MUSIC INFORMATION RETRIEVAL BIBLIOGRAPHY Information Seeking Behaviour and FRBR
Embracing Musicality
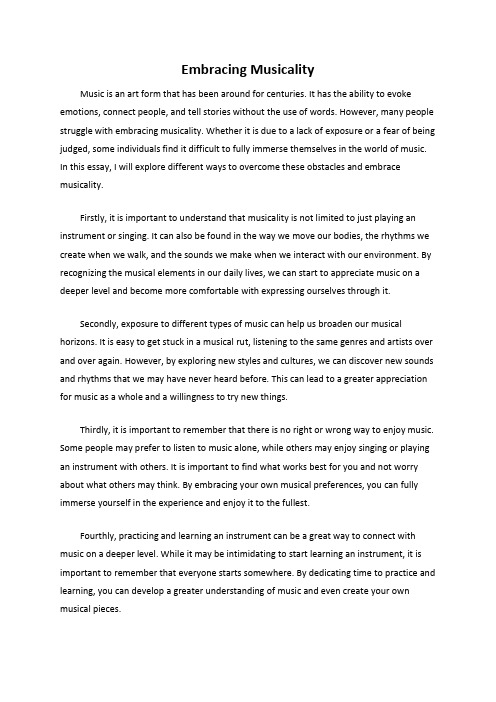
Embracing MusicalityMusic is an art form that has been around for centuries. It has the ability to evoke emotions, connect people, and tell stories without the use of words. However, many people struggle with embracing musicality. Whether it is due to a lack of exposure or a fear of being judged, some individuals find it difficult to fully immerse themselves in the world of music. In this essay, I will explore different ways to overcome these obstacles and embrace musicality.Firstly, it is important to understand that musicality is not limited to just playing an instrument or singing. It can also be found in the way we move our bodies, the rhythms we create when we walk, and the sounds we make when we interact with our environment. By recognizing the musical elements in our daily lives, we can start to appreciate music on a deeper level and become more comfortable with expressing ourselves through it.Secondly, exposure to different types of music can help us broaden our musical horizons. It is easy to get stuck in a musical rut, listening to the same genres and artists over and over again. However, by exploring new styles and cultures, we can discover new sounds and rhythms that we may have never heard before. This can lead to a greater appreciation for music as a whole and a willingness to try new things.Thirdly, it is important to remember that there is no right or wrong way to enjoy music. Some people may prefer to listen to music alone, while others may enjoy singing or playing an instrument with others. It is important to find what works best for you and not worry about what others may think. By embracing your own musical preferences, you can fully immerse yourself in the experience and enjoy it to the fullest.Fourthly, practicing and learning an instrument can be a great way to connect with music on a deeper level. While it may be intimidating to start learning an instrument, it is important to remember that everyone starts somewhere. By dedicating time to practice and learning, you can develop a greater understanding of music and even create your own musical pieces.Fifthly, attending live music events can be a great way to connect with others who share your love for music. Whether it is attending a concert or joining a local music group, being around others who appreciate music can be a great way to feel a sense of community and belonging.Lastly, it is important to approach music with an open mind and heart. Music has the ability to evoke strong emotions and connect people in ways that words cannot. By embracing musicality, we can tap into this powerful force and experience the beauty and joy that music has to offer.In conclusion, embracing musicality is about more than just listening to music. It is about recognizing the musical elements in our daily lives, exploring new genres and cultures, finding what works best for us, practicing and learning, connecting with others, and approaching music with an open mind and heart. By doing so, we can fully immerse ourselves in the world of music and experience its transformative power.。
音乐教育英文文献综述范文

音乐教育英文文献综述范文Music Education: A Comprehensive Literature ReviewMusic is a fundamental aspect of human culture, woven into the fabric of our social, emotional, and cognitive experiences. It has long been recognized as a powerful tool for personal and collective expression, as well as a vital component of education. In recent decades, there has been a growing body of research exploring the multifaceted benefits of music education, its impact on student development, and its role within the broader educational landscape. This comprehensive literature review aims to synthesize and analyze the existing scholarly literature on the subject of music education, providing a holistic understanding of its significance and potential implications for educational practices.The Importance of Music EducationThe value of music education extends far beyond the development of musical skills and appreciation. Numerous studies have demonstrated the positive impact of music education on various aspects of student development. Cognitively, music has been shown to enhance spatial-temporal reasoning, language skills, and overall academic performance. Psychologically, music education cancontribute to improved emotional regulation, social skills, and overall well-being. Moreover, music education has been linked to the development of creativity, critical thinking, and problem-solving abilities, all of which are essential for success in the 21st-century workforce.Cognitive Benefits of Music EducationOne of the most extensively researched areas in music education is its impact on cognitive development. Several studies have consistently found a strong correlation between music education and improved academic performance. For instance, a longitudinal study by Kinney (2008) examined the academic achievement of students who participated in school-based music programs compared to those who did not. The findings revealed that students involved in music education demonstrated significantly higher scores in standardized tests, particularly in the areas of mathematics and reading. Similarly, a meta-analysis by Hille and Schupp (2015) concluded that music education can lead to enhanced cognitive abilities, including increased memory, attention, and language processing skills.The neurological underpinnings of these cognitive benefits have also been explored. Researchers have discovered that musical training can induce structural and functional changes in the brain, particularly in areas associated with language, executive function, and spatial-temporal reasoning (Gaser & Schlaug, 2003; Moreno et al., 2011). These neuroplastic changes suggest that the cognitive benefits of music education extend beyond the musical domain, positively impacting a wide range of cognitive abilities.Emotional and Social Benefits of Music EducationIn addition to its cognitive advantages, music education has been shown to contribute to the emotional and social development of students. Numerous studies have found that participation in music programs can lead to improved emotional regulation, self-esteem, and social skills (Rickard et al., 2012; Schellenberg, 2004). Music can provide an outlet for emotional expression, fostering a sense of community and belonging among students (Kokotsaki & Hallam, 2007). Furthermore, music education often involves collaborative activities, such as ensemble performance, which can enhance teamwork, communication, and empathy (Hallam, 2010).The emotional and social benefits of music education are particularly relevant in the context of child and adolescent development. During these formative years, individuals undergo significant emotional and social changes, and music can play a crucial role in helping them navigate these challenges. By providing a supportive and nurturing environment, music education can contribute to the development of emotional intelligence, social competence, and overall well-being (Schellenberg, 2006).Music Education and the Development of Creativity and Critical ThinkingAlongside its cognitive and socio-emotional benefits, music education has been recognized as a powerful tool for fostering creativity and critical thinking skills. The process of music creation, performance, and analysis requires the application of divergent and convergent thinking, problem-solving, and decision-making (Burnard, 2012). Engaging in musical activities can enhance students' ability to think outside the box, consider multiple perspectives, and develop innovative solutions to complex problems.Furthermore, the study of music can cultivate critical thinking skills, as students are required to analyze, interpret, and evaluate musical works and their underlying elements (Elliot, 1995). This critical thinking process can be transferred to other academic domains, contributing to overall academic success and the development of21st-century skills.Music Education and the 21st-Century WorkforceAs the global economy and workforce continue to evolve, the importance of developing a diverse set of skills, including creativity, collaboration, and adaptability, has become increasingly recognized. Music education can play a vital role in preparing students for the demands of the 21st-century workforce.The skills acquired through music education, such as problem-solving, communication, and teamwork, are highly valued in the modern workplace (Biasutti, 2013). Employers in various industries are seeking individuals who can think critically, work effectively in teams, and demonstrate innovative thinking – all of which are fostered through music education. By integrating music education into the curriculum, schools can better equip students with the necessary skills and competencies to thrive in an ever-changing, global landscape.Challenges and Considerations in Music EducationDespite the well-documented benefits of music education, there are several challenges and considerations that must be addressed. One of the primary challenges is the issue of accessibility and equity. Music education programs are not equally available or accessible to all students, often disproportionately benefiting those from higher socioeconomic backgrounds (Elpus & Abril, 2011). Addressing this disparity is crucial to ensuring that the transformative power of music education is made available to all students, regardless of their socioeconomic status or background.Another challenge is the perceived prioritization of core academic subjects, such as mathematics and language arts, over the arts, including music (Abril & Gault, 2008). This perception can lead to themarginalization of music education within the curriculum, with limited resources and funding allocated to these programs. Advocating for the integration of music education as an essential component of a well-rounded education is crucial to ensuring its sustainability and widespread adoption.ConclusionThe existing body of research on music education presents a compelling case for its inclusion and expansion within educational systems. The cognitive, emotional, social, and creative benefits of music education have been well-documented, highlighting its potential to contribute to the holistic development of students. As the demands of the 21st-century workforce continue to evolve, the skills fostered through music education, such as critical thinking, problem-solving, and collaboration, become increasingly valuable.However, challenges related to accessibility, equity, and the perceived prioritization of core academic subjects over the arts must be addressed to ensure that all students have the opportunity to experience the transformative power of music education. By advocating for the integration of music education as an essential component of a comprehensive curriculum, educators, policymakers, and communities can work towards a future where the benefits of music education are accessible to all students, empowering them toreach their full potential and thrive in the ever-changing global landscape.。
介绍音乐的英语ppt课件

Viola
A large instrument with a deep, rich sound It is the largest of the string instruments
This symphony is the pinnacle of Beethoven's creative career and one of his most representative works. It is famous for its grand scale and profound emotions, expressing the ruthlessness of fate and the tenacity of humanity through music.
Summary: Music style refers to the characteristics and characteristics of music, including melody, rhythm, harmony, timbre, and other aspects. Different music styles reflect different cultural and historical backgrounds.
Cello
The largest and lowest matched string instrument It provides the bass line in an Orchestra
Bass
Flute
EVALUATION OF MUSICAL FEATURES FOR EMOTION CLASSIFICATION
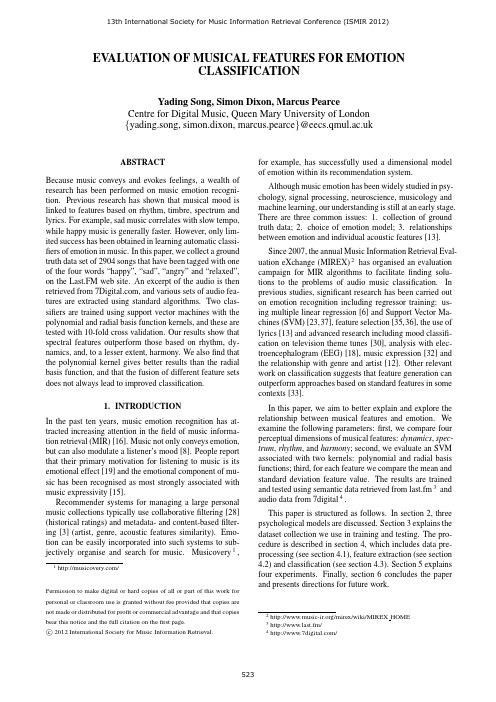
EV ALUATION OF MUSICAL FEATURES FOR EMOTIONCLASSIFICATIONYading Song,Simon Dixon,Marcus PearceCentre for Digital Music,Queen Mary University of London{yading.song,simon.dixon,marcus.pearce}@ABSTRACTBecause music conveys and evokes feelings,a wealth of research has been performed on music emotion recogni-tion.Previous research has shown that musical mood is linked to features based on rhythm,timbre,spectrum and lyrics.For example,sad music correlates with slow tempo, while happy music is generally faster.However,only lim-ited success has been obtained in learning automatic classi-fiers of emotion in music.In this paper,we collect a ground truth data set of2904songs that have been tagged with one of the four words“happy”,“sad”,“angry”and“relaxed”, on the Last.FM web site.An excerpt of the audio is then retrieved ,and various sets of audio fea-tures are extracted using standard algorithms.Two clas-sifiers are trained using support vector machines with the polynomial and radial basis function kernels,and these are tested with10-fold cross validation.Our results show that spectral features outperform those based on rhythm,dy-namics,and,to a lesser extent,harmony.We alsofind that the polynomial kernel gives better results than the radial basis function,and that the fusion of different feature sets does not always lead to improved classification.1.INTRODUCTIONIn the past ten years,music emotion recognition has at-tracted increasing attention in thefield of music informa-tion retrieval(MIR)[16].Music not only conveys emotion, but can also modulate a listener’s mood[8].People report that their primary motivation for listening to music is its emotional effect[19]and the emotional component of mu-sic has been recognised as most strongly associated with music expressivity[15].Recommender systems for managing a large personal music collections typically use collaborativefiltering[28] (historical ratings)and metadata-and content-basedfilter-ing[3](artist,genre,acoustic features similarity).Emo-tion can be easily incorporated into such systems to sub-jectively organise and search for music.Musicovery1, 1/Permission to make digital or hard copies of all or part of this work for personal or classroom use is granted without fee provided that copies are not made or distributed for profit or commercial advantage and that copies bear this notice and the full citation on thefirst page.c 2012International Society for Music Information Retrieval.for example,has successfully used a dimensional model of emotion within its recommendation system.Although music emotion has been widely studied in psy-chology,signal processing,neuroscience,musicology and machine learning,our understanding is still at an early stage. There are three common issues:1.collection of ground truth data;2.choice of emotion model;3.relationships between emotion and individual acoustic features[13].Since2007,the annual Music Information Retrieval Eval-uation eXchange(MIREX)2has organised an evaluation campaign for MIR algorithms to facilitatefinding solu-tions to the problems of audio music classification.In previous studies,significant research has been carried out on emotion recognition including regressor training:us-ing multiple linear regression[6]and Support Vector Ma-chines(SVM)[23,37],feature selection[35,36],the use of lyrics[13]and advanced research including mood classifi-cation on television theme tunes[30],analysis with elec-troencephalogram(EEG)[18],music expression[32]and the relationship with genre and artist[12].Other relevant work on classification suggests that feature generation can outperform approaches based on standard features in some contexts[33].In this paper,we aim to better explain and explore the relationship between musical features and emotion.We examine the following parameters:first,we compare four perceptual dimensions of musical features:dynamics,spec-trum,rhythm,and harmony;second,we evaluate an SVM associated with two kernels:polynomial and radial basis functions;third,for each feature we compare the mean and standard deviation feature value.The results are trained and tested using semantic data retrieved from last.fm3and audio data from7digital4.This paper is structured as follows.In section2,three psychological models are discussed.Section3explains the dataset collection we use in training and testing.The pro-cedure is described in section4,which includes data pre-processing(see section4.1),feature extraction(see section 4.2)and classification(see section4.3).Section5explains four experiments.Finally,section6concludes the paper and presents directions for future work.2/mirex/wiki/MIREX HOME3st.fm/4/2.PSYCHOLOGICAL EMOTION MODELS One of the difficulties in representing emotion is to distin-guish music-induced emotion from perceived emotion be-cause the two are not always aligned[5].Different psycho-logical models of emotion have been compared in a study of perceived emotion[7].Most music related studies are based on two popular approaches:categorical[10]and dimensional[34]mod-els of emotion.The categorical approach describes emo-tions with a limited number of innate and universal cate-gories such as happiness,sadness,anger and fear.The di-mensional model considers all affective terms arising from independent neurophysiological systems:valence(nega-tive to positive)and arousal(calm to exciting).Recently a more sophisticated model of music-induced emotion-the Geneva Emotion Music Scale(GEMS)model-consisting of9dimensions,has been proposed[42].Our results and analysis are based on the categorical model since we make our data collection through human-annotated social tags which are categorical in nature.3.GROUND-TRUTH DATA COLLECTIONAs discussed above,due to the lack of ground truth data, most researchers compile their own databases[41].Man-ual annotation is one of the most common ways to do this. However,it is expensive in terms offinancial cost and hu-man labour.Moreover,terms used may differ between in-dividuals.Different emotions may be described using the same term by different people which would result in poor prediction[38].However,with the emergence of music discovery and recommendation websites such as last.fm which support social tags for music,we can access rich human-annotated pared with the tradi-tional approach of web mining which gives noisy results, social tagging provides highly relevant information for mu-sic information retrieval(MIR)and has become an im-portant source of human-generated contextual knowledge [11].Levy[24]has also shown that social tags give a high quality source of ground truth data and can be effective in capturing music similarity[40].Thefive mood clusters proposed by MIREX[14](such as rollicking,literate,and poignant)are not popular in so-cial tags.Therefore,we use four basic emotion classes: happy,angry,sad and relaxed,considering these four emo-tions are widely accepted across different cultures and cover the four quadrants of the2-dimensional model of emo-tion[22].These four basic emotions are used as seeds to retrieve the top30tags from last.fm.We then obtain a list of songs labelled with the retrieved tags.Table1and table 2show an example of the retrieved results.Given the retrieved titles and the names of the singers, we use a public API to get previewfiles.The results cover different types of pop music,meaning that we avoid partic-ular artist and genre effects[17].Since the purpose of this step is tofind ground truth data,issues such as cold start, noise,hacking,and bias are not relevant[4,20].Most datasets on music emotion recognition are quiteHappy Angry Sad Relaxhappy angry sad relax happy hardcore angry music sad songs relax trance makes me happy angry metal happysad relax music happy music angry pop music sad song jazz relax happysad angry rock sad&beautiful only relax Table1.Top5tags returned by last.fmSinger TitleNoah And The Whale5Years TimeJason Mraz I’m YoursRusted Root Send Me On My WayRoyksopp Happy Up HereKaren O and the Kids All Is LoveTable2.Top songs returned with tags from the“happy”category.small(less than1000items),which indicates that2904 songs(see table3)for four emotions retrieved by social tags is a good size for the current experiments.The dataset will be made available5,to encourage other researchers to reproduce the results for research and evaluation.Emotion Number of SongsHappy753Angry639Sad763Relaxed749Overall2904Table3.Summary of ground truth data collection4.PROCEDURESThe experimental procedure consists of four stages:data collection,data preprocessing,feature extraction,and clas-sification,as shown infigure1.4.1Data PreprocessingAs shown in Table1,there is some noise in the data such as confusing tags and repeated songs.We manually remove data with the tag happysad which existed in both the happy and sad classes and delete the repeated songs,to make sure every song will only exist once in a single class.Moreover, we convert our dataset to standard wav format(22,050Hz sampling rate,16bit precision and mono channel).The song excerpts are either30seconds or60seconds,rep-resenting the most salient part of the song[27],therefore there is no need to truncate.At the end,we normalise the excerpts by dividing by the highest amplitude to mitigate the production effect of different recording levels.4.2Feature ExtractionAs suggested in the work of Saari and Eerola[35],two dif-ferent types of feature(mean and standard deviation)with 5The dataset can be found at https:///projects-/emotion-recognitionFigure1.Procedurea total of55features were extracted using the MIR tool-box6[21](shown in table4).The features are categorized into the following four perceptual dimensions of music lis-tening:dynamics,rhythm,spectral,and harmony.4.3ClassificationThe majority of music classification tasks[9](genre clas-sification[25,39],artist identification[29],and instrument recognition[31])have used k-nearest neighbour(K-NN) [26]and support vector machines(SVM)[2].In the case of audio input features,the SVM has been shown to per-form best[1].In this paper,therefore,we choose support vector ma-chines as our classifier,using the implementation of the se-quential minimal optimisation algorithm in the Weka data mining toolkit7.SVMs are trained using polynomial and radial basis function(RBF)kernels.We set the cost factor C=1.0,and leave other parameters unchanged.An in-ternal10-fold cross validation is applied.To better under-stand and compare features in four perceptual dimensions, our experiments are divided into four tasks.Experiment1:we compare the performance of the two kernels(polynomial and RBF)using various features.Experiment2:four classes(perceptual dimensions)of features are tested separately,and we compare the results tofind a dominant class.Experiment3:two types of feature descriptor,mean and standard deviation,are calculated.The purpose is to com-pare values for further feature selection and dimensionality reduction.6Version1.3.3:https://www.jyu.fi/music/coe/materials/mirtoolbox 7/ml/weka/Dimen.No.Features Acronyms Dynamics1-2RMS energy RMSm,RMSstd 3-4Slope Ss,Sstd5-6Attack As,Astd7Low energy LEm Rhythm1-2Tempo Ts,Tstd3-4Fluctuation peak(pos,mag)FPm,FMm5Fluctuation centroid FCm Spec.1-2Spectrum centroid SCm,SCstd3-4Brightness BRm,BRstd5-6Spread SPm,SPstd7-8Skewness SKm,SKstd9-10Kurtosis Km,Kstd11-12Rolloff95R95s,R95std13-14Rolloff85R85s,R85std15-16Spectral Entrophy SEm,SEstd17-18Flatness Fm,Fstd19-20Roughness Rm,Rstd21-22Irregularity IRm.IRstd23-24Zero crossing rate ZCRm,ZCRstd25-26Spectralflux SPm,SPstd27-28MFCC MFm,MFstd29-30DMFCC DMFm,DMFstd31-32DDMFCC DDm,DDstd Harmony1-2Chromagram peak CPm,CPstd3-4Chromagram centroid CCm,CCstd5-6Key clarity KCm,KCstd7-8Key mode KMm,KMstd9-10HCDF Hm,Hstd Table4.The feature set used in this work;m=mean,std =standard deviation.Experiment4:different combinations of feature classes (e.g.,spectral with dynamics)are evaluated in order to de-termine the best-performing model.5.RESULTS5.1Experiment1In experiment1,SVMs trained with two different kernels are compared.Previous studies[23]have found in the case of audio input that the SVM performs better than other classifiers(Logistic Regression,Random Forest,GMM, K-NN and Decision Trees).To our knowledge,no work has been reported explicitly comparing different kernels for SVMs.In emotion recognition,the radial basis func-tion kernel is a common choice because of its robustness and accuracy in other similar recognition tasks[1].Polynomial RBFFeature Class Accuracy Time Accuracy Time No.Dynamics37.20.4426.332.57Rhythm37.50.4434.523.25Harmony47.50.4136.627.410Spectral51.90.4048.114.332 Table5.Experiment1results:time=model building time, No.=number of features in each classThe results in table5show however that regardless of the features used,the polynomial kernel always achieved the higher accuracy.Moreover,the model construction times for each kernel are dramatically different.The av-erage construction time for the polynomial kernel is0.4 seconds,while the average time for the RBF kernel is24.2seconds,around60times more than the polynomial ker-nel.The following experiments also show similar results. This shows that polynomial kernel outperforms RBF in the task of emotion recognition at least for the parameter val-ues used here.5.2Experiment2In experiment2,we compare the emotion prediction re-sults for the following perceptual dimensions:dynamics, rhythm,harmony,and spectral.Results are shown infig-ure2).Dynamics and rhythm features yield similar re-sults,with harmony features providing better results,but the spectral class with32features achieves the highest ac-curacy of51.9%.This experiment provides a baseline model, and further exploration of multiple dimensions is performed in experiment4.parison of classification results for the four classes of features.5.3Experiment3In this experiment,we evaluate different types of feature descriptors,mean value and standard deviation for each feature across all feature classes,for predicting the emotion in music.The results in table6show that the use of both mean and standard deviation values gives the best results in each case.However,the processing time increased,so choosing the optimal descriptor for each feature is highly desirable.For example,choosing only the mean value in the harmony class,we lose2%of accuracy but increase the speed while the choice of standard deviation results in around10%accuracy loss.As the number of features in-creases,the difference between using mean and standard deviation will be reduced.However,more experiments are needed to explain why the mean in harmony and spectral features,and standard deviation values of dynamics and rhythm features have higher accuracy scores.5.4Experiment4In order to choose the best model,thefinal experiment fuses different perceptual features.As presented in table7, optimal accuracy is not produced by the combination of all features.Instead,the use of spectral,rhythm and harmony (but not dynamic)features produces the highest accuracy.Features Class Polynomial No.featuresDynamics all37.27Dynamics mean29.73Dynamics std33.83Rhythm all37.55Rhythm mean28.71Rhythm std34.21Harmony all47.510Harmony mean45.35Harmony std38.35Spectral all51.932Spectral mean49.616Spectral std47.516Spec+Dyn all52.339Spec+Dyn mean50.519Spec+Dyn std48.719Spec+Rhy all52.337Spec+Rhy mean49.817Spec+Rhy std47.817Spec+Har all53.342Spec+Har mean51.321Spec+Har std50.321Har+Rhy all49.115Har+Rhy mean45.66Har+Rhy std41.26Har+Dyn all48.817Har+Dyn mean46.98Har+Dyn std42.48Rhy+Dyn all41.712Rhy+Dyn mean32.04Rhy+Dyn std38.84parison of mean and standard deviation(std) features.Features Accuracy No.featuresSpec+Dyn52.339Spec+Rhy52.337Spec+Har53.342Har+Rhy49.115Har+Dyn48.817Rhy+Dyn41.712Spec+Dyn+Rhy52.444Spec+Dyn+Har53.849Spec+Rhy+Har54.047Dyn+Rhy+Har49.722All Features53.654Table7.Classification results for combinations of feature sets.6.CONCLUSION AND FUTURE WORKIn this paper,we collected ground truth data on the emo-tion associated with2904pop songs from last.fm tags.Au-dio features were extracted and grouped into four percep-tual dimensions for training and validation.Four experi-ments were conducted to predict emotion labels.The re-sults suggest that,instead of the conventional approach us-ing SVMs trained with a RBF kernel,a polynomial ker-nel yields higher accuracy.Since no single dominant fea-tures have been found in emotion recognition,we explored the performance of different perceptual classes of feature for predicting emotion in music.Experiment3found that dimensionality reduction can be achieved through remov-ing either mean or standard deviation values,halving the number of features used,with,in some cases,only2%ac-curacy loss.The last experiment found that inclusion of dynamics features with the other classes actually impairedthe performance of the classifier while the combination of spectral,rhythmic and harmonic features yielded optimal performance.In future work,we will expand this research both in depth and breadth,tofind features and classes of features which best represent emotion in music.We will examine higher-level dimensions such as temporal evolution fea-tures,as well as investigating the use of auditory ing the datasets retrieved from Last.fm,we will compare the practicability of social tags with other human-annotated datasets in emotion recognition.Through these studies of subjective emotion,we will develop methods for incorporating other empirical psychological data in a sub-jective music recommender system.7.ACKNOWLEDGEMENTSWe acknowledge the support of the Queen Mary University of London Postgraduate Research Fund(QMPGRF)and the China Scholarship Council.We would like to thank the reviewers and Emmanouil Benetos for their advice and comments.8.REFERENCES[1]K.Bischoff,C.S.Firan,R.Paiu,W.Nejdl,urier,and M.Sordo.Music Mood and Theme Classification -A Hybrid Approach.In10th International Society for Music Information Retrieval Conference,number Is-mir,pages657–662,2009.[2]E.Boser,N.Vapnik,and I.M.Guyon.Training Algo-rithm Margin for Optimal Classifiers.In ACM Confer-ence on Computational Learning Theory,pages144–152,1992.[3]P.Cano,M.Koppenberger,and N.Wack.Content-based Music Audio Recommendation.In Proceedings of the13th annual ACM international conference on Multimedia,number ACM,pages211–212,2005. [4]O.Celma.Foafing the Music:Bridging the SemanticGap in Music Recommendation.In The Semantic Web-ISWC,2006.[5]T.Eerola.Are the Emotions Expressed in Mu-sic Genre-specific?An Audio-based Evaluation of Datasets Spanning Classical,Film,Pop and Mixed Genres.Journal of New Music Research,40(March 2012):349–366,2011.[6]T.Eerola,rtillot,and P.Toiviainen.Predictionof Multdimensional Emotional Ratings in Music from Audio Using Multivariate Regression Models.In10th International Society for Music Information Retrieval Conference,number Ismir,pages621–626,2009. [7]T.Eerola and J.K.Vuoskoski.A Comparison of theDiscrete and Dimensional Models of Emotion in Mu-sic.Psychology of Music,39(1):18–49,August2010.[8]Y.Feng and Y.Zhuang.Popular Music Retrieval byDetecting Mood.In International Society for Music In-formation Retrieval Conference,volume2,pages375–376,2003.[9]Z.Fu,G.Lu,K.M.Ting,and D.Zhang.A Sur-vey of Audio-based Music Classification and Anno-tation.IEEE Transactions on Multimedia,13(2):303–319,2011.[10]K.Hevner.Experimental studies of the elements of ex-pression in music.The American Journal of Psychol-ogy,48:246–268,1936.[11]X.Hu,M.Bay,and J.S.Downie.Creating a SimplifiedMusic Mood Classification Grouth-truth Set.In Inter-national Conference on Music Information Retrieval, pages3–4,2007.[12]X.Hu and J.S.Downie.Exploring Mood Metadata:Relationships with Genre,Artist and Usage Metadata.In8th International Conference on Music Information Retrieval,2007.[13]X.Hu,J.S.Downie,and A.F.Ehmann.Lyric Text Min-ing in Music Mood Classification.In10th Interna-tional Society for Music Information Retrieval Confer-ence,number Ismir,pages411–416,2009.[14]X.Hu,J.S.Downie, urier,and M.Bay.The2007MIREX Audio Mood Classification Task:Les-son Learned.In International Society for Music Infor-mation Retrieval Conference,pages462–467,2008.[15]P.N.Juslin,J.Karlsson,E.Lindstr¨o m,A.Friberg,andE.Schoonderwaldt.Play it Again with Feeling:Com-puter Feedback in Musical Communication of Emo-tions.Journal of experimental psychology.Applied, 12(2):79–95,June2006.[16]Y.E.Kim,E.M.Schmidt,R.Migneco,B.G.Morton,P.Richardson,J.Scott,J.A.Speck,and D.Turnbull.Music Emotion Recognition:A State of the Art Re-view.In11th International Society for Music Informa-tion Retrieval Conference,number Ismir,pages255–266,2010.[17]Y.E.Kim, D.S.Williamson,and S.Pilli.TowardsQuantifying the Album Effect in Artist Identification.In International Society for Music Information Re-trieval Conference,2006.[18]S.Koelstra,C.Muhl,and M.Soleymani.Deap:ADatabase for Emotion Analysis Using Physiological Signals.IEEE Trans.on Affective Computing,pages1–15,2011.[19]C.L.Krumhansl.Music:A Link Between Cogni-tion and Emotion.American Psychological Society, 11(2):45–50,2002.[20]mere.Social Tagging and Music Information Re-trieval.Journal of New Music Research,37(2):101–114,June2008.[21]rtillot and P.Toiviainen.MIR in Matlab(II):AToolbox for Musical Feature Extraction from Audio.In International Conference on Music Information Re-trieval,number Ii,pages237–244,2007.[22]urier and J.Grivolla.Multimodal Music MoodClassification Using Audio and Lyrics.In Int.Conf.Machine Learning and Applications,pages1–6,2008.[23]urier,P.Herrera,M.Mandel,and D.Ellis.AudioMusic Mood Classification Using Support Vector Ma-chine.In MIREX task on Audio Mood Classification, pages2–4,2007.[24]M.Levy.A Semantic Space for Music Derived fromSocial Tags.In Austrian Compuer Society,volume1, page12.Citeseer,2007.[25]B.Lines,E.Tsunoo,G.Tzanetakis,and N.Ono.Be-yond Timbral Statistics:Improving Music Classifica-tion Using Percussive.IEEE Transactions on Audio, Speech and Language Processing,19(4):1003–1014, 2011.[26]T.M.Cover and P.E.Hart.Nearest Neighbor PatternClassification.IEEE Transactions on Information The-ory,13(1):21–27,1967.[27]K.F.MacDorman,S.Ough,and C.Ho.AutomaticEmotion Prediction of Song Excerpts:Index Construc-tion,Algorithm Design,and Empirical Comparison.Journal of New Music Research,36(4):281–299,De-cember2007.[28]T.Magno and C.Sable.A Comparison of Signal ofSignal-based Music Recommendation to Genre Labels, Collaborative Filtering,Musicological Analysis,Hu-man Recommendation and Random Baseline.In Pro-ceedings of the9th International Conference of Music Information Retrieval,pages161–166,2008.[29]M.Mandel.Song-level Features and Support Vec-tor Machines for Music Classification.In Proc.Inter-national Conference on Music Information Retrieval, 2005.[30]M.Mann,T.J.Cox,and F.F.Li.Music Mood Classi-fication of Television Theme Tunes.In12th Interna-tional Society for Music Information Retrieval Confer-ence,number Ismir,pages735–740,2011.[31]J.Marques and P.J.Moreno.A Study of Musical In-strument Classification Using Gaussian Mixture Mod-els and Support Vector Machines,1999.[32]L.Mion and G.D.Poli.Score-Independent AudioFeatures for Description of Music Expression.IEEE Transactions on Audio,Speech,and Language Pro-cessing,16(2):458–466,2008.[33]F.Pachet and P.Roy.Analytical Features:AKnowledge-Based Approach to Audio Feature Gener-ation.EURASIP Journal on Audio,Speech,and Music Processing,2009(2):1–23,2009.[34]J.A.Russell,A.Weiss,and G.A.Mendelsohn.Af-fect Grid:A Single-item Scale of Pleasure and Arousal.Journal of Personality and Social Psychology, 57(3):493–502,1989.[35]P.Saari,T.Eerola,and rtillot.Generalizabilityand Simplicity as Criteria in Feature Selection:Appli-cation to Mood Classification in Music.IEEE Trans-actions on Audio,Speech,and Language Processing, 19(6):1802–1812,2011.[36]E.M.Schmidt,D.Turnbull,and Y.E.Kim.FeatureSelection for Content-Based,Time-Varying Musical Emotion Regression Categories and Subject Descrip-tors.In Multimedia Information Retrieval,pages267–273,2010.[37]B.Schuller,J.Dorfner,and G.Rigoll.Determinationof Nonprototypical Valence and Arousal in Popular Music:Features and Performances.EURASIP Journal on Audio,Speech,and Music Processing,2010:1–19, 2010.[38]D.Turnbull,L.Barrington,and nckriet.Five Ap-proaches to Collecting Tags for Music.In Proceedings of the9th International Conference of Music Informa-tion Retrieval,pages225–230,2008.[39]G.Tzanetakis and P.Cook.Musical Genre Classifica-tion of Audio Signals.IEEE Transactions on Speech and Audio Processing,10(5):293–302,2002.[40]D.Wang,T.Li,and M.Ogihara.Tags Better ThanAudio Features?The Effect of Joint use of Tags and Audio Content Features for Artistic Style Clutering.In11th International Society on Music Information Retrieval Conference,number ISMIR,pages57–62, 2010.[41]D.Yang and W.S.Lee.Disambiguating Music Emo-tion Using Software Agents.In Proceedings of the5th International Conference on Music Information Re-trieval,pages52–58,2004.[42]M.Zentner,D.Grandjean,and K.R.Scherer.Emo-tions evoked by the sound of music:characterization, classification,and measurement.Emotion(Washing-ton,D.C.),8(4):494–521,August2008.。
英国蓝调音乐英语作文初中
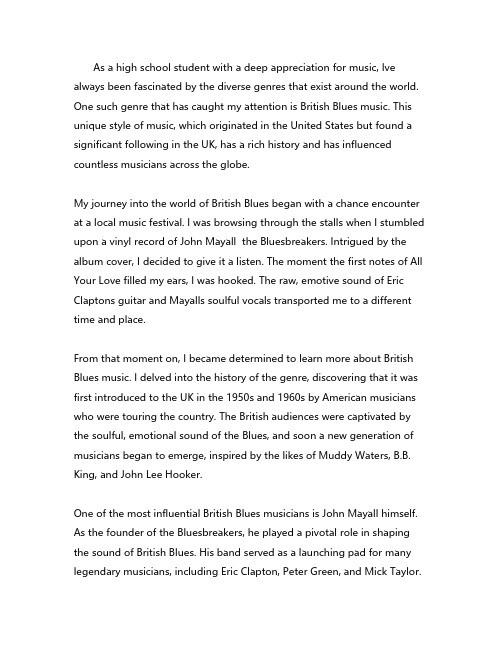
As a high school student with a deep appreciation for music, Ive always been fascinated by the diverse genres that exist around the world. One such genre that has caught my attention is British Blues music. This unique style of music, which originated in the United States but found a significant following in the UK, has a rich history and has influenced countless musicians across the globe.My journey into the world of British Blues began with a chance encounter at a local music festival. I was browsing through the stalls when I stumbled upon a vinyl record of John Mayall the Bluesbreakers. Intrigued by the album cover, I decided to give it a listen. The moment the first notes of All Your Love filled my ears, I was hooked. The raw, emotive sound of Eric Claptons guitar and Mayalls soulful vocals transported me to a different time and place.From that moment on, I became determined to learn more about British Blues music. I delved into the history of the genre, discovering that it was first introduced to the UK in the 1950s and 1960s by American musicians who were touring the country. The British audiences were captivated by the soulful, emotional sound of the Blues, and soon a new generation of musicians began to emerge, inspired by the likes of Muddy Waters, B.B. King, and John Lee Hooker.One of the most influential British Blues musicians is John Mayall himself. As the founder of the Bluesbreakers, he played a pivotal role in shaping the sound of British Blues. His band served as a launching pad for many legendary musicians, including Eric Clapton, Peter Green, and Mick Taylor.Mayalls music is characterized by its rich, harmonicadriven sound and his distinctive piano playing, which adds a unique touch to the traditional Blues style.Another notable figure in the British Blues scene is the late, great Stevie Ray Vaughan. Although he was an American musician, his influence on the British Blues scene cannot be overstated. Vaughans virtuosic guitar playing and soulful vocals inspired countless British musicians, including Gary Moore and Jeff Beck. His album Texas Flood is a testament to his incredible talent and has become a staple in the British Blues music library.As I continued to explore the world of British Blues, I was struck by the diversity of the musicians and the different styles they brought to the genre. From the gritty, raw sound of Peter Greens Fleetwood Mac to the more polished, melodic style of Dire Straits, there is something for everyone in the world of British Blues.One of the most striking aspects of British Blues music is its ability to evoke emotion. The lyrics often tell stories of heartbreak, loss, and struggle, which resonate with listeners on a deep level. The music itself, with its slow, mournful melodies and powerful guitar riffs, has the power to transport you to a different place, allowing you to feel the raw emotion of the music.In conclusion, my exploration of British Blues music has been an enriching and enlightening experience. The genre, with its rich history and diverse range of musicians, has left an indelible mark on the world of music. From the early pioneers like John Mayall to the modernday innovators, BritishBlues continues to inspire and captivate audiences around the world. As a high school student, I am grateful for the opportunity to have discovered this incredible genre and look forward to continuing my musical journey.。
Visualizing and Exploring Personal Music Libraries
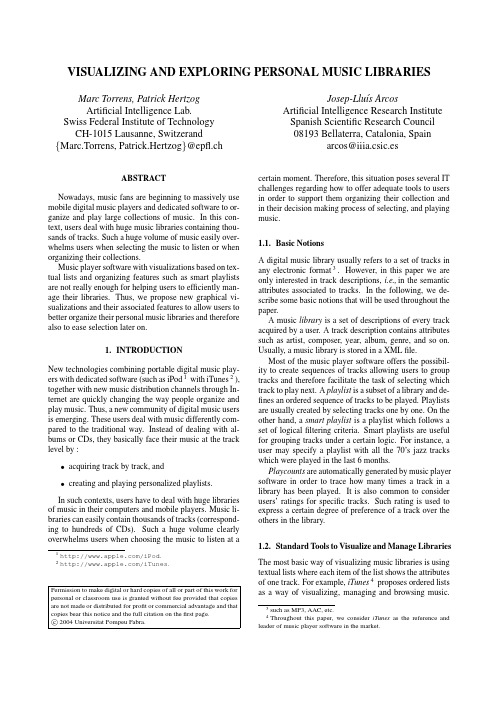
VISUALIZING AND EXPLORING PERSONAL MUSIC LIBRARIESMarc Torrens,Patrick HertzogArtificial Intelligence Lab. Swiss Federal Institute of Technology CH-1015Lausanne,Switzerand {Marc.Torrens,Patrick.Hertzog}@epfl.chJosep-Llu´ıs ArcosArtificial Intelligence Research Institute Spanish Scientific Research Council08193Bellaterra,Catalonia,Spainarcos@iiia.csic.esABSTRACTNowadays,music fans are beginning to massively use mobile digital music players and dedicated software to or-ganize and play large collections of music.In this con-text,users deal with huge music libraries containing thou-sands of tracks.Such a huge volume of music easily over-whelms users when selecting the music to listen or when organizing their collections.Music player software with visualizations based on tex-tual lists and organizing features such as smart playlists are not really enough for helping users to efficiently man-age their libraries.Thus,we propose new graphical vi-sualizations and their associated features to allow users to better organize their personal music libraries and therefore also to ease selection later on.1.INTRODUCTIONNew technologies combining portable digital music play-ers with dedicated software(such as iPod1with iTunes2), together with new music distribution channels through In-ternet are quickly changing the way people organize and play music.Thus,a new community of digital music users is emerging.These users deal with music differently com-pared to the traditional way.Instead of dealing with al-bums or CDs,they basically face their music at the track level by:•acquiring track by track,and•creating and playing personalized playlists.In such contexts,users have to deal with huge libraries of music in their computers and mobile players.Music li-braries can easily contain thousands of tracks(correspond-ing to hundreds of CDs).Such a huge volume clearly overwhelms users when choosing the music to listen at a 1/iPod.2/iTunes.Permission to make digital or hard copies of all or part of this work for personal or classroom use is granted without fee provided that copies are not made or distributed for profit or commercial advantage and that copies bear this notice and the full citation on thefirst page.c 2004Universitat Pompeu Fabra.certain moment.Therefore,this situation poses several IT challenges regarding how to offer adequate tools to users in order to support them organizing their collection and in their decision making process of selecting,and playing music.1.1.Basic NotionsA digital music library usually refers to a set of tracks in any electronic format3.However,in this paper we are only interested in track descriptions,i.e.,in the semantic attributes associated to tracks.In the following,we de-scribe some basic notions that will be used throughout the paper.A music library is a set of descriptions of every track acquired by a user.A track description contains attributes such as artist,composer,year,album,genre,and so on. Usually,a music library is stored in a XMLfile.Most of the music player software offers the possibil-ity to create sequences of tracks allowing users to group tracks and therefore facilitate the task of selecting which track to play next.A playlist is a subset of a library and de-fines an ordered sequence of tracks to be played.Playlists are usually created by selecting tracks one by one.On the other hand,a smart playlist is a playlist which follows a set of logicalfiltering criteria.Smart playlists are useful for grouping tracks under a certain logic.For instance,a user may specify a playlist with all the70’s jazz tracks which were played in the last6months.Playcounts are automatically generated by music player software in order to trace how many times a track in a library has been played.It is also common to consider users’ratings for specific tracks.Such rating is used to express a certain degree of preference of a track over the others in the library.1.2.Standard Tools to Visualize and Manage Libraries The most basic way of visualizing music libraries is using textual lists where each item of the list shows the attributes of one track.For example,iTunes4proposes ordered lists as a way of visualizing,managing and browsing music.3such as MP3,AAC,etc.4Throughout this paper,we consider iTunes as the reference and leader of music player software in the market.As shown in Figure1,track lists can be ordered alphanu-merically by any of the attributes,e.g.,by genre,artist, composer,album,year and so forth.1.2.1.Searching by keywordsA simple mechanism for searching a specific track in a music library is through a keyword-based search function-ality,as offered by ers can enter a keyword and then specify if the search has to be applied to all attributes or just to one of the predetermined ones(namely artists, albums,composers or track names).1.2.2.Searching byfiltersIn iTunes users can also search tracks by selecting three kind offilters:genres,artists and albums.The threefil-ters work with multiple selection and there is no order im-posed by the software.In a results window,thefiltered tracks are ers can then order the tracks al-phanumerically(ascendent or descendent)by any of the attributes.1.2.3.Standard playlists and smart playlistsIn order to organize and manage music libraries,iTunes offers the possibility of creating playlists in two different ways:1)to add tracks in a standard playlist by manually selecting them one by one from the library,or2)to define a set offiltering criteria from which the smart playlist is created.Thesefiltering criteria refer to one of the track attributes and are of the type contains,is,starts,ends,is before,etc.depending on the selected attribute.Other pa-rameters such as the size of the playlist can also be spec-ified,for instance,by the number of tracks,the duration of all tracks,etc.If such parameters are specified,then a selection argument can be chosen such as random,most played,highest rating,and similar others.Finally,iTunes allows users to decide if a smart playlist should be lively updated or not.A smart playlist with live updating is a playlist which is updated according to new tracks added to its associated music library.1.3.Related WorkTo our knowledge,there is not so much work done on visualizing and exploring personal music libraries based on the semantic attributes of tracks.However,there is a relevant research community working on how to visual-ize and organize music based on signal processing tech-niques.In that sense,Islands of Music[8,10]organizes music libraries without requiring genre or other attribute classification because it uses psycho-acoustic models,and then tracks are visualized using a metaphor of geograph-ical maps where islands resemble genres of music styles.A different approach using a heuristic version of Multi-dimensional Scaling(MDS)named FastMap is described in[1].With a similar goal to FastMap,Sonic Browser [6]uses sonic spatialization for navigating into audio li-braries and the Marsyas3D tool[12]proposes techniques based on principal component analysis for browsing audio libraries.Another related work is enlighten in[2]but it is more focussed on how to identify any potential misfits between the designers’views of a product or system,embodied in the device itself,and those of its users.Our work has an-other point of view since we do not consider how to build the whole system but just how to visualize and manage the existing music libraries of users.On the other hand,Beth Logan presented in[3]an ap-proach to form playlists from a given seed song.Their technique is based on their own audio content similarity measure introduced in[4].Paws and Eggen[9]propose to generate automatically playlists with an inductive learn-ing algorithm considering different context-of-use of mu-sic consumers.All these previous works are based on audio informa-tion and use signal processing techniques.Another related work is the Variations2project[5,7]at the Indiana Uni-versity.They exploit music bibliographic data for provid-ing visualization methods in order to assist music students and faculty members.The work presented in this paper is radically different focussing on the attributes associated to the tracks.1.4.The challengeMusic player software with visualizations based on textual lists and organizing features such as smart playlists are not enough for helping users to efficiently manage their libraries which may easily contain thousands of tracks. Thus,in order to avoid the current situation where users are clearly overwhelmed with the problem of selecting tracks,we propose new visualizations and their associated managing features.In the following,we describe our pro-posals and then we compare and evaluate them.We have basically explored three5different visualiza-tions which allow users to have a better overview of the contents of their music libraries and therefore to ease its organization.On the other hand,the twofirst visualiza-tions are shown to be very useful helping users to build playlists graphically instead of having to expressfiltering criteria which may may be confusing to users.2.VISUALIZATION TECHNIQUESThis section presents three different ways of graphically visualizing music libraries consideringfive criteria(thus,five dimensions)which are genre,artist,year and a quanti-tative criterion to be chosen by the user such as playcount, rating,added or last played date.The goal of these visual-izations is two-fold:a)to give an overview of the contents of a music library,and b)to visualize playlists and give some support to manage and organize them.Depending 5As we will see,the twofirst visualizations,using discs and rectan-gles,can be considered as variants of the same basic concept.on the visualization model users get different advantages since they have a different geometric expressiveness.All the explored techniques give a topologic overview of a music library regarding its tracks.2.1.Disc VisualizationThis visualization,called disc visualization,is based on well-known visualization charts in form of ers are used to manage such kind of visualizations which give good percentage and proportional overviews.However, this visualization is different than standard pie charts as we will see in the following sections.2.1.1.DescriptionAs shown in Figure2,the disc is divided in different sec-tors that represent each of the genres of the library6.The size of a sector is proportional to the number of tracks of the associated genre with respect to the whole library. Therefore,the size of a sector is directly proportional to the importance of the corresponding genre within the li-brary.At the same time,sectors are split in sub-sectors representing the artists of the associated genre.Again,the size of sub-sectors is proportional to the number of tracks of the artist.The radius of the disc,from the center to the perimeter,can be seen as the time axis:the center rep-resents the year of the oldest track of the library and over the perimeter the most recent tracks are positioned.Tracks are then depicted as points over the disc according to their attributes,i.e.,genre,artist,year.Tracks belonging to the same album are positioned contiguously,thus it has the ef-fect of producing like arcs of points representing albums. The order in which the albums are depicted is alphanu-meric,and the order for the tracks of the same album is the original order in the album.The quantitative attribute to be chosen by the user(for instance playcount,rating, last played date,added date,etc)is depicted according to different color tonalities.Colors are used to express the exact value for one track in its associated point.The mean value of all the tracks for one genre is also used to color the corresponding sector.Figure3illustrates how playlists and smart playlists can be shown using the disc visualization.Tracks of play-lists without any grouping logic can be depicted by using geometric forms different than regular points which are used in general for the rest of the songs.For instance, in Figure3,the playlist called“Jogging playlist”(num-ber4)is displayed by using bigger points in red.The other example in thefigure for playlists which do not fol-low any geometric logic is the“25last played”(number 3)whose tracks are represented as little red crosses.The other playlists(numbers1,2,and5)are shown as red re-gions since they follow a regular geometric form.The rest of the playlists(numbers6and7)are not highlighted since the user has not activated their corresponding checkboxes.6Thefigures illustrating the disc and rectangle approaches were gen-erated with a real music library of about2.500tracks.In such a visualization,the track currently being played could be highlighted and a path grouping the tracks to be played next could also be displayed.In this way,the user will get an idea of what regions of his library are going to be used in the current music sequence.2.1.2.Interaction PrinciplesIn this section,we describe how users interact with the disc visualization.Basically,the following principles have been identified:Navigation.The attributes of any track of the library can be visualized in textual form by just positioning the cursor over its point.For example,in Figure2,the cursor is over the track I don’t want a lover by Texas,so the song attributes are displayed in the bottom left corner.Also, when moving the cursor over the disc,the artist of the cor-responding sub-sector is highlighted as shown in Figure2 for the Texas group.In a similar way,the year is indicated with a circle,as illustrated in the example of Figure2with the circle of the year1989.Zoom.When a user is interested in getting a more detailed view of his library,he can zoom over any sec-tor of the disc.This zoom will then generate a disc with the same visualization and interaction principles but ap-plied to just the genre of the selected sector.Therefore,in thisfirst zoom level,sectors representing genres become sectors representing artists with sub-sectors representing albums.All the other dimensions and general principles remain the same.Finally,the latest zoom level when se-lecting a sector representing an artist would produce a disc where sectors are the albums of the selected artist,with-out any sub-sector.Thus,in this latest zoom level,users obtain a graphical representation of the tracks for a given artist.Playlist management.As explained in Section2.1.1 and shown in Figure3,the disc visualization can be nicely used to graphically display playlists and smart playlists. Moreover,this visualization can be used to edit or cre-ate new playlists with useful graphical help.The mecha-nism is based on considering playlists as sets and then be-ing able to construct set operations to form new playlists. Multiple playlists can be selected at the same time and then apply operations such as union,intersection,differ-ence,and so on.Since playlists are graphically visualized as sets,it is convenient and useful to apply set operations over them.The resulting playlists are also graphically dis-played.When creating(or editing)playlists with tools like the ones provided by iTunes(either directly selecting songs, or by constructing a set of logic rules for smart playlists), the disc visualization is useful for showing the playlist be-ing created step by step.So,at any moment of the cre-ation(or edition)of a playlist,the user can immediately see how the new playlist changes,its approximate size and its topology.Such procedures help users to have a better idea of which zones of the library are overused or underused,or the zones implied in each playlist.Standard search procedures.When using standard search procedures like the ones described in Section1.2, the disc visualization can also be of a great help by high-lighting thefiltered songs dynamically.In the same way that iTunes dynamically changes the list of tracks in the re-sults window,the visualization highlights the tracks graph-ically.2.2.Rectangle VisualizationThis visualization is similar to the disc visualization but using rectangles instead of discs.In the disc visualization, the time axis was represented along the radius of the disc, and in the rectangle visualization the time axis goes along the vertical axis.Similarly,for this visualization,the at-tribute genre goes along the horizontal axis.The result of this visualization is shown in Figure4.Even if both visualizations have similar features,they may give different user experiences with their advantages and downsides as described in Section3.2.2.1.Interaction PrinciplesThe main principles described for the disc visualization apply to this visualization,however the zoom functional-ity may be differently applied.Zoom.In this visualization,zooms can be done in the same way as for disc visualizations.When zooming over a genre(which is a sub-rectangle),the horizontal axis be-comes the artist dimension.Similarly,when zooming over an artist,the horizontal axis becomes the album dimen-sion.Another way of applying zooms in the rectangle visu-alization is to just consider that all the tracks in the li-brary are always shown,but the scale of the horizontal axis changes.Therefore,using this approach,the user ex-plores the whole library just by using a scroll bar for pan-ning over an specific zone.In this case,when zooming in,the horizontal axis still represents the genres,and the artists within each genre.With a second level of zoom,in addition to genres and artists,the axis also represents the albums for each artist.The horizontal axis and its scroll bar are accordingly adapted depending on the zoom level.2.3.Tree-Map VisualizationThis visualization is using Tree-Maps in a similar way as described in[11]but for visualizing music libraries.Figure5shows three different levels of zoom for the same library.In this visualization7,the size of rectan-gles are always proportional to the number of tracks in the attribute represented by the rectangle.At the same time, rectangles are recursively split in sub-rectangles showing other proportions.For example,in Figure5(a)rectangles are recursively split three times:the whole library(the parent rectangle)is split into genres,each genre is split 7For better showing the concept of Tree-Maps visualizations for mu-sic libraries,we assume that a sub-category of genre,called sub-genre, is available for each track.in its sub-genres,andfinally each sub-genre is split in its artists.Figure5(b)is showing the genre Rock,so there are rectangles representing Rock sub-genres like Rock and roll,Alternative,and so forth.At the same time,these rectangles are split into the artists of the associated sub-genre.Figure5(c)illustrates the visualization of the Rock and roll sub-genre,so rectangles representing artists are shown without anymore splitting.One could make a fur-ther recursion and split each artist rectangle by her/his al-bums.The color of each rectangle indicates a quantitative at-tribute to be chosen by the user,similarly as the previous visualizations,e.g.,playcount,last played date,ratings, and so on.However,in this visualization,since tracks are not depicted,only mean values are represented by differ-ent color tonalities.The interaction mechanism for the Tree-Map visualiza-tion is very straightforward for zooming:the user selects a rectangle,and the parent rectangle shows then the selected attribute.PARISONThe visualizations using discs and rectangles basically of-fer the same functionalities,while the Tree-Map visual-ization is more likely to be used just for giving a better overview of the contents of music libraries.This is be-cause the disc and the rectangle approaches are capable to show information at the track detail whereas it is unclear how to represent tracks using Tree-Maps.A comparison among the different presented visualizations and their fea-tures is summarized as follows:•Visualizations based on discs and rectangles offersimilar functionalities,but also different pros andcons due to their different geometric forms:–Discs give a better visual idea about the pro-portions of sectors and sub-sectors comparedto rectangles and sub-rectangles.–Track points are differently distributed in discsand rectangles.For libraries with more re-cent tracks than old ones,the points are betterplaced in the disc visualization.On the otherhand,libraries which are more homogeneouswith respect to the year of their tracks are bet-ter suited for the rectangle approach.–The zooming feature is more useable with rect-angles since the whole library space can berepresented with the help of scroll bars.Zoom-ing in the disc visualization implies to focus toa smaller portion of the library.–In the rectangle version,both coordinates arevisible(genre/artist/albums and year)thus thepositioning of tracks is easily understood byusers.In the disc representation,the year co-ordinate goes along the radius of the disc sopossibly more efforts could be required by usersto quickly understand it.•Tree-Map visualizations are more adequate to givean overview with respect to the number of tracksbelonging to each attribute represented by the sizeof its rectangles.•The Tree-Map approach is not very well-suited fordisplaying information about tracks or playlists.•Discs and rectangles can be used to visualize,andmore importantly to create and edit playlists.TheTree-Map representation does not offer this possi-bility because tracks are not shown.All the approaches presented in this paper(and also textual lists)should be regarded as complementary by con-sidering the above arguments.In this way,a complete mu-sic player software may allow users to choose among the different approaches.Also,it is feasible to automatically decide which approach has to be used depending on the topology of the library of the user and the action the user is considering,resulting in a really smart music organizer.4.CONCLUSIONSTextual list-based visualizations and organizing features such as smart playlists are not enough to really support users who deal with music collections of thousands of tracks.In order to assist music fans to better manage huge digital music libraries,we have proposed new visualiza-tions and their associated features.However,the proposed approaches should be regarded as complementary to more conventional tools like textual lists.We believe that advanced but yet simple visualizations are critical for supporting the process of exploring and therefore re-discovering personal music collections.Ac-tually,it seems reasonable to believe that many times users may be interested in rediscovering their own music instead of thinking about enlarging their collections.Moreover, users may be interested in exploring their music collec-tion to actually decide what to acquire or listen next.Cur-rently,this rediscovering process can be tedious by using textual lists,while the presented new approaches facilitate such task.er StudiesStronger andfinal arguments for validating the suggested approaches should be given by rigorous user studies.These user studies will be developed with different type of users considering at least factors like technology matureness, age,educational background,and topology of their libraries (size,recency of tracks,homogeneity). AcknowledgmentsWe would like to thank Michel Speiser for his great im-plementation of the ideas shown in this paper during a semester project at the EPFL.We also thank MusicStrands S.A.for providing XML parsers to read real iTunes li-braries.5.REFERENCES[1]Pedro Cano,Martin Kaltenbrunner,Fabien Gouyon,and Eloi Batlle.On the Use of FastMap for Audio In-formation Retrieval and Browsing.In International Conference on Music Information Retrieval(ISMIR 2002),Paris,France,October2002.[2]Iain W.Connell,Thomas R.G.Green,and Ann E.Blandford.Ontological Sketch Models:highlighting user-system misfits.In P.Palanque E.ONeill and P.Johnson,editors,Proceedings of Human Com-puter Interaction(HCI),Bath,England,September 2003.London Springer.[3]Beth Logan.Content-Based Playlist Generation:Ex-ploratory Experiments.In International Conference on Music Information Retrieval(ISMIR2002),Paris, France,October2002.[4]Beth Logan and Ariel Salomon.A Music Similar-ity Function Based on Signal Analysis.In IEEE International Conference on Multimedia and Expo (ICME),Tokyo,Japan,August2001.IEEE Press.[5]Indiana university digital music library project./.[6]D.O.Maidin and M.Fernstrom.The Best of TwoWorlds:Retrieving and Browsing.In Conference of Digital Audio Effects,2000.[7]Mark Notess and Natalia Minibayeva.Variations2:Toward Visual Interfaces for Digital Music Li-braries.In Second International Workshop on Visual Interfaces to Digital Libraries,2002.[8]Elias Pampalk,Andreas Rauber,and Dieter Merkl.Content-based Organization and Visualization of Music Archives.In ACM Multimedia,pages570–579,Juan les Pins,France,December2002.[9]Steffen Pauws and Berry Eggen.PATS:Realizationand User Evaluation of an Automatic Playlist Gener-ator.In International Conference on Music Informa-tion Retrieval(ISMIR2002),Paris,France,October 2002.[10]A.Rauber,E.Pampalk,and D.Merkl.The SOM-enhanced JukeBox:Organization and visualization of music collections based on perceptual models.Journal of New Music Research,32(2):193–210, 2003.[11]Ben Shneiderman.Tree Visualization with Tree-Maps:2-d Space-Filling Approach.ACM Transac-tions on Graphics,11(1):92–99,January1992. [12]G.Tzanetakis and P.Cook.Marsyas3D:A PrototypeAudio Browser-Editor using a Large Scale Immer-sive Visual Audio Display.In International Confer-ence on Auditory Display,2001.Figure1.Managing libraries with iTunes based on textual lists.Figure2.Visualizing music libraries by using discs.Figure3.Visualizing playlists and smart playlists in music libraries by using discs.Figure4.Visualizing music libraries by using rectangles.(a)Tree-Map visualization for a whole music library.(b)Tree-Map visualization for the Rock genre.(c)Tree-Map visualization for the Rock and roll sub-genreFigure5.Tree-Map visualizations applied to music libraries.Different zooms levels are shown for the same library.。
music似然函数
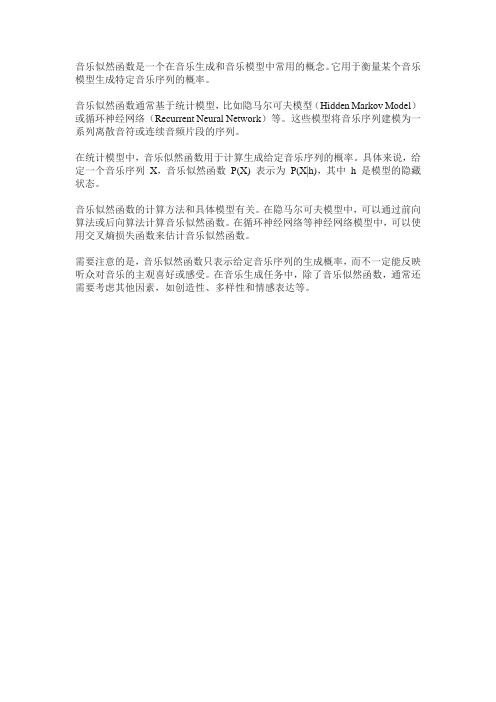
音乐似然函数是一个在音乐生成和音乐模型中常用的概念。
它用于衡量某个音乐模型生成特定音乐序列的概率。
音乐似然函数通常基于统计模型,比如隐马尔可夫模型(Hidden Markov Model)或循环神经网络(Recurrent Neural Network)等。
这些模型将音乐序列建模为一系列离散音符或连续音频片段的序列。
在统计模型中,音乐似然函数用于计算生成给定音乐序列的概率。
具体来说,给定一个音乐序列X,音乐似然函数P(X) 表示为P(X|h),其中h 是模型的隐藏状态。
音乐似然函数的计算方法和具体模型有关。
在隐马尔可夫模型中,可以通过前向算法或后向算法计算音乐似然函数。
在循环神经网络等神经网络模型中,可以使用交叉熵损失函数来估计音乐似然函数。
需要注意的是,音乐似然函数只表示给定音乐序列的生成概率,而不一定能反映听众对音乐的主观喜好或感受。
在音乐生成任务中,除了音乐似然函数,通常还需要考虑其他因素,如创造性、多样性和情感表达等。
音乐种类介绍英文
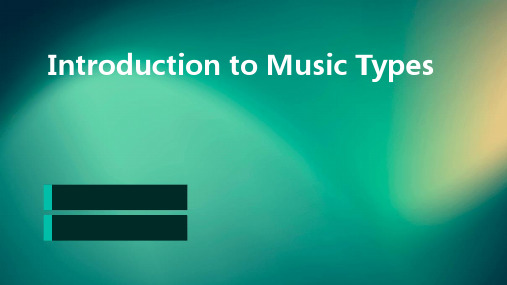
Classic Music
Period
01
Mid 18th century to early 19th century
Features
02
Clear musical form, simple harmony, moderate emotional
Introduction to Music Types
目 录
• Classic Music • Jazz • Rock • Pop
01 Classic Music
Baroque Music
Period: 17th to mid-18th century
Features: Using Baroque instruments such as the harpsichord and organ, with rigorous musical forms, developed counterpoint techniques, and restrained emotional expression.
Bebop
Bebop is a style of jazz music that emerged in the 1940s, known for its fast tempo, complex harmony, and virtual playing
Bebop musicians often played at a multiple fast tempo than traditional jazz, with more enriched memories and harmonies
Swing is a style of jazz music that originated in the 1930s, characterized by a strong rhythmic feel and a prospective swing feel
Singing-voicesep...
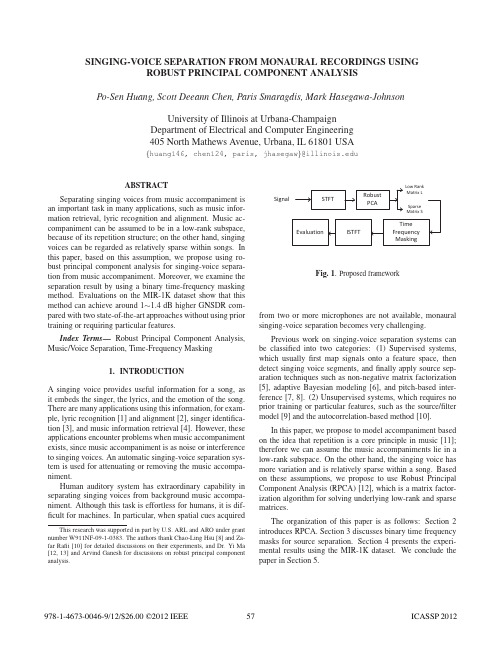
SINGING-VOICE SEPARATION FROM MONAURAL RECORDINGS USING ROBUST PRINCIPAL COMPONENT ANALYSISPo-Sen Huang,Scott Deeann Chen,Paris Smaragdis,Mark Hasegawa-JohnsonUniversity of Illinois at Urbana-ChampaignDepartment of Electrical and Computer Engineering405North Mathews Avenue,Urbana,IL61801USA{huang146,chen124,paris,jhasegaw}@ABSTRACTSeparating singing voices from music accompaniment is an important task in many applications,such as music infor-mation retrieval,lyric recognition and alignment.Music ac-companiment can be assumed to be in a low-rank subspace, because of its repetition structure;on the other hand,singing voices can be regarded as relatively sparse within songs.In this paper,based on this assumption,we propose using ro-bust principal component analysis for singing-voice separa-tion from music accompaniment.Moreover,we examine the separation result by using a binary time-frequency masking method.Evaluations on the MIR-1K dataset show that this method can achieve around1∼1.4dB higher GNSDR com-pared with two state-of-the-art approaches without using prior training or requiring particular features.Index Terms—Robust Principal Component Analysis, Music/V oice Separation,Time-Frequency Masking1.INTRODUCTIONA singing voice provides useful information for a song,as it embeds the singer,the lyrics,and the emotion of the song. There are many applications using this information,for exam-ple,lyric recognition[1]and alignment[2],singer identifica-tion[3],and music information retrieval[4].However,these applications encounter problems when music accompaniment exists,since music accompaniment is as noise or interference to singing voices.An automatic singing-voice separation sys-tem is used for attenuating or removing the music accompa-niment.Human auditory system has extraordinary capability in separating singing voices from background music accompa-niment.Although this task is effortless for humans,it is dif-ficult for machines.In particular,when spatial cues acquired This research was supported in part by U.S.ARL and ARO under grant number W911NF-09-1-0383.The authors thank Chao-Ling Hsu[8]and Za-far Rafii[10]for detailed discussions on their experiments,and Dr.Yi Ma [12,13]and Arvind Ganesh for discussions on robust principal component analysis.SignalFig.1.Proposed frameworkfrom two or more microphones are not available,monaural singing-voice separation becomes very challenging.Previous work on singing-voice separation systems can be classified into two categories:(1)Supervised systems, which usuallyfirst map signals onto a feature space,then detect singing voice segments,andfinally apply source sep-aration techniques such as non-negative matrix factorization [5],adaptive Bayesian modeling[6],and pitch-based inter-ference[7,8].(2)Unsupervised systems,which requires no prior training or particular features,such as the source/filter model[9]and the autocorrelation-based method[10].In this paper,we propose to model accompaniment based on the idea that repetition is a core principle in music[11]; therefore we can assume the music accompaniments lie in a low-rank subspace.On the other hand,the singing voice has more variation and is relatively sparse within a song.Based on these assumptions,we propose to use Robust Principal Component Analysis(RPCA)[12],which is a matrix factor-ization algorithm for solving underlying low-rank and sparse matrices.The organization of this paper is as follows:Section2 introduces RPCA.Section3discusses binary time frequency masks for source separation.Section4presents the experi-mental results using the MIR-1K dataset.We conclude the paper in Section5.2.ROBUST PRINCIPAL COMPONENT ANALYSIS Cand`e s et al.[12]proposed RPCA,which is a convex pro-gram,for recovering low-rank matrices when a fraction of their entries have been corrupted by errors,i.e.,when the ma-trix is sufficiently sparse.The approach,Principal Compo-nent Pursuit,suggests solving the following convex optimiza-tion problem:minimize ||L ||∗+λ||S ||1subject to L +S =Mwhere M ∈R n 1×n 2,L ∈R n 1×n 2,S ∈R n 1×n 2.||·||∗and ||·||1denote the nuclear norm (sum of singular values)and the L1-norm (sum of absolute values of matrix entries),respectively.λ>0is a trade-off parameter between the rank of L and the sparsity of S.As suggested in [12],using a value of λ=1/ max(n 1,n 2)is a good rule of thumb,which can then be adjusted slightly to obtain the best possible result.We explore λk =k/max(n 1,n 2)with different values of k ,thus testing different tradeoffs between the rank of L and the sparsity of S.Since music instruments can reproduce the same sounds each time they are played and music has,in general,an under-lying repeating musical structure,we can think of music as a low-rank signal.Singing voices,on the contrary,have more variation (higher rank)but are relatively sparse in the time and frequency domains.We can then think of singing voices as components making up the sparse matrix.By RPCA,we ex-pect the low-rank matrix L to contain music accompaniment and the sparse matrix S to contain vocal signals.We perform the separation as follows:First,we compute the spectrogram of music signals as matrix M ,calculated from the Short-Time-Fourier Transform (STFT).Second,we use the inexact Augmented Lagrange Multiplier (ALM)method [13],which is an efficient algorithm for solving RPCA problem,to solve L +S =|M |,given the input magnitude of M .Then by RPCA,we can obtain two output matrices L and S .From the example spectrogram,Figure 2,we can observe that there are formant structures in the sparse matrix S ,which indicates vocal activity,and musical notes in the low-rank matrix L .Note that in order to obtain waveforms of the esti-mated components,we record the phase of original sig-nals P =phase (M ),append the phase to matrix L and S by L (m,n )=Le jP (m,n ),S (m,n )=Se jP (m,n ),for m =1...n 1and n =1...n 2,and calculate the inverse STFT (ISTFT).The source codes and sound examples are available at /wiki/Software.3.TIME-FREQUENCY MASKINGGiven the separation results of the low-rank L and sparse S matrices,we can further apply binary time-frequency mask-ing methods for better separation results.We definebinary(a)Original MatrixM(b)Low-Rank MatrixL(c)Sparse Matrix MFig.2.Example RPCA results for yifen 201at SNR=5for (a)theoriginal matrix,(b)the low-rank matrix,and (c)the sparse matrix.time frequency masking M b as follows:M b (m,n )=1|S (m,n )|>gain ∗|L (m,n )|0otherwise(1)for all m =1...n 1and n =1...n 2.Once the time-frequency mask M b is computed,it is ap-plied to the original STFT matrix M to obtain the separation matrix X singing and X music ,as shown in Equation (2).X singing (m,n )=M b (m,n )M (m,n )X music (m,n )=(1−M b (m,n ))M (m,n )(2)for all m =1...n 1and n =1...n 2.To examine the effectiveness of the binary mask,we assign X singing =S and X music =L directly as the case with no mask .4.EXPERIMENTAL RESULTS4.1.DatasetWe evaluate our system using the MIR-1K dataset1.There are 1000song clips encoded with a sample rate of16kHz,with a duration from4to13sec.The clips were extracted from 110Chinese karaoke pop songs performed by both male and female amateurs.The dataset includes manual annotations of the pitch contours,lyrics,indices and types for unvoiced frames,and indices of the vocal and non-vocal frames.4.2.EvaluationFollowing the evaluation framework in[8,10],we create three sets of mixtures using the1000clips of the MIR-1K dataset.For each clip,the singing voice and the music accom-paniment were mixed at-5,0,and5dB SNRs,respectively. Zero indicates that the singing voice and the music are at the same energy levels,negative values indicate the energy of the music accompaniment is larger than the singing voice,and so on.For source separation evaluation,in addition to evaluating the Global Normalized Source to Distortion Ratio(GNSDR) as[8,10],we also evaluate our performance in terms of Source to Interference Ratio(SIR),Source to Artifacts Ratio (SAR),and Source to Distortion Ratio(SDR)by BSS-EV AL metrics[14].The Normalized SDR(NSDR)is defined as NSDR(ˆv,v,x)=SDR(ˆv,v)−SDR(x,v)(3) whereˆv is the resynthesized singing voice,v is the origi-nal clean singing voice,and x is the mixture.NSDR is for estimating the improvement of the SDR between the prepro-cessed mixture x and the separated singing voiceˆv.The GNSDR is calculated by taking the mean of the NSDRs over all mixtures of each set,weighted by their length.GNSDR(ˆv,v,x)= Nn=1w n NSDR(ˆv n,v n,x n)Nn=1w n(4)where n is the index of a song and N is the total number of the songs,and w n is the length of the n th song.Higher values of SDR,SAR,SIR,and GNSDR represent better separation quality2.4.3.ExperimentsIn the separation process,the spectrogram of each mixture is computed using a window size of1024and a hop size of 256(at Fs=16,000).Three experiments were run:(1)an eval-uation of the effect ofλk for controlling low rankness and sparsity,(2)an evaluation of the effect of the gain factor with 1https:///site/unvoicedsoundseparation/mir-1k2The suppression of noise is reflected in SIR.The artifacts introduced by the denoising process are reflected in SAR.The overall performance is reflected in SDR.a binary mask,and(3)a comparison of our results with the previous literature[8,10]in terms of GNSDR.(1)The effect ofλkThe valueλk=k/(max(n1,n2)can be used for trading off the rank of L with the sparsity of S.The matrix S is sparser with higherλk,and vice versa.Intuitively,for the source sep-aration problem,if the matrix S is sparser,there is less inter-ference in the matrix S;however,deletions of original signal components might also result in artifacts.On the other hand, if S matrix is less sparse,the signal contains less artifacts, but there is more interference from the other sources that ex-ist in matrix S.Experimental results(Figure3)show these trends for both the case of no mask and the case of binary mask(gain=1)at different SNR values.(a)no mask(b)binary maskparison between the case using(a)no mask and(b)a binary mask at SNR={-5,0,5},k(ofλk)={0.1,0.5,1,1.5,2,2.5}, and gain=1.(2)The effect of the gain factor with a binary maskThe gain factor adjusts the energy between the sparse matrix and the low-rank matrix.As shown in Figure4,we exam-ine different gain factors{0.1,0.5,1,1.5,2}atλ1where SNR={-5,0,5}.Similar to the effect ofλk,a higher gain factor results in a lower power sparse matrix S.Hence,there is larger interference and fewer artifacts at high gain,and vice versa.(3)Comparison with previous systemsFrom previous observations,moderate values forλk and the gain factor balance the separation results in terms of SDR, SAR,and SIR.We empirically chooseλ1(also suggested in [12])and gain=1to compare with previous literature on singing-voice separation in terms of GNSDR using the MIR-1K dataset[8,10].Hsu and Jang[8]performed singing-voice separation us-ing a pitch-based inference separation method on the MIR-1K dataset.Their method combines the singing-voice separa-tion method[7],the separation of the unvoiced singing-voice frames,and a spectral subtraction method.Rafii and Pardo proposed a singing-voice separation method by extracting theparison between various gain factors using a binarymask with λ1repeating musical structure estimated by the autocorrelation function [10].As shown in Figure 5,both our approach using binary mask and our approach using no mask achieve better GNSDR compared with previous approaches [8,10],with the no mask approach providing the best overall results.These methods are compared to an ideal situation where an ideal binary mask is used as the upper-bound performance of the singing-voice separation task with algorithms based on binary masking technique.parison between Hsu [8],Rafii [10],the binary mask ,the no mask ,and the ideal binary mask cases in terms of GNSDR.5.CONCLUSION AND FUTURE WORKIn this paper,we proposed an unsupervised approach which applies robust principal component analysis on singing-voice separation from music accompaniment for monaural record-ings.We also examined the parameter λk in RPCA and the gain factor with a binary mask in detail.Without using prior training or requiring particular features,we are able to achieve around 1∼1.4dB higher GNSDR compared with two state-of-the-art approaches,by taking into account the rank of mu-sic accompaniment and the sparsity of singing voices.There are several ways in which this work could be ex-tended,for example,(1)to investigate dynamic parameter se-lection methods according to different contexts,or (2)to ex-pand current work to speech noise reduction,since in many situations,the noise spectrogram is relatively low-rank,while the speech spectrogram is relatively sparse.6.REFERENCES[1] C.-K.Wang,R.-Y .Lyu,and Y .-C.Chiang,“An automaticsinging transcription system with multilingual singing lyric recognizer and robust melody tracker,”in Proc.of Interspeech ,2003,pp.1197–1200.[2]H.Fujihara,M.Goto,J.Ogata,K.Komatani,T.Ogata,andH.G.Okuno,“Automatic synchronization between lyrics and music cd recordings based on viterbi alignment of segregated vocal signals,”in Proc.of ISM ,122006,pp.257–264.[3] A.Berenzweig,D.P.W.Ellis,and wrence,“Using voicesegments to improve artist classification of music,”in AES 22nd International Conference:Virtual,Synthetic,and Enter-tainment Audio ,2002.[4]H.Fujihara and M.Goto,“A music information retrieval sys-tem based on singing voice timbre,”in ISMIR ,2007,pp.467–470.[5]S.Vembu and S.Baumann,“Separation of vocals from poly-phonic audio recordings,”in ISMIR ,2005,pp.337–344.[6] A.Ozerov,P.Philippe,F.Bimbot,and R.Gribonval,“Adap-tation of Bayesian models for single-channel source separation and its application to voice/music separation in popular songs,”Audio,Speech,and Language Processing,IEEE Transactions on ,vol.15,no.5,pp.1564–1578,July 2007.[7]Yipeng Li and DeLiang Wang,“Separation of singing voicefrom music accompaniment for monaural recordings,”Audio,Speech,and Language Processing,IEEE Transactions on ,vol.15,no.4,pp.1475–1487,May 2007.[8] C.-L.Hsu and J.-S.R.Jang,“On the improvement of singingvoice separation for monaural recordings using the MIR-1K dataset,”Audio,Speech,and Language Processing,IEEE Transactions on ,vol.18,no.2,pp.310–319,Feb.2010.[9]J.-L.Durrieu,G.Richard, B.David,and C.Fevotte,“Source/filter model for unsupervised main melody extraction from polyphonic audio signals,”Audio,Speech,and Language Processing,IEEE Transactions on ,vol.18,no.3,pp.564–575,March 2010.[10]Z.Rafii and B.Pardo,“A simple music/voice separationmethod based on the extraction of the repeating musical struc-ture,”in ICASSP ,May 2011,pp.221–224.[11]H.Schenker,Harmony ,University of Chicago Press,1954.[12]Emmanuel J.Cand`e s,Xiaodong Li,Yi Ma,and John Wright,“Robust principal component analysis?,”J.ACM ,vol.58,pp.11:1–11:37,Jun.2011.[13]Z.Lin,M.Chen,L.Wu,and Y .Ma,“The augmented La-grange multiplier method for exact recovery of corrupted low-rank matrices,”Tech.Rep.UILU-ENG-09-2215,UIUC,Nov.2009.[14] E.Vincent,R.Gribonval,and C.Fevotte,“Performance mea-surement in blind audio source separation,”Audio,Speech,and Language Processing,IEEE Transactions on ,vol.14,no.4,pp.1462–1469,July 2006.。
2024届浙江省宁波市高三下学期第二次模拟考试英语试题

宁波市2023学年第二学期高考与选考模拟考试英语试卷注意事项:1.答卷前,考生务必将自己的姓名、准考证号填写在答题卡上。
2.回答选择题时,选出每小题答案后,用铅笔把答题卡上对应题目的答案标号涂黑。
如需改动,用橡皮擦干净后,再选涂其他答案标号。
回答非选择题时,将答案写在答题卡上,写在本试卷上无效。
3.考试结束后,将本试卷和答题卡一并交回。
第一部分听力(共两节,满分30分)做题时,先将答案标在试卷上。
录音内容结束后,你将有两分钟的时间将试卷上的答案转涂到答题卡上。
第一节(共5小题;每小题1.5分,满分7.5分)听下面5段对话.每段对话后有一个小题,从题中所给的A、B、C三个选项中选出最佳选项,并标在试卷的相应位置。
听完每段对话后,你都有10秒钟的时间来回答有关小题和阅读下一小题。
每段对话仅读一遍。
1. Where does the conversation probably take place?A. In a hospital.B. In a factory.C. In a hotel.2. When will the speakers arrive at the airport probably?A. At 3: 30.B. At 4: 30.C. At 5: 30.3. What suggestion does the woman give to the man?A. Avoiding working at night.B. Getting all the parts from Japan.C. Buying a new washing machine.4. What are the speakers talking about?A. How to get to New York.B. How to enter for a course.C. How to get a driving licence.5. What kept the woman awake last night?A. The heat.B. The repairs of the power.C. The storm.第二节(共15小题;每小题1.5分,满分22.5分)听下面5段对话或独白。
必修二Unit5Musics单词详解
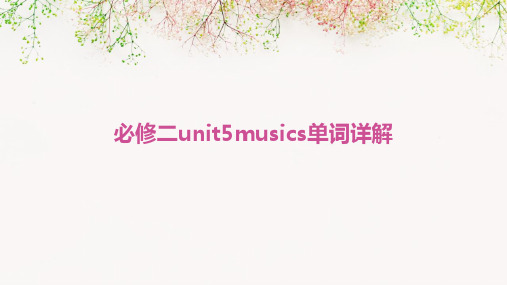
古典音乐时期以海顿、莫扎特和贝多芬等作曲家为代表,其作品结构严谨、逻辑清晰,追求平衡与和 谐。古典音乐时期的作品如莫扎特的《安魂曲》和贝多芬的《命运交响曲》等,都展现了其高度的艺 术价值。
浪漫音乐时期
总结词
浪漫音乐时期是欧洲音乐的另一高峰期 ,其特点为情感丰富、个性化强烈和民 族特色鲜明。
VS
详细描述
浪漫音乐时期的作品多以个人情感和社会 问题为主题,如肖邦的《圆舞曲》和柴可 夫斯基的《胡桃夹子》等。浪漫音乐时期 也注重民族特色,如德沃夏克的《斯拉夫 舞曲》和格里格的《皮尔金特》等作品, 展现了各国的民族风情。
05
音乐与社会文化
音乐与教育
音乐教育
音乐作为教育的一部分,能够培养学生的审美观念 、创造力和团队协作能力。
03
音乐术语
和声
01
02
03
04
和声定义
和声是指两个或多个音符同时 发出的声音,通过不同音符的 组合,创造出丰富的音响效果 。
和声的功能
在音乐作品中,和声可以起到 建立背景、塑造情感、引导旋 律走向等作用,是构成音乐作 品的重要元素之一。
和声的分类
根据不同的分类标准,和声可 以分为许多类型,如按照声部 可以分为高音和低音,按照音 程关系可以分为密集和开放等 。
调性的运用
在作曲中,调性的运用非常重 要,通过精心设计,可以创造 出富有表现力的音乐作品。
04
音乐历史
中世纪音乐
总结词
中世纪音乐是欧洲历史上最早的音乐时期,其特点为宗教性 、单声部和即兴性。
详细描述
中世纪音乐以宗教为主要内容,多为合唱形式,以圣咏为主 要表现形式,强调声音的和谐与纯净。同时,中世纪音乐也 具有即兴性,乐师们会根据旋律即兴演奏或演唱。
2019统编人教版高中英语必修第二册unit 5《Music》全单元教案教学设计
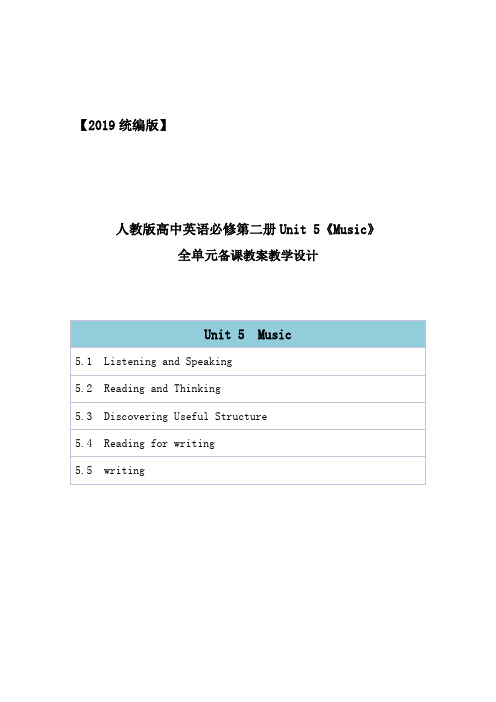
【2019统编版】人教版高中英语必修第二册Unit 5《Music》全单元备课教案教学设计Unit 5 MusicListening and Speaking【教学目标】1. Instruct students to get main facts by listening and motivate them to talk about the topics about music, the types of music, and how the music makes them feel.2. Develop students’ sense of cooperative learning and individual thinking capability.3. Develop students’ different listening skills to solve different listening comprehensive problems.4. Help students to understand how to use the structures “past participle as adverbial”.【教学重难点】Prompt students to talk about the related topics, such as types of music they know, their favourite type of music, how music makes them feel, and how to use past participle as adverbial.【教学过程】Step 1 Lead inThe teacher is advised to talk with their students about music.Boys and girls, before we listen, let’s work in pairs and discuss what type of music you know.Which type is your favorite? How does it make you feel? Share your ideas with partners.I know Chinese traditional music/classical music/country music/hip-hop/jazz/pop music/Latin music/rap/rock/punk…I like classical music. It makes me feel full of energy and happy.Step 2: PredictionAfter their small talk, the teacher can move on by finishing the following task: See the pictures and give the correct answers.1. What are the people doing in the picture below?2. Match the pictures with the correct types of music.A. Chinese traditionalB. classicalC. country musicD. hip-hop1_______________2_______________3_______________4_______________Step 3: Summary of the main ideaListeningI. Play the radio about The Sound of Music (音乐之声), and let students finish the following tasks.A star has come out to tell me1.___________________ to goBut deep in the dark-green shadowsAre voices that urge me to staySo I pause and I wait and I listenFor one more sound for one more lovely thing2.___________________ might say…The hills are alive with the sound of musicWith songs they have sung 3.__________________The hills fill my heart with the sound of musicMy heart 4.__________________ every song it hearsMy heart wants to beat like the wings of the birds that rise from the lake to the treesMy heart wants to sigh like the chime that flies from a church on a breezeTo laugh like a brook 5.__________________ and falls over stones in its wayTo sing through the night like a lark who is 6._____________I go to the hills when my heart is lonelyI know I will hear what I’ve heard beforeMy heart will 7.______________ the sound of musicAnd I’ll sing once moreII. The reporter paraphrased some of the answers the students gave him. Listen to the interviews again and complete the sentences with the words you hear.1. A: Country music touches my heart.B: So you like music that’s _______of _______?2. A: When I listen to hip-hop, I just have to move!B: So it makes you want to _______?3. A: Classical music makes me feel like I’m sitting beside a quiet stream and enjoying nature.B: So to you, it’s _______ and _______?Learning new wordsList the new words in the lesson, tell students the meaning of these words and give some examples.News words: classical, energy, soul…Talking projectGuide students to do speaking practice.I. Talk in pairs. Interview each other about music. Use the picture below for ideas.A: What kind of music do you like?B: I like techno music.A: What makes it so special to you?B: I like to listen to it when l exercise. It gives me energy.II. Work in pairs or groups and role play a conversation.●Suppose you are a reporter and interviewing the students who about music.➢I like to…➢Chinese traditional song/classical music/hip-hop music/country music…➢Listen to/play/sing…Unit 5 MusicReading and Thinking【教学目标】1. To acquire the basic usage of the new words and express concerning how computers and the Internet help us experience music.2. Enable students to understand the main information and text structure of the reading text.3. Enable students to understand past participle as adverbial.【教学重难点】1. Guide students to pay attention to reading strategies, such as prediction, self-questioning and scanning.2. To talk about the advantages and disadvantages of being a member of virtual choir.3. Lead students to understand past participle as adverbial;【教学过程】Step 1 PredictionAsk students the question.How can computers and the Internet help us experience music differently?Step 2: Learning new wordsLearn words:perform,enable,prove,award,and fall in love with…New words practiceIn order to have a good _______________ (perform), I have made good preparations for it.At present, developing the ___________ (able) of the students is an important task in our daily teaching activity.Step 3: Learning sentence patternsIntroduce the sentence patterns in the lesson and give some examples and explanation1. as 引导定语从句,意为“正如,正像”2. 过去分词(短语)作状语as引导定语从句的常用句式有:as is known to all 众所周知as we all know我们都知道as we can see正如我们所看到的as is reported正如报道的as is often the case这是常有的事as is mentioned above如上所述Step 4: Fast reading tasksGuide student to read the article quickly, teach some reading skills and do some exercises.Task of the first fast reading:Read quickly and figure out the key words of each paragraph.•Paragraph 1: enable•Paragraph 2: award•Paragraph 3: performanceTask of the second fast reading:1. What is mainly discussed in this passage?2. Which paragraph mentions background information about the virtual choir?3. Which paragraph mentions the conclusion of the virtual choir?Step 5: Careful reading tasksGuide student to read the article carefully and do some exercises.1. What is the attitude towards the virtual choir?2. Why does the virtual choir prove to be a good influence on the lives of many people?3. If you want to take part in a virtual choir, you need….Step 6: Study reading tasksAnalyze two difficult sentences in the text.1. Imagine having the opportunity to sing together with hundreds of other people while you are at home alone.2. A virtual choir enables them to add their voices to those of other individuals and become part of the global community.Step 7 Homework:Review what we have learned and find out the key language points in the text.Unit 5 MusicDiscovering Useful Structure【教学目标】1. Get students to have a good understanding the basic usage of past word segmentation as past segmentation as predicative and adverbial.2. Strengthen students’ great interest in grammar learning.3. Instruct students to express their ideas with this grammar correctly.【教学重难点】How to enable students to use the structure and meaning of past word segmentation as past segmentation as predicative and adverbial.【教学过程】Step 1 Lead-inGive some messages and ask students to guess who she is.英语过去分词的句子。
关于音乐付费的英语作文
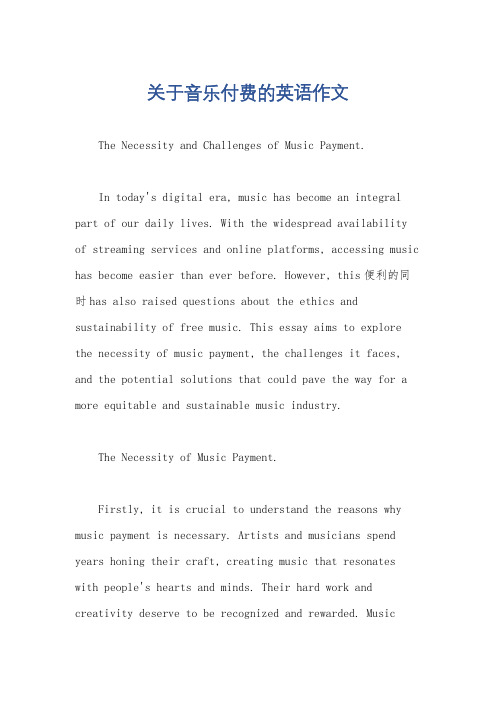
关于音乐付费的英语作文The Necessity and Challenges of Music Payment.In today's digital era, music has become an integral part of our daily lives. With the widespread availability of streaming services and online platforms, accessing music has become easier than ever before. However, this便利的同时has also raised questions about the ethics and sustainability of free music. This essay aims to explore the necessity of music payment, the challenges it faces, and the potential solutions that could pave the way for a more equitable and sustainable music industry.The Necessity of Music Payment.Firstly, it is crucial to understand the reasons why music payment is necessary. Artists and musicians spend years honing their craft, creating music that resonates with people's hearts and minds. Their hard work and creativity deserve to be recognized and rewarded. Musicpayment not only ensures that artists are fairly compensated for their efforts but also encourages them to continue creating and innovating.Moreover, music payment supports the sustainability of the music industry. Music production, distribution, and promotion require significant resources and investments. Payment for music helps to fund these operations, ensuring that the industry can continue to thrive and provide us with the diverse and rich musical experiences we enjoy.Additionally, music payment also acts as a deterrent against piracy and illegal downloads. When music is freely available, it becomes easier for individuals to obtain it without paying for it, leading to losses for artists and the music industry. By making music available for payment, we can reduce piracy and ensure that artists receive the royalties they deserve.Challenges Facing Music Payment.Despite the necessity of music payment, severalchallenges hinder its widespread adoption. One of the primary challenges is the perception that free music is the norm. Many individuals are accustomed to accessing music without paying for it, and convincing them to change their habits can be difficult.Another challenge is the variety of music streaming services and platforms available. With so many options, it can be confusing for consumers to determine which service offers the best value for their money. This leads to a situation where many individuals may opt for the free tier of a streaming service or choose not to pay for music at all.Additionally, the cost of music payment can be abarrier for some individuals. While some streaming services offer affordable subscription plans, others can be quite pricey. This can prevent some people, especially those on a tight budget, from accessing paid music.Potential Solutions.To overcome these challenges and promote music payment, several solutions can be considered. Firstly, education and awareness-raising campaigns can be conducted to inform individuals about the importance of music payment and the benefits it brings to artists and the music industry.Secondly, streaming services can offer more transparent pricing and value-added features to attract consumers. By providing a clear breakdown of subscription costs and exclusive content, streaming services can make it easierfor individuals to understand the value of paying for music.Moreover, collaborations and partnerships between streaming services, artists, and labels can help to promote music payment. By working together, they can create marketing campaigns and promotional activities that encourage individuals to pay for music and support artists.In conclusion, music payment is crucial for recognizing and rewarding the hard work and creativity of artists. It supports the sustainability of the music industry, acts asa deterrent against piracy, and ensures that music remainsa vibrant and diverse art form. While challenges such as perception, variety, and cost exist, solutions like education, transparent pricing, and collaborations can help to promote music payment and create a more equitable and sustainable music industry.。
livemusic
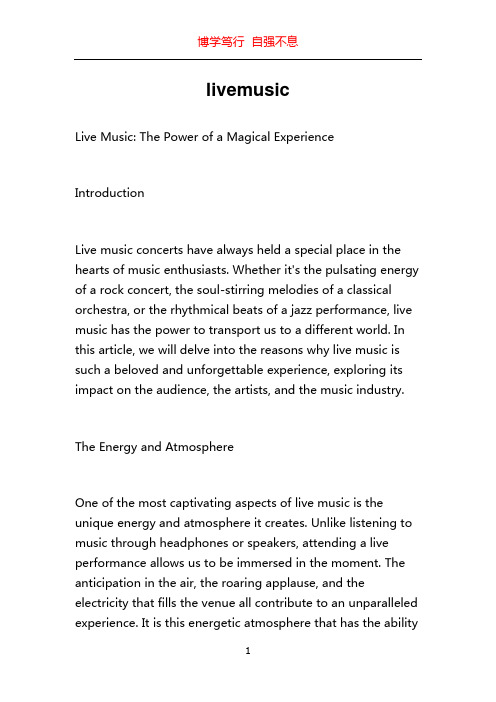
livemusicLive Music: The Power of a Magical ExperienceIntroductionLive music concerts have always held a special place in the hearts of music enthusiasts. Whether it's the pulsating energy of a rock concert, the soul-stirring melodies of a classical orchestra, or the rhythmical beats of a jazz performance, live music has the power to transport us to a different world. In this article, we will delve into the reasons why live music is such a beloved and unforgettable experience, exploring its impact on the audience, the artists, and the music industry.The Energy and AtmosphereOne of the most captivating aspects of live music is the unique energy and atmosphere it creates. Unlike listening to music through headphones or speakers, attending a live performance allows us to be immersed in the moment. The anticipation in the air, the roaring applause, and the electricity that fills the venue all contribute to an unparalleled experience. It is this energetic atmosphere that has the abilityto unite people from different walks of life, igniting a shared love for music and creating a sense of camaraderie among concert-goers.The Emotional ConnectionMusic has a profound ability to evoke emotions, and live performances take this connection to another level. When the artist is on stage, pouring their heart and soul into their craft, it resonates with the audience in a way that recorded music simply cannot achieve. The raw emotions conveyed through voice, instruments, and lyrics have the power to move us, bringing tears to our eyes, creating a surge of joy, or awakening deep-seated emotions we didn't even know existed. It is in these magical moments that live music becomes a personal and transformative experience for each individual present.The Intimate Connection with ArtistsOne of the most unique aspects of live music is the opportunity to witness the talent and skill of artists up close and personal. Regardless of whether you are in a small venue or a massive arena, the energy and intensity of a live performance are palpable. The connection formed betweenthe artist and the audience is electric, with each side feeding off the energy of the other. It is not uncommon for artists to engage in banter, interact with the crowd, or even surprise fans with impromptu moments of improvisation. This intimacy fosters a sense of admiration and respect for the artists, cultivating a deep appreciation for their craft.The Impact on ArtistsLive music performances also hold immense significance for the artists themselves. For many musicians, performing in front of a live audience is what drives them to create music in the first place. The energy and feedback from the crowd fuel their passion and inspire them to continue pushing boundaries artistically. Moreover, live performances allow artists to connect with their fans on a personal level, creating lifelong memories and building a loyal following. In this digital age, where music is often consumed through screens and headphones, the importance of this direct connection cannot be overstated.The Thriving Music IndustryLive music plays a crucial role in the music industry's overall health and vitality. Concerts and festivals provide artists witha significant source of income, enabling them to continue making music. Furthermore, these events have a ripple effect, benefitting various sectors of the industry, such as sound engineers, lighting technicians, venue owners, and merchandise sellers. The economic impact of live music is substantial, contributing to local economies and keeping the industry flourishing.ConclusionLive music has an undeniable power to captivate, inspire, and unite people in a way that few other art forms can. It transcends barriers of age, language, and culture, creating a shared experience that leaves a lasting impact on all who attend. The energy, the emotional connection, the intimate interaction with artists, the impact on musicians, and the thriving music industry all contribute to the magic of live music. So, next time you have the opportunity to attend a live concert, embrace it with open arms, and let the music take you on an unforgettable journey.。
2025版高考英语一轮总复习重点单词必修第二册Unit5Music
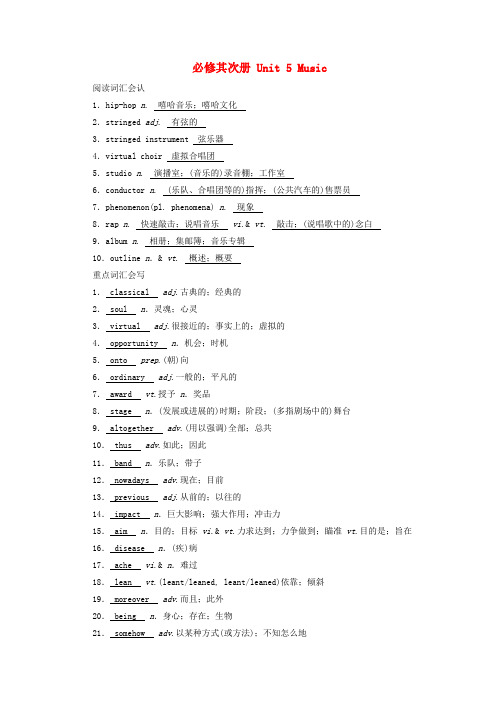
必修其次册 Unit 5 Music阅读词汇会认1.hip-hop n. 嘻哈音乐;嘻哈文化2.stringed adj. 有弦的3.stringed instrument 弦乐器4.virtual choir 虚拟合唱团5.studio n. 演播室;(音乐的)录音棚;工作室6.conductor n. (乐队、合唱团等的)指挥;(公共汽车的)售票员7.phenomenon(pl. phenomena) n. 现象8.rap n. 快速敲击;说唱音乐vi.& vt. 敲击;(说唱歌中的)念白9.album n. 相册;集邮簿;音乐专辑10.outline n.& vt. 概述;概要重点词汇会写1. classical adj.古典的;经典的2. soul n.灵魂;心灵3. virtual adj.很接近的;事实上的;虚拟的4. opportunity n.机会;时机5. onto prep.(朝)向6. ordinary adj.一般的;平凡的7. award vt.授予n.奖品8. stage n.(发展或进展的)时期;阶段;(多指剧场中的)舞台9. altogether adv.(用以强调)全部;总共10. thus adv.如此;因此11. band n.乐队;带子12. nowadays adv.现在;目前13. previous adj.从前的;以往的14. impact n.巨大影响;强大作用;冲击力15. aim n.目的;目标vi.& vt.力求达到;力争做到;瞄准vt.目的是;旨在16. disease n.(疾)病17. ache vi.& n.难过18. lean vt.(leant/leaned, leant/leaned)依靠;倾斜19. moreover adv.而且;此外20. being n.身心;存在;生物21. somehow adv.以某种方式(或方法);不知怎么地拓展词汇会变1. energy n.能源;能量;精力→ energetic adj.精力足够的2. composition n.成分;(音乐、艺术、诗歌的)作品→ compose v.作曲,谱写→ composer n.作曲者;作曲家3. perform vi.& vt.表演;履行;执行→performance n.表演;演技;表现→performer n.表演者;演员4. enable vt.使能够;使可能→ able adj.有实力的→ ability n.实力→disable vt.使丢失实力;使残疾→disability n.残疾;缺陷→unable adj.不能的;没有实力的→ disabled adj.丢失实力的;有残疾的5. prove vt.证明;呈现→ proof n.证据6. original adj.原来的;独创的;原作的n.原件;原作→ originally adv.最初;原来→ origin n.起源7. gradual adj.慢慢的;渐进的→ gradually adv.慢慢地8. capable adj.有实力的;有才能的→incapable adj.没有实力的→capacity n.容量;实力9. relief n.(焦虑、苦痛的)减轻或消退;(不快过后的)宽慰、轻松或解脱→relieve vt.使减轻;缓解→ relieved adj.轻松的;解脱的10. cure vt.治愈;治好(疾病);解决(问题) n.药物;治疗;(解决问题、改善糟糕状况的)措施→ curable adj.可治愈的→ incurable adj.不行治愈的11. unemployed adj.失业的;待业的→ employ vt.雇用;利用→ employment n.就业;运用;雇用;工作→ employer n.雇主→ employee n.雇员12. romantic adj.浪漫的n.浪漫的人→ romance n.浪漫气氛13. equipment n.设备;装备→ equip vt.装备;配备14. talent n.天才;天资;天赋→ talented adj.有天赋的;天才的15. piano n.钢琴→ pianist n.钢琴家16. assume vt.以为;假设→ assumption n.假设;推断→ assuming conj.假设;假定17. addition n.添加;加法;增加物→additional adj.附加的;另外的→add vt.添加;增加18. treatment n.治疗;对待;处理→ treat vt.治疗;对待;款待n.乐趣;款待19. satisfaction n.满意;满意;欣慰→satisfy vt.使满意→satisfied adj.满意的→ satisfying adj.令人满意的→ satisfactory adj.令人满意的20. various adj.各种不同的;各种各样的→variety n.多样性→vary v.变更21. repetition n.重复;重做→ repeat vt.重复22. reaction n.反应;回应→ react vi.(对……)作出反应运用巩固提能1.(2024·全国Ⅱ卷)Giving employees the tools enables (使能够) them to communicate honestly.2.(剑桥高阶)There's evidence to suggest that child abuse is not just a recent phenomenon (现象).3.(朗文当代)Moodie has been awarded (授予) a golf scholarship at the University of Hawaii.4.(2024·全国Ⅱ卷)He pushed a chair onto (朝向) the balcony, and climbed up to see them.5.(2024·全国甲卷)It presents live entertainment, including pop, rock, folk, jazz, musicals, dance, world music, films and classical (古典的) music.6.(2024·全国甲卷)It is hard to name a comedy star who hasn't been on the stage (舞台) here.7.(2024·全国甲卷)However, after I went to high school, somehow (不知怎么地) I became distant from him.8.(2024·全国Ⅱ卷)What does Levine want to explain by mentioning the rubber band (带子)?9. Nowadays (现在) more and more people have come to realize the importance of a balanced diet to their health.10.I do hope that ordinary (一般的) people can live a rich and happy life.11.Helen became a capable (有实力的) student and finally she received a university degree in English literature.12.(牛津高阶)Muscular aches (ache) and pains can be soothed by a relaxing massage.13.(2024·江苏卷)Others also looked at the phone boxes and saw business opportunities (opportunity).14.Boys of five to eight years old are very energetic and they seem to have limitless energy .(energy)15.The performer performed so well that we all applauded his performance .(perform)16.The disabled person has a lot of trouble with his life due to hisdisability .However, his new facility enables him to walk freely.(able) 17. Originally the book described the origin of hip-hop;however, he canceled it from the original .(origin)18.Some people thought his disease was incurable ,while others thought it curable .At last, an old specialist found a cure for it and cured him of the disease.(cure)19.Mr Johnson is unemployed .How he wishes an employer could employ him!(employ)20.The patient is under medical treatment now and is being treated with a new drug.(treat)21.To my satisfaction ,I got a satisfying/satisfactory result of the exam, which made my mother quite satisfied .(satisfy)22.Lang Lang has a talent for music and he is a talented pianist.(talent) 23.Our classroom is equipped with the most advanced equipment .(equip)。
一篇关于音乐的英语作文必修二第五单元
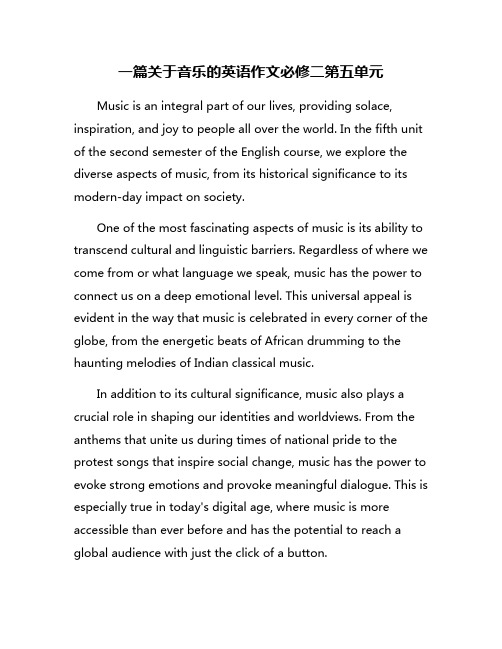
一篇关于音乐的英语作文必修二第五单元Music is an integral part of our lives, providing solace, inspiration, and joy to people all over the world. In the fifth unit of the second semester of the English course, we explore the diverse aspects of music, from its historical significance to its modern-day impact on society.One of the most fascinating aspects of music is its ability to transcend cultural and linguistic barriers. Regardless of where we come from or what language we speak, music has the power to connect us on a deep emotional level. This universal appeal is evident in the way that music is celebrated in every corner of the globe, from the energetic beats of African drumming to the haunting melodies of Indian classical music.In addition to its cultural significance, music also plays a crucial role in shaping our identities and worldviews. From the anthems that unite us during times of national pride to the protest songs that inspire social change, music has the power to evoke strong emotions and provoke meaningful dialogue. This is especially true in today's digital age, where music is more accessible than ever before and has the potential to reach a global audience with just the click of a button.Furthermore, music has the ability to heal and uplift our spirits in times of need. Whether we're feeling stressed, sad, or overwhelmed, music has a way of soothing our souls and bringing us back to a place of calm and peace. This therapeutic effect is well-documented in scientific studies, which show that music has the power to reduce anxiety, lower blood pressure, and even improve cognitive function.In conclusion, music is a powerful force that has the ability to unite, inspire, and heal us in ways that words alone cannot. By exploring the rich history and diverse influences of music in the fifth unit of our English course, we gain a deeper appreciation for the role that music plays in shaping our world and our identities. Whether we're listening to a classical symphony or rocking out to a pop anthem, music has the power to move us in ways that are truly universal.。
- 1、下载文档前请自行甄别文档内容的完整性,平台不提供额外的编辑、内容补充、找答案等附加服务。
- 2、"仅部分预览"的文档,不可在线预览部分如存在完整性等问题,可反馈申请退款(可完整预览的文档不适用该条件!)。
- 3、如文档侵犯您的权益,请联系客服反馈,我们会尽快为您处理(人工客服工作时间:9:00-18:30)。
MUSIC INFORMATION RETRIEVAL BIBLIOGRAPHY
Information Seeking Behaviour and FRBR
Andrew Hankinson
MUMT611: Music Information Retrieval
McGill University
Dr. Ichiro Fujinaga
Bates, Marcia J. 1989. e design of browsing and berrypicking techniques for the online search interface. Online Review 13 (5): 407-24.
–––. 2002. Toward an integrated model of information seeking and searching. In Proceedings of the e Fourth International Conference on Information Needs, Seeking and Use in
Different Contexts, Lisbon, Portugal.
Blandford, Ann, and Hanna Stelmaszewska. 2002. Usability of musical digital libraries: A multimodal analysis. In Proceedings of the ird International Conference on Music
Information Retrieval, Paris, France.
Buchanan, George. 2006. FRBR: Enriching and integrating digital libraries. In Proceedings of the 6th ACM/IEEE-CS joint conference on Digital libraries, Chapel Hill, NC, USA.
Connell, Iain W., Ann E. Blandford, and omas R.G. Green. 2002. Usability of a music digital library: An OSM case study. In Proceedings of the ird International Conference on Music Information Retrieval, Paris, France.
Downie, J. Stephen. 2004. A sample of music information retrieval approaches. Journal of the American Society for Information Science and T echnology 55 (12): 1033-36.
Futrelle, Joe, and J. Stephen Downie. 2002. Interdisciplinary communities and research issues in music information retrieval. In Proceedings of the ird International Conference on Music Information Retrieval, Paris, France.
Hemmasi, Harriette. 2002. Why not MARC? In Proceedings of the ird International Conference on Music Information Retrieval, Paris, France.
IFLA Study Group on the functional requirements for bibliographic records. 1998.
Functional Requirements for Bibliographic Records: Final Report. Vol. 19, UBCIM
Publications - New Series. Munich, Germany: K.G. Saur.
IFLA UBCIM Working Group on Functional Requirements and Numbering of Authority Records. 2005. Functional Requirements for Authority Records: A Conceptual Model -
Draft. http://www.ifl/VII/d4/FRANAR-Conceptual-M-Draft-e.pdf Accessed 4 April, 2007.
Kuhlthau, Carol C. 1991. Inside the search process: Information seeking from the user's perspective. Journal of the American Society for Information Science 42 (5): 361-71. Lee, Jin Ha, and J. Stephen Downie. 2004. Survey of music information needs, uses and seeking behaviours: Preliminary findings. In Proceedings of the Fifth International
Conference on Music Information Retrieval, Barcelona, Spain.
Minibayeva, Natalia, and Jon W. Dunn. 2002. A digital library data model for music. In Proceedings of the Second ACM/IEEE-CS Joint Conference on Digital Libraries, Portland, Oregon.
Morville, Peter. 2005. Ambient Findability. Sebastopol, CA: O'Reilly.
Notess, Mark. 2004. ree looks at users: A comparison of methods for studying digital library use. Information Research 9 (3). /ir/9-3/paper177.html Accessed 3 April, 2007.
–––, and Margaret B. Swan. 2003. Predicting user satisfaction from subject satisfaction. In Proceedings of the Conference on Human factors in Computing Systems, Ft. Lauderdale. Smiraglia, Richard P. 2001. Musical works as information retrieval entities: Epistemological perspectives. In Proceedings of the Second Annual International Symposium on Music
Information Retrieval, Bloomington, Indiana.
Taheri-Panah, S., and A. MacFarlane. 2004. Music information retrieval systems: Why do individuals use them and what are their needs? In Proceedings of the Fifth International Conference on Music Information Retrieval, Barcelona, Spain.
Vellucci, Sherry L. 1997. Bibliographic relationships in music catalogs. Lanham, Md. ; London: Scarecrow Press.
–––. 1999. Metadata for music. Fontes Artis Musicae 46 (3-4): 205-17.。