视音频编解码技术发展现状和展望(四)
音视频编解码技术的现状与发展

音视频编解码技术的现状与发展随着互联网的普及和网络速度的提升,音视频资讯的获取变得越来越容易。
我们可以随时随地打开手机或电脑,观看视频、听音乐。
但是,这些视频和音频文件都离不开一个重要的技术——编解码技术。
本文将会探讨音视频编解码技术的现状与发展。
一、什么是编解码技术?在了解编解码技术的现状和发展前,我们需要先了解编解码技术是什么。
简单来说,音视频编解码技术就是把多媒体信号经过压缩算法转化为压缩格式的技术,以便于传输、存储和处理。
音视频编解码技术分为编码和解码两个过程,编码器把采集到的音视频数据压缩为压缩格式的数据,解码器把压缩格式的数据解压缩还原为原始音视频数据。
二、音视频编解码技术的现状1.国际标准的制定随着技术的发展,音视频编解码技术也在不断地被探索和研究,多种音视频编解码技术涌现出来并被广泛使用。
但是,如果缺乏统一的国际标准,那么不同厂商的设备和软件之间就会出现互不兼容的问题。
为了解决这个问题,国际标准组织ITU-T、ISO、IEC联合制定了许多音视频编解码标准,如H.264、H.265、MPEG-4、MPEG-2、VP9等等。
这些标准规定了音视频编解码的各种规范和参数,使得音视频编解码技术得到了广泛应用。
2.压缩效率的提升音视频编解码技术的核心之一就是压缩技术。
在压缩技术优化的过程中,压缩效率的提升一直是音视频编解码技术的发展方向之一。
现在,H.264是市场上最为流行的视频编码标准之一,其压缩后的视频质量和压缩比(压缩前和压缩后的数据量之比)都很优秀。
除此之外,H.265标准也日渐流行,在保证视频质量的前提下,其压缩比可以达到更高的水平。
3.应用领域的不断扩展音视频编解码技术应用领域的不断扩展也是其现状之一。
在早期,音视频编解码技术主要被应用在广播电视等领域。
随着技术和网络的发展,音视频编解码技术被应用到更多的领域,如在线视频、视频会议、社交应用、游戏直播等。
随着人们对视频沟通需求的不断增长,音视频编解码技术的应用领域将会更加广泛。
浅谈数字视音频编解码与电视传输网络技术
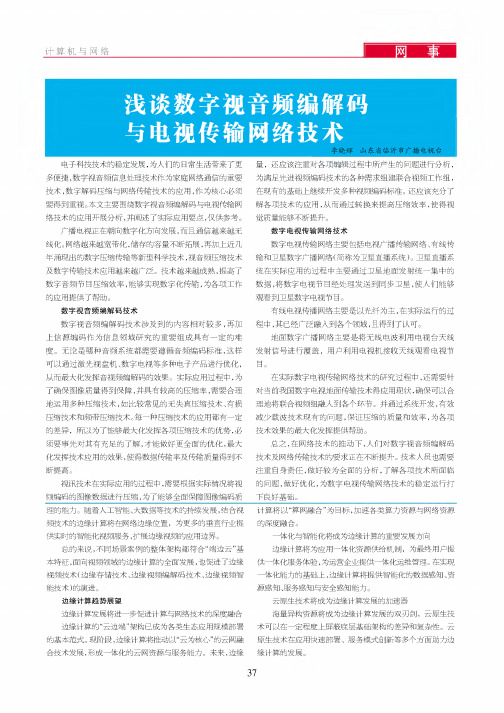
计算机与网络李晓辉山东省临沂市广播电视台电子科技技术的稳定发展,为人们的日常生活带来了更多便捷,数字视音频信息处理技术作为家庭网络通信的重要技术,数字解码压缩与网络传输技术的应用,作为核心必须要得到重视。
本文主要围绕数字视音频编解码与电视传输网络技术的应用开展分析,并阐述了实际应用要点,仅供参考。
广播电视正在朝向数字化方向发展,而且通信越来越无线化。
网络越来越宽带化,储存的容量不断拓展,再加上近几年涌现出的数字压缩传输等新型科学技术,视音频压缩技术及数字传输技术应用越来越广泛。
技术越来越成熟,提高了数字音频节目压缩效率,能够实现数字化传输,为各项工作的应用提供了帮助。
数字视音频编解码技术数字视音频编解码技术涉及到的内容相对较多,再加上信源编码作为信息领域研究的重要组成具有一定的难度。
无论是哪种音频系统都需要遵循音频编码标准,这样可以通过激光视盘机、数字电视等多种电子产品进行优化,从而最大化发挥音视频编解码的效果。
实际应用过程中,为了确保图像质量得到保障,并具有较高的压缩率,需要合理地运用多种压缩技术,如比较常见的无失真压缩技术、有损压缩技术和频带压缩技术。
每一种压缩技术的应用都有一定的差异,所以为了能够最大化发挥各项压缩技术的优势,必须要事先对其有充足的了解,才能做好更全面的优化,最大化发挥技术应用的效果,使得数据传输率及传输质量得到不断提咼。
视讯技术在实际应用的过程中,需要根据实际情况将视频编码的图像数据进行压缩,为了能够全面保障图像编码质理的能力。
随着人工智能、大数据等技术的持续发展,结合视频技术的边缘计算将在网络边缘位置,为更多的垂直行业提供实时的智能化视频服务,扩展边缘视频的应用边界。
总的来说,不同场景案例的整体架构都符合“端边云”基本特征,面向视频领域的边缘计算的全面发展,也促进了边缘视频技术(边缘存储技术、边缘视频编解码技术、边缘视频智量,还应该注重对各项编辑过程中所产生的问题进行分析,为满足先进视频编码技术的各种需求组建联合视频工作组,在现有的基础上继续开发多种视频编码标准。
H.264编码技术和发展趋势
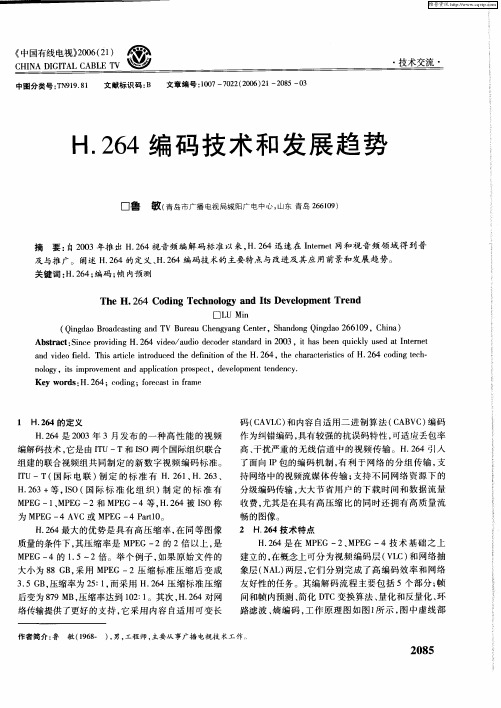
敏 (9 8 ) 男 , 16 一 , 工程 师, 主要从事广播 电视技 术工作.
2 8 0 5
维普资讯
鱼 敏:.6 编码技术和发展趋势 H 24
盟
塑 96 0 年第2 期 1
因为画面图像 的左右 、 上下肯定都有一定的相关性 , 所
持 网络 中的视 频流 媒体 传 输 ; 支持 不 同 网络 资 源 下 的 分级 编码传 输 , 大大 节 省 用 户 的下 载 时 间和 数 据 流 量
组建的联合视频组共同制 定的新数字视频编码标准。 IU—T( 际 电 联 ) 定 的 标 准 有 H 2 1 H. 6 、 T 国 制 . 6 、 2 3
H.6 24是 在 MP G一2、 E 一4技 术 基 础 之 上 E MP G
建立的 , 在概念上可分 为视频编码层 ( L ) V C 和网络抽 象层 ( A ) N L 两层 , 它们分别完 成了高编码效率和 网络 友好 性 的任务 。其 编 解 码 流 程 主 要包 括 5个 部 分 ; 帧
文献标识码 : B
H 2 4编 码 技 术 和 发 展 趋 势 .6
口鲁 敏 ( 青岛市广播电 视局城阳广电 中心, 东青岛26 9 山 61 ) 0
摘
要 : 03 自20 年推 出 H 2 4 .6 视音频编解码标准以来, .6 H 24迅速在 I e t n me 网和视音频领域得到普 t
1 H.6 2 4的定义
码 ( A L ) 内容 自适 用 二 进 制 算 法 ( A V 编 码 CVC和 C B C)
H 24是 20 年 3月发布 的一种高性能 的视频 .6 03
编解 码技术 , 是 由 IU— 它 T T和 IO 两个 国际组 织联 合 S
音频行业发展趋势及相关技术趋势
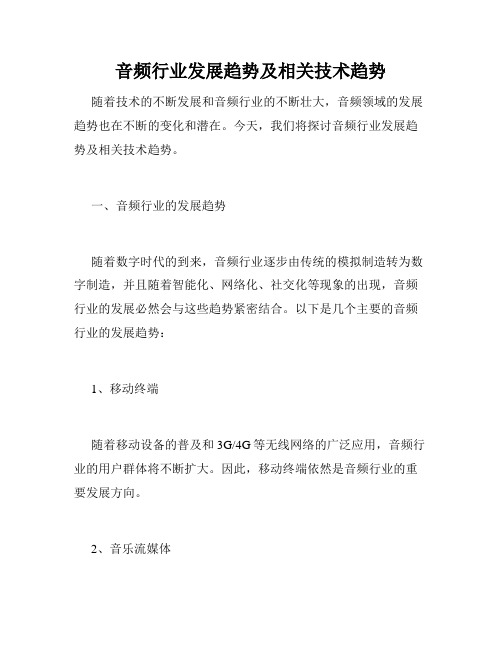
音频行业发展趋势及相关技术趋势随着技术的不断发展和音频行业的不断壮大,音频领域的发展趋势也在不断的变化和潜在。
今天,我们将探讨音频行业发展趋势及相关技术趋势。
一、音频行业的发展趋势随着数字时代的到来,音频行业逐步由传统的模拟制造转为数字制造,并且随着智能化、网络化、社交化等现象的出现,音频行业的发展必然会与这些趋势紧密结合。
以下是几个主要的音频行业的发展趋势:1、移动终端随着移动设备的普及和3G/4G等无线网络的广泛应用,音频行业的用户群体将不断扩大。
因此,移动终端依然是音频行业的重要发展方向。
2、音乐流媒体在音频领域,流媒体音乐服务将成为未来发展的主导形式之一。
通过音乐流媒体,消费者可以访问到大量的、全球的音乐数据。
同时,内容制作者能够跨越地理限制更加轻易地发布音频作品,以吸引更多的听众。
3、智能化智能音频将成为未来的主流。
以智能音箱为例,它们配备了人工智能语音助手,可执行各种任务,如搜索铃声、音乐、天气等,这将显著改善用户体验。
通过人工智能语音交互和合成技术,智能音频也可以实现更多的混合和新颖的体验,让用户更多地突破听觉界限。
二、相关技术趋势除音频行业的发展趋势外,技术也在不断地突破和发展,以下是几个主要的技术趋势:1、虚拟现实在虚拟现实技术的帮助下,用户可以在更加重视的音频环境中进行沉浸式的交互体验。
随着虚拟现实技术的不断拓展和深入,沉浸式音频体验将会更加普遍。
举个例子,你戴上虚拟现实头盔,就仿佛置身于现场,可以体验现场音乐演出前排的沉浸式音效。
2、3D音频和空间音频3D音频和空间音频可被视作对传统音频“平面”的一种补充。
通过这些新技术,用户可以在听音效果上得到更加丰富和生动的体验。
结合虚拟现实技术,通过空间音频用户可以体验仿佛置身于现场的效果。
研究表明,空间音频体验的增强可使听众更好的记忆他们听到的内容。
3、人工智能人工智能技术将会在音频行业各个领域施加影响,如音乐推荐、语音合成、音频识别等。
2024年数字电视编解码器市场前景分析
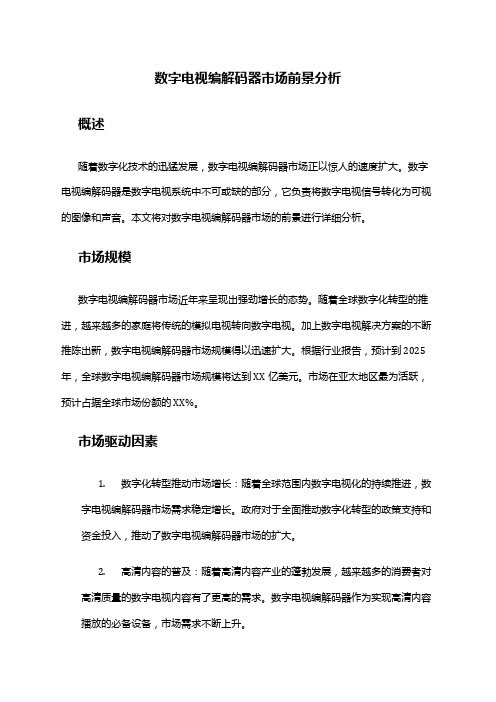
数字电视编解码器市场前景分析概述随着数字化技术的迅猛发展,数字电视编解码器市场正以惊人的速度扩大。
数字电视编解码器是数字电视系统中不可或缺的部分,它负责将数字电视信号转化为可视的图像和声音。
本文将对数字电视编解码器市场的前景进行详细分析。
市场规模数字电视编解码器市场近年来呈现出强劲增长的态势。
随着全球数字化转型的推进,越来越多的家庭将传统的模拟电视转向数字电视。
加上数字电视解决方案的不断推陈出新,数字电视编解码器市场规模得以迅速扩大。
根据行业报告,预计到2025年,全球数字电视编解码器市场规模将达到XX亿美元。
市场在亚太地区最为活跃,预计占据全球市场份额的XX%。
市场驱动因素1.数字化转型推动市场增长:随着全球范围内数字电视化的持续推进,数字电视编解码器市场需求稳定增长。
政府对于全面推动数字化转型的政策支持和资金投入,推动了数字电视编解码器市场的扩大。
2.高清内容的普及:随着高清内容产业的蓬勃发展,越来越多的消费者对高清质量的数字电视内容有了更高的需求。
数字电视编解码器作为实现高清内容播放的必备设备,市场需求不断上升。
3.5G技术的发展:5G技术的普及将极大提升数字电视的传输速度和质量,进一步推动了数字电视编解码器市场的发展。
高速稳定的5G网络为数字电视编解码器的运行提供了良好的基础,为市场的增长创造了有利条件。
市场挑战1.市场竞争加剧:数字电视编解码器市场竞争激烈,市场参与者众多,产品同质化程度较高。
为了在激烈的市场竞争中脱颖而出,厂商需要不断创新、提高产品性能,并降低成本。
2.专利技术限制:在数字电视编解码器市场,专利技术对于厂商来说具有重要意义。
某些技术的专利掌握在少数厂商手中,其他厂商进入市场时可能会受到技术限制,从而增加了市场准入的难度。
3.法规与标准的变化:数字电视编解码器市场受到政府政策和技术标准的影响较大。
频繁变化的法规和标准对厂商的生产和销售带来一定的不确定性,需要不断跟进和调整。
2023年视频解码器行业市场分析现状

2023年视频解码器行业市场分析现状视频解码器是一种对视频进行解码的设备或软件,用于将压缩的视频数据解码为可视化的图像和声音。
随着互联网的快速发展和视频内容的普及,视频解码器行业也迅速崛起。
这篇文章将对视频解码器行业的市场分析进行现状分析。
在过去几年中,视频解码器行业经历了快速的增长。
这主要是由于以下几个因素的影响:1. 视频内容的快速增长:随着互联网带宽的扩大和4G、5G等移动网络的普及,视频内容的数量和质量都得到了显著提升。
越来越多的用户愿意通过在线视频平台观看电影、电视剧和短视频等内容,这就需要视频解码器进行解码和播放。
2. 视频编码技术的发展:视频编码技术的快速发展也推动了视频解码器行业的增长。
目前最常用的视频编码标准是H.264和H.265,它们通过对视频进行压缩来减少数据传输和存储的成本。
视频解码器需要能够对这些编码标准进行解码,以便将压缩的视频转换为可视化的图像和声音。
3. 移动设备的普及:移动设备市场的快速增长也为视频解码器行业带来了机会。
越来越多的人使用智能手机和平板电脑观看视频内容,这就需要视频解码器进行解码和播放。
同时,移动设备的处理能力也得到了显著提升,可以更好地支持高清视频解码。
尽管视频解码器行业在过去几年中取得了快速增长,但仍然面临一些挑战和竞争:1. 压缩技术的不断发展:视频压缩技术在不断发展,新的视频编码标准不断出现。
尽管H.264和H.265目前是主流的编码标准,但未来可能会出现更先进的压缩技术。
视频解码器行业需要紧跟技术发展的步伐,不断更新和升级解码器,以适应不断变化的市场需求。
2. 市场竞争的加剧:随着视频解码器行业的增长,市场竞争也日益激烈。
许多公司进入这个行业,提供各种各样的视频解码器产品和服务。
在这种竞争环境中,视频解码器企业需要不断提高产品质量、降低价格,以赢得市场份额。
3. 视频内容版权的问题:视频解码器行业还面临着版权问题。
一些视频内容提供商对其内容进行了版权保护,视频解码器需要确保只有经过授权的用户可以观看受版权保护的内容。
音频网络传输技术的发展与应用
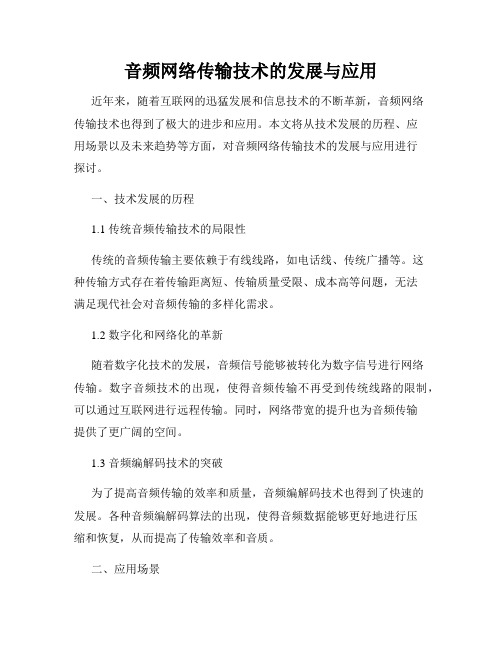
音频网络传输技术的发展与应用近年来,随着互联网的迅猛发展和信息技术的不断革新,音频网络传输技术也得到了极大的进步和应用。
本文将从技术发展的历程、应用场景以及未来趋势等方面,对音频网络传输技术的发展与应用进行探讨。
一、技术发展的历程1.1 传统音频传输技术的局限性传统的音频传输主要依赖于有线线路,如电话线、传统广播等。
这种传输方式存在着传输距离短、传输质量受限、成本高等问题,无法满足现代社会对音频传输的多样化需求。
1.2 数字化和网络化的革新随着数字化技术的发展,音频信号能够被转化为数字信号进行网络传输。
数字音频技术的出现,使得音频传输不再受到传统线路的限制,可以通过互联网进行远程传输。
同时,网络带宽的提升也为音频传输提供了更广阔的空间。
1.3 音频编解码技术的突破为了提高音频传输的效率和质量,音频编解码技术也得到了快速的发展。
各种音频编解码算法的出现,使得音频数据能够更好地进行压缩和恢复,从而提高了传输效率和音质。
二、应用场景2.1 音乐和娱乐领域随着音频网络传输技术的发展,现在人们可以通过互联网随时随地收听音乐、观看视频等。
各种音频在线平台如音乐App、视频网站等的出现,使得音乐和娱乐变得更加便捷和丰富。
用户只需连接网络,便可畅享高品质的音频内容。
2.2 会议和教育领域音频网络传输技术的应用还扩展到了会议和教育领域。
通过网络进行音频会议,可以实现不同地域之间的沟通和交流,提高工作效率。
同时,在线教育平台也借助音频网络传输技术,为学生提供高质量的远程教育服务。
2.3 车载音频系统随着智能汽车的快速发展,车载音频系统也得到了很大的改进。
通过将音频系统与互联网连接,车主可以随时收听在线音乐、订阅有声读物等。
音频网络传输技术的应用使得驾车过程更加愉悦和丰富。
三、未来趋势3.1 更高的音质和传输速度随着网络带宽的进一步提升,未来音频网络传输技术将会更加普及和发达。
新一代音频编解码技术的应用,将进一步提高音频传输的质量和传输速度,使用户可以享受更高品质的音频体验。
2024年数字信号处理器市场发展现状
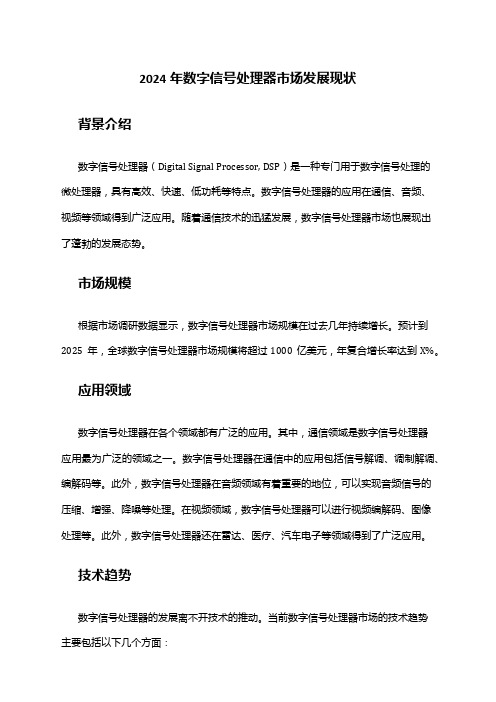
2024年数字信号处理器市场发展现状背景介绍数字信号处理器(Digital Signal Processor, DSP)是一种专门用于数字信号处理的微处理器,具有高效、快速、低功耗等特点。
数字信号处理器的应用在通信、音频、视频等领域得到广泛应用。
随着通信技术的迅猛发展,数字信号处理器市场也展现出了蓬勃的发展态势。
市场规模根据市场调研数据显示,数字信号处理器市场规模在过去几年持续增长。
预计到2025年,全球数字信号处理器市场规模将超过1000亿美元,年复合增长率达到X%。
应用领域数字信号处理器在各个领域都有广泛的应用。
其中,通信领域是数字信号处理器应用最为广泛的领域之一。
数字信号处理器在通信中的应用包括信号解调、调制解调、编解码等。
此外,数字信号处理器在音频领域有着重要的地位,可以实现音频信号的压缩、增强、降噪等处理。
在视频领域,数字信号处理器可以进行视频编解码、图像处理等。
此外,数字信号处理器还在雷达、医疗、汽车电子等领域得到了广泛应用。
技术趋势数字信号处理器的发展离不开技术的推动。
当前数字信号处理器市场的技术趋势主要包括以下几个方面:1. 高性能随着通信和多媒体应用的迅猛发展,用户对于数字信号处理器性能的要求越来越高。
数字信号处理器需要具备高处理能力和低延迟的特点,以满足复杂的信号处理需求。
2. 低功耗低功耗是数字信号处理器市场的一个重要趋势。
数字信号处理器需要在提供高性能的同时保持低功耗,以应对移动设备的发展和节能环保的需求。
3. 集成化随着半导体工艺的进步,数字信号处理器市场向着集成化发展。
集成化的数字信号处理器可以减少系统的成本和占用空间,并提高整体性能。
4. 实时性实时性是数字信号处理器市场的一个重要需求。
数字信号处理器需要能够快速响应和处理信号,以满足实时通信、音视频等应用的要求。
市场竞争格局数字信号处理器市场竞争激烈,主要厂商包括德州仪器(Texas Instruments)、美国ADI公司(Analog Devices Inc.)、NXP半导体等。
2024年视频解码器市场前景分析

2024年视频解码器市场前景分析概述视频解码器是一种广泛应用于数字媒体领域的技术,其作用是将压缩编码的视频数据进行解码还原为可显示的视频信号。
随着数字媒体产业的迅猛发展,视频解码器市场也呈现出蓬勃的增长态势。
本文将对视频解码器市场前景进行分析,深入探讨其发展趋势和潜在机遇。
市场发展趋势1. 视频流媒体的兴起随着互联网的普及和带宽的提升,视频流媒体服务如Netflix、YouTube等逐渐成为人们娱乐和学习的主要方式。
为了提供高质量的视频体验,视频解码器需具备高效的解码能力和优质的视频渲染性能。
2. 8K及以上分辨率的普及随着技术的进步,8K及以上分辨率的视频开始渐渐普及。
这种超高清晰度的视频需要更加复杂和高效的解码算法,从而对视频解码器提出了更高的要求。
3. AI技术的应用AI技术在视频解码器中的应用也呈现出巨大的潜力。
通过使用深度学习算法,视频解码器可以实现更有效的视频压缩和解码,从而提升视频播放的性能和质量。
市场机遇和挑战1. 市场机遇随着数字媒体产业的繁荣,视频解码器市场面临着巨大的机遇。
随着互联网的全球化普及,视频解码器的市场需求将会不断增长。
同时,随着人们对高质量视频体验的追求,对于优质视频解码器的需求也将不断增加。
2. 技术挑战视频解码器市场面临着一些技术挑战。
首先,随着分辨率的提升,视频数据的处理量将会成倍增加,对解码器的计算能力和内存需求提出了更高的要求。
其次,与解码性能相对应的是压缩比率的提升,解码器需要具备较好的算法设计和实现能力。
此外,AI技术的应用也给视频解码器的研发带来了挑战,需要解决算法优化和硬件支持等问题。
市场竞争态势1. 主要厂商目前,视频解码器市场上存在着若干主要的厂商,包括英特尔、NVIDIA、ARM等。
这些厂商在视频解码器的研发和推广方面具有较强实力和技术积累。
而随着人工智能技术的兴起,一些新兴厂商也开始涉足视频解码器市场,如华为、海思等。
2. 竞争模式当前视频解码器市场竞争激烈,主要表现为技术竞争和价格竞争。
音频技术的发展现状与未来趋势

音频技术的发展现状与未来趋势随着科技的快速发展,音频技术也在不断创新和改进。
音频技术广泛应用于音乐、电影、媒体、通信等领域,为人们带来了极大的便利和享受。
本文将探讨音频技术的发展现状以及未来的趋势。
一. 音频技术的发展现状1. 高清音质的普及随着音频技术的不断进步,高清音质的普及成为了一个主要的趋势。
传统的音质无法满足人们对音频的需求,而高清音质能够还原更真实的声音,带给人们更好的听觉享受。
比如,现在市面上的音频设备和音响系统都支持高清音质的播放,让用户能够更好地享受音乐和电影带来的震撼。
2. 虚拟现实的崛起虚拟现实技术的兴起也对音频技术提出了更高的要求。
虚拟现实技术能够让用户身临其境地感受到不同场景的视觉和听觉体验,而音频技术在其中扮演着重要角色。
通过使用立体声和3D声音技术,音频可以更加真实地还原现实世界的声音效果,提升用户的沉浸感。
3. 人工智能的应用人工智能的快速发展也给音频技术带来了革新。
语音助手如Siri、Alexa和谷歌助手等已经成为人们日常生活中不可或缺的部分。
人工智能的发展使得语音识别和语音合成技术得到了巨大的突破,使得音频技术在语音领域得到了广泛的应用。
二. 音频技术的未来趋势1. 空间音频技术随着虚拟现实和增强现实技术的发展,人们对于音频的要求也越来越高。
未来,空间音频技术有望成为热门的趋势。
通过使用更高级的声音处理算法和音响系统,空间音频技术能够模拟不同的音频环境,让用户感受到更加真实的声音效果。
2. 个性化音频体验随着音频技术的发展,个性化音频体验也将成为未来的趋势之一。
音频设备和应用程序将会根据用户的个人偏好和听觉特征来调整声音效果,以提供更适合用户的音频体验。
例如,根据用户的听力损失情况对音量和频率进行自动调整,使得每个人都能获得最佳的音频效果。
3. 物联网与音频技术的结合未来,随着物联网技术的普及,音频技术和物联网将会更加紧密地结合在一起。
用户可以通过智能音箱、智能音频设备等与其他物联网设备进行互联,实现更智能化和便捷化的音频体验。
关于下一代编解码技术的工作随想
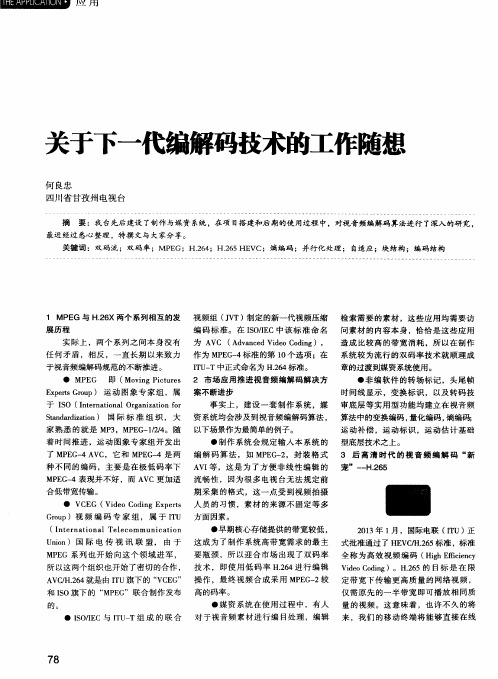
展 历 程
编 码 标 准。 在 I S O / I E C中该 标 准 命 名 问素材 的 内容本 身 ,恰 恰是 这些 应用
运动 补偿 ,运动 标识 ,运动 估计 基 础
S t a n d a r d i z a t i o n) 国 际 标 准 组 织 , 大 资 系统 均会涉及到 视音频编解码 算法 , 算法 中的变换 编码 , 量化编码 , 熵编码; 家 熟 悉 的就 是 MP 3 ,MP E G 一 1 / 2 / 4 。随 以下场景作为最简单 的例子 。 着 时 间推进 ,运 动 图象专 家组 开发 出 ●制 作系 统会 规定 输入 本 系统 的 型 底 层 技 术 之 上 。
7 8
( q u a d t r e e )的部 分 区域进行 A L F ,如 档案 博物 馆 藏 ,平 面传 媒类 ,广播 电 笔者 相 信 H. 2 6 5还是 能 够 一显 身 手 ,
果 是基 于 部分 区域 的 A L F ,还 必须 传 视 行业 ,互 联 网传播 行业 等 行业 与机 实际 上 电视 台的播控 系统 后端 至广 电 递指示 区域 信息的附加信息。
和I S O旗下 的 “ MP E G”联合 制作发 布 高的码率 。
的。
●媒 资 系统 在使 用过 程 中 ,有人 量 的视频 。这 意味 着 ,也许 不 久 的将
●I S O / I E C与 I T U — T组 成 的联 合 对 于视 音频 素材 进行 编 目处 理 ,编 辑 来 ,我 们 的移 动终 端将 能够直 接在 线
4 . 5并 行化设计
2023年数字电视编解码器行业市场发展现状
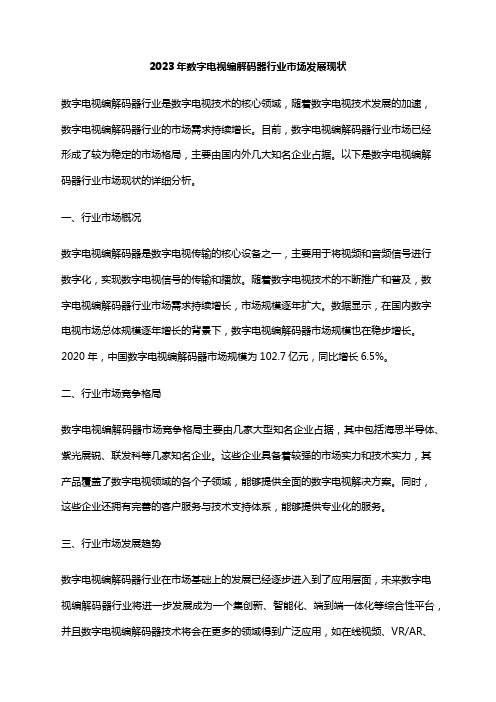
2023年数字电视编解码器行业市场发展现状数字电视编解码器行业是数字电视技术的核心领域,随着数字电视技术发展的加速,数字电视编解码器行业的市场需求持续增长。
目前,数字电视编解码器行业市场已经形成了较为稳定的市场格局,主要由国内外几大知名企业占据。
以下是数字电视编解码器行业市场现状的详细分析。
一、行业市场概况数字电视编解码器是数字电视传输的核心设备之一,主要用于将视频和音频信号进行数字化,实现数字电视信号的传输和播放。
随着数字电视技术的不断推广和普及,数字电视编解码器行业市场需求持续增长,市场规模逐年扩大。
数据显示,在国内数字电视市场总体规模逐年增长的背景下,数字电视编解码器市场规模也在稳步增长。
2020年,中国数字电视编解码器市场规模为102.7亿元,同比增长6.5%。
二、行业市场竞争格局数字电视编解码器市场竞争格局主要由几家大型知名企业占据,其中包括海思半导体、紫光展锐、联发科等几家知名企业。
这些企业具备着较强的市场实力和技术实力,其产品覆盖了数字电视领域的各个子领域,能够提供全面的数字电视解决方案。
同时,这些企业还拥有完善的客户服务与技术支持体系,能够提供专业化的服务。
三、行业市场发展趋势数字电视编解码器行业在市场基础上的发展已经逐步进入到了应用层面,未来数字电视编解码器行业将进一步发展成为一个集创新、智能化、端到端一体化等综合性平台,并且数字电视编解码器技术将会在更多的领域得到广泛应用,如在线视频、VR/AR、自动驾驶等领域。
未来,数字电视编解码器行业市场将会强化个性化需求、升级需求以及网络化趋势这三个主要市场趋势,为行业发展提供了广阔的空间。
总体来看,数字电视编解码器行业市场已经进入到了快速发展的阶段,未来数字电视编解码器行业将会进一步拓展其市场空间,增强智能化应用场景,满足数字生活的不断升级需求。
在这个领域的企业要抓住机遇,抢占市场份额,发挥自身优势,不断提高技术水平,提供质量更好的产品与服务。
网络音频技术的发展趋势和应用场景

网络音频技术的发展趋势和应用场景随着互联网的普及,网络音频技术的应用越来越广泛。
它不仅可以通过网络传输音频信号,而且可以实现音频的编解码、处理、存储等功能。
尤其是在近年来,随着音频内容的迅速增长,网络音频技术得到了极大的发展,并且在各个领域中得到了广泛应用。
那么,网络音频技术的发展趋势和应用场景是怎样的呢?下面我们从三个方面来论述。
一、网络音频编解码技术的发展趋势网络音频编解码(Codec)技术是当今网络音频技术中最核心的技术之一,其主要作用是将音频的模拟信号转换成数字信号,又将数字信号转换为模拟信号,以达到在网络上传输和存储音频的目的。
如今,网络音频编解码技术发展呈现以下趋势:1. 高效性网络音频技术要求编解码器在保留高质量音频的同时,必须保持高效。
因此,随着技术进步的发展,越来越多的音频编解码器开始更好地结合了质量和效率。
2. 实时性随着网络带宽的逐渐提高、延迟的逐渐降低,网络音频技术应用的领域不断扩大,对实时性的要求也越来越高。
声音在网络中的传输需要确保声音的信息不会因为延迟而丢失。
3. 跨平台网络音频技术面对的是各种不同的设备和平台,不同厂家之间的原始格式虽然有些差异,但是网络音频技术要满足不同设备和平台之间的互通性。
二、网络音频技术的应用场景网络音频技术的应用场景非常广泛,它已经成功地应用于音乐、广播、新媒体、在线教育、电商、智能汽车等领域。
下面列举了其中的几个应用场景:1. 在线音乐随着音乐产业的不断发展,越来越多的用户倾向于使用在线音乐服务,例如Spotify、Apple Music等。
利用网络音频技术,用户可以简便地从在线音乐服务中下载或流式播放高质量的音乐。
2. 在线广播在线广播也是网络音频技术的一个重要应用场景,其需要的声音传输和编解码技术相对比较简单,但是广播的内容涵盖面非常广泛,因此要求在传输和编解码中要有高效性和准确性。
3. 在线教育在线教育早已融入人们的学习生活。
网络音频技术的发展让媒体教学变得更加多样化和丰富,可以在课上直播和录播,每个人都可以在各自的时间和地点学习。
前途光明道路曲折 SVAC标准前景分析
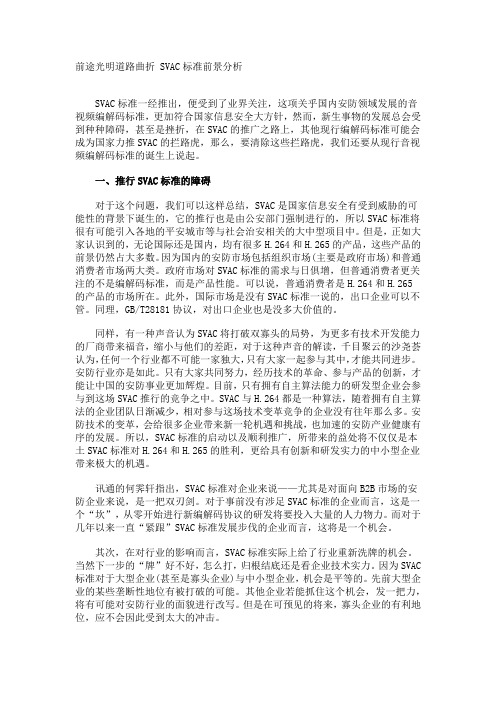
前途光明道路曲折 SVAC标准前景分析SVAC标准一经推出,便受到了业界关注,这项关乎国内安防领域发展的音视频编解码标准,更加符合国家信息安全大方针,然而,新生事物的发展总会受到种种障碍,甚至是挫折,在SVAC的推广之路上,其他现行编解码标准可能会成为国家力推SVAC的拦路虎,那么,要清除这些拦路虎,我们还要从现行音视频编解码标准的诞生上说起。
一、推行SVAC标准的障碍对于这个问题,我们可以这样总结,SVAC是国家信息安全有受到威胁的可能性的背景下诞生的,它的推行也是由公安部门强制进行的,所以SVAC标准将很有可能引入各地的平安城市等与社会治安相关的大中型项目中。
但是,正如大家认识到的,无论国际还是国内,均有很多H.264和H.265的产品,这些产品的前景仍然占大多数。
因为国内的安防市场包括组织市场(主要是政府市场)和普通消费者市场两大类。
政府市场对SVAC标准的需求与日俱增,但普通消费者更关注的不是编解码标准,而是产品性能。
可以说,普通消费者是H.264和H.265的产品的市场所在。
此外,国际市场是没有SVAC标准一说的,出口企业可以不管。
同理,GB/T28181协议,对出口企业也是没多大价值的。
同样,有一种声音认为SVAC将打破双寡头的局势,为更多有技术开发能力的厂商带来福音,缩小与他们的差距,对于这种声音的解读,千目聚云的沙尧荟认为,任何一个行业都不可能一家独大,只有大家一起参与其中,才能共同进步。
安防行业亦是如此。
只有大家共同努力,经历技术的革命、参与产品的创新,才能让中国的安防事业更加辉煌。
目前,只有拥有自主算法能力的研发型企业会参与到这场SVAC推行的竞争之中。
SVAC与H.264都是一种算法,随着拥有自主算法的企业团队日渐减少,相对参与这场技术变革竞争的企业没有往年那么多。
安防技术的变革,会给很多企业带来新一轮机遇和挑战,也加速的安防产业健康有序的发展。
所以,SVAC标准的启动以及顺利推广,所带来的益处将不仅仅是本土SVAC标准对H.264和H.265的胜利,更给具有创新和研发实力的中小型企业带来极大的机遇。
音频科技的发展现状与未来趋势

音频科技的发展现状与未来趋势随着科技的不断进步和人们生活水平的提高,音频科技也在快速发展。
从最初的录音机到今天的智能音箱,音频科技已经在我们日常生活中起到了不可或缺的作用。
本文将探讨音频科技的发展现状以及未来的趋势。
近年来,随着无线技术的成熟和智能设备的普及,无线音频技术得到了广泛应用。
蓝牙耳机和无线音箱已经成为人们生活中常见的配件,不再需要纠缠的线缆,让人们更方便地享受音频娱乐。
与此同时,随着互联网的发展,音频流媒体服务也得到了迅猛发展,音乐、播客和有声书等内容都可以通过网络随时随地访问。
这不仅丰富了人们的娱乐选择,还为音频科技的发展提供了更广阔的空间。
除了无线技术的应用,声音识别技术也是音频科技中的重要一环。
随着人工智能的进步,声音识别技术的准确度和实用性也在不断提高。
声控智能助手可以通过声音指令来执行各种任务,比如播放音乐、查找信息、控制智能家居等。
人们不再需要手动操控设备,只需要用声音来交互,实现了更加便捷和智能化的操作体验。
音频科技的发展也渗透到了各个领域。
在汽车行业,车载音响系统已经从简单的收音机升级为具有多声道立体声效果的高级音响系统。
一些高端汽车还配备了主动降噪系统,通过分析车内的噪音来源并发出相反的声波来抵消噪音,提供更好的驾乘体验。
此外,无人驾驶也可以利用音频传感器来检测周围的声音,实现更精确的环境感知。
音频技术还在医疗领域发挥着重要作用。
听觉辅助设备,如助听器和人工耳蜗,帮助那些有听力问题的人恢复听觉功能,提高生活质量。
音频技术还广泛应用于声波疗法和声波刀等医疗设备中,用于治疗各种疾病,如肿瘤和创伤。
未来,音频科技有望得到更多的突破和创新。
首先,随着5G技术的普及,音频流媒体服务将进一步提升,高清音频内容将更加流畅地传输到用户设备上。
其次,虚拟现实和增强现实技术也将与音频科技紧密结合,创造出逼真的声音效果,提升用户的沉浸感。
此外,声音合成技术和声音分析算法的进步将实现更加自然、逼真的人工声音生成,带来更多创新的音频应用。
视频编码技术的新进展与应用前景

视频编码技术的新进展与应用前景视频编码是当今互联网领域中广泛应用的一个技术,涉及到多媒体信息的处理和传输。
可以说,视频编码对于视频网站、短视频、在线教育、在线会议等领域的快速发展起着至关重要的作用。
不断地持续创新是视频编码技术的核心,为了提高传输和存储效率,各种编码技术愈加被追捧,技术进步不断推动着视频编码技术的发展,让我们一同探讨一下视频编码技术的新进展与应用前景。
一、HEVC编码标准HEVC (High Efficiency Video Coding)是一种视频压缩标准,继承了H.264/AVC AVC的优点,同时将其压缩率提高了50%左右。
HEVC对于4K、8K等高分辨率的视频有很好的效果,可以将视频的数据压缩到更小的尺寸,降低了传输和存储的成本,并且在保证视觉效果的前提下,能够实现更加高效的数据传输,有着广泛的应用前景。
二、AV1编码技术AV1是由Alliance for Open Media组织实现的最新视频编解码器,能够比HEVC和H.264/AVC在同等条件下提供更好的图像质量,同时实现更高的视频压缩性能。
它采用了先进的技术来减少视频文件的大小,如多输出量量化、非精细分割、深度学习、帧内预测等。
AV1压缩密度更高,能够让网站流量更少,更快速的启动视频,并且支持HDR和WCG等新的视频标准,具有明显的优势。
三、AI技术在视频编码中的应用近年来,AI技术的飞速发展为视频编码技术的发展注入了新的活力,人工智能视频编码技术可以实现更加智能的视音频数据处理和压缩,同样可以降低数据传输和存储的成本,并且可以为用户提供更好的视觉体验。
在视频编码中,AI技术可以运用在如下领域:1)视频内容的识别与分割,根据不同的物体区域来实现动态量化;2)基于AI的视频提取技术,提供更新、更精确的视频画面移动特征的提取;3)根据用户设备的特性,选择最佳的视频编码参数等等。
AI技术为视频编码技术开拓了广泛的应用领域。
音视频编解码技术研究与应用面临的挑战
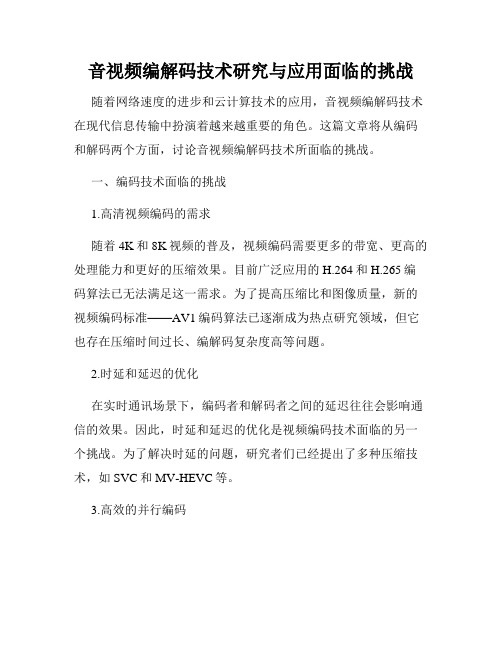
音视频编解码技术研究与应用面临的挑战随着网络速度的进步和云计算技术的应用,音视频编解码技术在现代信息传输中扮演着越来越重要的角色。
这篇文章将从编码和解码两个方面,讨论音视频编解码技术所面临的挑战。
一、编码技术面临的挑战1.高清视频编码的需求随着4K和8K视频的普及,视频编码需要更多的带宽、更高的处理能力和更好的压缩效果。
目前广泛应用的H.264和H.265编码算法已无法满足这一需求。
为了提高压缩比和图像质量,新的视频编码标准——AV1编码算法已逐渐成为热点研究领域,但它也存在压缩时间过长、编解码复杂度高等问题。
2.时延和延迟的优化在实时通讯场景下,编码者和解码者之间的延迟往往会影响通信的效果。
因此,时延和延迟的优化是视频编码技术面临的另一个挑战。
为了解决时延的问题,研究者们已经提出了多种压缩技术,如SVC和MV-HEVC等。
3.高效的并行编码并行编码技术能够提高编码的速度和效率,特别适用于大规模的视频数据。
但是,并行编码技术需要克服很多困难,如编码器和解码器之间的数据依赖性、束缚和资源限制等。
4.可扩展的编码可扩展编码技术允许编码器根据网络和设备的情况,自动调整视频的分辨率和码率,以适应网络流量的需求。
但是,实现可扩展编码技术需要克服很多挑战,如数据同步和码率控制等。
二、解码技术面临的挑战1.实时解码实时解码需要处理大量的数据,这就需要在短时间内完成解码功能。
因此,在一些场景中,如视频通信和监控等实时性要求较高的应用场景,解码技术面临更严峻的挑战。
2.网络环境的不稳定性网络环境是解码技术面临的另一个挑战。
从视频源发送到接收方,视频数据需要经过各种网络设备。
其中,网络延迟、网络出错和丢包等因素都会影响视频数据的传输质量,进而影响解码质量。
3.视频解码质量的提升视频解码质量的提升是解码技术面临的另一个挑战。
在图像压缩过程中,由于带宽和存储容量的限制,一些细节信息往往会失真或丢失,进而影响视频解码的质量。
音视频编解码技术的现状和未来发展
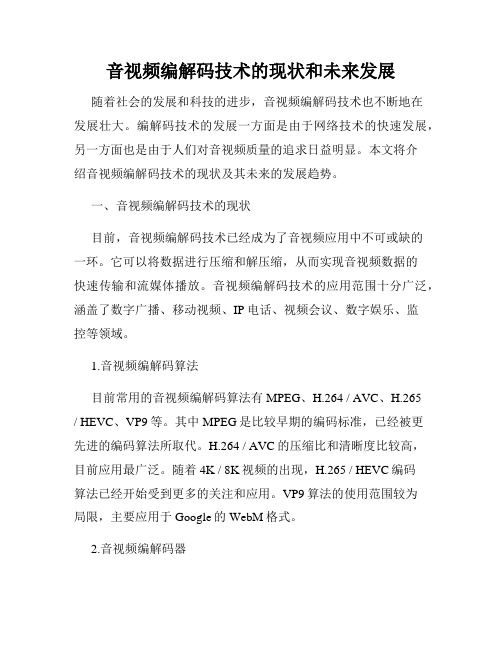
音视频编解码技术的现状和未来发展随着社会的发展和科技的进步,音视频编解码技术也不断地在发展壮大。
编解码技术的发展一方面是由于网络技术的快速发展,另一方面也是由于人们对音视频质量的追求日益明显。
本文将介绍音视频编解码技术的现状及其未来的发展趋势。
一、音视频编解码技术的现状目前,音视频编解码技术已经成为了音视频应用中不可或缺的一环。
它可以将数据进行压缩和解压缩,从而实现音视频数据的快速传输和流媒体播放。
音视频编解码技术的应用范围十分广泛,涵盖了数字广播、移动视频、IP电话、视频会议、数字娱乐、监控等领域。
1.音视频编解码算法目前常用的音视频编解码算法有MPEG、H.264 / AVC、H.265/ HEVC、VP9等。
其中MPEG是比较早期的编码标准,已经被更先进的编码算法所取代。
H.264 / AVC的压缩比和清晰度比较高,目前应用最广泛。
随着4K / 8K视频的出现,H.265 / HEVC编码算法已经开始受到更多的关注和应用。
VP9算法的使用范围较为局限,主要应用于Google的WebM格式。
2.音视频编解码器音视频编解码器是用来处理和解析音视频数据的软硬件系统。
常见的编解码器软件有FFmpeg、x264、x265等。
硬件编解码器通常是嵌入式设备或专用处理器芯片,可以提供高速的性能和流媒体处理能力。
3.音视频编解码技术的应用音视频编解码技术已经应用于多种领域,包括数字广播、移动视频、在线教育、视频监控等。
其中,网络视频应用的发展非常迅速,较为成功的代表有优酷、爱奇艺、腾讯视频等娱乐平台。
随着全球市场不断扩大,音视频编解码技术的应用领域也会越来越广泛。
二、音视频编解码技术的未来发展趋势尽管音视频编解码技术已经得到广泛的应用,但是未来它仍然有很多的进步空间。
以下是音视频编解码技术未来的发展趋势:1.更高的压缩比和更高的清晰度音视频编解码技术的目的是将音视频数据进行压缩,以便更高效的传输。
未来,我们期望有更高的压缩比和更高的清晰度,从而满足日益增长的带宽需求,同时提高视频质量。
- 1、下载文档前请自行甄别文档内容的完整性,平台不提供额外的编辑、内容补充、找答案等附加服务。
- 2、"仅部分预览"的文档,不可在线预览部分如存在完整性等问题,可反馈申请退款(可完整预览的文档不适用该条件!)。
- 3、如文档侵犯您的权益,请联系客服反馈,我们会尽快为您处理(人工客服工作时间:9:00-18:30)。
视音频编解码技术发展现状和展望(四)4视音频编解码技术展望由于数字视频编码的核心是对信号进行压缩,所以不断提高编码压缩效率仍是混合编码的主要发展目标。
但是追求更高的压缩效率需要对传统的“变换+运动补偿+基于视觉的量化+熵编码”框架有所突破,给视频编码性能带来新的提升。
可伸缩的视频编码技术因为具有良好的网络适应性,所以围绕它的应用,尤其是网络环境下的应用,会越来越多。
可以预见,在未来的网络视频监控中,可伸缩技术将是保证网络传输质量的一个重要实现技术。
而多视点编码方法的研究会集中在多视点视频的采集与校准,场景深度及几何信息获取(立体匹配),多视点视频编码,多视点视频通信,新视图渲染以及最终的交互或立体显示等6大关键上,这些技术的突破会为自由视点电视(FTV)、立体电视(3DTV)和沉浸感视频会议的应用提供技术支持。
作为SVC、MVC等各类视频编码的基础,混合框架的编码仍有很强的生命力。
同时随着网络、通信、娱乐业对数字媒体的广泛需求,A VS、H.264这一代标准被普遍接受,相应的产品开发工作相当重要。
包括编解码芯片、整机和系统。
应用领域涉及数字电视、卫星电视、移动电视、手机电视、网络电视、时移电视机、新一代光盘存储媒体、安防监控、智能交通、会议电视、可视电话、数字摄像机等等。
其中,安防监控领域是音视频编解码技术的主要应用领域之一。
编解码技术在这个领域的应用,需要结合安防监控领域的特殊需求进行研究。
只有在这个方向掌握有自主知识产权的核心技术,我国的安防监控产业才能健康持续的发展。
5 参考文献1. ThomasWiegand, G.J.S., Senior Member, IEEE, Gisle Bjøntegaard, and Ajay Luthra, SeniorMember, IEEE, Overview of the H.264/AVC Video Coding Standard. IEEE TRANSACTIONS ON CIRCUITS AND SYSTEMS FOR VIDEO TECHNOLOGY, 2003. 13(7): p. 17.2. 周秉锋, 郑.叶., JVT草案中的核心技术综述.软件学报, 2004. 15(1): p. 11.3. Ostermann, J., Hybrid Coding: Where Can Future Ga ins Come from?” 2005.4. Julien Reichel, H.S., Mathias Wien, Scalable Video Coding – Working Draft 2, JVT, Editor.2005.5. Dr Francesco Ziliani, J.-C.M., Scalable Video Coding In Digital Video Security. 2005. p. 19.6. Wallace Kai-Hong Ho; Wai-Kong Cheuk; Lun, D.P.-K., Content-based scalable H.263 videocoding for road traffic monitoring. IEEE Transactions on Multimedia, 2005. 7(4): p. 9.7. Ser-Nam Lim; Davis, L.S.E., A., Scalable image-based multi-camera visual surveillancesystem, in AVSS.2003. 2003.8. Nicolas, H., Scalable video compression scheme for tele-surveillance applications based oncast shadow detection and modelling, in Image Processing, 2005. ICIP 2005. IEEE International Conference on. 2005.9. May, A.T., J.; Hobson, P.; Ziliani, F.; Reichel, J.;, Scalable video requirements for surveillanceapplications. Intelligent Distributed Surveilliance Systems, IEE, 2004: p. 4.10. 陶钧, 王., 张军, 姜志宏, 三维小波视频编码的可伸缩性研究.小型微型计算机系统,2005. 26(2).11. ping., L.Y., A true th ree2dimension wavelet transfo rmtechnique and its app lication, in Video Image Coding-Electronic Engineering. 2002. p. 52-59.12. Wen-Hsiao Peng, C.-Y.T., Tihao Chiang, and Hsueh-Ming Hang, Advances of MPEG ScalableVideo Coding Standard. 2005.13. Robert T. Collins, A.J.L., Takeo Kanade,, et al., A System for Video Surveillance andMonitoring. 2000, The Robotics Institute, Carnegie Mellon University, Pittsburgh PA. p. 69.14. Ismail Haritaoglu, M., IEEE, David Harwood, Member, IEEE, and Larry S. Davis, Fellow,IEEE, W4:Real-Time Surveillance of People and Their Activities.IEEE TRANSACTIONS ON PATTERN ANALYSIS AND MACHINE INTELLIGENCE, 2000. 22(8).15. A VC, I.-T.R.I.I.-. Advanced Video Coding for Generic Audiovisual Service. 2005.16. Robert Pless, T.B.y., and Yiannis Aloimonos, Detecting Independent Motion: The Statistics ofTemporal Continuity. IEEE TRANSACTIONS ON PA TTERN ANALYSIS AND MACHINE INTELLIGENCE, 2000. 22(8): p. 6.17. L. Wixson, M., IEEE Computer Society, Detecting Salient Motion by Accumulating Directionally-Consistent Flow. IEEE TRANSACTIONS ON PA TTERN ANALYSIS AND MACHINE INTELLIGENCE, 2000. 22(8).18. Medioni, I.C.G., Detecting and Tracking Moving Objects for Video Surveillance. 1999.19. Tarak Gandhi, M.M.T., et al., Motion Analysis of OmniDirectional Video Streams for a MobileSentry. 2003.20. Kakadiaris, C.B.o.a.I.A., et al., A Convex Penalty Method For Optical Human MotionTracking. 2003.21. Zhang, Z.M., et al., Independent Motion Detection Directly from Compressed SurveillanceVideo. 2003.22. G., W.G.a.B. An introduction to the Kalman filter.2000 [cited; Available from:.23. A., I.M.a.B., Condensation—conditional density propagation for visual tracking. InternationalJournal of Computer Vision, 1998. 25(1): p. 24.24. Pavlović V, R.J., Cham T-J and Murphy K., A dynamic Bayesian network approach to figuretracking using learned dynamic models, in IEEE International Conference on Computer Vision,. 1999: Corfu, Greece,.25. Chris Stauffer, M., IEEE, and and M. W. Eric L. Grimson, IEEE, Learning Patterns of ActivityUsing Real-Time Tracking.IEEE TRANSACTIONS ON PA TTERN ANALYSIS AND MACHINE INTELLIGENCE, 2000. 22(8).26. Bouthemy, Y.R.a.P., Real-Time Tracking of Moving Persons by Exploiting Spatio-TemporalImage Slices.IEEE TRANSACTIONS ON PATTERN ANALYSIS AND MACHINE INTELLIGENCE, 2000. 22(8).27. Jinman Kang, I.C., Gérard Medioni, U.C.V.G. IRIS, and U.o.S. California, Multi-ViewsTracking Within and Across Uncalibrated Camera Streams. 2003.28. Hang-Bong Kang, S.-H.C., D.o.c. Eng., and T.C.U.o. Korea, Adaptive Object Tracking usingBayesian Network and Memory. 2004.29. Bruno Müller Junior, R.d.O.A., I.d. Computaçao, and U.E.d. Campinas, Distributed RealTimeSoccer Tracking. 2004.30. R. Cucchiara, C.G., G. Tardini and D.d.I.I.-U.o.M.a.R. Emilia, Track-based and Object-basedOcclusion for People Tracking Refinement in Indoor Surveillance. 2004.31. Cash J. Costello, C.P.D., Amit Banerjee, Hesky Fisher, A.P. Laboratory, and J.H. University,Scheduling an Active Camera to Observe People. 2004.32. Marco Cristani, M.B., Vittorio Murino, U.o. Verona, and D.d. Informatica, MultilevelBackground Initialization using Hidden Markov Models. 2003.33. Collins, R.T.L., A.J.; Fujiyoshi, H.; Kanade, T., Algorithms for cooperative multisensorsurveillance. Proceedings of the IEEE, 2001. 89(10): p. 12.34. Utsumi A, M.H., Ohya J and Yachida M., Multiple-view-based tracking of multiple humans.,in IEEE International Conference on Pattern Recognition. 1998: Brisbane, Australia,.35. J., C.Q.a.A., Tracking human motion using multiple cameras., in International Conference onPattern Recognition. 1996: Vienna.36. Marchesotti, L.M., L.; Regazzoni, C.;, A video surveillance architecture for alarm generationand video sequences retrieval, in 2002 International Conference on Image Processing (ICIP2002). 2002.37. Sangho Park, J.K.A., et al., Recognition of Two-person Interactions Using a HierarchicalBayesian Network. 2003.38. Spagnolo, M.L.T.D.O.P., I.o.I.S. for, and Automation, Human Activity Recognition forAutomatic VisualSurveillance of Wide Areas. 2004.(作者单位:武汉大学国家多媒体软件工程技术研究中心)。