指纹识别毕业设计外文翻译
基于单片机的指纹识别英文资料和中文翻译
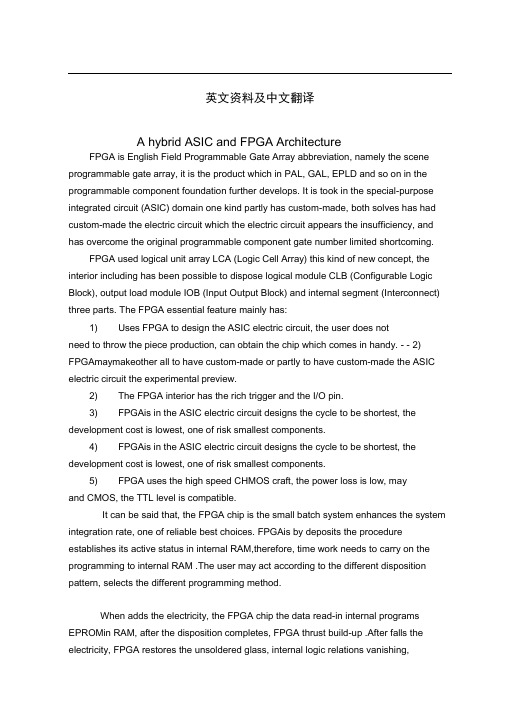
英文资料及中文翻译A hybrid ASIC and FPGA ArchitectureFPGA is English Field Programmable Gate Array abbreviation, namely the scene programmable gate array, it is the product which in PAL, GAL, EPLD and so on in the programmable component foundation further develops. It is took in the special-purpose integrated circuit (ASIC) domain one kind partly has custom-made, both solves has had custom-made the electric circuit which the electric circuit appears the insufficiency, and has overcome the original programmable component gate number limited shortcoming.FPGA used logical unit array LCA (Logic Cell Array) this kind of new concept, the interior including has been possible to dispose logical module CLB (Configurable Logic Block), output load module IOB (Input Output Block) and internal segment (Interconnect) three parts. The FPGA essential feature mainly has:1) Uses FPGA to design the ASIC electric circuit, the user does notneed to throw the piece production, can obtain the chip which comes in handy. - - 2) FPGAmaymakeother all to have custom-made or partly to have custom-made the ASIC electric circuit the experimental preview.2) The FPGA interior has the rich trigger and the I/O pin.3) FPGAis in the ASIC electric circuit designs the cycle to be shortest, the development cost is lowest, one of risk smallest components.4) FPGAis in the ASIC electric circuit designs the cycle to be shortest, the development cost is lowest, one of risk smallest components.5) FPGA uses the high speed CHMOS craft, the power loss is low, mayand CMOS, the TTL level is compatible.It can be said that, the FPGA chip is the small batch system enhances the system integration rate, one of reliable best choices. FPGAis by deposits the procedure establishes its active status in internal RAM,therefore, time work needs to carry on the programming to internal RAM .The user may act according to the different disposition pattern, selects the different programming method.When adds the electricity, the FPGA chip the data read-in internal programs EPROMin RAM, after the disposition completes, FPGA thrust build-up .After falls the electricity, FPGA restores the unsoldered glass, internal logic relations vanishing,therefore, FPGA can use repeatedly. The FPGAprogramming does not need the special-purpose FPGAprogrammer, only must use general EPROM, the PROM programmer then. When needs to revise the FPGA function, only must trade piece of EPROM then. Thus, identical piece FPGA, the different programming data, may have the different electric circuit function. Therefore the FPGA use is extremely flexible. FPGA has many kinds of disposition pattern: Parallel principal-mode -like is piece of FPGA adds piece of EPROM the way; The host may support piece of PROM from the pattern to program multi-piece FPGA;The serial pattern mayuse serial PROMto program FPGA;The peripheral pattern mayFPGAtake the microprocessor the peripheral, programs by the microprocessor to it.In the electrical observation and control system, needs to gather each kind of simulation quantity signal, the digital quantity signal frequently, and carries on corresponding processing to them. In the ordinary circumstances, in the observation and control system with ordinary MCU (for example 51, 196and so on monolithic integrated circuits or control DSP) is may complete thesystem task. 。
单片机的指纹识别电子密码锁设计:英文资料和中文翻译---论文篇
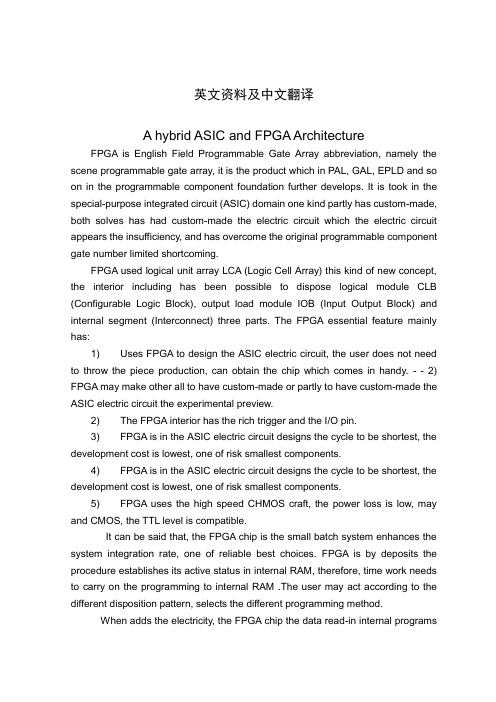
英文资料及中文翻译A hybrid ASIC and FPGA ArchitectureFPGA is English Field Programmable Gate Array abbreviation, namely the scene programmable gate array, it is the product which in PAL, GAL, EPLD and so on in the programmable component foundation further develops. It is took in the special-purpose integrated circuit (ASIC) domain one kind partly has custom-made, both solves has had custom-made the electric circuit which the electric circuit appears the insufficiency, and has overcome the original programmable component gate number limited shortcoming.FPGA used logical unit array LCA (Logic Cell Array) this kind of new concept, the interior including has been possible to dispose logical module CLB (Configurable Logic Block), output load module IOB (Input Output Block) and internal segment (Interconnect) three parts. The FPGA essential feature mainly has:1) Uses FPGA to design the ASIC electric circuit, the user does not need to throw the piece production, can obtain the chip which comes in handy. - - 2) FPGA may make other all to have custom-made or partly to have custom-made the ASIC electric circuit the experimental preview.2) The FPGA interior has the rich trigger and the I/O pin.3) FPGA is in the ASIC electric circuit designs the cycle to be shortest, the development cost is lowest, one of risk smallest components.4) FPGA is in the ASIC electric circuit designs the cycle to be shortest, the development cost is lowest, one of risk smallest components.5) FPGA uses the high speed CHMOS craft, the power loss is low, may and CMOS, the TTL level is compatible.It can be said that, the FPGA chip is the small batch system enhances the system integration rate, one of reliable best choices. FPGA is by deposits the procedure establishes its active status in internal RAM, therefore, time work needs to carry on the programming to internal RAM .The user may act according to the different disposition pattern, selects the different programming method.When adds the electricity, the FPGA chip the data read-in internal programsEPROM in RAM, after the disposition completes, FPGA thrust build-up .After falls the electricity, FPGA restores the unsoldered glass, internal logic relations vanishing, therefore, FPGA can use repeatedly. The FPGA programming does not need the special-purpose FPGA programmer, only must use general EPROM, the PROM programmer then. When needs to revise the FPGA function, only must trade piece of EPROM then. Thus, identical piece FPGA, the different programming data, may have the different electric circuit function. Therefore the FPGA use is extremely flexible. FPGA has many kinds of disposition pattern: Parallel principal-mode -like is piece of FPGA adds piece of EPROM the way; The host may support piece of PROM from the pattern to program multi-piece FPGA; The serial pattern may use serial PROM to program FPGA; The peripheral pattern may FPGA take the microprocessor the peripheral, programs by the microprocessor to it.In the electrical observation and control system, needs to gather each kind of simulation quantity signal, the digital quantity signal frequently, and carries on corresponding processing to them. In the ordinary circumstances, in the observation and control system with ordinary MCU (for example 51, 196 and so on monolithic integrated circuits or control DSP) is may complete the system task.。
fingerprintidentification(指纹识别)
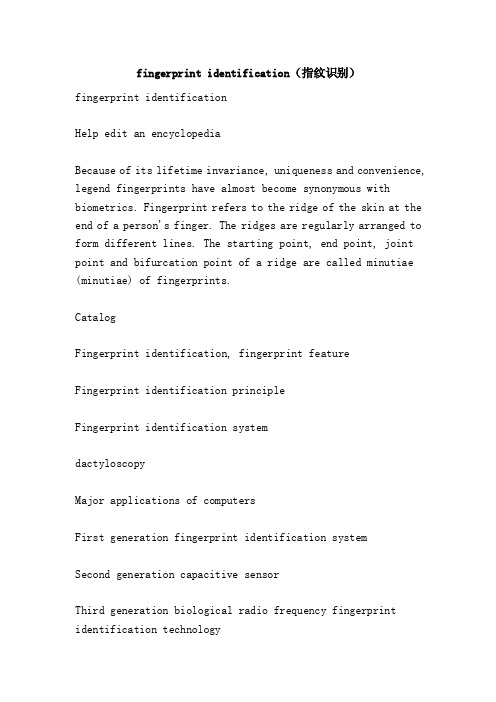
fingerprint identification(指纹识别)fingerprint identificationHelp edit an encyclopediaBecause of its lifetime invariance, uniqueness and convenience, legend fingerprints have almost become synonymous with biometrics. Fingerprint refers to the ridge of the skin at the end of a person's finger. The ridges are regularly arranged to form different lines. The starting point, end point, joint point and bifurcation point of a ridge are called minutiae (minutiae) of fingerprints.CatalogFingerprint identification, fingerprint featureFingerprint identification principleFingerprint identification systemdactyloscopyMajor applications of computersFirst generation fingerprint identification systemSecond generation capacitive sensorThird generation biological radio frequency fingerprint identification technologyAlgorithm overview, fingerprint identification, fingerprint featuresFingerprint identification principleFingerprint identification systemdactyloscopyMajor applications of computersFirst generation fingerprint identification systemSecond generation capacitive sensorThird generation biological radio frequency fingerprint identification technologyAlgorithm overviewEdit this section of fingerprint identification profilesFingerprint characteristicsEnglish Name: (fingerprinting)Fingerprint identification principleFingerprint identification refers to the identification of minutiae by comparing the minutiae of different fingerprints.Because each person's fingerprints are different, that is, between the ten fingers of the same person, there is also a significant difference between fingerprints, so fingerprints can be used for identification. In fact, in ancient China, early use of fingerprint (fingerprint) to sign. In 1684, Grew, the botanical engineer, published his first scientific paper on fingerprints. Fingerprint identification principleIn 1809, Bewick used his fingerprints as a trademark. In 1823, Purkije dissected the fingerprints into nine categories. In 1880, Faulds in nature called for fingerprints to be used to identify criminals. In 1891, Galton proposed the famous Galton classification system. After that, the British, American, German and other police departments have adopted fingerprint identification as the main method of identification. Along with the development of computer and information technology, FBI and Paris police station began to research and develop automatic fingerprint identification system (AFIS) for criminal case detection in 60s. At present, the automatic identification system has been widely used in police stations all over the world. In 90s, automatic fingerprint identification system for personal identification was developed and applied. Because each stamp of the range is not exactly the same, focus on the different will lead to different degree of deformation, and there are a lot of fuzzy fingerprint, feature extraction and how to realize the correct matching, fingerprint recognition technology is the key. Fingerprint identification technology involves many fields, such as image processing, pattern recognition, machine learning, computer vision, mathematical morphology and wavelet analysis.Edit this section of fingerprint identification systemPreprocessing system of fingerprint identificationFingerprint identification system is a typical pattern recognition system, including fingerprint image acquisition, processing, feature extraction and peer to peer module. Fingerprint image acquisition: through a special fingerprint acquisition instrument, you can collect living fingerprint images. At present, fingerprint acquisition instruments mainly include living optics, capacitive and pressure-sensitive devices. For the technical indicators such as resolution and acquisition area, the public security industry has formed international and domestic standards, but other standards are still lacking. According to the fingerprint area can be divided into rolling press fingerprint and plane fingerprints, public security industry commonly used rolling press fingerprint. In addition, fingerprint images can be obtained by scanners, digital cameras, and so on. Fingerprint image compression: large capacity fingerprint database must be compressed and stored to reduce storage space. The main methods include JPEG, WSQ, EZW and so on. Fingerprint image processing includes fingerprint area detection, image quality judgment, direction and frequency estimation, image enhancement, two value reduction and thinning of fingerprint images.Edit this section of fingerprint classificationFingerprint identification securityThe pattern is the basic classification of fingerprints, whichis divided into the basic pattern of the center pattern and the triangle. Of or relating to the shape of a central line. In our country, the ten fingerprint analysis divides the fingerprint into three major types and nine forms.In general, the automatic fingerprint identification system divides the fingerprint into arcuate (arc, account, shape), skip (left, right, left), bucket and miscellaneous patterns. Extraction form and detail features of fingerprint: fingerprint features including Center (upper and lower) and delta (left and right), the details of fingerprint feature points including the starting point and end point, combining ridge point and bifurcation point. Fingerprint matching: it can be coarsely matched according to the shape of the fingerprint, and then use the fingerprint shape and minutiae to make the exact matching, and give the similarity score of the two fingerprints. According to the different applications, the similarity score of the fingerprint is sorted or given whether the result of the same fingerprint is same.Edit the main application of this paragraphFingerprint recognition keyboardApplications in computersIn today's computer applications, including many very confidential file protection, most of them use the "user ID+ password" approach to user authentication and access control. However, if the password is forgotten or stolen by others, the security problems of the computer system and files arethreatened. With the development of science and technology, fingerprint identification technology has gradually begun to enter the computer world. At present, many companies and research institutions in the field of fingerprint recognition technology achieved great progress breakthrough, many application products introduced the combination of fingerprint identification and traditional IT technology perfect, these products have been recognized by more and more users. Fingerprint identification technology is applied in the business field of the relatively high security requirements, and a well-known brand in the business field of mobile office building Fujitsu, Samsung and IBM and other international has a fingerprint recognition system technology and application of mature, the following brief introduction on the application of fingerprint identification system in the notebook computer.First generation fingerprint identification systemAs we all know, two years ago, some brands of notebooks used fingerprint identification technology for user login identification, the first generation of optical fingerprint readerHowever, the fingerprint system introduced at the time belonged to the optical identification system, which should belong to the first generation of fingerprint identification technology. Optical fingerprint recognition system because light can not penetrate the surface of the skin (dead skin layer), so can only scan the surface of finger skin, or scan the dead skin layer, but not deep dermis. In this case, the cleanliness of the finger surface directly affects the recognition effect. If the user'sfinger is stuck with more dust, there may be an identification error. And, if people follow a finger, a fingerprint fingerprint recognition system, it may also be, for users, it is not very safe and stable.Second generation capacitive sensorLater, the second generation of capacitive sensors, capacitive sensor technology is the use of alternating command and arrangement and sensor panels, alternating plate of the second generation capacitive sensorsThe forms are two capacitive plates, and the fingerprint valleys and ridges become dielectric between plates. The dielectric constant sensor to detect changes between the generated fingerprint image. But because the sensor surface is the use of silicon material is easy to damage lead to loss of life, and it is through fingerprint valleys and ridges to form a concave convex fingerprint image so the dirty fingers wet fingers, fingers difficult low recognition rate.Third generation biological radio frequency fingerprint identification technologyTo today, the third generation of RF biological fingerprint recognition technology (radio frequency fingerprint core technology principle leather (line collector)), RF sensor technology is through the sensor itself emits trace RF signal to penetrate the epidermal layer, finger control layer texture measurement, to obtain the best fingerprint image. Therefore, on the dry finger, the Han third generation biological RFsensorsDry fingers, fingers and other difficulties through the fingers can be as high as 99@%, fingerprint anti-counterfeiting ability, fingerprint recognition principle of sensor only to human dermal skin reaction, fundamentally eliminate the artificial fingerprint, wide temperature range: especially suitable for cold or hot special area.Radio frequency technology is the most reliable and powerful solution because RF sensors produce high quality images. In addition, the high quality image also allows the reduction of the sensor, no need to sacrifice reliability certification, thereby reducing the cost and makes the application of RF sensor thought to any field of mobile and unfettered in size.Edit this section algorithm overviewAs people usually put in fingerprint into dustpan, bucket type, in the research of automatic fingerprint recognition, fingerprint is divided into five types: arch, left and right ring ring, pointed arch, vortex type (i.e. "bucket"). The main purpose of fingerprint classification is to facilitate the management of large capacity fingerprint libraries, and to reduce the search space and speed up the fingerprint matching process. Fingerprint classification is based on the overall flow direction of fingerprint ridges or valleys, and the core point of fingerprints. Many researchers have tried to solve the problem of fingerprint classification, but the classification algorithm still has a higher recognition rate. How to improve the accuracy of fingerprint classification is a key problem inautomatic fingerprint identification research.。
指纹识别技术的研究与设计--指纹图像预处理之二 计算机科学与技术专业毕业设计 毕业论文
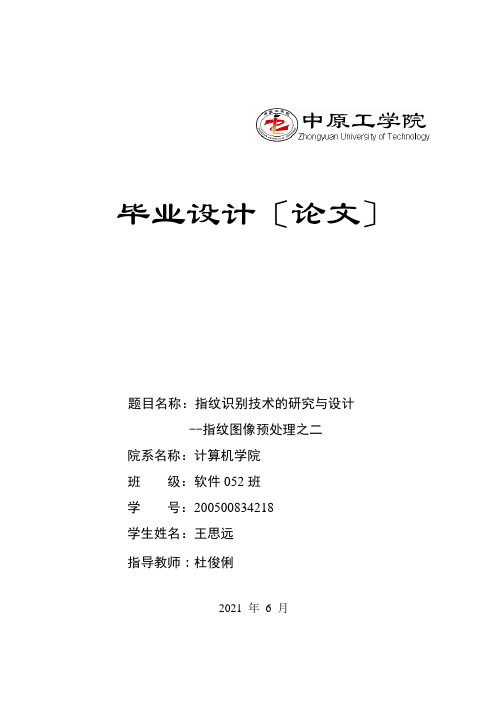
毕业设计〔论文〕题目名称:指纹识别技术的研究与设计--指纹图像预处理之二院系名称:计算机学院班级:软件052班学号:200500834218学生姓名:王思远指导教师:杜俊俐2021 年6 月指纹识别技术的研究与设计--指纹图像预处理之二The Fingerprint Recognition Technology's Research and Designs---Second fingerprint image pretreatment院系名称:计算机学院班级:软件052班学号:200500834218学生姓名:王思远指导教师:杜俊俐2021 年6 月摘要指纹识别作为一种生物鉴定技术,为人类的个体的定义提供了一个到目前为止最为快捷和可信的方法。
随着指纹识别的普及,人们之间的信任本钱将大大降低,提高人类社会活动的效率。
对于想从事和已经从事网络商务的公司来讲,确认交易人的身份是解决信用问题的第一步,而且是最重要的一步。
在信息时代,一种平安便捷的身份认证方式显得越发重要。
“在网络上,没有人知道你是一只狗,〞在这种情况下,任何基于网络环境下的交往活动都被蒙上了一层技术意义的灰色。
不可防止,所有基于这种网络技术根底之上的经济活动也因此被深深打上了不真实和不被信任的烙印。
随着科技的进步,指纹识别技术已经开始慢慢进入计算机世界中。
目前许多公司和研究机构都在指纹识别技术领域取得了很大突破性进展,推出许多指纹识别与传统IT技术完美结合的应用产品,这些产品已经被越来越多的用户所认可。
对指纹图片进行识别前的预处理工作,希望不吝指正。
关键词:指纹、图像、预处理、指纹识别技术。
AbstractThe fingerprint recognition takes one bioassay technology, has provided for humanity's individual's definition so far most quickly and the credible method.Along with fingerprint recognition popularization, between people's trust cost will reduce greatly, raises the efficiency which the human society moves. Regarding wanted to be engaged in with already was engaged in the network commerce the company saying that the seal bargain person's status was solves the credit problem first step, moreover was most important one step. In the information age, one security convenient status authentication way appears even more important. “in the network, nobody knew that you are a dog,〞in this case, any based on network environment under contact by Mongolia in a technical significance gray. Inevitable, possessed the economic activity therefore deeply has also been gotten based on this kind of networking foundation above not real and not the brand mark which trusted.Along with the technical progress, the fingerprint recognition technology already started to enter in slowly the computer world. At present many companies and the development facility have made the very big breakthrough progress in the fingerprint recognition area of technology, promotes many fingerprint recognitions and the traditional IT technology perfect union application product, these products were already approved by more and more users.Before this system uses Visual the C++ 6.0 for fingerprint pictures to carry on the recognition the pretreatment work, hoped that the liberal with points out mistakes.Key word: Image, pretreatment, fingerprint recognition technology.目录摘要 (I)Abstract............................................................................................................ I I 第1章指纹识别概述 (5)1.1 指纹识别技术 (5)1.1.1 指纹识别的简介 (5)1.1.2 指纹识别的关键技术 (5)1.1.3 指纹识别的研究历程 (5)1.2 预处理技术 (6)指纹识别技术的应用 (6)指纹识别的可靠性 (7)第2章指纹识别系统的需求分析 (9)2.1 指纹识别系统的目标 (9)2.2 指纹识别系统的功能需求 (9)2.2.1 指纹图象的点运算 (9)2.2.2 指纹图像的几何变换 (9)2.2.3 指纹图像的图像增强 (9)2.2.4 指纹图像的形态学变换 (9)2.2.5 指纹图像的图像分割 (10)2.2.6 查看直方图 (10)2.3 指纹识别系统的性能需求 (10)2.3.1 运行平台的低要求 (10)2.3.2 系统的实时性 (10)2.3.3 系统的有效性 (10)2.4 指纹识别系统的结构 (10)2.4.1 指纹识别系统框架图 (10)2.4.2 指纹识别系统模块功能划分 (12)指纹识别模块 (12)2.5 开发工具 (12)第3章指纹识别系统中预处理的设计与实现 (13)3.1 预处理功能模块的设计 (13)3.2 预处理模块的详细设计 (15)3.2.1 点运算模块 (15)3.2.2 几何变换模块 (18)3.2.3 图像增强模块 (22)3.2.4 形态学变换模块 (25)3.2.5 图像分析模块 (26)3.2.6 查看模块 (28)3.3 预处理实验结果与分析 (30)3.3.1 预处理实验结果 (30)3.3.2 预处理结果的分析 (38)第4章系统工程目录说明 (39)4.1 系统工程目录 (39)4.2 系统主要的类 (40)4.2.1 CDlgIntensity类的成员函数与成员数据 (40)4.2.2 CDlgLinerPara类的成员函数与成员数据 (41)4.2.3 CDlgMidFilter类的成员函数与成员数据 (43)4.2.4 CDlgPointStre类的成员函数与成员数据 (44)4.2.5 CDlgPointThre类的成员函数与成员数据 (46)4.2.6 CDlgPointWin类的成员函数与成员数据 (48)4.2.7 CDlgSmooth类的成员函数与成员数据 (49)第5章系统的调试、运行与测试 (52)5.1 程序设计中出现的问题与解决方法 (52)5.1.1 点运算 (52)5.1.2 几何变换 (54)5.1.3 图像增强 (56)5.1.4 形态学变换和图像分析 (57)5.2 系统测试局部结果显示 (59)5.2.1 图像信息载入出错显示: (59)5.2.2 功能处理过程中出错提示: (59)5.2.3 提示用户保存信息显示: (59)5.2.4 主界面显示: (59)第6章结论 (61)参考文献 (62)致谢 (63)附录 (64)第1章指纹识别概述1.1 指纹识别技术指纹识别的简介指纹识别技术通过分析指纹的局部特征,从中抽取详尽的特征点,从而可靠地确认个人身份。
指纹识别系统本科毕业论文
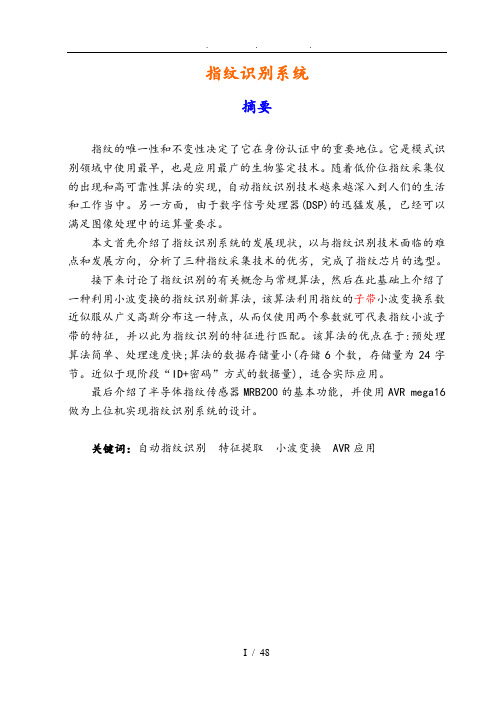
指纹识别系统摘要指纹的唯一性和不变性决定了它在身份认证中的重要地位。
它是模式识别领域中使用最早,也是应用最广的生物鉴定技术。
随着低价位指纹采集仪的出现和高可靠性算法的实现,自动指纹识别技术越来越深入到人们的生活和工作当中。
另一方面,由于数字信号处理器(DSP)的迅猛发展,已经可以满足图像处理中的运算量要求。
本文首先介绍了指纹识别系统的发展现状,以与指纹识别技术面临的难点和发展方向,分析了三种指纹采集技术的优劣,完成了指纹芯片的选型。
接下来讨论了指纹识别的有关概念与常规算法,然后在此基础上介绍了一种利用小波变换的指纹识别新算法,该算法利用指纹的子带小波变换系数近似服从广义高斯分布这一特点,从而仅使用两个参数就可代表指纹小波子带的特征,并以此为指纹识别的特征进行匹配。
该算法的优点在于:预处理算法简单、处理速度快;算法的数据存储量小(存储6个数,存储量为24字节。
近似于现阶段“ID+密码”方式的数据量),适合实际应用。
最后介绍了半导体指纹传感器MRB200的基本功能,并使用AVR mega16做为上位机实现指纹识别系统的设计。
关键词:自动指纹识别特征提取小波变换 AVR应用Fingerprint Identification SystemAbstractFingerprint has important state in identity verification for its exclusivity and invariability. Along with the emergence of the low cost fingerprint sensor and the realization of the highly reliable algorithm, the automated fingerprint identification technique is applied more and more in our life and work. On the other hand, because of the fast development of the digital signal processor (DSP), the DSP already can satisfy the request of abounding computing in image processing.First, this paper introduces the developing actuality, difficulties and direction on fingerprint identification technology, and analyses the advantages and disadvantages of the technology of fingerprint capturing; and at last selects the fingerprint sensor.So then, This paper discusses the relevant concept and the traditional algorithm of fingerprint verification, then put forward a new fingerprint verification algorithm based on wavelet transformation. This algorithm is on the base of the character that the wavelet subband coefficient approximately obedient to the generalized Gaussian density. So two parameters can represent the wavelet subband and can be used to match fingerprints as fingerprint's character. The algorithm's advangtage is simplity of pre-processing algorithm, fast processing speed; small data memory needed by the algorithm (need to save 6 data, 24 byte. Be close to the capacity of `ID+password'), which suit real application.Finally,this article introduces basic functions of the semiconductor fingerprint sensor MBF200, and uses AVR mega16 as upper machine to realize the design of fingerprint identification system.Key words:Automated fingerprint identification, Feature extraction, Wavelet transformation, AVR application目录第一章绪论11.1 引言11.2 指纹识别技术的研究背景21.3 指纹识别原理41.4 指纹识别技术面临的难点以与发展方向51.5 指纹采集技术比较71.6 特征提取与匹配91.7 性能评价91.8 本文所做工作与论文结构11第二章指纹识别算法122.1 指纹识别的基本知识122.1.1 总体特征122.1.2 局部特征132.2 指纹的特征提取与匹配算法142.2.1基于特征点的特征提取与匹配算法152.2.2 基于指纹纹理特性的新算法162.3 指纹奇异点检测算法222.3.1 指纹主向图的求取232.3.2 指纹奇异点的提取26第三章指纹识别系统的硬件设计293.1 MRB200指纹模块简介293.1.1 系统特点293.1.2 接口定义303.1.3 安装连接313.1.4 命令格式313.2 ATmega16 单片机介绍323.3 指纹识别系统的硬件设计333.4 指纹识别系统的软件设计39第四章总结与展望404.1 总结404.2 展望41参考文献42致43第一章绪论1.1 引言随着网络时代的来临,人们对于安全性的要求越来越高,如何准确的鉴定个人身份,保护信息安全是当今信息化时代必须解决的一个关键性社会问题。
一个利用模糊匹配算法的指纹识别系统(外文文献翻译)
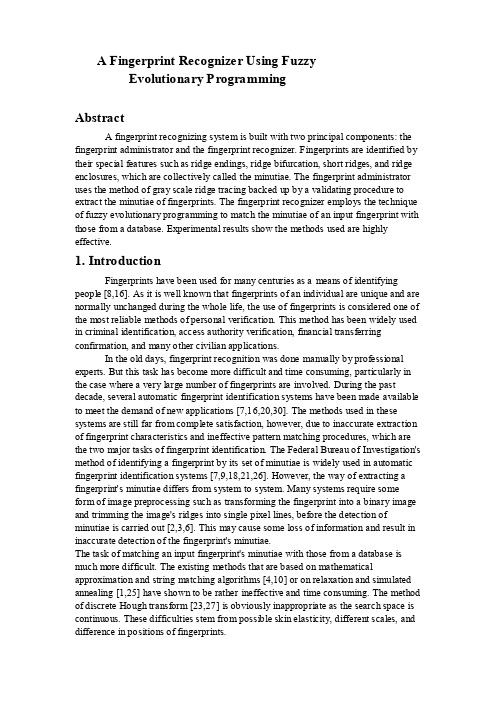
A Fingerprint Recognizer Using FuzzyEvolutionary ProgrammingAbstractA fingerprint recognizing system is built with two principal components: the fingerprint administrator and the fingerprint recognizer. Fingerprints are identified by their special features such as ridge endings, ridge bifurcation, short ridges, and ridge enclosures, which are collectively called the minutiae. The fingerprint administrator uses the method of gray scale ridge tracing backed up by a validating procedure to extract the minutiae of fingerprints. The fingerprint recognizer employs the technique of fuzzy evolutionary programming to match the minutiae of an input fingerprint with those from a database. Experimental results show the methods used are highly effective.1. IntroductionFingerprints have been used for many centuries as a means of identifying people [8,16]. As it is well known that fingerprints of an individual are unique and are normally unchanged during the whole life, the use of fingerprints is considered one of the most reliable methods of personal verification. This method has been widely used in criminal identification, access authority verification, financial transferring confirmation, and many other civilian applications.In the old days, fingerprint recognition was done manually by professional experts. But this task has become more difficult and time consuming, particularly in the case where a very large number of fingerprints are involved. During the past decade, several automatic fingerprint identification systems have been made available to meet the demand of new applications [7,16,20,30]. The methods used in these systems are still far from complete satisfaction, however, due to inaccurate extraction of fingerprint characteristics and ineffective pattern matching procedures, which are the two major tasks of fingerprint identification. The Federal Bureau of Investigation's method of identifying a fingerprint by its set of minutiae is widely used in automatic fingerprint identification systems [7,9,18,21,26]. However, the way of extracting a fingerprint's minutiae differs from system to system. Many systems require some form of image preprocessing such as transforming the fingerprint into a binary image and trimming the image's ridges into single pixel lines, before the detection of minutiae is carried out [2,3,6]. This may cause some loss of information and result in inaccurate detection of the fingerprint's minutiae.The task of matching an input fingerprint's minutiae with those from a database is much more difficult. The existing methods that are based on mathematical approximation and string matching algorithms [4,10] or on relaxation and simulated annealing [1,25] have shown to be rather ineffective and time consuming. The method of discrete Hough transform [23,27] is obviously inappropriate as the search space is continuous. These difficulties stem from possible skin elasticity, different scales, and difference in positions of fingerprints.In this paper, we adopt the method of direct gray scale minutiae detection proposed in [5,15,17,29] improved by a backup validating procedure to eliminate false minutiae. As for minutiae matching, we employed the technique of fuzzy evolutionary programming, which has been used successfully in speaker identification [11], images clustering [12,13], and fuzzy algebraic operations [14]. Experimental results show this approach is also highly effective in fingerprint recognition. The paper is organized as follows. Section 2 discusses the characteristics of fingerprints that are commonly used to identify individuals. The method of extracting these fingerprint characteristics is described in Section 3. Section 4 presents the major component of our fingerprint recognizing system, which employs the technique of fuzzy evolutionary programming to identify a fingerprint. In Section 5, we present some experimental results that justify the methods used. The last section summarizes the paper and indicates some related future works.2. Fingerprint characteristicsA fingerprint is a textural image containing a large number of ridges that form groups of almost parallel curves (Figure 1). It has been established that fingerprint's ridges are individually unique and are unlikely to change during the whole life.Although the structure of ridges in a fingerprint is fairly complex, it is well known [7] that a fingerprint can be identified by its special features such as ridge endings, ridge bifurcation, short ridges, and ridge enclosures. These ridge features are collectively called the minutiae of the fingerprint. It is also reported in [7] that for automatic detection of a fingerprint, it suffices to focus on two types of minutiae, namely ridge endings and bifurcation. Figure 2 shows the forms of various minutiae of a fingerprint.A full fingerprint normally contains between 50 to 80 minutiae. A partial fingerprint may contain fewer than 20 minutiae. According to the Federal Bureau of Investigation [7], it suffices to identify a fingerprint by matching 12 minutiae, but it has been reported that in most cases 8 matched minutiae are enough.3. Minutiae extractionFor convenience, we represent a fingerprint image in reverse gray scale. That is, the dark pixels of the ridges are assigned high values where as the light pixels of the valleys are given low values. Figure 3 shows a section of ridges in this representation.In a fingerprint, each minutia is represented by its location (x, y) and the local ridge direction . Figure 4 shows the attributes of a fingerprint's minutia. The process of minutiae detection starts with finding a summit point on a ridge, then continues by tracing the ridge until a minutia, which can be either a ridge ending or bifurcation, is encountered. Details of these tasks are described in the following subsections.3.1 Finding a ridge summit pointTo find a summit point on a ridge, we start from a point x= (x1, x2) and compute the direction angle b y using the gradient method described in [5,17]. Then the vertical section orthogonal to the direction i s constructed (To suppress light noise, the section gray values are convoluted with a Gaussian weight function). The point in this section with maximum gray level is a summit pointon the nearest ridge.The direction angle a t a point x mentioned above is computed as follows. A 9 9 neighborhood around x is used to determine the trend of gray level change. At each pixel u = (u1, u2) in this neighborhood, a gradient vector v(u) = (v1(u), v2(u)) is obtained by applying the operator h = (h1, h2) withto the gray levels in a neighborhood of u. That is,where y runs over the eight neighboring pixels around u and g(y) is the gray level of pixel y in the image. The angle r epresents the direction of the unit vector t that is(almost) orthogonal to all gradient vectors v. That is, t is chosen so that is minimum.3.2 Tracing a ridgeThe task of tracing a ridge line to detect minutiae is described in the following algorithm. This algorithm also constructs a traced image of the fingerprint. Every time a new summit point of the ridge is found, its location in the traced image is assigned a high gray value and the surrounding pixels are given lower gray levels if they have not been marked.Algorithm 1 (Ridge tracing)Start from a summit point x of a ridge.RepeatCompute the direction angle ϕa t x;Move μp ixels from x along the direction ϕt o another point y;Find the next summit point z on the ridge, which is the local maximum of the section orthogonal to direction a t point y; Set x = z;Until point x is a termination point (i.e. a minutia or offvalid area).Determine if the termination point x is a valid minutia,if so record it.End Algorithm 1There are three criteria used to terminate tracing a ridge. The first stopping condition is that when the current point is out of the area of interest. That is, the current point is within 10 pixels from the border, as experiments show that there are rarely any minutiae close to the edges of the image. The second criterion determines a ridge ending: the section at the current point contains no pixels with gray levels above a pre-specified threshold. In this case, the previous point on the ridge is recorded as a ridge endpoint. The last stopping condition corresponds to the case of a possible bifurcation: the current point is detected to be on another ridge that has been marked on the traced image.Algorithm 1 is backed up by a checking procedure that determines if a termination point is a valid minutia. The procedure is expressed as follows. Algorithm 2 (Elimination of false minutiae)If the current ridge end is close to another ridge end with almost opposite direction, then delete both of them and join the gap, as they are simply broken ends of the same ridge.If the current bifurcation point is close to the end of one of its branch, then delete both of them, as short branch of a bifurcation is normally a result of light noise in the image.If the current termination is close to more than two other terminations, then delete all of them, as they are likely caused by damaged ridges in the image.The above algorithms form one major component of our fingerprint recognizing system, called the Fingerprint Administrator. Figure 7 depicts theuser-interface feature of the Fingerprint Administrator. The input fingerprint image is displayed in the left box, and the result of ridge tracing and detection of minutia is shown in a traced image in the right box. Observe that in the traced image,the ridge summits are shown in black color, their surrounding of five pixels is colored red, and the detected minutiae are marked with yellow tangent vectors. If the Save button is clicked, the coordinates of the detected minutiae and their associated direction angles are saved in a database in the form of linked list.The Fingerprint Administrator is used to extract minutiae of known fingerprints and store them in a database. It is also used to extract minutiae of an input fingerprint for the purpose of identification. Experimental results are reported in Section 5.4. Fingerprint recognitionThe primary purpose of our fingerprint recognizing system is to calculate the matching degree of the target fingerprint with the images in a database and to decide if it belongs to a particular individual. A fingerprint is said to match one image in the database if the degree of matching between its minutiae and that of the image in the database is higher than some pre-specified acceptance level. The method of calculating this matching degree is based on our fuzzy evolutionary programming technique, which is described below.Consider two fingerprints that are represented by their sets of minutiaewhere , for. Observe that the two sets may not have the same number of points, and that the order of thepoints in each set is possibly arbitrary. The principal task is to find a transformationthat transforms the set of minutiae P into the set Q. Here, srepresents a scaling factor, a n angle of rotation, and a translation in thexy-plane. Thus, the transform of a minutia is defined by:Also, in order to calculate the degree of matching, we associate with each minutia F(p) a fuzzy set (also denoted by F(p) for convenience) the membership function ofwhich is defined by:whereand represents a fuzzy subset of the real line defined as follows:Intuitively, we allow some degree of tolerance in matching the minutiae F(p) and q, but this tolerance decreases rapidly when the two minutiae are far apart. The matching degree between two sets F(P) and Q is defined as:The task of finding a transformation F to match two sets of minutiae P and Q consists of two phases. First the rotation angle i s estimated by the following algorithm.A lgorithm 3 (Estimation of rotation)Divide the interval [- π, π] into K subintervals,k = 1,…, K. ,Set up an integer array and a real array,and initialize them to 0. For each i = 1,…, m and each j = 1,…, n doFind an index k such that k ≤ - < k+1;Increment c[k] by 1 and increment [k] by ( - ).Find the index k* such that c[k*] is maximum.Let be defined byHaving established the rotation angle , the remaining parameters s and x, y of the transformation F are estimated by the following algorithm.Algorithm 4 (Fuzzy evolutionary programming)Generate a population ofwhere the parameter values are randomly taken from appropriate intervals.For each k = 1,…, m, compute the fitness:RepeatFor each k = 1,…, m, generate an offspringAlgorithms 3 and 4 form the principal component ofour system: the fingerprint recognizer. The fingerprintrecognizer receives an input fingerprint, calls thefingerprint administrator to extract the fingerprint'sminutiae, then tries to match this set of minutiae withthose in the database, until either a good match is found,or the database is exhausted. Experiments are discussed inthe next section.5. Experimental resultsA number of fingerprints of various types, including plain and tented arch, ulna and radial loop, plain whorl,central pocket whorl, double whorl, and accidental whorl,are fed to the system's fingerprint administrator for minutiae detection. The resulting traced images (as depicted in Figure 7) are manually inspected and the results are shown in Table 1.We note also that the fingerprints' ridges are accurately traced, broken ridges are effectively rejoined,and the short ridges and spur ridges are correctly adjusted.Manual checking confirms that most of those anomalies in the fingerprints are result of light noise, skin cut or distortion. The adjustment was realized by the system's process of eliminating false minutiae (Algorithm 2).A number of fingerprints are taken from the database and are modified by various rotations, resizing, and shifting, for use in testing experiments. We also add random light noise to the test images. The results of the fingerprint recognizer's performance are summarized in Table 2.6. Conclusion and future workWe have presented a fingerprint recognizing system that uses the method of gray scale ridge tracing backed up by a validating procedure to detect fingerprint's minutiae and that employs the technique of fuzzy evolutionary programming to match two sets of minutiae in order to identify a fingerprint. The experimental results show that the system is highly effective with relatively clean fingerprints. However, for poorly inked and badly damaged fingerprints, the system appears to be not so successful. In order to handle those bad types of fingerprints, we are working on the addition of a preprocessing component that also adopts the fuzzy evolutionary approach to reconstruct and enhance the fingerprints before they are processed by the system.Also, it is possible to connect the system with a live fingerprint scanner that obtains a person's fingerprint directly and sends it to the system for identification.These are the objectives of our future work.2、译文一个利用模糊匹配算法的指纹识别系统摘要一个指纹识别系统首先要包括两个部分:指纹管理程序和指纹识别器。
基于arm的指纹识别毕业设计
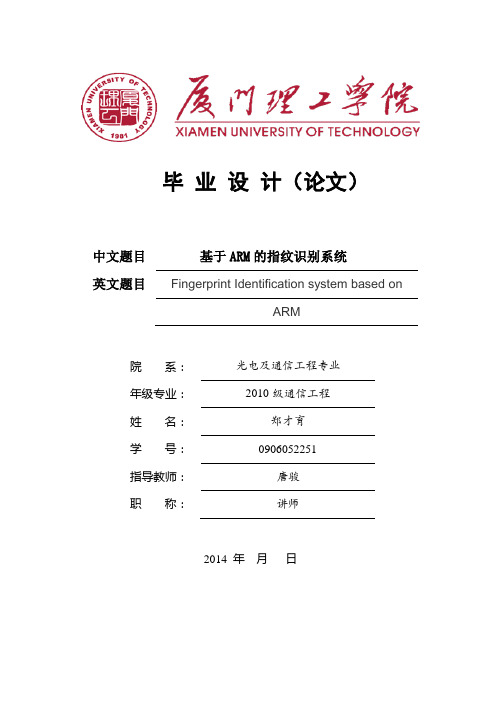
毕业设计(论文)中文题目基于ARM的指纹识别系统英文题目Fingerprint Identification system based onARM院系:光电及通信工程专业年级专业:2010级通信工程姓名:郑才育学号:0906052251指导教师:唐骏职称:讲师2014 年月日基于ARM的指纹识别系统毕业设计(论文)诚信声明书本人郑重声明:在毕业设计(论文)工作中严格遵守学校有关规定,恪守学术规范;我所提交的毕业设计(论文)是本人在唐骏指导教师的指导下独立研究、撰写的成果,设计(论文)中所引用他人的文字、研究成果,均已在设计(论文)中加以说明;在本人的毕业设计(论文)中未剽窃、抄袭他人的学术观点、思想和成果,未篡改实验数据。
本设计(论文)和资料若有不实之处,本人愿承担一切相关责任。
学生签名:年月日2基于ARM的指纹识别系统基于ARM的指纹识别系统设计摘要世界正朝着互联化的方向发展,而物联网正是这个数字革命的核心之一。
在目前流行的物联网技术中,要求嵌入式终端能够提供成熟且价格便宜的生物特征识别技术,目前来说指纹识别的技术应用最为广泛,我们不仅在门禁、考勤系统中可以看到指纹识别技术的身影,市场上有了更多指纹识别的应用:如手机、指纹锁、银行支付验证都可应用指纹识别的技术。
在指纹识别控制领域,也会用到各种微控制器,本文采用了三星半导体S3C6410作为控制核心,S3C6410应用了专为要求高性能、低成本、低功耗的嵌入式消费类电子设计的ARM11内核。
按性能分成两个不同的系列:该系列内核时钟频率已经达到532MHz。
指纹识别基于两种特征点来识别:(i)组成指纹的指纹整体特征结构(ii)局部的特征点。
本文提出了一种可以在自动指纹识别系统中使用的基于特征点的指纹识别算法。
本文提到的方法基于从细化提取的特征点,二值化一个指纹图像分割图。
该系统采用在指纹分类的指纹索引匹配,大大提高了匹配算法的性能。
关键字:ARM11,指纹识别,特征识别,图像处理3基于ARM的指纹识别系统AbstractThe world is moving in the direction of the development of the Internet, the Internet of is one of the core of the digital revolution. In the current network technology, the embedded terminal capable of providing biometric technology is mature and the price is cheap, at present technology of fingerprint recognition is the most widely, we can not only see the fingerprint recognition technology in access control, attendance system, fingerprint recognition application is more on the market: such as mobile phone, fingerprint lock, bank payment verification can be applied to fingerprint recognition technology.In the fingerprint recognition and control field, we will also use a variety of micro controller, this paper uses Samsung S3C6410 as the control core, S3C6410 application designed for high performance, low cost, low power embedded consumer electronic design based on ARM11 kernel. According to performance is divided into two different series: this series of core clock frequency has reached 532MHz.Fingerprint identification two feature points based on: (I) to identify the fingerprint feature structure fingerprint (II) feature local. This paper proposes a can be used in automatic fingerprint recognition system of fingerprint recognition algorithm based on feature points. The methods mentioned in this article is based on the feature points extracted from refined, two value segmentation image of a fingerprint image. The system adopts fingerprint indexing in the fingerprint classification matching, greatly improves the performance of matching algorithm.Keywords: ARM11,Fingerprint Identifier,Feature Point,Image Processing.4基于ARM的指纹识别系统目录第一章概述 (1)1.1指纹课题的研究与应用背景 (1)1.1.1指纹采集器原理与类型 (1)1.2 嵌入式系统概述 (2)1.3指纹算法概述 (4)1.3.1指纹预处理算法 (4)1.3.2指纹图像识别算法 (4)第二章系统设计方案 (5)2.1指纹识别系统的概述 (5)2.2指纹系统硬件设计方案 (5)2.2.1指纹采集器介绍 (6)第三章指纹预处理算法的设计 (8)3.1指纹图片畸变处理算法: (8)3.2指纹图像的分割计算: (10)3.3指纹均衡 (11)3.4指纹收敛处理 (13)3.5指纹的平滑处理: (14)3.6指纹的增强处理: (14)3.7指纹的二值化细化处理: (15)4纹识别算法的设计 (18)4.1指纹图像的特征 (18)4.2指纹图像特征提取算法 (19)4.3特征点的匹配算法 (20)5.指纹识别系统的实现 (22)5基于ARM的指纹识别系统5.1软件运行环境的设置 (22)5.2指纹采集器接口设计 (23)5.3显示及UI功能的实现 (26)第六章系统调试与测试 (27)6.1指纹算法的验证: (27)6.2指纹采集接口的调试部分 (28)6.3系统运行的测试: (29)参考文献 (29)6基于ARM的指纹识别系统第一章概述1.1指纹课题的研究与应用背景信息化的特点就是能够实现事物的数字化和隐秘化,传统的身份管理如IC卡,磁卡,钥匙存在着易丢失,伪造等缺点,相比较于传统的身份辨识,人的指纹永远无法改变,因此指纹识别在安防领域,司法领域扮演及其重要的作用,在民用领域,随着采集器的多样化和嵌入式微控制器的发展,指纹识别更多地走进了人们的生活中。
和指纹识别技术有关的英语作文
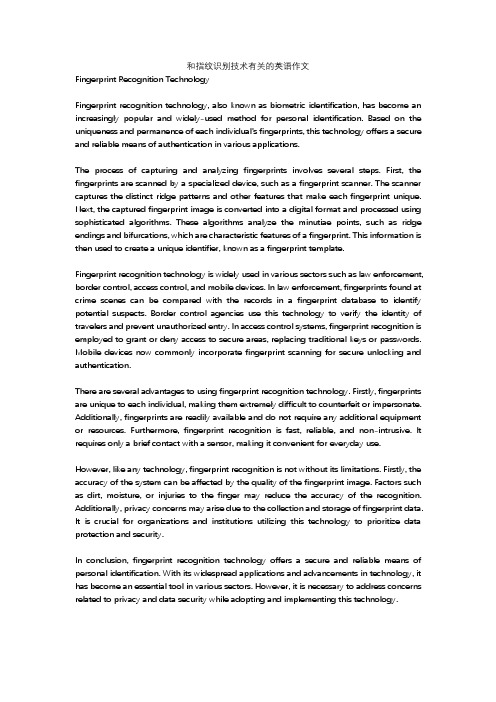
和指纹识别技术有关的英语作文Fingerprint Recognition TechnologyFingerprint recognition technology, also known as biometric identification, has become an increasingly popular and widely-used method for personal identification. Based on the uniqueness and permanence of each individual's fingerprints, this technology offers a secure and reliable means of authentication in various applications.The process of capturing and analyzing fingerprints involves several steps. First, the fingerprints are scanned by a specialized device, such as a fingerprint scanner. The scanner captures the distinct ridge patterns and other features that make each fingerprint unique. Next, the captured fingerprint image is converted into a digital format and processed using sophisticated algorithms. These algorithms analyze the minutiae points, such as ridge endings and bifurcations, which are characteristic features of a fingerprint. This information is then used to create a unique identifier, known as a fingerprint template.Fingerprint recognition technology is widely used in various sectors such as law enforcement, border control, access control, and mobile devices. In law enforcement, fingerprints found at crime scenes can be compared with the records in a fingerprint database to identify potential suspects. Border control agencies use this technology to verify the identity of travelers and prevent unauthorized entry. In access control systems, fingerprint recognition is employed to grant or deny access to secure areas, replacing traditional keys or passwords. Mobile devices now commonly incorporate fingerprint scanning for secure unlocking and authentication.There are several advantages to using fingerprint recognition technology. Firstly, fingerprints are unique to each individual, making them extremely difficult to counterfeit or impersonate. Additionally, fingerprints are readily available and do not require any additional equipment or resources. Furthermore, fingerprint recognition is fast, reliable, and non-intrusive. It requires only a brief contact with a sensor, making it convenient for everyday use.However, like any technology, fingerprint recognition is not without its limitations. Firstly, the accuracy of the system can be affected by the quality of the fingerprint image. Factors such as dirt, moisture, or injuries to the finger may reduce the accuracy of the recognition. Additionally, privacy concerns may arise due to the collection and storage of fingerprint data. It is crucial for organizations and institutions utilizing this technology to prioritize data protection and security.In conclusion, fingerprint recognition technology offers a secure and reliable means of personal identification. With its widespread applications and advancements in technology, it has become an essential tool in various sectors. However, it is necessary to address concerns related to privacy and data security while adopting and implementing this technology.。
计算机专业指纹识别操作系统毕业论文外文文献翻译及原文
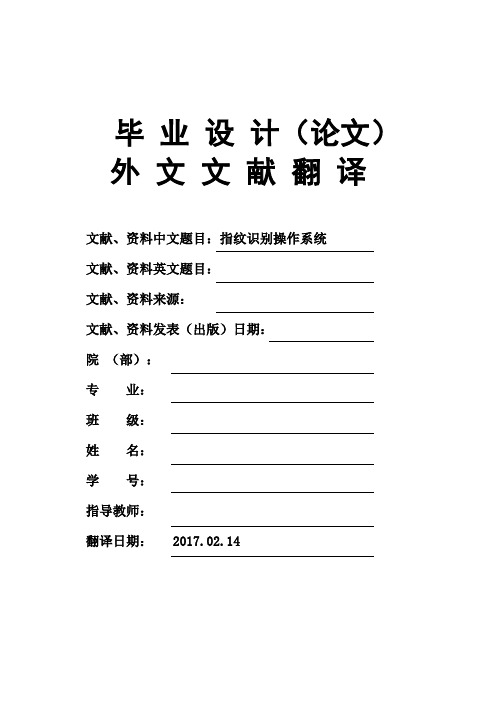
毕业设计(论文)外文文献翻译文献、资料中文题目:指纹识别操作系统文献、资料英文题目:文献、资料来源:文献、资料发表(出版)日期:院(部):专业:班级:姓名:学号:指导教师:翻译日期: 2017.02.14摘要:本文拟在提出一种可以区分protocol指纹识别的方法,用帧描述指纹识别代替建立帧系统获得主机信息与系统配对从而分辨出主机操作系统的类别。
实验的结果表明这种方法能够有效的辨别操作系统,这一方法比其他例如nmap 和xprobe的系统更为隐秘。
关键词:传输控制)协议/ 协议指纹识别操作系统辨别远程主机的操作系统,这是一个很重要的领域。
了解主机操作系统可以分析和获取一些信息,例如记忆管理,CPU的类型。
这些信息对于计算机网络的攻击与防御非常重要。
主要的辨别是通过TCP/IP指纹识别来完成的。
几乎所有的操作系统的定制他们自己的协议栈都通过以下的RFC。
这种情况导致一个实例,每个协议栈会有细节上的不同。
这些不同的细节就是所知道的使辨别操作系统称为可能的指纹识别。
Nmap、Queso在传输层里使用指纹。
他们将特殊数据包发送到目标并分析返回的数据包,在指纹库中寻找配对的指纹,以便得到的结果。
指纹库中的信息受指定的探测信息的影响.很难区分类似的操作系统(例如:windows98/2000/xp)Xprobe主要是利用ICMP协议,这是利用五种包来识别操作系统。
它能够提供的在所有可能的情况下确实是操作系统的概率。
主要不足是它过分依赖ICMP协议议定书。
SYNSCAN是在应用协议中与目标主机联系时,使用的一些典型的指纹识别方法。
指纹库对在这个领域有限制。
Ring,Ttbit查明操作系统所使用TCP / IP 的性能特点。
因为这种性能受网络环境极大。
其结果往往是不完全确定的。
文献分析资料中的行动而获得的拦截(如一些同步的要求,一个封闭的端口如何响应连接请求)。
虽然这种方式是有效,它在少数特定操作系统区分上述的各种系统,都没有完整的描述指纹系统,引起他们进行分辨的主要是依靠部分的TCP/IP。
外文翻译
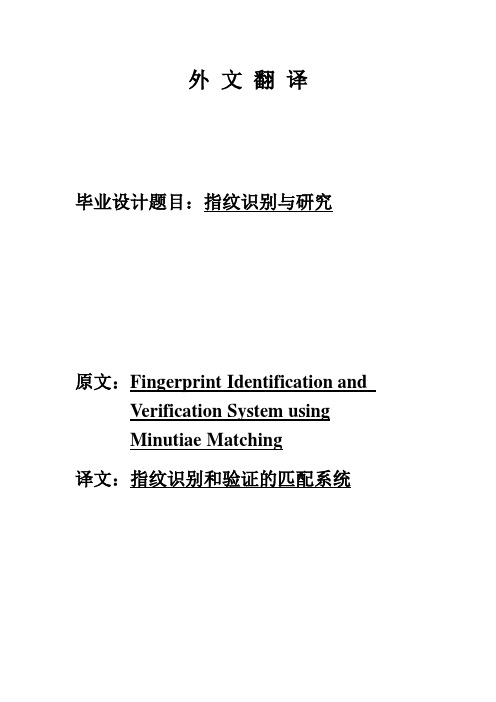
外文翻译毕业设计题目:指纹识别与研究原文:Fingerprint Identification and Verification System usingMinutiae Matching译文:指纹识别和验证的匹配系统原文:Fingerprint Identification and Verification System using MinutiaeMatchingAbstract: Fingerprints are the most widely used biometric feature for person identification and verification in the field of biometric identification. Fingerprints possess two main types of features that are used for automatic fingerprint identification and verification: (i) global ridge and furrow structure that forms a special pattern in the central region of the fingerprint and (ii) Minutiae details associated with the local ridge and furrow structure.This paper presents the implementation of a minutiae based approach to fingerprint identification and verification and serves as a review of the different techniques used in various steps in the development of minutiae based Automatic Fingerprint Identification Systems (AFIS). The technique conferred in this paper is based on the extraction of minutiae from the thinned, binarized and segmented version of a fingerprint image. The system uses fingerprint classification for indexing during fingerprint matching which greatly enhances the performance of the matching algorithm. Good results (~92% accuracy) were obtained using the FVC2000 fingerprint databases.1. INTRODUCTIONFingerprints have been in use for biometric recognition since long because of their high acceptability, immutability and individuality. Immutability refers to the persistence of the fingerprints over time whereas individuality is related to the uniqueness of ridge details across individuals. The probability that two fingerprints are alike is 1 in 1.9 x 1015[1]. These features make the use of fingerprints extremely effective in areas where the provision of a high degree of security is an issue. The major steps involved in automated fingerprint recognition include a) Fingerprint Acquisition, b) Fingerprint Segmentation, c) Fingerprint Image Enhancement, d) Feature Extraction e) Minutiae Matching, f) Fingerprint Classification.Fingerprint acquisition can either be offline (inked) or Online (Live scan). In the inked method an imprint of an inked finger is first obtained on a paper, which is then scanned. This method usually produces images of very poor quality because of the non-uniform spread of ink and is therefore not exercised in online AFIS. For online fingerprint image acquisition, capacitative or opticalfingerprint scanners such as URU 4000, etc. are utilized which make use of techniques such as frustrated total internal reflection (FTIR)[2], ultrasound total internal reflection[3], sensing of differential capacitance[4] and non contact 3D scanning[5]for image development. Live scan scanners offer much greater image quality, usually a resolution of 512 dpi, which results in superior reliability during matching in comparison to inked fingerprints.Segmentation refers to the separation of fingerprint area (foreground) from the image background [6]. Segmentation is useful to avoid extraction of features in the noisy areas of fingerprints or the background. A Simple thresholding technique [7] proves to be ineffective because of the streaked nature of the fingerprint area. The presence of noise in a fingerprint image requires more vigorous techniques for effective fingerprint segmentation. A good segmentation method should exhibit the following characteristics [8]:·It should be insensitive to image contrast·It should detect smudged or noisy regions·Segmentation results should be independent of whether the input image is an enhanced image or a raw image·The segmentation results should be independent of image qualityRen et al. [8] proposed an algorithm for segmentation that employs feature dots, which are then used to obtain a close segmentation curve. The authors claim that their method surpasses directional field and orientation based methods [9,10,11] for fingerprint image segmentation. Shen et al. [12] proposed a Gabor filter based method in which eight Gabor filters are convolved with each image block and the variance of the filter response is used both for fingerprint segmentation and quality specification. Xian et al. [13] proposed a segmentation algorithm that exploits a block’s cluster degree, mean and variance. An optimal linear classifier is used for classification with morphological post-processing to remove classification errors. Bazen et al. [14] proposed a pixel wise technique for segmentation involving a linear combination of three feature vectors (i.e. gradient coherence, intensity mean and variance). A final morphological post-processing step is performed to eliminate holes in both the foreground and background. In spite of its high accuracy this algorithm has a very high computational complexity, which makes it impractical for real time processing. Klein et al.[15] proposed an algorithm that employs HMMs to remove the problem offragmented segmentation encountered during the use of different segmentation algorithms.For a good quality fingerprint feature extraction is much easier, efficient and reliable in comparison to a relatively lower quality fingerprint. The quality of fingerprints is degraded by skin conditions (e.g. wet or dry, cuts and bruises), sensor noise, non-uniform contact with sensor surface, and inherently low quality fingerprint images (e.g. those of elderly people, laborers). A significant percentage of fingerprints are of poor quality, which must be enhanced for the recognition process to be effective. Thereare two major objectives of fingerprint enhancement i.e. i) to increase the contrast between ridges and valleys and ii) to connect broken ridges. These objectives can be fulfilled by using a contextual filter whose characteristics vary according to the local context to be used for fingerprint enhancement instead of conventional image filters. The filter should posses the following characteristics:·It should provide a low pass (averaging) effect alongthe ridge direction with the aim of linking small gaps and filling impurities due to pores or noise.·It should have a band pass (differentiating) effect in the direction orthogonal to the ridges in order to increase the discrimination between ridges and valleys and top separate parallel linked ridges.Sherlock et al.[16]proposed an algorithm for fingerprint image enhancement that employs position-dependent Fourier-domain-filtering-based orientation smoothing and thresholding technique. Greenberg et al. [17] proposed two schemes for fingerprint enhancement. One method uses local histogram equalization, Wiener filtering, and image binarization whereas the other method uses a unique anisotropic filter for direct grayscale enhancement. O’Gorman et al. [18,19] proposed a contextual filter based approach that utilizes four main parameters of fingerprint images at a given resolution i.e. maxima and minima of the ridge and valley widths to form a mother filter whose rotated versions are then convolved with the image to yield the enhanced output. Hong et al. [20] proposed an effective method based on Gabor filters for image enhancement. Gabor filters fulfill the requirements of a good fingerprint enhancement filter mentioned earlier as they possess both the differentiating and averaging effects. Slight modifications to this technique were made by Greenberg et al. [21]. Jiangwei et al. [22]modified the technique given by [20]. Their approachmodels the sinusoidal shape of the ridge-valley structure in a closer manner and gives better results. Tico et al. [23] made use of a ridge detecting technique based on the second directional derivative of the image to carry out fingerprint enhancement. Watson et al. [24]multiplied the Fourier transform of each 32x32 block by its power spectrum raised to a power k to produce an efficient technique that can find its use in online fingerprint recognition systems. Fingerprints possess two major types of features: special type of pattern formed by the ridge and furrow structures in the central region of the fingerprints and minutiae detailsassociated with local ridges and furrows. Minutiae are minute details such as ridge endings (a point where a ridge ends abruptly) or a ridge bifurcation (where a ridge breaks up into two ridges). Minutiae are characterized by the position, direction and type (Ridge ending or bifurcation). The global features are used for fingerprint classification into six major classes whereas the minutiae details are used for fingerprint based person identification. Fingerprint analysts utilize the minutiae information for fingerprint identification and it is the most established technique in the field of AFIS. The accuracy of the technique is dependent upon the precision in the extraction of minutiae. There are two major type of methods that are used for minutiae extraction i) binarization based methods and ii) direct gray scale methods. In binarization-based methods some information is lost during binarization that can degrade the performance of the minutiae extractor. Direct gray scale methods overcome these problems but may be difficult to implement and time consuming to operate. The typical approach for a binarization-based method involves a priori enhancement, binarization and then thinning. Various binarization and thinning approaches are discussed in [25, 26, 27, 28, 29]. Once a binary skeleton has been obtained, a simple image scan allows the pixels corresponding to the minutiae to be detected by finding the crossing number. The minutiae obtained as a result of minutiae extraction need to be filtered in order to remove the false minutiae introduced. Various minutiae filtering techniques are proposed in [30,31].During enrollment the minutiae set obtained from an individual’s fingerprint is stored as a template for that subject. In the authentication module, the fingerprint given as input is compared or matched with the templates to provide the decision of authentication. This process is known as minutiae matching. For minutiae matching to be effective the input fingerprint should be registered to the template fingerprint using the minutiae information of both the fingerprints. After registration the minutiae sets are compared using the spatial distance, which must be smaller thana particular threshold for two minutiae to be declared as matched. A minutia matching is usually performed by using Hough transform[32,33]or by pre- alignment [34].Fingerprint classification is the process of classifying a given fingerprint into a number of classes such as left loop, right loop, whorl, arch and tented arch, double loop etc. Fingerprint classification is used for indexing in the fingerprint database and can greatly improve the performance of an AFIS. A typical fingerprint classification approach is based on the extraction of fingerprint singular points (core and delta) and the implementation of a rule based classification system that takes its decision based on the number and placement of core and delta in a fingerprint. Different classification algorithms are proposed in [35,36,37].The rest of the paper is organized as follows: Section II gives details about the method used, section III exhibits the results with section IV giving the conclusions.II. IMPLEMENTATION OF THE AFISThis paper introduces a prototype automatic identity authentication system that is capable of authenticating the identity of an individual, using fingerprints. The main components of the AFIS are1.Fingerprint database2.Fingerprint features database3.Enrollment Module4.Authentication module.The fingerprint database stores the fingerprint images. for this purpose. The features extracted from these fingerprints are stored in the features database along with the person ID. The objective of the enrollment module is to admit a person using his/her ID and fingerprints into a fingerprints database after the process of feature extraction. These features form a template that is used to determine or verify the identity of the subject, formulating the process of authentication. The component of the AFIS used for authentication is referred to as the authentication module. Figure 1 illustrates the different steps involved in the development of the AFIS. The details of these steps are given in the following subsections.Fingerprint segmentation is an important part of a fingerprint identification and verification system. However the time spent in segmentation is also crucial. The algorithms presented in [13] and [14]work quite well in the extraction of the required region but these algorithms have veryhigh computational cost.We have developed an efficient algorithm that works with acceptable performance and has a lower computational cost. This algorithm is based only on the block coherence of an image. Coherence gives us a measure of how well the gradients of the fingerprint image are pointing in the same direction. In a window of size WxW around a pixel, the coherence is defined as:yy xx xy yy xx W y s x s Wy s x s G G G G G G G G G coh ++-==∑∑22,,,,4)(),(),( (1)Where Gx and Gy are the local gradients along X and Y directions respectively. The resulting gradient coherence image is smoothed by the use of a Gaussian smoothing filter to give a coherence image C(x, y). The smoothed image is then binarized to produce a segmentation mask CB. The binarization is carried out by global thresholding asOtherwise S M y i C j i C cC B 5.0),(01),(->⎩⎨⎧= (2)Fig. 1: Steps required for feature extractionA B C DA. Orientation Field,B. Ridge Frequency ImageC. Enhanced ImageD. Thinned Image IV . Minutiae ExtractionMinutiae extraction was carried out using the crossing number approach [39]. Crossing number of pixel ‘p’ is defined as half the sum of the differences between pairs of adjacent pixels defining the 8-neighborhood of ‘p’. Mathematically∑=--=8.118mod )()(21)(i i i p val p val p cn (3) Where p0 to p7 are the pixels belonging to an ordered sequence of pixels defining the 8-neighborhood of p and val (p) is the pixel value.Fig.2: cn (p)=2,cn (p)=3 and cn (p)=1 representing a nonminutiae region, a bifurcation and a ridge endingCrossing numbers 1 and 3 correspond to ridge endings and ridge bifurcations respectively. An intermediate ridge point has a crossing number of 2. The minutiae obtained from this algorithm must be filtered to preserve only the true minutiae. The different types of false minutiae introduced during minutiae extraction include spike, bridge, hole, break, Spur, Ladder, and Misclassified Border areas. (See figure 5)Fig. 3 Types of false minutiaeA BC DE FA. Spike,B. Bridge,C. Hole,D.Break,E. SpurF. LadderThe number of minutiae in a given area is also limited therefore the minutiae density must also be kept in check. In order to filter out these false minutiae a 3 level-filtering process is applied: Level 1: Removes the false ridge endings created as a result of the application of minutiae extraction algorithm at the ends of the thinned image.Level 2: Removes the first five types of minutiae mentioned above using the rule based morphological minutiae filtering approach given by [40].Level 3: This stage limits the maximum number of minutiae present in the thinned image to a pre-specified threshold.A minutiae m is described by the triplet m={x, y, θ}, where x, y indicate the minutiae location coordinates and θ denotes the minutiae orientation, which is the orientation evaluated for the minutiae location from the orientation image obtained during the enhancement process. The minutiae type is not being used during the matching process since minutiae type can be inverted due to enhancement and binarization steps.Fig.4. Filtered and Unfiltered Minutiae SetsV . Minutiae MatchingLet T and I be the representation of the template and input fingerprint, respectively. Let the minutiae sets of the twofingerprints be given by:{}{}m i y x m m m m T i i i i m ..1,,,,...,,21===θ {}{}n j y x m m m m I i i i i n ..1,,,,...,,....21===θA minutia mj ’in I and a minutia mi in T are considered to be matched if their spatial and orientation differences are within specified thresholds 0r and 0θ. Minutia matching was carried out by using the approach given in [32]. In this approach the minutiae sets are first registered using a derivative of the Hough transform (Figure. 5) followed by fingerprint matching using spatial and orientation-based distance computation. The matching algorithm returns a percentage match score, which is then used to take the match-no match decision based on the security criterion.Fig. 5 A. Unregistered, B.Registered Minutiae SetsVI. Fingerprint ClassificationFingerprint classification was carried out by the extraction of the singular points from the fingerprint image using the approach presented by [41](see figure 8). After the extraction of singular points the approach mentioned in [42] was used to perform a rule-based classification. The introduction of fingerprint classification greatly improves the matching time especially for large databases.Fig. 6 Original Image an the extracted singular pointsIII. RESULTS AND DISCUSSIONThe system was tested on the FVC 2000 database [38]. The database used was developed using low cost capacitative fingerprint scanners. The database contains a total of about 800 fingerprints of 110 different fingers. The accuracy of the system is quantified in terms of false acceptance ratio (FAR) and the false rejection ration (FRR). An FAR of 1% was obtained for an FRR of 7% for this database. The Equal error rate (FAR=FRR) for the system mentioned was found to be 5% that180of rotation in a fingerprint image. The implies an accuracy of 95%. The system can handle 0system was implemented using MA TLAB 6.5 [43]and the time taken for processing a single fingerprint is 12s on a 2.2 GHz P4.with a 496MB RAM These results have been obtained without code optimization. The system will be implemented in C++ after code optimization, which would further reduce the total processing time.IV. CONCLUSION:The paper presents an overview of the different steps involved in the development of a fingerprint based person identification and verification system. We have also proposed the design and development of a minutiae based AFIS. The system developed is still a prototype version and needs improvements for decreasing the time spent during fingerprint processing and the reduction in the number of false acceptances and rejections made by the algorithm. Work is currently underway on some modifications to the matching mechanism, which would further improve the system’s accuracy.译文指纹识别和验证的匹配系统摘要:指纹是在生物识别领域中使用最广泛的生物特征。
A201004-1314 指纹识别系统(英文版)
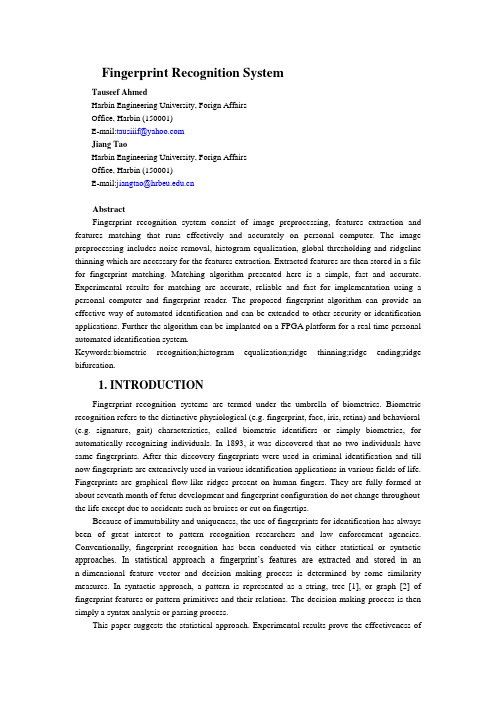
Fingerprint Recognition SystemTauseef AhmedHarbin Engineering University, Forign AffairsOffice, Harbin (150001)E-mail:******************Jiang TaoHarbin Engineering University, Forign AffairsOffice, Harbin (150001)E-mail:******************.cnAbstractFingerprint recognition system consist of image preprocessing, features extraction and features matching that runs effectively and accurately on personal computer. The image preprocessing includes noise removal, histogram equalization, global thresholding and ridgeline thinning which are necessary for the features extraction. Extracted features are then stored in a file for fingerprint matching. Matching algorithm presented here is a simple, fast and accurate. Experimental results for matching are accurate, reliable and fast for implementation using a personal computer and fingerprint reader. The proposed fingerprint algorithm can provide an effective way of automated identification and can be extended to other security or identification applications. Further the algorithm can be implanted on a FPGA platform for a real time personal automated identification system.Keywords:biometric recognition;histogram equalization;ridge thinning;ridge ending;ridge bifurcation.1. INTRODUCTIONFingerprint recognition systems are termed under the umbrella of biometrics. Biometric recognition refers to the distinctive physiological (e.g. fingerprint, face, iris, retina) and behavioral (e.g. signature, gait) characteristics, called biometric identifiers or simply biometrics, for automatically recognizing individuals. In 1893, it was discovered that no two individuals have same fingerprints. After this discovery fingerprints were used in criminal identification and till now fingerprints are extensively used in various identification applications in various fields of life. Fingerprints are graphical flow-like ridges present on human fingers. They are fully formed at about seventh month of fetus development and fingerprint configuration do not change throughout the life except due to accidents such as bruises or cut on fingertips.Because of immutability and uniqueness, the use of fingerprints for identification has always been of great interest to pattern recognition researchers and law enforcement agencies. Conventionally, fingerprint recognition has been conducted via either statistical or syntactic approaches. In statistical approach a fingerprint’s features are extracted and stored in an n-dimensional feature vector and decision making process is determined by some similarity measures. In syntactic approach, a pattern is represented as a string, tree [1], or graph [2] of fingerprint features or pattern primitives and their relations. The decision making process is then simply a syntax analysis or parsing process.This paper suggests the statistical approach. Experimental results prove the effectiveness ofthis method on a computer platform, hence making it suitable for security applications with a relatively small database. The preprocessing of fingerprints is carried out using modified basic filtering methods which are substantially good enough for the purpose of our applications with reasonable computational time. Block diagram for the complete process is shown in Figure.1.2. IMAGE PREPROCESSINGFor the proper and true extraction of minutiae, image quality is improved and image preprocessing is necessary for the features extraction because we cannot extract the required points from the original image. First of all, any sort of noise present in the image is removed. Order statistics filters are used to remove the type of noise which occurs normally at image acquisition. Afterwards the following image preprocessing techniques are applied to enhance the fingerprint images for matching.2.1 Histogram EqualizationThis method is used where the unwanted part of the image is made lighter in intensity so as to emphasize the desired the desired part. Figure 2(a) shows the original image and Figure 2(b) histogram equalization in which the discontinuities in the small areas are removed. For the histogram equalization, let the input and the output level for an arbitrary pixel be i and l, respectively. Then the accumulation of histogram from 0 to i ( 0 ≤i ≤255,0 ≤k ≤255) is given bywhere H(k) is the number of pixel with gray level k, i.e. histogram of an area, and C(i) is also known as cumulative frequency.2.2 Dynamic ThresholdingBasic purpose of thresholding is to extract the required object form the background. Thresholding is simply the mapping of all data points having gray level more that average gray level. The results of thresholding are shown in Figure 3.2.3 Ridgeline ThinningBefore the features can be extracted, the fingerprints have to be thinned or skeletonised so that all ridges are one pixel thick. When a pixel is decided as a boundary pixel, it is deleted directly form the image [3-5] or flagged and not deleted until the entire image been scanned [6-7]. There are deficiencies in both cases. In the former, deletion of each boundary pixel will change the object in the image and hence affect the object symmetrically. To overcome this problem, some thinning algorithms use several passes in one thinning iteration. Each pass is an operation to remove boundary pixels from a given direction. Pavlidis [8] and Fiegin and Ben-Yosef [9] have developed effective algorithms using this method. However, both the time complexity and memory requirement will increase. In the latter, as the pixels are only flagged, the state of the bitmap at the end of the last iteration will used when deciding which pixel to delete. However, if this flag map is not used to decide whether a current pixel is to be deleted, the information generated from processing the previous pixels in current iteration will be lost. In certain situations the final skeleton may be badly distorted. For example, a line with two pixels may be completely deleted. Recently, Zhou, Quek and Ng [10] have proposed an algorithm that solves the problem described earlier and is found to perform satisfactorily while providing a reasonable computational time. The thinning effect is illustrated in Figure 43. FEATURES EXTRACTIONThe two basic features extracted from the image are ridge endings and ridge bifurcation. For fingerprint images used in automated identification, ridge endings and bifurcation are referred toas minutiae. To determine the location of these features in the fingerprint image, a 3x3 window mask is used (Figure 5). M is the detected point and X1… X8 are its neighboring points in a clockwise direction. If X n is a black pixel, then its response R (n) will be 1 or otherwise it will be 0. If M is an ending, the response of the matrix will bewhere R(9)=R(1). For M to be a bifurcation,for example, if a bifurcation is encountered during extraction, mask will contain the pixel information such as R(1) = R(3)= R(4) = R(6) = R(7) = 0, R(2) = R(5) = R(8) = R(9) = 1, andFor all the minutiae detected in the interpolated thinned image, the coordinates and their minutiae type is save as feature file. At the end of feature extraction, a feature record of the fingerprint is formed.4. MATCHINGFingerprint matching is the central part of this paper. The proposed technique is based on structural model of fingerprints [11]. One of the major breakthroughs of this method is its ability to mach fingerprints that are shifted, rotated and stretched. This is achieved by a different matching approach. As it is clear that this algorithm matches the two fingerprint images captured at different time. This matching is based on the minutiae identification and minutiae type matching. Matching procedure is complex due to two main reasons;1) The minutiae of the fingerprint captured may have different coordinates2) The shape of the fingerprint captured at different time may be different due to stretching.An automated fingerprint identification system that is robust must have following criteria:1) Size of features file must be small2) Algorithm must be fast and robust3) Algorithm must be rotationally invariant4) Algorithm must be relatively stretch invariantTo achieve these criteria, the structural matching method described by Hrechak and McHugh [11] is adopted as the basis of our recognition algorithm, with changes made to the algorithm, to provide more reliable and improving overall matching speed. This matching represents the local identification approach, in which local identified features, their type and orientation is saved in features file, is correlated with the other image’s extracted features file. The model is shown in Figure 6.For each extracted features on the fingerprint, a neighborhood of some specified radius R about the central feature is defined and then Euclidean distance and relative angles between the central point and the other point is noted with the point’s type. Since the distance among the point remains the same throughout the life. So this technique works well for the rotated and shifted images.5. CONCLUSIONA fingerprint recognition algorithm that is fast, accurate and reliable has been successfully implemented. This algorithm can be modified, introducing the ridgeline count, and then could be used in online and real time automated identification and recognition system.REFERENCES[1] MOAYER, B., and FU, K.S.: ‘A tree system approach for fingerprint pattern recognition’, IEEE Trans., 1986,PAMT-8, (3), pp. 376-387[2] ISENOR, D.K., and ZAKY, S.G.: ‘Fingerprint identification using graph matching’, Pattern Recognit., 1986,19, (2) pp. 113-122[3] TAMURA, H.: ‘A comparison of line thinning algorithms from digital geometry viewpoint’. Proceedings of fourth international joint conference on Pattern Recognition, Kyoto, Nov. 1978, pp. 715-719[4] HILDITCH, C.J.: ’Linear skeleton from square cupboards’, Machine Intel., 1969, 4, pp.403-420[5] NACCACHE, N.J., and SH INCHAL, R.: ‘An investigation into the skeletonization approach of Hilditch’,Pattern Recognit., 1984, 17, (3), pp. 279-284[6] JANG, B.K., and CHIN, P.T.: ‘Analysis of thinning algorithms using mathematical morphology’, IEEE Trans.,Pattern Anal. Machine Intel., 1990, 12, (6), pp. 541-551[7] XU, W., and WANG, C.: ‘CGT: a fast thinning algorithm implemented on sequential computer’, IEEE Trans.,1987, SMC-17, (5), pp. 847-851[8] PAVLIDIS, T.: ‘Algorithm for graphical and image processing’, Comput. Graph. Image Process., 1982, 20,pp133-157[9] FEIGIN, G., and BEN YOSEF, N.: ‘Line thinning algorithm’, Proc. SPIE – Int. Soc. Opt. Eng., 1984, 397, pp.108-112[10]ZHOU, R.W., QUEK, C., and NG, G.S.: ‘Novel single-pass thinning algorithm’, Pattern Recognit. Lett., 1995,16, (12), pp.1267-1275[11]HRECHAK, A.K., and MCHUGH, J.A.: ‘Automated fingerprint recognition using structural matching’,Pattern Recognit., 1990, 23, (8), pp. 893-904[12]GOUNZALEZ, R.C., and WOOD, R.E.: ‘Digital image processing’ (Pearson Education, 2002)AUTHORS BREIF INTRODUCTIONTauseef Ahmed, born in 1982, Master of Engineering in Communication & Information Systems at Harbin Engineering University, currently researching and developing the software based EPIRB.Previously holds a Computer Engineering degree from Pakistan.Jiang Tao Ph.D, born in 1973, professor, senior member of China Institute of Communications,Heilongjiang Western Returned Scholars Association / Communications Section Member, IEEE EMC Chapter, the antenna branch, communications branch member, IEEE Harbin Section EMC Chapter Vice Chairman. Research interests are electromagnetic compatibility, antenna design, radio navigation and positioning. He got the technological achievements COSTIND second prize a,Heilongjiang Province Department of Education Teaching Achievement Award 1, second prize a,textbook, a total of 40 articles.。
文献翻译--指纹识别系统

Fingerprint Recognition SystemAbstractFingerprint recognition system consist of image preprocessing, features extraction and features matching that runs effectively and accurately on personal computer. The image preprocessing includes noise removal, histogram equalization, global thresholding and ridgeline thinning which are necessary for the features extraction. Extracted features are then stored in a file for fingerprint matching. Matching algorithm presented here is a simple, fast and accurate. Experimental results for matching are accurate, reliable and fast for implementation using a personal computer and fingerprint reader. The proposed fingerprint algorithm can provide an effective way of automated identification and can be extended to other security or identification applications. Further the algorithm can be implanted on a FPGA platform for a real time personal automated identification system.Keywords:biometric recognition;histogram equalization;ridge thinning;ridge ending;ridge bifurcation.1. INTRODUCTIONFingerprint recognition systems are termed under the umbrella of biometrics. Biometric recognition refers to the distinctive physiological (e.g. fingerprint, face, iris, retina) and behavioral (e.g. signature, gait) characteristics, called biometric identifiers or simply biometrics, for automatically recognizing individuals. In 1893, it was discovered that no two individuals have same fingerprints. After this discovery fingerprints were used in criminal identification and till now fingerprints are extensively used in various identification applications in various fields of life. Fingerprints are graphical flow-like ridges present on human fingers. They are fully formed at about seventh month of fetus development and fingerprint configuration do not change throughout the life except due to accidents such as bruises or cut on fingertips.Because of immutability and uniqueness, the use of fingerprints for identification has always been of great interest to pattern recognition researchers and law enforcement agencies. Conventionally, fingerprint recognition has been conducted via either statistical or syntactic approaches. In statistical approach a fingerprint’s features are extracted and stored in an n-dimensional feature vector and decision making process is determined by some similarity measures. In syntactic approach, a pattern is represented as a string, tree [1], or graph [2] of fingerprint features or pattern primitives and their relations. The decision making process is then simply a syntax analysis or parsing process.This paper suggests the statistical approach. Experimental results prove the effectiveness of this method on a computer platform, hence making it suitable for security applications with a relatively small database. The preprocessing of fingerprints is carried out using modified basic filtering methods which are substantially good enough for the purpose of our applications with reasonable computational time. Block diagram for the complete process is shown in Figure.1.2. IMAGE PREPROCESSINGFor the proper and true extraction of minutiae, image quality is improved and image preprocessing is necessary for the features extraction because we cannot extract the required points from the original image. First of all, any sort of noise present in the image is removed. Order statistics filters are used to remove the type of noise which occurs normally at image acquisition. Afterwards the following image preprocessing techniques are applied to enhance the fingerprint images for matching.2.1 Histogram EqualizationThis method is used where the unwanted part of the image is made lighter in intensity so as to emphasize the desired the desired part. Figure 2(a) shows the original image and Figure 2(b) histogram equalization in which the discontinuities in the small areas are removed. For the histogram equalization, let the input and the output level for an arbitrary pixel be i and l, respectively. Then the accumulation of histogram from 0 to i ( 0 ≤i ≤255,0 ≤k ≤255) is given bywhere H(k) is the number of pixel with gray level k, i.e. histogram of an area, and C(i) is also known as cumulative frequency.2.2 Dynamic ThresholdingBasic purpose of thresholding is to extract the required object form the background. Thresholding is simply the mapping of all data points having gray level more that average graylevel. The results of thresholding are shown in Figure 3.2.3 Ridgeline ThinningBefore the features can be extracted, the fingerprints have to be thinned or skeletonised so that all ridges are one pixel thick. When a pixel is decided as a boundary pixel, it is deleted directly form the image [3-5] or flagged and not deleted until the entire image been scanned [6-7]. There are deficiencies in both cases. In the former, deletion of each boundary pixel will change the object in the image and hence affect the object symmetrically. To overcome this problem, some thinning algorithms use several passes in one thinning iteration. Each pass is an operation to remove boundary pixels from a given direction. Pavlidis [8] and Fiegin and Ben-Yosef [9] have developed effective algorithms using this method. However, both the time complexity and memory requirement will increase. In the latter, as the pixels are only flagged, the state of the bitmap at the end of the last iteration will used when deciding which pixel to delete. However, if this flag map is not used to decide whether a current pixel is to be deleted, the information generated from processing the previous pixels in current iteration will be lost. In certain situations the final skeleton may be badly distorted. For example, a line with two pixels may be completely deleted. Recently, Zhou, Quek and Ng [10] have proposed an algorithm that solves the problem described earlier and is found to perform satisfactorily while providing a reasonable computational time. The thinning effect is illustrated in Figure 43. FEATURES EXTRACTIONThe two basic features extracted from the image are ridge endings and ridge bifurcation. For fingerprint images used in automated identification, ridge endings and bifurcation are referred to as minutiae. To determine the location of these features in the fingerprint image, a 3x3 window mask is used (Figure 5). M is the detected point and X1… X8 are its neighboring points in a clockwise direction. If X n is a black pixel, then its response R (n) will be 1 or otherwise it will be 0.If M is an ending, the response of the matrix will bewhere R(9)=R(1). For M to be a bifurcation,for example, if a bifurcation is encountered during extraction, mask will contain the pixel information such as R(1) = R(3)= R(4) = R(6) = R(7) = 0, R(2) = R(5) = R(8) = R(9) = 1, andFor all the minutiae detected in the interpolated thinned image, the coordinates and their minutiae type is save as feature file. At the end of feature extraction, a feature record of the fingerprint is formed.4. MATCHINGFingerprint matching is the central part of this paper. The proposed technique is based on structural model of fingerprints [11]. One of the major breakthroughs of this method is its ability to mach fingerprints that are shifted, rotated and stretched. This is achieved by a different matching approach. As it is clear that this algorithm matches the two fingerprint images captured at different time. This matching is based on the minutiae identification and minutiae type matching. Matching procedure is complex due to two main reasons;1) The minutiae of the fingerprint captured may have different coordinates2) The shape of the fingerprint captured at different time may be different due to stretching.An automated fingerprint identification system that is robust must have following criteria:1) Size of features file must be small2) Algorithm must be fast and robust3) Algorithm must be rotationally invariant4) Algorithm must be relatively stretch invariantTo achieve these criteria, the structural matching method described by Hrechak and McHugh [11] is adopted as the basis of our recognition algorithm, with changes made to the algorithm, to provide more reliable and improving overall matching speed. This matching represents the localidentification approach, in which local identified features, their type and orientation is saved in features file, is correlated with the other image’s extracted fe atures file. The model is shown in Figure 6.For each extracted features on the fingerprint, a neighborhood of some specified radius R about the central feature is defined and then Euclidean distance and relative angles between the central point and the other point is noted with the point’s type. Since the distance among the point remains the same throughout the life. So this technique works well for the rotated and shifted images.5. CONCLUSIONA fingerprint recognition algorithm that is fast, accurate and reliable has been successfully implemented. This algorithm can be modified, introducing the ridgeline count, and then could be used in online and real time automated identification and recognition system.REFERENCES[1] MOAYER, B., and FU, K.S.: ‘A tree system approach for fingerprint pattern recognition’, IEEE Trans., 1986,PAMT-8, (3), pp. 376-387[2] ISENOR, D.K., and ZAKY, S.G.: ‘Fingerprint identification using graph matching’, Pattern Recognit., 1986,19, (2) pp. 113-122[3] TAMURA, H.: ‘A comparison of line thinning algorithms from digital geometry viewpoint’. Proceedings of fourth international joint conference on Pattern Recognition, Kyoto, Nov. 1978, pp. 715-719[4] HILDITCH, C.J.: ’Linear skeleton from square cupboards’, Machine Intel., 1969, 4, pp.403-420[5] NACCACHE, N.J., and SHINCHAL, R.: ‘An investigation into the skeletonization approach of Hilditch’,Pattern Recognit., 1984, 17, (3), pp. 279-284[6] JANG, B.K., and CHIN, P.T.: ‘Analysis of thinning algorithms using mathematical morphology’, IEEE Trans.,Pattern Anal. Machine Intel., 1990, 12, (6), pp. 541-551[7] XU, W., and WANG, C.: ‘CGT: a fast thinning algorithm implemented on sequential computer’, IEEETrans.,1987, SMC-17, (5), pp. 847-851[8] PAVLIDIS, T.: ‘Algorithm for graphical and image processing’, Comput. Graph. Image Process., 1982, 20,pp133-157[9] FEIGIN, G., and BEN YOSEF, N.: ‘Line thinning algorithm’, Proc. SPIE – Int. Soc. Opt. Eng., 1984, 397, pp.108-112[10]ZHOU, R.W., QUEK, C., and NG, G.S.: ‘Novel single-pass thinning a lgorithm’, Pattern Recognit. Lett., 1995,16, (12), pp.1267-1275[11]HRECHAK, A.K., and MCHUGH, J.A.: ‘Automated fingerprint recognition using structural matching’,Pattern Recognit., 1990, 23, (8), pp. 893-904[12]GOUNZALEZ, R.C., and WOOD, R.E.: ‘Digital image processing’ (Pearson Education, 2002)指纹识别系统摘要指纹识别系统包括图像预处理、特征提取和在个人计算机上进行有效、准确地匹配的功能。
指纹锁毕业设计
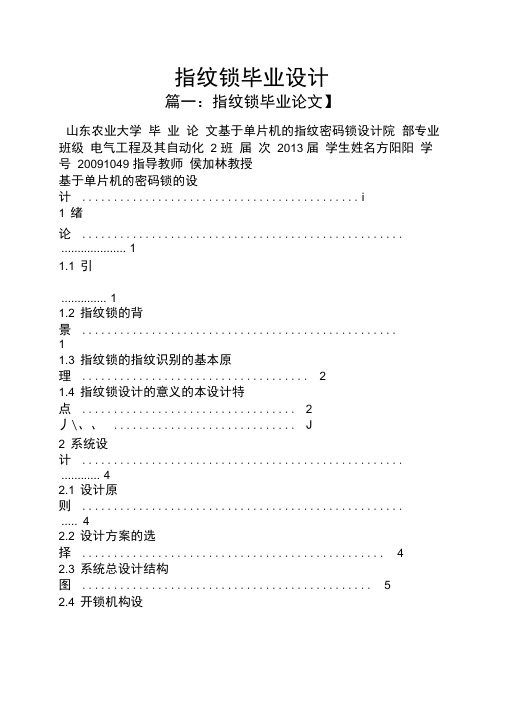
指纹锁毕业设计篇一:指纹锁毕业论文】山东农业大学毕业论文基于单片机的指纹密码锁设计院部专业班级电气工程及其自动化 2 班届次2013 届学生姓名方阳阳学号20091049 指导教师侯加林教授基于单片机的密码锁的设计 (i)1 绪论................................................... .. (1)1.1 引 (1)1.2 指纹锁的背景..................................................11.3 指纹锁的指纹识别的基本原理 (2)1.4 指纹锁设计的意义的本设计特点 (2)丿\、、............................. J2 系统设计................................................... (4)2.1 设计原则................................................... .. (4)2.2 设计方案的选择 (4)2.3 系统总设计结构图 (5)2.4 开锁机构设计..................................................3 主要元器件介绍...................................................73.1 89c51 单片介绍 (7)3.1.1 时钟电路...................................................83.1.2 复位电路 (9)3.2 stm32 单片机介绍 (10)3.2.1 stm32f103 性能特点 (11)3.2.2stm32f103 的系统作用 (12)3.3 sb2000m 指纹识别模块介绍 (13)3.3.1 结构................................................... .. (13)3.3.2 产品规格...................................................133.4 rs-232c 连接器介绍 (14)3.5 gsm 设备的介绍 (15)4 硬件电路的设计 (18)4.1 系统电源的设计 (18)4.2 系统的备用电源的设计 (19)4.2.1 备用电源的供给设计 (19)4.2.2 备用电源的使用选择设计 (21)4.3 开锁电路的设计 (22)4.4 指纹识别模块电路的设计 (23)4.5 总电路的设计 (24)5 软件程序的设计 (25)5.1 程序设计语言的选择及步骤 (25)5.1.1 c语言简介 (25)5.1.2 c语言特点...... . (25)5.1.3 c语言优点...... . (25)5.2 keil简介 (26)5.3 开锁程序的设计及流程图 (27)结论................................................... (28)致谢................................................... (29)参考文献................................................... .. (30)附录一:程序................................................... ....... 31 附录二:电路接线图及实物图 (42)design of fingerprint lock based onmcu (i)1 the firstchapter ............................................................................................ .. (1)1.1introduction ................................................................................ . (1)1.2 the background of fingerprintlock (1)1.3 the basicprinciples ......................................................................................... .. (2)1.4 the designcharacteristics ................................................................................ .. (2)2 the secondchapter ............................................................................................. . (4)2.1designprinciple ........................................................................................... (4)2.2designoptions ............................................................................................. (4)2.3the systemstructure .......................................................................................... .. 52.4 lock mechanismdesign (5)3 the thirdchapter ............................................................................................ (7)3.1 89c51introduction ..................................................................................... . (7)3.1.1 clockcircuit ............................................................................................... (8)3.1.2 resetcircuit ............................................................................................... .. 93.2stm32 ............................................................................................... (10)3.2.1 stm32f103features (11)3.2.2stm32f103systems (12)3.3sb2000m .......................................................................................... (13)3.3.1structure .......................................................................................... .. (13)3.3.2specification ..................................................................................... .. (13)3.4 rs-232c ................................................................................................ .. (14)3.5gsm .................................................................................................. (15)4 the fourthchapter ............................................................................................ .. (18)4.1 power supply systerm design (18)4.2 standbypower ............................................................................................... .. (19)4.2.1design .............................................................................................. .. (19)4.2.2 designoptions .............................................................................................214.3 lock circuitdesign ..............................................................................................224.4 the fingerprint identification circuit (23)4.5 the totalcircuit ............................................................................................... .. 245 the fifthchapter ............................................................................................ .. (25)5.1 selection and step of programming language 255.1.1 clanguage .......................................................................................... (25)5.1.2 c languagefeatures (25)5.1.3 c languageadvantages (25)5.2keil ................................................................................................... . (26)5.3 lock program design ............................................................27conclusions ...................................................................................... .. (28)thanks .............................................................................................. .. (29)reference ......................................................................................... .. (30)appendix a :programs ......................................................................................... . (31)appendix b :circuit design and the real figure 42【篇二:指纹锁的设计与实现】(2009 届)本科毕业设计(论文)资料湖南工业大学教务处2009 届本科毕业设计(论文)资料设计说明书(或毕业论文)第一部分(2009 届)本科毕业设计(论文)指纹锁的设计与实现2009 年06 月摘要随着信息技术的快速发展,传统的身份识别方式(诸如密码、证件等)已经不能满足不断增加的安全需要,身份识别已经成为信息安全领域所面临的一个难题。
基于无线网络的指纹识别考勤仪的设计的外文文献原稿和译文
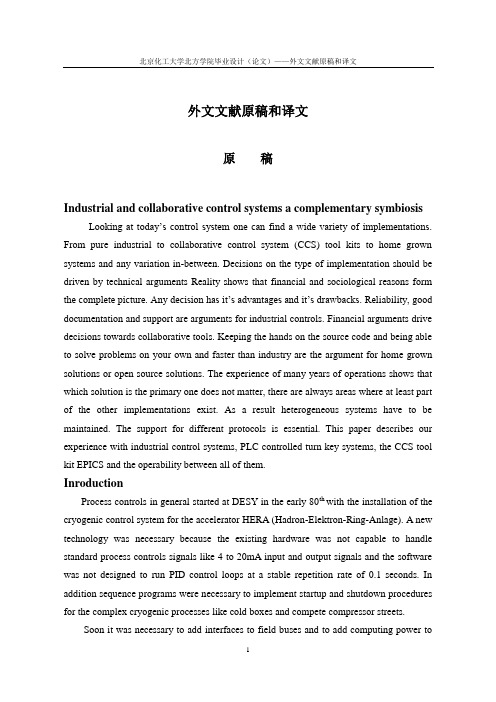
外文文献原稿和译文原稿Industrial and collaborative control systems a complementary symbiosis Looking at today’s control system one can find a wide variety of implementations. From pure industrial to collaborative control system (CCS) tool kits to home grown systems and any variation in-between. Decisions on the type of implementation should be driven by technical arguments Reality shows that financial and sociological reasons form the complete picture. Any decision has it’s advantages and it’s drawbacks. Rel iability, good documentation and support are arguments for industrial controls. Financial arguments drive decisions towards collaborative tools. Keeping the hands on the source code and being able to solve problems on your own and faster than industry are the argument for home grown solutions or open source solutions. The experience of many years of operations shows that which solution is the primary one does not matter, there are always areas where at least part of the other implementations exist. As a result heterogeneous systems have to be maintained. The support for different protocols is essential. This paper describes our experience with industrial control systems, PLC controlled turn key systems, the CCS tool kit EPICS and the operability between all of them.InroductionProcess controls in general started at DESY in the early 80th with the installation of the cryogenic control system for the accelerator HERA (Hadron-Elektron-Ring-Anlage). A new technology was necessary because the existing hardware was not capable to handle standard process controls signals like 4 to 20mA input and output signals and the software was not designed to run PID control loops at a stable repetition rate of 0.1 seconds. In addition sequence programs were necessary to implement startup and shutdown procedures for the complex cryogenic processes like cold boxes and compete compressor streets.Soon it was necessary to add interfaces to field buses and to add computing power tocryogenic controls. Since the installed D/3 system[1] only provided an documented serial connection on a multibus board, the decision was made to implement a DMA connection to VME and to emulate the multibus board’s functionality. The necessary computing power for temperature conversions came from a Motorola MVME 167 CPU and the field bus adapter to the in house SEDAC field bus was running on an additional MVME 162. The operating system was VxWorks and the application was the EPICS toolkit.Since this implementation was successful it was also implemented for the utility controls which were looking for a generic solution to supervise their distributed PLC’s.A slection of process control systems at desyDCS (D/3)As a result of a market survey the D/3 system from GSE was selected for the HERA cryogenic plant. The decision was fortunate because of the DCS character of the D/3. The possibility to expand the system on the display- and on the I/O side helped to solve the increasing control demands for HERA. The limiting factor for the size of the system is not the total number of I/O but the traffic on the communication network. This traffic is determined by the total amount of archived data not by the data configured in the alarm system. The technical background of this limitation is the fact that archived data are polled from the display servers whereas the alarms are pushed to configured destinations like alarm-files, (printer) queues or displays.SCADA Systems with DCS Features (Cube)The fact that the D/3 system mentioned above had some hard coded limitations with respect to the Y2K problem was forcing us to look for an upgrade or a replacement of the existing system. As a result of a call for tender the company Orsi with their product Cube came into play [2]. The project included a complete replacement of the installed functionality. This included the D/3 as well as the integration of the DESY field bus SEDAC and the temperature conversion in VME. The project started promising. But soon technical and organizational problems were pushing the schedule to it’s limits which were determined by the HERA shutdown scheduled at that time. The final acceptance test at the vendors site showed dramatic performance problems. Two factors could be identified as the cause of these problems. The first one was related to the under estimated CPU load of the6th grade polynomial temperature conversion running at 1 Hz. The second one was the additional CPU load caused by the complex functionality of the existing D/3 system. Here it was underestimated that each digital and analog i nput and output channel had it’s own alarm limits in the D/3 system. In a SCADA like system as Cube the base functionality of a channel is to read the value and make it available to the system. Any additional functionality must be added. Last not least the load on the network for polling all the alarm limits – typically for a SCADA system –was also driving the network to it’s limits.Finally the contract with Orsi was cancelled and an upgrade of the D/3 system was the only possible solution. It was finally carried out in march 2003.In any case it should be mentioned that the Cube approach had the advantage of a homogeneous configuration environment (for the Cube front end controllers) – compared with heterogeneous environments for ‘pure’ SCADA systems.SCADA (PVSS-II)The H1 experiment at the HERA accelerator decided to use PVSS-II for an upgrade of their slow control systems[3]. The existing systems were developed by several members of the H1 collaboration and were difficult to maintain. The decision to use PVSS as a replacement was driven by the results of an extensive survey carried out at CERN by the Joint Controls Project [4]. PVSS is a ‘pure’ Supervisory And Data Acquisition System (SCADA). It provides a set of drivers for several field buses and generic socket libraries to implement communication over TCP/IP. The core element is the so called event manager. It collects the data (mostly by polling) from the I/O devices and provides an event service to the attached management services like: control manager, database manager, user interface, API manager and the built in HTTP server. The PVSS scripting library allows to implement complex sequences as well as complex graphics. Compared with other SCADA systems PVSS comes with one basic feature: it provides a true object oriented API to the device’s data.One major disadvantage of SCADA systems is the fact that two databases, the one for the PLC and the one for the SCADA system must be maintained. Integrated environments try to overcome this restriction.EPICSEPICS has emerged at DESY from a problem solver to a fully integrated control system. Starting from the data collector and number cruncher for the cryogenic control system, EPICS made it’s way to become the core application for the DESY utility group. In addition it is used wherever data is available through VME boards or by means of Industry Pack (IP) modules. For those cryogenic systems which are not controlled by the D/3 system EPICS is used with it’s complete functionality. In total about 50 Input Output Controller (IOC) are operational processing about 25 thousand records.1 EPICS as a SCADA SystemThe utility group ( water, electrical power, compressed air, heating and air conditioning) is using a variety of PLC’s spread out over the whole DESY site. EPICS is used to collect the data from these PLC’s over Profibus (FMS and DP) and over Ethernet (Siemens H1 and TCP). The IOC’s provide the interfaces to the buses and collect the data. The built in alarm checking of the EPICS records is used to store and forward alarm states to the alarm handler (alh) of the EPICS toolkit. In addition tools like the channel archiver and the graphic display (dm2k) are used. The default name resolution (by UDP broadcast) and the directory server (name server) are used to connectclient and server applications over TCP. All of these are basically SCADA functions.The textual representation of all configuration files ( for the IOC, the graphic tool, the alarm handler and the archiver) provides a flexible configuration scheme. At DESY the utility group has developed a set of tools to create IOC databases and alarm configuration files from Oracle. This way the controls group provides the service to maintain the EPICS tools and the IOC’s while the users can concentrate on th e equipment being controlled.2 EPICS as a DCS SystemBesides the basic components of a SCADA system EPICS also provides a full flavoured Input Output Controller (IOC). The IOC provides all of the function a DCS system requires, such as: a standard set of properties implemented in each record, built in alarm checking processed during the execution of each record; control records like PID etc.; configuration tools for the processing engine. The flexible naming scheme and the default display and alarm properties for each record ease the connection between the operator tools and the IOC’s. The flexible data acquisition supports the poll mode as well as the publishsubscribe mode. The latter reduces the traffic drastically.ConclusionsDepending on the size and the requirements for a controls project the combination of commercial solutions and solutions based on a collaborative approach is possible in any rate between 0 and 100 percent. This applies for all levels from implementation to long term support. Special requirements on safety issues or a lack of manpower might turn the scale commercial. The necessity to interface special hardware, special timing requirements, the ‘having the code in my hands’ argument or the initial costs for commercial solutions will turn the scale collaborative. As long as collaborative approaches like EPICS stay up to date and run as stable and robust as commercial solutions, both will keep their position in the controls world in a complementary symbiosis.译文工业控制系统和协同控制系统当今的控制系统被广泛运用于许多领域。
指纹识别(Fingerprint Recognition)PPT英文版

Development Prospect and Application Area
Fingerprint anti-theft device (汽 车指纹防盗装置)
Capture fingerprints through the optical imaging(光学成像) or semiconductor sensor (半导体感应)
Fingerprint Recognition System
Step 1
Step 2 Step 3
•Fingerprint image acquisition and preprocessing
•Extracting the required feature point
•Matching information with database and outputting the fingerprint processing results
Development Prospect and Application Area
Become civilian
Replace the identification code and password
Development Prospect and Application Area Combine the feature of the biological recognition technology and the fingerprint. Face recognition
Two important characteristic of fingerprint were found in 19th century.
Different finger has different range pattern.
电子信息工程专业外文翻译--一个混杂的指纹自动识别系统(中文)
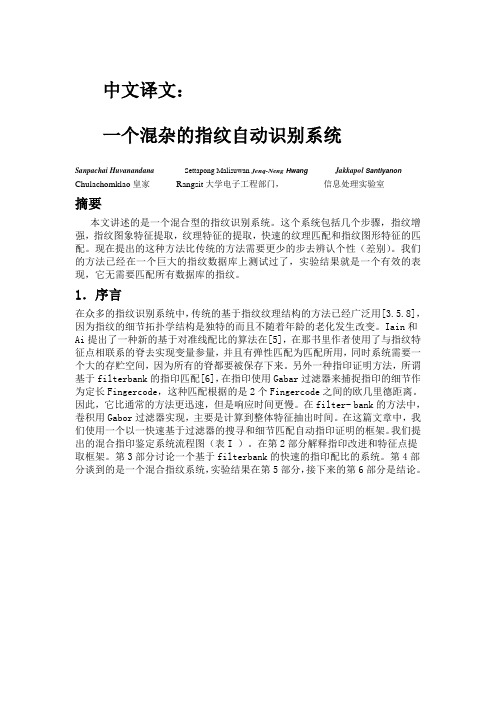
中文译文:一个混杂的指纹自动识别系统Sanpachai Huvanandana Settapong Malisuwan Jenq-Neng Hwang Jakkapol Santiyanon Chulachomklao皇家Rangsit大学电子工程部门,信息处理实验室摘要本文讲述的是一个混合型的指纹识别系统。
这个系统包括几个步骤,指纹增强,指纹图象特征提取,纹理特征的提取,快速的纹理匹配和指纹图形特征的匹配。
现在提出的这种方法比传统的方法需要更少的步去辨认个性(差别)。
我们的方法已经在一个巨大的指纹数据库上测试过了,实验结果就是一个有效的表现,它无需要匹配所有数据库的指纹。
1.序言在众多的指纹识别系统中,传统的基于指纹纹理结构的方法已经广泛用[3.5.8],因为指纹的细节拓扑学结构是独特的而且不随着年龄的老化发生改变。
Iain和Ai提出了一种新的基于对准线配比的算法在[5],在那书里作者使用了与指纹特征点相联系的脊去实现变量参量,并且有弹性匹配为匹配所用,同时系统需要一个大的存贮空间,因为所有的脊都要被保存下来。
另外一种指印证明方法,所谓基于filterbank的指印匹配[6],在指印使用Gabar过滤器来捕捉指印的细节作为定长Fingercode,这种匹配根据的是2个Fingercode之间的欧几里德距离。
因此,它比通常的方法更迅速,但是响应时间更慢。
在filter- bank的方法中,卷积用Gabor过滤器实现,主要是计算到整体特征抽出时间。
在这篇文章中,我们使用一个以一快速基于过滤器的搜寻和细节匹配自动指印证明的框架。
我们提出的混合指印鉴定系统流程图(表I )。
在第2部分解释指印改进和特征点提取框架。
第3部分讨论一个基于filterbank的快速的指印配比的系统。
第4部分谈到的是一个混合指纹系统,实验结果在第5部分,接下来的第6部分是结论。
图表1.一个混杂的指纹识别系统2.改进和特征点提取2.1 (LRO)估计优选的脊方向被定义为显示统治地方结构取向的定向领域。
- 1、下载文档前请自行甄别文档内容的完整性,平台不提供额外的编辑、内容补充、找答案等附加服务。
- 2、"仅部分预览"的文档,不可在线预览部分如存在完整性等问题,可反馈申请退款(可完整预览的文档不适用该条件!)。
- 3、如文档侵犯您的权益,请联系客服反馈,我们会尽快为您处理(人工客服工作时间:9:00-18:30)。
摘要:本文拟在提出一种可以区分protocol指纹识别的方法,用帧描述指纹识别代替建立帧系统获得主机信息与系统配对从而分辨出主机操作系统的类别。
实验的结果表明这种方法能够有效的辨别操作系统,这一方法比其他例如nmap 和xprobe的系统更为隐秘。
关键词:传输控制)协议/ 协议指纹识别操作系统辨别远程主机的操作系统,这是一个很重要的领域。
了解主机操作系统可以分析和获取一些信息,例如记忆管理,CPU的类型。
这些信息对于计算机网络的攻击与防御非常重要。
主要的辨别是通过TCP/IP指纹识别来完成的。
几乎所有的操作系统的定制他们自己的协议栈都通过以下的RFC。
这种情况导致一个实例,每个协议栈会有细节上的不同。
这些不同的细节就是所知道的使辨别操作系统称为可能的指纹识别。
Nmap、Queso在传输层里使用指纹。
他们将特殊数据包发送到目标并分析返回的数据包,在指纹库中寻找配对的指纹,以便得到的结果。
指纹库中的信息受指定的探测信息的影响.很难区分类似的操作系统(例如:windows98/2000/xp)Xprobe主要是利用ICMP协议,这是利用五种包来识别操作系统。
它能够提供的在所有可能的情况下确实是操作系统的概率。
主要不足是它过分依赖ICMP协议议定书。
SYNSCAN是在应用协议中与目标主机联系时,使用的一些典型的指纹识别方法。
指纹库对在这个领域有限制。
Ring,Ttbit查明操作系统所使用TCP / IP 的性能特点。
因为这种性能受网络环境极大。
其结果往往是不完全确定的。
文献分析资料中的行动而获得的拦截(如一些同步的要求,一个封闭的端口如何响应连接请求)。
虽然这种方式是有效,它在少数特定操作系统区分上述的各种系统,都没有完整的描述指纹系统,引起他们进行分辨的主要是依靠部分的TCP/IP。
这篇文章的目的就是要简绍一种新的方法来解决这些问题。
它们都被吓跑的方式来描述指纹的OS integrallty ,造成诉讼程序的确定只能依靠部分TCP / IP协议。
本文提出了一种新的方法来解决这一问题:它是指纹操作系统,是通过利用科技来获取一些信息,获取的信息的一些技术,查明操作系统。
第二章我们提出一些基本的方法的概念,第三章用帧技术来提出描述和匹配协定指纹,第四章,是完成这种方法的算法,第五部分,利用实验来验证他的有效有效性并分析结果最后第六部分是总结全文,及未来的发展方向。
该程序是为了获取信息,提取指纹和匹配的指纹库里的记录,以便知道类型。
本节确定获取信息的方法,采取的做法和通信的状况,还区分指纹。
这些工作为下一节如何建立一个帧系统来识别指纹做好准备要插入“表”或“数字” ,请粘贴下文所述数据。
所有表格和数字必须使用连续数字( 1 , 2 , 3等),并有一个标题放在下面的数字(“ FigCaption ” )或在表的上面(“ FigTalbe ” )用8pt字体和从风格兰中下拉菜单中的类别中选择指定的样式“标题”。
在本文中,我们提出了一个方法,以确定操作系统的远程主机。
该方法使用帧技术来识别指纹,弥补探针和监控获得的信息和从资料中摘取信息来与指纹库中的匹配,最后识别操作系统。
通过实验,该方法与nmap and xprobe. 相比,能准确识别远程的主机的操作系统。
在未来,我们计划为每个种操作系统汇编更多的指纹,使算法(规则系统)将更加智能化,以提高识别的精度(准确性)。
This paper present a method that classify the fingerprint of protocol(电脑之间通信与资料传送所遵守的规则), use the frame to describe the fingerprint in order to create the frame system, get the information of host(主机)to match the system to identify the type of OS inremote host. Result from experimental(实验性的)appears that this method can identify the OS effectively, the action of is more secretly than other systems such as nmap and xprobe (x-probe:X探针).Key words: TCP/IP Fingerprint OSIt is an important field that identify what OS in remote host. Mastering the OS can analyse and acquire some information such as memory management、the kind of CPU. These information is important for computer network attack and computer network defense.The main way to identify is through the TCP/IP fingerprint to finish. Nearly all kind of OS customize(定制)their own’s protocol stack by following the RFC. This instance cause the fact that every protocol stack has some different details during implementing. These details are known as fingerprint which make it possible to identify the OS .Nmap、Queso[1] use the fingerprint in transport layer. They send the particular packets to the target and analyse the retured packets, matching the fingerprint in the fingerprint warehouse in order to get the result. The information in the warehouse is affected by the specified message for probing. It hardly to distinguish the similar OS (eg.windows98/2000/xp).Xprobe[2] mainly use the ICMP which make use of five kinds of packets in ICMP to identify OS. It can give the probability of all possible situation which maybe the indeed OS. The main shortage is it excessively depend on ICMP Protocol.SYNSCAN[3] use some typical fields’ fingerprint to identify when it communicaties with target host in application protocol. The warehouse of fingerprint have limited types of field.Ring 、Ttbit[5][6] identify the OS using the performance character of TCP/IP. Because this kind of character is affected by network environment greatly. The result is often not exactly.Literature[7] analysis the action in messages which are acquired through interception(eg. The number of SYN request, a closed port how to response a connection request).Although this way is availability, it only distinguish a few given OSAbove all the kinds of system, they all be scare of a way to describe the fingerprint of OS integrallty, which cause the proceeding of identify only depend on a part of TCP/IP . This paper propose a new method to resolve the problem: it uniformly the fingerprint of OS, acquire the message by some technology, identify the OS at last.The rest of the paper is organized as followed: Section Ⅱ we present based concept of this method. Section Ⅲpresent how to describe and match the protocol fingerprint using frame technology. Section Ⅳpresent an algorithm to implement the method and Section Ⅴuseexperiment to validate its effectiveness and analysis the result. Finally Section Ⅵ present the concluding remark and possible future work.The proceeding of identify is to acquire message, extract the fingerprint and match the record of fingerprint warehouse, in order to know the type. This section define the measure which are to acquire message, the action and status of communication, also classify the fingerprint. These work are all prepared for the next section which how to built a frame system describing the fingerprint.To insert “Tables” or “Figures”, please paste the data as stated below. All tables and figures must be given sequential numbers (1, 2, 3, etc.) and have a caption placed below the figure (“FigCaption”) or above the table(“FigTalbe”) being described, using 8pt font and ple ase make use of the specified style “caption” from the drop-down menu of style categories ConclusionIn this paper, we have presented a method for identifying OS of remote host. The method use frame technology to express the fingerprint, make up of Probe and Monitor to get message and abstract the information from the message to match the warehouse of fingerprint, identify the OS at last. Through experiment, this method can exactly identify the OS of remote hose with more secretly and less number of packets comparing with nmap and xprobe.In the future, we plan to collect more fingerprint for each kind of OS, make the algorithm(规则系统) to be more intelligent, in order to improve the precision(准确性) of identify.无论是日本媒体的打算激发一种民族自强不息的人,或者是美国媒体进一步宣扬“中国威胁论”总之,最近,一些外国媒体一直鼓吹中国已经成为“世界工厂” 。