分类与决策树
分类分析--决策树(经典决策树、条件推断树)

分类分析--决策树(经典决策树、条件推断树)分类分析--决策树决策树是数据挖掘领域中的常⽤模型。
其基本思想是对预测变量进⾏⼆元分离,从⽽构造⼀棵可⽤于预测新样本单元所属类别的树。
两类决策树:经典树和条件推断树。
1 经典决策树经典决策树以⼀个⼆元输出变量(对应威斯康星州乳腺癌数据集中的良性/恶性)和⼀组预测变量(对应九个细胞特征)为基础。
具体算法如下:(1) 选定⼀个最佳预测变量将全部样本单元分为两类,实现两类中的纯度最⼤化(即⼀类中良性样本单元尽可能多,另⼀类中恶性样本单元尽可能多)。
如果预测变量连续,则选定⼀个分割点进⾏分类,使得两类纯度最⼤化;如果预测变量为分类变量(本例中未体现),则对各类别进⾏合并再分类。
(2) 对每⼀个⼦类别继续执⾏步骤(1)。
(3) 重复步骤(1)~(2),直到⼦类别中所含的样本单元数过少,或者没有分类法能将不纯度下降到⼀个给定阈值以下。
最终集中的⼦类别即终端节点(terminal node)。
根据每⼀个终端节点中样本单元的类别数众数来判别这⼀终端节点的所属类别。
(4) 对任⼀样本单元执⾏决策树,得到其终端节点,即可根据步骤3得到模型预测的所属类别。
上述算法通常会得到⼀棵过⼤的树,从⽽出现过拟合现象。
结果就是,对于训练集外单元的分类性能较差。
为解决这⼀问题,可采⽤10折交叉验证法选择预测误差最⼩的树。
这⼀剪枝后的树即可⽤于预测。
R中的rpart包⽀持rpart()函数构造决策树,prune()函数对决策树进⾏剪枝。
下⾯给出判别细胞为良性或恶性的决策树算法实现。
(1)使⽤rpart()函数创建分类决策树:#⽣成树:rpart()函数可⽤于⽣成决策树library(rpart)set.seed(1234)dtree <- rpart(class ~ ., data=df.train, method="class",parms=list(split="information"))#rpart() 返回的cptable值中包括不同⼤⼩的树对应的预测误差,因此可⽤于辅助设定最终的树的⼤⼩。
深入浅出决策树分类(精彩故事引入)

03
数据划分
将数据集划分为训练集、验证集和测试集,以便进行模型训练和评估。
特征工程在决策树分类中应用示例
特征选择
01
通过计算特征重要性、绘制特征相关性热力图等方法,选择对
分类结果影响较大的特征。
特征构造
02
根据业务理解和数据探索,构造新的特征以增强模型的表达能
力。
特征转换
03
通过特征离散化、特征交互等方式,将原始特征转换为更适合
04 决策树分类实践案例分析
数据集选择与预处理操作指南
01
数据集选择
选择适合决策树分类的数据集,如Iris、Titanic等经典数据集,或根据
实际业务需求选择相关数据集。
02
数据预处理
包括数据清洗(处理缺失值、异常值等)、特征编码(将非数值特征转
换为数值特征)、特征缩放(将不同特征缩放到同一尺度)等操作。
采用适当的剪枝策略,简化决策树结 构,避免过拟合现象。
集成学习
将多个决策树结合起来,形成随机森 林等集成学习方法,提高分类性能和 稳定性。
特征选择
在构建决策树前,进行特征选择和降 维处理,以提高分类效率和准确性。
引入领域知识
在构建决策树时引入领域知识,指导 决策树的生成和剪枝过程。
06 拓展知识:集成学习方法 在决策树中应用
Bagging是一种并行式的集成学习 方法,它基于自助采样法,通过有 放回地重复采样来生成多个不同的 数据集,然后对每个数据集独立地 训练出一个基学习器,最后将这些 基学习器的预测结果进行结合。
Boosting是一种串行式的集成学 习方法,它通过改变训练样本的 权重,使得每轮训练都更加关注 于前一轮被错误分类的样本,从 而逐步提高学习器的性能。
分类及其应用案例
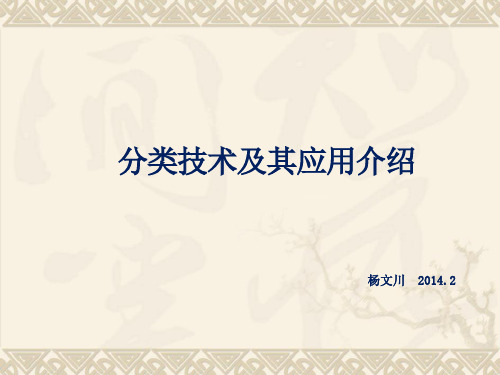
添加数据源节点
编辑“数据源节点”
节点重命名
加入表节点
“选择”节点
A1="?"
or @NULL(A2) or @NULL(A3) or A4="?" or A5="?" or A6="?" or A7="?" or @NULL(A8) or A9="?" or A10="?" or @NULL(A11) or A12="?" or A13="?" or @NULL(A14) or @NULL(A15) or A16="?"
设S代表训练数据集,由s个样本组成。A是
S的某个属性,有m个不同的取值,根据这 些取值可以把S划分为m个子集,Si表示第i 个子集(i=1,2,…,m),|Si|表示子集Si中的 样本数量。那么:
| Si | | Si | Split _ Info( S , A) ( log 2 ) s s i 1
If (年龄<40) and (职业=“学生” or职业=“教师”) Then 信用等级 =“优” If (年龄<40) and (职业!=“学生”and职业!=“教师”) Then 信用等级 =“良” If (年龄≥40) and (月薪<1000) Then 信用等级=“差” If (年龄≥40) and (月薪≥1000 and月薪≤3000) Then 信用等级=“良” If (年龄≥40) and (月薪>3000) Then 信用等级=“优”
,
“类型”节点
“抽样”节 点
“C5.0”节点
生成的决策树模型
决策树分类算法的研究及其在电力营销中的应用
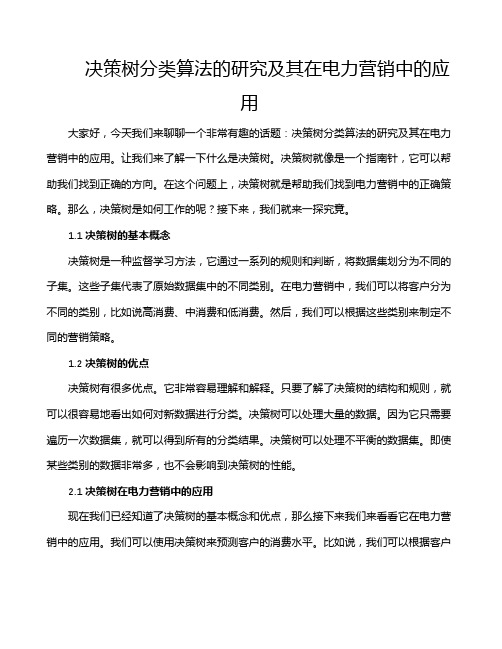
决策树分类算法的研究及其在电力营销中的应用大家好,今天我们来聊聊一个非常有趣的话题:决策树分类算法的研究及其在电力营销中的应用。
让我们来了解一下什么是决策树。
决策树就像是一个指南针,它可以帮助我们找到正确的方向。
在这个问题上,决策树就是帮助我们找到电力营销中的正确策略。
那么,决策树是如何工作的呢?接下来,我们就来一探究竟。
1.1 决策树的基本概念决策树是一种监督学习方法,它通过一系列的规则和判断,将数据集划分为不同的子集。
这些子集代表了原始数据集中的不同类别。
在电力营销中,我们可以将客户分为不同的类别,比如说高消费、中消费和低消费。
然后,我们可以根据这些类别来制定不同的营销策略。
1.2 决策树的优点决策树有很多优点。
它非常容易理解和解释。
只要了解了决策树的结构和规则,就可以很容易地看出如何对新数据进行分类。
决策树可以处理大量的数据。
因为它只需要遍历一次数据集,就可以得到所有的分类结果。
决策树可以处理不平衡的数据集。
即使某些类别的数据非常多,也不会影响到决策树的性能。
2.1 决策树在电力营销中的应用现在我们已经知道了决策树的基本概念和优点,那么接下来我们来看看它在电力营销中的应用。
我们可以使用决策树来预测客户的消费水平。
比如说,我们可以根据客户的年龄、性别、职业等因素,构建一个决策树模型。
然后,我们可以用这个模型来预测客户的消费水平。
这样一来,我们就可以根据客户的消费水平来制定不同的营销策略。
2.2 如何构建一个有效的决策树模型要构建一个有效的决策树模型,我们需要遵循以下几个步骤:(1) 确定目标变量:在电力营销中,我们的目标变量是客户的消费水平。
所以我们需要先确定这个目标变量。
(2) 选择特征:特征是指影响目标变量的因素。
在电力营销中,我们可以选择很多特征,比如说客户的年龄、性别、职业等。
但是要注意的是,特征的数量不能太多,否则会导致过拟合的问题。
一般来说,我们可以选择10个左右的特征。
(3) 划分数据集:我们需要将原始数据集划分为训练集和测试集。
决策树算法介绍

3.1 分类与决策树概述3.1。
1 分类与预测分类是一种应用非常广泛的数据挖掘技术,应用的例子也很多。
例如,根据信用卡支付历史记录,来判断具备哪些特征的用户往往具有良好的信用;根据某种病症的诊断记录,来分析哪些药物组合可以带来良好的治疗效果。
这些过程的一个共同特点是:根据数据的某些属性,来估计一个特定属性的值。
例如在信用分析案例中,根据用户的“年龄”、“性别”、“收入水平"、“职业”等属性的值,来估计该用户“信用度”属性的值应该取“好”还是“差",在这个例子中,所研究的属性“信用度"是一个离散属性,它的取值是一个类别值,这种问题在数据挖掘中被称为分类。
还有一种问题,例如根据股市交易的历史数据估计下一个交易日的大盘指数,这里所研究的属性“大盘指数”是一个连续属性,它的取值是一个实数。
那么这种问题在数据挖掘中被称为预测.总之,当估计的属性值是离散值时,这就是分类;当估计的属性值是连续值时,这就是预测.3。
1.2 决策树的基本原理1.构建决策树通过一个实际的例子,来了解一些与决策树有关的基本概念.表3—1是一个数据库表,记载着某银行的客户信用记录,属性包括“姓名”、“年龄”、“职业”、“月薪”、..。
..。
、“信用等级”,每一行是一个客户样本,每一列是一个属性(字段)。
这里把这个表记做数据集D.银行需要解决的问题是,根据数据集D,建立一个信用等级分析模型,并根据这个模型,产生一系列规则。
当银行在未来的某个时刻收到某个客户的贷款申请时,依据这些规则,可以根据该客户的年龄、职业、月薪等属性,来预测其信用等级,以确定是否提供贷款给该用户。
这里的信用等级分析模型,就可以是一棵决策树. 在这个案例中,研究的重点是“信用等级”这个属性。
给定一个信用等级未知的客户,要根据他/她的其他属性来估计“信用等级”的值是“优”、“良"还是“差”,也就是说,要把这客户划分到信用等级为“优”、“良"、“差"这3个类别的某一类别中去.这里把“信用等级”这个属性称为“类标号属性”。
使用决策树进行分类和回归的方法
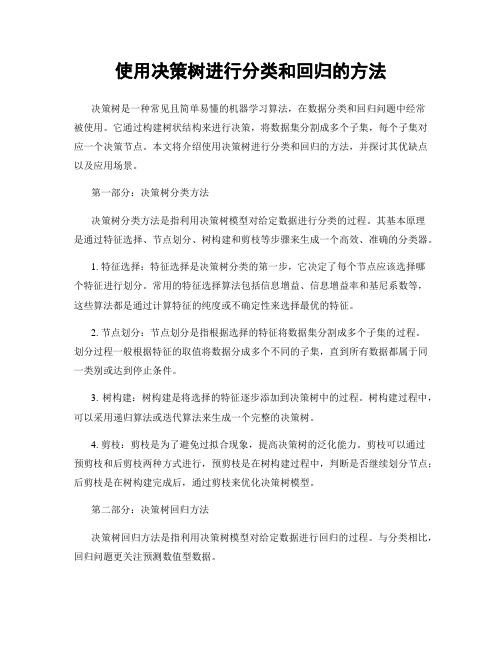
使用决策树进行分类和回归的方法决策树是一种常见且简单易懂的机器学习算法,在数据分类和回归问题中经常被使用。
它通过构建树状结构来进行决策,将数据集分割成多个子集,每个子集对应一个决策节点。
本文将介绍使用决策树进行分类和回归的方法,并探讨其优缺点以及应用场景。
第一部分:决策树分类方法决策树分类方法是指利用决策树模型对给定数据进行分类的过程。
其基本原理是通过特征选择、节点划分、树构建和剪枝等步骤来生成一个高效、准确的分类器。
1. 特征选择:特征选择是决策树分类的第一步,它决定了每个节点应该选择哪个特征进行划分。
常用的特征选择算法包括信息增益、信息增益率和基尼系数等,这些算法都是通过计算特征的纯度或不确定性来选择最优的特征。
2. 节点划分:节点划分是指根据选择的特征将数据集分割成多个子集的过程。
划分过程一般根据特征的取值将数据分成多个不同的子集,直到所有数据都属于同一类别或达到停止条件。
3. 树构建:树构建是将选择的特征逐步添加到决策树中的过程。
树构建过程中,可以采用递归算法或迭代算法来生成一个完整的决策树。
4. 剪枝:剪枝是为了避免过拟合现象,提高决策树的泛化能力。
剪枝可以通过预剪枝和后剪枝两种方式进行,预剪枝是在树构建过程中,判断是否继续划分节点;后剪枝是在树构建完成后,通过剪枝来优化决策树模型。
第二部分:决策树回归方法决策树回归方法是指利用决策树模型对给定数据进行回归的过程。
与分类相比,回归问题更关注预测数值型数据。
1. 划分依据:决策树回归方法中,每个节点的划分依据不再是纯度或不确定性的度量,而是基于平方误差、均方差或平均绝对误差等度量指标。
划分依据是为了找到能够使子集数据的目标值尽量接近的特征。
2. 节点划分和树构建:节点划分和树构建的过程与分类问题类似,不同之处在于目标值的处理。
在回归问题中,节点划分过程应根据目标值的大小将数据集划分成多个子集。
3. 预测值计算:决策树回归模型的预测值是通过对决策树进行遍历,找到与待预测样本最匹配的叶子节点,并返回该节点的目标值作为预测结果。
6. 决策树分类
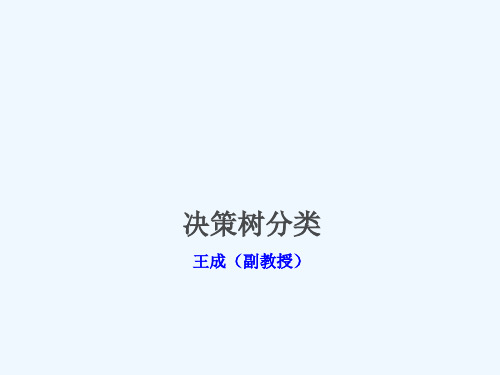
收入
学生 信用
买了电脑 收入=高的有4个, 其中2个为“否”
<30
高
否
一般 否
收入=中的有6个, 其中2个为“否”
<30
高
否
好
否
收入=低的有4个, 其中1个为“否”
30-40 高
否
一般 是
>40
中
否
一般 是
Info收入(D)
>40
低
是
一般 是
>40
低
是
好
否
30-40 低
是
好
是
<30
中
否
一般 否
<30
信息熵 (Entropy)
假如我错过了一个有32支球队参加的足球赛,赛后我问一 个知道比赛结果的观众“哪支球队是冠军”?他不愿意直 接告诉我,而让我猜,每猜一次,他要收一元钱才肯告诉 我是否猜对,那我需要付多少钱才能知道谁是冠军呢?
我可以把球队编号,从1到32,然后问“冠军球队在1-16 号中吗?”,假如他告诉我猜对了,我就接着问“冠军在 1-8号中吗?”,假如他说猜错了,那我就知道冠军在9-16 号中。这样只要5次,我就能知道哪支球队是冠军
决策树提供了一种展示在什么条件下会得到什么类别这类 规则的方法。
下例是为了解决这个问题而建立的一棵决策树,从中可以 看到决策树的基本组成部分:决策结点、分支和叶结点
决策树
下图给出了一个商业上使用的决策树的例子。它表示了一 个关心电子产品的用户是否会购买PC(buys_computer)的 知识,用它可以预测某条记录(某个人)的购买意向
是
一般 是
= Info(D) - Info收入(D)
决策树名词解释
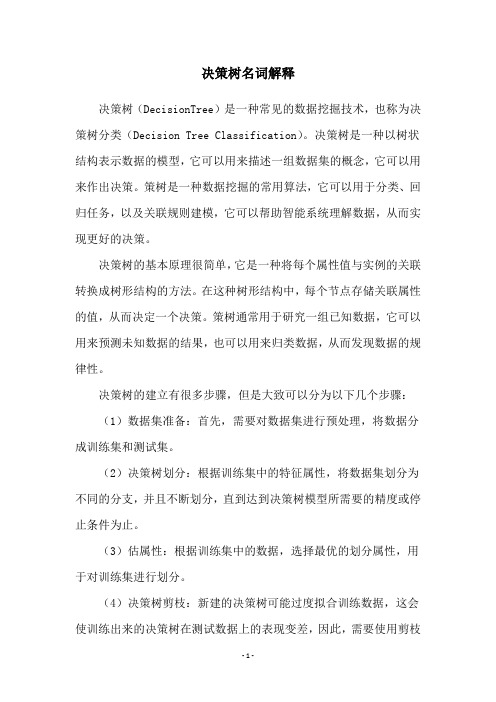
决策树名词解释决策树(DecisionTree)是一种常见的数据挖掘技术,也称为决策树分类(Decision Tree Classification)。
决策树是一种以树状结构表示数据的模型,它可以用来描述一组数据集的概念,它可以用来作出决策。
策树是一种数据挖掘的常用算法,它可以用于分类、回归任务,以及关联规则建模,它可以帮助智能系统理解数据,从而实现更好的决策。
决策树的基本原理很简单,它是一种将每个属性值与实例的关联转换成树形结构的方法。
在这种树形结构中,每个节点存储关联属性的值,从而决定一个决策。
策树通常用于研究一组已知数据,它可以用来预测未知数据的结果,也可以用来归类数据,从而发现数据的规律性。
决策树的建立有很多步骤,但是大致可以分为以下几个步骤:(1)数据集准备:首先,需要对数据集进行预处理,将数据分成训练集和测试集。
(2)决策树划分:根据训练集中的特征属性,将数据集划分为不同的分支,并且不断划分,直到达到决策树模型所需要的精度或停止条件为止。
(3)估属性:根据训练集中的数据,选择最优的划分属性,用于对训练集进行划分。
(4)决策树剪枝:新建的决策树可能过度拟合训练数据,这会使训练出来的决策树在测试数据上的表现变差,因此,需要使用剪枝算法,来减少决策树的过拟合现象。
(5)测试:根据训练好的决策树,对测试集数据进行分类,统计测试集分类正确率,从而对决策树进行评估。
决策树在实际应用中可以用于社会决策分析、企业决策分析、关联规则挖掘等应用场景,但是决策树也有若干缺点。
其一,决策树生成过程中属性之间的关系可能非线性,而决策树假设属性之间的关系是线性的,因此可能导致决策树模型的准确性不足。
其二,决策树的剪枝操作可能会过度剪枝,也影响模型的准确性。
总之,决策树是一种常用的数据挖掘技术,它可以用于推理和预测数据,它可以用来帮助智能系统理解数据,从而改善决策效率。
但是,因为决策树的局限性,仍然需要其他的数据挖掘技术来提高决策的准确性。
分类决策树的基本原理
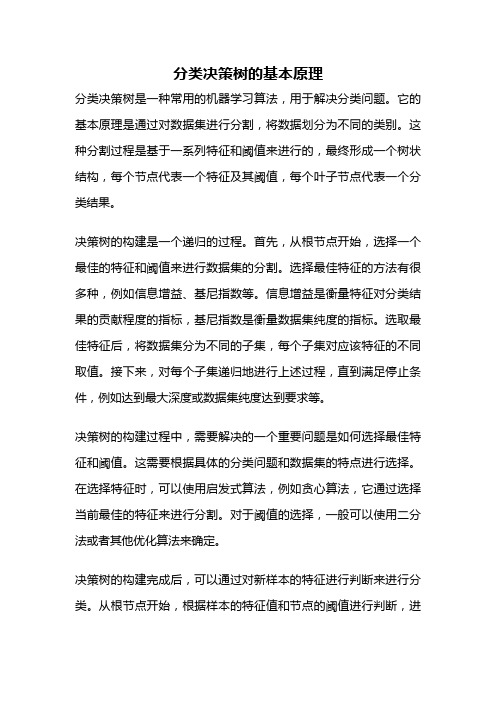
分类决策树的基本原理分类决策树是一种常用的机器学习算法,用于解决分类问题。
它的基本原理是通过对数据集进行分割,将数据划分为不同的类别。
这种分割过程是基于一系列特征和阈值来进行的,最终形成一个树状结构,每个节点代表一个特征及其阈值,每个叶子节点代表一个分类结果。
决策树的构建是一个递归的过程。
首先,从根节点开始,选择一个最佳的特征和阈值来进行数据集的分割。
选择最佳特征的方法有很多种,例如信息增益、基尼指数等。
信息增益是衡量特征对分类结果的贡献程度的指标,基尼指数是衡量数据集纯度的指标。
选取最佳特征后,将数据集分为不同的子集,每个子集对应该特征的不同取值。
接下来,对每个子集递归地进行上述过程,直到满足停止条件,例如达到最大深度或数据集纯度达到要求等。
决策树的构建过程中,需要解决的一个重要问题是如何选择最佳特征和阈值。
这需要根据具体的分类问题和数据集的特点进行选择。
在选择特征时,可以使用启发式算法,例如贪心算法,它通过选择当前最佳的特征来进行分割。
对于阈值的选择,一般可以使用二分法或者其他优化算法来确定。
决策树的构建完成后,可以通过对新样本的特征进行判断来进行分类。
从根节点开始,根据样本的特征值和节点的阈值进行判断,进而遍历树的节点,直到达到叶子节点,得到分类结果。
决策树具有很多优点。
首先,决策树易于理解和解释,可以生成直观的规则。
其次,决策树可以处理多类别问题,并且可以处理连续和离散的特征。
此外,决策树可以处理缺失数据,不需要对数据进行预处理。
最后,决策树算法的时间复杂度较低,适合处理大规模的数据集。
然而,决策树也存在一些缺点。
首先,决策树容易出现过拟合问题,特别是当树的深度较大时。
过拟合会导致决策树对训练样本的分类效果很好,但对未知样本的分类效果较差。
为了解决过拟合问题,可以使用剪枝等方法。
其次,决策树对于一些复杂的问题,可能无法找到最优解。
此外,决策树对于数据集中噪声和异常值较为敏感,容易受到干扰。
如何使用决策树算法进行分类
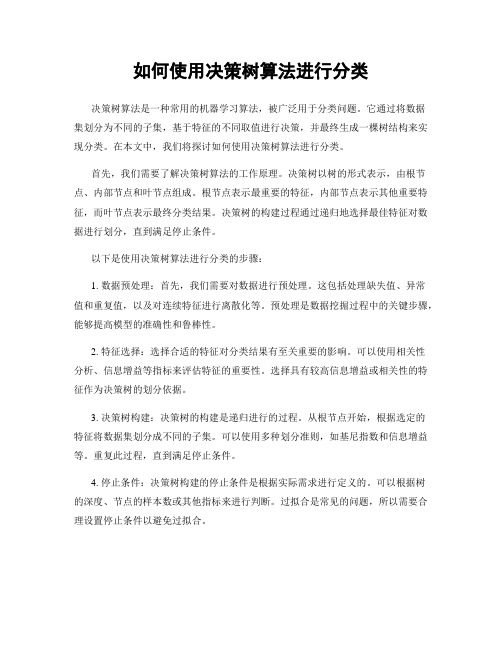
如何使用决策树算法进行分类决策树算法是一种常用的机器学习算法,被广泛用于分类问题。
它通过将数据集划分为不同的子集,基于特征的不同取值进行决策,并最终生成一棵树结构来实现分类。
在本文中,我们将探讨如何使用决策树算法进行分类。
首先,我们需要了解决策树算法的工作原理。
决策树以树的形式表示,由根节点、内部节点和叶节点组成。
根节点表示最重要的特征,内部节点表示其他重要特征,而叶节点表示最终分类结果。
决策树的构建过程通过递归地选择最佳特征对数据进行划分,直到满足停止条件。
以下是使用决策树算法进行分类的步骤:1. 数据预处理:首先,我们需要对数据进行预处理。
这包括处理缺失值、异常值和重复值,以及对连续特征进行离散化等。
预处理是数据挖掘过程中的关键步骤,能够提高模型的准确性和鲁棒性。
2. 特征选择:选择合适的特征对分类结果有至关重要的影响。
可以使用相关性分析、信息增益等指标来评估特征的重要性。
选择具有较高信息增益或相关性的特征作为决策树的划分依据。
3. 决策树构建:决策树的构建是递归进行的过程。
从根节点开始,根据选定的特征将数据集划分成不同的子集。
可以使用多种划分准则,如基尼指数和信息增益等。
重复此过程,直到满足停止条件。
4. 停止条件:决策树构建的停止条件是根据实际需求进行定义的。
可以根据树的深度、节点的样本数或其他指标来进行判断。
过拟合是常见的问题,所以需要合理设置停止条件以避免过拟合。
5. 决策树剪枝:决策树构建完成后,可能出现过拟合的情况。
剪枝是通过裁剪决策树的一些子树来减少过拟合。
剪枝可以通过预剪枝或后剪枝来实现。
预剪枝是在构建树的过程中进行剪枝,而后剪枝是在构建完成后再进行剪枝。
6. 分类预测:完成决策树的构建和剪枝后,我们可以使用分类预测来对新样本进行分类。
从根节点开始,根据特征的取值进行递归判断,直到达到叶节点。
叶节点的分类结果即为预测结果。
决策树算法的优点在于易于理解和解释,而且可以处理非线性关系。
决策树算法在客户分类中的应用

决策树算法在客户分类中的应用在当今信息时代,企业已经积累了大量的客户数据。
如何利用这些数据来更好地理解客户并进行分类成为了企业面临的一个重要挑战。
决策树算法作为一种常用的机器学习方法,被广泛应用于客户分类中。
本文将探讨决策树算法在客户分类中的应用,并讨论其优势和局限性。
一、决策树算法简介决策树算法是一种基于树形结构的预测模型,在分类问题中广泛应用。
决策树由根节点、内部节点和叶节点组成,根节点代表一个特征,内部节点代表特征的取值,叶节点代表分类结果。
通过对训练数据进行分割,决策树可以根据特征的取值预测目标变量的类别。
二、客户分类中的决策树算法应用1. 特征选择在客户分类中,我们需要选择一组合适的特征来构建决策树。
特征选择是决策树算法中的关键步骤。
常用的特征选择方法包括信息增益、信息增益比和基尼指数等。
通过计算不同特征的重要性,我们可以选择最相关的特征来构建决策树,从而实现客户分类。
2. 构建决策树模型一旦选择了特征,我们就可以开始构建决策树模型。
决策树算法通过不断地分割数据集来生成一个树形结构,从而实现对客户分类的预测。
决策树的分割过程基于特征的取值,使得每个子节点上的样本尽可能属于同一类别。
通过递归建立决策树的过程,我们可以得到一个完整的分类模型。
3. 模型评估在构建决策树模型之后,我们需要评估其性能。
常用的评估指标包括准确率、召回率、F1值等。
通过比较模型预测的结果与实际类别,我们可以评估模型在客户分类中的表现,并对其进行优化调整。
三、决策树算法在客户分类中的优势1. 简单直观决策树算法具有直观的特点,易于理解和解释。
通过决策树模型,我们可以清晰地看到分类的过程,了解每个节点的取值对分类结果的影响。
这使得决策树算法在客户分类中更具可解释性,能够帮助企业深入了解客户特征和需求。
2. 非参数化决策树算法是一种非参数化方法,对数据的分布没有要求。
这意味着我们可以直接使用原始数据进行分类,无需对数据进行任何预处理。
分类模型——决策树
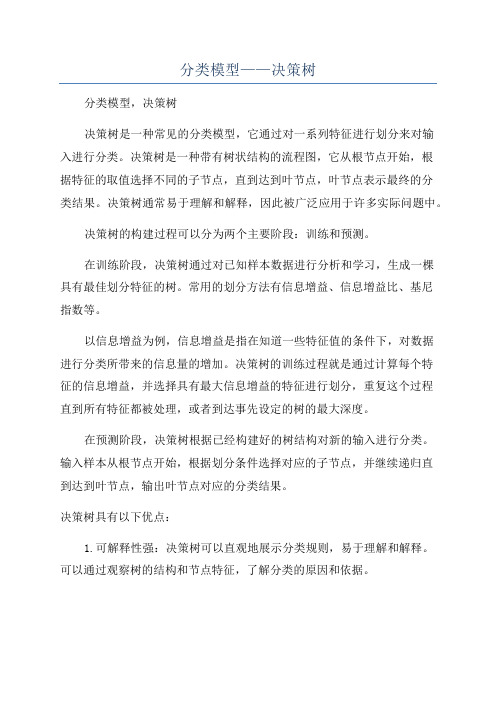
分类模型——决策树分类模型,决策树决策树是一种常见的分类模型,它通过对一系列特征进行划分来对输入进行分类。
决策树是一种带有树状结构的流程图,它从根节点开始,根据特征的取值选择不同的子节点,直到达到叶节点,叶节点表示最终的分类结果。
决策树通常易于理解和解释,因此被广泛应用于许多实际问题中。
决策树的构建过程可以分为两个主要阶段:训练和预测。
在训练阶段,决策树通过对已知样本数据进行分析和学习,生成一棵具有最佳划分特征的树。
常用的划分方法有信息增益、信息增益比、基尼指数等。
以信息增益为例,信息增益是指在知道一些特征值的条件下,对数据进行分类所带来的信息量的增加。
决策树的训练过程就是通过计算每个特征的信息增益,并选择具有最大信息增益的特征进行划分,重复这个过程直到所有特征都被处理,或者到达事先设定的树的最大深度。
在预测阶段,决策树根据已经构建好的树结构对新的输入进行分类。
输入样本从根节点开始,根据划分条件选择对应的子节点,并继续递归直到达到叶节点,输出叶节点对应的分类结果。
决策树具有以下优点:1.可解释性强:决策树可以直观地展示分类规则,易于理解和解释。
可以通过观察树的结构和节点特征,了解分类的原因和依据。
2.适用性广泛:决策树可以处理多类别问题,并且对于连续特征和离散特征都可以进行分类。
同时,决策树也可以用于处理缺失值、处理不平衡数据等问题。
3.计算效率高:决策树的训练和预测过程都可以在较短的时间内完成。
决策树的训练过程由于每次选择一个最优特征进行划分,因此可以减少特征的空间。
然而,决策树也存在一些缺点:1.容易过拟合:决策树容易生成过于复杂的模型,导致过拟合的问题。
过拟合会导致在训练集上表现很好,但在测试集上表现较差。
2.对噪声敏感:决策树对于噪声和异常值比较敏感。
当训练数据中包含大量噪声时,决策树可能会产生错误的分类结果。
3.不稳定性:当输入数据稍有变化时,决策树的结构可能会发生较大的变化,导致预测结果不稳定。
分类模型归纳总结

分类模型归纳总结在机器学习和数据挖掘领域,分类是一种常见的任务,它旨在根据给定的特征将数据点分为不同的类别。
分类模型是用于解决分类问题的数学模型。
本文将对一些常见的分类模型进行归纳总结,包括逻辑回归、决策树、支持向量机和随机森林等。
一、逻辑回归(Logistic Regression)逻辑回归是一种广泛应用于分类问题的线性模型。
它通过将输入特征与权重相乘,并通过一个激活函数(如sigmoid函数)将结果映射到[0, 1]的范围内,从而预测样本属于某个类别的概率。
逻辑回归具有简单、高效的特点,适用于二分类问题。
二、决策树(Decision Tree)决策树是一种基于树结构的分类模型。
它通过将特征空间划分为多个矩形区域,每个区域对应一个类别,从而实现对样本进行分类。
决策树具有易解释、易理解的特点,可处理离散和连续特征,并且具备较好的鲁棒性。
三、支持向量机(Support Vector Machine)支持向量机是一种经典的分类模型,通过在特征空间中构造最优超平面,将不同类别的样本分开。
支持向量机可处理线性可分和线性不可分的问题,在高维空间中表现出色,并具有一定的抗噪能力。
四、随机森林(Random Forest)随机森林是一种集成学习方法,由多个决策树组成。
它通过对训练集随机采样,并对每个采样子集构建一个决策树,最终通过投票或平均等方式得到分类结果。
随机森林具有较高的准确性和较好的泛化能力,对于处理高维数据和大规模数据集具有一定优势。
五、朴素贝叶斯分类器(Naive Bayes Classifier)朴素贝叶斯分类器是一种基于贝叶斯定理的概率分类模型。
它假设各个特征之间相互独立,并根据训练数据计算类别的先验概率和特征的条件概率,从而进行分类预测。
朴素贝叶斯分类器简单、高效,并在处理文本分类等领域表现突出。
六、神经网络(Neural Networks)神经网络是一类模拟人脑结构和功能的机器学习模型。
它包含输入层、隐藏层和输出层,通过不同层之间的连接权重进行信息传递和特征提取,最终实现分类任务。
分类决策树decisiontreeclassifier详解介绍
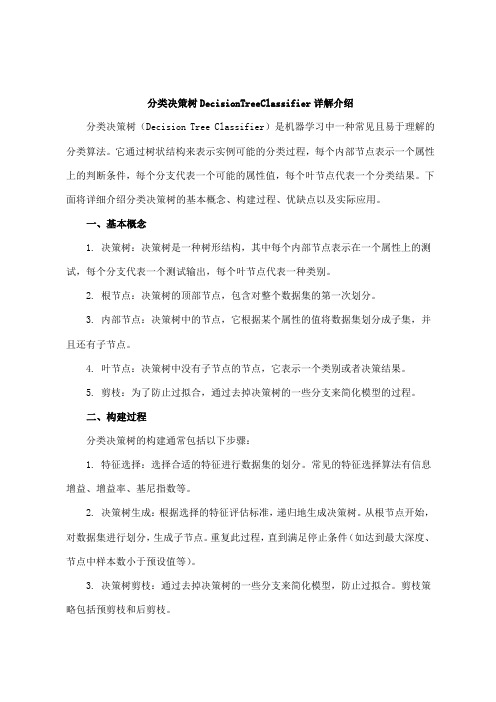
分类决策树DecisionTreeClassifier详解介绍分类决策树(Decision Tree Classifier)是机器学习中一种常见且易于理解的分类算法。
它通过树状结构来表示实例可能的分类过程,每个内部节点表示一个属性上的判断条件,每个分支代表一个可能的属性值,每个叶节点代表一个分类结果。
下面将详细介绍分类决策树的基本概念、构建过程、优缺点以及实际应用。
一、基本概念1. 决策树:决策树是一种树形结构,其中每个内部节点表示在一个属性上的测试,每个分支代表一个测试输出,每个叶节点代表一种类别。
2. 根节点:决策树的顶部节点,包含对整个数据集的第一次划分。
3. 内部节点:决策树中的节点,它根据某个属性的值将数据集划分成子集,并且还有子节点。
4. 叶节点:决策树中没有子节点的节点,它表示一个类别或者决策结果。
5. 剪枝:为了防止过拟合,通过去掉决策树的一些分支来简化模型的过程。
二、构建过程分类决策树的构建通常包括以下步骤:1. 特征选择:选择合适的特征进行数据集的划分。
常见的特征选择算法有信息增益、增益率、基尼指数等。
2. 决策树生成:根据选择的特征评估标准,递归地生成决策树。
从根节点开始,对数据集进行划分,生成子节点。
重复此过程,直到满足停止条件(如达到最大深度、节点中样本数小于预设值等)。
3. 决策树剪枝:通过去掉决策树的一些分支来简化模型,防止过拟合。
剪枝策略包括预剪枝和后剪枝。
三、优缺点1. 优点:- 易于理解和解释:决策树的结构直观,易于理解和解释,适合非专业人士使用。
- 对数据预处理要求较低:决策树算法能够处理数值型和离散型数据,不需要过多的数据预处理。
- 能够处理非线性关系:决策树能够自动捕捉特征与目标变量之间的非线性关系。
- 对异常值和缺失值不敏感:决策树算法在一定程度上对异常值和缺失值具有鲁棒性。
2. 缺点:- 容易过拟合:决策树在生成过程中可能会过于复杂,导致过拟合现象。
决策树(CART算法)针对中文文本分类
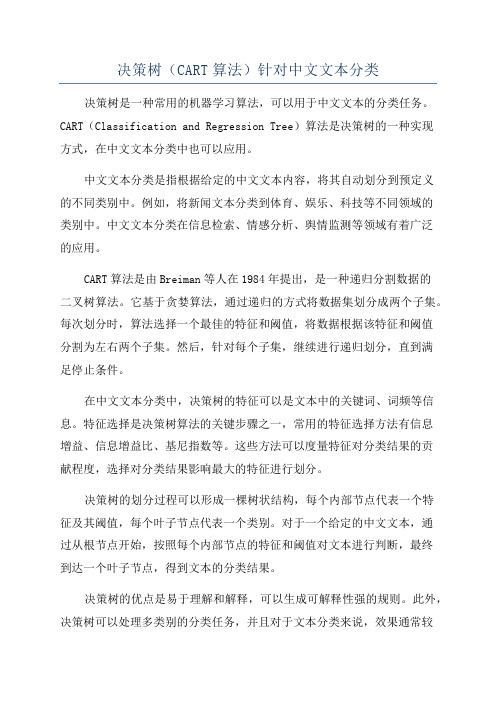
决策树(CART算法)针对中文文本分类决策树是一种常用的机器学习算法,可以用于中文文本的分类任务。
CART(Classification and Regression Tree)算法是决策树的一种实现方式,在中文文本分类中也可以应用。
中文文本分类是指根据给定的中文文本内容,将其自动划分到预定义的不同类别中。
例如,将新闻文本分类到体育、娱乐、科技等不同领域的类别中。
中文文本分类在信息检索、情感分析、舆情监测等领域有着广泛的应用。
CART算法是由Breiman等人在1984年提出,是一种递归分割数据的二叉树算法。
它基于贪婪算法,通过递归的方式将数据集划分成两个子集。
每次划分时,算法选择一个最佳的特征和阈值,将数据根据该特征和阈值分割为左右两个子集。
然后,针对每个子集,继续进行递归划分,直到满足停止条件。
在中文文本分类中,决策树的特征可以是文本中的关键词、词频等信息。
特征选择是决策树算法的关键步骤之一,常用的特征选择方法有信息增益、信息增益比、基尼指数等。
这些方法可以度量特征对分类结果的贡献程度,选择对分类结果影响最大的特征进行划分。
决策树的划分过程可以形成一棵树状结构,每个内部节点代表一个特征及其阈值,每个叶子节点代表一个类别。
对于一个给定的中文文本,通过从根节点开始,按照每个内部节点的特征和阈值对文本进行判断,最终到达一个叶子节点,得到文本的分类结果。
决策树的优点是易于理解和解释,可以生成可解释性强的规则。
此外,决策树可以处理多类别的分类任务,并且对于文本分类来说,效果通常较好。
然而,决策树也存在一些限制,如容易过拟合和对输入数据分布敏感等问题。
因此,在应用决策树进行中文文本分类时,需要注意适当的预处理和参数设置,以避免这些问题。
总而言之,CART算法是决策树分类的一种常用实现方式,在中文文本分类中有着广泛的应用。
通过选择合适的特征和阈值,决策树可以将中文文本自动划分到不同的类别中。
虽然决策树在处理中文文本分类问题上具有优势,但仍需结合实际应用需求和数据特点来进行合理选择和调整。
数学建模决策树分类模型
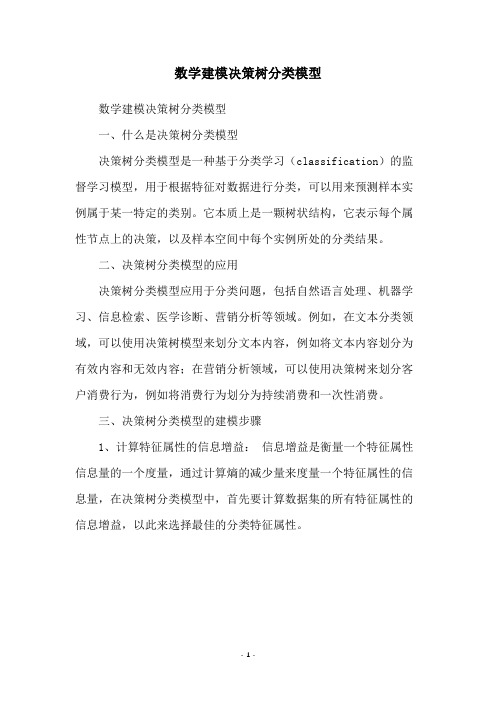
数学建模决策树分类模型
数学建模决策树分类模型
一、什么是决策树分类模型
决策树分类模型是一种基于分类学习(classification)的监督学习模型,用于根据特征对数据进行分类,可以用来预测样本实例属于某一特定的类别。
它本质上是一颗树状结构,它表示每个属性节点上的决策,以及样本空间中每个实例所处的分类结果。
二、决策树分类模型的应用
决策树分类模型应用于分类问题,包括自然语言处理、机器学习、信息检索、医学诊断、营销分析等领域。
例如,在文本分类领域,可以使用决策树模型来划分文本内容,例如将文本内容划分为有效内容和无效内容;在营销分析领域,可以使用决策树来划分客户消费行为,例如将消费行为划分为持续消费和一次性消费。
三、决策树分类模型的建模步骤
1、计算特征属性的信息增益:信息增益是衡量一个特征属性信息量的一个度量,通过计算熵的减少量来度量一个特征属性的信息量,在决策树分类模型中,首先要计算数据集的所有特征属性的信息增益,以此来选择最佳的分类特征属性。
- 1 -。
决策树分类方法
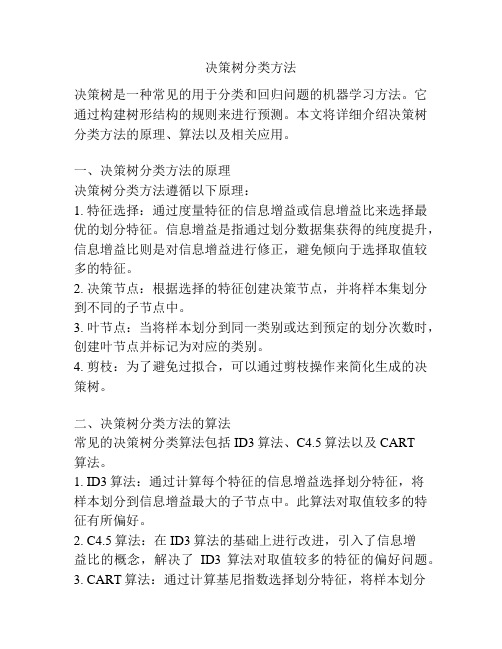
决策树分类方法决策树是一种常见的用于分类和回归问题的机器学习方法。
它通过构建树形结构的规则来进行预测。
本文将详细介绍决策树分类方法的原理、算法以及相关应用。
一、决策树分类方法的原理决策树分类方法遵循以下原理:1. 特征选择:通过度量特征的信息增益或信息增益比来选择最优的划分特征。
信息增益是指通过划分数据集获得的纯度提升,信息增益比则是对信息增益进行修正,避免倾向于选择取值较多的特征。
2. 决策节点:根据选择的特征创建决策节点,并将样本集划分到不同的子节点中。
3. 叶节点:当将样本划分到同一类别或达到预定的划分次数时,创建叶节点并标记为对应的类别。
4. 剪枝:为了避免过拟合,可以通过剪枝操作来简化生成的决策树。
二、决策树分类方法的算法常见的决策树分类算法包括ID3算法、C4.5算法以及CART算法。
1. ID3算法:通过计算每个特征的信息增益选择划分特征,将样本划分到信息增益最大的子节点中。
此算法对取值较多的特征有所偏好。
2. C4.5算法:在ID3算法的基础上进行改进,引入了信息增益比的概念,解决了ID3算法对取值较多的特征的偏好问题。
3. CART算法:通过计算基尼指数选择划分特征,将样本划分到基尼指数最小的子节点中。
此算法适用于分类和回归问题。
三、决策树分类方法的应用决策树分类方法广泛应用于各个领域,以下是几个常见的应用场景:1. 信用评估:通过构建决策树模型,根据客户的个人信息和历史数据预测其信用等级,用于信贷风险评估和贷款审批。
2. 疾病诊断:通过决策树模型,根据患者的病症和医学检测结果预测其患有何种疾病,用于辅助医生的诊断决策。
3. 电商推荐:通过决策树模型,根据用户的历史购买记录和个人喜好预测其对某些商品的偏好程度,从而进行个性化商品推荐。
4. 欺诈检测:通过构建决策树模型,根据用户的账户行为和交易记录预测其是否存在欺诈行为,用于金融等领域的欺诈检测。
四、决策树分类方法的优缺点决策树分类方法具有以下优点:1. 易于理解和解释:决策树模型的结果具有很好的可解释性,可以通过树形结构直观地看出预测结果的原因。
机器学习 第3章 决策树与分类算法
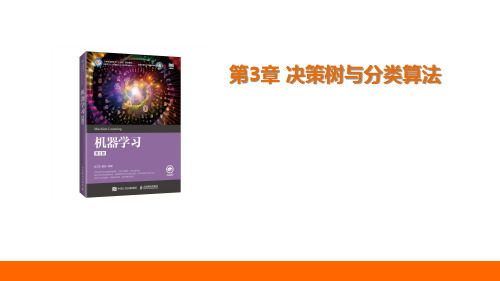
议程
CART算法
议程
CART算法示例
以下是基于sklearn库的CART算法示例代码。通过构建决策树(采用Gini指标)对随机生成(通过np.random.randint方法)的数字进行分类,自变量X为100x4的矩阵,随机生成的数字大于10,因变量Y为大于2的100x1矩阵。树的最大深度限制为3层,训练完成之后将树可视化显示。
议程
C4.5算法
议程
C5.0算法
C5.0算法是Quinlan在C4.5算法的基础上提出的商用改进版本,目的是对含有大量数据的数据集进行分析 C5.0算法与C4.5算法相比有以下优势: 决策树构建时间要比C4.5算法快上数倍,同时生成的决策树规模也更小,拥有更少的叶子结点数 使用了提升法(boosting),组合多个决策树来做出分类,使准确率大大提高 提供可选项由使用者视情况决定,例如是否考虑样本的权重、样本错误分类成本等
议程
ID3 算法
脊椎动物训练样本集以“饮食习性”作为分支属性的分裂情况 “饮食习性”为“肉食动物”的分支中有3个正例、5个反例,其熵值为:
议程
ID3 算法
同理,计算出“饮食习性”分类为“草食动物”的分支与分类为“杂食动物”的分支中的熵值分别为 设“饮食习性”属性为Y,由此可以计算得出,作为分支属性进行分裂之后的信息增益为
import numpy as np import random from sklearn import tree from graphviz import Source np.random.seed(42) X=np.random.randint(10, size=(100, 4)) Y=np.random.randint(2, size=100) a=np.column_stack((Y,X)) clf = tree.DecisionTreeClassifier(criterion='gini',max_depth=3) clf = clf.fit(X, Y) graph = Source(tree.export_graphviz(clf, out_file=None)) graph.format = 'png' graph.render('cart_tree',view=True)
使用决策树算法进行多分类的步骤方法
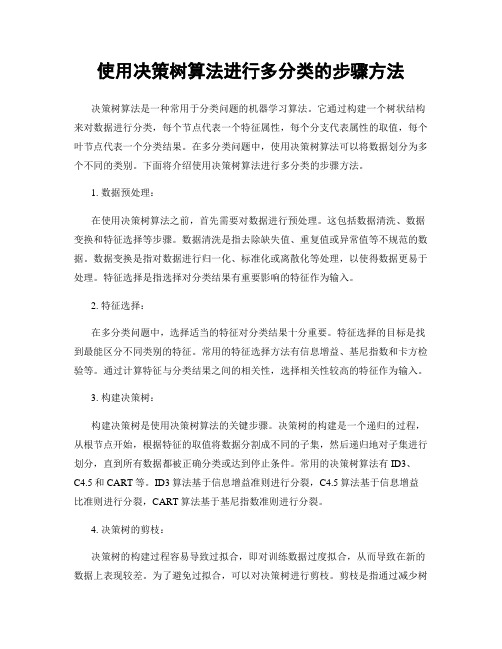
使用决策树算法进行多分类的步骤方法决策树算法是一种常用于分类问题的机器学习算法。
它通过构建一个树状结构来对数据进行分类,每个节点代表一个特征属性,每个分支代表属性的取值,每个叶节点代表一个分类结果。
在多分类问题中,使用决策树算法可以将数据划分为多个不同的类别。
下面将介绍使用决策树算法进行多分类的步骤方法。
1. 数据预处理:在使用决策树算法之前,首先需要对数据进行预处理。
这包括数据清洗、数据变换和特征选择等步骤。
数据清洗是指去除缺失值、重复值或异常值等不规范的数据。
数据变换是指对数据进行归一化、标准化或离散化等处理,以使得数据更易于处理。
特征选择是指选择对分类结果有重要影响的特征作为输入。
2. 特征选择:在多分类问题中,选择适当的特征对分类结果十分重要。
特征选择的目标是找到最能区分不同类别的特征。
常用的特征选择方法有信息增益、基尼指数和卡方检验等。
通过计算特征与分类结果之间的相关性,选择相关性较高的特征作为输入。
3. 构建决策树:构建决策树是使用决策树算法的关键步骤。
决策树的构建是一个递归的过程,从根节点开始,根据特征的取值将数据分割成不同的子集,然后递归地对子集进行划分,直到所有数据都被正确分类或达到停止条件。
常用的决策树算法有ID3、C4.5和CART等。
ID3算法基于信息增益准则进行分裂,C4.5算法基于信息增益比准则进行分裂,CART算法基于基尼指数准则进行分裂。
4. 决策树的剪枝:决策树的构建过程容易导致过拟合,即对训练数据过度拟合,从而导致在新的数据上表现较差。
为了避免过拟合,可以对决策树进行剪枝。
剪枝是指通过减少树的深度或节点数来降低模型复杂度。
常用的剪枝方法有预剪枝和后剪枝。
预剪枝是指在构建决策树的过程中,根据一定的准则提前停止划分,而后剪枝是指先构建完整的决策树,然后通过减少节点来降低模型复杂度。
5. 模型评估:在构建完决策树后,需要对模型进行评估,以了解其分类性能。
常用的评估指标有准确率、精确率、召回率和F1值等。
如何使用决策树算法进行分类
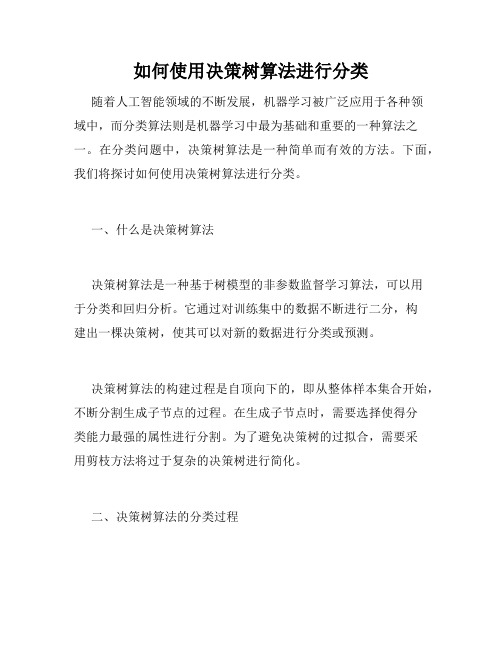
如何使用决策树算法进行分类随着人工智能领域的不断发展,机器学习被广泛应用于各种领域中,而分类算法则是机器学习中最为基础和重要的一种算法之一。
在分类问题中,决策树算法是一种简单而有效的方法。
下面,我们将探讨如何使用决策树算法进行分类。
一、什么是决策树算法决策树算法是一种基于树模型的非参数监督学习算法,可以用于分类和回归分析。
它通过对训练集中的数据不断进行二分,构建出一棵决策树,使其可以对新的数据进行分类或预测。
决策树算法的构建过程是自顶向下的,即从整体样本集合开始,不断分割生成子节点的过程。
在生成子节点时,需要选择使得分类能力最强的属性进行分割。
为了避免决策树的过拟合,需要采用剪枝方法将过于复杂的决策树进行简化。
二、决策树算法的分类过程决策树算法的分类过程可以分为两个步骤:决策树的构建和分类预测。
1. 决策树的构建在构建决策树时,需要使用训练数据进行学习,并选择最优特征进行节点的划分。
构建过程中,需要注意以下几点:(1)特征选择:决策树的好坏主要取决于属性的选择。
基于信息增益或基尼指数来进行属性选择都是常用的方式。
(2)节点划分:选择了最优特征后,需要将数据集按照该特征的属性值进行划分。
(3)树的生长:重复以上步骤,直到每个叶子节点都是同一类别的样本,或者无法继续进行特征选择为止。
2. 分类预测在构建好决策树后,就可以使用它进行分类预测了。
分类预测的过程是从根节点开始,按照特征进行判断,最终到达某个叶子节点,该叶子节点上的类别即为预测类别。
三、决策树算法的优缺点决策树算法具有以下优点:(1)易于理解和解释:决策树算法生成的决策树可以很清晰地展现出数据的分类情况,方便理解和解释。
(2)处理分类和连续性变量:决策树算法可以处理包括分类和连续性变量在内的各种类型的数据。
(3)高效:在分类预测时,决策树算法的复杂度是O(log2n),效率较高。
然而决策树算法也存在一些缺点:(1)容易过拟合:在样本数量较少或者属性数量较多的情况下,容易出现过拟合现象。
- 1、下载文档前请自行甄别文档内容的完整性,平台不提供额外的编辑、内容补充、找答案等附加服务。
- 2、"仅部分预览"的文档,不可在线预览部分如存在完整性等问题,可反馈申请退款(可完整预览的文档不适用该条件!)。
- 3、如文档侵犯您的权益,请联系客服反馈,我们会尽快为您处理(人工客服工作时间:9:00-18:30)。
ID 1 2 3 4 5 6 7 8 9 10 11 12 13 14 15
Age Young Young Young Young Young Middle Middle Middle Middle Middle Old Old Old Old Old
Has_job No No Yes Yes No No No Yes No No No No Yes Yes No
No
No Yes Yes No No No Yes Yes
10
11 12 13
Middle
Old Old Old
No
No No Yes
Yes
Yes Yes No
Excellent
Excellent Good Good
Yes
Yes Yes Yes
14
15
Old
Old
Yes
No
No
No
Excellent
Age
Has_job
Own_home
Credit
Class
1
2 3 4 5 6 7 8 9
Young
Young Young Young Young Middle Middle Middle Middle
No
No Yes Yes No No No Yes No
No
No No Yes Nood Good Fair Fair Fair Good Good Excellent
No
No Yes Yes No No No Yes Yes
10
11 12 13
Middle
Old Old Old
No
No No Yes
Yes
Yes Yes No
Excellent
Excellent Good Good
Fair
Good Good Fair Fair Fair Good Good Excellent
No
No Yes Yes No No No Yes Yes
10
11 12 13
Middle
Old Old Old
No
No No Yes
Yes
Yes Yes No
Excellent
Excellent Good Good
银 行 贷 款 数 据 集
ID
Age
Has_job
Own_home
Credit
Class
1
2 3 4 5 6 7 8 9
Young
Young Young Young Young Middle Middle Middle Middle
No
No Yes Yes No No No Yes No
No
No No Yes No No No Yes Yes
分类与预测
Vicky
银行个人住房贷款审批
银行个人客户提出住房贷款申请,根据历史 数据发现:部分贷款客户不能按时还款。为尽量 降低这种现象,需要发现不能按时还款客户的特 征,以便对以后住房贷款申请的审批提供依据。 2006年年底,由SAS机构与招商银行启动了全 行个人住房贷款评分卡开发与推广项目。 该项目利用客户的历史数据构建评分卡模型, 然后将该模型应用到新客户上,最后决定是否接 受新客户的贷款申请。
Yes
Yes Yes Yes
14
15
Old
Old
Yes
No
No
No
Excellent
Fair
Yes
No
银行贷款案例数据集的基尼指数: gini=1-(6/15)2-(9/15)2=0.48
分类误差(classification error)
CE最大=? 1-1/2 CE最小=? 0
(目标变量为二元变量)
Excellent Excellent Excellent Good Good Excellent Fair
分类与预测
• 分类:
– 目标变量为非数值型
• 预测:
– 目标变量为数值型
建模 模型评估
• 根据历史数据集(已知目标变 量),构建模型描述目标变量 与输入变量之间的关系,并依 据模型来分类或预测新数据( 目标变量值未知)。 分类模型也称为分类器。
裂分后数据集的熵EntropyAge(T)= 5/15* Entropy(T1)+ 5/15* Entropy(T2)+ 5/15* Entropy(T3) = 5/15*(− 3/5*log2(3/5) − 2/5*log2(2/5) )+ 5/15*(− 3/5*log2(3/5) − 2/5*log2(2/5) )+ 5/15*(− 1/5*log2(1/5) − 4/5*log2(4/5) )=0.888 信息增益Gain(age)=0.971-0.888=0.083
信息增益
Information Gain = 裂分前数据集的熵 – 裂分后各子数据集的熵 加权和 其中:权重为每个子集中的观测数在裂分前总 观测数中所占的比例
案例数据集基于own_home属性划分
ID 1 2 3 4 5 6 7 8 9 Age Young Young Young Young Young Middle Middle Middle Middle Has_job No No Yes Yes No No No Yes No Own_home No No No Yes No No No Yes Yes Credit Fair Good Good Fair Fair Fair Good Good Excellent Class No No Yes Yes No No No Yes Yes
纯度与混杂度
混杂度的常用测度指标
• 信息熵 ( Entropy) • 基尼指数( Gini Index) • 分类误差(classification error)
信息熵 ( Entropy)
Pj 是数据集合中类别j的相对比例. entropy = pi log2 pi
i
什么情况下,熵最小? 什么情况下,熵最大?
分类方法
•决策树方法 •贝叶斯分类法 •LOGISTIC回归 •神经网络方法 •K近邻分类法 •SVM分类法 ……….
决策树(decision tree)
Root
Node
Leaf 规则1:If refund=no and (marst=single or marst=divorced) and taxincome>80k then cheat=yes ……
Yes
Yes Yes Yes
14
15
Old
Old
Yes
No
No
No
Excellent
Fair
Yes
No
银行贷款案例数据集的分类误差: CE=1- 9/15=6/15=0.4
二、建立决策树
常用算法 二叉或多叉 信息熵 • ID3-ID5,C4,C4.5,C5.0 • CART(Classification and Regression Trees分 类与回归树) (C&RT) 二叉 GINI 指数 • CHAID(chi-squared automatic interaction detection,卡方自动交互检测)
Yes:6 No Yes:3
No:0
No:6
裂分前数据集的熵:Entropy(T0)=−6/15*log2(6/15) − 9/15*log2(9/15)=0.971
划分后数据集的熵EntropyOwn_home(T)= 6/15* Entropy(T1)+ 9/15* Entropy(T2) = 6/15*(− 6/6*log2(6/6) − 0/0*log2(0/6) )+ 9/15*(− 3/9*log2(3/9) − 6/9*log2(6/9) =0.551 信息增益Gain(ownhome)=0.971-0.551=0.42
has_job
Yes:6
信息增益分别为: No:0 Yes Gain(hasjob)=0.324 Gain(credit)=0.363 Yes:5 Gain(ownhome)=0.42 No:0 Gain(age)=0.971-0.888=0.083
案例数据集基于age属性划分
ID 1 2 3 4 5 6 7 8 9 Age Young Young Young Young Young Middle Middle Middle Middle Has_job No No Yes Yes No No No Yes No Own_home No No No Yes No No No Yes Yes Credit Fair Good Good Fair Fair Fair Good Good Excellent Class No No Yes Yes No No No Yes Yes
银行贷款申请 No Fair
No No Yes No No No Yes Yes Yes Yes Yes No No No Good Good Fair Fair Fair Good Good
Own_home
Credit
Class No No Yes Yes No No No Yes Yes Yes Yes Yes Yes Yes No
规则1:If refund=no and marst=married then cheat=no 模 …… 型 应 用
分类的过程
• 数据集分区
– 训练集:建立模型 – 验证集:调整和选择模型 – 测试集:评估模型的预测能力
• 建立模型 • 评估并选择模型 • 运用模型 新数据(打分集)
思考:分类模型在什么情况下不适合用于新数据?