新技术介绍优缺点.
环保新技术:比较太阳能与氢能
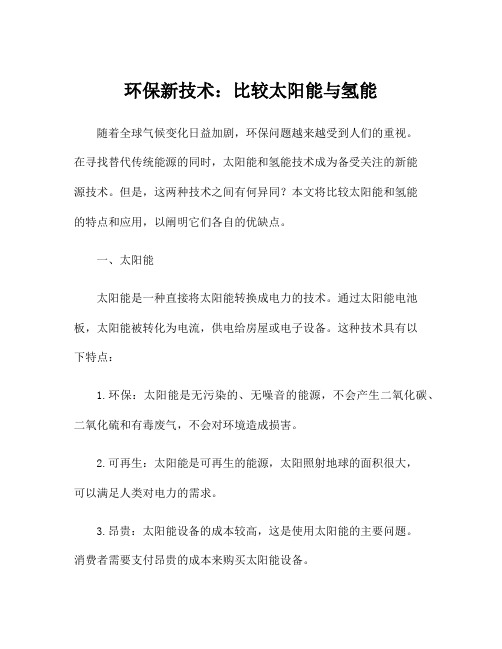
环保新技术:比较太阳能与氢能随着全球气候变化日益加剧,环保问题越来越受到人们的重视。
在寻找替代传统能源的同时,太阳能和氢能技术成为备受关注的新能源技术。
但是,这两种技术之间有何异同?本文将比较太阳能和氢能的特点和应用,以阐明它们各自的优缺点。
一、太阳能太阳能是一种直接将太阳能转换成电力的技术。
通过太阳能电池板,太阳能被转化为电流,供电给房屋或电子设备。
这种技术具有以下特点:1.环保:太阳能是无污染的、无噪音的能源,不会产生二氧化碳、二氧化硫和有毒废气,不会对环境造成损害。
2.可再生:太阳能是可再生的能源,太阳照射地球的面积很大,可以满足人类对电力的需求。
3.昂贵:太阳能设备的成本较高,这是使用太阳能的主要问题。
消费者需要支付昂贵的成本来购买太阳能设备。
4.取决于天气:太阳能的使用取决于天气条件。
在天气阴沉的地方效率较低,且在晚上和阴雨天等条件下,太阳能无法发电。
二、氢能氢能是一种通过将氢气和氧气反应后来产生能量的技术。
通过氢燃料电池,氢气和氧气反应,释放出电子,生成电力。
这种技术具有以下特点:1.环保:在氢燃烧过程中,唯一的废物是水蒸气,不会对环境造成任何污染。
2.可再生:氢气是可再生能源,它可以通过太阳能、风能和水力发电厂等设施来制造。
3.昂贵:从制造氢气燃料电池到建造氢燃料站,氢燃料的成本非常高,并且建设氢基础设施需要大量的资金投入。
4.安全隐患:尽管氢气在燃烧时不会产生有害物质,但氢气是一种易燃的气体,一旦泄漏,很容易引发爆炸。
三、比较太阳能和氢能都是新能源技术,尽管它们各有优势和局限,但也存在一些相似之处。
1.环保:两种能源技术均为环保的能源,不会对环境造成任何污染和破坏。
2.可再生:太阳能和氢气都是可再生的能源,是未来能源发展的方向。
3.需求:两种能源技术都需要大量的投资和研发来降低成本和提高效率,从而让更多的人接受并应用它们。
然而,太阳能和氢能也存在一些差异。
首先,太阳能是一种更成熟且可靠的技术,而氢燃料电池仍处于发展阶段。
自主研发和引进技术的优缺点、效益等方面的比较分析
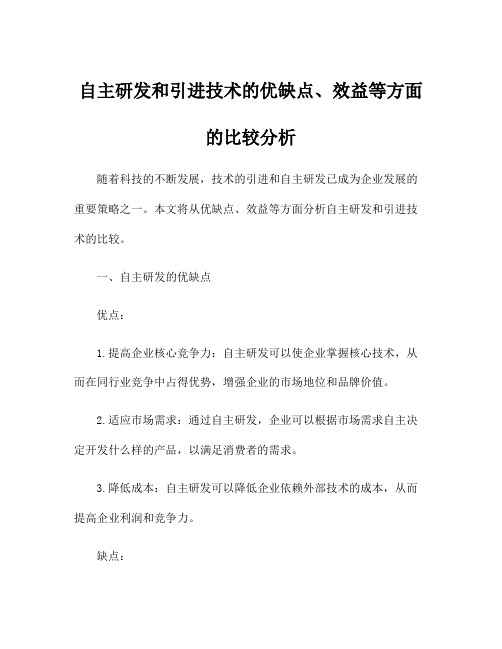
自主研发和引进技术的优缺点、效益等方面的比较分析随着科技的不断发展,技术的引进和自主研发已成为企业发展的重要策略之一。
本文将从优缺点、效益等方面分析自主研发和引进技术的比较。
一、自主研发的优缺点优点:1.提高企业核心竞争力:自主研发可以使企业掌握核心技术,从而在同行业竞争中占得优势,增强企业的市场地位和品牌价值。
2.适应市场需求:通过自主研发,企业可以根据市场需求自主决定开发什么样的产品,以满足消费者的需求。
3.降低成本:自主研发可以降低企业依赖外部技术的成本,从而提高企业利润和竞争力。
缺点:1.时间周期较长:科研试验需要耗费大量时间,尤其是在高科技领域,需要投入大量资金和人力,而且研发成功的可能性也较低。
2.高风险:新技术的开发和掌握需要十分复杂的过程,成功的机率不高。
尤其是在研发后可能会出现竞争对手已经赶超了之前技术水平的情况下,该项研发的意义就被打了折扣。
3.必须有强大的科研实力:需要大量专业人才、研究设备和技术,企业必须拥有雄厚的自主研发力量,否则研发费用就会成为白费。
二、引进技术的优缺点优点:1.节省时间:引进技术可以快速实现企业技术水平的提升,进而更好地适应市场需求,提升企业的核心竞争力。
2.降低风险:引进先进的技术已经经过市场检验,有一定的市场保障,成功率较高,不会像自主研发那样存在很大的风险。
3.节约成本:与自主研发相比,引进的技术已经经过市场考验,企业可以通过购买成熟的技术产品以降低研发成本。
而且,购买现成的技术也可以避免运营成本和资金的浪费。
缺点:1.可能造成市场竞争压力:随着市场不断扩大,市场不断提高对技术产品的要求,如果企业不能在市场竞争中保持优势,就可能被其他更先进的技术所替代。
2.技术依赖性较强:企业过于依赖引进技术,也会面临技术停滞甚至是滞后的风险,从而成为同行业竞争的弱势企业。
3.可能会存在技术壁垒:一些国际公司进行技术转让时,会设置技术壁垒,让企业在技术上受到限制,这会阻碍企业在自主研发方面的发展。
营销推广中的新技术和新趋势
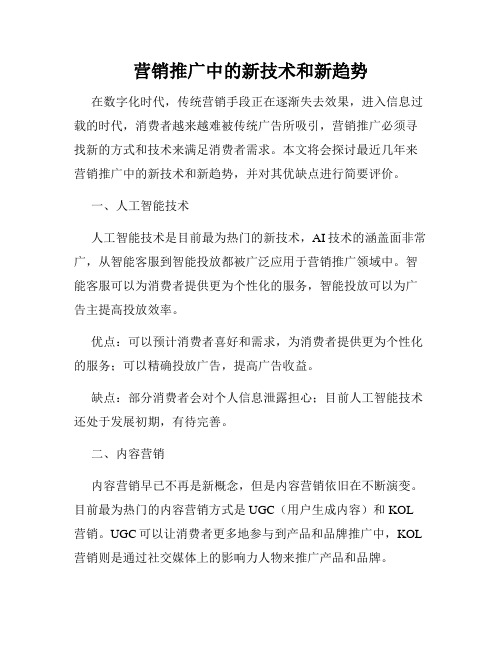
营销推广中的新技术和新趋势在数字化时代,传统营销手段正在逐渐失去效果,进入信息过载的时代,消费者越来越难被传统广告所吸引,营销推广必须寻找新的方式和技术来满足消费者需求。
本文将会探讨最近几年来营销推广中的新技术和新趋势,并对其优缺点进行简要评价。
一、人工智能技术人工智能技术是目前最为热门的新技术,AI技术的涵盖面非常广,从智能客服到智能投放都被广泛应用于营销推广领域中。
智能客服可以为消费者提供更为个性化的服务,智能投放可以为广告主提高投放效率。
优点:可以预计消费者喜好和需求,为消费者提供更为个性化的服务;可以精确投放广告,提高广告收益。
缺点:部分消费者会对个人信息泄露担心;目前人工智能技术还处于发展初期,有待完善。
二、内容营销内容营销早已不再是新概念,但是内容营销依旧在不断演变。
目前最为热门的内容营销方式是UGC(用户生成内容)和KOL 营销。
UGC可以让消费者更多地参与到产品和品牌推广中,KOL 营销则是通过社交媒体上的影响力人物来推广产品和品牌。
优点:内容营销可以增加消费者对品牌和产品的认知度和好感度;KOL营销引领了市场新潮流,提高了品牌曝光度。
缺点:UGC需要消费者自发参与,难以控制内容质量;KOL 营销可以引发虚假流量问题,需要注意粉丝的质量。
三、虚拟现实技术虚拟现实技术也是近几年来备受关注的新技术,如何把虚拟现实技术融入到营销推广中成为了一个热门话题。
虚拟现实技术可以让消费者更加直观地感受到产品和品牌,提供更加个性化的营销体验。
优点:可以增强消费者对产品和品牌的沉浸感,提高品牌认知度和忠诚度;可以提供更为生动和直观的营销体验。
缺点:虚拟现实技术需要较高的技术和资金支持,还处于发展初期;部分消费者可能会对此技术产生不适应和排斥。
四、数据分析数据分析一直都是营销推广中不可或缺的一环,但是随着大数据时代的到来,数据分析的重要性更加突出。
数据分析可以为广告主提供更为明确和精准的用户画像,帮助广告主更好地了解消费者需求和行为。
当今食品车间领域杀菌技术优缺点介绍
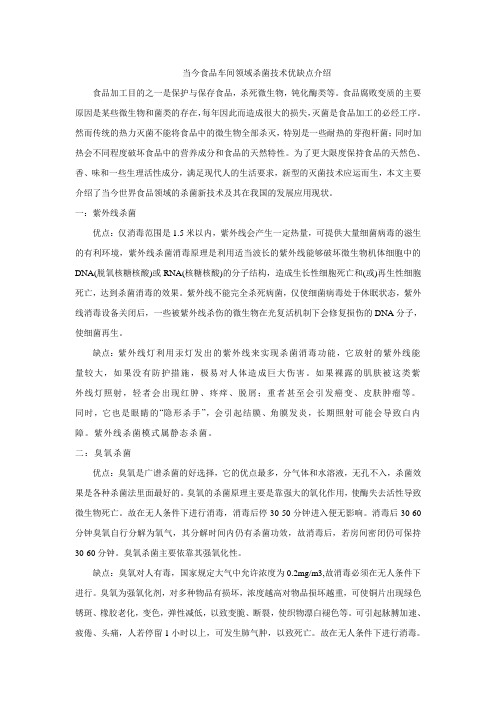
当今食品车间领域杀菌技术优缺点介绍食品加工目的之一是保护与保存食品,杀死微生物,钝化酶类等。
食品腐败变质的主要原因是某些微生物和菌类的存在,每年因此而造成很大的损失,灭菌是食品加工的必经工序。
然而传统的热力灭菌不能将食品中的微生物全部杀灭,特别是一些耐热的芽孢杆菌;同时加热会不同程度破坏食品中的营养成分和食品的天然特性。
为了更大限度保持食品的天然色、香、味和一些生理活性成分,满足现代人的生活要求,新型的灭菌技术应运而生,本文主要介绍了当今世界食品领域的杀菌新技术及其在我国的发展应用现状。
一:紫外线杀菌优点:仅消毒范围是1.5米以内,紫外线会产生一定热量,可提供大量细菌病毒的滋生的有利环境,紫外线杀菌消毒原理是利用适当波长的紫外线能够破坏微生物机体细胞中的DNA(脱氧核糖核酸)或RNA(核糖核酸)的分子结构,造成生长性细胞死亡和(或)再生性细胞死亡,达到杀菌消毒的效果。
紫外线不能完全杀死病菌,仅使细菌病毒处于休眠状态,紫外线消毒设备关闭后,一些被紫外线杀伤的微生物在光复活机制下会修复损伤的DNA分子,使细菌再生。
缺点:紫外线灯利用汞灯发出的紫外线来实现杀菌消毒功能,它放射的紫外线能量较大,如果没有防护措施,极易对人体造成巨大伤害。
如果裸露的肌肤被这类紫外线灯照射,轻者会出现红肿、疼痒、脱屑;重者甚至会引发癌变、皮肤肿瘤等。
同时,它也是眼睛的“隐形杀手”,会引起结膜、角膜发炎,长期照射可能会导致白内障。
紫外线杀菌模式属静态杀菌。
二:臭氧杀菌优点:臭氧是广谱杀菌的好选择,它的优点最多,分气体和水溶液,无孔不入,杀菌效果是各种杀菌法里面最好的。
臭氧的杀菌原理主要是靠强大的氧化作用,使酶失去活性导致微生物死亡。
故在无人条件下进行消毒,消毒后停30-50分钟进入便无影响。
消毒后30-60分钟臭氧自行分解为氧气,其分解时间内仍有杀菌功效,故消毒后,若房间密闭仍可保持30-60分钟。
臭氧杀菌主要依靠其强氧化性。
物联网技术的优缺点分析
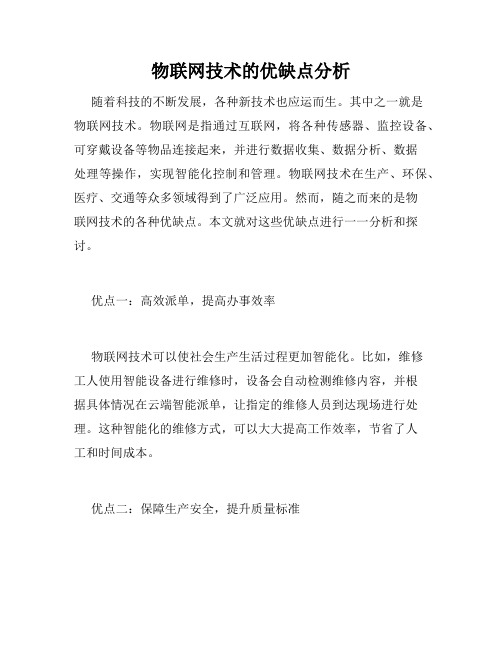
物联网技术的优缺点分析随着科技的不断发展,各种新技术也应运而生。
其中之一就是物联网技术。
物联网是指通过互联网,将各种传感器、监控设备、可穿戴设备等物品连接起来,并进行数据收集、数据分析、数据处理等操作,实现智能化控制和管理。
物联网技术在生产、环保、医疗、交通等众多领域得到了广泛应用。
然而,随之而来的是物联网技术的各种优缺点。
本文就对这些优缺点进行一一分析和探讨。
优点一:高效派单,提高办事效率物联网技术可以使社会生产生活过程更加智能化。
比如,维修工人使用智能设备进行维修时,设备会自动检测维修内容,并根据具体情况在云端智能派单,让指定的维修人员到达现场进行处理。
这种智能化的维修方式,可以大大提高工作效率,节省了人工和时间成本。
优点二:保障生产安全,提升质量标准物联网技术可以为生产环境带来更高的安全性和质量标准。
例如,在工厂生产过程中,可以通过智能设备实时检测机器运行状态、物料存储状态、物料使用状态等,避免误操作和危险事件发生。
此外,物联网技术也可以提供关键的数据监测,以便于在进行生产过程中进行及时的优化和调整。
这不仅可以确保生产效率和效果,还能够帮助企业降低成本,保护环境和员工的安全。
优点三:帮助发展智慧城市随着各种物品智能化的普及,物联网技术也可以帮助城市建设智慧城市。
例如,公共交通系统可以通过物联网技术实现实时监测,优化公共交通出行路线,缓解交通拥堵;智能道路的建设也可以通过物联网技术实现风险识别和预防,以提高交通安全性等等。
缺点一:数据泄露与隐私侵犯虽然物联网技术在数据处理和管理方面具有很大的优势,但是也面临着数据泄露和隐私侵犯的风险。
特别是当它关联到个人隐私数据时,隐私泄露风险会更大。
因此,安全保护机制必须一直在不断地完善,才能确保物联网技术的安全性和可靠性。
缺点二:采购成本高昂,制约了普及在实施物联网技术时,尤其是在中小企业,其设备的采购、设施改造、技术培训等成本都比较高。
这一点限制了物联网技术的普及和推广。
技术进步的优缺点
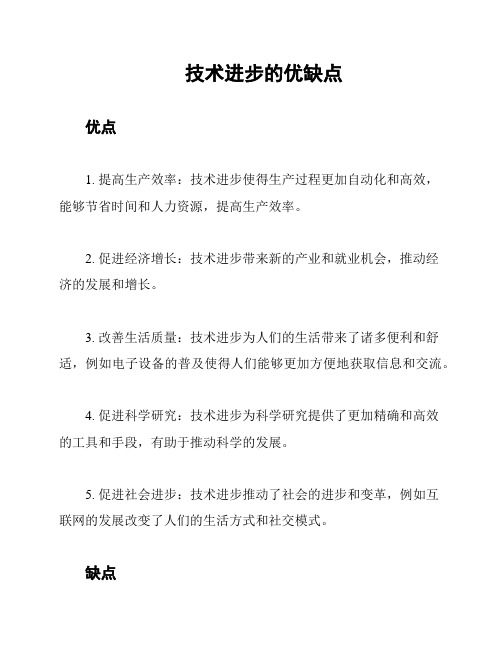
技术进步的优缺点
优点
1. 提高生产效率:技术进步使得生产过程更加自动化和高效,
能够节省时间和人力资源,提高生产效率。
2. 促进经济增长:技术进步带来新的产业和就业机会,推动经
济的发展和增长。
3. 改善生活质量:技术进步为人们的生活带来了诸多便利和舒适,例如电子设备的普及使得人们能够更加方便地获取信息和交流。
4. 促进科学研究:技术进步为科学研究提供了更加精确和高效
的工具和手段,有助于推动科学的发展。
5. 促进社会进步:技术进步推动了社会的进步和变革,例如互
联网的发展改变了人们的生活方式和社交模式。
缺点
1. 就业压力增加:技术进步导致一些传统工作被取代,导致部
分人员失去工作机会,增加了就业压力。
2. 社会不平等加剧:技术进步可能加剧社会的不平等现象,富
人更容易从技术进步中受益,而贫穷的人却更难享受新技术带来的
好处。
3. 隐私问题:技术进步使得个人信息更容易被获取和滥用,造
成个人隐私的泄露和侵犯。
4. 环境问题:技术进步也带来了一些环境问题,例如电子废物
的产生和电子设备对环境的污染。
5. 人与人之间的隔阂:技术进步可能导致人们与现实世界的社
交和人际交往减少,对人际关系产生一定的负面影响。
综上所述,技术进步带来了许多优点,如提高生产效率和改善
生活质量,但也存在一些缺点,如就业压力增加和社会不平等加剧。
我们需要在促进技术进步的同时,也要关注和解决其带来的问题,以实现科技和社会的共同发展。
工程测量新技术
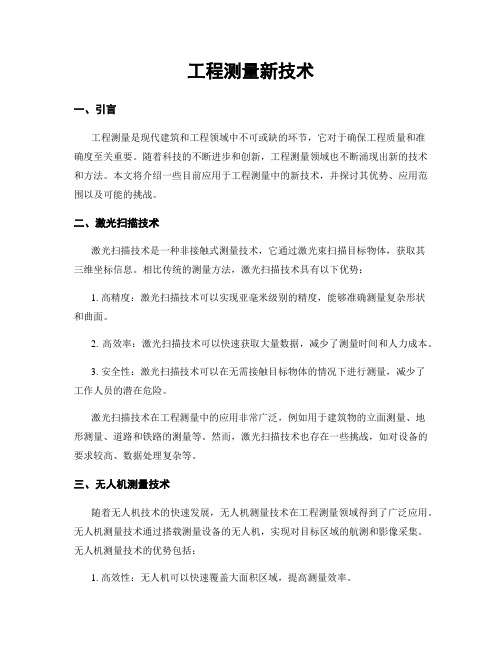
工程测量新技术一、引言工程测量是现代建筑和工程领域中不可或缺的环节,它对于确保工程质量和准确度至关重要。
随着科技的不断进步和创新,工程测量领域也不断涌现出新的技术和方法。
本文将介绍一些目前应用于工程测量中的新技术,并探讨其优势、应用范围以及可能的挑战。
二、激光扫描技术激光扫描技术是一种非接触式测量技术,它通过激光束扫描目标物体,获取其三维坐标信息。
相比传统的测量方法,激光扫描技术具有以下优势:1. 高精度:激光扫描技术可以实现亚毫米级别的精度,能够准确测量复杂形状和曲面。
2. 高效率:激光扫描技术可以快速获取大量数据,减少了测量时间和人力成本。
3. 安全性:激光扫描技术可以在无需接触目标物体的情况下进行测量,减少了工作人员的潜在危险。
激光扫描技术在工程测量中的应用非常广泛,例如用于建筑物的立面测量、地形测量、道路和铁路的测量等。
然而,激光扫描技术也存在一些挑战,如对设备的要求较高、数据处理复杂等。
三、无人机测量技术随着无人机技术的快速发展,无人机测量技术在工程测量领域得到了广泛应用。
无人机测量技术通过搭载测量设备的无人机,实现对目标区域的航测和影像采集。
无人机测量技术的优势包括:1. 高效性:无人机可以快速覆盖大面积区域,提高测量效率。
2. 灵活性:无人机可以灵活调整飞行路径和高度,适应不同的测量需求。
3. 多源数据融合:无人机可以搭载多种传感器,如摄像机、激光雷达等,实现多源数据的融合,提供更全面的测量结果。
无人机测量技术在土地测量、矿山测量、森林资源调查等领域有着广泛的应用。
然而,无人机测量技术也面临着飞行安全、数据处理和隐私保护等方面的挑战。
四、全站仪技术全站仪技术是一种集光学、机械、电子、计算机于一体的高精度测量设备。
它通过测量目标点的水平角度、垂直角度和斜距,计算出目标点的三维坐标。
全站仪技术的优势包括:1. 高精度:全站仪技术可以实现毫米级别的测量精度,适用于高精度测量需求。
2. 多功能性:全站仪可以进行角度测量、距离测量、坐标测量等多种测量任务。
医学超声影像新技术综述
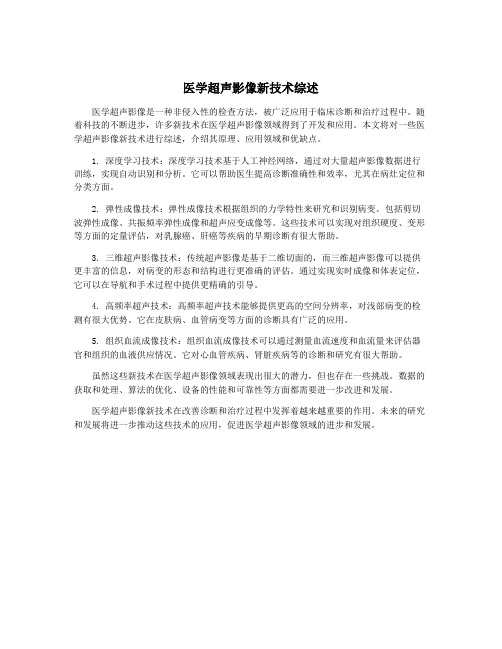
医学超声影像新技术综述医学超声影像是一种非侵入性的检查方法,被广泛应用于临床诊断和治疗过程中。
随着科技的不断进步,许多新技术在医学超声影像领域得到了开发和应用。
本文将对一些医学超声影像新技术进行综述,介绍其原理、应用领域和优缺点。
1. 深度学习技术:深度学习技术基于人工神经网络,通过对大量超声影像数据进行训练,实现自动识别和分析。
它可以帮助医生提高诊断准确性和效率,尤其在病灶定位和分类方面。
2. 弹性成像技术:弹性成像技术根据组织的力学特性来研究和识别病变。
包括剪切波弹性成像、共振频率弹性成像和超声应变成像等。
这些技术可以实现对组织硬度、变形等方面的定量评估,对乳腺癌、肝癌等疾病的早期诊断有很大帮助。
3. 三维超声影像技术:传统超声影像是基于二维切面的,而三维超声影像可以提供更丰富的信息,对病变的形态和结构进行更准确的评估。
通过实现实时成像和体表定位,它可以在导航和手术过程中提供更精确的引导。
4. 高频率超声技术:高频率超声技术能够提供更高的空间分辨率,对浅部病变的检测有很大优势。
它在皮肤病、血管病变等方面的诊断具有广泛的应用。
5. 组织血流成像技术:组织血流成像技术可以通过测量血流速度和血流量来评估器官和组织的血液供应情况。
它对心血管疾病、肾脏疾病等的诊断和研究有很大帮助。
虽然这些新技术在医学超声影像领域表现出很大的潜力,但也存在一些挑战。
数据的获取和处理、算法的优化、设备的性能和可靠性等方面都需要进一步改进和发展。
医学超声影像新技术在改善诊断和治疗过程中发挥着越来越重要的作用。
未来的研究和发展将进一步推动这些技术的应用,促进医学超声影像领域的进步和发展。
儿童口腔临床防治新技术研究

儿童口腔临床防治新技术研究随着医学技术的不断进步,儿童口腔临床防治也在不断发展。
目前,各种新技术已经广泛应用于儿童口腔临床防治中,取得了良好的效果。
本文将重点介绍几种新技术的应用及其优缺点。
一、口腔镜技术口腔镜技术是一种以口腔镜作为检查和治疗工具的技术。
该技术可以直接观察到口腔内的病变部位,无需切开口腔组织,能够减少手术创伤,缩短手术时间,恢复迅速。
在儿童口腔临床防治中,口腔镜技术被广泛应用于龋齿、牙髓炎、牙周病等病例的治疗。
相比于传统治疗方法,口腔镜技术可以减少牙体对牙齿的损伤,保护了牙齿的健康。
二、数字化印模技术数字化印模技术是一种以数字化印模代替传统手工印模的技术。
该技术采用激光扫描或三维成像技术获取牙齿和牙槽骨的数据,通过计算机处理和分析,生成精确的虚拟印模。
在儿童口腔临床防治中,数字化印模技术被广泛应用于牙冠修复、义齿修复等方面。
相比于传统手工印模,数字化印模具有精度高、时间短、舒适度好等优点。
三、激光技术激光技术是一种以激光器为主要工具的技术。
该技术可以用来进行牙髓炎、龋齿等的治疗。
激光治疗不仅可以精准地消除病原体,而且对牙体的侵蚀更少,不会损害牙体的健康。
在儿童口腔临床防治中,激光技术被广泛应用于龋齿的治疗。
与传统治疗方法相比,激光治疗可以减少牙体对牙齿的损伤,恢复更快。
四、电动牙刷电动牙刷是一种采用电力驱动的牙刷,它可以通过其超高频振动来有效去除牙齿表面的污渍和牙菌斑。
在儿童口腔临床防治中,电动牙刷被广泛应用于预防龋齿和牙周病。
相比于传统牙刷,电动牙刷更加智能化, 可以更好的保护牙齿健康。
总之,新技术的不断出现为儿童口腔临床防治带来了很大的便利。
但是,在应用新技术的同时,医生需注意新技术的优缺点,合理选择治疗方案,确保治疗效果最大化,也更好地保护了孩子们的口腔健康。
新技术在医学中的应用
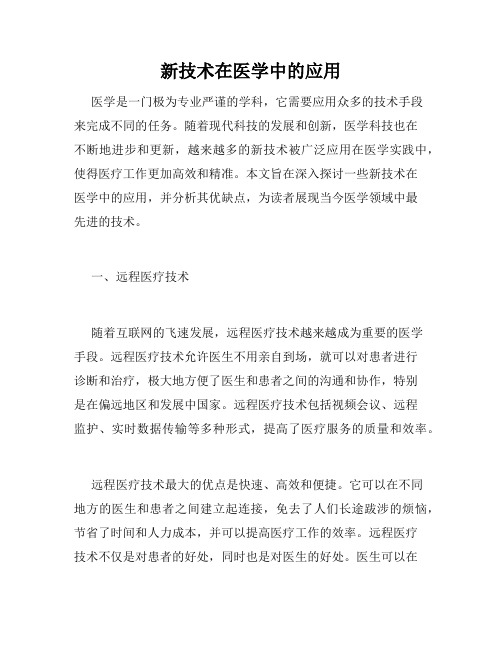
新技术在医学中的应用医学是一门极为专业严谨的学科,它需要应用众多的技术手段来完成不同的任务。
随着现代科技的发展和创新,医学科技也在不断地进步和更新,越来越多的新技术被广泛应用在医学实践中,使得医疗工作更加高效和精准。
本文旨在深入探讨一些新技术在医学中的应用,并分析其优缺点,为读者展现当今医学领域中最先进的技术。
一、远程医疗技术随着互联网的飞速发展,远程医疗技术越来越成为重要的医学手段。
远程医疗技术允许医生不用亲自到场,就可以对患者进行诊断和治疗,极大地方便了医生和患者之间的沟通和协作,特别是在偏远地区和发展中国家。
远程医疗技术包括视频会议、远程监护、实时数据传输等多种形式,提高了医疗服务的质量和效率。
远程医疗技术最大的优点是快速、高效和便捷。
它可以在不同地方的医生和患者之间建立起连接,免去了人们长途跋涉的烦恼,节省了时间和人力成本,并可以提高医疗工作的效率。
远程医疗技术不仅是对患者的好处,同时也是对医生的好处。
医生可以在家里或卫生中心的电脑前,随时随地对患者进行远程诊断,加快诊断和治疗进程,专注于患者的健康问题。
然而,远程医疗技术也有它的一些缺点和局限性。
最主要的是数据安全性和隐私保护的问题,防止患者的信息被窃取和滥用,以及诊断和治疗的准确性有待进一步提高。
除此之外,远程医疗技术使用的设备和通信网络也需要足够稳定和安全,以免影响医疗工作的质量。
二、3D打印技术3D打印技术是一种将数字模型转换成实体物品的先进技术。
它通过不断叠加薄片来构建三维实体物件,并可以制作出各种形状和尺寸的高精度构件。
在医学领域中,3D打印技术可以应用于制造人身上的各种医疗器械和部件(比如假体、牙齿模型、外科器械等),或者用来打印出人体器官模型,方便医生进行手术操作的前期模拟。
相比传统的手工制造或部件加工,3D打印技术具有很多优势。
它可以快速制造出高精度构件,减少了人力成本和时间浪费,大大提高了生产的效率和工艺的质量。
同时,3D打印技术可以普及快速定制和个性化医疗服务,根据不同的患者需求、身体特点、病情应用不同的材料打印器械和部件,可以更好地满足患者的个性化需求。
新技术汇报Masquelet技术
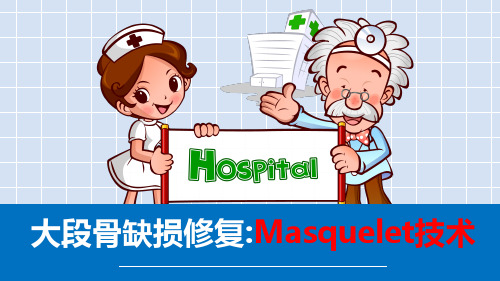
B
第二阶段:置入PMMA后6~8周,在不损伤诱导膜的前提下 ,取出PMMA,将足量的颗粒状自体松质骨填充骨缺损部位 ,再用钢板、髓内钉或外固定支架稳定固定患肢。
诱导膜在组织形态学上与滑膜组织相似,厚为0.5~2.0 mm,不具 有渗透性,可以防止周围软组织长入骨缺损,同时又避免了植入
病例
一期术后拍片
二期术中
二期术后第二天
感谢您的聆听!
Masquelet技术要点
第二阶段: a.纵向切开诱导膜,应用与骨膜下剥离相同的技术将其剥离; b.取出骨水泥后要打开髓腔以促进血液循环; c.骨移植材料: 1.自体松质骨是骨移植材料的“金标准”; 2.自体骨移植不能满足大节段骨缺损时,需要额外添加同种异体移植物或骨 替代物,但同种异体骨的含量不应超过1/3 ; 3.植骨应该松散地充填于骨缺损处,并超过两断端;植骨充填过紧会损伤血 管,影响血管长入,加速骨的坏死; 4.植骨后缝合诱导膜,创造一个密闭环境。
大段骨缺损修复:Masquelet技术
Masquelet技术优缺点
1986年,法国学者Masquelet等首次利用诱导膜和自体骨移植相结合的方法成功治 愈长为25 cm的大段骨缺损。
1.适用范围广,特别适用于开 放性、感染性骨长段缺损 2.操作简便,易于掌握
优点
3.膜内成骨、骨折愈合快,愈 合率高 4.并发症较少
1.需多次手术 2.二期需植入自体骨、植骨量 大
缺点
3.发生骨不愈合需进一步手术 4.潜在感染可能
Masquelet技术:主要包括2个相对独立的阶段
Masquelet技术应用的前提是骨缺损区有良好且完整的软组织覆盖。
A
第一阶段:需要对创面的骨和软组织进行彻底清创,将 聚甲基丙烯酸甲酯(polymethylmethacrylate, PMMA)填充 于骨缺损区,连接两断端,必要时行肌皮瓣转移覆盖修 复缺损软组织,然后根据骨折具体情况选用合适的固定方式 对骨折进行稳定的固定。 PMMA主要有2个作用:首先起到力学支撑的作用,防 止纤维组织长入骨缺损区,为后期植骨的生长提供良好 的生物微环境;其次,PMMA周围形成的诱导膜起到生物 保护作用,既可以促进植入骨的重建和再血管化,又避
虚拟仿真施工技术的优缺点总结及解决措施
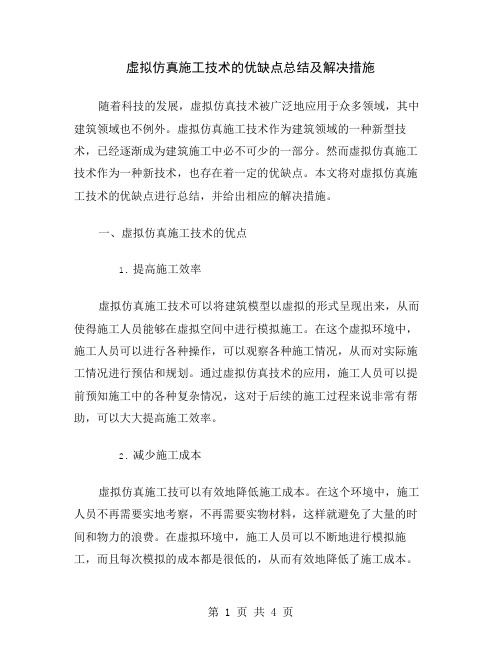
虚拟仿真施工技术的优缺点总结及解决措施随着科技的发展,虚拟仿真技术被广泛地应用于众多领域,其中建筑领域也不例外。
虚拟仿真施工技术作为建筑领域的一种新型技术,已经逐渐成为建筑施工中必不可少的一部分。
然而虚拟仿真施工技术作为一种新技术,也存在着一定的优缺点。
本文将对虚拟仿真施工技术的优缺点进行总结,并给出相应的解决措施。
一、虚拟仿真施工技术的优点1.提高施工效率虚拟仿真施工技术可以将建筑模型以虚拟的形式呈现出来,从而使得施工人员能够在虚拟空间中进行模拟施工。
在这个虚拟环境中,施工人员可以进行各种操作,可以观察各种施工情况,从而对实际施工情况进行预估和规划。
通过虚拟仿真技术的应用,施工人员可以提前预知施工中的各种复杂情况,这对于后续的施工过程来说非常有帮助,可以大大提高施工效率。
2.减少施工成本虚拟仿真施工技可以有效地降低施工成本。
在这个环境中,施工人员不再需要实地考察,不再需要实物材料,这样就避免了大量的时间和物力的浪费。
在虚拟环境中,施工人员可以不断地进行模拟施工,而且每次模拟的成本都是很低的,从而有效地降低了施工成本。
3.提高施工质量虚仿真施工技术可以有效地提高施工质量。
虚拟环境会模拟各种环境下的施工情况,施工人员可以通过模拟进行多次调试和测试,从而发现施工中潜在的问题和不足,并进行及时的提出和解决。
这样做可以大大提高施工质量,减少各种不必要的错误和浪费。
4.增强施工安全性虚拟仿真施工技术能够极大地增强施工的安全性。
在虚拟环境中,施工人员可以进行各种模拟施工,从而预估可能发生的事故和危险。
施工人员可以在虚拟环境中找出潜在的危险,从而在实际施工中避免类似事故的发生,这样可以大大保障施工安全。
二、虚拟仿真施工技术的缺点1.依赖于计算机技术虚拟仿真施工技术需要依赖于计算机技术,因此对计算机软硬件的要求比较高。
如果计算机不能满足要求,就无法实现虚拟仿真施技术。
并且计算机软硬件的更新换代速度比较快,如果不能及时跟进更新,就很容易被淘汰。
施工技术的优缺点与整改策略

施工技术的优缺点与整改策略一、施工技术的优缺点施工技术是在建筑工程中起着关键作用的重要因素之一。
不同的施工技术会对项目的质量、进度和成本产生直接影响。
本文将探讨施工技术的优缺点,并提出相应的整改策略。
A. 优点1. 提高效率:采用现代化施工技术可以大幅提升施工效率。
例如,预制构件的使用减少了现场加工和安装时间,加快了项目进展。
自动化设备和机械化作业也能够节省人力资源和时间成本。
2. 提升质量:先进的施工技术可以保证项目质量更加稳定可靠。
如使用BIM (建筑信息模型)技术进行设计与管理,可以极大程度上减少设计错误,并为施工过程提供精确数据支持。
此外,精细化测量技术和现代检测仪器也有助于提高质量监控水平。
3. 减少安全风险:新兴的施工技术在提供高效率的同时,也能减小事故风险。
比如,在高空作业中使用无人机巡检,避免了人工登高的危险。
机器人在危险区域进行作业,有效减少了劳动者暴露在有害环境下的风险。
B. 缺点1. 技术成本较高:引入新的施工技术需要投入大量的资金用于设备购置和人员培训。
特别是对于中小型企业来说,经济负担可能过重。
此外,技术迭代也可能导致频繁的更新和维护成本。
2. 技术难度与风险:新技术往往伴随着学习曲线和技术难题。
操作不当可能导致事故发生,且解决问题所需时间可能较长。
此外,一些新技术还可能存在供应链不稳定、专业人才匮乏等问题。
3. 依赖于外部环境:施工技术很大程度上受制于现场环境限制。
建筑材料供应、现场设备安装等方面都对施工效率产生影响。
同时,天气等自然因素也会对施工进度造成不可预测的干扰。
二、整改策略A. 提高效率1. 加强项目管理和组织:优化项目计划,确保施工进度合理安排,并适时调整以应对变化。
合理安排资源和工人,避免物资短缺和人力不足的情况。
2. 推行信息技术应用:引入BIM技术,改善设计与施工之间的配合与沟通,减少误差。
同时使用移动设备和建筑管理软件进行实时数据监控和交流。
3. 考虑预制构件:在项目规划初期就考虑使用预制构件,减少现场加工时间。
s-zorb技术的优缺点(个人整理珍藏版)

S-Zorb的技术优缺点中国石化S-Zorb装置已经开始运行,由于是一个全新的技术,还在不断完善中。
由于汽油质量升级需要,可能还有更多的装置要上此装置。
可能很快进入基础设计阶段,以下是我收集到此技术的优缺点。
优点1、适应于长周期运行。
2、适应原料硫含量的变化。
3、能够保证产品收率。
4、全运转周期反应及产品性质稳定。
5、氢气用量低。
6、汽油吸附脱硫(S-Zorb)技术是专为汽油脱硫开发的新技术。
该技术具有脱硫率高(产品汽油硫含量可低于10ppm)、耗氢少、能耗低、辛烷值损失少、液收高、操作费用低的特点。
S-Zorb技术的开发与应用有益于清洁城市的建设和发展。
7、高适应性。
根据康菲公司的介绍,S-Zorb并不像其他一些工艺路线那样不得不以减少油品总产量作为脱硫的代价。
康菲公司在其网站上还指出,S-Zorb技术适用于几乎所有进料,即,无论是重组分、轻组分、还是混合油气,经可以未经预处理或分馏直接送进S-Zorb装置脱硫。
而该装置在生产过程中并不产生大量的轻组分,从而有助于缓解出口油汽的过高压力。
与此同时,S-Zorb对各种现有的全厂总流程有很强的适应性。
其中,S-Zorb的再生单元对吸附剂的连续再生能力使S-Zorb装置具有很强的连续生产能力。
作为针对催化石脑油设计的非固定床的长效工艺流程,S-Zorb装置可以与催化装置采用同样的检修周期,这样,可以最大限度缩短检修期、最大限度增加油品产量。
8、低造价及低运行费用。
根据康菲公司的宣传资料,在综合考虑运行周期、辛烷值、保证总产量及各种操作参数的基础上,S Zorb技术具有相对较低的操作费用。
康菲公司还介绍说,S Zorb装置的主要工艺设备(流化床反应器及再生器)并没有显著增加投资,原因是,这些设备均采用普通碳钢材料、较低操作压力和较高的空速,这些因素均使得这些设备的成本大幅度降低。
9、可得到较高的液体收率。
单质Ni的加氢活性更高,苯加氢就是用的金属Ni作为加氢催化剂。
主要的给水处理新技术及其优缺点
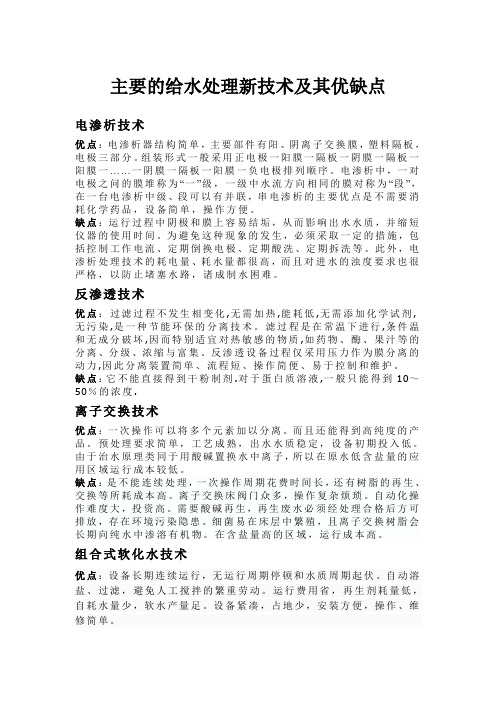
主要的给水处理新技术及其优缺点电渗析技术优点:电渗析器结构简单,主要部件有阳、阴离子交换膜,塑料隔板,电极三部分。
组装形式一般采用正电极一阳膜一隔板一阴膜一隔板一阳膜一……一阴膜一隔板一阳膜一负电极排列顺序。
电渗析中,一对电极之问的膜堆称为“一”级,一级中水流方向相同的膜对称为“段”,在一台电渗析中级、段可以有并联,串电渗析的主要优点是不需要消耗化学药品,设备简单,操作方便。
缺点:运行过程中阴极和膜上容易结垢,从而影响出水水质,并缩短仪器的使用时间。
为避免这种现象的发生,必须采取一定的措施,包括控制工作电流、定期倒换电极、定期酸洗、定期拆洗等。
此外,电渗析处理技术的耗电量、耗水量都很高,而且对进水的浊度要求也很严格,以防止堵塞水路,诸成制水困难。
反渗透技术优点:过滤过程不发生相变化,无需加热,能耗低,无需添加化学试剂,无污染,是一种节能环保的分离技术。
滤过程是在常温下进行,条件温和无成分破坏,因而特别适宜对热敏感的物质,如药物、酶、果汁等的分离、分级、浓缩与富集。
反渗透设备过程仅采用压力作为膜分离的动力,因此分离装置简单、流程短、操作简便、易于控制和维护。
缺点:它不能直接得到干粉制剂.对于蛋白质溶液,一般只能得到10~50%的浓度.离子交换技术优点:一次操作可以将多个元素加以分离。
而且还能得到高纯度的产品。
预处理要求简单,工艺成熟,出水水质稳定,设备初期投入低。
由于治水原理类同于用酸碱置换水中离子,所以在原水低含盐量的应用区域运行成本较低。
缺点:是不能连续处理,一次操作周期花费时间长,还有树脂的再生、交换等所耗成本高。
离子交换床阀门众多,操作复杂烦琐。
自动化操作难度大,投资高。
需要酸碱再生,再生废水必须经处理合格后方可排放,存在环境污染隐患。
细菌易在床层中繁殖,且离子交换树脂会长期向纯水中渗溶有机物。
在含盐量高的区域,运行成本高。
组合式软化水技术优点:设备长期连续运行,无运行周期停顿和水质周期起伏。
煤矿新技术、新工艺、新设备和新材料
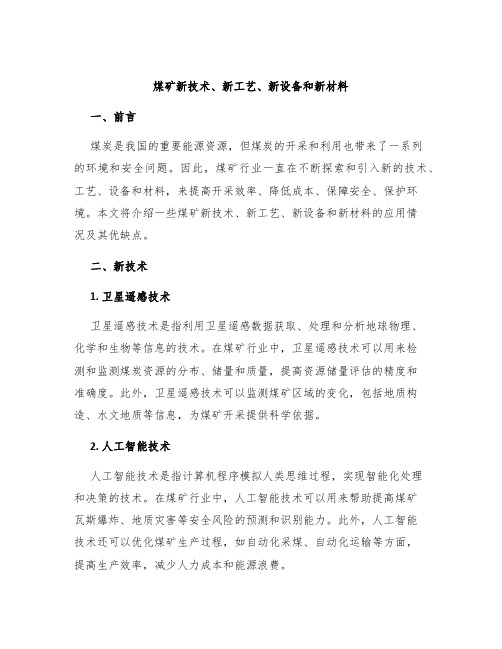
煤矿新技术、新工艺、新设备和新材料一、前言煤炭是我国的重要能源资源,但煤炭的开采和利用也带来了一系列的环境和安全问题。
因此,煤矿行业一直在不断探索和引入新的技术、工艺、设备和材料,来提高开采效率、降低成本、保障安全、保护环境。
本文将介绍一些煤矿新技术、新工艺、新设备和新材料的应用情况及其优缺点。
二、新技术1. 卫星遥感技术卫星遥感技术是指利用卫星遥感数据获取、处理和分析地球物理、化学和生物等信息的技术。
在煤矿行业中,卫星遥感技术可以用来检测和监测煤炭资源的分布、储量和质量,提高资源储量评估的精度和准确度。
此外,卫星遥感技术可以监测煤矿区域的变化,包括地质构造、水文地质等信息,为煤矿开采提供科学依据。
2. 人工智能技术人工智能技术是指计算机程序模拟人类思维过程,实现智能化处理和决策的技术。
在煤矿行业中,人工智能技术可以用来帮助提高煤矿瓦斯爆炸、地质灾害等安全风险的预测和识别能力。
此外,人工智能技术还可以优化煤矿生产过程,如自动化采煤、自动化运输等方面,提高生产效率,减少人力成本和能源浪费。
3. 3D打印技术3D打印技术是指利用计算机控制技术,将数字模型逐层打印成实体模型的技术。
在煤矿行业中,3D打印技术可以用来制造模型、零部件、工具等,以替代传统的生产方式,减少时间和成本。
此外,在煤矿灾害救援方面,利用3D打印技术可以制造出特种救援设备,提高应对突发事件的能力。
三、新工艺1. 光伏发电光伏发电是指利用太阳能光电效应,将光能转化为电能的技术。
在煤矿行业中,光伏发电可以用来提供煤矿用电,减少传统电力的使用,同时降低煤矿生产过程中的能源消耗,实现绿色低碳生产。
2. 水泥矿尾固化技术水泥矿尾固化技术是指将煤矿废弃物和水泥等物料混合固化的技术。
在煤矿行业中,采用水泥矿尾固化技术可以有效地处理煤矿废弃物、减少对环境的污染,同时利用煤炭企业本身的产废资源来生产水泥材料,实现资源循环利用。
3. 条带煤压紧成形技术条带煤压紧成形技术是指将煤炭通过压缩成特定形状,以提高其堆积密度、降低运输成本的技术。
施工中的建筑工程施工新技术应用
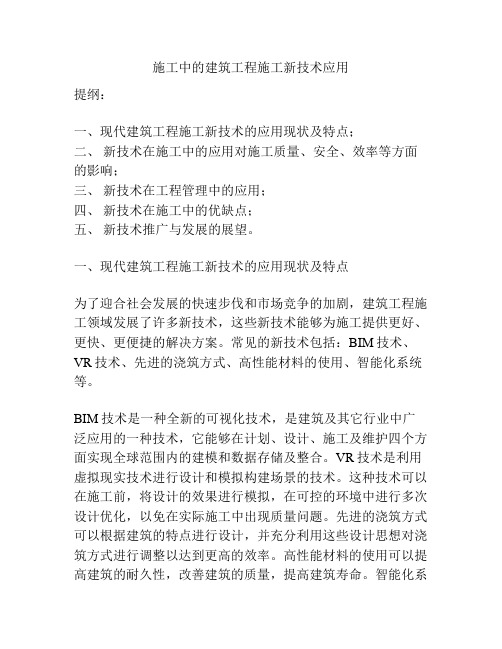
施工中的建筑工程施工新技术应用提纲:一、现代建筑工程施工新技术的应用现状及特点;二、新技术在施工中的应用对施工质量、安全、效率等方面的影响;三、新技术在工程管理中的应用;四、新技术在施工中的优缺点;五、新技术推广与发展的展望。
一、现代建筑工程施工新技术的应用现状及特点为了迎合社会发展的快速步伐和市场竞争的加剧,建筑工程施工领域发展了许多新技术,这些新技术能够为施工提供更好、更快、更便捷的解决方案。
常见的新技术包括:BIM技术、VR技术、先进的浇筑方式、高性能材料的使用、智能化系统等。
BIM技术是一种全新的可视化技术,是建筑及其它行业中广泛应用的一种技术,它能够在计划、设计、施工及维护四个方面实现全球范围内的建模和数据存储及整合。
VR技术是利用虚拟现实技术进行设计和模拟构建场景的技术。
这种技术可以在施工前,将设计的效果进行模拟,在可控的环境中进行多次设计优化,以免在实际施工中出现质量问题。
先进的浇筑方式可以根据建筑的特点进行设计,并充分利用这些设计思想对浇筑方式进行调整以达到更高的效率。
高性能材料的使用可以提高建筑的耐久性,改善建筑的质量,提高建筑寿命。
智能化系统可以将整个施工过程进行自动化管理,减少工人的疲劳和错误率,提高施工的效率和安全性。
二、新技术在施工中的应用对施工质量、安全、效率等方面的影响新技术应用于施工,在施工成本和效率的提高方面取得了显著的成效。
新技术的应用为施工的质量、安全和效率带来了重要的影响。
1、提高施工质量。
由于新技术的应用,使施工质量得到了显著提高。
BIM技术能够对建筑的各个部位进行详细的模拟和构建,这样可以让施工员在施工过程中更加清楚地了解施工位置和构造形式,在避免出现设计失误的同时,可有效的提高施工质量。
高性能材料的使用可以提高建筑的抗震性能,并在一定程度上提高施工质量。
2、提高施工安全。
新技术的应用对施工安全的风险进行了有效的控制和解决。
例如,VR技术的应用能够使施工人员在施工前在虚拟环境中进行多次模拟,可降低建筑安全事故的发生概率。
空气质量监测和治理的新技术
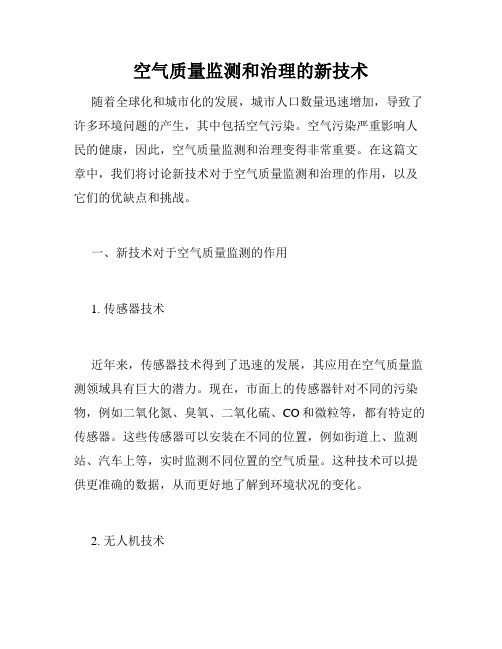
空气质量监测和治理的新技术随着全球化和城市化的发展,城市人口数量迅速增加,导致了许多环境问题的产生,其中包括空气污染。
空气污染严重影响人民的健康,因此,空气质量监测和治理变得非常重要。
在这篇文章中,我们将讨论新技术对于空气质量监测和治理的作用,以及它们的优缺点和挑战。
一、新技术对于空气质量监测的作用1. 传感器技术近年来,传感器技术得到了迅速的发展,其应用在空气质量监测领域具有巨大的潜力。
现在,市面上的传感器针对不同的污染物,例如二氧化氮、臭氧、二氧化硫、CO和微粒等,都有特定的传感器。
这些传感器可以安装在不同的位置,例如街道上、监测站、汽车上等,实时监测不同位置的空气质量。
这种技术可以提供更准确的数据,从而更好地了解到环境状况的变化。
2. 无人机技术随着无人机技术的快速发展,越来越多的机构开始使用无人机来监测空气质量。
无人机的特点在于可以飞行到空气质量监测站无法到达的区域,并能够进行空中拍摄。
相对于传统的监测方法,无人机技术可以提供更多的空气质量数据,尤其是在城市交通拥堵、建筑工地等区域,传统监测无法涵盖到的地方。
3. 人工智能人工智能技术可以将大量的空气质量数据进行分析和处理,从而提取更加有用的信息和知识。
人工智能可以自动识别和分析大量的数据,建立空气质量预测模型,通过对不同因素的分析,发现环境和天气状况对空气质量的影响。
这种技术可以帮助环保管理部门快速地了解环保情况,并及时采取有效的空气质量治理措施。
二、新技术对于空气质量治理的作用1. 雾炮治理技术雾炮治理技术是一种新型的空气治理方式。
它通过使用雾状液体对于粉尘颗粒进行捕捉,以达到净化空气的目的。
与传统的空气治理方式不同,雾炮治理技术不需要使用耗能和污染的空气净化设备,不会对环境和人体健康产生负面影响。
尤其是在城市建筑工地、露天矿山、物流码头等各类污染源较为分散的场所,雾炮治理技术可以取得较好的治理效果。
2. 智能交通管理系统智能交通管理系统可以在交通拥堵时,通过控制车辆流量,减少尾气排放量,达到一定的空气净化效果。
如何避免企业对新技术的过度依赖
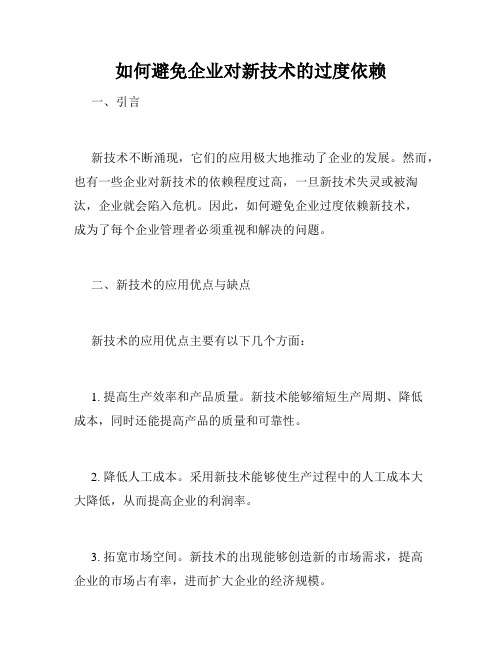
如何避免企业对新技术的过度依赖一、引言新技术不断涌现,它们的应用极大地推动了企业的发展。
然而,也有一些企业对新技术的依赖程度过高,一旦新技术失灵或被淘汰,企业就会陷入危机。
因此,如何避免企业过度依赖新技术,成为了每个企业管理者必须重视和解决的问题。
二、新技术的应用优点与缺点新技术的应用优点主要有以下几个方面:1. 提高生产效率和产品质量。
新技术能够缩短生产周期、降低成本,同时还能提高产品的质量和可靠性。
2. 降低人工成本。
采用新技术能够使生产过程中的人工成本大大降低,从而提高企业的利润率。
3. 拓宽市场空间。
新技术的出现能够创造新的市场需求,提高企业的市场占有率,进而扩大企业的经济规模。
但是,新技术的应用也存在一些缺点:1. 对技术人员的要求较高。
新技术的应用需要专业的技术人才进行支持,企业需要投入较大的人力、物力和财力去培训和招募合适的技术人才。
2. 存在技术风险。
新技术的应用需要消耗大量的科研和开发成本,同时也存在技术不成熟、对环境的影响等风险,企业需要进行风险评估并进行合理的风险控制。
三、如何避免企业对新技术的过度依赖1. 多元化技术投资企业在引入新技术时,应该注重多元化技术投资,即同时投资多种技术,避免企业仅仅依靠某一种技术。
这样,即使一种技术出现问题或被淘汰,企业仍可以有其他技术作为补充,保证企业的稳定发展。
2. 建立完善的应急预案企业应该根据新技术的特点,制定合理的应急预案。
应急预案应包括新技术的故障排除、备用设备的准备和应对措施等内容,以应对可能出现的意外情况,保证企业的稳定运转。
3. 加强技术研发企业应当加强技术研发,不断探索新技术,了解市场的发展趋势,并使自身具有新技术的研究和开发能力。
这样,企业就可以更好地适应市场变化,提高竞争力。
4. 建立多种盈利模式企业应该建立多种盈利模式,通过多元化的实践和实验,寻找出适合自己的盈利模式,不仅仅依赖单一的技术产品,而是通过丰富的产品线和销售渠道来增加收入,以保证企业的长期发展。
- 1、下载文档前请自行甄别文档内容的完整性,平台不提供额外的编辑、内容补充、找答案等附加服务。
- 2、"仅部分预览"的文档,不可在线预览部分如存在完整性等问题,可反馈申请退款(可完整预览的文档不适用该条件!)。
- 3、如文档侵犯您的权益,请联系客服反馈,我们会尽快为您处理(人工客服工作时间:9:00-18:30)。
Update on Gene Expression Analysis,Proteomics,and Network Discovery Gene Expression Analysis,Proteomics,andNetwork Discovery1Sacha Baginsky,Lars Hennig,Philip Zimmermann,and Wilhelm Gruissem*Department of Biology and Zurich-Basel Plant Science Center,ETH Zurich Universita¨tstrasse2, 8129Zurich,SwitzerlandTechnological advances in biological experimenta-tion are now enabling researchers to investigate living systems on an unprecedented scale by studying ge-nomes,proteomes,or molecular networks in their entirety.Genomics technologies have led to a para-digm shift in biological experimentation because they measure(profilemost or even all components of one class(e.g.transcripts,proteins,etc.in a highly parallelway.Whether gene expression analysis using micro-arrays,proteome and metabolome analysis using mass spectrometry,or large-scale screens for genetic interactions,high-throughput profiling technologies provide a rich source of quantitative biological information that allows researchers to move beyond a reductionist approach by both integrating and un-derstanding interactions between multiple compo-nents in cells and organisms(Fig.1;for a recent update of bioinformatics tools,see Pitzschke andHirt,2010.Currently,most genomics experiments involve profiling tr anscripts,proteins,or metabolites. Increasing efforts to complement molecular data with phenotypic information will further advance our un-derstanding of the quantitative relationships between molecules in directing systems behavior and function. In the following Update we will briefly review recent advances in thefield and highlight advantages and limitations of current approaches to develop models of genetic and molecular networks that aim to describe emergent properties of plant systems.GENOMICS TECHNOLOGIES:THE POWER OF GENOME-SCALE QUANTITATIVE DATA RESOLUTION PROFILING TRANSCRIPTOMES Transcriptprofiling offers the largest coverage and a wide dynamic range of gene expression information and can often be performed genome wide.Micro-arrays are currently most popular for transcript profiling and can be readily afforded by many laboratories.Various commercial and academic micro-array platforms exist that vary in genome coverage, availability,specificity,and sensitivity(Table I.Micro-arrays manufactured by Affymetrix are probably most commonly used in plant biology(Redman et al.,2004; Rehrauer et al.,2010,but commercial arrays from Agilent or arrays from the academic Complete Arabi-dopsis Transcriptome MicroArray(CATMAconsor-tium are often used as well(for review,see Busch and Lohmann,2007.Serial analysis of gene expression (SAGEand massively parallel signature sequencing (MPSSare well-established alternatives to microar-rays.Both techniques can be superior to microarrays because they do not depend on prior probe selection. More recently,direct sequencing of transcripts by high-throughput sequencing technologies(RNA-Seq has become an additional alternative to microarrays and is superseding SAGE and MPSS(Busch and Lohmann,2007.Like SAGE and MPPS,RNA-Seq does not depend on genome annotation for prior probe selection and avoids biases introduced dur-ing hybridization of microarrays.On the other hand, RNA-Seq poses novel algorithmic and logistic chal-lenges,and current wet-lab RNA-Seq strategies re-quire lengthy library preparation procedures. Therefore,RNA-Seq is the method of choice in projects using nonmodel organisms and for transcript discov-ery and genome annotation.Because of their robust sample processing and analysispipelines,often micro-arrays are still a preferable choice for projects that involve large numbers of samples for profiling tran-scripts in model organisms with well-annotated ge-nomes.Tools such as Genevestigator(Hruz et al.,2008 and MapMan(Usadel etal.,2009allow researchers to organize large gene expression datasets and analyze them for relational networks within a single experi-ment or across many experiments(contextual meta-analysis.PROFILING EPIGENOMES AND TRANSCRIPTION FACTOR BINDINGMuch control of gene expression occurs at the level of transcription,and information on genome-wide chromatin profiles(epigenomesand transcription factor binding to promoters is needed to decipher1This work was supported by the European Union(EU FrameworkProgram6,AGRON-OMICS;grant no.LSHG–CT–2006–037704,the Swiss National Science Foundation,CTI(Swiss Innovation Promotion Agency,ETH Zurich,and the Functional Genomics Center Zurich for our profiling experiments.*Corresponding author;e-mail wgruissem@ethz.ch.The author responsible for distribution of materials integra l to the findings presented in this article in accordance with the policy described in the Instructions forAuthors(is: Wilhelm Gruissem(wgruissem@ethz.ch./cgi/doi/10.1104/pp.109.150433the inherent logic of transcriptional regulation.Chromatin immunoprecipitation (ChIPcoupled to microarray analysis (ChIP-chipor high-throughput sequencing (ChIP-Seqcan generate such data.In plants,DNA methylation,repressive and activating chromatin marks,as well as histone variants have been mapped onto the genome (for review,see Zhang,2008,but because such marks are expected to differ between cell types and developmental stages,more targeted epigenome profiling is needed in thefuture.Targeted analysis of DNA methylation during seed development,forinstance,revealed unexpected genome-wide demethylation (Gehring et al.,2009;Hsieh et al.,2009.ChIP-chip was also used for global mapping of binding sites of transcription factors such as TGA2and SEPALLATA3and to refine definitions of binding mot ifs that were previously determined by in vitro experiments (Thibaud-Nissen etal.,2006;Kaufmann et al.,2009.It was found that SEPAL-LATA3is a key component in the regulatory tran-scriptional network underlying the formation of floral organs.In a comparative experiment ChIP-chip and ChIP-Seq gave very similar results (Kaufmann etal.,2009.This is encouraging because bias introduced by the profiling technology seems not to severely con-found studies on global protein-binding profiles.Cur-rently,work is going on in several laboratories to establish a compendium of transcription factor binding sites in Arabidopsis (Arabidopsis thaliana .Thus,more genome-wide data sets are in reach that could provide causal explanations for transcriptional profiles.PROFILING PROTEOMESGene expression is a highly regulated,multistep process,and it is impossible to predict the exact protein concentration or activity from the measure-ment of mRNA levels.Proteomics has therefore be-come a key tool in systems biology because it provides quantitative and structural information about pro-teins,which are the major functional determinants of cells.Phenotypic alterations associated with genetic perturbations often result from changes in protein accumulation or stability,or changes in protein p osttranslational modifications,which can disrupt protein-protein interactions and network connectivity (Gstaiger and Aebersold,2009.Quantitative protein information complements data from transcriptional profiling and metabolomics.It represents a key link between different levels of gene expression regulation and provides insights into their causal relationships.Unlike transcriptional profiling,however,comprehen-sive proteome analysis remains challenging,and in-formation about proteome complexity and dynamics is far from complete (Cox and Mann,2007.Moreover,the rate of metabolite synthesis is often controlled by regulatory posttranslational modifications of enzymes and not only by their rmation about quantitative relationships between RNA and protei n accumulation,posttranslational protein modifications,and metabolite levels is therefore required to fully understand regulatory circuits that control systems behavior and function.Protein quantification can be absolute or relative (Table I.While relative protein quantification mostly depends on stable isotopes,absolute quantification of comprehensive protein sets is much more difficult.Recent improvements in statistical dataevaluation and increasing accuracy of mass spectrometry instru-ments allow quantifying large numbers of proteins in shotgun-type experiments on the basis of spectral counting (Lu et al.,2007.This method is reliable and comparable to most other quantification methods,including two-dimensional PAGE-based protein stain-ing;however,the protein dataset must be very large.More accurate information about the exact in vivo concentration of individual proteins requires special-ized targeted approaches.Current methods for absolute protein quantification include isotope dilution strategies using isotopically labeled peptides as internal standards (for acompre-Figure 1.Relationships between supracellular com-ponents (biological systems,intracellular compo-nents,and the function and behavior of these components are revealed by the interaction of indi-vidual components.Systems biological approaches aim at modeling these interactions to find primary relationships and to distinguish causality and effect.The understanding of how these interactions are regulated allows making predictions on function,behavior,and survival.Gene Expression Analysis,Proteomics,and Network Discoveryhensive review,see Brun et al.,2009.Signature pep-tides for internal standardization are characteristic for a protein of interest,and are often referred to as proteotypicpeptides(PTPs.In AQUA,PTPs are added to analytical protein samples in known concen-trations.The protein samples are subsequently scan-ned for PTPs of ing the extracted ion chromatograms the native peptide can then be quan-tified relative to the added PTP(Kus ter et al.,2005.A modification of this strategy accounts for quantifica-tion errors derived from incomplete tryptic digest of the analytical sample.In QconCAT(for quantification concatamer,a synthetic protein with concatenated,isotopically labeled PTPs is expressed as recombinant protein in a biological system,added to the sample prior to Trypsin treatment and carried through the digestion procedure,such that losses from incomplete tryptic digestion will also affect the quantity of the PTPs.Both the AQUA and the QconCAT strategies are incompatible with upstream fractionation techniques, which is a potential problem in biomarker quantifi-cation.A way around this constraint is offered by the protein standard absolute quantification strategy, which uses isotopically labeled protein standards that are added to the sample prior to fractionation. Several prediction tools exist that help to define theTable I.Advantages and disadvantages of various technologies for the measurement of transcript and protein abundanceA sys tematic performance assessment for the different protein quantification techniques was recently conducted(Turck et al.,2007and a detailed description of the different quantification techniques along with examples for application in the plantfield is available(Baginsky,2009.Technologies Advantages Disadvantages TranscriptsMPSS Sequences do not need to be known inadvanceRelatively expensive,laboriousMicroarrays Genome wide,relatively cheap,streamlined handling,oligos Sequences must be known in advance; limited sensitivity due to hybridizationQuantitative reverse transcription-PCR High precision and high sensitivityIncreasingly multiplexed Not genome wide;data normalization sensitive to method/choice of reference genesHigh-throughput sequencing Sequences do not need to be known inadvance;possibility to sequence veryshort sequences Expensive at the moment,few solutions for downstream analysis;direct read outProteinsRelative quantification via iTRAQ Established labeling protocol with stableisotopes,good reproducibility,relevantregulation factor can be determinedfrom the data,multiplexing to up toeight samples,produces good qualitytandem mass spectrometry spectra Cost and effort,the analysis software is still not optimal,fluctuations between different s oftwares possibleRelative quantification via stable isotope labeling with amino acids in cell culture Established protocol for the labelingof cell culture proteins,reliablequantification possibleRestricted to cell cultureRelative quantification via extra cted ion chromatograms Comes at no additional costs,softwaretools for alignment and normalizationare available(e.g.SuperHirn;Mueller et al.,2007Only applicable to very similarsamples and very similar liquidchromatography-mass spectrometryruns,done within a small timewindow,baseline normalization issometimes a problemAbsolute quantification via AQUA peptides Highly sensitive absolute quantificationon the basis of isotopically labeledPTPs,targeted analyses possiblevia specific scan methods(e.g.SRMFi nding suitable PTPs and characteristic parent to daughter ion transitionsnot straightforward,selectivity of the PTP transitions not always unambiguousAbsolute quantification via QconCAT Excellent for the quantification of proteincomplex stoichiometry,lower costcompared to AQUA,PTPs aresynthesized in a biological system Unsuitable for the quantification of posttranslational modifications, optimization necessary,exact quantification of the standardis vital,incompatible with sample fractionationAbsolute quan tification via protein standard absolute quantification Excellent for the quantification ofindividual,low abundance proteins,compatible with fractionationRestricted to few proteins,up scalingdifficult,quantifications ofposttranslational modifications notpossibleAbsolute quantification via normalized spectral counting(APEX;Lu et al.,2007No additional costs,produces reliableresults with large-scale datasetsQuantification of individual proteinsmust be validated by additional tools,unreliable for small datasetsBaginsky et al.most suitable PTPs for the detection and quantification of specificproteins.However,only experimental data provide the necessary reliability for PTP selection because in practice PTP prediction often deviates from experimental observations.Therefore,efforts are under way to catalogue PTPs for model organism proteomes.Proteome maps for Arabidopsis generated PTPs for4,105proteins,many of which may be opti-mal for the detection of proteins in different organs (Baerenfaller et al.,2008.Similar quantitative approaches are also used for metabolites,because in addition to RNA and protein levels,understanding the function and behavior of metabolic networks requires global information about metabolite concentrations andfluxes as well.In recent year s,much progress has been made in metabolic profiling,and the interested reader is referred to recent reviews(e.g.Issaq et al.,2009,and refs.therein.TRANSCRIPTS AND MORE TRANSCRIPTS:WHAT CAN WE LEARN FROM GENE EXPRESSION ANALYSIS?During the analysis of large gene expression data-sets the researcher is often confronted with several questions.How do we interpret a mathematical rela-tionship between genes or between genes and condi-tions?For example,does a high correlation between two genes mean that they are coregulated,or could one of them be the positive regulator of the other?Or can we assume that they are involved in the same pathway or biological process?Although it is not possible to answer these questions conclusively from gene expression data alone,a number of parallel approaches can be useful to distinguish between dif-ferent scenarios.For example,Gene Ontology enrich-ment analysis can provide confidence that a given gene cluster is enriched in genes that areknown to have a common function,cellular location,or biolog-icalprocess.Similarly,conserved cis-regulatory ele-ments in the promoters of genes from the same cluster indicate that they are likely coregulated.Although these methods do not establish proof of the nature of the relationship between genes,they allow formulat-ing hypotheses that can be tested in the laboratory.In summary,although gene expression analysis by itself is rather descriptive(i.e.describing how genes re-spond to various test conditions or tissues,it is a valuable validation tool and an excellent starting point to study novel cellular process and to formulate novel hypotheses.A major challenge of genome-scale transcription analysis is the very large number of predictors(genes compared to a generally small number of measure-ments(microarrays.Without appropriate statistical measures to correct for multiple testing and including false discovery rates,almost any approach will yield significantgenes,including many false positives.The creation of large databases in recent years has brought an additional layer of complexity and precautions to take(see Table II.For example,large databases such as Genevestigator(Hruz et al.,2008not only profile a large number of genes,but also allow contextual meta-Table II.Overview of some of the most popular plant gene expression microarray platforms and the number of available experimentsin ArrayExpressThe Arabidopsis ATH1array is the most frequently used microarray,followed by the CATMA25k and23k arrays.In all,approximately750 Arabidopsis microarray experiments have been published so far.Rice(Oryza sativaand barley(Hordeum vulgareare the second and third plant species in terms of microarray experiments published.Soybean(Glycine maxalso has a high number of arrays,but this is due to a single very large experiment containing2,521arrays.IPK,Leibniz Institute of Plant Genetics and Crop Plant Research;TIGR,The Institute for Genomic Research.Species ProviderArrayFormatArray Name Experiments ArraysArabidopsis Affymetrix8K AG41352Affymetrix22K ATH15548,895Agilent22K Arabidopsis234253Agilent44K Arabidopsis3760CATMA25K CATMA2_URGV to CATMA2.3_URGV83851CATMA23K CATMA Arabidopsis23K array501,290TIGR26K TIGR Arabidopsis whole genome6264 Rice Affymetrix57K GeneChip Rice Genome Array29418Agilent21K Agilent Rice Oligo Microarray22164 Barley Affymetrix22K GeneChip Barley Genome Array351,165IPK6K+4K IPK barley PGRC1_A and B7324 Medicago Affymetrix61K GeneChip Medicago Genome Array19218 Maize Affymetrix17K GeneChip Maize Genome Array22370 Soybean Affymetrix61K GeneChip Soybean Genome Array223,236 Tomato(SolanumlycopersicumAffymetrix10K GeneChip Tomato Genome Array6127 Grape(Vitis viniferaAffymetrix16K GeneChip Vitis vinifera Genome Array6239 Wheat(Triticum aestivumAffymetrix61K GeneChip Wheat Genome Array25811 Total96819,037Gene Expression Analysis,Proteomics,and Network Discoveryanalysis of several hundred conditions,each of which is covered by only a small number of replicates(usu-ally3–5.While some genes will respond to a small number of conditions and therefore their expression is easier to contextualize and interpret,other genes will respond to dozens or hundreds of conditions.It is often very difficult to distinguish primary effects from secondary effects,because the intensity of the effect does not necessarily relate to the direct involvement of the corresponding condition in regulating a specific target gene.Breaking down these effects into local patterns(e.g.by using a biclustering algorithm;Prelic et al.,2006helps infinding out conditions that are more directly linked to the gene of interest.APPROACHING THE TARGET:FROM ORGANS TO TISSUES AND CELLSMost transcript and protein profiling experiments analyze mixtures of tissues containing different cell types and organelles.This approach reveals certain global patterns,but quantitative analyses and model-ing is limited with such complexdata.Therefore meth-ods for organ(or bettercell-type-specific transcript and proteinprofiling as well as for organelle-specific proteomics are needed.Four types of approaches are now commonly used to sample RNA and/or proteins from selected celltypes:(1micropipetting,(2laser capture microdissection(LCM,(3protoplasting and sorting,and(4polysome immunopurification(for review,see Zanetti etal.,2005;Hennig,2007;Nelson et al.,2008.Micropipetting using microcapillaries directly ex-tracts the contents from selected cells.It has been successfully applied to various leaf cell types and for phloem but extraction is more difficult from internal cells.LCM involves sectioning of frozen orembedded tissue,and subsequent dissection of the region of interest using laser excision.Applications of LCM include studies of vascular tissue,epidermis,and pericycle in maize(Zea maysand seed development in Arabidopsis.Micropipetting and LCM are usually very l abor intensive and difficult for isolation of small cells such as in meristems.Because of the limited amount of material that can be captured,they work well for transcript profiling,which can use amplifica-tion steps,but provide only a very small coverage of the proteome.As an alternative,protoplasting and cell sorting offers rapid and accurate isolation of RNA from small cells.Specific tissues or cell types that are labeled by expression of GFP are isolated by proto-plasting and sorted through afluorescence-activated cell lions of cells can be processed within 1to2h,but care has to be taken to exclude changes in gene expression profiles by sample processing. This technique was successfully applied to measure genome-wide expression profiles in more than15root regions,establishing a compendium of digital in situ data(Birnbaum etal.,2003;Cartwright et al.,2009.It will be interesting to test whether this approach can also be used for protein profiling.Polysome immuno-purification is based on the tissue-specific expression of the FLAG-tagged ribosomal protein L18in trans-genicplants(Zanetti et al.,2005.In contrast to micro-pipetting,LCM,and sorting of protoplasts,which all can be used to isolate total cellular RNA,polysomeimmunopurification can be used to isolat e transcripts that are associated with ribosomes(translatome.Dis-crepancies between total RNA levels and representa-tion translatome can reveal regulation at the level of translation(Mustroph et al.,2009.In the future,trans-latome datasets,which bridge transcriptomics and proteomics,can help to interpret unusual transcript-to-protein ratios(see below.Alternatively,it is possible to identify cell-type-specific transcripts and proteins by comparing wild-type plants with mutants that lack specific cells or tiss ue types.In Arabidopsis,for instance,a series of homeotic mutants that lack variousfloral organs was used to identify several hundreds offloral organ-specific genes(Wellmer et al.,2004.If no appropriate mutants exist,specific cell types can be genetically abla ted by expression of acell-autonomous toxin,such as diphtheria toxin subunit A or RNase,under the control of cell-type-specific promoters.Again,these approaches have been proven to work for transcript profiling(Tung et al.,2005but it remains to be tested wh ether they could be useful for protein profiling.DECREASING COMPLEXITY BY ORGANIZING ORGAN AND SUBCELLULAR PROTEOMES Systematic analysis of accurate protein localization is essential to understand cellular networks in the context of compartmentalization,which is a funda-mental design principle of eukaryotic anelle proteomics has therefore become a very active re-searchfield.Until recently,the protein inventory of cell organelles was based on proteins from isolated organ-elles,such as mitochondria,chloroplast,and peroxi-somes(Lilley and Dupree,2007;Baginsky,2009.This approach has limitations because true low-abundant organelle proteins often cannot be distinguished from contaminating proteins.Two approaches have been used to deal with this problem.First,a recently reported isolation procedure for mitochondria used the electrostatic characteristics of the mitochondrial surface to separate mitochondria from other organelles in an electricfield.This procedure results in mito-chondria preparations with higher purity,but the yield is low(Eubel et al.,2007.Second,information about the quantitative distribution of proteins along density gradients has been used to determine if a protein was enriched by the organellar isolation procedure.In practice,the abundance distribution profile of un-known proteins is compared to known organelle marker proteins.This strategy is referred to as protein correlation profiling(Foster et al.,2006or LOPIT (Dunkley et al.,2006.Baginsky et al.Gene Expression Analysis, Proteomics, and Network Discovery Both procedures, however, are of limited use for the analysis of proteome dynamics in response to a stimulus because the long time that is needed to isolate and purify organelles affects their proteome properties. This is especially critical for transient posttranslational proteinmodifications. Thus, proteome dynamics is best analyzed at the cell or tissue level, followed by sorting of proteins into their respective organelle a posteriori. This strategy is now possible because substantial information about the protein complement of different cell organelles has accumulated (a comprehensive collection of proteome databases is for example available in Lu and Last, 2009. The SUBA database is most suitable for this purpose, because it is frequently updated and well maintained. SUBA generates lists of organelle proteins using reliability criteria, for example evidence from several different proteomics studies, targeting prediction, or GFP-localization assays, or a combination of this information (Heazlewood et al., 2007. For the chloroplast, two proteome reference tables have been established (Yu et al., 2008; Reiland et al., 2009. The overlap between these two proteome reference tables has generated a list of 1,156 proteins that can be considered high-confidence chloroplast proteins. Although the number of organelle proteins is constantly increasing, it is not clear when an organelle proteome can be considered complete. Organelle proteomes are dynamic and functional organelle proteomes differ sign ificantly during development, in different cell types or tissues, and in different conditions. This problem can be addressed by considering organelles as cellular subnetworks and applying fluxbalance modeling to assess network consistency. Initial modeling approaches with mitochondria and chloroplasts focused on a limited number of reactions, such as those of the Calvin cycle, amino acid biosynthesis, or the tricarboxylic acid cycle. Also, mitochondrial network reconstructions based on proteomics data are available and the existing models allow prediction of metabolite accumulation for a limited number of metabolites (Vo and Palsson, 2007. A recent flux-balance model of the primary metabolism in Chlamydomonas reinhardtii localized reactions into chloroplasts, mitochondria, and the cytosol and assessed systematically the contribution of different organelles to biomass production (Boyle and Morgan, 2009. The above examples illustrate the excellent suitability of metabolic network reconstruction to identify gaps in existing knowledge. different levels, a comparison between transcript and protein accumulation can provide information about the rate of protein translation and thedegree of posttranscriptional regulation. We have recently analyzed the correlation between protein and transcript abundance in representative samples from different plant organs and found mostly positive correlations in the range from 0.5 to 0.68 (Baerenfaller et al., 2008. The lowest correlation was observed for seeds, which accumulate stable storage proteins whose abundance is largely uncoupled from transcription. The highest correlation was obtained in leaves, suggesting that the most abundant photosynthetic proteins are predominantly regulated at the transcriptional level. It is clear that such a genome-scale analysis only offers a global view of regulatory events and does not allow a systematic assessment of individual enzyme regulation. A more refined comparison of protein and transcript levels showed that the correlation between transcript and protein abundance can vary significantly between different pathways (Kleffmann et al., 2004 and most likely also between different enzymes in the same pathway. Figure 2 shows an example of a correlation analysis of a representative leaf transcriptome and proteome for a selection of 345 genes/proteins from primary and secondary metabolism pathways. Although the data was collected from various sources and summarized (see also Baerenfaller et al., 2008, the protein-to-transcript ratio was similar for most proteins, indicating that this analysis is robust. The ma- THE CHALLENGE OF DATA INTEGRATION: GENOME-SCALE ANALYSIS OF RNA-PROTEIN CORRELATIONS Quantitative information about protein accumulation at genome scale offers entirely new insights into network function and the behavior of organs, tissues, and cells. Because gene expression is regulated at Plant Physiol. Vol. 152, 2010 Figure 2. Correlation analysis of transcript and protein abundance in Arabidopsis leaves based on 345 genes from various primary and secondary metabolism pathways. Transcript abundance was calculated as a representative expression vector derived from multiple Affymetrix ATH1 array measurements from leaf samples (data from Genevestigator, Hruz et al., 2008. The proteome data was obtained from distinct leaf samples. Approximately 20% of these genes/proteins had ratios of protein to transcript abundance deviating strongly from 1. 407。