了安全着陆,还需要对开伞时的飞行高度进行约束㊂综上所述,再入飞行器轨迹优化问题的终端约束条件为v (t f )=v f ,h (t f )ȡh f ,min(7)式中:t f 为再入轨迹的终端时刻,v f 为开伞时飞行器的速度,h f ,min 允许的最低开伞高度㊂1.5㊀目标函数轨迹优化的目标函数可根据实际设计需求选取㊂最大横向航程是衡量飞行器再入机动能力的重要指标㊂本文选取最大横向航程作为优化指标㊂由于ϕ0=0ʎ,ψ0=0ʎ,因而目标函数可写为J =min㊀ϕ(t f )(8)式中:ϕ(t f )<0,式(8)使横向航程最大化㊂综上所述,跳跃式再入返回轨迹优化问题描述为:求解最优控制变量u (t )=σ(t ),使得目标函数最小化,并且满足再入动力学方程组(1),初始条件(2),轨迹路径约束(3)~(5)以及终端约束(7)㊂2㊀跳跃式再入轨迹优化方法2.1㊀自适应稀疏局部配点法轨迹优化问题本质上属于最优控制问题㊂以Bolza 型最优控制问题为例,可描述为:求解控制变量u (t )ɪm ,使得如下目标函数最小化J =M (x (t 0),t 0,x (t f ),t f )+ʏt f t 0L (x (t ),u (t ),t )d t(9)式中:M :n ˑˑn ˑң,L :n ˑm ˑң,x ɪn ,u ɪm ,t ɪ[t 0,t f ]⊆㊂状态方程为̇x =f (x (t ),u (t ),t ),t ɪ[t 0,t f ](10)端点条件为E (x (t 0),t 0,x (t f ),t f )=0(11)路径约束为C (x (t ),u (t ),t )ɤ0,t ɪ[t 0,t f ](12)式中:f :n ˑR m ˑңn ,E :n ˑˑR m ˑңR e ,C :n ˑR m ˑңc ㊂方程(9)-(12)所描述的问题称为连续Bolza 型最优控制问题㊂本文采用局部配点法求解轨迹优化问题㊂首先利用积分变换τ=(t -t 0)/(t f -t 0)将轨迹优化问题(方程(9)~(12))的时间区间变换至归一化的时间区间τɪ[0,1]㊂假设单位区间[0,1]上的N 个离散节点为G ={τi :τi ɪ[0,1],i =0,1, ,N ;τ0=0,τN =τf =1;τi <τi +1,i =0,1, ,N -1}(13)式中:τi 称为离散节点或网格节点,τi 在[0,1]上可以均匀分布,也可以非均匀分布㊂记x i =x (τi ),u i =u (τi ),对于状态方程(10),基于q 阶Runge-Kutta(RK)方法的离散格式为312第2期赵吉松等:探月返回飞行器跳跃式再入轨迹优化x i+1=x i+Δt㊃h iðqj=1βj f ij,(i=0,1, ,N-1)(14)式中:Δt=t f-t0,h i=τi+1-τi,f ij=f(x ij,u ij,τij;t0, t f),x ij,u ij和τij为中间变量,x ij由下式给出x ij =x i+Δt㊃h iðql=1αjl f il,1ɤjɤq(15)式中:τij=τi+h iρj,u ij=u(τij),ρj,βj,αjl均为已知常数并且满足0ɤρ1ɤ ɤρqɤ1㊂当αjl=0(lȡj)时,为显式格式,否则为隐式格式㊂采用类似的方法,可将目标函数离散化㊂常用的离散格式有梯形格式(q=2),Hermite-Simpson格式(q=3,简记为HS格式),经典四阶Runge-Kutta格式(q=4)等㊂由此离散得到的非线性规划问题是求解变量X,U,U-,t0和t f使得如下目标函数最小J=M(x0,t0,x f,t f)+ΔtðN-1i=0h iðq j=1βj L ij(16)并且满足如下约束ξi =x i+1-x i-Δt㊃h iðqj=1βj f ij=0(17)Ci=C(x i,u i,τi;t0,t f)ɤ0(18)C-ij=C(x ij,u ij,τij;t0,t f)ɤ0,τijɪG-(19)E(x0,t0,x f,t f)=0(20)式中:L il=L(x il,u il,τil),i=0,1, ,Nτ-1;X= {x0,x1, ,x Nτ},U={u0,u1, ,u Nτ};G-={τilɪ[0,1]:τil∉G,0ɤi<Nτ,1ɤlɤq};X-={x il:τilɪG-},U-={u il:τilɪG-}㊂本研究采用HS格式,该格式需要用到区间中点的变量和函数值,为此将区间中点的控制量也作为优化变量并且在区间中点添加路径约束,即G-=τ-i+1=τi+h i2,i=0,1, ,N-1{}(21) HS格式得到的NLP的优化变量为(x0,x1, ,x N; u0,u1, ,u N;u-1,u-2, ,u-N;t0,t f),目标函数为J=M(x0,t0,x f,t f)+ΔtðN-1i=0h i6(L i+4L-i+1+L i+1)(22)约束条件为ξi=x i+1-x i-Δt h i6(f i+4f-i+1+f i+1)=0(23)Ci=C(x i,u i,τi;t0,t f)ɤ0(24)C-i+1=C( x i+1,u-i+1,τ-i+1;t0,t f)ɤ0(25)E(x0,t0,x f,t f)=0(26)其中x i+1=(x i+x i+1)2+h i8(f i-f i+1)f-i+1=f( x i+1,u-i+1,τ-i+1;t0,t f)L-i+1=L( x i+1,u-i+1,τ-i+1;t0,t f)在数值优化时,为了使轨迹优化问题具有实际物理意义,还需要添加时间差约束Δt=t f-t0>0(27)求解方程(22)~(27)所示的NLP问题即可得到轨迹优化问题的最优解㊂本文采用美国斯坦福大学基于SQP算法开发的SNOPT[11]求解NLP问题㊂为了提高轨迹优化的求解效率和精度,本文应用稀疏差分算法[18]提高NLP偏导数的计算效率,应用节点细化技术[19]动态调整离散节点分布,提高对跳跃式再入轨迹的适应能力㊂在采用SNOPT求解NLP时,为SNOPT提供NLP的一阶偏导数矩阵(即雅克比矩阵,定义为目标函数和全部约束对全部自变量的偏导数矩阵)能够显著提高优化的计算效率,但是偏导数矩阵的计算量比较大㊂稀疏差分法[18]通过分析偏导数矩阵的稀疏特性,将其中的占绝大多数的零元素识别出来,并且将其中的非零元素的值通过矩阵链式求导运算分解为原始轨迹优化问题的偏导数,然后采用稀疏差分方法计算,从而大幅度减小偏导数的计算量㊂节点细化技术[19]的原理是数据压缩,每次优化出一条轨迹之后,根据轨迹的变化特性,在光滑区域对离散节点进行压缩,减少节点数量,在非光滑区域插入一些新节点,使之分布更密,然后再次优化轨迹,综合效果是采用较少的离散节点高精度描述轨迹㊂稀疏差分法和节点细化技术都具有很强的通用性和鲁棒性,对于本文的优化问题,只需要设置用于判断轨迹是否光滑的阈值参数即可(判断每个节点处的控制量与采用其周围节点的插值结果的差异是否超过阈值,若超过,则认为轨迹不光滑,否则认为轨迹光滑)㊂412㊀㊀㊀㊀㊀㊀㊀㊀㊀㊀㊀㊀㊀㊀㊀㊀宇航学报第42卷2.2㊀跳跃式再入轨迹优化方法对于跳跃式再入轨迹,由于二次再入段对首次再入段的误差非常敏感,若将首次再入段和二次再入段作为整体进行优化会导致二次再入轨迹的优化精度较低㊂本文在自适应稀疏局部配点法的基础上,提出一种能够高精度求解跳跃式再入轨迹优化问题的方法㊂该方法的关键之处是从跳跃轨迹的最高点开始,对二次再入轨迹进行重新优化㊂如图2所示,首先,将跳跃式再入轨迹作为整体进行优化,得到最优倾侧角随时间变化曲线;然后,按照最优倾侧角积分再入动力学方程组得到最优轨迹;当积分至跳跃轨迹的最高点时,以实际积分得到的状态变量作为新的初始条件(其它条件保持不变),对二次再入轨迹重新优化,求解新的最优倾侧角㊂考虑到优化计算需要耗费一些时间,为了使得方法在制导跟踪领域具有实用性,本文方法在解出二次优化问题的最优倾侧角之前,仍然采用整体优化得到的最优倾侧角作为二次再入轨迹的控制输入㊂因为在跳跃轨迹的最高点附近大气非常稀薄,气动力对轨迹的影响非常小,所以这样近似处理是合理的㊂图2㊀跳跃式再入轨迹优化策略Fig.2㊀Optimization strategy for skip entry trajectory本文方法的具体流程如下:1)应用基于稀疏差分法和节点自适应细化技术的局部配点法求解由方程(1)~(8)描述的跳跃式再入轨迹优化问题,得到最优倾侧角σ1(t )㊂2)以σ1(t )为控制变量,采用四阶Runge-Kutta 方法积分由方程组(1)~(2)描述的微分方程组初值问题至跳跃轨迹的最高点(即最大高度处),得到最高点的状态变量x 1,f 以及对应的时刻t 1,f ㊂3)以t 1,f 和x 1,f 作为新的初始条件,对由方程组(1)和(3)~(8)描述的再入轨迹优化问题进行重新优化(即二次优化),得到新的最优倾侧角σ2(t )和终端时刻t 2,f ,记下二次优化的耗时为Δt 2㊂4)从跳跃轨迹的最高点开始,以x 1,f 为初始条件,以σ1(t )为控制变量,继续积分再入动力学方程组(1)至t 2,1=t 1,f +Δt 2,得到状态变量x 2,1㊂5)以t 2,1和x 2,1为初始条件,以σ2(t )为控制变量,积分再入动力学方程组(1)至终端时刻t 2,f ㊂6)将在步骤2)㊁4)和5)中积分得到的三段轨迹(含各段采用的控制变量)组合在一起,即可得到最优跳跃式再入轨迹和相应的控制变量㊂相对于间接法和直接打靶法等其它方法,配点法的优势之一是对优化初值的敏感性较低㊂尽管如此,良好的优化初值仍然有利于加速轨迹优化的收敛㊂本文在对跳跃式再入轨迹进行第一次优化时,状态变量的优化初值选取初始状态变量和终端状态变量的连线(对于终端状态给定的变量)或者初值状态变量常值(对于终端状态没有给定的变量),控制变量的优化初值取σ(t )=-45ʎ㊂在后续的节点细化以及二次优化时,均利用前一步得到的最优轨迹通过插值提供较为准确的优化初值㊂3㊀仿真结果本文的仿真研究采用的模型参数来自文献[1,20]㊂飞行器质量m =8382kg,升力系数C L =0.3892,阻力系数C D =1.3479,气动力参考面积A =19.635m 2㊂大气密度随高度变化特性采用美国1976版标准大气模型插值计算㊂控制变量的边界约束参数:σmin =-180ʎ,σmax =0ʎ㊂再入初始条件为:h (t 0)=121.92km,θ(t 0)=0ʎ,ϕ(t 0)=0ʎ,v (t 0)=10.98km /s,ψ(t 0)=0ʎ,γ(t 0)=-5.576ʎ㊂终端约束条件:h (t f )ȡ10km,v (t f )=150m /s㊂再入轨迹的路径约束参数为:过载上限n max =3.5;热流上限Q ㊃max =260W /cm 2,驻点半径r =3m;动压上限q max =15kPa㊂本文采用的计算平台为MacBook Air (处理器Intel Core i5-5250U 1.6GHz,单核参与运算;内存DDR34GB;操作系统Windows 10企业版;编程语言MATLAB R2009a)㊂本文中给出的优化耗时为10次优化的平均耗时㊂3.1㊀方法1(整体优化)为了对比,本文首先不考虑二次优化,在整个512第2期赵吉松等:探月返回飞行器跳跃式再入轨迹优化时间区间对跳跃式再入轨迹进行优化㊂采用本文3.1节介绍的自适应稀疏局部配点法求解该问题㊂图3为优化出的最优控制变量随时间变化曲线㊂图4为优化出的最优高度和速度随时间变化曲线㊂图3和图4中的圆圈表示离散最优解(总共采用142个非均匀分布的离散节点)㊂图3中的细实线为对离散控制变量进行插值得到的连续控制变量随时间变化曲线㊂图4中的细实线为根据图3所示的最优控制变量采用四阶Runge-Kutta 数值积分方法对再入动力学方程组进行数值积分得到的结果㊂图3㊀最优倾侧角变化曲线(方法1)Fig.3㊀Optimal bank angle profile (method 1)由图4可知,在初次再入阶段,数值积分结果与离散最优解的差异微小,但是从飞行器跳跃出大气层开始,数值积分结果与离散最优解逐渐出现了明显的差异㊂在轨迹终端,数值积分得到的终端高度为7361.8m,终端速度为106.1m /s,均不满足开伞条件㊂数值积分结果与离散最优解的高度差异高达-4768.3m,速度差异高达-43.9m /s,显然超出了合理范围㊂这种差异主要是因为在初次再入段,数值积分结果与离散最优解存在微小的误差(由于数值离散造成的,不可避免),而跳跃式再入轨迹的跳跃段和二次再入段对首次再入段的误差非常敏感,使得首次再入段的误差沿轨迹累积并传播,最终导致轨迹的终端误差过大㊂对于常规轨迹优化问题,数值积分结果与离散最优解也存在误差,只是误差通常非常小,其影响可以忽略不计㊂理论上,增加离散节点的数量能够减小这种误差,但是实际上如果离散节点数量过多,NLP 的规模过大,优化算法的收敛性通常会变差,甚至无法收敛[21]㊂图4㊀最优高度和速度变化曲线(方法1)Fig.4㊀Optimal altitude and velocity profiles (method 1)3.2㊀方法2(整体优化+二次优化)为了解决数值积分结果和离散最优解差异过大问题,本文在跳跃式再入轨迹整体优化结果的基础上,从跳跃的最高点(对应的时间t 1,f =711.2s)开始,对二次再入轨迹重新优化,具体方法参见2.2节㊂图5给出优化的控制变量随时间变化曲线,图6给出优化的高度和速度随时间变化曲线㊂其中,跳跃最高点之前的轨迹是通过对再入轨迹整体优化得到(参见2.1节),跳跃最高点之后的轨迹为二次优化的结果(本文考虑了二次优化的耗时,因而连接点相对最高点略微右移)㊂图5和图6中的圆圈表示二次优化得到的离散最优解(采用84个非均匀分布的节点)㊂图5中的细实线是对离散控制变量进行插值得到的连续控制变量随时间变化曲线,图6中的细实线是根据图5所示的最优控制变量对再入动力学方程组进行数值积分得到的结果㊂对比图5和前述图3可知,对完整再入轨迹优化和对二次再入轨迹重新优化得到的二次再入控制变量差别不大㊂对比图6和图4可知,引入二次优612㊀㊀㊀㊀㊀㊀㊀㊀㊀㊀㊀㊀㊀㊀㊀㊀宇航学报第42卷化之后,二次再入轨迹的数值积分结果与离散最优解非常接近,终端高度误差仅为2.12218m,终端速度误差仅为0.02580m /s,很好地满足了再入飞行器的开伞条件约束㊂可见,通过对二次再入段重新优化显著提高了跳跃式再入轨迹的优化精度㊂图5㊀最优控倾侧角变化曲线(方法2)Fig.5㊀Optimal bank angle profile (method 2)图6㊀最优高度和速度变化曲线(方法2)Fig.6㊀Optimal altitude and velocity profiles (method 2)图7给出优化的三维再入轨迹,其中圆圈为离散最优解,细实线为数值积分结果㊂为了便于展示轨迹特征,图7中还给出了飞行器在地球表面投影点的轨迹㊂可见,为了取得最大横向航程,飞行器在首次再入过程中利用气动力改变速度方向进行转弯,在二次再入过程中进一步利用气动力进行横向机动㊂图8给出再入过程中过载等路径约束沿轨迹变化曲线㊂可见,最优轨迹的过载㊁热流和动压都满足路径约束要求,其中过载在首次再入段的最低点附近达到上限,其它约束均未达到上限㊂图7㊀三维最优跳跃式再入轨迹(方法2)Fig.7㊀Optimal three-dimensional entry trajectory (method 2)表1给出方法1(整体优化)和方法2(整体优化+二次优化)的优化结果对比㊂对于方法2,表中还给出了不同的二次优化耗时Δt 2对数值积分终端误差的影响㊂从表1中可以看出,通过对二次再入轨迹重新优化,可以将终端时刻的速度误差和高度误差降低3~4个数量级,从而使得飞行器的开伞条件得到严格满足㊂在目标函数方面,两种方法解出的最大横向航程几乎没有差别㊂需要强调的是,方法1的优化结果对应的数值积分终端误差过大㊁不满足开伞条件,实际上并不是可行轨迹㊂在本算例中,二次再入轨迹重新优化消耗的时间为Δt 2=2.4s㊂为了使方法具有实用性,本文在二次优化得到新的控制变量之前,仍然采用整体优化得到的控制变量作为输入对再入动力学方程组进行积分㊂虽然二次优化得出的控制变量和整体优化得出的控制变量存在差异,但是仿真结果表明这样处理对二次再入轨迹几乎没有影响㊂目前,国产宇航芯片的主频可达到300MHz [22],而本文采用的计算平台的处理器主频为1.6GHz,考虑到内存和软件等性能的差异,预计宇航级计算机的计算性能与712第2期赵吉松等:探月返回飞行器跳跃式再入轨迹优化图8㊀最优轨迹的路径约束(方法2) Fig.8㊀Path constraints along optimal trajectory(method2)本文采用的计算平台相差10~20倍㊂进一步仿真研究表明,即使在计算能力较低的宇航级计算机上(以计算能力降低至1/20为例),二次优化的耗时会显著增加,但是上述处理方式对二次再入轨迹仍然几乎没有影响,如表1所示㊂因为跳跃最高点的高度为117.8km,大气非常稀薄,气动力非常小,因而在最高点附近采用不够准确的控制变量积分再入动力学方程组对轨迹几乎没有影响㊂因此,本文提出的处理方法使得二次再入轨迹的优化是一种准实时优化,在再入轨迹的制导领域具有应用潜力㊂表1㊀不同方法的优化结果对比Table1㊀Comparison of the optimization results that areobtained from different methods目标函数ϕ/(ʎ)终端状态误差Δh/mΔv/(m㊃s-1)Δϕ/(ʎ)方法1-6.96080-4768.31548-43.932300.00635方法2(Δt2=2.4s)-6.95794 2.122180.025800.00001方法2(Δt2=48.0s)-6.95793 3.388710.040770.00001 4 结论探月返回飞行器跳跃式再入返回轨迹优化问题是复杂的多约束㊁非线性最优控制问题,特别是二次再入轨迹对首次再入段的误差非常敏感,给优化带来挑战㊂本文提出一种高精度求解跳跃式再入轨迹优化问题的方法㊂该方法首先应用节点自适应稀疏配点法对完整跳跃式再入轨迹进行优化,然后根据优化得到的控制变量积分再入动力学方程组,当积分到跳跃轨迹的最高点时,以积分得到的状态变量值作为新的初始条件,对二次再入轨迹重新优化㊂在求解二次再入轨迹期间(约2~3s),继续基于整体优化解出的最优控制积分再入动力学方程组,直到优化算法重新优化出二次再入段的最优控制,然后根据新的最优控制积分再入动力学方程组至轨迹终端㊂仿真结果表明:与不采用二次优化的方法相比,采用二次优化能够将跳跃式再入轨迹的终端高度和速度误差降低3~4个数量级㊂此外,在跳跃的最高点附近进行的二次优化是一种准实时优化,在再入轨迹的精确制导领域具有应用潜力㊂参㊀考㊀文㊀献[1]㊀Brunner C W,Lu P.Skip entry trajectory planning and guidance[J].Journal of Guidance Control and Dynamics,2008,31(5):1210-1219.[2]㊀南英,陆宇平,龚平.登月返回地球再入轨迹的优化设计[J].宇航学报,2009,30(5):1842-1847.[Nan Ying,LuYu-ping,Gong Ping.Optimal reentry trajectory design forMooncraft returning to the earth[J].Journal of Astronautics,2009,30(5):1842-1847.][3]㊀王大轶,郭敏文.航天器大气进入过程制导方法综述[J].宇航学报,2015,36(1):1-8.[Wang Da-yi,Guo Min-wen.Review of spacecraft entry guidance[J].Journal of Astronautics,2015,36(1):1-8.]812㊀㊀㊀㊀㊀㊀㊀㊀㊀㊀㊀㊀㊀㊀㊀㊀宇航学报第42卷[4]㊀崔平远,赵泽端,朱圣英.火星大气进入段轨迹优化与制导技术研究进展[J].宇航学报,2019,40(6):611-620.[CuiPing-yuan,Zhao Ze-duan,Zhu Sheng-ying.Research progress oftrajectory optimization and guidance techniques for marsatmospheric entry[J].Journal of Astronautics,2019,40(6):611-620.][5]㊀Istratie V.Optimal entry into atmosphere with minimum heat andconstraints[C].AIAA Atmospheric Flight Mechanics Confe-rence and Exhibit,Monterey,CA,Aug.5-8,2002. [6]㊀王银,陆宇平,张崇峰.月球返回舱跳跃再入弹道特性分析与优化设计[J].南京航空航天大学学报,2010,42(1):21-24.[Wang Yin,Lu Yu-ping,Zhang Chong-feng.Skip-entrytrajectory characteristic analysis and optimal design for lunarreturn vehicle[J].Journal of Nanjing University of Aeronauticsand Astronautics,2010,42(1):21-24.][7]㊀杜昕,刘会龙.探月飞船跳跃式再入轨迹可达域分析[J].载人航天,2017,4(2):163-167.[Du Xin,Liu Hui-long.Analysis of reachable sets of lunar module skip entry trajectory[J].Manned Spaceflight,2017,4(2):163-167.] [8]㊀崔乃刚,赵彪,郭继峰,等.低升阻比飞行器月球返回再入轨迹特性分析[J].哈尔滨工业大学学报,2013,45(7):30-34.[Cui Nai-gang,Zhao Biao,Guo Ji-feng,et al.Trajectorycharacteristics analysis of low lift-drag-ratio vehicle for lunarreturn entry[J].Journal of Harbin Institute of Technology,2013,45(7):30-34.][9]㊀曾亮,张洪波,郑伟.飞船跳跃式再入第一次再入能量管理方法[J].宇航学报,2015,36(10):1155-1162.[Zeng Liang,Zhang Hong-bo,Zheng Wei.Energy management method forcapsule skip reentry in first entry[J].Journal of Astronautics,2015,36(10):1155-1162.][10]㊀Betts J T.Practical methods for optimal control and estimationusing nonlinear programming,advances in design and controlseries[M].Philadelphia:Soc.for Industrial and Applied Mat-hematics,2009.[11]㊀Gill P E,Murray W,Saunders M A.SNOPT:An SQP algorithmfor large-scale constrained optimization[J].SIAM Journal onOptimization,2002,12(4):979-1006.[12]㊀Biegler L T,Zavala V rge-scale nonlinear programmingusing IPOPT:An integrating framework for enterprise-widedynamic optimization[J].Computers and Chemical Engin-eering,2009,33(3):575-582.[13]㊀Darby C L,Hager W W,Rao A V.Direct trajectory optimizationusing a variable low-order adaptive pseudospectral method[J].Journal of Spacecraft and Rockets,2011,48(3):433-445.[14]㊀Betts J T,Huffman W P.Mesh refinement in direct transcriptionmethods for optimal control[J].Optimal Control Applicationsand Methods,1998,19(1):1-21.[15]㊀Jain S,Tsiotras P.Multiresolution-based direct trajectoryoptimization[C].The46th IEEE Conference on Decision andControl,Piscataway,NJ,Dec.12-14,2007. [16]㊀Zhao Ji-song,Li Shuang.Modified multiresolution technique formesh refinement in numerical optimal control[J].Journal ofGuidance Control and Dynamics,2017,40(12):3328-3338.[17]㊀赵吉松,张建宏,李爽.高超声速滑翔飞行器再入轨迹快速㊁高精度优化[J].宇航学报,2019,40(9):17-26.[Zhao Ji-song,Zhang Jian-hong,Li Shuang.Rapid and high accurateapproach for hypersonic glide vehicle reentry trajectoryoptimization[J].Journal of Astronautics,2019,40(9):17-26.][18]㊀赵吉松.求解轨迹优化问题的局部配点法的稀疏性研究[J].宇航学报,2017,38(12):49-58.[Zhao Ji-song.Exploiting sparsity in local collocation methods for solvingtrajectory optimization problems[J].Journal of Astronautics,2017,38(12):49-58.][19]㊀赵吉松,谷良贤,佘文学.配点法和网格细化技术用于非光滑轨迹优化[J].宇航学报,2013,34(11):1442-1450.[ZhaoJi-song,Gu Liang-xian,She Wen-xue.Application of localcollocation method and mesh refinement to nonsmooth trajectoryoptimization[J].Journal of Astronautics,2013,34(11):1442-1450.][20]㊀Brown J D,Bogdanoff D W,Yates L A,et al.Free-flightdynamic aero data for a lifting CEV capsule[C].The46th AIAAAerospace Sciences Meeting and Exhibit,Reno,Nevada,January7-10,2008.[21]㊀Schlegel M,Stockmann K,Binder T,et al.Dynamicoptimization using adaptive control vector parameterization[J].Computers and Chemical Engineering,2005,29(8):1731-1751.[22]㊀北京微电子技术研究所.产品谱系[OL]2020[2020]./n3/n1497/n1501/index.html作者简介:赵吉松(1984-),男,博士,讲师,主要从事飞行器总体设计与轨迹优化等方面的研究㊂通信地址:南京市秦淮区御道街29号(210016)电话:182****2336E-mail:zhaojisongjinling@912第2期赵吉松等:探月返回飞行器跳跃式再入轨迹优化。
openmv大津法自适应阈值 -回复

openmv大津法自适应阈值-回复【openmv大津法自适应阈值】导语:在图像处理和计算机视觉领域,自适应阈值是一项重要的技术。
自适应阈值的目的是根据图像中的像素灰度值来动态地确定不同区域的阈值,以有效地分割图像。
OpenMV是一款基于MicroPython的高性能计算机视觉模块,可以实现图像分析、目标检测、颜色识别等多种应用。
本文将介绍OpenMV中的大津法自适应阈值算法,以及具体的步骤和原理。
第一部分:背景介绍1.1 图像阈值分割图像阈值分割是一种常见的图像处理方法,通过将图像像素灰度值与事先设定的阈值进行比较,将图像分割为不同的区域。
然而,传统的全局阈值方法无法有效地处理光照不均匀或者目标区域中存在着不同灰度值的情况。
为了解决这个问题,自适应阈值方法被提出。
1.2 大津法自适应阈值大津法(Otsu’s method)是一种常用的自适应阈值算法,它是由日本学者大津展之于1979年提出的。
该算法的基本思想是寻找图像的最佳阈值,使得目标和背景之间的类间方差最大化。
通过最大化类间方差,大津法能够自适应地确定不同区域的阈值,从而实现有效的图像分割。
第二部分:OpenMV大津法自适应阈值算法步骤2.1 加载图像首先,我们需要通过OpenMV读取并加载图像。
OpenMV提供了诸多图像加载函数,如`sensor.snapshot()`函数可以实时获取摄像头的当前图像。
2.2 灰度化处理在大津法自适应阈值算法中,我们需要将原始图像转换为灰度图像。
通过`img.to_grayscale()`函数,我们可以将彩色图像转换为灰度图像。
2.3 直方图计算在自适应阈值算法中,计算图像直方图是一个重要的步骤。
通过直方图,我们可以统计不同灰度值的像素数量分布,并基于此来确定阈值。
在OpenMV中,我们可以使用`histogram()`函数来计算图像的灰度直方图。
2.4 计算类间方差大津法的关键步骤是寻找图像的最佳阈值,使得类间方差最大化。
ChatGPT技术的自适应学习与模型更新策略

ChatGPT技术的自适应学习与模型更新策略ChatGPT是OpenAI开发的一种自然语言处理技术,能够生成与人类非常相似的文本。
它的核心是一个深度学习模型,通过大规模训练来提高生成文本的质量和流畅度。
然而,由于模型的巨大复杂性和数据的多样性,它有时候会生成一些不准确的或者有争议的内容。
为了解决这个问题,OpenAI采取了一系列的自适应学习和模型更新策略。
首先,ChatGPT会利用用户的反馈来不断改进自身。
当用户发现模型生成的文本有问题时,可以通过反馈机制告诉ChatGPT。
例如,用户可以指出某部分文本不合理,或者提供正确的答案。
这些反馈会被OpenAI收集并进行分析,以改进模型的性能。
反馈机制使得ChatGPT能够不断从错误中学习,并逐渐提升自身的准确性和信息质量。
其次,自适应学习还包括了用户界面的优化。
OpenAI鼓励用户主动提供反馈,并致力于改进用户界面的设计,使得用户可以更方便地通过反馈机制与ChatGPT进行交互。
用户界面的优化不仅提高了用户的参与度,也促进了ChatGPT技术的不断发展。
除了用户反馈,OpenAI还采取了多样化的数据源,包括互联网上的公开文本和私有合作伙伴提供的数据,来训练模型。
通过引入各种不同领域、不同主题的数据,可以使ChatGPT更全面地了解不同的问题和需求。
这种多样化的数据训练有助于提高模型的灵活性和准确性,使其能够适应各种不同的语境和使用场景。
另外,模型更新策略也是ChatGPT技术中的重要组成部分。
OpenAI会定期更新ChatGPT的模型,以跟进新的技术进展和改进。
通过不断的模型更新,OpenAI能够将最新的研究成果和改进应用到ChatGPT中,进一步提高其性能和功能。
然而,模型更新也可能带来一些挑战。
一方面,模型更新可能会引入新的问题或者错误,甚至可能导致模型性能的下降。
为了确保模型的质量,OpenAI会在更新模型之前进行大规模的测试和评估。
另一方面,模型更新可能还会导致用户与旧模型训练的ChatGPT产生差异。
- 1、下载文档前请自行甄别文档内容的完整性,平台不提供额外的编辑、内容补充、找答案等附加服务。
- 2、"仅部分预览"的文档,不可在线预览部分如存在完整性等问题,可反馈申请退款(可完整预览的文档不适用该条件!)。
- 3、如文档侵犯您的权益,请联系客服反馈,我们会尽快为您处理(人工客服工作时间:9:00-18:30)。
J Geod(2009)83:403–410DOI10.1007/s00190-008-0226-9ORIGINAL ARTICLEAdaptive collocation with application in height system transformation Y.Yang·A.Zeng·J.ZhangReceived:10October2007/Accepted:31March2008/Published online:15May2008©Springer-Verlag2008Abstract In collocation applications,the prior covariance matrices or weight matrices between the signals and the observations should be consistent to their uncertainties;other-wise,the solution of collocation will be distorted.To balance the covariance matrices of the signals and the observations, a new adaptive collocation estimator is thus derived in which the corresponding adaptive factor is constructed by the ratio of the variance components of the signals and the obser-vations.A maximum likelihood estimator of the variance components is thus derived based on the collocation func-tional model and stochastic model.A simplified Helmert type estimator of the variance components for the colloca-tion is also introduced and compared to the derived maximum likelihood type estimator.Reasonable and consistent cova-riance matrices of the signals and the observations are arri-ved through the adjustment of the adaptive factor.The new adaptive collocation with related adaptive factor constructed by the derived variance components is applied in a trans-formation between the geodetic height derived by GPS and orthometric height.It is shown that the adaptive collocation is not only simple in calculation but also effective in balan-cing the contribution of observations and the signals in the collocation model.Y.Yang(B)Xi’an Research Institute of Surveying and Mapping,No.1Middle Yanta Road,Xian710054,Chinae-mail:yuanxi@;yuanxi_yang@A.ZengXi’an Information Division of Surveying and Mapping,36Xiying Road,Xian710054,ChinaA.Zeng·J.ZhangSchool of Geological and Surveying Engineering,Chang’an University,126Yanta Road,Xian710054,China Keywords Collocation·Adaptive estimation·Variance component estimation·geodetic height·orthometric height 1IntroductionCollocation is usually applied in approximation in gravity field(Tscherning1978).It can also be applied in coordi-nate transformation(You and Hwang2006)and height datum transformation(Featherstone and Sproule2006).To control the outlier influences,robust collocation is studied(Schaffrin 1986;Yang1992).In the transformation of different height systems,the collocation method can also be applied tofit the errors that remained after the functional transformation of the two height systems or in a synthetic transformation pro-cess combining the functional transformation(trend-fitting) and stochasticfitting.The covariance function of the signals (stochastic part of the transformation)is,however,a key pro-blem in the synthetic height transformation procedure(Yang 1992).Once the covariance function is chosen,the coeffi-cients of the function are then determined by the observa-tions and/or the known values of the parameters.The prior determined covariance elements are usually not changed in the collocation process.Similar approach is kriging,which has also been widely researched and applied(Journel and Huijbregt1989;Robe-son1997;Karniefi1990;Oliver and Webster1990;Felus et al.2005).In theory,the kriging and collocation are equiva-lent from the expressions.The kriging evaluates the parame-ters and signals simultaneously;the dimension of the normal equation is larger than that of collocation,because the collo-cation estimates the trend parameters and signals separately. Furthermore,the collocation is only suitably applied in the smooth and stable stochasticfields to estimate the parameters and signals(Bian and Menz2000).404Y.Yang et al.The collocation results are sometimes distorted.This is due predominantly to the well-known distortions of the prior weight matrix of the measurements and thefitted covariance matrix of the signals.In theory,the weight matrices bet-ween the measurements and signals should be consistent to their actual contributions to the unknown parameters and signal estimates.In other words,the covariance matrices of the measurements and signals should reflect their actual uncertainties.Otherwise,the collocation results for the height transformation will be distorted.Incomplete knowledge of variance matrix of the obser-vations occurs in many geodetic application(Teunissen and Amiri-Simkooei2008).In collocation,the incomplete know-ledge of variance matrices of the measurements and signals will result in systematic errors similar to the influences of the functional model errors in the error effect point of view. Refitting of the residuals of the collocation is a way to reduce the systematic error influences(Yang and Liu2002).In the view point of stochastic model error influences,the errors of the covariance elements of the signals can partly be adjusted through the variance factor.Thus,the variance component estimation can be employed in adjusting the ratio of the prior weights of the signals and the measurements(Koch and Kusche2002;Shen and Liu2002;Xu et al.2006;Yang and Xu2003).Following Koch and Kusche’s idea to use the ratio of the variance components to overcome the ill-conditioned problem,we employ the ratio of the variance components of measurements and signals to adjust their weight matrices.In geodetic applications,a lot of variance component esti-mation methods have been proposed and researched,such as minimum norm quadratic unbiased estimator(MINQUE) (Rao1971),best invariant quadratic unbiased estimates (BIQUE)(Caspary1987;Sjöeberg1984),the maximum likelihood estimates(Koch1986)or the restricted maximum likelihood estimation(Searle et al.1992)and least squares(LS)variance component estimation(Teunissen and Amiri-Simkooei2007).An often used variance component estimation of Helmert type is analyzed with respect to trans-lation invariance and unbiasedness,which is also genera-lized into variance and covariance component estimation (Grafarend1980).The best invariance component estima-tor is studied and its application to the generalized multiva-riate adjustment of heterogeneous deformation observations is proposed(Schaffrin1981).Using the orthogonal comple-ment likelihood function and rank factorization(Schaffrin 1983),an iterative procedure for the maximum likelihood estimates of the variance and covariance components is deri-ved(Koch1986).It is shown that BIQUE,MINQUE and LS variance component estimates are identical with Helmert’s estimate under the Gaussian distribution(Amiri-Simkooei 2007;Ou1989;Yu1992).Bayesian inference for variance components is researched by Koch(1987).Furthermore,the variance component estimation is proposed to be applied in the determination of gravityfield by different types of obser-vations and solving the regularization problems(Koch andKusche2002;Xu et al.2006),as well as in adaptive naviga-tion(Yang and Xu2003;Yang and Gao2005).This paper generalizes the maximum likelihood estima-tion of the variance component to the collocation model andemploys the variance component ratio to set up an adaptivefactor to balance the contribution of the measurements andprior signal information.Thus,a new adaptive collocationprocedure is derived by introducing the adaptive factor.2Least square collocation estimatorsAssume that we have an n×1vector L of measurements,thecorresponding error vector e with the expectation E(e)=0and covariance matrix e=σ2e P−1e,an m×1vector X of trend parameters,and a u×1signal vector S with prior cova-riance matrix S=σ2s P−1S,which has functional relation with measurements,whereσ2e andσ2s are variance compo-nents,P e and P S are the weight matrices of e and S,respec-tively.The observational equation isL=AX+BS+e(1) where A denotes an n×m design matrix with rank m and B is an n×u coefficient matrix.The corresponding error equation isV=AˆX+BˆS−L(2)whereˆX is the estimated vector of the trend parameters and ˆS is the estimated signal vector.If the signal vector S ,which has no relation with the measurements,is correlated with the measured signal vector S,that is, SS = T S S=0,and if the unmeasured signal vector needs to be estimated,then Eq.2can be changed intoV=AˆX+[B0]ˆSˆS−Lwhere0is a zero matrix.The covariance matrix between the measured signals S and unmeasured signals S must be evaluated atfirst,which is the task of covariance function fitting.Assume that the signals and the measurement noises are uncorrelated,by using the least squares collocation principle (Koch1977;Krarup1978;Moritz1980)V T P e V+ˆS T P SˆS=min(3) we getˆX=(A T PL A)−1A T PL L(4) andˆS=S P L(L−AˆX)(5)Adaptive collocation with application in height system transformation 405whereP L =(B S B T + e )−1(6)The signal estimates at unmeasured stations are ˆS= S S P L (L −A ˆX )(7)3Adaptive collocation estimatorsIt is different from the standard collocation that the objectfunction of the adaptive collocation is similar to the adaptive Kalman filter (Yang et al.2001;Yang and Gao 2006) =V T P e V +αˆST P S ˆS =min (8)where αis an adaptive factor,which balances the contributionof the measurements and signals to the parameter estimates.By derivation we haveA T P e A A T P e BB T P e A B T P e B +αP S ˆX ˆS =A T P e LB T P e L (9)The stepwise estimators are ˆX=(A T ¯P L A )−1A T ¯P L L (10)ˆS=¯ S B T ¯P L (L −A ˆX )(11)The signal estimator at the unmeasured stations is ˆS =¯ S S ¯ −1SˆS (12)where ¯PL ,¯ S and ¯ S S denote the evaluated matrices by using the adaptive factor,they are respectively expressed as ¯PL =(B S B T /α+ e )−1=α(B S B T +α e )−1(13)¯ S =1α S (14)¯ S S =1αSS(15)Let˜PL =(B S B T +α e )−1(16)then we have ¯PL =α˜P L (17)Substituting Eqs.(14)–(16)into Eqs.(10)–(12),we have ˆX=(A T ˜P L A )−1A T ˜P L L (18)ˆS= S B T ˜P L (L −A ˆX )(19)ˆS =1α S S (1αS )−1ˆS = S S −1SˆS (20)Equations 7and 20have the same form,but the estimatedvalues are not equivalent,because the estimated signals ˆSof the measured stations is changed by the adaptive factor.Ifthe adaptive factor α=1,the solutions provided by the two formulae will be equal.If the measurement outliers are taken into account,the object function may further be expressed as =n i =1P i ρ(V i )+αˆST P S ˆS =min (21)where ρ(V i )is convex,continuous and not decreased func-tion of the residual V i ,P i is the weight element of the weight matrix P e .Equation 21is called an adaptively robust collo-cation object function,in which the robust object function is employed for dealing with the measurement outliers and adaptive factor αis introduced for balancing the prior weight matrices of the measurements and the signals.Similar to the robust collocation (Yang 1992)and adaptive Kalman filter (Yang and Gao 2006),after taking the deri-vatives of the object function with respect to the unknown parameters,we haveA T ¯P e A A T ¯P e BB T ¯P e A B T ¯P e B +αP S ˆX ˆS = A T ¯P e L B T ¯P e L (22)where ¯Pe denotes the equivalent weight matrix of the mea-surement vector which is determined by a weight function (Yang 1991,1992).4Adaptive factor determined by variance components It has been explained in Sect.1that the weight matrices of the signals and measurements should adapt to their pro-per uncertainties.In actual computation,however,the cova-riance matrix of the signals evaluated by the chosen cova-riance function and the known data points and the priori covariance matrix of the measurements may be inaccurate,which result in the weight matrices of the measurements and the signals unsuitable.Thus,we use an adaptive factor αto adjust the weight matrices and balance the contribution of the measurements and signals to the parameter estimates.Different statistical principles have different variance com-ponent estimators.In this section,two types of variance component estimators are derived based on the maximum likelihood estimation and Helmert estimation,respectively.An adaptive factor is presented.4.1Maximum likelihood estimator of variance components Assume that the measurement vector L follows normal dis-tribution L ∼N (AX , L )(23)406Y .Yang et al.where L includes the variance components σ2s and σ2eof the stochastic signals and observational errors,that is L =σ2s B Q s B T +σ2eQ e (24)where Q s and Q e are the cofactor matrices of the signals and measurements,respectively.Then the likelihood func-tion of the unknown parameter vector X and the variancecomponents σ2s and σ2eis (Koch 1986;Ou 1989)l (X ,σ2s ,σ2e |L )=(2π)−n2| L |−12×exp {−12(L −AX )T −1L (L −AX )}(25)Taking the logarithm of the Eq.25and neglecting the constant terms,we haveln l (x ,σ2s ,σ2e |L )=−ln | L |−(L −AX )T −1L (L −AX )=−ln | L |−tr [ −1L×(L −AX )(L −AX )T ](26)Taking the derivatives of Eq.26with respect to σ2s and σ2e,respectively,we obtain∂ln l ∂σ2s =−tr (B Q S B T −1L )+tr [ −1L B Q S B T −1L ×(L −AX )(L −AX )T ](27)∂ln l e=−tr (Q e −1L )+tr [ −1L Q e −1L ×(L −AX )(L −AX )T ](28)Let the derivatives be equal to zeros and considering−1L=P L ,we get tr [P L B Q S B T P L (L −AX )(L −AX )T ]=tr (B Q S B T P L )(29)tr [P L Q e P L (L −AX )(L −AX )T ]=tr (Q e P L )(30)The right-hand term of Eq.29can be expressed as tr (B Q S B T P L )=tr (B Q S B T P L L P L )=tr [B Q S B T P L (B Q S B T σ2s +Q e σ2e )P L ]=tr (B Q S B T P L B Q S B T P L )σ2s +tr (B Q S B T P L Q e P L )σ2e(31)The right-hand term of the Eq.30can be expressed as tr (Q e P L )=tr (Q e P L B Q S B T P L )σ2s +tr (Q e P L Q e P L )σ2e(32)Combining the Eqs.29–32and expressing L −AX as theresidual vector V ,we arrive attr (B Q S B T P L B Q S B T P L )tr (B Q S B T P L Q e P L )tr (B Q S B T P L Q e P L )tr (Q e P L Q e P L ) × ˆσ2sˆσ2e = V TP L B Q S B T P L V V T P L Q e P L V(33)If B =I ,then we get the estimator of the variance com-ponents as ˆσ2s ˆσ2e=tr (Q S P L Q S P L )tr (Q S P L Q e P L )tr (Q S P L Q e P L )tr (Q e P L Q e P L )−1×V TP L Q S P L V V T P L Q e P L V(34)It is interesting that the new estimators of variance compo-nents make the calculation procedure simpler than the Hel-mert type estimates,because they do not have the need to calculate the normal matrix and its inverse.After solving ˆσ2s and ˆσ2e ,the adaptive factor αcan be set up like (Yang et al.2001;Yang and Xu 2003;Yang and Gao 2005)α=ˆσ2e /ˆσ2s (35)in which ˆσ2s =0must be satisfied.If the variance componentof the signals is large,that is,their weight matrix should be reduced.The adaptive factor expressed by Eq.35just adapts the weight matrix of the signals to its proper one and also adapts the signals to their proper contributions to the parameter estimates.Having the adaptive factor α,we re-estimate the trendparameter vector ˆXand the signal vectors ˆS and ˆS by using Eqs.16and 18–20.4.2Simplified estimator of variance componentsIf the signals are viewed as pseudo observations,then the variance components with respect to the two kinds of obser-vations can be obtained (Koch and Kusche 2002).We start from the error equationsV =A ˆX+B ˆS −L with P e = −1e V S =ˆS −0with P S = −1S(36)where “0”denotes the prior expectation vector of the signals.Then the corresponding Helmert type of variance component estimator is like (Koch and Kusche 2002)ˆσ2e=V T P e Vr e(37)ˆσ2s =ˆS T P s ˆS r s(38)where r e and r s are the partial redundancies,i.e.,the contri-butions of the observations L and the prior signal informa-tion S to the overall redundancy r =n +u −m of the model of Eq.1.The partial redundancies are computed fromAdaptive collocation with application in height system transformation 407(Koch and Kusche 2002)r e =n −tr1σ2eN −1N e (39)r s =u −tr1σ2s N −1N s(40)withN =⎡⎣1σ2e A T P e A 1σ2e A T P e B 1σ2e B T P e A 1σ2e B T P e B +1σ2s P S⎤⎦,N e =⎡⎣1σ2e A T P e A 1σ2e A T P e B 1σ2eB T P e A1σ2eB T P e B⎤⎦,N s =001σ2eP SAfter solving the estimates of variance components ˆσ2s andˆσ2eas well as the adaptive factor α,we can re-estimate the trend parameter vector ˆXand the signal vectors ˆS and ˆS in the same way as listed in Sect.4.1.The formulas above may be derived by the maximum like-lihood method,by the best invariant quadratic unbiased esti-mation or Helmert’s method (see Koch 2000,p.146;Koch and Kusche 2002).5An actual computation and analysisThree hundred and sixty two GPS/leveling stations in the area of Southern China with two different heights are cho-sen for the height transformation (see Fig.1),in which the geodetic heights are referenced to the geocentric reference frame ITRF97and the normal heights are referenced to the Chinese height datum 1985defined by the mean sea surface of Huanghai Sea determined by tide gauge observations at Qingdao.To check the accuracy of the applied transforma-tion methods,332stations are employed for computing the transformation parameters and the signals,and the rest 30stations are served as checking stations,that is,the geodetic heights of the 30stations are transformed into the orthome-tric heights,then compared with the observed orthometric ones and the discrepancies as well as the RMS are obtained.The polynomial model is chosen as the functional model to express the trend of the height differences.Considering the stochastic part,we employ the following error model V i =K m =1m n =0ˆαmn d B m −n i d L n i +ˆSi −(h i −H i )(41)where V i is the residual of i th pseudo-observation (h i −H i ),which is also defined in Eq.2;ˆαmn denotes the estimated unknown coefficients,which construct the unknown para-meter vector in Eq.2;d B i and d L i are the centered lati-tude and longitude coordinates,which construct the design matrix in Eq.2;h i and H i denote the ellipsoidal heightandFig.1Distribution of common stations with the heights of two sys-tems (The blue triangles are the reference data stations,the red stars denote the checking stations,which do not take part in the collocation)orthometric height of the i th GPS/leveling station;and Kdenotes the order of the polynomial model.The Gaussian covariance function of the signals is chosen (Moritz 1980;You and Hwang 2006)C (d )=C 0e −k2d 2(42)where C 0and k are unknown parameters to be estimated and C (d )denotes the covariance between the i th point and the j th point with distance d .A method proposed by Mikhail and Ackermann (1976)to fit the empirical covariance function is employed.Firstly,the data stations (332reference stations)are divided into m groups,each of which with nearly equal distance d i (i =1,...,m ).Secondly,the initial covariance is obtained by the mean of all possible products l i l j with the distance d iC (d i )=1n in ii ,jl i l j(43)where n i denotes the number of pseudo-observations in the i th group;C (d i )is regarded as the covariance at the distance d i ,and the pseudo-observations are expressed as l i =(h i −H i )−K =2 m =1m n =0ˆαmn d B m −n i d L ni(44)Thirdly,we get the unknown parameter estimates in the cho-sen Gaussian function,C 0=0.0123,k =0.0162,based on the m covariance elements by using least squares estimation.408Y.Yang et al.Table1Root mean square residuals of heights at data stations(m)σ20Scheme Minimum Maximum RMSσ20=1X LS−0.3850.3040.105X C−0.3750.3070.100X MAC−0.3290.2960.085X HAC−0.3260.2950.085σ20=0.1X C−0.3390.2960.088X MAC−0.3290.2960.085X HAC−0.3260.2950.085The root mean square residuals calculated by the differences of thefittedheight values by collocation methods and the measurementsThe RMS of the transformed orthometric heights from thechecking stations is computed byRMS=1nni=1( H i)21/2(45)where n=30is the number of checking stations and H i is the difference between the computed and known orthometric height at the i th checking station.The smaller the RMS is, the better the transformation scheme is.In the computation,following four schemes are perfor-med:Scheme1:Least squares estimation based on a polynomial model,expressed as X LSScheme2:Collocation,expressed as X CScheme3:Adaptive collocation based on maximum likeli-hood estimates of variance components,expres-sed as X MACScheme4:Adaptive collocation based on Helmert type estimates of variance components,expressedas X HAC.To show how the adaptive collocation proposed in this paper adapts to the weight ratios of the measurements and signals,the initial scale variance is supposed as1.0and 0.1,respectively.Table1shows the minimum,maximum Table2RMS of the heights at checking stations(m)σ20Scheme Minimum Maximum RMSσ20=1X LS−0.2090.1970.102 X C−0.1970.1710.096X MAC−0.1940.1110.070X HAC−0.1920.1050.069σ20=0.1X C−0.1950.1280.076 X MAC−0.1940.1110.070X HAC−0.1920.1050.069 The root mean square errors calculated from the differences of thefitted height values by collocation methods and the known values which do not take part in the collocation,by using Eq.(45)Table3The error region of thefitted heights at check stationsσ20Scheme<5cm[5cm,10cm]>10cmσ20=1X LS9(30%)12(40%)9(30%) X C10(33%)12(40%)8(27%)X MAC17(57%)9(30%)4(13%)X HAC17(57%)10(33%)3(10%)σ20=0.1X C16(53%)8(27%)6(20%) X MAC17(57%)9(30%)4(13%)X HAC17(57%)10(33%)3(10%)In table3,the percentages reflect the absolute error region of thefitted height values by collocation methodsvalues and root mean squares of residuals of height measu-rements at reference stations for different schemes,respecti-vely.Figure2presents distribution of residuals of the heights at the reference stations.The minimum and maximum values and RMS of the transformed heights at the checking stations are shown in Table2,and the intervals of the corresponding discrepancies between the transformed orthometric heights and the original ones are shown in Table3.If the scale fac-torσ20=1is chosen,then the adaptive factorα=50.61 after three iterations,by using Scheme3;whileα=50.60after four iterations,by using Scheme4.Ifσ20=0.1is cho-sen,then the adaptive factorα=5.04after three iterations,Fig.2Residual distribution of the measured stations(σ20equals 1.0and0.1,respectively)Adaptive collocation with application in height system transformation409 Fig.3Iterative adaptivefactors(σ20equals1.0and0.1,respectively)by using Scheme3;whileα=5.03after three iterations, by using Scheme4.The adaptive factorαcorresponding to different schemes are shown in Fig.3.It shows that the priori variance scale will not influence the adaptive collo-cation results,but it will affect the nonadaptive collocation results.From the computation results,the following facts have been drawn:1.The initial value of scale factorσ20has significant effects on the transformed heights for both of the colloca-tion method and least squares polynomialfitting method, which has been reflected by the residuals of the measured stations and the discrepancies of the transformed heights of the checking stations.It means that the inconsistent variance components between the measurements and the signals will result in systematic errors of the transformed heights.2.In theory and practice,the weight matrices of the measurements and signals should reflect their uncertainties when applying collocation.In actual computation,however, it may have errors in thefitted variance–covariance matrix of the signals,which may lead to a biased variance scale of the signals,thus,leading to inconsistent weight matrices for the measurements and the signals.Thus,it is reasonable to employ the variance components to construct an adaptive factor,to adapt the ratio of the weight matrices of the signals and measurements to their proper value,and in turn to adjust their contributions to the parameter estimates.3.For the two kinds of adaptive collocation,the different initial values ofσ20do not influence transformed heights, since the adaptive factor plays a role in balancing the contri-butions of the stochastic models between the measurements and signals according to their actual uncertainties,which keeps the weight matrices between the measurements and signals in a reasonable ratio,and keeps the collocation results stable.4.The RMS of the checking stations shows that the adap-tive collocation based on the ratio of the estimated variance components is superior to the standard collocation and the polynomialfitting in transforming the geodetic heights to the orthometric heights.6ConclusionsThe determination of the covariance function is a key pro-blem in collocation.If the prior weight matrices of the signals and the measurements inversed from the related covariance matrices are consistent,in other words,the two weight matrices reflect their uncertainties of the measurements and signals,the collocation results are stable and reliable.Other-wise,the standard collocation results will be distorted. Usually,it is difficult to make the prior weight matrices of the signals and the measurements consistent in practice;thus, the standard collocation may be influenced in some cases.The adaptive collocation by introducing an adaptive fac-torα,which makes the weight matrices of the signals and measurements consistent,balances the contributions of the measurements and signals to the estimated parameters.It should be pointed that the adaptive factorαdetermined by variance component estimates may be influenced by the preliminary values of the prior covariance matrices.Thus, the preliminary covariance matrices should be as precise as possible.Acknowlegments The project is sponsored by Natural Science Foundations of China(40474001and40774001)and Natural863 Foundations of China(2007AA12Z331).The authors are indebted to Dr.J.Kusche and C.Kotsakis as well as other anonymous reviewers for their helpful comments and suggestions.ReferencesAmiri-Simkooei AR(2007)Least-squares variance component estima-tion:theory and GPS applications.PhD Thesis,Delft University of Technology,Publication on Geodesy,64,Netherlands Geodetic Commission,DelftBian S,Menz J(2000)Collocation:a synonym of kriging in geodesy.Math Geol5:43–48410Y.Yang et al.Caspary WF(1987)Concepts of network and deformation analysis.School of Surveying,the University of New South Wales,Ken-sington,pp97–111Featherstone WE,Sproule DM(2006)Fitting AUSGeio98to the Aus-tralian height datum using GPS-leveling and least squares collo-cation:Application of a cross-validation technique.Survey Rev 38(301):573–582Felus Y A,Saalfeld A,Schaffrin B(2005)Delauney triangulation struc-tured kringing for surface interpolation.Survey Land Inf Sci 65(1):27–36Grafarend E(1980)An introduction to the variance–covariance-component estimation of Helmert type.Z Vermessungswes4:161–180Journel AG,Huijbregt J(1989)Mining geo-statistics.Academic Press, LondonKarniefiAD(1990)Application of kriging technique to areal precipi-tation mapping in Arizona.GeoJournal22(4):391–398Koch KR(1977)Least squares adjustment and collocation.Bull Géod 51:127–135Koch KR(1986)Maximum likelihood estimate of variance compo-nents.Bull Géod60:329–328.Ideas by Pope AJKoch KR(1987)Bayesian inference for variance components.Manuscr Geod12:309–313Koch KR(2000)Einführung in die Bayes-Statistik,Springer,Berlin, Heidelberg,New York,Barcelona,Hongkong,London,Mailand, Paris,Singapur,Tokio,pp146Koch KR,Kusche J(2002)Regularization of geopotential determina-tion from satellite data by variance components.J Geod76:259–268Krarup T(1978)Some remarks about collocation.In:Moritz H, Suenkel H(eds)Approximate methods in geodesy.H.Wichmann Verlag,Karlsruhe,pp193–209Mikhail EM,Ackermann F(1976)Observation and least squares, Harper and Row,New York,pp418–421Moritz H(1980)Statistical foundations of collocation.Boll Geod Sci Affini2:131–150Oliver MA,Webster R(1990)Kriging:method of interpolation for geo-graphical information system.Int J Geogr Inf Sys4(3):313–332 Ou Z(1989)Estimation of variance and covariance components.Bull Géod63:139–148Rao CR(1971)Estimation of variance and covariance components—MINQUE theory.J Multivar Anal1:257–275Robeson SM(1997)Spherical methods for spatial interpolation:review and evaluation.Cartogr Geog Inf sys24(1):3–20Schaffrin B(1981)Best invariant covariance component estimators and its application to the generalized multivariate adjustment of hete-rogeneous deformation observations.Bull Géod55:73–85 Schaffrin B(1983)Varianz–kovarianz-komponentenschätzung bei der Ausgleichung heterogener Wiederholungsmessungen.C282, Deutsche Geodatische Kommission,MunchenSchaffrin B(1986)On robust collocation.In:Sanso F(ed)Proceedings of thefirst marussi symposium on mathematical geodesy Milano, 3–6June1985,pp343–361Searle SR,Casella G,McCulloch CE(1992)Variance components.Wiley,New YorkShen YZ,Liu DJ(2002)An unbiased estimate of the variance of unit weight after regularization.Geomat Inf Sci.Wuhan Univ27:604–606(in Chinese)Sjöberg LE(1984)Non negative variance component estimation in the Gauss–Helmet adjustment model.Manuscr Geod9:247–280 Teunissen PJG,Amiri-Simkooei AR(2008)Least-squares variance component estimation.J Geod82:65–82Tscherning CC(1978)Collocation and least squares methods as a tool for handling gravityfield dependent data obtained through space research techniques.In:Hieber S,Guyenne TD(eds)on space oceanography,navigation and geodynamics European workshop.European Space Agency,Paris pp141–149Xu PL,Shen Y,Fukuda Y,Liu YM(2006)Variance components esti-mation in linear inverse ill-posed models.J Geod80:69–81 Yang Y(1991)Robust Bayesian estimation.Bull Geod65(3):145–150 Yang Y(1992)Robustfying collocation.Manuscr Geod17(1):21–28 Yang Y,Gao W(2005)Comparison of adaptive factor in Kalmanfilters on navigation results.J Navigat58:471–478Yang Y,Gao W(2006)An optimal adaptive Kalmanfilter.J Geod 80:177–183Yang Y,He H,Xu T(2001)Adaptively robustfiltering for kinematic geodetic positioning.J Geod75:109–116Yang Y,Liu N(2002)A new resolution of collocation by two minimi-zation steps.Acta Geod Cartogr Sin31(3):192–195(in Chinese) Yang Y,Xu T(2003)An adaptive Kalmanfilter based on sage window weights and variance components.J Navigat56:231–240You RJ,Hwang HW(2006)Coordinate transformation between two geodetic datums of Taiwan by least-squares collocation.J Survey Eng132(2):64–70Yu Z(1992)A generalization theory of estimation of variance-covariance components.Manuscr Geod17:295–301。