科学文献
科技文献的定义
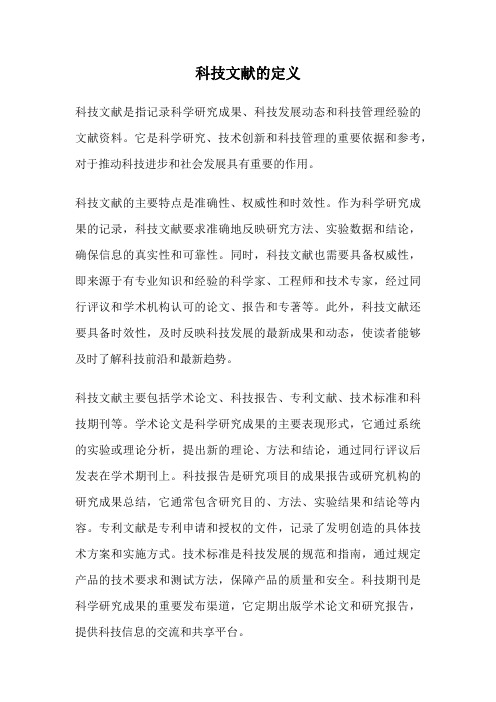
科技文献的定义科技文献是指记录科学研究成果、科技发展动态和科技管理经验的文献资料。
它是科学研究、技术创新和科技管理的重要依据和参考,对于推动科技进步和社会发展具有重要的作用。
科技文献的主要特点是准确性、权威性和时效性。
作为科学研究成果的记录,科技文献要求准确地反映研究方法、实验数据和结论,确保信息的真实性和可靠性。
同时,科技文献也需要具备权威性,即来源于有专业知识和经验的科学家、工程师和技术专家,经过同行评议和学术机构认可的论文、报告和专著等。
此外,科技文献还要具备时效性,及时反映科技发展的最新成果和动态,使读者能够及时了解科技前沿和最新趋势。
科技文献主要包括学术论文、科技报告、专利文献、技术标准和科技期刊等。
学术论文是科学研究成果的主要表现形式,它通过系统的实验或理论分析,提出新的理论、方法和结论,通过同行评议后发表在学术期刊上。
科技报告是研究项目的成果报告或研究机构的研究成果总结,它通常包含研究目的、方法、实验结果和结论等内容。
专利文献是专利申请和授权的文件,记录了发明创造的具体技术方案和实施方式。
技术标准是科技发展的规范和指南,通过规定产品的技术要求和测试方法,保障产品的质量和安全。
科技期刊是科学研究成果的重要发布渠道,它定期出版学术论文和研究报告,提供科技信息的交流和共享平台。
科技文献的利用可以促进科学研究的发展和技术创新的进步。
科学家和工程师可以通过查阅和分析科技文献,了解前人的研究成果和经验,避免重复劳动和错误,为自己的研究工作奠定基础。
科技管理人员可以通过研究科技文献,掌握科技发展的动态和趋势,制定科技政策和计划,引导科技创新和产业升级。
工程师和技术人员可以通过科技文献,学习新的技术方法和应用案例,提高自己的专业能力和技术水平。
科技文献是科学研究、技术创新和科技管理的重要资源和工具。
科技工作者应该重视科技文献的收集和利用,不断更新自己的知识和技能,推动科技进步和社会发展。
同时,科技出版机构和科技管理部门也应该加强对科技文献的管理和服务,提高科技文献的质量和影响力,为科技创新提供有力支持。
高中生如何有效阅读科学文献
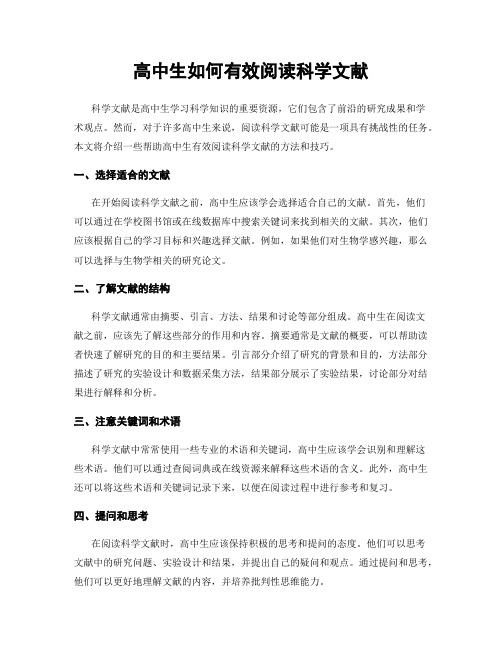
高中生如何有效阅读科学文献科学文献是高中生学习科学知识的重要资源,它们包含了前沿的研究成果和学术观点。
然而,对于许多高中生来说,阅读科学文献可能是一项具有挑战性的任务。
本文将介绍一些帮助高中生有效阅读科学文献的方法和技巧。
一、选择适合的文献在开始阅读科学文献之前,高中生应该学会选择适合自己的文献。
首先,他们可以通过在学校图书馆或在线数据库中搜索关键词来找到相关的文献。
其次,他们应该根据自己的学习目标和兴趣选择文献。
例如,如果他们对生物学感兴趣,那么可以选择与生物学相关的研究论文。
二、了解文献的结构科学文献通常由摘要、引言、方法、结果和讨论等部分组成。
高中生在阅读文献之前,应该先了解这些部分的作用和内容。
摘要通常是文献的概要,可以帮助读者快速了解研究的目的和主要结果。
引言部分介绍了研究的背景和目的,方法部分描述了研究的实验设计和数据采集方法,结果部分展示了实验结果,讨论部分对结果进行解释和分析。
三、注意关键词和术语科学文献中常常使用一些专业的术语和关键词,高中生应该学会识别和理解这些术语。
他们可以通过查阅词典或在线资源来解释这些术语的含义。
此外,高中生还可以将这些术语和关键词记录下来,以便在阅读过程中进行参考和复习。
四、提问和思考在阅读科学文献时,高中生应该保持积极的思考和提问的态度。
他们可以思考文献中的研究问题、实验设计和结果,并提出自己的疑问和观点。
通过提问和思考,他们可以更好地理解文献的内容,并培养批判性思维能力。
五、扩展阅读阅读科学文献不仅限于一篇文章,高中生可以通过扩展阅读来深入了解某个主题。
例如,他们可以查阅相关的综述文章、书籍或其他研究论文,以获取更全面的知识。
扩展阅读还可以帮助高中生了解当前研究领域的热点问题和最新进展。
六、记录和总结在阅读科学文献时,高中生应该养成记录和总结的习惯。
他们可以使用笔记本或电子工具来记录重要的观点、关键词和疑问。
此外,他们还可以将阅读的内容进行总结和归纳,以便后续的学习和复习。
如何阅读科学文献
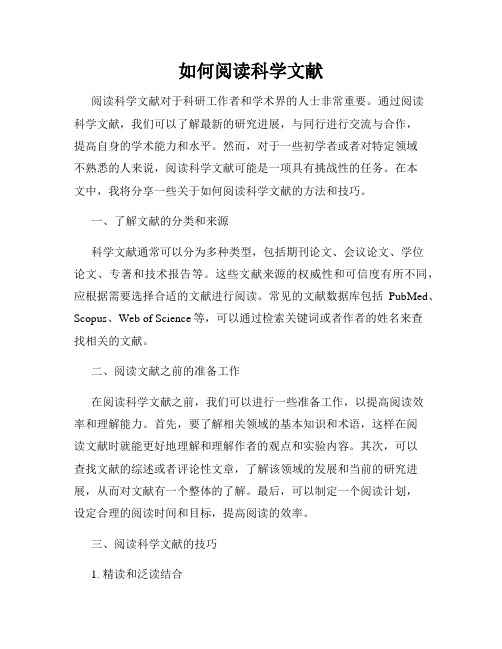
如何阅读科学文献阅读科学文献对于科研工作者和学术界的人士非常重要。
通过阅读科学文献,我们可以了解最新的研究进展,与同行进行交流与合作,提高自身的学术能力和水平。
然而,对于一些初学者或者对特定领域不熟悉的人来说,阅读科学文献可能是一项具有挑战性的任务。
在本文中,我将分享一些关于如何阅读科学文献的方法和技巧。
一、了解文献的分类和来源科学文献通常可以分为多种类型,包括期刊论文、会议论文、学位论文、专著和技术报告等。
这些文献来源的权威性和可信度有所不同,应根据需要选择合适的文献进行阅读。
常见的文献数据库包括PubMed、Scopus、Web of Science等,可以通过检索关键词或者作者的姓名来查找相关的文献。
二、阅读文献之前的准备工作在阅读科学文献之前,我们可以进行一些准备工作,以提高阅读效率和理解能力。
首先,要了解相关领域的基本知识和术语,这样在阅读文献时就能更好地理解和理解作者的观点和实验内容。
其次,可以查找文献的综述或者评论性文章,了解该领域的发展和当前的研究进展,从而对文献有一个整体的了解。
最后,可以制定一个阅读计划,设定合理的阅读时间和目标,提高阅读的效率。
三、阅读科学文献的技巧1. 精读和泛读结合对于篇幅较长或者对自己比较重要的文献,可以进行精读。
在精读时,要认真阅读摘要和介绍部分,了解研究的背景和目的,然后逐段、逐句进行仔细阅读,理解作者的实验设计和研究结果。
在阅读过程中,可以做一些标记或者写下关键点,以便于后续的回顾和整理。
对于篇幅较长或者对自己不是很重要的文献,可以进行泛读。
在泛读时,可以关注文献的结构、图表和重点段落,了解作者的主要观点和研究结果。
泛读可以帮助快速获取信息,筛选出对自己研究有用的文献。
2. 多角度阅读在阅读科学文献时,要注意从多个角度进行思考和分析。
可以思考文献的创新点、实验设计、结果解释以及与其他相关文献的联系。
可以尝试用自己的话总结和表达作者的观点和结论,以帮助更好地理解文献内容。
科学文献的名词解释

科学文献的名词解释
文献:
1. Abstract
2. Bibliographic Database
3. Editor
4. Index
一、Abstract:
抽象是科学文献中概述性段落的内容,即文章的摘要,它在文献的开头,能简要介绍文献的内容和目的,是科学文献查找、分析和利用的重要基础。
Abstract由一般性问题、技术方法、重要结果、结论和指出事实的综述性的评论组成,概述文献的内容及方法,是检索文献信息的重要依据。
二、Bibliographic Database:
文献数据库是以文献数据为基础建立起来的文献信息体系,其主要内容是存储文献信息(如书籍、期刊、报纸、图书、报告摘要、摘录、贴文等)的元数据,并提供跨文献的快速检索的功能。
一般而言,文献数据库由代表文献的描述性元数据(如题名、作者、出版社、出版日期等)和用于检索所建立的全文索引组成。
三、Editor:
编辑是指组织、审查和整理文献内容,以便发表的这类编辑服务活动。
编辑可以编排、修改、撰写、组织文献,编辑从文献撰写、组织和修订等方面起着协调作用,以确保出版物的质量。
四、Index:
索引是科学文献检索的一个重要技术工具,它的主要目的是使读者能够轻松找到所需要的信息。
索引包括有关文献的词汇表,能够提供有用的参考资料,而无需检查整个文献的文本内容。
另外,索引还可以帮助读者了解文献的整体框架,有利于从文献中快速获取信息。
十大科技文献源
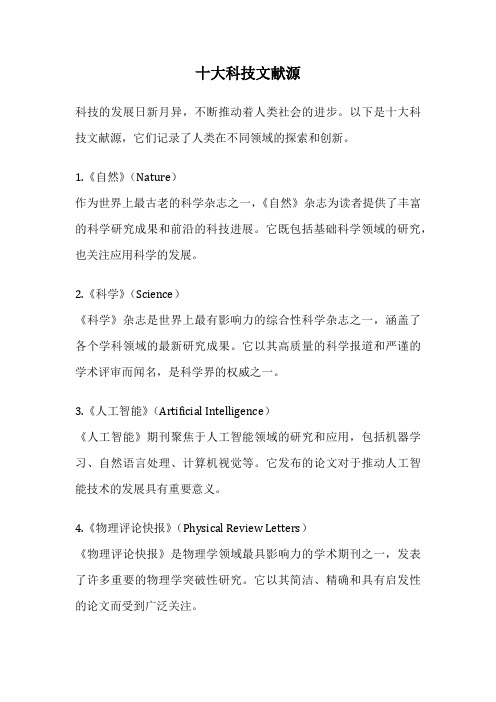
十大科技文献源科技的发展日新月异,不断推动着人类社会的进步。
以下是十大科技文献源,它们记录了人类在不同领域的探索和创新。
1.《自然》(Nature)作为世界上最古老的科学杂志之一,《自然》杂志为读者提供了丰富的科学研究成果和前沿的科技进展。
它既包括基础科学领域的研究,也关注应用科学的发展。
2.《科学》(Science)《科学》杂志是世界上最有影响力的综合性科学杂志之一,涵盖了各个学科领域的最新研究成果。
它以其高质量的科学报道和严谨的学术评审而闻名,是科学界的权威之一。
3.《人工智能》(Artificial Intelligence)《人工智能》期刊聚焦于人工智能领域的研究和应用,包括机器学习、自然语言处理、计算机视觉等。
它发布的论文对于推动人工智能技术的发展具有重要意义。
4.《物理评论快报》(Physical Review Letters)《物理评论快报》是物理学领域最具影响力的学术期刊之一,发表了许多重要的物理学突破性研究。
它以其简洁、精确和具有启发性的论文而受到广泛关注。
5.《细胞》(Cell)《细胞》杂志是细胞生物学和分子生物学领域的顶级期刊之一,报道了该领域的最新研究成果和突破性发现。
它对于理解生命的基本机制和疾病的发生机理具有重要意义。
6.《计算机视觉国际会议》(Conference on Computer Vision and Pattern Recognition)计算机视觉是人工智能领域的一个重要分支,该会议是该领域最重要的学术会议之一。
它汇集了来自全球的顶尖研究人员,分享了最新的计算机视觉技术和应用。
7.《美国国家科学院院刊》(Proceedings of the National Academy of Sciences)《美国国家科学院院刊》是美国国家科学院的官方期刊,发表了各个学科领域的重要研究成果。
它是一本跨学科的期刊,涵盖了自然科学、社会科学和工程技术等领域。
8.《医学》(The Lancet)《医学》杂志是世界上最具影响力的医学期刊之一,发表了许多重要的医学研究。
科学阅读的方法和技巧
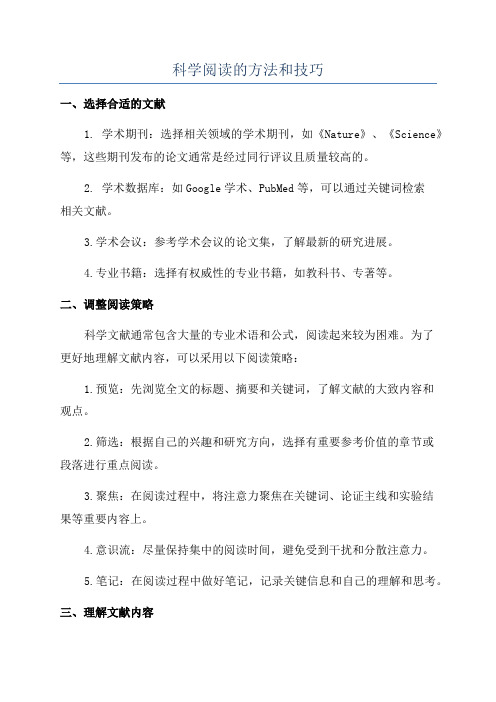
科学阅读的方法和技巧一、选择合适的文献1. 学术期刊:选择相关领域的学术期刊,如《Nature》、《Science》等,这些期刊发布的论文通常是经过同行评议且质量较高的。
2. 学术数据库:如Google学术、PubMed等,可以通过关键词检索相关文献。
3.学术会议:参考学术会议的论文集,了解最新的研究进展。
4.专业书籍:选择有权威性的专业书籍,如教科书、专著等。
二、调整阅读策略科学文献通常包含大量的专业术语和公式,阅读起来较为困难。
为了更好地理解文献内容,可以采用以下阅读策略:1.预览:先浏览全文的标题、摘要和关键词,了解文献的大致内容和观点。
2.筛选:根据自己的兴趣和研究方向,选择有重要参考价值的章节或段落进行重点阅读。
3.聚焦:在阅读过程中,将注意力聚焦在关键词、论证主线和实验结果等重要内容上。
4.意识流:尽量保持集中的阅读时间,避免受到干扰和分散注意力。
5.笔记:在阅读过程中做好笔记,记录关键信息和自己的理解和思考。
三、理解文献内容科学文献通常采用科学语言和专业术语,为了更好地理解文献内容,可以采用以下方法:1.查阅词典和参考书:查阅相关课本、词典、参考书籍等,弄清楚不熟悉的术语和概念。
2.加深背景知识:扩大自己的科学背景知识,了解相关领域的基本原理和理论框架。
3.多角度理解:通过阅读多个文献,了解不同研究观点和方法,从不同角度思考和分析问题。
4.精确解释:将复杂的概念或内容用自己的语言重新解释一遍,以确保自己理解透彻。
四、分析论证逻辑科学文献通常有一定的逻辑结构,分析论证逻辑有助于深入理解文献内容。
可以采用以下方法:1.总结主旨:通过阅读摘要、引言和结论等部分,总结文献的主旨和观点。
4.反思批判:对文献的内容进行批判性思考,发现可能存在的问题和不足之处。
五、扩展思考和应用1.感知科学思维:思考作者是如何发现问题、提出假设、设计实验和得出结论的,借鉴科学思维方法。
2.拓展思考:将文献中的观点与自己的观点进行比较和对比,思考存在的差异和原因,并以此为基础进行拓展思考。
科学文献阅读技巧详解
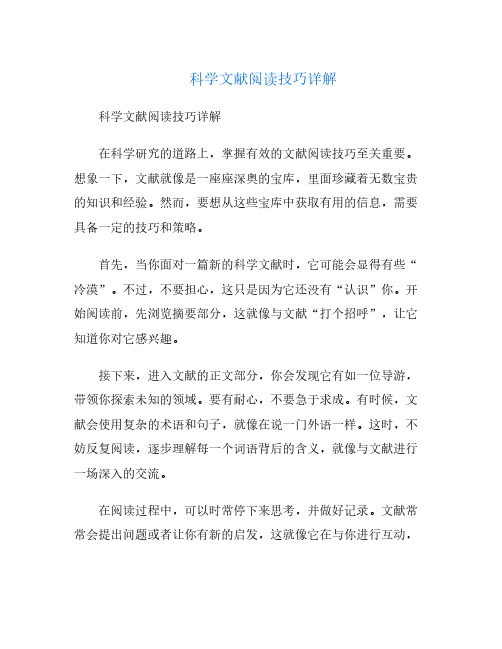
科学文献阅读技巧详解科学文献阅读技巧详解在科学研究的道路上,掌握有效的文献阅读技巧至关重要。
想象一下,文献就像是一座座深奥的宝库,里面珍藏着无数宝贵的知识和经验。
然而,要想从这些宝库中获取有用的信息,需要具备一定的技巧和策略。
首先,当你面对一篇新的科学文献时,它可能会显得有些“冷漠”。
不过,不要担心,这只是因为它还没有“认识”你。
开始阅读前,先浏览摘要部分,这就像与文献“打个招呼”,让它知道你对它感兴趣。
接下来,进入文献的正文部分,你会发现它有如一位导游,带领你探索未知的领域。
要有耐心,不要急于求成。
有时候,文献会使用复杂的术语和句子,就像在说一门外语一样。
这时,不妨反复阅读,逐步理解每一个词语背后的含义,就像与文献进行一场深入的交流。
在阅读过程中,可以时常停下来思考,并做好记录。
文献常常会提出问题或者让你有新的启发,这就像它在与你进行互动,促使你深入思考。
记得,做好笔记非常重要,这有助于你将碎片化的信息整理成有条理的知识体系。
此外,不要忽视文献中的图表和数据。
它们就像文献的“视觉演示”,通过直观的方式展示研究结果。
深入理解图表背后的数据,有助于你更全面地把握文献的核心内容。
最后,要保持批判性思维。
就像与一位智者交谈一样,不要轻易接受文献中的每一个观点。
要学会提出问题,评估实验设计的有效性,并思考研究结果的可能局限性。
这样,你才能更好地理解文献,甚至为未来的研究提供新的思路和方法。
总结来说,科学文献阅读并非一项简单的任务,它需要技巧和耐心。
通过与文献建立良好的互动关系,你将能够开启一段充满发现和启发的学术之旅。
不断地练习和改进阅读技巧,相信你定能在科学研究的道路上越走越远。
中国科学引用文献格式

中国科学(Science China)的引用文献格式通常遵循国际通用的科技文献引用规范,以下是一种常见的引用格式:
期刊文章:
作者. 文章标题. 刊名, 年份, 卷号(期号): 起始页码-结束页码.
例如:
王明, 李华, 赵丽. 量子通信的新进展. 科学通报, 2021, 66(12): 1234-1256.
书籍:
作者. 书名. 版本(初版可省略). 出版地: 出版社, 出版年份.
例如:
张三. 物理学导论. 第3版. 北京: 高等教育出版社, 2018.
学位论文:
作者. 论文标题. 学位级别. 授予单位, 年份.
例如:
李四. 量子计算的研究. 博士学位论文. 清华大学, 2022.
请注意,具体的引用格式可能会根据期刊或出版机构的要求有所不同,因此在撰写论文时应参照目标期刊或出版社的投稿指南进行调整。
科学文献阅读的注意事项

科学文献阅读的注意事项科学文献阅读的注意事项在探索科学文献的广阔海洋时,如同旅行者在陌生土地上航行。
每一篇文献都是知识的岛屿,而你则是一位探险家,渴望在这些岛屿上发现宝藏。
然而,要想从这些文献中获得宝贵的知识和洞见,你需要具备一定的技巧和注意事项。
首先,作为一位文献的探险家,你应该学会如何“与文献对话”。
文献有时像是一个沉默的学者,蕴藏着无限的智慧。
当你打开一篇文献时,不要仅仅停留在表面信息的浏览上。
要善于提出问题,文献便会像是回答你的问题一样,逐渐揭示其内涵和深度。
其次,了解文献的“语言和风格”也是非常重要的。
每一篇文献都有其独特的表达方式和专业术语。
有时候,文献可能会使用复杂的句子结构或专业术语,这并不意味着你需要被吓倒。
相反,你可以像是学习一门新语言一样,逐步熟悉并理解这些语言的规则和习惯用法。
此外,保持“批判性思维”的能力也是阅读科学文献不可或缺的技能。
作为文献的探险家,你需要时刻保持怀疑和探索的精神。
不要轻信一切,而是要学会分析和评估文献的内容。
提出质疑,寻找证据,这样才能真正理解文献背后的意图和科学观点。
进一步地,要善于“比较和综合”不同的文献。
科学文献世界就像是一个多面体,不同的角度和视角可能带来不同的认知和发现。
因此,阅读多篇相关的文献,比较它们之间的异同,从中获取更为全面和深入的理解。
最后,永远不要忘记“记录和引用”的重要性。
在你的探险旅程中,收集到的每一个知识宝藏都应该被妥善保存和记录。
正确引用文献不仅是对知识贡献者的尊重,也是维护学术诚信的基础。
综上所述,阅读科学文献不仅是获取知识的途径,更是一种探险和发现的过程。
作为一名文献的探险家,你需要具备探索精神、批判思维、比较能力和记录技巧。
只有这样,你才能在科学的海洋中畅行无阻,发现属于你的知识宝藏。
科技文献定义

科技文献定义科技文献是指记录和传播科学技术研究成果的文献资源。
它包括了科学研究的论文、学术期刊、会议论文集、科技报告、技术标准、专利文献等形式。
科技文献是科学研究和技术创新的重要载体,对于推动科技进步和促进学术交流具有重要意义。
科技文献的特点主要体现在以下几个方面。
首先,科技文献以学术性和专业性为特征,通常由专业学者、科研机构和科技企业发表或出版。
其次,科技文献具有较高的可信度和权威性,经过严格的同行评审程序,确保了内容的科学性和准确性。
再次,科技文献具有时效性,记录了最新的科学研究成果和技术发展动态。
最后,科技文献通过各种渠道和方式进行传播,包括印刷出版、电子期刊、学术会议、数据库等形式。
科技文献的编写和发布过程通常包括以下几个环节。
首先,科学研究人员进行实验和研究,形成研究成果。
然后,他们将研究成果整理和撰写成论文的形式。
在撰写过程中,他们需要对已有的相关文献进行综述和引用,以确保研究的完整性和可信度。
接下来,研究人员选择合适的学术期刊或会议进行投稿。
投稿后,编辑和审稿人会对论文进行评审和修改意见,最终确定是否接受和出版论文。
对于已经出版的科技文献,读者可以通过各种渠道获取和阅读,包括图书馆、互联网、数据库等。
科技文献在科学研究和技术创新中起着重要的作用。
首先,科技文献记录了科学研究的过程和结果,为其他研究人员提供了重要的参考和借鉴。
其次,科技文献促进了学术交流和合作,科研人员可以通过阅读文献了解最新的研究进展,开展合作研究项目。
同时,科技文献也为科技政策制定和决策提供了参考依据,对于推动科技进步和社会发展具有重要意义。
然而,科技文献的管理和利用也面临一些挑战。
首先,科技文献数量庞大,涉及的学科和领域也非常广泛,如何有效管理和检索文献成为了一个重要问题。
其次,科技文献的版权和知识产权保护也是一个亟待解决的问题,如何平衡科研人员的知识共享和出版机构的经济利益是一个难题。
此外,科技文献的语言和专业性也限制了一部分人群的阅读和理解,如何提高科技文献的传播和普及成为了一个重要课题。
科学探索的科学文献与资源获取

科学探索的科学文献与资源获取在科学研究中,科学文献与资源的获取是非常重要的一环。
科学研究者需要准确、全面地了解各个领域的研究进展,以便能够针对性地开展自己的研究工作。
本文将介绍一些科学文献与资源获取的途径和方法,以帮助科研人员更好地开展科学探索。
1. 学术期刊学术期刊是科学研究者常用的资源之一。
在学术期刊中,研究者可以了解到最新的研究成果、方法和理论。
常见的学术期刊有《Science》、《Nature》等。
获取学术期刊的方法有多种,可以通过图书馆提供的电子数据库、在线期刊数据库或者直接购买期刊订阅服务等途径。
2. 学术会议学术会议是科学研究者交流和分享研究成果的重要场所。
研究者不仅可以借此结识同行,并与他们进行深入的学术讨论,还可以听取其他研究者的报告和演讲,了解最新的研究动态。
科学研究者可以通过查阅相关会议的官方网站、论文集或者参与会议的身份来获取相关资源。
3. 在线学术资源随着互联网的普及,许多学术资源已经通过在线平台提供给研究者使用。
一些知名机构和大学提供了免费的学术资源数据库,例如:Google学术、中国知网、PubMed等。
在这些平台上,研究者可以搜索到各个领域的学术论文、研究报告和会议论文等,并且可以免费获取或付费购买。
4. 合作与交流与其他研究者的合作和交流也是获取科学文献和资源的一种重要途径。
研究者可以通过与同行的合作进行资源的共享,例如相互交换自己的研究成果和论文。
此外,加入学术组织、参加学术研讨会等也是获取科学文献和资源的良好机会。
5. 专业图书馆和研究机构专业图书馆和研究机构通常都有丰富的学术资源和图书馆藏。
科研人员可以通过办理借阅证或者到图书馆进行现场查阅的方式来获取所需的科学文献和资源。
此外,一些大型研究机构还会提供科学文献的数字化资源或者专门的研究资源库,供科研人员使用。
总之,科学文献和资源的获取对于科学探索来说至关重要。
研究者可以通过学术期刊、学术会议、在线学术资源、合作与交流以及专业图书馆和研究机构等多种途径,获取到最新的研究成果和资源。
学习窍门如何有效阅读科学文献

学习窍门如何有效阅读科学文献有效阅读科学文献的学习窍门科学文献是科研工作者获取最新科研进展、提升科研水平的重要来源。
然而,由于科学文献既深入又繁杂,对于普通读者来说进行有效阅读并非易事。
本文将介绍一些学习窍门,帮助读者有效阅读科学文献。
一、清楚阅读目的在开始阅读科学文献之前,务必明确阅读的目的。
例如,是为了了解某一具体问题的最新研究进展,还是为了获取某方面的背景知识。
明确阅读目的有助于筛选和整理重要信息,提高阅读效率。
二、抓住重点信息科学文献通常包含大量的数据、实验方法和讨论等内容。
为了有效阅读,可以通过以下方式抓住重点信息:1.注重摘要部分:摘要是论文内容的提炼,通过仔细阅读摘要,可以初步了解论文的主要观点、方法和结论,帮助读者判断是否需要深入阅读全文。
2.关注引言和讨论部分:这两个部分通常包含了研究的背景、意义、现有研究进展以及未来研究方向等信息。
通过阅读这些部分,可以更好地了解文献的研究背景和意义。
3.快速浏览实验方法:对于非专业领域的读者来说,实验方法可能相对较难理解。
可以通过快速浏览实验方法来了解研究所用的技术、仪器等,以及实验方案是否合理可行。
三、跳过细节部分科学文献中会有许多细节部分,如大量的实验数据、图表、推导过程等。
对于一般读者来说,可以适当跳过这些细节,将重点放在主要观点、结论和讨论部分上。
当然,如果读者对某些实验数据或细节感兴趣,也可以深入阅读。
四、积极阅读批评意见科学文献的评审过程通常由同行专家进行,他们会在论文中提出不同的观点、建议和批评。
对于读者来说,阅读这些批评意见有助于深入了解研究的优点、不足之处以及可能的局限性。
同时,批评意见也能提醒读者对研究结果持有适度的怀疑态度。
五、及时记录关键信息在阅读科学文献时,及时记录关键信息非常重要。
可以通过以下方式进行记录:1.摘录关键语句:将论文中的重要观点、结论或者给出的实验数据等关键信息摘录下来,以备后续查阅和引用。
2.记笔记:将自己的思考、对文献的批评意见或者扩展思路等记录在笔记本中,这有助于巩固对文献的理解和记忆。
10本必读的科学文献,汇总了最新研究成果!
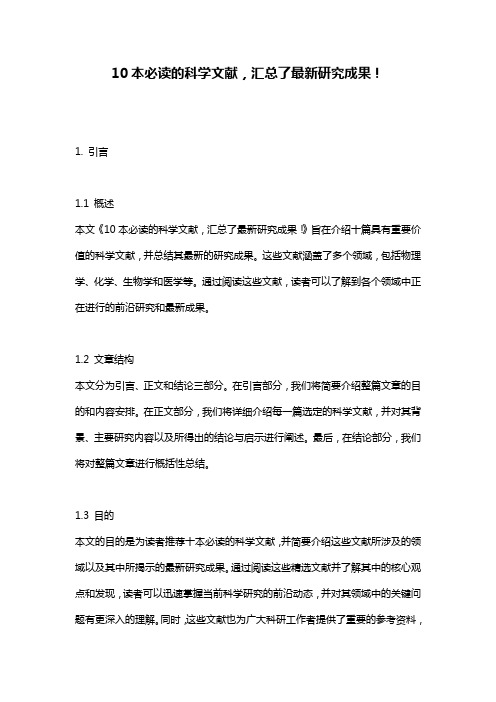
10本必读的科学文献,汇总了最新研究成果!1. 引言1.1 概述本文《10本必读的科学文献,汇总了最新研究成果!》旨在介绍十篇具有重要价值的科学文献,并总结其最新的研究成果。
这些文献涵盖了多个领域,包括物理学、化学、生物学和医学等。
通过阅读这些文献,读者可以了解到各个领域中正在进行的前沿研究和最新成果。
1.2 文章结构本文分为引言、正文和结论三部分。
在引言部分,我们将简要介绍整篇文章的目的和内容安排。
在正文部分,我们将详细介绍每一篇选定的科学文献,并对其背景、主要研究内容以及所得出的结论与启示进行阐述。
最后,在结论部分,我们将对整篇文章进行概括性总结。
1.3 目的本文的目的是为读者推荐十本必读的科学文献,并简要介绍这些文献所涉及的领域以及其中所揭示的最新研究成果。
通过阅读这些精选文献并了解其中的核心观点和发现,读者可以迅速掌握当前科学研究的前沿动态,并对其领域中的关键问题有更深入的理解。
同时,这些文献也为广大科研工作者提供了重要的参考资料,激发他们在相应领域进行更具创新性的研究。
希望本文能为读者提供有益启示,并促进科学知识的传播和应用。
2. 文献一: XXXX2.1 背景介绍文献一是XXXX,这是一个重要的科学研究领域,并且已经引起了广泛的关注。
在背景介绍中,我们将引入该领域的发展历程、当前研究状态以及该文献所涉及的主要问题和挑战。
2.2 主要研究内容在这一部分中,我们将详细介绍文献一的主要研究内容。
我们将阐述作者们所采用的方法、实验设计和数据分析等方面的细节。
此外,我们还将呈现他们的实验结果,并进行解读和讨论。
2.3 结论与启示结论与启示部分将总结并评估文献一的重要发现。
我们将强调这些发现对该领域的贡献以及其对相关领域进一步研究的意义。
同时,我们还会探讨存在的局限性和可能需要解决的未解决问题。
请注意,在填写“XXXX”的位置时,请提供实际的文献标题或描述,以便能够针对具体情境作出回答。
3. 文献二: XXXX3.1 背景介绍在本节中,我们将介绍文献二的背景信息。
如何有效地进行科学文献阅读

如何有效地进行科学文献阅读科学文献是科学研究的重要成果之一,阅读科学文献对于研究者来说至关重要。
然而,由于科学文献的数量庞大和内容的复杂性,有效地进行科学文献阅读变得至关重要。
本文将介绍一些有效的方法和技巧,帮助读者提高科学文献阅读的效率和质量。
一、准备阅读前的工作在开始阅读科学文献之前,需要进行一些准备工作。
首先,明确阅读的目的和需求。
根据自己的研究课题和问题,确定需要了解的领域和方向。
其次,建立一个合适的文献检索策略。
可以使用科学文献数据库,如Google Scholar、PubMed等来检索相关文献。
在检索时,使用关键词和逻辑运算符能够更准确地获得所需的文献。
最后,确定阅读的时间和地点。
选择一个安静、舒适的环境,避免干扰,以提高阅读的效果。
二、快速浏览和筛选文献在获取到一系列相关文献后,首先进行快速浏览和筛选。
阅读文献的标题、摘要和关键词,初步了解文献的内容和相关性。
根据自己的需求和判断,筛选出与研究主题相关且有可能有价值的文献。
这一步的目的是快速获得文献的概述,避免浪费时间在与研究无关的文献上。
三、详细阅读和理解文献在筛选出潜在有价值的文献后,进行详细阅读和理解。
首先,注意文献的结构和内容组织方式。
大部分科学文献一般包括引言、方法、结果和讨论等部分,对于不同的学科领域可能会有一些差异。
其次,重点关注文献的核心内容,有目的地进行阅读。
读者可以根据自己的需求,关注文献的方法和结果,也可以参考讨论部分来了解作者的观点和结论。
四、做好笔记和总结在阅读过程中,及时做好笔记和总结对于进一步理解和应用文献的内容至关重要。
读者可以使用摘要、标注和备注等工具,记录关键信息和自己的想法。
可以根据文献的不同部分,制作一个清晰的笔记和总结,以备后续查阅和参考。
同时,将文献与已有的知识体系整合,形成一个完整的理解框架。
五、批判性地思考和评估文献在阅读科学文献时,需要保持批判性思维,对文献进行评估和思考。
首先,评估文献的可靠性和可信度。
科学文献搜索技巧分享

科学文献搜索技巧分享
在现代信息化社会中,科学文献搜索技巧显得尤为重要。
作为信息的守门者,我将分享一些精湛的搜索技巧,帮助您更轻松地掌握和利用宝贵的学术资源。
首先,像一个聪明的导航员一样,关键词是您探索信息海洋的罗盘。
选择准确、具体的关键词,可以大大提高搜索效率。
试想一下,如果您要探索“人工智能在医疗中的应用”,将“人工智能”、“医疗”和“应用”作为您的引导词是何等明智!
其次,搜索引擎就像一位热心的图书管理员,总是乐于帮助您寻找宝藏。
利用搜索引擎的高级搜索功能,例如限定搜索范围、时间范围或特定文件类型,可以精准定位您需要的学术文献。
这就像是您的私人助理,为您过滤海量信息,呈现最相关的结果。
再者,不要忘记专业数据库就像您的私人图书馆,藏书丰富而精选。
许多学术期刊和数据库提供高质量的文献资源,如PubMed、IEEE Xplore、Google Scholar等。
熟悉并善用这些资源,将极大地扩展您的信息获取范围。
此外,订阅警报服务犹如一位忠实的侍者,时刻关注您的需求。
通过设定文献警报,您可以及时获得最新发表的相关研究成果,保持信息更新和领先。
最后,像一个不断学习的智者一样,不断改进搜索策略和技巧。
信息技术的进步迅猛,新的搜索工具和技术层出不穷。
保持好奇心和求知欲,参与学术交流与讨论,将不断提升您的文献搜索能力。
总而言之,科学文献搜索技巧是一门艺术,需要耐心、智慧和技巧。
希望以上分享的技巧能够为您在学术探索的道路上提供有力的支持,让信息的海洋不再是遥不可及的神秘迷宫,而是您探索知识的乐园和成就的源泉。
sci文献检索方法
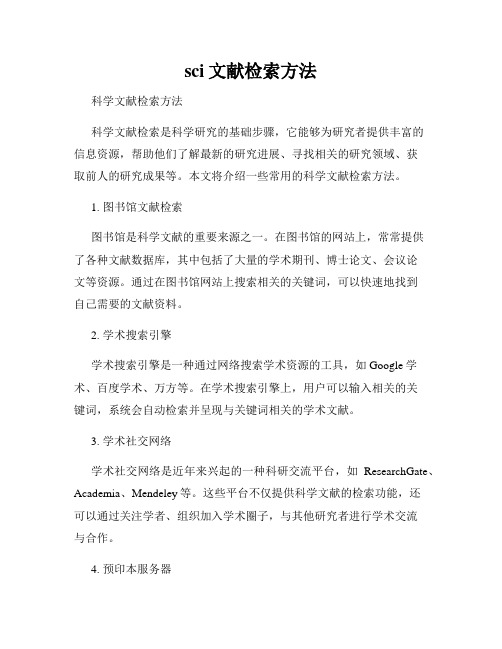
sci文献检索方法科学文献检索方法科学文献检索是科学研究的基础步骤,它能够为研究者提供丰富的信息资源,帮助他们了解最新的研究进展、寻找相关的研究领域、获取前人的研究成果等。
本文将介绍一些常用的科学文献检索方法。
1. 图书馆文献检索图书馆是科学文献的重要来源之一。
在图书馆的网站上,常常提供了各种文献数据库,其中包括了大量的学术期刊、博士论文、会议论文等资源。
通过在图书馆网站上搜索相关的关键词,可以快速地找到自己需要的文献资料。
2. 学术搜索引擎学术搜索引擎是一种通过网络搜索学术资源的工具,如Google学术、百度学术、万方等。
在学术搜索引擎上,用户可以输入相关的关键词,系统会自动检索并呈现与关键词相关的学术文献。
3. 学术社交网络学术社交网络是近年来兴起的一种科研交流平台,如ResearchGate、Academia、Mendeley等。
这些平台不仅提供科学文献的检索功能,还可以通过关注学者、组织加入学术圈子,与其他研究者进行学术交流与合作。
4. 预印本服务器预印本服务器是科学研究中一个新兴的科研交流平台,如arXiv、bioRxiv等。
研究者可以在这些平台上发布自己的研究成果,其他研究者可以通过搜索关键词找到与自己研究方向相关的预印本,获取最新的研究动态。
5. 个人联系与建议除了以上几种常用的文献检索方式,与其他研究者直接联系也是获取文献资源的一种方式。
在学术会议上结交同行,参加研讨会、讲座等也是获取文献建议的途径。
可以向有经验的学者或研究者请教,获取他们的经验与建议。
他们通常会给出一些建议性的文献资料。
总结:科学文献检索是科学研究的重要一环,合理有效地进行文献检索对于研究的开展和深入具有重要的意义。
本文介绍了几种常用的文献检索方法,包括图书馆文献检索、学术搜索引擎、学术社交网络、预印本服务器以及个人联系与建议等。
研究者可以根据自己的需求和研究领域选择适合的方法进行文献检索,以便更好地开展科研工作。
科学文献概述

科学文献概述科学文献是科学研究中不可或缺的重要资源,它记录了科学家们的研究成果、实验数据和理论探索,为科学研究提供了有力的支持和指导。
本文将对科学文献的概念、特点以及使用方法进行概述,旨在帮助读者更好地理解和利用科学文献。
一、科学文献的概念科学文献是指科学研究者在进行科学研究过程中所发表的学术论文、研究报告、学位论文、会议论文等形式的文献资料。
它包含了科学家们的研究成果、实验数据、理论探索等内容,是科学研究的重要产出和交流方式。
二、科学文献的特点1. 学术性:科学文献是由科学研究者发表的,具有一定的学术性和专业性。
它经过同行评议,经过严格的学术审查和筛选,保证了其内容的可靠性和科学性。
2. 更新性:科学文献反映了科学研究的最新进展和成果,具有很强的时效性。
科学家们通过发表文献来及时分享自己的研究成果,使得科学研究能够不断推进和发展。
3. 可信性:科学文献是经过同行评议和学术审查的,其内容经过了严格的验证和检验,具有较高的可信性。
科学研究者可以通过查阅相关文献来获取可靠的信息和数据。
4. 多样性:科学文献的形式多样,包括学术论文、研究报告、学位论文、会议论文等。
不同形式的文献适用于不同的研究领域和目的,科学研究者可以根据自己的需要选择合适的文献来源。
三、科学文献的使用方法1. 文献检索:科学研究者可以通过文献检索工具(如数据库、图书馆目录等)来查找和获取相关的科学文献。
在进行文献检索时,可以根据关键词、作者、出版年限等进行筛选和过滤,以获取符合自己研究需求的文献。
2. 文献阅读:科学研究者在获取到相关文献后,需要进行仔细阅读和理解。
阅读科学文献时,可以先浏览摘要和关键词,了解文献的主要内容和研究方法;然后再深入阅读全文,理解作者的实验设计、数据分析和结论推断。
3. 文献引用:科学研究者在撰写学术论文或研究报告时,需要引用相关的科学文献来支持自己的观点和结论。
在引用文献时,需要遵循相应的引用格式和规范,确保引用的准确性和规范性。
科普类文献
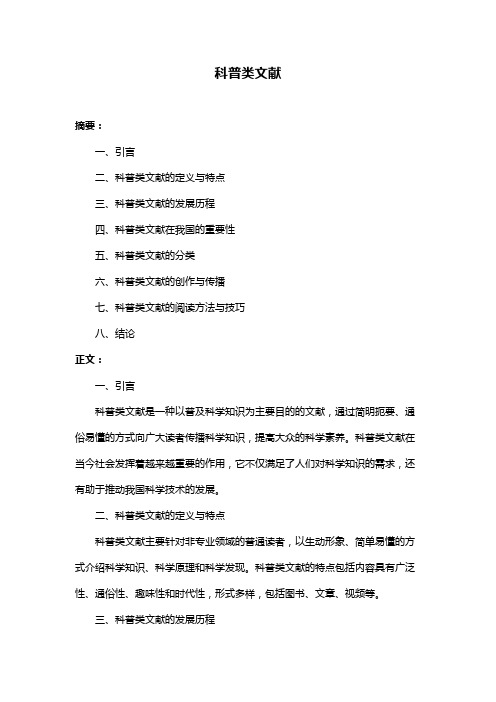
科普类文献摘要:一、引言二、科普类文献的定义与特点三、科普类文献的发展历程四、科普类文献在我国的重要性五、科普类文献的分类六、科普类文献的创作与传播七、科普类文献的阅读方法与技巧八、结论正文:一、引言科普类文献是一种以普及科学知识为主要目的的文献,通过简明扼要、通俗易懂的方式向广大读者传播科学知识,提高大众的科学素养。
科普类文献在当今社会发挥着越来越重要的作用,它不仅满足了人们对科学知识的需求,还有助于推动我国科学技术的发展。
二、科普类文献的定义与特点科普类文献主要针对非专业领域的普通读者,以生动形象、简单易懂的方式介绍科学知识、科学原理和科学发现。
科普类文献的特点包括内容具有广泛性、通俗性、趣味性和时代性,形式多样,包括图书、文章、视频等。
三、科普类文献的发展历程科普类文献源远流长,可以追溯到古代的民间传说和神话。
随着科学技术的进步,科普类文献逐渐发展为独立的领域,涌现出了许多脍炙人口的科普作品。
四、科普类文献在我国的重要性在我国,科普类文献对于提高全民科学素质、推动科技创新和培养人才具有重要意义。
政府也高度重视科普工作,出台了一系列政策措施,推动科普类文献的创作与传播。
五、科普类文献的分类科普类文献根据内容和形式可以分为多种类型,如基础科学普及、应用科学普及、科学史普及、科学幻想等。
六、科普类文献的创作与传播科普类文献的创作需要作者具备丰富的科学知识、写作技巧和教育经验。
传播途径包括传统纸质媒体、网络媒体、影视媒体等。
七、科普类文献的阅读方法与技巧阅读科普类文献时,要注意挑选适合自己水平的书籍和文章,采用轻松、愉快的心态来学习。
同时,要善于运用批判性思维,辨别真伪,避免被伪科学所误导。
八、结论科普类文献是传播科学知识、提高全民科学素质的重要载体。
sci中的相关文献

sci中的相关文献SCI(Science Citation Index)是科学引文索引,它涵盖了各个学科领域的学术期刊和会议论文,收录了世界上最重要的科学文献,并通过引文分析来评估文献的质量和影响力。
在SCI数据库中可以找到各个学科领域的相关文献。
以下是几个常见学科领域的SCI相关文献:1. 医学和生命科学领域:包括人类医学、生物学、生物化学、分子生物学、遗传学等。
相关期刊包括《Nature Medicine》、《The New England Journal of Medicine》、《Cell》等。
2. 工程科学和技术领域:包括机械工程、电子工程、化学工程、材料科学等。
相关期刊包括《Advanced Materials》、《IEEE Transactions on Industrial Electronics》、《Chemical Engineering Science》等。
3. 自然科学领域:包括物理学、化学、地球科学、天文学等。
相关期刊包括《Nature》、《Physical Review Letters》、《Chemical Reviews》、《Earth and Planetary Science Letters》等。
4. 社会科学领域:包括经济学、心理学、社会学、政治学等。
相关期刊包括《The American Economic Review》、《Psychological Science》、《American Journal of Sociology》等。
这些只是一部分常见的学科领域和相关期刊,实际上SCI涉及的学科领域非常广泛,覆盖了几乎所有的学术领域。
要获取更具体的相关文献信息,您可以登录SCI数据库进行搜索,或者参考相关学术期刊的官方网站。
科学研究 方法 的参考文献
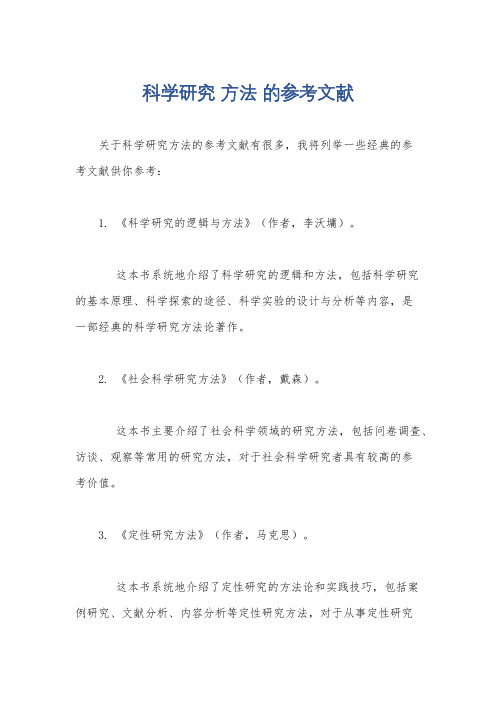
科学研究方法的参考文献
关于科学研究方法的参考文献有很多,我将列举一些经典的参
考文献供你参考:
1. 《科学研究的逻辑与方法》(作者,李沃墉)。
这本书系统地介绍了科学研究的逻辑和方法,包括科学研究
的基本原理、科学探索的途径、科学实验的设计与分析等内容,是
一部经典的科学研究方法论著作。
2. 《社会科学研究方法》(作者,戴森)。
这本书主要介绍了社会科学领域的研究方法,包括问卷调查、访谈、观察等常用的研究方法,对于社会科学研究者具有较高的参
考价值。
3. 《定性研究方法》(作者,马克思)。
这本书系统地介绍了定性研究的方法论和实践技巧,包括案
例研究、文献分析、内容分析等定性研究方法,对于从事定性研究
的学者和学生提供了宝贵的指导。
4. 《定量研究方法》(作者,柯林斯)。
这本书主要介绍了定量研究的基本原理和技术方法,包括实验设计、数据采集与分析、统计推断等内容,对于从事定量研究的学者和学生具有较高的参考价值。
以上是一些关于科学研究方法的经典参考文献,它们涵盖了科学研究的基本原理、途径和方法,对于帮助你全面了解科学研究方法具有重要意义。
希望对你有所帮助。
- 1、下载文档前请自行甄别文档内容的完整性,平台不提供额外的编辑、内容补充、找答案等附加服务。
- 2、"仅部分预览"的文档,不可在线预览部分如存在完整性等问题,可反馈申请退款(可完整预览的文档不适用该条件!)。
- 3、如文档侵犯您的权益,请联系客服反馈,我们会尽快为您处理(人工客服工作时间:9:00-18:30)。
header for SPIE usePoint-based warping with optimized weighting factorsof displacement vectorsRainer Pielot a, Michael Scholz b, Klaus Obermayer b, Eckart D. Gundelfinger a and Andreas Hess aa Leibniz Institute for Neurobiology, Brenneckestr. 6,D-39118 Magdeburg, Germanyb TU Berlin, Department of Computer Science, Sekr. FR 2-1, Franklinstr. 28/29,D-10587 Berlin, GermanyABSTRACTThe accurate comparison of inter-individual 3D image brain datasets requires non-affine transformation techniques (warping) to reduce geometric variations. Constrained by the biological prerequisites we use in this study a landmark-based warping method with weighted sums of displacement vectors, which is enhanced by an optimization process. Furthermore, we investigate fast automatic procedures for determining landmarks to improve the practicability of 3D warping. This combined approach was tested on 3D autoradiographs of Gerbil brains. The autoradiographs were obtained after injecting a non-metabolizable radioactive glucose derivative into the Gerbil thereby visualizing neuronal activity in the brain. Afterwards the brain was processed with standard autoradiographical methods. The landmark-generator computes corresponding reference points simultaneously within a given number of datasets by Monte-Carlo-techniques. The warping function is a distance weighted exponential function with a landmark-specific weighting factor. These weighting factors are optimized by a computational evolution strategy. The warping quality is quantified by several coefficients (correlation coefficient, overlap-index, and registration error). The described approach combines a highly suitable procedure to automatically detect landmarks in autoradiographical brain images and an enhanced point-based warping technique, optimizing the local weighting factors. This optimization process significantly improves the similarity between the warped and the target dataset.Keywords:Warping, Brain Imaging, Registration, Landmark Detection1.INTRODUCTIONGeometric transformation techniques play an important role for matching information of individual brain image datasets against a reference system to reduce inter-individual variations1. Such transformations consist in the simplest affine case of repositioning by translation and rotation of the image dataset relative to the reference system. Additional transforming the shape of the brain requires non-affine transformations (warping)1-3. Implementations of warping algorithms can be based on low-level information, such as intensity distributions, or on high-level information like structural information, such as corresponding points or contours of important anatomic elements. Intensity-based warping algorithms maximize local intensity similarities between the datasets; the definition of the spatial relationships can be based e.g. local cross correlation4 or squared differences of pixel intensities5. Since our interest is the inter-individual local spatial distribution of grayvalue differences instead of subject-specific shape variations intensity-based warping is not appropriate for our kind of biological analysis. Another possibility to match individual image datasets is to find corresponding reference points (landmarks) and touse them for calculation of the transformation (point-based warping). In this study, we use a distance-weighted method of warping with weighted sums of displacement vectors6-7, which is extended by an iterative optimization process8.The manual setting of such reference points between large 3D image datasets depends on prior knowledge of the expert and is very time-consuming. To overcome these problems several approaches for an automatic extraction of landmarks by incorporating knowledge about anatomic features were developed9-10. These algorithms utilize anatomic structures such as sulcal-ventricular curves and gyral crests1 as matching criteria. We approach this problem by investigating fast automatic procedures for determing landmarks, which are independent of prior anatomic knowledge in our model.To evaluate the results, three different similarity functions were applied to the datasets before and after matching against a reference template. The mean linear cross correlation coefficient measures the intensity similarity between all datasets according to correspondent grayvalue information. The overlap index describes the geometric shape correspondence of the datasets. The registration error utilizes structural information in terms of characteristic brain description points to measure the similarity.The combined approach was tested on reconstructed 3D autoradiographs of brains of Mongolian Gerbils (Meriones unguiculatus).This paper is organized as follows: The next section describes the preparation of the brains and the digitization of the autoradiographs. The preprocessing of the image-stacks (reconstruction) by image alignment is outlined in Section 3. Sections 5 and 6 describe the detection of the landmarks and the subsequent warping procedure. Section 6 describes the quality measures, which evaluate the warping results. The results are presented in Section 7 and discussed in Section 8.2.TISSUE-PREPARATIONThe autoradiographs were obtained after intraperitoneal injection of radioactive non-metabolizable 2-fluoro-2-deoxyglucose11 (2FDG) into gerbils12. This method visualizes brain activity due to locally increased excitatory neuronal metabolic activity. The gerbils were acoustically stimulated (pure tones at 70 db) with a stimulating frequency of 1 and 8 kHz. After 45 min the animals were sacrificed and the brains were removed. Then the brains were sectioned on a cryostat and after drying at 60°C autoradiographed with a KODAK NMB X-ray film. After 2 weeks exposure, the films were developed and digitized with a CCD camera (768*512 pixel, 8 bit/pixel). All animal experiments were performed in accordance with the guidelines and regulations defined by the German Animal Welfare Act. Permission was obtained from the Regierungspraesidium Dessau.3.IMAGE PREPROCESSINGReconstructions of image-stacks of physically sectioned slices are performed in a first step by image alignment. For this purpose, each slice is rotated and translated in order to maximize the spatial relations between adjacent slices. In this study we use a combinational reconstruction method13, consisting of principal axes alignment, which is fast automatic technique and a cross-correlation-based method, resulting in a high reconstruction quality14.The intensity of autoradiographs depends on various parameters like the position of the brain slice on the X-ray film and exposure time. Therefore, the images within one image stack may have a different grayvalue distribution and thus resulting in an inhomogeneous data volume. To solve this problem, the datasets were z-transformed to normalize the distribution of grayvalues:σµ-X=Z(1) X and Z are grayvalues of the voxel, µ the average grayvalue and σ the standard deviation.NDMARK DETECTIONIn this study we use an approach to define corresponding reference points, which is based on Monte-Carlo-techniques to detect edges of anatomical structures. In our model, an edge is defined by the grayvalue transition of adjacent voxels, which exceeds a certain threshold value. This value strongly depends on the level of noise and contrast in the image. A three-dimensional grid is placed on each dataset to define non-overlapping subvolumes. Within each subvolume random movements of a single point perform the search for edges. This procedure stops within each a certain subvolume, if an edge is found. Subvolumes in which no edge is found after a maximum number of iterations do not contribute a reference point. Only if a given subvolume contributes a reference point in all datasets, a reference point is generated for this subvolume in the template by averaging the positions of all corresponding reference points of the individual datasets. Consequently, the spatial difference of an individual reference point and the corresponding one in the reference template defines a displacement vector for the warping to be performed.Monte-Carlo-techniques are generally based on random numbers. In this study we use a pseudo random number generator, which is initialized with a certain seed number. As an approach to minimize the influence of the seed number, the final position of each reference point is the average of 10 runs. The pseudo random number generator, used in this study, is taken from the tested standard libraries (MIPSpro C-compiler 7.1). The quality of the pseudo random number generator is tested negatively for autocorrelation.In order to detect different displacement sizes, we use a multiscaling procedure. Two differently sized grids are used successively: First a coarse grid with large first-order subvolumes (1920) is used to detect large distortions (Fig. 1a). Afterwards, each large subvolume is divided into four smaller second-order subvolumes (7680) (Fig. 1b). If the search procedure within the four second-order subvolumes detects more than one surface in the corresponding first-order subvolume, the displacement vector in the latter one is eliminated to avoid non-corresponding surfaces, otherwise both are taken.The Monte-Carlo search was performed in each dataset separately. As mentioned above, the reference points of the template for a given subvolume are defined as the mean positions of the reference points of each individual dataset. Therefore each individual landmark couple is build up by a reference point of the individual dataset and the corresponding one of the reference template. The subsequent warping procedure matches each dataset with the information of all individual landmarks to the template.a bFigure 1: Grids of non-overlapping subvolumes. At the beginning of the Monte-Carlo search each point is located in the center of selected subvolumes. a) Coarse grid (1920 subvolumes). b) Fine grid (7680 subvolumes). For a better illustration four different anatomical structures were manually segmented (septum (SE), striatum (ST), thalamus (TH), andhippocampus (H)) and then surface rendered including the outline of the brain (Visualization software: A MIRA (Indeed GmbH)).5.WARPINGAs described above, the presented warping method is based on displacement vectors, which are defined by the spatial difference of corresponding reference points (landmarks) in the individual datasets and in the reference template. The transformation function determines the displacement of each voxel by the weighted sum of all displacement vectors.The distribution of the generated landmarks across the 3D dataset is very irregular. Simple distance-weighted warping methods determine the transformation only dependent on the absolute distance of the reference points to a given voxel. Therefore landmark-free regions are transformed with the information of the nearest available landmark, which can be localized in another structure. On the other side, there may exist regions with many landmarks lying close together describing even different transformation directions. The transformation of such regions is more or less determined by an average of these displacement vectors. One approach to overcome this problem is to determine each displacement not only by the spatial difference of a given voxel to each landmark but also by a landmark-specific weighting factor8. The weighting factor determines the influence of that specific landmark on the transformation. At the beginning all weighting factors have identical values, so that the displacement of a given voxel is mainly influenced by the closest landmarks.The following transformation function T(P) describes the transformation of the voxel P=(x,y,z) based on the reference points {i1L}={(x i,y i,z i)|0<=i<=M-1} in the source dataset and the corresponding points }{0i L={(u i,v i,w i)|0<=i<=M-1} in the reference template:T(P)=P+wi(P)(Lii=0M-1∑−L i1)wi(P)i=0M-1∑(2)The weighting function w i(P) consists of the landmark-specific weighting factor βi and the distance function d i(P) of the point P to the reference point {i1L}:wi=e−βi d i(P)(3) The distance function d i(P) bases on the city-block metric to reduce computational efforts by avoiding the computation of the square root:d i(P)=|(x-x i)|+ |(y-y i)|+ |(z-z i)|(4) Calculation of the Euclidian distance instead of the city-block distance does not improve the warping results (data not shown).As outlined above it is essential to select the weighting factors optimally due to their strong influence on the warping result. To optimize these weighting factors, we used a computational evolution strategy, which consists of the following steps: At the beginning, a population of identical sets of weighting factors is generated. The fitness of each set is determined by warping of the dataset to the template and then by calculating a registration error to quantify the similarity. The registration error is the sum of the distance values between all so-called registration points of the warped dataset and the corresponding ones of the reference template. Registration points are a small number of manually defined points at characteristic positions in all datasets. In this study, the registration points are defined by one expert. Here, we use 28 registration points, which are located on structures which can be easily identified (Fig. 2).Figure 2: Registration points. For a better illustration only one slice with registration points, for which the z-coordinates correspond ± 1 slice to the z-level of this slice, is shown here (16 out of 28 registration points in the 3D dataset are placed here).For the reference template the registration points are generated by averaging the coordinates of the registration points of the individual datasets around a given position. This registration error has to be minimized by the optimization procedure.Therefore after each generation, the set with the lowest registration error is duplicated and replaces the worst set. Then the weighting factors of all sets other than the fittest set are mutated randomly with normal distributed step sizes. This procedure prevents the optimization to run into deadlocks. These steps are iterated for a constant number of generations,which was selected to obtain a stable fitness. In this study, the optimization was realized with a population of 500 sets of local weighting factors and ended after 1,000 generation cycles.6. SIMILARITY MEASURESThe quality of the warping is determined by comparison of the mean linear cross correlation coefficient, the overlap index and the registration error between the datasets before, after warping and after optimized warping to the reference template.The mean linear cross correlation coefficient measures the intensity similarity between two datasets according to correspondent grayvalue information. The maximum value of 1.0 is reached, when the highest grayvalues exist at the same location in the two 3D datasets. A normalized image cross correlation c is defined as 14:()()∑∑∑∑∑∑∑∑∑=x y z z y x T x y z z y x W x y zz y x T z y x W I I I I c 2),,(2),,(),,(),,((5)I W(x,y,z) and I T(x,y,z) describe the grayvalues of the voxels of the datasets W and T at the position (x,y,z ). The summation is only performed in the area of overlap between the datasets.The overlap index o describes the extent of the volume of overlap, which quantifies the geometric correspondence of the two datasets to be compared. The overlap index is defined as:T W WTN N N o +=*2(6)The overlap volume between the datasets W and T is described by N WT . N W , N T are the overlap volumes of the datasets W and T , respectively. Values of o are between 0 (no overlap) and 1.0 (perfect overlap).The determination of the registration error is already described in the previous section. The registration error reaches 0when the positions of the registration points in the transformed datasets become identical (perfect match). As mentioned before, the optimization procedure is based on this similarity function.7. RESULTS Figure 3 shows as an example a surface view of an individual brain dataset together with defined landmarks (M=1363).Figure 3: Surface view of an individual brain dataset together with 1363 generated landmarks. The small lines at the points describe the displacement vectors. As in Fig. 1, four different regions (septum (SE), striatum (ST), thalamus (TH), and hippocampus (H)) were segmented manually and surface rendered together with the outer surface of the gerbil brain by the visualization software A MIRA (Indeed GmbH). The cerebellum (CE) was not segmented.Most of the landmarks are located on the surface of the structures. Some landmarks are defined within the region of the cerebellum, which is not shown as a segmented structure in this figure. As described above, the positions of the individual reference points are averaged in order to generate a reference template. Then all datasets are warped to this template. Figure 4 shows as an example a slice of a dataset before transformation (Fig. 4a) and after transformation (Fig. 4b). The contour of the corresponding slice of another dataset is shown to illustrate the increase in overlap after warping.a bFigure 4: a) Slice of a dataset before transformation b) same slice after transformation. Black contour: Outline of the corresponding slice of another dataset. The arrows point to positions where a better overlap is achieved after warping. Tab. 1 shows the averaged results of the similarity measure (3 datasets).Table 1: Results of similarity measures (averaged values of all brain datasets).All calculations were performed on a SGI Origin 200 (MIPS R10000/180 MHz). The landmark generation required 7,228 s / dataset computation time, the warping procedure including the optimization required 13,027 s / dataset computation time (averaged values, single CPU).8.DISCUSSIONIn our analysis the first step of an accurate comparison of inter-individual brain datasets is to define individual reference points. Then the reference template is generated by averaging the positions of the corresponding individual reference points. The landmarks provide the geometrical information for the subsequent warping of the individual brain datasets to thistemplate. Finally the increase of similarity between the transformed objects is quantified. The presented approach combines a fast fully automatic procedure to detect landmarks and a point-based warping technique, which optimizes the influence of displacement vectors.The presented landmark generator is a highly suitable tool for detecting landmarks simultaneously in large 3D brain datasets without incorporating any prior knowledge. The average positions of the landmarks are successfully used to generate a reference template.The optimization of the weighting factors improves the similarity of the warped datasets and hence decreases the inter-individual differences in all cases tested. Similarity measures between the warped objects show the increase of similarity after warping. Optimizing the local influence of each landmark results in an accurate warping with very good local adjustments, which justifies the relatively high computational effort of the optimization procedure.For biological questions demanding quantitative evaluation of grayvalues the above described algorithms proved to be a useful tool to make complex biological structures inter-individually more comparable and facilitate a quantitative group comparison.ACKNOWLEDGEMENTSThis work was supported by the Bundesministerium für Bildung und Forschung (BMBF) of the Federal Republic of Germany (AZ 10963, Forschungsverbund “Virtual Brain”)REFERENCES1. A.W. Toga, Brain Warping, Academic Press, San Diego, 19992.G. Wolberg, Digital Image Warping, IEEE Computer Society Press, 19903. A.W. Toga, “Visualization and warping of multimodality brain imagery,” in Functional Neuroimaging, R.W. Thatcher,M. Hallet, T. Zeffiro, E.R. John, and M. Huerta, eds., pp. 171-180. Academic Press, San Diego, 19944.R. Bajcsy, S. Kovacic, “Multiresolution elastic matching,” Computer Vision, Graphics and Image Processing, 46, pp.1-21, 19895.G.E. Christensen, R.D. Rabbit and M.I. Miller, “Deformable templates using large deformation kinematics,” IEEETransactions on Image Processing, 5(10), pp. 1435-1447, 19966. D. Shepard, “A two-dimensional interpolation function for irregularly-spaced data,” Proc. 1968 ACM NationalConference, pp. 517-524, 19687.R. Franke and G. Nielson, “Smooth interpolation of large sets of scattered data,” Int J for Numerical Methods inEngineering, 15, pp.1691-1704, 19808.R. Pielot, M. Scholz, K. Obermayer, E.D. Gundelfinger and A. Hess, “Optimiertes Warping durch gewichtete Summenvon Verschiebungsvektoren – eine neue Methode zur Reduktion von interindividuellen Variabilitäten von Hirndaten,”in Bildverarbeitung für die Medizin 1999, H. Evers, G. Glombitza, T. Lehmann and H.-P. Meinzer, eds., pp. 417-421, 19999.J. Declerck, G. Subsol, J.-P. Thirion and N. Ayache, “Automatic retrieval of anatomical structures in three-dimensionalmedical images,” INRIA Technical Report, p. 2485, 199510.C. Davatzikos, “Spatial normalization of three-dimensional brain images using deformable models,” J Comp AssistedTomography, 20(4) pp. 656-665, 199611.L. Sokoloff, M. Reivich, C. Kennedy, M.H. Des Rosiers, C.S. Patlak, K.D. Pettigrew, O. Sakurada and M. Shinohara,“The (14C)deoxyglucose method for the measurement of local cerebral glucose utilization: Theory, procedure, and normal values in the concious and anaesthetized albino rat,” J Neurochem, 28, pp. 897-916, 197712.H. Scheich, P Heil, G. Langner, “Functional organization of auditory cortex in the mongolian gerbil (Merionesunguiculatus) II. Tonotopic 2-deoxyglucose,” European J of Neuroscience, 5, pp. 898-914, 199313.L.S. Hibbard and R.A. Hawkins, “Objective image alignment for three-dimensional reconstruction of digitalautoradiograms,” J Neurosci Methods, 26, pp. 55-74, 198814.A. Hess, K. Lohmann, E.D. Gundelfinger and H. Scheich, “A new method for reliable and efficient reconstruction of 3-dimensional images from autoradiographs of brain sections,” J Neurosci Methods, 84, pp. 77-86, 199815.R.C Gonzalez, Digital Image Processing, Reading, MA:Addison Wesley, 1992。