Multi-dimensionalmodellingofspray,in-cylinderair motionandfuel–airmixinginadirect-injectionengine
多尺度特征融合的脊柱X线图像分割方法
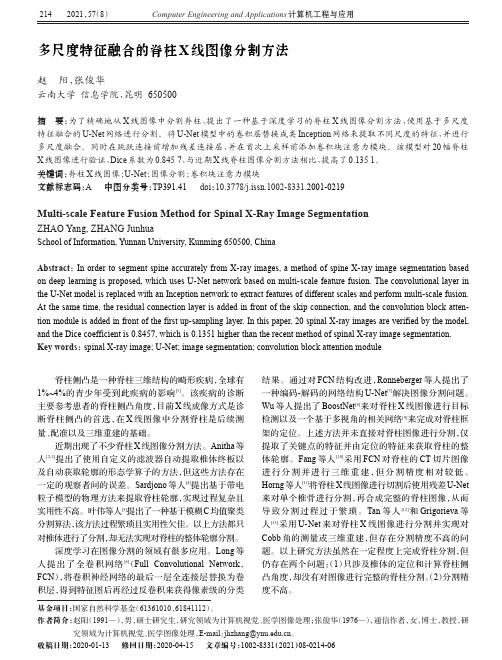
脊柱侧凸是一种脊柱三维结构的畸形疾病,全球有1%~4%的青少年受到此疾病的影响[1]。
该疾病的诊断主要参考患者的脊柱侧凸角度,目前X线成像方式是诊断脊柱侧凸的首选,在X线图像中分割脊柱是后续测量、配准以及三维重建的基础。
近期出现了不少脊柱X线图像分割方法。
Anitha等人[2-3]提出了使用自定义的滤波器自动提取椎体终板以及自动获取轮廓的形态学算子的方法,但这些方法存在一定的观察者间的误差。
Sardjono等人[4]提出基于带电粒子模型的物理方法来提取脊柱轮廓,实现过程复杂且实用性不高。
叶伟等人[5]提出了一种基于模糊C均值聚类分割算法,该方法过程繁琐且实用性欠佳。
以上方法都只对椎体进行了分割,却无法实现对脊柱的整体轮廓分割。
深度学习在图像分割的领域有很多应用。
Long等人提出了全卷积网络[6](Full Convolutional Network,FCN),将卷积神经网络的最后一层全连接层替换为卷积层,得到特征图后再经过反卷积来获得像素级的分类结果。
通过对FCN结构改进,Ronneberger等人提出了一种编码-解码的网络结构U-Net[7]解决图像分割问题。
Wu等人提出了BoostNet[8]来对脊柱X线图像进行目标检测以及一个基于多视角的相关网络[9]来完成对脊柱框架的定位。
上述方法并未直接对脊柱图像进行分割,仅提取了关键点的特征并由定位的特征来获取脊柱的整体轮廓。
Fang等人[10]采用FCN对脊柱的CT切片图像进行分割并进行三维重建,但分割精度相对较低。
Horng等人[11]将脊柱X线图像进行切割后使用残差U-Net 来对单个椎骨进行分割,再合成完整的脊柱图像,从而导致分割过程过于繁琐。
Tan等人[12]和Grigorieva等人[13]采用U-Net来对脊柱X线图像进行分割并实现对Cobb角的测量或三维重建,但存在分割精度不高的问题。
以上研究方法虽然在一定程度上完成脊柱分割,但仍存在两个问题:(1)只涉及椎体的定位和计算脊柱侧凸角度,却没有对图像进行完整的脊柱分割。
融合多尺度通道注意力的开放词汇语义分割模型SAN
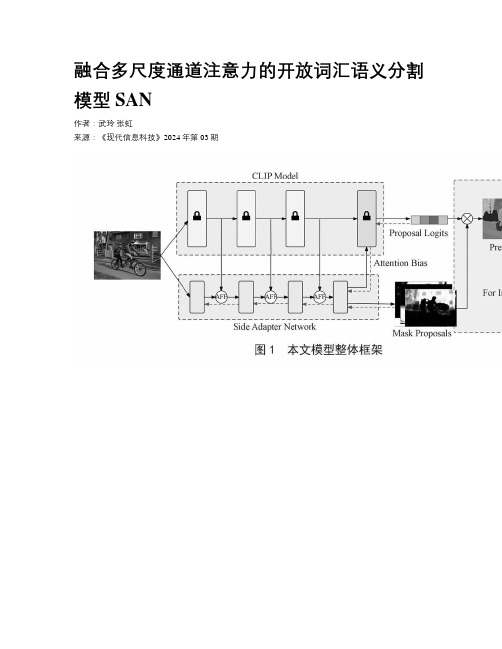
融合多尺度通道注意力的开放词汇语义分割模型SAN作者:武玲张虹来源:《现代信息科技》2024年第03期收稿日期:2023-11-29基金项目:太原师范学院研究生教育教学改革研究课题(SYYJSJG-2154)DOI:10.19850/ki.2096-4706.2024.03.035摘要:随着视觉语言模型的发展,开放词汇方法在识别带注释的标签空间之外的类别方面具有广泛应用。
相比于弱监督和零样本方法,开放词汇方法被证明更加通用和有效。
文章研究的目标是改进面向开放词汇分割的轻量化模型SAN,即引入基于多尺度通道注意力的特征融合机制AFF来改进该模型,并改进原始SAN结构中的双分支特征融合方法。
然后在多个语义分割基准上评估了该改进算法,结果显示在几乎不改变参数量的情况下,模型表现有所提升。
这一改进方案有助于简化未来开放词汇语义分割的研究。
关键词:开放词汇;语义分割;SAN;CLIP;多尺度通道注意力中图分类号:TP391.4;TP18 文献标识码:A 文章编号:2096-4706(2024)03-0164-06An Open Vocabulary Semantic Segmentation Model SAN Integrating Multi Scale Channel AttentionWU Ling, ZHANG Hong(Taiyuan Normal University, Jinzhong 030619, China)Abstract: With the development of visual language models, open vocabulary methods have been widely used in identifying categories outside the annotated label. Compared with the weakly supervised and zero sample method, the open vocabulary method is proved to be more versatile and effective. The goal of this study is to improve the lightweight model SAN for open vocabularysegmentation, which introduces a feature fusion mechanism AFF based on multi scale channel attention to improve the model, and improve the dual branch feature fusion method in the original SAN structure. Then, the improved algorithm is evaluated based on multiple semantic segmentation benchmarks, and the results show that the model performance has certain improvement with almost no change in the number of parameters. This improvement plan will help simplify future research on open vocabulary semantic segmentation.Keywords: open vocabulary; semantic segmentation; SAN; CLIP; multi scale channel attention 0 引言識别和分割任何类别的视觉元素是图像语义分割的追求。
语义分析的一些方法

语义分析的一些方法语义分析的一些方法(上篇)•5040语义分析,本文指运用各种机器学习方法,挖掘与学习文本、图片等的深层次概念。
wikipedia上的解释:In machine learning, semantic analysis of a corpus is the task of building structures that approximate concepts from a large set of documents(or images)。
工作这几年,陆陆续续实践过一些项目,有搜索广告,社交广告,微博广告,品牌广告,内容广告等。
要使我们广告平台效益最大化,首先需要理解用户,Context(将展示广告的上下文)和广告,才能将最合适的广告展示给用户。
而这其中,就离不开对用户,对上下文,对广告的语义分析,由此催生了一些子项目,例如文本语义分析,图片语义理解,语义索引,短串语义关联,用户广告语义匹配等。
接下来我将写一写我所认识的语义分析的一些方法,虽说我们在做的时候,效果导向居多,方法理论理解也许并不深入,不过权当个人知识点总结,有任何不当之处请指正,谢谢。
本文主要由以下四部分组成:文本基本处理,文本语义分析,图片语义分析,语义分析小结。
先讲述文本处理的基本方法,这构成了语义分析的基础。
接着分文本和图片两节讲述各自语义分析的一些方法,值得注意的是,虽说分为两节,但文本和图片在语义分析方法上有很多共通与关联。
最后我们简单介绍下语义分析在广点通“用户广告匹配”上的应用,并展望一下未来的语义分析方法。
1 文本基本处理在讲文本语义分析之前,我们先说下文本基本处理,因为它构成了语义分析的基础。
而文本处理有很多方面,考虑到本文主题,这里只介绍中文分词以及Term Weighting。
1.1 中文分词拿到一段文本后,通常情况下,首先要做分词。
分词的方法一般有如下几种:•基于字符串匹配的分词方法。
此方法按照不同的扫描方式,逐个查找词库进行分词。
机械加工简明对照表

(1)机械加工工艺Machining Technologe刨planing;shaping表面加工facing槽焊slot welding超级精密加工superfinish超声波机械加工ultrasonic machining车削turning齿纹接合serrated joint冲孔punching吹管焊接torch welding粗加工rough working粗磨coarse grinding锉光file finishing锉削file cutting搭焊lap welding搭铆接lap riveting点焊spot welding电镀electroplating电焊electric welding电弧焊arc welding电弧切削arc cutting电解切割electrolytic cutting电抛光electropolishing镀铬chroming镀金gold plating镀锌zincification镀银silver plating;silvering 对接铆butt riveting多刀切削multicutting缝焊seam welding高速切割high-speed cutting 攻丝tapping刮削scraping滚花knurling焊接welding回火tempering火焰淬火flame hardening火焰切割flame cutting机械精加工finish machining 机械切割machine cut激光切割laser cutting加工方法working process加工技术machining technique 剪shearing交叉铆接cross riveting铰接articulated (twist) joint 金属切割metal cutting精密加工super-finish镜面磨削mirror face grinding 锯sawing拉削broaching冷焊cold welding立铣end milling链型铆接chain rivetinf螺纹铣削thread milling螺旋缝焊spiral welding螺旋切割screw cutting铆钉搭接rivet lap铆接riveting密封sealing密封焊接seal welding磨光polishing磨削grinding抛光burnishment;polishing 喷漆spray-painting平焊downward welding平接butting平铣face milling气割gas cutting气焊gas ( / autogenous ) welding 气力铆pneumatic rivetinf铅封lead-seal切削cutting氢焊hydrogen welding人工铆接hand riveting双排铆接double riveting酸刻法etching镗孔boring套接sleeve joint铜焊braze welding;brazing退火annealing微机械加工micromachining无切削工艺chipless metal working 无屑加工chipless machining铣milling细切割smooth cut旋削rotary cut旋转磨削rotary grinding仰焊upward welding氧乙炔焊oxygen- acetylene welding乙炔焊acetylene welding真空焊接vacuum welding重切割heavy welding铸焊forge welding自动焊接automatic welding最精加工superfinishing(2)机械制造工艺Machine Manufacturing Technology半机械化semi-machanization;semimechanized 半自动化semi-automation;semiautomatic部件吻合fit部件装配major assembly差动总成differential assembly大气腐蚀atmospheric corrosion仿形制造profile modelling机器制造machine building机器装配machine assembling机械化mechanization;mechanized检修overhaul连续生产线serial production line流水线assembly line切削寿命working durability;service life试车test working:trial run遥测telemetering遥控remote control;telemechanics制造工艺manufacturing engineering装配线assembly ilne自动化automation;automatic自动生产线automatic production line总装配final assembly总装配图assembly drawing总装配线general assembly line组件装配minor assembly组装件assembly parts作业线production line(3)公差、变形和腐蚀Tolerance, Deformation and Corrosion变形deformation测定误差error at ( / of ) measurement低温腐蚀low temperature corrosion电化腐蚀electrochemical corrosion负偏离minus deviation高温腐蚀high temperature corrosion公差(容许误差)allowable error;tolerance 厚度(深度)depth加工留量allowance绝对变形absolute deformation绝对误差absolute error刻度误差error of graduation裂缝crack疲劳fatigue偏差deviation平均误差average error切削深度cutting depth上偏差upper deviation误差error下偏差lower daviation相对变形relative deformation相对误差relative error永久变形residual deformation。
生物膜数学模型研究进展
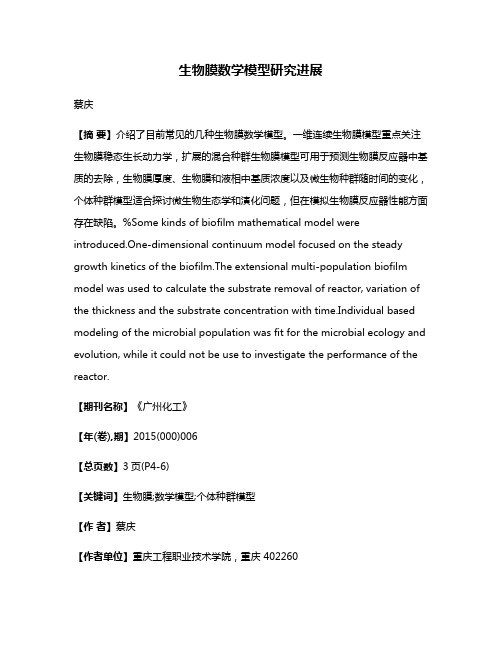
生物膜数学模型研究进展蔡庆【摘要】介绍了目前常见的几种生物膜数学模型。
一维连续生物膜模型重点关注生物膜稳态生长动力学,扩展的混合种群生物膜模型可用于预测生物膜反应器中基质的去除,生物膜厚度、生物膜和液相中基质浓度以及微生物种群随时间的变化,个体种群模型适合探讨微生物生态学和演化问题,但在模拟生物膜反应器性能方面存在缺陷。
%Some kinds of biofilm mathematical model were introduced.One-dimensional continuum model focused on the steady growth kinetics of the biofilm.The extensional multi-population biofilm model was used to calculate the substrate removal of reactor, variation of the thickness and the substrate concentration with time.Individual based modeling of the microbial population was fit for the microbial ecology and evolution, while it could not be use to investigate the performance of the reactor.【期刊名称】《广州化工》【年(卷),期】2015(000)006【总页数】3页(P4-6)【关键词】生物膜;数学模型;个体种群模型【作者】蔡庆【作者单位】重庆工程职业技术学院,重庆 402260【正文语种】中文【中图分类】X703生物膜由多种细菌构成,同时也包含真菌、藻类、酵母菌、原生动物等微生物、侵蚀产物和水,所有组分通过胞外聚合物(EPS)固定在一起,形成一个复杂的动态变化的有机体。
燃气轮机英文词汇[整理文档]
![燃气轮机英文词汇[整理文档]](https://img.taocdn.com/s3/m/d4aaf2a164ce0508763231126edb6f1aff0071b8.png)
燃气轮机词汇表(A-I)(2007-07-02 20:36:14)转载英文xxAAcceptance test 验收实验Actual enthalpy drop (enthalpy rise)实际焓降(焓增)Actuating oil system 压力油系统Aero-derivative gas turbine, aircraft-derivative gas turbine 航空衍生(派生)型燃气机轮Air charging system 空气冲气系统Air film cooling 气膜冷却Air intake duck 进气道Alarm and protection system 报警保护系统Annulus drag loss 环壁阻力损失Area heat release rate 面积热强度Aspect ratio 展弦比Atomization 雾化Atomized particle size 雾化细度Automatic starting time of gas turbine 燃气机轮的自动起动时间Auxiliary loads 辅助负荷Availability 可用性Average continuous running time of gas turbine 燃气机轮平均连续运行时间Axial displacement limiting device 轴向位移保护装置Axial flow turbine 轴流式透平Axial thrust 轴向推力BBase load rated output 基本负荷额定输出功率Black start 黑起动Blade 叶片Blade 叶片Blade height 叶(片)高(度)Blade inlet angle (叶片)进口角Blade outlet angle (叶片)出口角Blade profile 叶型Blade profile thickness 叶型厚度Blade root xxBleed air/extraction air 抽气Blow-off 放气Blow-off value 放气阀Burner outlet temperature 透平进口温度By-pass control 旁路控制By-pass control 旁路控制CCamber angle 叶型转折角Camber line 中弧线Carbon deposit 积碳Casing 气缸Cascade xxCenter support system 定中系统Centripetal turbine 向心式(透平)Choking 堵塞Choking limit 阻塞极限Chord 弦长Closed-cycle 闭式循环Cogeneration 热电联供Cold starting 冷态起动Combined cycle 联合循环Combined cycle with multi-pressure level Rankine cycle 多压朗肯循环的联合循环Combined cycle with single pressure level Rankine cycle 单压朗肯循环的联合循环Combined supercharged boiler and gas turbine cycle 增压锅炉型联合循环Combustion chamber 燃烧室Combustion intensity 燃烧强度Combustion zone 燃烧区Combustion efficiency 燃烧室效率Combustion inspection 燃烧室检查Combustion outer casing 燃烧室外壳Combustion outlet temperature 透平进口温度Combustion specific pressure loss 燃烧室比压力损失Compactness factor 紧凑系数Compressor 压气机Compressor characteristic curves 压气机特性线Compressor disc 压气机轮盘Compressor input power 压气机输入功率Compressor intake anti-icing system 压气机进气防冰系统Compressor rotor 压气机转子Compressor turbine 压气机透平Compressor washing system 压气机清洗系统Compressor wheel 压气机叶轮Constant power operation 恒功率运行Constant temperature operation 恒温运行Control system 控制系统Convection cooling 对流冷却Cooled blade 冷却叶片Cooling down 冷却盘车Corrected flow 折算流量Corrected output 折算输出功率Corrected speed 折算转速Corrected thermal efficiency 折算热效率Critical speed 临界转速Critical speed of rotor 转子临界速度Cross flame tube/inter-connector/cross fire tube/cross light tube 联烟管confidence interval 置信区间DDead band 迟缓率Dead center 死点Deep stall 渐进失速Degree of reaction 反动度Design condition 设计工况Deviation angle 落后角Diaphragm 隔板Diffuser 扩压器Dilution of rotation 旋转方向Disc-coupled vibration 轮系振动Disc-friction loss 轮盘摩擦损失Dual fuel nozzle 双燃料喷嘴Dual fuel system 双燃料系统Dynamic balancing 动平衡EElectro-hydraulic control system 电液调节系统Enclosures 罩壳End plate 端板Energy effectiveness 能量有效度Equivalence ratio 当量比Evaporative 蒸发冷却器Excess air ratio 过量空气比Exhaust duct 排气道Exhaust gas flow 排气流量External loss 外损失Extraction gas turbine/bled gas turbine 抽气式燃气轮机FFast start 快速起动Final temperature difference 端差Flame detector 火焰检测器Flame failure limit 熄火极限Flame-failure tripping device 火焰失效遮断装置Flame-holder 火焰稳定器Flame-out ripping device 熄火遮断装置Flexible rotor 挠性(柔性)转子Flow coefficient 流量系数Flow inlet angle 进气角Flow outlet angle 出气角Flow passage 通流部分Flow pattern 流型Flow turning angle 气流转折角Free piston turbine 自由活塞燃气轮机Front 额线Fuel coefficient 燃料系数Fuel consumption 燃料消耗量Fuel control system 燃料控制系统Fuel flow control valve 燃料流量控制阀Fuel injection pressure 燃料喷嘴压力Fuel injection pump 燃料注入泵Fuel injector 燃料喷嘴Fuel shut-off valve 燃料流量控制阀Fuel supply system 燃料供给系统Fuel treatment equipment 燃料处理设备Fuel-air ratio 燃料空气比GGas expander turbine 气体膨胀透平Gas flow bending stress 气流弯应力Gas fuel nozzle 气体燃料喷嘴Gas generator 燃气发生器Gas temperature controller 燃气温度控制器Gas turbine 燃气轮机Gas turbine power plant 燃气轮机动力装置Governing system 调节系统HHeader 联箱Heat balance 热平衡Heat consumption 热耗Heat exchanger plant 换热器板Heat exchanger tube 换热器管Heat loss 热损失Heat rate 热耗率Heat recovery steam generator/HRSG 余热锅炉Heat transfer rate of heating surface 受热表面的传热率Heat utilization 热能利用率Heater 加热器Heating surface area 受热面积High oil temperature protection device (润)滑油温过高保护装置High pressure turbine 高压透平Hollow blade 空心叶片Hot corrosion 热腐蚀Hot section inspection 热通道检查Hot starting 热态起动IIdle time 惰转时间Idling speed 空负荷转速Ignition 点火Ignition equipment 点火装置Ignition speed 点火转速Impingement cooling 冲击冷却Impulse turbine 冲动式透平Incidence 冲角Inlet air flow 进口空气流量Inlet casing(plenum)进气缸(室)Inlet condition 进气参数Inlet guide vanes 进口导叶Inlet pressure 进口压力Inlet temperature 进口温度Inner casing 内气缸Intake air filter 进口过滤器Integral(tip)shroud 叶冠Intercooled cycle 中间冷却循环(间冷循环)Intercooler 中间冷却器Intermediate pressure turbine 内燃式燃气机轮Internal efficiency 内效率Internal loss 内损失Isentropic efficiency 等熵效率Isentropic efficiency 等熵效率Isentropic power 等熵功率LLagging 保温层Leaving velocity loss 余速损失Level pressure control 基准压力调节Light-off 着火Limit power 极限功率Load dump test 甩负荷试验Load rejection test 甩负荷试验Loading time 加载时间Locking piece 锁口件Logarithmic-mean temperature difference 对数平均温差Long shank blade root 长颈叶根Low fuel pressure protection device 燃料压力过低保护装置Low oil pressure protection device (润)滑油压力过低保护装置Low pressure turbine 低压透平Lubrication system 润滑油系统MMain gear 负荷齿轮箱(主齿轮箱)Major inspection 关键(部件)检查Major overhaul xxManual tripping device 手动遮断装置Mass to power ratio(mobile applications)质量功率比(用于移动式燃气机轮)Matrix 蓄热体Maximum continuous power 最大连续功率Maximum momentary speed 飞升转速Mechanical efficiency 机械效率Mechanical efficiency 机械效率Mechanical loss 机械损失Method of modelling stage 模型级法Method of plane cascade 平面叶栅法Mobile gas turbine 移动式燃气机轮Moving blade/rotor blade 动叶片Multi-shaft gas turbine 多轴燃气机轮Multi-spool gas turbine 多转子燃气机轮NNew and clean condition 新的和清洁的状态No-load operation 空负荷运行Normal start 正常起动Number of starts 起动次数OOff-design condition 变工况Open-cycle 开式循环Operating point 运行点Outer casing 外壳Outer casing 外壳Outlet condition 出气参数Outlet guide vanes 出口导叶Outlet pressure 出口压力Outlet pressure 出口压力Outlet pressure 出口压力Outlet temperature 出口温度Outlet temperature 出口温度Outlet temperature/burner outlet temperature 燃烧室出口温度Output limit 极限输出功率Output performance diagram 输出功率性能图Overspeed control device 超速控制装置Overspeed trip device 超速遮断装置Overtemperature control device 超温控制装置Overtemperature detector 超温检测器Overtemperature protective device 超温保护装置PPackaged gas turbine 箱式燃气轮机Particle separator 颗粒分离器Peak load rated output 尖峰负荷额定输出功率Performance map/characteristic map 特性图Pitch 节距Plate type recuperator 板式回热器Polytropic efficiency 多变效率Polytropic efficiency 多变效率Power recovery turbine 能量回收透平Power turbine 动力透平Power-heat ratio 功热比Precooler 预冷器Pressure level control 压力控制Pressure ratio 膨胀比(压比)Pressure ratio 膨胀比(压比)Primary air 一次空气Primary zone 一次燃烧区Profile loss 型面损失Protection system 保护系统Protective device test 保护设备试验Purging 清吹RRadial flow turbine 径流式透平Rate of load-up 负荷上升率Rated condition 额定工况Rated output 额定输出功率Rated speed 额定转速Reaction turbine 反动失透平Recirculating zone 回流区Recuperator 表面式回热器Referred output 折算输出功率Referred speed 折算转速Referred thermal efficiency 折算热效率Regenerative cycle 回热循环Regenerator 回热器Regenerator 回热器Regenerator effectiveness 回热度Reheat cycle 再热循环Reheat factor 重热系数Relative dead center 相对死点Reliability 可靠性Reserve peak load output 备用尖峰负荷额定输出功率(应急尖峰符合额定输出功率)rigid rotor 刚性转子Rotating regenerator 回转式回热器(再生式回热器)Rotating stall 旋转失速Rotor blade loss 动叶损失Rotor blade/rotor bucket 动叶片Rotor without blades 转子体SSealing 气封Secondary air 二次空气Secondary flow loss 二次流损失Secondary zone 二次燃烧区Self-sustaing speed 自持转速Semi-base-load rated output 半基本负荷额定输出功率(中间负荷额定输出功率)Semiclosed-cycle 半闭式循环Shaft output 轴输出功率Shafting vibration 轴系振动Shell 壳体Shell and tube recuperator 壳管式回热器Silencer 消音器Simple cycle 简单循环Single-shaft gas turbine 单轴燃气机轮Site conditions 现场条件Site rated output 现场额定输出功率Soot blower 吹灰器Spacer 隔叶块Specific fuel consumption 燃料消耗率Specific power 比功率Speed changer 转速变换器Specific changer/synchronizer 转速变换器Speed governor 转速调节器Speed governor droop 转速不等率Spray cone angle 雾化锥角Stabilization time 稳定性时间Stage 级Stage efficiency 级效率Stagger angle 安装角Stall 失速Standard atmosphere 标准大气Standard rated output 标准额定输出功率Standard reference conditions 标准参考条件start 起动Starter cut-off 起动机脱扣Starting characteristics diagram 起动特性图Starting characteristics test 起动特性试验Starting equipment 起动设备Starting time 起动时间Static balancing 静平衡Stationary blade 静叶片Stationary blade loss 静叶损失Stationary gas turbine 固定式燃气机轮Stator 静子Steady-state incremental speed regulation 稳态转速增量调节Steady-state speed 静态转速Steady-state speed droop 静态转速不等率Steady-state speed regulation 静态转速调节Steam and/or water injection 蒸汽和/或水的喷注Steam injection gas turbine 注蒸汽燃气机轮Steam/water injection equipment 注蒸汽/注水设备Steam-air ratio 蒸汽空气比Steam-gas power ratio 蒸燃功比Stoichiometric fuel-air ratio 理论(化学计量)燃烧空气比Straight blade 直叶片Surge 旋转失速Surge limit 喘振边界Surge margin 喘振裕度Surge-preventing device 防喘装置Swirler 旋流器TTemperature effectiveness 温度有效率Temperature pattern factor 温度场系数Temperature ratio 温比Thermal blockage 热(悬)挂Thermal efficiency 热效率Thermal fatigue 热疲劳Thermal shock 热冲击Thermodynamic performance test 热力性能试验Throat area (叶栅)喉部面积Tip-hub ratio 轮毂比Total pressure loss coefficient 全压损失系数Total pressure loss for air side 空气侧压全损失Total pressure loss for gas side 燃气侧压全损失Total pressure recover factor 全压恢复系数Transpiration cooling 发散冷却Tube bundle/tube nest 管束Tube plate 管板Turbine 透平Turbine characteristic curves 透平特性线Turbine diaphragm 透平隔板Turbine disc 透平轮盘Turbine entry temperature 透平进口温度Turbine nozzle 透平喷嘴Turbine power output 透平输出功率Turbine reference inlet temperature 透平参考进口温度Turbine rotor 透平转子Turbine rotor inlet temperature 透平转子进口温度Turbine trip speed 燃气轮机跳闸转速Turbine trip speed 燃气轮机跳闸转速Turbine washing equipment 透平清洗设备Turbine wheel 透平叶轮Turbine gear 盘车装置Turning/barring 盘车Twisted blade 扭叶片UVVane xxVane xxVariable stator blade 可调静叶片Variable stator blade 可调静叶片Variable-geometry gas turbine 变几何燃气机轮Velocity coefficient 速度系数Velocity ratio 速比Velocity triangle 速度三角形Volumetric heat release rate 容积热强度WWheel efficiency 轮周效率Working fluid heater 工质加热器Working fluid heater efficiency 工质加热器效率21/ 21。
3D-Printing-Technology
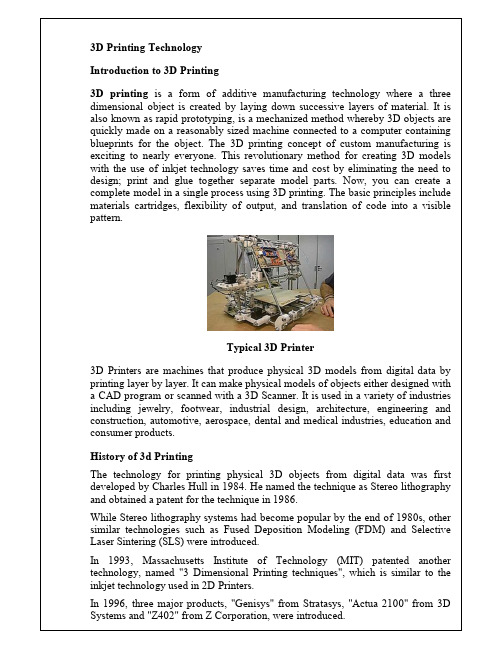
3D Printing Technology Introduction to 3D Printing 3D printing is a form of additive manufacturing technology where a three dimensional object is created by laying down successive layers of material. It is also known as rapid prototyping, is a mechanized method whereby 3D objects are quickly made on a reasonably sized machine connected to a computer containing blueprints for the object. The 3D printing concept of custom manufacturing is exciting to nearly everyone. This revolutionary method for creating 3D models with the use of inkjet technology saves time and cost by eliminating the need to design; print and glue together separate model parts. Now, you can create a complete model in a single process using 3D printing. The basic principles include materials cartridges, flexibility of output, and translation of code into a visible pattern.Typical 3D Printer 3D Printers are machines that produce physical 3D models from digital data by printing layer by layer. It can make physical models of objects either designed with a CAD program or scanned with a 3D Scanner. It is used in a variety of industries including jewelry, footwear, industrial design, architecture, engineering and construction, automotive, aerospace, dental and medical industries, education and consumer products. History of 3d Printing The technology for printing physical 3D objects from digital data was first developed by Charles Hull in 1984. He named the technique as Stereo lithography and obtained a patent for the technique in 1986. While Stereo lithography systems had become popular by the end of 1980s, other similar technologies such as Fused Deposition Modeling (FDM) and Selective Laser Sintering (SLS) were introduced. In 1993, Massachusetts Institute of Technology (MIT) patented another technology, named "3 Dimensional Printing techniques", which is similar to the inkjet technology used in 2D Printers. In 1996, three major products, "Genisys" from Stratasys, "Actua 2100" from 3D Systems and "Z402" from Z Corporation, were introduced.In 2005, Z Corp. launched a breakthrough product, named Spectrum Z510, which was the first high definition color 3D Printer in the market. Another breakthrough in 3D Printing occurred in 2006 with the initiation of an open source project, named Reprap, which was aimed at developing a selfreplicating 3D printer. MANUFACTURING A MODEL WITH THE 3D PRINTERThe model to be manufactured is built up a layer at a time. A layer of powder is automatically deposited in the model tray. The print head then applies resin in the shape of the model. The layer dries solid almost immediately. The model tray then moves down the distance of a layer and another layer of power is deposited in position, in the model tray. The print head again applies resin in the shape of the model, binding it to the first layer. This sequence occurs one layer at a time until the model is completeVery recently Engineers at the University of Southampton in the UK have designed, printed, and sent skyward the world’s first aircraft manufactured almost entirely via 3-D printing technology. The UAV dubbed SULSA is powered by an electric motor that is pretty much the only part of the aircraft not created via additive manufacturing methods.World’s First 3D Printed Plane Takes Flight Created on an EOS EOSINT P730 nylon laser sintering machine, its wings, hatches and control surfaces basically everything that makes up its structure and aerodynamic controls was custom printed to snap together. It requires no fasteners and no tools to assemble. Current 3D Printing Technologies Stereo lithography - Stereo lithographic 3D printers (known as SLAs or stereo lithography apparatus) position a perforated platform just below the surface of a vat of liquid photo curable polymer. A UV laser beam then traces the first slice of an object on the surface of this liquid, causing a very thin layer of photopolymer to harden. The perforated platform is then lowered very slightly and another slice is traced out and hardened by the laser. Another slice is then created, and then another, until a complete object has been printed and can be removed from the vat of photopolymer, drained of excess liquid, and cured. Fused deposition modelling - Here a hot thermoplastic is extruded from a temperature-controlled print head to produce fairly robust objects to a high degree of accuracy. Selective laser sintering (SLS) - This builds objects by using a laser to selectively fuse together successive layers of a cocktail of powdered wax, ceramic, metal, nylon or one of a range of other materials. Multi-jet modelling (MJM)- This again builds up objects from successive layers of powder, with an inkjet-like print head used to spray on a binder solution that glues only the required granules together.The VFlash printer, manufactured by Canon, is low-cost 3D printer. It’s known to build layers with a light-curable film. Unlike other printers, the VFlash builds its parts from the top down. Desktop Factory is a startup launched by the Idealab incubator in Pasadena, California. Fab@home, an experimental project based at Cornell University, uses a syringe to deposit material in a manner similar to FDM. The inexpensive syringe makes it easy to experiment with different materials from glues to cake frosting. The Nanofactory 3D printing technologies are introduced that are related to the nanotechnologies. . 3D Printing Capabilities: As anticipated, this modern technology has smoothed the path for numerous new possibilities in various fields. The list below details the advantages of 3D printing in certain fields. 1. Product formation is currently the main use of 3D printing technology. These machines allow designers and engineers to test out ideas for dimensional products cheaply before committing to expensive tooling and manufacturing processes. 2. In Medical Field, Surgeons are using 3d printing machines to print body parts for reference before complex surgeries. Other machines are used to construct bone grafts for patients who have suffered traumatic injuries. Looking further in the future, research is underway as scientists are working on creating replacement organs. 3. Architects need to create mockups of their designs. 3D printing allows them to come up with these mockups in a short period of time and with a higher degree of accuracy. 4. 3D printing allows artists to create objects that would be incredibly difficult, costly, or time intensive using traditional processes. 3D Saves Time and Cost Creating complete models in a single process using 3D printing has great benefits. This innovative technology has been proven to save companies time, manpower and money. Companies providing 3D printing solutions have brought to life an efficient and competent technological product.Compiled by-Gaurav Tyagi, Technical Director/DIO, NIC-Muzaffarnagar, UP Sources/3dprinting.html /wiki/3D_printing /3d-printers/ /ir/library/pdf/DEC0702.pdf /intro-to-3-d-printing.html /773374/3d-printing-technologies。
A_review_of_feature_selection_techniques_in_bioinformatics

A review of feature selection techniques in bioinformaticsAbstractFeature selection techniques have become an apparent need in many bioinformatics applications. In addition to the large pool of techniques that have already been developed in the machine learning and data mining fields, specific applications in bioinformatics have led to a wealth of newly proposed techniques.In this article, we make the interested reader aware of the possibilities of feature selection, providing a basic taxonomy of feature selection techniques, and discussing their use, variety and potential in a number of both common as well as upcoming bioinformatics applications.1 INTRODUCTIONDuring the last decade, the motivation for applying feature selection (FS) techniques in bioinformatics has shifted from being an illustrative example to becoming a real prerequisite for model building. In particular, the high dimensional nature of many modelling tasks in bioinformatics, going from sequence analysis over microarray analysis to spectral analyses and literature mining has given rise to a wealth of feature selection techniques being presented in the field.In this review, we focus on the application of feature selection techniques. In contrast to other dimensionality reduction techniques like those based on projection (e.g. principal component analysis) or compression (e.g. using information theory), feature selection techniques do not alter the original representation of the variables, but merely select a subset of them. Thus, they preserve the original semantics of the variables, hence, offering the advantage of interpretability by a domain expert.While feature selection can be applied to both supervised and unsupervised learning, we focus here on the problem of supervised learning (classification), where the class labels are known beforehand. The interesting topic of feature selection for unsupervised learning (clustering) is a more complex issue, and research into this field is recently getting more attention in several communities (Liu and Yu, 2005; Varshavsky et al., 2006).The main aim of this review is to make practitioners aware of the benefits, and in some cases even the necessity of applying feature selection techniques. Therefore, we provide an overview of the different feature selection techniques for classification: we illustrate them by reviewing the most important application fields in the bioinformatics domain, highlighting the efforts done by the bioinformatics community in developing novel and adapted procedures. Finally, we also point the interested reader to some useful data mining and bioinformatics software packages that can be used for feature selection.Previous SectionNext Section2 FEATURE SELECTION TECHNIQUESAs many pattern recognition techniques were originally not designed to cope with large amounts of irrelevant features, combining them with FS techniques has become a necessity in many applications (Guyon and Elisseeff, 2003; Liu and Motoda, 1998; Liu and Yu, 2005). The objectives of feature selection are manifold, the most important ones being: (a) to avoid overfitting andimprove model performance, i.e. prediction performance in the case of supervised classification and better cluster detection in the case of clustering, (b) to provide faster and more cost-effective models and (c) to gain a deeper insight into the underlying processes that generated the data. However, the advantages of feature selection techniques come at a certain price, as the search for a subset of relevant features introduces an additional layer of complexity in the modelling task. Instead of just optimizing the parameters of the model for the full feature subset, we now need to find the optimal model parameters for the optimal feature subset, as there is no guarantee that the optimal parameters for the full feature set are equally optimal for the optimal feature subset (Daelemans et al., 2003). As a result, the search in the model hypothesis space is augmented by another dimension: the one of finding the optimal subset of relevant features. Feature selection techniques differ from each other in the way they incorporate this search in the added space of feature subsets in the model selection.In the context of classification, feature selection techniques can be organized into three categories, depending on how they combine the feature selection search with the construction of the classification model: filter methods, wrapper methods and embedded methods. Table 1 provides a common taxonomy of feature selection methods, showing for each technique the most prominent advantages and disadvantages, as well as some examples of the most influential techniques.Table 1.A taxonomy of feature selection techniques. For each feature selection type, we highlight a set of characteristics which can guide the choice for a technique suited to the goals and resources of practitioners in the fieldFilter techniques assess the relevance of features by looking only at the intrinsic properties of the data. In most cases a feature relevance score is calculated, and low-scoring features are removed. Afterwards, this subset of features is presented as input to the classification algorithm. Advantages of filter techniques are that they easily scale to very high-dimensional datasets, they are computationally simple and fast, and they are independent of the classification algorithm. As a result, feature selection needs to be performed only once, and then different classifiers can be evaluated.A common disadvantage of filter methods is that they ignore the interaction with the classifier (the search in the feature subset space is separated from the search in the hypothesis space), and that most proposed techniques are univariate. This means that each feature is considered separately, thereby ignoring feature dependencies, which may lead to worse classification performance when compared to other types of feature selection techniques. In order to overcome the problem of ignoring feature dependencies, a number of multivariate filter techniques were introduced, aiming at the incorporation of feature dependencies to some degree.Whereas filter techniques treat the problem of finding a good feature subset independently of the model selection step, wrapper methods embed the model hypothesis search within the feature subset search. In this setup, a search procedure in the space of possible feature subsets is defined, and various subsets of features are generated and evaluated. The evaluation of a specific subset of features is obtained by training and testing a specific classification model, rendering this approach tailored to a specific classification algorithm. To search the space of all feature subsets, a search algorithm is then ‘wrapped’ around the classification model. However, as the space of feature subsets grows exponentially with the number of features, heuristic search methods are used to guide the search for an optimal subset. These search methods can be divided in two classes: deterministic and randomized search algorithms. Advantages of wrapper approaches include the interaction between feature subset search and model selection, and the ability to take into account feature dependencies. A common drawback of these techniques is that they have a higher risk of overfitting than filter techniques and are very computationally intensive, especially if building the classifier has a high computational cost.In a third class of feature selection techniques, termed embedded techniques, the search for an optimal subset of features is built into the classifier construction, and can be seen as a search in the combined space of feature subsets and hypotheses. Just like wrapper approaches, embedded approaches are thus specific to a given learning algorithm. Embedded methods have the advantage that they include the interaction with the classification model, while at the same time being far less computationally intensive than wrapper methods.Previous SectionNext Section3 APPLICATIONS IN BIOINFORMATICS3.1 Feature selection for sequence analysisSequence analysis has a long-standing tradition in bioinformatics. In the context of feature selection, two types of problems can be distinguished: content and signal analysis. Content analysis focuses on the broad characteristics of a sequence, such as tendency to code for proteins or fulfillment of a certain biological function. Signal analysis on the other hand focuses on the identification of important motifs in the sequence, such as gene structural elements or regulatory elements.Apart from the basic features that just represent the nucleotide or amino acid at each position in a sequence, many other features, such as higher order combinations of these building blocks (e.g.k-mer patterns) can be derived, their number growing exponentially with the pattern length k. As many of them will be irrelevant or redundant, feature selection techniques are then applied to focus on the subset of relevant variables.3.1.1 Content analysisThe prediction of subsequences that code for proteins (coding potential prediction) has been a focus of interest since the early days of bioinformatics. Because many features can be extracted from a sequence, and most dependencies occur between adjacent positions, many variations of Markov models were developed. To deal with the high amount of possible features, and the often limited amount of samples, (Salzberg et al., 1998) introduced the interpolated Markov model (IMM), which used interpolation between different orders of the Markov model to deal with small sample sizes, and a filter method (χ2) to select only relevant features. In further work, (Delcher et al., 1999) extended the IMM framework to also deal with non-adjacent feature dependencies, resulting in the interpolated context model (ICM), which crosses a Bayesian decision tree with a filter method (χ2) to assess feature relevance. Recently, the avenue of FS techniques for coding potential prediction was further pursued by (Saeys et al., 2007), who combined different measures of coding potential prediction, and then used the Markov blanket multivariate filter approach (MBF) to retain only the relevant ones.A second class of techniques focuses on the prediction of protein function from sequence. The early work of Chuzhanova et al. (1998), who combined a genetic algorithm in combination with the Gamma test to score feature subsets for classification of large subunits of rRNA, inspired researchers to use FS techniques to focus on important subsets of amino acids that relate to the protein's; functional class (Al-Shahib et al., 2005). An interesting technique is described in Zavaljevsky et al. (2002), using selective kernel scaling for support vector machines (SVM) as a way to asses feature weights, and subsequently remove features with low weights.The use of FS techniques in the domain of sequence analysis is also emerging in a number of more recent applications, such as the recognition of promoter regions (Conilione and Wang, 2005), and the prediction of microRNA targets (Kim et al., 2006).3.1.2 Signal analysisMany sequence analysis methodologies involve the recognition of short, more or less conserved signals in the sequence, representing mainly binding sites for various proteins or protein complexes. A common approach to find regulatory motifs, is to relate motifs to gene expressionlevels using a regression approach. Feature selection can then be used to search for the motifs that maximize the fit to the regression model (Keles et al., 2002; Tadesse et al.,2004). In Sinha (2003), a classification approach is chosen to find discriminative motifs. The method is inspired by Ben-Dor et al. (2000) who use the threshold number of misclassification (TNoM, see further in the section on microarray analysis) to score genes for relevance to tissue classification. From the TNoM score, a P-value is calculated that represents the significance of each motif. Motifs are then sorted according to their P-value.Another line of research is performed in the context of the gene prediction setting, where structural elements such as the translation initiation site (TIS) and splice sites are modelled as specific classification problems. The problem of feature selection for structural element recognition was pioneered in Degroeve et al. (2002) for the problem of splice site prediction, combining a sequential backward method together with an embedded SVM evaluation criterion to assess feature relevance. In Saeys et al. (2004), an estimation of distribution algorithm (EDA, a generalization of genetic algorithms) was used to gain more insight in the relevant features for splice site prediction. Similarly, the prediction of TIS is a suitable problem to apply feature selection techniques. In Liu et al. (2004), the authors demonstrate the advantages of using feature selection for this problem, using the feature-class entropy as a filter measure to remove irrelevant features.In future research, FS techniques can be expected to be useful for a number of challenging prediction tasks, such as identifying relevant features related to alternative splice sites and alternative TIS.3.2 Feature selection for microarray analysisDuring the last decade, the advent of microarray datasets stimulated a new line of research in bioinformatics. Microarray data pose a great challenge for computational techniques, because of their large dimensionality (up to several tens of thousands of genes) and their small sample sizes (Somorjai et al., 2003). Furthermore, additional experimental complications like noise and variability render the analysis of microarray data an exciting domain.In order to deal with these particular characteristics of microarray data, the obvious need for dimension reduction techniques was realized (Alon et al., 1999; Ben-Dor et al., 2000; Golub et al., 1999; Ross et al., 2000), and soon their application became a de facto standard in the field. Whereas in 2001, the field of microarray analysis was still claimed to be in its infancy (Efron et al., 2001), a considerable and valuable effort has since been done to contribute new and adapt known FS methodologies (Jafari and Azuaje, 2006). A general overview of the most influential techniques, organized according to the general FS taxonomy of Section 2, is shown in Table 2.Table 2.Key references for each type of feature selection technique in the microarray domain3.2.1 The univariate filter paradigm: simple yet efficientBecause of the high dimensionality of most microarray analyses, fast and efficient FS techniques such as univariate filter methods have attracted most attention. The prevalence of these univariate techniques has dominated the field, and up to now comparative evaluations of different classification and FS techniques over DNA microarray datasets only focused on the univariate case (Dudoit et al., 2002; Lee et al., 2005; Li et al., 2004; Statnikov et al., 2005). This domination of the univariate approach can be explained by a number of reasons:the output provided by univariate feature rankings is intuitive and easy to understand;the gene ranking output could fulfill the objectives and expectations that bio-domain experts have when wanting to subsequently validate the result by laboratory techniques or in order to explore literature searches. The experts could not feel the need for selection techniques that take into account gene interactions;the possible unawareness of subgroups of gene expression domain experts about the existence of data analysis techniques to select genes in a multivariate way;the extra computation time needed by multivariate gene selection techniques.Some of the simplest heuristics for the identification of differentially expressed genes include setting a threshold on the observed fold-change differences in gene expression between the states under study, and the detection of the threshold point in each gene that minimizes the number of training sample misclassification (threshold number of misclassification, TNoM (Ben-Dor etal.,2000)). However, a wide range of new or adapted univariate feature ranking techniques has since then been developed. These techniques can be divided into two classes: parametric and model-free methods (see Table 2).Parametric methods assume a given distribution from which the samples (observations) have been generated. The two sample t-test and ANOVA are among the most widely used techniques in microarray studies, although the usage of their basic form, possibly without justification of their main assumptions, is not advisable (Jafari and Azuaje, 2006). Modifications of the standard t-test to better deal with the small sample size and inherent noise of gene expression datasets include a number of t- or t-test like statistics (differing primarily in the way the variance is estimated) and a number of Bayesian frameworks (Baldi and Long, 2001; Fox and Dimmic, 2006). Although Gaussian assumptions have dominated the field, other types of parametrical approaches can also be found in the literature, such as regression modelling approaches (Thomas et al., 2001) and Gamma distribution models (Newton et al.,2001).Due to the uncertainty about the true underlying distribution of many gene expression scenarios, and the difficulties to validate distributional assumptions because of small sample sizes,non-parametric or model-free methods have been widely proposed as an attractive alternative to make less stringent distributional assumptions (Troyanskaya et al., 2002). Many model-free metrics, frequently borrowed from the statistics field, have demonstrated their usefulness in many gene expression studies, including the Wilcoxon rank-sum test (Thomas et al., 2001), the between-within classes sum of squares (BSS/WSS) (Dudoit et al., 2002) and the rank products method (Breitling et al., 2004).A specific class of model-free methods estimates the reference distribution of the statistic using random permutations of the data, allowing the computation of a model-free version of the associated parametric tests. These techniques have emerged as a solid alternative to deal with the specificities of DNA microarray data, and do not depend on strong parametric assumptions (Efron et al., 2001; Pan, 2003; Park et al., 2001; Tusher et al., 2001). Their permutation principle partly alleviates the problem of small sample sizes in microarray studies, enhancing the robustness against outliers.We also mention promising types of non-parametric metrics which, instead of trying to identify differentially expressed genes at the whole population level (e.g. comparison of sample means), are able to capture genes which are significantly disregulated in only a subset of samples (Lyons-Weiler et al., 2004; Pavlidis and Poirazi, 2006). These types of methods offer a more patient specific approach for the identification of markers, and can select genes exhibiting complex patterns that are missed by metrics that work under the classical comparison of two prelabelled phenotypic groups. In addition, we also point out the importance of procedures for controlling the different types of errors that arise in this complex multiple testing scenario of thousands of genes (Dudoit et al., 2003; Ploner et al., 2006; Pounds and Cheng, 2004; Storey, 2002), with a special focus on contributions for controlling the false discovery rate (FDR).3.2.2 Towards more advanced models: the multivariate paradigm for filter, wrapperand embedded techniquesUnivariate selection methods have certain restrictions and may lead to less accurate classifiers by, e.g. not taking into account gene–gene interactions. Thus, researchers have proposed techniques that try to capture these correlations between genes.The application of multivariate filter methods ranges from simple bivariate interactions (Bø and Jonassen, 2002) towards more advanced solutions exploring higher order interactions, such as correlation-based feature selection (CFS) (Wang et al., 2005; Yeoh et al., 2002) and several variants of the Markov blanket filter method (Gevaert et al., 2006; Mamitsuka, 2006; Xing et al., 2001). The Minimum Redundancy-Maximum Relevance (MRMR) (Ding and Peng, 2003) and Uncorrelated Shrunken Centroid (USC) (Yeung and Bumgarner, 2003) algorithms are two other solid multivariate filter procedures, highlighting the advantage of using multivariate methods over univariate procedures in the gene expression domain.Feature selection using wrapper or embedded methods offers an alternative way to perform a multivariate gene subset selection, incorporating the classifier's; bias into the search and thus offering an opportunity to construct more accurate classifiers. In the context of microarray analysis, most wrapper methods use population-based, randomized search heuristics (Blanco et al., 2004; Jirapech-Umpai and Aitken, 2005; Li et al., 2001; Ooi and Tan, 2003), although also a few examples use sequential search techniques (Inza et al., 2004; Xiong et al., 2001). An interesting hybrid filter-wrapper approach is introduced in (Ruiz et al., 2006), crossing a univariatelypre-ordered gene ranking with an incrementally augmenting wrapper method.Another characteristic of any wrapper procedure concerns the scoring function used to evaluate each gene subset found. As the 0–1 accuracy measure allows for comparison with previous works, the vast majority of papers uses this measure. However, recent proposals advocate the use of methods for the approximation of the area under the ROC curve (Ma and Huang, 2005), or the optimization of the LASSO (Least Absolute Shrinkage and Selection Operator) model (Ghosh and Chinnaiyan, 2005). ROC curves certainly provide an interesting evaluation measure, especially suited to the demand for screening different types of errors in many biomedical scenarios.The embedded capacity of several classifiers to discard input features and thus propose a subset of discriminative genes, has been exploited by several authors. Examples include the use of random forests (a classifier that combines many single decision trees) in an embedded way to calculate the importance of each gene (Díaz-Uriarte and Alvarez de Andrés, 2006; Jiang et al., 2004). Another line of embedded FS techniques uses the weights of each feature in linear classifiers, such as SVMs (Guyon et al., 2002) and logistic regression (Ma and Huang, 2005). These weights are used to reflect the relevance of each gene in a multivariate way, and thus allow for the removal of genes with very small weights.Partially due to the higher computational complexity of wrapper and to a lesser degree embedded approaches, these techniques have not received as much interest as filter proposals. However, an advisable practice is to pre-reduce the search space using a univariate filter method, and only then apply wrapper or embedded methods, hence fitting the computation time to the available resources.3.3 Mass spectra analysisMass spectrometry technology (MS) is emerging as a new and attractive framework for disease diagnosis and protein-based biomarker profiling (Petricoin and Liotta, 2003). A mass spectrum sample is characterized by thousands of different mass/charge (m / z) ratios on the x-axis, each with their corresponding signal intensity value on the y-axis. A typical MALDI-TOF low-resolution proteomic profile can contain up to 15 500 data points in the spectrum between 500 and 20 000 m / z, and the number of points even grows using higher resolution instruments.For data mining and bioinformatics purposes, it can initially be assumed that each m / z ratio represents a distinct variable whose value is the intensity. As Somorjai et al. (2003) explain, the data analysis step is severely constrained by both high-dimensional input spaces and their inherent sparseness, just as it is the case with gene expression datasets. Although the amount of publications on mass spectrometry based data mining is not comparable to the level of maturity reached in the microarray analysis domain, an interesting collection of methods has been presented in the last 4–5 years (see Hilario et al., 2006; Shin and Markey, 2006 for recent reviews) since the pioneering work of Petricoin et al.(2002).Starting from the raw data, and after an initial step to reduce noise and normalize the spectra from different samples (Coombes et al., 2007), the following crucial step is to extract the variables that will constitute the initial pool of candidate discriminative features. Some studies employ the simplest approach of considering every measured value as a predictive feature, thus applying FS techniques over initial huge pools of about 15 000 variables (Li et al., 2004; Petricoin et al., 2002), up to around 100 000 variables (Ball et al.,2002). On the other hand, a great deal of the current studies performs aggressive feature extraction procedures using elaborated peak detection and alignment techniques (see Coombes et al., 2007; Hilario et al., 2006; Shin and Markey, 2006 for a detailed description of these techniques). These procedures tend to seed the dimensionality from which supervised FS techniques will start their work in less than 500 variables (Bhanot et al., 2006; Ressom et al., 2007; Tibshirani et al., 2004). A feature extraction step is thus advisable to set the computational costs of many FS techniques to a feasible size in these MS scenarios. Table 3 presents an overview of FS techniques used in the domain of mass spectrometry. Similar to the domain of microarray analysis, univariate filter techniques seem to be the most common techniques used, although the use of embedded techniques is certainly emerging as an alternative. Although the t-test maintains a high level of popularity (Liu et al., 2002; Wu et al., 2003), other parametric measures such as F-test (Bhanot et al., 2006), and a notable variety of non-parametric scores (Tibshirani et al., 2004; Yu et al., 2005) have also been used in several MS studies. Multivariate filter techniques on the other hand, are still somewhat underrepresented (Liu et al., 2002; Prados et al., 2004).Table 3.Key references for each type of feature selection technique in the domain of mass pectrometryWrapper approaches have demonstrated their usefulness in MS studies by a group of influential works. Different types of population-based randomized heuristics are used as search engines in the major part of these papers: genetic algorithms (Li et al., 2004; Petricoin et al., 2002), particle swarm optimization (Ressom et al., 2005) and ant colony procedures (Ressom et al., 2007). It is worth noting that while the first two references start the search procedure in ∼ 15 000 dimensions by considering each m / z ratio as an initial predictive feature, aggressive peak detection and alignment processes reduce the initial dimension to about 300 variables in the last two references (Ressom et al., 2005; Ressom et al., 2007).An increasing number of papers uses the embedded capacity of several classifiers to discard input features. Variations of the popular method originally proposed for gene expression domains by Guyon et al. (2002), using the weights of the variables in the SVM-formulation to discard features with small weights, have been broadly and successfully applied in the MS domain (Jong et al., 2004; Prados et al., 2004; Zhang et al., 2006). Based on a similar framework, the weights of the input masses in a neural network classifier have been used to rank the features'importance in Ball et al. (2002). The embedded capacity of random forests (Wu et al., 2003) and other types of decision tree-based algorithms (Geurts et al., 2005) constitutes an alternative embedded FS strategy.Previous SectionNext Section4 DEALING WITH SMALL SAMPLE DOMAINSSmall sample sizes, and their inherent risk of imprecision and overfitting, pose a great challenge for many modelling problems in bioinformatics (Braga-Neto and Dougherty, 2004; Molinaro et al., 2005; Sima and Dougherty, 2006). In the context of feature selection, two initiatives have emerged in response to this novel experimental situation: the use of adequate evaluation criteria, and the use of stable and robust feature selection models.4.1 Adequate evaluation criteria。
DBN-Hinton-简洁
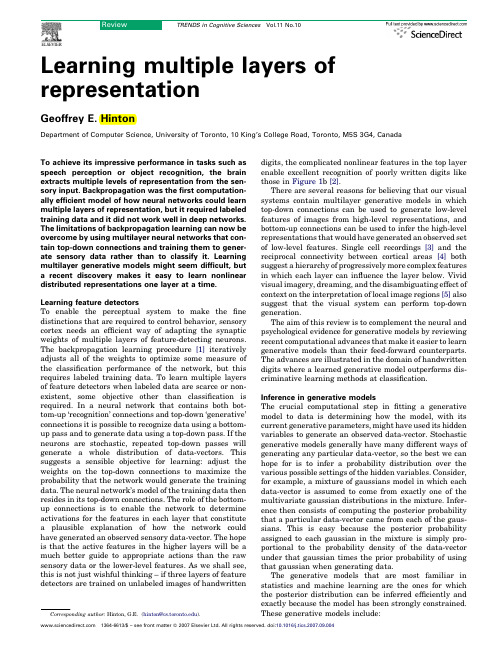
Learning multiple layers of representationGeoffrey E.HintonDepartment of Computer Science,University of Toronto,10King’s College Road,Toronto,M5S 3G4,CanadaTo achieve its impressive performance in tasks such as speech perception or object recognition,the brain extracts multiple levels of representation from the sen-sory input.Backpropagation was the first computation-ally efficient model of how neural networks could learn multiple layers of representation,but it required labeled training data and it did not work well in deep networks.The limitations of backpropagation learning can now be overcome by using multilayer neural networks that con-tain top-down connections and training them to gener-ate sensory data rather than to classify it.Learning multilayer generative models might seem difficult,but a recent discovery makes it easy to learn nonlinear distributed representations one layer at a time.Learning feature detectorsTo enable the perceptual system to make the fine distinctions that are required to control behavior,sensory cortex needs an efficient way of adapting the synaptic weights of multiple layers of feature-detecting neurons.The backpropagation learning procedure [1]iteratively adjusts all of the weights to optimize some measure of the classification performance of the network,but this requires labeled training data.To learn multiple layers of feature detectors when labeled data are scarce or non-existent,some objective other than classification is required.In a neural network that contains both bot-tom-up ‘recognition’connections and top-down ‘generative’connections it is possible to recognize data using a bottom-up pass and to generate data using a top-down pass.If the neurons are stochastic,repeated top-down passes will generate a whole distribution of data-vectors.This suggests a sensible objective for learning:adjust the weights on the top-down connections to maximize the probability that the network would generate the training data.The neural network’s model of the training data then resides in its top-down connections.The role of the bottom-up connections is to enable the network to determine activations for the features in each layer that constitute a plausible explanation of how the network could have generated an observed sensory data-vector.The hope is that the active features in the higher layers will be a much better guide to appropriate actions than the raw sensory data or the lower-level features.As we shall see,this is not just wishful thinking –if three layers of feature detectors are trained on unlabeled images of handwrittendigits,the complicated nonlinear features in the top layer enable excellent recognition of poorly written digits like those in Figure 1b [2].There are several reasons for believing that our visual systems contain multilayer generative models in which top-down connections can be used to generate low-level features of images from high-level representations,and bottom-up connections can be used to infer the high-level representations that would have generated an observed set of low-level features.Single cell recordings [3]and the reciprocal connectivity between cortical areas [4]both suggest a hierarchy of progressively more complex features in which each layer can influence the layer below.Vivid visual imagery,dreaming,and the disambiguating effect of context on the interpretation of local image regions [5]also suggest that the visual system can perform top-down generation.The aim of this review is to complement the neural and psychological evidence for generative models by reviewing recent computational advances that make it easier to learn generative models than their feed-forward counterparts.The advances are illustrated in the domain of handwritten digits where a learned generative model outperforms dis-criminative learning methods at classification.Inference in generative modelsThe crucial computational step in fitting a generative model to data is determining how the model,with its current generative parameters,might have used its hidden variables to generate an observed data-vector.Stochastic generative models generally have many different ways of generating any particular data-vector,so the best we can hope for is to infer a probability distribution over the various possible settings of the hidden variables.Consider,for example,a mixture of gaussians model in which each data-vector is assumed to come from exactly one of the multivariate gaussian distributions in the mixture.Infer-ence then consists of computing the posterior probability that a particular data-vector came from each of the gaus-sians.This is easy because the posterior probability assigned to each gaussian in the mixture is simply pro-portional to the probability density of the data-vector under that gaussian times the prior probability of using that gaussian when generating data.The generative models that are most familiar in statistics and machine learning are the ones for which the posterior distribution can be inferred efficiently and exactly because the model has been strongly constrained.These generative modelsinclude:TRENDS in Cognitive Sciences Vol.11No.10Corresponding author:Hinton,G.E.(hinton@ ).1364-6613/$–see front matter ß2007Elsevier Ltd.All rights reserved.doi:10.1016/j.tics.2007.09.004Factor analysis –in which there is a single layer of gaussian hidden variables that have linear effects on the visible variables (see Figure 2).In addition,independent gaussian noise is added to each visible variable [6–8].Given a visible vector,it is impossible to infer the exact state of the factors that generated it,but it is easy to infer the mean and covariance of the gaussian posterior distribution over the factors,and this is sufficient to enable the parameters of the model to be improved. Independent components analysis –which generalizes factor analysis by allowing non-gaussian hidden vari-ables,but maintains tractable inference by eliminating the observation noise in the visible variables and using the same number of hidden and visible variables.These restrictions ensure that the posterior distribution collapses to a single point because there is only one setting of the hidden variables that can generate each visible vector exactly [9–11].Mixture models –in which each data-vector is assumed to be generated by one of the component distributions in the mixture and it is easy to compute the density under each of the component distributions.If factor analysis is generalized to allow non-gaussian hidden variables,it can model the development of low-level visual receptive fields [12].However,if the extra con-straints used in independent components analysis are not imposed,it is no longer easy to infer,or even to represent,the posterior distribution over the hidden vari-ables.This is because of a phenomenon known as explain-ing away [13](see Figure 3b).Multilayer generative modelsGenerative models with only one hidden layer are much too simple for modeling the high-dimensional and richly struc-tured sensory data that arrive at the cortex,but they have been pressed into service because,until recently,it was too difficult to perform inference in the more complicated,multilayer,nonlinear models that are clearly required.There have been many attempts to develop multilayer,nonlinear models [14–18].In Bayes nets (also called belief nets),which have been studied intensively in artificial intelligence and statistics,the hidden variables typically have discrete values.Exact inference is possible if every variable only has a few parents.This can occur in Bayes nets that are used to formalize expert knowledge in limited domains [19],but for more densely connected Bayes nets,exact inference is generally intractable.It is important to realize that if some way can be found to infer the posterior distribution over the hidden variables for each data-vector,learning a multilayer generative model is relatively straightforward.Learning is also straightforward if we can get unbiased samples from the posterior distribution.In this case,we simply adjust the parameters so as to increase the probability that the sampled states of the hidden variables in each layerwouldFigure 1.(a)The generative model used to learn the joint distribution of digit images and digit labels.(b)Some test images that the network classifies correctly even though it has never seen thembefore.Figure 2.The generative model used in factor analysis.Each real-valued hidden factor is chosen independently from a gaussian distribution,N (0,1),with zero mean and unit variance.The factors are then linearly combined using weights (W jk )and gaussian observation noise with mean (m i )and standard deviation (s i )is added independently to each real-valued variable (i ).TRENDS in Cognitive Sciences Vol.11No.10429generate the sampled states of the hidden or visible variables in the layer below.In the case of the logistic belief net shown in Figure 3a,which will be a major focus of this review,the learning rule for each training case is a version of the delta rule [20].The inferred state,h i ,of the ‘postsynaptic’unit,i ,acts as a target value and the prob-ability,ˆhi ,of activating i given the inferred states,h j ,of all the ‘presynaptic’units,j ,in the layer above acts as a prediction:D w ji /h j ðh i Àˆhi Þ(Equation 1)where D w ji is the change in the weight on the connectionfrom j to i .If i is a visible unit,h i is replaced by the actual state of i in the training example.If training vectors are selected with equal probability from the training set and the hidden states are sampled from their posterior distribution given the training vector,the learning rule in Equation 1has a positive expected effect on the probability that the gen-erative model would produce exactly the N training vectors if it was run N times.Approximate inference for multilayer generative modelsThe generative model in Figure 3a is defined by the weights on its top-down,generative connections,but it also has bottom-up,recognition connections that can be used to perform approximate inference in a single,bottom-up pass.The inferred probability that h j =1is s (S i h i r ij ).This inference procedure is fast and simple,but it is incorrect because it ignores explaining away.Surprisingly,learning is still possible with incorrect inference because there is a more general objective function that the learning rule in Equation 1is guaranteed to improve [21,22].Instead of just considering the log probability of gen-erating each training case,we can also take the accuracy ofthe inference procedure into account.Other things being equal,we would like our approximate inference method to be as accurate as possible,and we might prefer a model that is slightly less likely to generate the data if it enables more accurate inference of the hidden representations.So it makes sense to use the inaccuracy of inference on each training case as a penalty term when maximizing the log probability of the observed data.This leads to a new objective function that is easy to maximize and is a ‘vari-ational’lower-bound on the log probability of generating the training data [23].Learning by optimizing a vari-ational bound is now a standard way of dealing with the intractability of inference in complex generative models [24–27].An approximate version of this type of learning has been proposed as a model of learning in sensory cortex (Box 1),but it is slow in deep networks if the weights are initialized randomly.A nonlinear module with fast exact inferenceWe now turn to a different type of model called a ‘restricted Boltzmann machine’(RBM)[28](Figure 4a).Despite its undirected,symmetric connections,the RBM is the key to finding an efficient learning procedure for deep,directed,generative models.Images composed of binary pixels can be modeled by using the hidden layer of an RBM to model the higher-order correlations between pixels [29].To learn a good set of feature detectors from a set of training images,we start with zero weights on the symmetric connections between each pixel i and each feature detector j .Then we repeatedly update each weight,w ij ,using the difference between two measured,pairwise correlations D w i j ¼e ð<v i h j >data À<v i h i >recon Þ(Equation 2)where e is a learning rate,<v i h j >data is the frequency with which pixel i and feature detector j are on togetherwhenFigure 3.(a)A multilayer belief net composed of logistic binary units.To generate fantasies from the model,we start by picking a random binary state of 1or 0for each top-level unit.Then we perform a stochastic downwards pass in which the probability,ˆhi ,of turning on each unit,i ,is determined by applying the logistic function s (x )=1/(1+exp(Àx ))to the total input S j h j w ji that i receives from the units,j ,in the layer above,where h j is the binary state that has already been chosen for unit j .It is easy to give each unit an additional bias,but to simplify this review biases will usually be ignored.r ij is a recognition weight.(b)An illustration of ‘explaining away’in a simple logistic belief net containing two independent,rare,hidden causes that become highly anticorrelated when we observe the house jumping.The bias of À10on the earthquake unit means that,in the absence of any observation,this unit is e 10times more likely to be off than on.If the earthquake unit is on and the truck unit is off,the jump unit has a total input of 0,which means that it has an even chance of being on.This is a much better explanation of the observation that the house jumped than the odds of e À20,which apply if neither of the hidden causes is active.But it is wasteful to turn on both hidden causes to explain the observation because the probability of them both happening is approximately e À20.430TRENDS in Cognitive Sciences Vol.11No.10the feature detectors are being driven by images from the training set,and <v i h j >recon is the corresponding frequency when the feature detectors are being driven by recon-structed images.A similar learning rule can be used for the biases.Given a training image,we set the binary state,h j ,of each feature detector to be 1with probabilityp ðh j ¼1Þ¼s ðb j þXiv i w i j Þ(Equation 3)where s ( )is the logistic function,b j is the bias of j and v i isthe binary state of pixel i .Once binary states have been chosen for the hidden units we produce a ‘reconstruction’of the training image by setting the state of each pixel to be 1with probabilityp ðv i ¼1Þ¼s ðb i þXjh j w i j Þ(Equation 4)The learned weights and biases directly determine theconditional distributions p (h j v )and p (v j h )using Equations 3and 4.Indirectly,the weights and biases define the joint and marginal distributions p (v ,h ),p (v )and p (h ).Sampling from the joint distribution is difficult,but it can be done by using ‘alternating Gibbs sampling’.This starts with a random image and then alternates between updating all of the features in parallel using Equation 3and updating all of the pixels in parallel using Equation 4.After Gibbs sampling for sufficiently long,the network reaches ‘thermal equilibrium’.The states of pixels and feature detectors still change,but the probability of finding the system in any particular binary configuration does not.By observing the fantasies on the visible units at thermal equilibrium,we can see the distribution over visible vectors that the model believes in.The RBM has two major advantages over directed models with one hidden layer.First,inference is easy because there is no explaining away:given a visible vector,the posterior distribution over hidden vectors factorizes into a product of independent distributions for each hidden unit.So to get a sample from the posterior we simply turn on each hidden unit with a probability given by Equation 3.Box 1.The wake-sleep algorithmFor the logistic belief net shown in Figure 3a,it is easy to improve the generative weights if the network already has a good set of recognition weights.For each data-vector in the training set,the recognition weights are used in a bottom-up pass that stochastically picks a binary state for each hidden unit.Applying the learning rule in Equation 1will then follow the gradient of a variational bound on how well the network generates the training data [22].It is not so easy to compute the derivatives of the bound with respect to the recognition weights,but there is a simple,approx-imate learning rule that works well in practice.If we generate fantasies from the model by using the generative weights in a top-down pass,we know the true causes of the activities in each layer,so we can compare the true causes with the predictions made by the approximate infererence procedure and adjust the recognition weights,r ij ,to maximize the probability that the predictions are correct:D r i j /h i h j Às ðXih i r i j Þ (Equation 5)The combination of approximate inference for learning the gen-erative weights,and fantasies for learning the recognition weights isknown as the ‘wake-sleep’algorithm [22].Figure 4.(a)Two separate restricted Boltzmann machines (RBMs).The stochastic,binary variables in the hidden layer of each RBM are symmetrically connected to the stochastic,binary variables in the visible layer.There are no connections within a layer.The higher-level RBM is trained by using the hidden activities of the lower RBM as data.(b)The composite generative model produced by composing the two RBMs.Note that the connections in the lower layer of the composite generative model are directed.The hidden states are still inferred by using bottom-up recognition connections,but these are no longer part of the generative model.TRENDS in Cognitive Sciences Vol.11No.10431Second,as we shall see,it is easy to learn deep directed networks one layer at a time by stacking yer-by-layer learning does not work nearly as well when the individual modules are directed,because each directed module bites off more than it can chew:it tries to learn hidden causes that are marginally independent.This is generally beyond its abilities so it settles for a generative model in which independent causes generate a poor approximation to the data distribution.Learning many layers of features by composing RBMs After an RBM has been learned,the activities of its hidden units(when they are being driven by data)can be used as the‘data’for learning a higher-level RBM.To understand why this is a good idea,it is helpful to consider decompos-ing the problem of modeling the data distribution,P0,into two subproblems by picking a distribution,P1,that is easier to model than P0.Thefirst subproblem is to model P1and the second subproblem is to model the transform-ation from P1to P0.P1is the distribution obtained by applying p(h j v)to the data distribution to get the hidden activities for every data-vector in the training set.P1is easier for an RBM to model than P0because it is obtained from P0by allowing an RBM to settle towards a distri-bution that it can model perfectly:its equilibrium distri-bution.The RBM’s model of P1is p(h),the distribution over hidden vectors when the RBM is sampling from its equi-librium distribution.The RBM’s model of the transform-ation from P1to P0is p(v j h).After thefirst RBM has been learned,we keep p(v j h)as part of the generative model and we keep p(h j v)as a quick way of performing inference,but we throw away our model of P1and replace it by a better model that is obtained, recursively,by treating P1as the training data for the second-level RBM.This leads to the composite generative model shown in Figure4b.To generate from this model we need to get an equilibrium sample from the top-level RBM, but then we simply perform a single downwards pass through the bottom layer of weights.So the composite model is a curious hybrid whose top two layers form an undirected associative memory and whose lower layers form a directed generative model.It is shown in reference [30]that if the second RBM is initialized appropriately,the gain from building a better model of P1always outweighs the loss that comes from the fact that p(h j v)is no longer the correct way to perform inference in the composite genera-tive model shown in Figure4b.Adding another hidden layer always improves a variational bound on the log probability of the training data unless the top-level RBM is already a perfect model of the data it is trained on. Modeling images of handwritten digitsFigure1a shows a network that was used to model the joint distribution of digit images and their labels.It was learned one layer at a time and the top-level RBM was trained using‘data’-vectors that were constructed by concatenat-ing the states of ten winner-take-all label units with500 binary features inferred from the image.After greedily learning one layer of weights at a time,all the weights were fine-tuned using a variant of the wake-sleep algorithm(see reference[30]for details).Thefine-tuning significantly improves the ability of the model to generate images that resemble the data,but without the initial layer-by-layer learning,thefine-tuning alone is hopelessly slow.The model was trained to generate both a label and an image,but it can be used to classify new images.First,the recognition weights are used to infer binary states for the 500feature units in the second hidden layer,then alter-nating Gibbs sampling is applied to the top two layers with these500features heldfixed.The probability of each label is then represented by the frequency with which it turns ing an efficient version of this method,the network significantly outperforms both backpropagation and sup-port vector machines[31]when trained on the same data [30].A demonstration of the model generating and recog-nizing digit images is at my homepage(www.cs.toronto. edu/$hinton).Instead offine-tuning the model to be better at generating the data,backpropagation can be used to fine-tune it to be better at discrimination.This works extremely well[2,20].The initial layer-by-layer learning finds features that enable good generation and then the discriminativefine-tuning slightly modifies these features to adjust the boundaries between classes.This has the great advantage that the limited amount of information in the labels is used only for perturbing features,not for creating them.If the ultimate aim is discrimination it is possible to use autoencoders with a single hidden layer instead of restricted Boltzmann machines for the unsuper-vised,layer-by-layer learning[32].This produces the best results ever achieved on the most commonly used bench-mark for handwritten digit recognition[33].Modeling sequential dataThis review has focused on static images,but restricted Boltzmann machines can also be applied to high-dimen-sional sequential data such as video sequences[34]or the joint angles of a walking person[35].The visible and hidden units are given additional,conditioning inputs that come from previous visible frames.The conditioning inputs have the effect of dynamically setting the biases of the visible and hidden units.These conditional restricted Boltzmann machines can be composed by using the sequence of hidden activities of one as the training data for the next.This creates multilayer distributed repres-entations of sequences that are far more powerful than the representations learned by standard methods such as hidden Markov models or linear dynamical systems[34]. Concluding remarksA combination of three ideas leads to a novel and effective way of learning multiple layers of representation.Thefirst idea is to learn a model that generates sensory data rather than classifying it.This eliminates the need for large amounts of labeled data.The second idea is to learn one layer of representation at a time using restricted Boltz-mann machines.This decomposes the overall learning task into multiple simpler tasks and eliminates the infer-ence problems that arise in directed generative models. The third idea is to use a separatefine-tuning stage to improve the generative or discriminative abilities of the composite model.432TRENDS in Cognitive Sciences Vol.11No.10 Versions of this approach are currently being applied to tasks as diverse as denoising images[36,37],retrieving documents[2,38],extracting opticalflow[39],predicting the next word in a sentence[40]and predicting what movies people will like[41].Bengio and Le Cun[42]give further reasons for believing that this approach holds great promise for artificial intelligence applications,such as human-level speech and object recognition,that have proved too difficult for shallow methods like support vector machines[31]that cannot learn multiple layers of representation.The initial successes of this approach to learning deep networks raise many ques-tions(see Box2).There is no concise definition of the types of data for which this approach is likely to be successful,but it seems most appropriate when hidden variables generate richly structured sensory data that provide plentiful infor-mation about the states of the hidden variables.If the hidden variables also generate a label that contains little information or is only occasionally observed,it is a bad idea to try to learn the mapping from sensory data to labels using discriminative learning methods.It is much more sensiblefirst to learn a generative model that infers the hidden variables from the sensory data and then to learn the simpler mapping from the hidden variables to the labels.AcknowledgementsI thank Yoshua Bengio,David MacKay,Terry Sejnowski and my past and present postdoctoral fellows and graduate students for helping me to understand these ideas,and NSERC,CIAR,CFI and OIT for support.References1Rumelhart, D.E.et al.(1986)Learning representations by back-propagating errors.Nature323,533–5362Hinton,G.E.and Salakhutdinov,R.R.(2006)Reducing the dimensionality of data with neural networks.Science313,504–507 3Lee,T.S.et al.(1998)The role of the primary visual cortex in higher level vision.Vision Res.38,2429–24544Felleman,D.J.and Van Essen,D.C.(1991)Distributed hierarchical processing in the primate cerebral cortex.Cereb.Cortex1,1–475Mumford, D.(1992)On the computational architecture of the neocortex.II.The role of cortico-cortical loops.Biol.Cybern.66, 241–2516Dayan,P.and Abbott,L.F.(2001)Theoretical Neuroscience: Computational and Mathematical Modeling of Neural Systems,MIT Press7Roweis,S.and Ghahramani,Z.(1999)A unifying review of linear gaussian models.Neural Comput.11,305–3458Marks,T.K.and Movellan,J.R.(2001)Diffusion networks,products of experts,and factor analysis.In Proceedings of the International Conference on Independent Component Analysis(Lee,T.W.et al., eds),pp.481–485,/article/marks01diffusion.html9Bell,A.J.and Sejnowski,T.J.(1995)An information-maximization approach to blind separation and blind deconvolution.Neural Comput.7,1129–115910Hyva¨rinen,A.et al.(2001)Independent Component Analysis,Wiley 11Bartlett,M.S.et al.(2002)Face recognition by independent component analysis.IEEE Trans.Neural Netw.13,1450–146412Olshausen, B.A.and Field, D.(1996)Emergence of simple-cell receptivefield properties by learning a sparse code for natural images.Nature381,607–60913Pearl,J.(1988)Probabilistic Inference in Intelligent Systems:Networks of Plausible Inference,Morgan Kaufmann14Lewicki,M.S.and Sejnowski,T.J.(1997)Bayesian unsupervised learning of higher order structure.In Advances in Neural Information Processing Systems(Vol.9)(Mozer,M.C.et al.,eds),pp.529–535,MIT Press15Hoyer,P.O.and Hyva¨rinen,A.(2002)A multi-layer sparse coding network learns contour coding from natural images.Vision Res.42, 1593–160516Portilla,J.et al.(2004)Image denoising using Gaussian scale mixtures in the wavelet domain.IEEE Trans.Image Process.12, 1338–135117Schwartz,O.et al.(2006)Soft mixer assignment in a hierarchical generative model of natural scene statistics.Neural Comput.18,2680–271818Karklin,Y.and Lewicki,M.S.(2003)Learning higher-order structures in natural work14,483–49919Cowell,R.G.et al.(2003)Probabilistic Networks and Expert Systems, Springer20O’Reilly,R.C.(1998)Six principles for biologically based computational models of cortical cognition.Trends Cogn.Sci.2,455–46221Hinton,G.E.and Zemel,R.S.(1994)Autoencoders,minimum description length,and Helmholtz free energy.Adv.Neural Inf.Process.Syst.6,3–1022Hinton,G.E.et al.(1995)The wake-sleep algorithm for self-organizing neural networks.Science268,1158–116123Neal,R.M.and Hinton,G.E.(1998)A new view of the EM algorithm that justifies incremental,sparse and other variants.In Learning in Graphical Models(Jordan,M.I.,ed.),pp.355–368,Kluwer Academic Publishers24Jordan,M.I.et al.(1999)An introduction to variational methods for graphical models.Mach.Learn.37,183–23325Winn,J.and Jojic,N.(2005)LOCUS:Learning object classes with unsupervised segmentation,Tenth IEEE International Conference on Computer Vision(Vol.1),pp.756–763,IEEE Press26Bishop, C.M.(2006)Pattern Recognition and Machine Learning, Springer27Bishop,C.M.et al.(2002)VIBES:a variational inference engine for Bayesian networks.Adv.Neural Inf.Process.Syst.15,793–80028Hinton,G.E.(2007)Boltzmann Machines,Scholarpedia29Hinton,G.E.(2002)Training products of experts by minimizing contrastive divergence.Neural Comput.14,1711–1800Box2.Questions for future researchHow might this type of algorithm be implemented in cortex?Inparticular,is the initial perception of sensory input closelyfollowed by a reconstruction that uses top-down connections?Computationally,the learning procedure for restricted Boltzmannmachines does not require a‘pure’reconstruction.All that isrequired is that there are two phases that differ in the relativebalance of bottom-up and top-down influences,with synapticpotentiation in one phase and synaptic depression in the other.Can this approach deal adequately with lateral connections andinhibitory interneurons?Currently,there is no problem inallowing lateral interactions between the visible units of a‘semirestricted’Boltzmann machine[30,43].Lateral interactionsbetween the hidden units can be added when these become thevisible units of the higher-level,semirestricted Boltzmann ma-chine.This makes it possible to learn a hierarchy of undirectedMarkov random fields,each of which has directed connections tothe field below as suggested in ref.[44].This is a more powerfultype of generative model because each level only needs toprovide a rough specification of the states at the level below:Thelateral interactions at the lower level can settle on the fine detailsand ensure that they obey learned constraints.Can we understand the representations that are learned in thedeeper layers?In a generative model,it is easy to see what adistributed pattern of activity over a whole layer means:simplygenerate from it to get a sensory input vector(e.g.an image).It ismuch harder to discover the meaning of activity in an individualneuron in the deeper layers because the effects of that activitydepend on the states of all the other nonlinear neurons.The factthe some neurons in the ventral stream can be construed as facedetectors is intriguing,but I can see no good reason to expectsuch simple stories to be generally applicable.TRENDS in Cognitive Sciences Vol.11No.10433 。
多模态话语分析Multimoda...

多模态话语分析Multimodal_Discourse_Analysis__Systemic_Functional_ Perspectives__Open_Linguistics_Multirnodal Discourse Analysis Systemic-Functional PerspectivesOpen Linguistics Series Series EditorRobin Fawcett, Cardiff UniversityThe series is 'open' in two related ways. First, it is not confined to works associated withany one school of linguistics. For almost two decades the series has played a significantrole in establishing and maintaining the present climate of 'openness' in linguistics, andwe intend to maintain this tradition. However, we particularly welcome works whichexplore the nature and use of language through modelling its potential for use in socialcontexts, or through a cognitive model of language - or indeed a combination of the two.The series is also 'open' in the sense that it welcomesworks that open out 'core'linguistics in various ways: to give a central place to the description of natural texts and theuse of corpora; to encompass discourse 'above the sentence'; to relate language to othersemiotic systems; to apply linguistics in fields such as education, language pathology andlaw; and to explore the areas that lie between linguistics and its neighbouring disciplinessuch as semiotics, psychology, sociology, philosophy, and cultural and literary studies.Continuum also publishes a series that offers a forum for primarily functionaldescriptions of languages or parts of languages ? Functional Descriptions of Language.Relations between linguistics and computing are covered in the Communication in ArtificialIntelligence series, two series, Advances in Applied Linguistics and Communication in Public Life,publish books in applied linguistics and the series Modern Pragmatics in Theory and Practicepublishes both social and cognitive perspectives on themaking of meaning in languageuse. We also publish a range of introductory textbooks on topics in linguistics, semioticsand deaf studies.Recent titles in this seriesClassroom Discourse Analysis: A Functional Perspective, Frances ChristieConstruing Experience through Meaning: A Language-based Approach to Cognition,M. A. K. Halliday and Christian M. I. M. MatthiessenCulturally Speaking: Managing Rapport through Talk across Cultures, Helen Spencer-Oatey ed.Educating Eve: The 'Language Instinct' Debate, Geoffrey SampsonEmpirical Linguistics, Geoffrey SampsonGenre and Institutions: Social Processes in the Workplace and School, Frances Christie andJ. R. Martin edsThe Intonation Systems of English, Paul TenchLanguage Policy in Britain and France: The Processes of Policy, Dennis AgerLanguage Relations across Bering Strait: Reappraising theArchaeological and Linguistic Evidence,Michael FortescueLearning through Language in Early Childhood, Clare PainterPedagogy and the Shaping of Consciousness: Linguistic and Social Processes, Frances Christie ed.Register Analysis: Theory and Practice, Mohsen Ghadessy ed.Relations and Functions within and around Language, Peter H. Fries, Michael Cummings,David Lockwood and William Spruiell edsResearching Language in Schools and Communities: Functional Linguistic Perspectives,Len Unsworth ed.Summary Justice: Judges Address Juries, Paul Robertshaw Syntactic Analysis and Description: A Constructional Approach, David G. LockwoodThematic Developments in English Texts, Mohsen Ghadessy ed.Ways of Saying: Ways of Meaning. Selected Papers of Ruqaiya Hasan. Carmen Cloran, DavidButt and Geoffrey Williams edsWords, Meaning and Vocabulary: An Introduction to Modern English Lexicology, Howard Jacksonand Etienne Zé AmvelaWorking with Discourse: Meaning beyond the Clause, J. R. Martin and David RoseMultimodal Discourse Analysis Systemic-Functional PerspectivesEdited by Kay L. O'HallorancontinuumLONDO N NE W YORKContinuumThe Tower Building 15 East 26th Street11 York Road New YorkLondon SE1 7NX NY 10010Kay L. O'Halloran 2004All rights reserved. No part of this publication may be reproduced or transmittedin any form or by any means, electronic or mechanical, including photocopying,recording, or any information storage or retrieval system, without prior permissionin writing from the publishers.British Library Cataloguing-in-Publication DataA catalogue record for this book is available from the British Library.ISBN: 0-8264-7256-7Library of Congress Cataloguing-in-Publication DataA catalogue record for this book is available from the Library of Congress.Typeset by RefineCatch Limited, Bungay, SuffolkPrinted and bound in Great Britain by MPG Books Ltd, Bodmin, CornwallContentsIntroduction 1Kay L. O'HallomnPart IThree-dimensional material objects in space1 Opera Ludentes: the Sydney Opera House at work and play 11Michael O'Toole2 Making history in From Colony to Nation: a multimodal analysisof a museum exhibition in Singapore 28Alfred Pang Kah Meng3 A semiotic study of Singapore's Orchard Road and MarriottHotel 55Safeyaton AliasPart IIElectronic media and film4 Phase and transition, type and instance: patterns in media textsas seen through a multimodal concordancer 83Anthony P. Baldry5 Visual semiosis in film 109Kay L. O'Halloran6 Multisemiotic mediation in hypertext 131Arthur Kok Kum ChiewPart IIIPrint media7 The construal of Ideational meaning in print advertisements 163Cheong Tin Yuenvi CONTENTS8 Multimodality in a biology textbook 196Libo Guo9 Developing an integrative multi-semiotic model 220Victor Lim FeiIndex 247This book is dedicated to my mother, Janet O'HalloranThis page intentionally left blank Introduction Kay L. O'HalloranMulti-modal Discourse Analysis is a collection of researchpapers in the field ofmultimodality. These papers are concerned with developing the theory andpractice of the analysis of discourse and sites which make use of multiplesemiotic resources; for example, language, visual images, space and archi-tecture. New social semiotic frameworks are presented for the analysis of arange of discourse genres in print media, dynamic and static electronicmedia and three-dimensional objects in space. The theoretical approachinforming these research efforts is Michael Halliday's 1994 systemic-functional theory of language which is extended to other semiotic resources.These frameworks, many of which are inspired by Michael O'Toole's 1994approach in The Language of Displayed Art, are also used to investigate mean-ing arising from the integrated use of semiotic resources.The research presented here represents the early stages in a shift of focusin linguistic enquiry where language use is no longer theorized as an isolatedphenomenon see, for example, Baldry, 2000; Kress, 2003; Kress and vanLeeuwen, 1996, 2001; ledema, 2003; Ventola et al., forthcoming. Theanalysis and interpretation of language use is contextualized in conjunctionwith other semiotic resources which are simultaneously used for the con-struction of meaning. For example, in addition to linguistic choices and theirtypographical instantiation on the printed page,1 multimodal analysis takesinto account the functions and meaning of the visual images, together withthe meaning arising from the integrated use of the two semiotic resources.To date, the majority of research endeavours in linguistics have tended toconcentrate solely on language while ignoring, or at least downplaying, thecontributions of other meaning-making resources. This has resulted inrather an impoverished view of functions and meaning of discourse.Language studies are thus undergoing a major shift to account fully formeaning-making practices as evidenced by recent research in multimodalityfor example, Baldry, 2000; Callaghan and McDonald, 2002; ledema, 2001;Jewitt, 2002; Martin, forthcoming; Kress, 2000, 2003; Kress et al., 2001:Kress and van Leeuwen, 1996, 2001; Lemke, 1998, 2002, 2003; O'Halloran,1999a, 2000, 2003a, 2003b; Royce, 2002; Thibault, 2000; Unsworth, 2001;Ventola et al., forthcoming; Zammit and Callow, 1998.Multimodal Discourse Analysis contains an invited paper by Michael2 INTRODUCTIONO'Toole, a founding scholar in the extension ofsystemic-functional theoryto semiotic resources other than language. The collection also features aninvited contribution from Anthony Baldry, a forerunner in the use of inform-ation technology for the development of multimodal theory and practice.The remaining seven research papers have been completed by KayO'Halloran and her postgraduate students in the Semiotics Research GroupSRG in the Department of English Language and Literature at theNational University of Singapore. The SRG has been actively involved inresearch in systemic-functional approaches to multimodality over theperiod 1999-2003.The papers are organized into sections according to the medium of thediscourse: Part I which is concerned with three-dimensional material objectsin space, Part II which deals with electronic media and film and Part IIIwhich contains investigations into print media. The theoretical advancespresented in this volume are illustrated through the analysis of a range ofmultimodal discourses and sites, some of which are Singaporean. Thesecontributions represent a critical yet sensitive interpretation of everydaydiscourses in Singapore. Thus, like all discourse, they are grounded in localknowledge, but due to the universality of the semiotic model being used,they are applicable to similar texts in any culture. A brief synopsis of eachpaper in this collection is given below.In Michael O'Toole's opening paper in Part I, 'Opera Ludentes: theSydney Opera House at work and play', a systemic-functional analysis ofarchitecture O'Toole, 1990, 1994 is used to consider inturn the Experien-tial, Interpersonal and Textual functions ofJ0rn Utzon's 1957-73 SydneyOpera House and its parts, both internally and in relation to its physical andsocial context. In this paper, the usual definition of 'functionalism' in archi-tecture is significantly extended. Like language, the building embodies anExperiential function: its practical purposes, the 'lexical content' of its com-ponents theatre, stage, seats, lights, and so forth and the relations of whodoes what to whom, and when and where. It also embodies a 'stance' vis-a-vis the viewer and user its facade, height, transparency, resemblance toother buildings or objects which also reflects the power relations betweengroups of users. That is, it embodies an Interpersonal function like lan-guage. The Sydney Opera House also embodies a Textualfunction: its partsconnect with each other and combine to make a coherent 'text', and itrelates meaningfully to its surrounding context of streets, quays, harbour,nearby buildings and cityscape, and by 'meaningful' here we include delib-erate dramatic contrast as well as harmonious blending in. In the analysis,certain features are discovered to be multifunctional, marking 'hot spots' ofmeaning in the total building complex. In terms of all three functions, theOpera House emerges as a playful building: Opera Ludentes. Utzon's build-ing started its life as a focus of architectural and political controversy andmost discourses about the building are still preoccupied with the politics ofits conception, competition, controversies and completion by different archi-tects. A semiotic rereading of the building can relate itsstructure and designINTRODUCTION 3to the 'social semiotic' of both Sydney in the 1960s and to the internationalcommunity of its users today.The museum is located as the next site for semiotic study in Alfred Pang's'Making history in From Colony to Nation: a multimodal analysis of a museumexhibition in Singapore'. Pang discusses how systemic-functional theory isproductive in fashioning an interpretative framework that facilitates a multi-modal analysis of a museum exhibition. The usefulness of this frameworkis exemplified in the critical analyses of particular displays in From Colony toNation, an exhibition at the Singapore History Museum SHM that displaysSingapore's political constitutional history. From this analysis, Pang explainshow the museum as a discursive site powerfully constitutes and maintainsparticular social structures through the primary composite medium of anexhibition. Of interest is the relationship between the museum, nation andhistory and how the multimodal representation of history in From Colony toNation ideologically positions the visitor to a particular style of imagining a'nation' Anderson, 1991.Safeyaton Alias investigates the semiotic makeup of the city in 'A semioticstudy of Singapore's Orchard Road and Marriott Hotel'. Like a written text,the city stores information and 'presents particular transformations andembeddings of a culture's knowledge of itself and of the world' Preziosi,1984: 50-51. In this paper, a rank-scale framework for the functions andsystems in the three-dimensional multi-semiotic city is proposed. The focus inthis paper, however, is the analysis of the built forms ofOrchard Road andthe Marriott Hotel. Safeyaton discusses how these built forms transmit mes-sages which are articulated through choices in a range of metafunctionallybased systems. This paper discusses the intertextuality and the discourses thatconstruct Singapore as a city that survives on consumerism and capitalism.In Part II on electronic media and film, Anthony Baldry's opening paper,'Phase and transition, type and instance: patterns in media texts as seenthrough a multimodal concordancer', explores the use of computer tech-nology for capturing 'the slippery eel-like' to quote Baldry dynamics ofsemiosis. Baldry demonstrates that the online multimodal concordancer, theMultimodal Corpus Authoring MCA system, provides new possibilities forthe analysis and comparison of film and videotexts. Thistype of concord-ancing transcends in vitro approaches by preserving the dynamic text, insofaras this is ever possible, in its original form. The relational properties of themultimodal concordancer also allow a researcher to embark on a quest forpatterns and types. Taking the crucial semiotic units of phase and transitionas its starting point, Baldry shows that, when examining the semiotic andstructural units that make up a video, a multimodal concordancer far out-strips multimodal transcription in the quest for typical patterns.Kay O'Halloran further explores the use of computer technology forthe semiotic analysis of dynamic images in 'Visual semiosis in film'. A sys-temic-functional model which incorporates the visual imagery and thesoundtrack for the analysis of film is introduced. Inspiredby O'Toole's1999 representation of systemic choices in paintings in the interactive4 INTRODUCTIONCD-ROM Engaging with Art., O'Halloran uses video-editing software AdobePremiere 6.0 to discuss the analysis of the temporal unfolding of semioticchoices in the visual images for two short extracts from Roman Polanski's1974 film Chinatown. While film narrative involves staged and directedbehaviour to achieve particular effects, the analysis of film is at least a firststep to understanding semiosis in everyday life. The analysis demonstratesthe difficulty of capturing and interpreting the complexity of dynamicsemiotic activity.Attention turns to hypertext in Arthur Kok's 'Multisemiotic mediation inhypertext'. In this paper, Kok explores how hypertext represents reality andengages the user, and how instantiations of different semiotic resources arearranged and co-deployed for this purpose. This paper formulates a workingdefinition and a theoretical model of hypertext which contains differentorders of abstraction. As with many papers in this collection, the semioticanalysis is employed through extending previously developed systemic-functional frameworks Halliday, 1994; Kress and van Leeuwen, 1996;O'Toole, 1994. Via an examination of the semiotic choices made inSingapore's Ministry of Education MOE homepage, this analysis seeks tounderstand how the objectives of an institution become translated, trans-mitted and received through the hypertext medium. In the process, anaccount of the highly elusive process of intersemiosis, the interaction ofmeanings across different semiotic instantiations, is given.In Part III on print media, in the first paper, 'The construal of ideationalmeaning in print advertisements', Cheong Yin Yuen proposes a genericstructure potential for print advertisements which incorporates visual andverbal components. Cheong also investigates lexicogrammatical strategiesfor the expansion of ideational meaning which occur through the inter-action of the linguistic text and visual images. Through the analysis of fiveadvertisements, Cheong develops a new vocabulary to discuss the strategieswhich account for semantic expansions of ideational meaning in these texts;namely, the Bi-directional Investment of Meaning, Contextual Propensity,Interpretative Space, Semantic Effervescence and Visual Metaphor.Moving to the field of education, Guo Libo investigates the multi-semioticnature of introductory biology textbooks in 'Multimodality in a biologytextbook'. These books invariably contain words and visual images: forexample, diagrams, photographs, and mathematical and statistical graphs.Drawing upon the work of sociological studies of biology texts and followingO'Toole 1994, Lemke 1998 and O'Halloran 1999b, this paper proposessocial semiotic frameworks for the analysis of schematic drawings and math-ematical or statistical graphs in biology. The frameworks are used to analysehow the various semiotic resources interact with each other to make meaningin selected pages from the biology textbook Essential Cell Biology Alberts et al.,1998. The article concludes by reiterating Johns's 1998: 194 claim that inteaching English for Academic Purposes to science and engineering stu-dents, due attention must be given to the visual as well as the linguisticmeaning in what is termed Visual/Textual interactivity' ibid.: 186.。
Extensions-of-the-Spalart-Allmaras-turbulence-model-to-account-for-wall-roughness

Extensions of the Spalart–Allmaras turbulence model to accountfor wall roughnessB.Aupoixa,*,P.R.SpalartbaONERA/DMAE Centre d Õ E tudes et de Recherches de Toulouse,B.P.4025,2,Avenue E douard Belin,31055Toulouse Cedex 4,France bBoeing Commercial Airplanes,P.O.Box 3707,Seattle,WA 98124,USAReceived 23November 2002;accepted 15March 2003AbstractThis paper describes extensions of the Spalart–Allmaras model to surface roughness,developed independently by Boeing and ONERA.They are rather simple and numerically benign,yield similar predictions,and are in fair agreement with experiments.They do not provide a description of the flow near the roughness elements,but rely instead on the ‘‘equivalent sand grain’’approach.In that sense,they are not self-contained.The uncertain accuracy of the separate correlations,such as Dirling Õs,needed to determine the sand grain size presents a challenge,as always.The roughness height must be much smaller than the boundary layer thickness,but the full range of roughness Reynolds number is covered.Some test cases reveal an incompatibility between the predicted effect of roughness on heat transfer and on skin friction.i.e.if the sand grain size is adjusted for skin friction,the heat transfer is too high.Ó2003Elsevier Science Inc.All rights reserved.Keywords:Boundary layer;Equivalent sand grain;Heat transfer;Pressure gradient;Skin friction;Turbulence modelling;Wall roughness1.Extension of the Spalart–Allmaras model 1.1.Basic Spalart–Allmaras modelThe Spalart–Allmaras (S–A)turbulence model solvesonly one transport equation for the quantity ~m,which is equivalent to the eddy viscosity m t far from walls.The transport equation has been constructed empirically to reproduce flows of increasing complexity.The transport equation,neglecting transition terms,reads (Spalart and Allmaras,1994)D ~m D t ¼c b 1e S ~mÀc w 1f w ~md!2þ1r ½div ð½~m þm grad ~m Þþc b 2grad ~mÁgrad ~m ð1Þwhere d is the distance to the nearest wall.The model has been tuned so that,close to solid surfaces but out-side the viscous region,it fits the logarithmic region,i.e.~m¼u s j d ;e S ¼u sj dð2Þwhere u s is the friction velocity based upon the wall friction s w (u s ¼ffiffiffiffiffiffiffiffiffiffis w =q p )and j the von K a rm a n con-stant.The turbulent viscosity m t is linked to the trans-ported variable ~mby m t ¼f v 1~m ;f v 1¼v 3v 3þc 3v 1;v ¼~mm ð3Þand e S is linked to the vorticity S (which reduces to j o u =o y j in thin shear flows),bye S ¼S þ~m j 2d2f v 2;f v 2¼1Àv 1þv f v 1ð4ÞFinally,f w is a function of the ratio r ~m =ðe S j 2d 2Þ,andboth equal unity in the log layer.Eq.(1)is in balance provided c w 1¼c b 1=j 2þð1þc b 2Þ=r .1.2.Modelling roughness effectsIt is assumed that the roughness-element size in any direction is small compared with the boundary layer thickness so that,above the roughnesses,the flow is averaged over numerous roughness elements the exact location of which is not accounted for.Two such ‘‘macroscopic’’strategies can then be used to account*Corresponding author.Tel.:+33-5-6225-2804;fax:+33-5-6225-2583.E-mail address:bertrand.aupoix@onecert.fr (B.Aupoix).0142-727X/03/$-see front matter Ó2003Elsevier Science Inc.All rights reserved.doi:10.1016/S0142-727X(03)00043-2International Journal of Heat and Fluid Flow 24(2003)454–462/locate/ijhfffor wall roughness in Navier–Stokes computations, without solving theflow equations around each rough-ness element(see e.g.Patel(1998)).In both strategies, the boundary of the calculation domain is smooth,and the velocity boundary condition is zero.•The‘‘discrete element approach’’accounts for the roughness by extra terms in theflow equations which represent theflow blockage due to the roughnesses and the drag and heatflux on roughness elements (see,e.g.Coleman et al.(1983)or Aupoix(1994)for the derivation of the equations).This approach re-quires drastic changes in theflow equations and has rarely been used in practical applications.•The‘‘equivalent sand grain approachÕlinks the real roughness to an idealized roughness,with reference to NikuradseÕs experiments(1933),the height of the equivalent sand grain being deduced from the real roughness shape with the help of empirical correla-tions,usually the correlation proposed by Dirling (1973)and Grabow and White(1975).The roughness effect is mimicked by increasing the turbulent eddy viscosity in the wall region to obtain higher skin fric-tion and wall heatflux levels.Here again,two kinds of model can be considered:Models in which the eddy viscosity is null at the boundary.They can be interpreted as models inwhich the virtual‘‘wall’’corresponds to the bot-tom of the roughnesses.The roughness correctionthen mainly acts through a reduction of the turbu-lence damping in the wall region.Models in which the eddy viscosity isfinite at the boundary.They can be interpreted as models inwhich the virtual wall is located part-way up theroughnesses.Unpublished studies at ONERA haveshown that this approach better accounts for small roughnesses.The increase in skin friction due to wall roughness can be directly related to changes in the velocity profiles, as will be shown in Fig.9where the profile is plotted in wall variables i.e.the normalized velocity uþ¼u=u s versus the normalized wall distance yþ¼yu s=m.For high enough Reynolds numbers and at heights much larger than the roughness height,the logarithmic region and the outer layer or‘‘wake’’are simply shifted compared to the smooth-wall case.Accordingly,the roughness modifications of the models vanish in those regions.It must be remembered that the edge value of the nor-malized velocity isuþe¼u es¼ffiffiffiffiffi2fsð5Þso that,tofirst order,as the wake shape is unaffected by roughness,the increase in the skin friction coefficient C f is directly related to the downward shift D uþof the profile.Conversely,the effect of riblets would be an upward shift and a reduction of C f(see e.g.Baron and Quadrio(1993)).Nikuradse(1933)provided relations between this shift D uþand the normalized roughness heighthþs¼h s u s=m for the specific sand grain roughnesses of various heights h s he investigated.Once the shape of the sand or other roughness element is set,the classical analysis of the turbulent boundary layer leads directly to such a D uþðhþsÞrelationship.As a result,good predictions can be achieved only if the shift D uþof the velocity profile is reproduced for anynormalized sand grain roughness height hþsand the equivalent sand grain roughness h s is correctly estimated from the true shape of the considered rough surface. This estimate is far from trivial,and note that for most types of‘‘real-life’’roughness,the sand grain size h s is several times larger than the depth of thegrooves B.Aupoix,P.R.Spalart/Int.J.Heat and Fluid Flow24(2003)454–462455or other irregularities that have the same effect on the velocity profile.Ignoring this would lead to a severe under-estimate of roughness effect in some cases.1.3.Boeing extensionThis extension(Spalart,2000)was designed in the same spirit as the original model and to preserve its behaviour in the wall region(Eq.(2))but non-zero values of~m and m t are now expected at the wall to mimic roughness effects.For that,the wall condition~m¼0is replaced byo~m o n ¼~mdð6Þwhere n is along the wall normal and the distance d has to be increased.The simplest way is to impose an offset d¼d minþd0where d min is the distance to the wall and d0ðh sÞa length that will be adjusted.It will be a simple linear relationship,and the viscous functions of the model will be calibrated in thefinite-Reynolds-number r e gime.For very rough surfaces,in the fully rough regime(hþs >70),Nikuradse has shown that the velocity pro-files,in the logarithmic region,obeyuþ¼1jlnyh sþ8:5ð7ÞThe molecular viscosity m does not appear.As the roughness effect is strong,the eddy viscosity should be large compared to the gas viscosity even at the wall,and m t¼~m.Therefore,the momentum equation reduces tou2 s ¼m to uo y¼u s j do uo yð8Þthe solution of which readsuþ¼1j½lnðyþd0ÞÀlnðd0Þ ð9ÞIdentification of these two velocity profile expressions yieldsd0¼expðÀ8:5jÞh s%0:03h sð10ÞTo achieve good predictions for smaller roughnesses, the f v1function in Eq.(3)is altered by modifying v asv¼~mmþc R1h sd;c R1¼0:5ð11ÞThis definition and value of c R1give a dependence ofD uþon hþs which is close to that given by Schlichting(1979)after Nikuradse.The balance of the transport equation imposes that all terms have the same behaviour with respect to d as for smooth surfaces,so that the definition of e S is unchangede S¼Sþ~m22f v2;f v2¼1À~mv1ð12ÞRegrettably,there is a misprint in the expression of f v2inSpalart(2000).1.4.ONERA extensionAs previous unpublished ONERA studies have fa-vored models using a non-zero value of the wall turbu-lent viscosity,especially for intermediate roughnessheights,it was decided to impose such a value for~m tosimulate wall roughness effects.The required wall value was determined by solvingthe one-dimensional problem in the wall region.Ne-glecting advection,Eq.(1)reads,in wall variables,i.e.making terms dimensionless with the viscosity m and thefriction velocity u s0¼c b1~Sþ~mþÀc w1f w~mþdþ!2þ1roo yþ~mþo~mþo yþ!24þc b2o~mþo yþ!235ð13Þwhile the momentum equation reduces too uþo yþÀh u0v0iþ¼ð1þmþtÞo uþo yþ¼1ð14ÞAt the wall,the value of~mþis imposed.The otherboundary condition is imposed far in the logarithmicregion where Eq.(14)reduces to~mþ¼mþt¼j yþÀ1.Eq.(13)is solved using a pseudo-unsteady approach.Thevelocity gradient which appears through e S is deducedfrom Eq.(14).Once a solution is obtained for~m and e S,the velocity profile can be deduced by simple integra-tion,and the shift D uþis determined.It turned out that even imposing very large wallvalues for~mþyielded small values of the velocity shift asthe sink term)c w1f wð~m=dÞ2in the transport equationbecame large and suppressed the effect of the imposedwall condition.An offset in the wall distance d has to beintroduced.To be consistent with the behaviour oversmooth walls,the distance d is expressed asdþ¼dþminþ~mþwð15Þwhere~m w is the imposed wall value for~m.This newboundary condition preserves the solution~mþ¼j dþð16Þwhich is still the solution of Eqs.(13)and(14).There-fore,the solutions over smooth and rough walls arelinked by½~mþðyþÞrough¼~mþyþ"þ~mþwj!#smoothð17Þand the same relation holds for the velocity profile sothat the velocity shift reads456 B.Aupoix,P.R.Spalart/Int.J.Heat and Fluid Flow24(2003)454–462D uþ¼uþ~mþwj !"#smoothð18ÞThis allows to get analytical relationships between the wall eddy viscosity and the equivalent sand grainroughness for mildly rough surfaces~mþw =j<3ÀÁand forvery rough surfaces~mþw =j>50ÀÁ.These relations are used to build thefinal model form:~mþw ¼ð0:377ln hþsÀ0:447ÞexpÀhþs70þ1:25710À2hþs1ÀexpÀhþs70!þmax0;loghþs10min1;1:36expÀhþs250;25expÀhþs100ð19Þparison of the two extensionsThe Boeing model only refers to the sand grain roughness height,whereas the ONERA model also needs the friction velocity.This leads to different be-haviours close to two-dimensional separation where the friction velocity tends to zero.The Boeing model then predicts stronger effects of the wall roughness.It must be pointed out that the ONERA model leads to no nu-merical problem at separation:u s is null,and so is hþs and hence~m w and d0.Both extensions change the wall boundary condition, either imposing the wall value or providing a mixed condition.In both cases,the wall distance d is modified so that the model is non-local:the information about the offset d0has to be known,i.e.eachfield point has to be related to a point on the surface.This represents a slight but unfortunate deviation from the‘‘charter’’of the S–A model.The Boeing change of d is simpler in that it only depends upon the roughness height and remains the same during computational iterations.Finally,the Boeing model requires changing the expression of v in the damping function f v1.2.ValidationBoth extensions have been implemented in the ON-ERA two-dimensional boundary layer code CLI C2and compared to other roughness models such as mixing length model(van Driest,1956;Rotta,1962;Krogstad, 1991),k–e models(Blanchard,1977)or k–x model (Wilcox,1988)for various experiments.The results of other models,which are either irrelevant or very close to the present ones,are not given here for the sake of clarity of thefigures.Only a selection of pertinent test-cases is reported.In allfigures,the solid line corresponds to the prediction of the S–A model over a smooth-wall, to highlight roughness effects.Unless otherwise specified,the equivalent sand grain roughness is deduced from DirlingÕs correlation which links the sand grain roughness h s to the mean roughness height h ash¼a h s a¼60:95KÀ3:78if K<4:9150:0072K1:9if K>4:915(K¼LhA sA p4=3ð20Þwhere•h is the mean roughness height,•L is the mean distance between roughness elements,i.e.if there are N roughness elements on an area S,L¼ffiffiffiffiffiffiffiffiffiS=Np,•A p is the surface of the roughness projected on a plane normal to theflow direction,•A s is the wetted surface of the roughness directly ex-posed to the upstreamflow.Initial profiles are generated automatically by the code from the prescribed value of the momentum thickness,assuming local self-similarity and using a mixing length model which accounts for wall roughness. The quantity~m is then deduced from the eddy viscosity.2.1.Blanchard’s experimentsBlanchard(1977)conducted experiments over various surfaces,including sand grain paper of various heights and wire meshes.We present predictions for a sand grain paper the average height of which is0.425mm. Blanchard estimated that the equivalent sand grain roughness height was twice the height of his roughness. This is not fully consistent with the equivalent sand grain roughness which can be deduced from DirlingÕs correlation and the simplified surface representation Blanchard proposed using cones,but he pointed to the large scatter in the correlation.Thefirst case corresponds to a zero pressure gradient flow,with an external velocity of45m sÀ1.This gives anormalized equivalent sand grain roughness height hþs about150,i.e.a fully rough regime.Both models predict a skin friction evolution in fair agreement with experi-ments as shown in Fig.1.The Boeing extension gives slightly higher predictions.For a rougher surface,ve-locity profiles predicted by both extensions are indis-tinguishable and in fair agreement with experiments. Recall that the models were not calibrated on thisflow.Fig.2shows predictions for a positive pressure gra-dientflow.As the pressure gradient is moderate,the friction velocity does not decrease much,and normalizedB.Aupoix,P.R.Spalart/Int.J.Heat and Fluid Flow24(2003)454–462457equivalent sand grain roughness height hþs remains about150.The agreement with experiment remains good,and the discrepancy between the models is smaller.2.2.Acharya et al.experimentsAcharya et al.(1986)conducted experiments on sur-faces specifically machined to reproduce aged turbine blade surfaces.Two surfaces,named SRS1and SRS2 for‘‘simulated rough surface’’have been considered,for a constant external velocity of19m sÀ1.Equivalent sand grain roughness heights have been evaluated from Dir-lingÕs correlation and surface statistics given in TaradaÕs thesis(1987).Surface SRS1gives a normalized equivalent sand grain roughness height hþs about25,i.e.a transitionally rough regime.Fig.3shows that both models predict the skin friction fairly well,the ONERA model giving higher values and therefore better agreement.Surface SRS2gives a normalized equivalent sand grain roughness height hþsabout70,i.e.the lower limit of the fully rough regime.Fig.4shows that both models are in excellent agreement with experiments.2.3.MSU experimentsMany experiments over rough surfaces have been performed at Mississippi State University(MSU).Hosni et al.(1993,1991)investigated boundary layers over spheres,hemispheres and cones arranged in staggered rows in a low-speed wind tunnel designed to perform heat transfer measurements.Skin friction was deduced458 B.Aupoix,P.R.Spalart/Int.J.Heat and Fluid Flow24(2003)454–462from the Reynolds stress hÀu0v0i above the roughnesses, corrected via a momentum balance around the rough-nesses.The data were in fair agreement with the skin friction estimate from the von K a rm a n equation.Heat fluxes were deduced from an energy balance for each heated wall plate,accounting for losses by conduction and radiation.Only results for hemispheres,1.27mm in diameter, will be presented here.The case of a spacing-over-height ratio of ten,i.e.for a weakly rough surface,is not pre-sented here.All test cases are for zero pressure gradient flows.The equivalent sand grain height h s is determined from DirlingÕs correlation.Thefirst surface is covered with hemispheres with a spacing of twice their height.For an external velocity of 12m sÀ1,the normalized equivalent sand grain rough-ness height hþsis about45,i.e.a transitionally roughregime.Both extensions under-predict both the skin friction and the Stanton number,as shown in Figs.5 and6.Other roughness models yield similar predictions. Here again,the ONERA extension gives slightly higher and better levels than the Boeing one.When the velocity is increased to58m sÀ1,the nor-malized equivalent sand grain roughness height hþsis about220,i.e.a fully rough regime.Then,the agreement between predictions and measurements is excellent as shown in Figs.7and8.The velocity profiles in wall variables are plotted in Fig.9.The shift D uþof the logarithmic region and of the wake is about ten wall units.Both models give similar profiles,except very close to the wall where the notion of mean velocity profile makes limited sense.B.Aupoix,P.R.Spalart/Int.J.Heat and Fluid Flow24(2003)454–462459Fig.10illustrates the increase of the quantity ~min the wall region.The two models take somewhat different values at the wall.In the logarithmic and wake region,they give similar eddy viscosity levels,reaching more than twice the level on a smooth surface.The eddy viscosity is the product of a turbulence velocity scale,which is proportional to the friction velocity u s ,and a turbulence length scale which is linked,in the outer re-gion,to the boundary layer thickness.Therefore the eddy viscosity increase is due to both increases of the friction level and of the boundary layer thickness.The second surface is covered with hemispheres with a spacing of four times their height.For an external velocity of 12m s À1,the normalized equivalent sand grain roughness height h þs is about 10,i.e.a transition-ally rough regime.Both extensions give identical resultsbut under-predict both the skin friction and the Stantonnumber,as shown in Figs.11and 12.Other roughness models yield similar predictions.When the external velocity is increased to 58m s À1,the normalized equivalent sand grain roughness height h þs is about 50,i.e.a transitionally rough regime similar to the first MSU case,but for a higher range of values of the Reynolds number R h based upon the bound-ary layer momentum thickness.As for the first MSU case,the skin friction is under-estimated while the Stanton number is fairly well reproduced (Figs.13and 14).Both extensions give similar predictions,whatever the roughness regime.Which extension gives a slightly higher skin friction depends upon thenormalized0.00.51.01.52.02.5X (M)*10-3C f /2Cf MSU Smooth ONERA BoeingFig.11.Skin friction predictions––MSU experiment––hemispheres with spacing/height ratio of four––U ¼12m s À1.0.00.51.01.52.02.5X (m)*10-3S tSt MSU Smooth ONERABoeingFig.12.Stanton number predictions––MSU experiment––hemispheres with spacing/height ratio of four––U ¼12m s À1.460 B.Aupoix,P.R.Spalart /Int.J.Heat and Fluid Flow 24(2003)454–462equivalent sand grain roughness height h þs .The predic-tions are comparable to those of the best tested rough-ness models,again showing that having a single equation is not a serious obstacle to useful modelling.As regards the MSU experiments,for high values of h þs ,predictions are in good agreement with experiments while roughness effects are under-estimated for the same surfaces in the transitionally rough regime.However,good predictions are achieved in the transitionally rough regime for the Acharya et al.experiments.Either the relation D u þðh þs Þproposed by Nikuradse and which has been used to calibrate models is failing and the good predictions in the transitionally rough regime are inci-dental,or a given roughness does not correspond always to the same equivalent sand grain roughness height,which means that the correlations are not accurate and complete enough.A closer inspection of the predictions reveals that the heat transfer increase due to roughnesses is overesti-mated compared to the skin friction increase.A striking example is the last test-case for which the skin friction is under-estimated while the Stanton number is fairly well predicted.This is a well-known drawback of the equivalent sand grain approach as the thermal and dy-namical problems are solved similarly,the same increase being applied to the turbulent viscosity and conductivity.Assuming a linear relation between the velocity and total enthalpy profiles,the analogy factor s reads:s ¼St C f =2/ðk þk t Þo h iðm þm t Þo uo y/ðk þk t Þðm þm t Þ/1P mð21Þwhere P m is a mixed Prandtl number which increases from the gas Prandtl number (0.72)for smooth surfaces to the turbulent Prandtl number (0.9)for fully rough surfaces.Figs.15and 16show that,although there isX (M)01234*10-3C f / 2Cf MSU Smooth ONERABoeingFig.13.Skin friction predictions––MSU experiment––hemispheres with spacing/height ratio of four––U ¼58m s À1.B.Aupoix,P.R.Spalart /Int.J.Heat and Fluid Flow 24(2003)454–462461some scatter in the data,the decrease of the analogy factor is under-predicted by the models,compared with experiments,when the surface becomes rougher.This is consistent with Dipprey and SaberskyÕs results(1963) and the idea that the skin friction increase is mainly due to pressure drag on the roughnesses while the heat-transfer increase is a viscous phenomenon and is more closely linked to the wetted surface increase.Therefore, the Reynolds analogy no longer holds for rough sur-faces,while the modelling implemented here still uses it. Corrections based on functions of d=h s may be devised in the future.3.ConclusionTwo extensions of the S–A turbulence model have been derived.Both assume a non-zero-eddy viscosity at the wall and change the definition of the distance d,so that the model becomes non-local.The Boeing extension only uses the roughness height while the ONERA also refers to the friction velocity.The modifications are ra-ther minor.The extensions could be used instead of the original S–A f t1term to trip boundary layers;a rough band would be placed along the transition line.However there is no decisive advantage over the f t1form and the f t2term would still be needed.Tests on a variety of two-dimensional experiments show that these extensions give similar predictions,in fair agreement with other roughness models and,gen-erally,with experiments.No test is available close en-ough to separation to differentiate the models for low skin friction levels.However,comparisons raise doubts about the universality of the equivalent sand grain size, which appears to depend upon theflow regime for a given surface.In other words,for a given roughness shape,the optimal sand grain roughness height h s is not simply proportional to the physical size of the rough-ness.While h was expected to depend onflow direction, for instance with grooves,a dependence on the friction velocity is a further disappointment and inconvenience. Moreover,the over-prediction of roughness effects on heat transfer compared with the effects on skin friction, using the equivalent sand grain approach and a uniform turbulent Prandtl number,is unfortunately repeatable. AcknowledgementsThe ONERA work was divided between the ARCAE research program funded by the French Ministry of Defence and the research project‘‘Unsteady Transi-tional Flows in Axial Turbomachines’’funded by the European Commission under contract number G4RD-CT-2001-00628.It is thefirst authorÕs pleasure to ac-knowledge P.Baubias and G.Fontaine for their con-tributions to this work.ReferencesAcharya,M.,Bornstein,J.,Escudier,M.P.,1986.Turbulent boundary layers on rough surfaces.Experiments in Fluids4(1),33–47. Aupoix,B.,1994.Modelling of boundary layers over rough surfaces.In:Benzi,R.(Ed.),Advances in Turbulence V,Sienna.Fifth European Turbulence Conference.Kluwer,pp.16–20.Baron, A.,Quadrio,M.,1993.Some preliminary results on the influence of riblets on the structure of a turbulent boundary layer.International Journal of Heat and Fluid Flow14(3),223–230. Blanchard, A.,1977.Analyse Exp e rimentale et Th e orique de la Structure de la Turbulence dÕune Couche Limite sur Paroi Rugueuse.Ph.D.thesis,Universit e de Poitiers U.E.R.-E.N.S.M.A. Coleman,H.W.,Hodge, B.K.,Taylor,R.P.,1983.Generalized roughness effects on turbulent boundary layer heat transfer––a discrete element predictive approach for turbulentflow over rough surfaces.Air Force Armament Laboratory AFATL-TR-83-90, Mississippi State University.Dipprey,D.F.,Sabersky,R.H.,1963.Heat and momentum transfer in smooth and rough tubes at various Prandtl numbers.International Journal of Heat and Mass Transfer6,329–353.Dirling Jr.,R.B.,1973.A method for computing rough wall heat transfer rates on reentry nosetips.AIAA Paper73-763,AIAA8th Thermophysics Conference,Palm Springs,California.Grabow,R.M.,White, C.O.,1975.Surface roughness effects on nosetip ablation characteristics.AIAA Journal13(5),605–609. Hosni,M.H.,Coleman,H.W.,Taylor,R.P.,1991.Measurements and calculations of rough-wall heat transfer in the turbulent boundary layer.International Journal of Heat and Mass Transfer34(4/5), 1067–1082.Hosni,M.H.,Coleman,H.W.,Gardner,J.W.,Taylor,R.P.,1993.Roughness element shape effects on heat transfer and skin friction in rough-wall turbulent boundary layer.International Journal of Heat and Mass Transfer36(1),147–153.Krogstad,P. A.,1991.Modification of the van Driest damping function to include the effects of surface roughness.AIAA Journal 296,888–894.Nikuradse,J.,1933.Str€o mungsgesetze in rauhen Rohren.Tech.Rept 361,VDI-Forschungsheft.Patel,V.C.,1998.Perspective:flow at high Reynolds number and over rough surfaces––Achilles heel of CFD.Journal of Fluids Engi-neering120,434–444.Rotta,J.,1962.Turbulent boundary layers in incompressibleflows.Progress in Aeronautical Sciences2,73–82.Schlichting,H.,1979.Boundary-Layer Theory,seventh ed.McGraw-Hill,New York.Spalart,P.,2000.Trends in turbulence treatments.AIAA Paper2000-2306,Fluids2000,Denver.Spalart,P.R.,Allmaras,S.R.,1994.A one-equation turbulence model for aerodynamicfl Recherche A e rospatiale1,5–21. Tarada, F.H.A.,1987.Heat Transfer to Rough Turbine Blading.Ph.D.thesis,University of Sussex.van Driest,E.R.,1956.On turbulentflow near a wall.Journal of Aeronautical Sciences23(11),1007–1011.Wilcox,D.C.,1988.Reassessment of the scale-determining equation for advanced turbulence models.AIAA Journal26(11),1299–1310.462 B.Aupoix,P.R.Spalart/Int.J.Heat and Fluid Flow24(2003)454–462。
From Data Mining to Knowledge Discovery in Databases
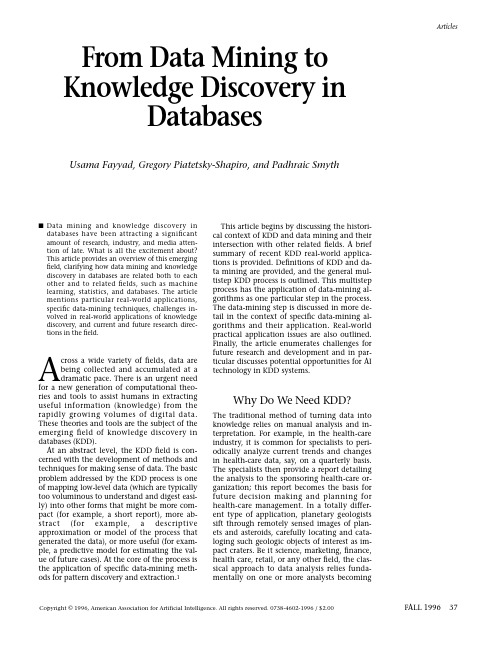
s Data mining and knowledge discovery in databases have been attracting a significant amount of research, industry, and media atten-tion of late. What is all the excitement about?This article provides an overview of this emerging field, clarifying how data mining and knowledge discovery in databases are related both to each other and to related fields, such as machine learning, statistics, and databases. The article mentions particular real-world applications, specific data-mining techniques, challenges in-volved in real-world applications of knowledge discovery, and current and future research direc-tions in the field.A cross a wide variety of fields, data arebeing collected and accumulated at adramatic pace. There is an urgent need for a new generation of computational theo-ries and tools to assist humans in extracting useful information (knowledge) from the rapidly growing volumes of digital data. These theories and tools are the subject of the emerging field of knowledge discovery in databases (KDD).At an abstract level, the KDD field is con-cerned with the development of methods and techniques for making sense of data. The basic problem addressed by the KDD process is one of mapping low-level data (which are typically too voluminous to understand and digest easi-ly) into other forms that might be more com-pact (for example, a short report), more ab-stract (for example, a descriptive approximation or model of the process that generated the data), or more useful (for exam-ple, a predictive model for estimating the val-ue of future cases). At the core of the process is the application of specific data-mining meth-ods for pattern discovery and extraction.1This article begins by discussing the histori-cal context of KDD and data mining and theirintersection with other related fields. A briefsummary of recent KDD real-world applica-tions is provided. Definitions of KDD and da-ta mining are provided, and the general mul-tistep KDD process is outlined. This multistepprocess has the application of data-mining al-gorithms as one particular step in the process.The data-mining step is discussed in more de-tail in the context of specific data-mining al-gorithms and their application. Real-worldpractical application issues are also outlined.Finally, the article enumerates challenges forfuture research and development and in par-ticular discusses potential opportunities for AItechnology in KDD systems.Why Do We Need KDD?The traditional method of turning data intoknowledge relies on manual analysis and in-terpretation. For example, in the health-careindustry, it is common for specialists to peri-odically analyze current trends and changesin health-care data, say, on a quarterly basis.The specialists then provide a report detailingthe analysis to the sponsoring health-care or-ganization; this report becomes the basis forfuture decision making and planning forhealth-care management. In a totally differ-ent type of application, planetary geologistssift through remotely sensed images of plan-ets and asteroids, carefully locating and cata-loging such geologic objects of interest as im-pact craters. Be it science, marketing, finance,health care, retail, or any other field, the clas-sical approach to data analysis relies funda-mentally on one or more analysts becomingArticlesFALL 1996 37From Data Mining to Knowledge Discovery inDatabasesUsama Fayyad, Gregory Piatetsky-Shapiro, and Padhraic Smyth Copyright © 1996, American Association for Artificial Intelligence. All rights reserved. 0738-4602-1996 / $2.00areas is astronomy. Here, a notable success was achieved by SKICAT ,a system used by as-tronomers to perform image analysis,classification, and cataloging of sky objects from sky-survey images (Fayyad, Djorgovski,and Weir 1996). In its first application, the system was used to process the 3 terabytes (1012bytes) of image data resulting from the Second Palomar Observatory Sky Survey,where it is estimated that on the order of 109sky objects are detectable. SKICAT can outper-form humans and traditional computational techniques in classifying faint sky objects. See Fayyad, Haussler, and Stolorz (1996) for a sur-vey of scientific applications.In business, main KDD application areas includes marketing, finance (especially in-vestment), fraud detection, manufacturing,telecommunications, and Internet agents.Marketing:In marketing, the primary ap-plication is database marketing systems,which analyze customer databases to identify different customer groups and forecast their behavior. Business Week (Berry 1994) estimat-ed that over half of all retailers are using or planning to use database marketing, and those who do use it have good results; for ex-ample, American Express reports a 10- to 15-percent increase in credit-card use. Another notable marketing application is market-bas-ket analysis (Agrawal et al. 1996) systems,which find patterns such as, “If customer bought X, he/she is also likely to buy Y and Z.” Such patterns are valuable to retailers.Investment: Numerous companies use da-ta mining for investment, but most do not describe their systems. One exception is LBS Capital Management. Its system uses expert systems, neural nets, and genetic algorithms to manage portfolios totaling $600 million;since its start in 1993, the system has outper-formed the broad stock market (Hall, Mani,and Barr 1996).Fraud detection: HNC Falcon and Nestor PRISM systems are used for monitoring credit-card fraud, watching over millions of ac-counts. The FAIS system (Senator et al. 1995),from the U.S. Treasury Financial Crimes En-forcement Network, is used to identify finan-cial transactions that might indicate money-laundering activity.Manufacturing: The CASSIOPEE trou-bleshooting system, developed as part of a joint venture between General Electric and SNECMA, was applied by three major Euro-pean airlines to diagnose and predict prob-lems for the Boeing 737. To derive families of faults, clustering methods are used. CASSIOPEE received the European first prize for innova-intimately familiar with the data and serving as an interface between the data and the users and products.For these (and many other) applications,this form of manual probing of a data set is slow, expensive, and highly subjective. In fact, as data volumes grow dramatically, this type of manual data analysis is becoming completely impractical in many domains.Databases are increasing in size in two ways:(1) the number N of records or objects in the database and (2) the number d of fields or at-tributes to an object. Databases containing on the order of N = 109objects are becoming in-creasingly common, for example, in the as-tronomical sciences. Similarly, the number of fields d can easily be on the order of 102or even 103, for example, in medical diagnostic applications. Who could be expected to di-gest millions of records, each having tens or hundreds of fields? We believe that this job is certainly not one for humans; hence, analysis work needs to be automated, at least partially.The need to scale up human analysis capa-bilities to handling the large number of bytes that we can collect is both economic and sci-entific. Businesses use data to gain competi-tive advantage, increase efficiency, and pro-vide more valuable services to customers.Data we capture about our environment are the basic evidence we use to build theories and models of the universe we live in. Be-cause computers have enabled humans to gather more data than we can digest, it is on-ly natural to turn to computational tech-niques to help us unearth meaningful pat-terns and structures from the massive volumes of data. Hence, KDD is an attempt to address a problem that the digital informa-tion era made a fact of life for all of us: data overload.Data Mining and Knowledge Discovery in the Real WorldA large degree of the current interest in KDD is the result of the media interest surrounding successful KDD applications, for example, the focus articles within the last two years in Business Week , Newsweek , Byte , PC Week , and other large-circulation periodicals. Unfortu-nately, it is not always easy to separate fact from media hype. Nonetheless, several well-documented examples of successful systems can rightly be referred to as KDD applications and have been deployed in operational use on large-scale real-world problems in science and in business.In science, one of the primary applicationThere is an urgent need for a new generation of computation-al theories and tools toassist humans in extractinguseful information (knowledge)from the rapidly growing volumes ofdigital data.Articles38AI MAGAZINEtive applications (Manago and Auriol 1996).Telecommunications: The telecommuni-cations alarm-sequence analyzer (TASA) wasbuilt in cooperation with a manufacturer oftelecommunications equipment and threetelephone networks (Mannila, Toivonen, andVerkamo 1995). The system uses a novelframework for locating frequently occurringalarm episodes from the alarm stream andpresenting them as rules. Large sets of discov-ered rules can be explored with flexible infor-mation-retrieval tools supporting interactivityand iteration. In this way, TASA offers pruning,grouping, and ordering tools to refine the re-sults of a basic brute-force search for rules.Data cleaning: The MERGE-PURGE systemwas applied to the identification of duplicatewelfare claims (Hernandez and Stolfo 1995).It was used successfully on data from the Wel-fare Department of the State of Washington.In other areas, a well-publicized system isIBM’s ADVANCED SCOUT,a specialized data-min-ing system that helps National Basketball As-sociation (NBA) coaches organize and inter-pret data from NBA games (U.S. News 1995). ADVANCED SCOUT was used by several of the NBA teams in 1996, including the Seattle Su-personics, which reached the NBA finals.Finally, a novel and increasingly importanttype of discovery is one based on the use of in-telligent agents to navigate through an infor-mation-rich environment. Although the ideaof active triggers has long been analyzed in thedatabase field, really successful applications ofthis idea appeared only with the advent of theInternet. These systems ask the user to specifya profile of interest and search for related in-formation among a wide variety of public-do-main and proprietary sources. For example, FIREFLY is a personal music-recommendation agent: It asks a user his/her opinion of several music pieces and then suggests other music that the user might like (<http:// www.ffl/>). CRAYON(/>) allows users to create their own free newspaper (supported by ads); NEWSHOUND(<http://www. /hound/>) from the San Jose Mercury News and FARCAST(</> automatically search information from a wide variety of sources, including newspapers and wire services, and e-mail rele-vant documents directly to the user.These are just a few of the numerous suchsystems that use KDD techniques to automat-ically produce useful information from largemasses of raw data. See Piatetsky-Shapiro etal. (1996) for an overview of issues in devel-oping industrial KDD applications.Data Mining and KDDHistorically, the notion of finding useful pat-terns in data has been given a variety ofnames, including data mining, knowledge ex-traction, information discovery, informationharvesting, data archaeology, and data patternprocessing. The term data mining has mostlybeen used by statisticians, data analysts, andthe management information systems (MIS)communities. It has also gained popularity inthe database field. The phrase knowledge dis-covery in databases was coined at the first KDDworkshop in 1989 (Piatetsky-Shapiro 1991) toemphasize that knowledge is the end productof a data-driven discovery. It has been popular-ized in the AI and machine-learning fields.In our view, KDD refers to the overall pro-cess of discovering useful knowledge from da-ta, and data mining refers to a particular stepin this process. Data mining is the applicationof specific algorithms for extracting patternsfrom data. The distinction between the KDDprocess and the data-mining step (within theprocess) is a central point of this article. Theadditional steps in the KDD process, such asdata preparation, data selection, data cleaning,incorporation of appropriate prior knowledge,and proper interpretation of the results ofmining, are essential to ensure that usefulknowledge is derived from the data. Blind ap-plication of data-mining methods (rightly crit-icized as data dredging in the statistical litera-ture) can be a dangerous activity, easilyleading to the discovery of meaningless andinvalid patterns.The Interdisciplinary Nature of KDDKDD has evolved, and continues to evolve,from the intersection of research fields such asmachine learning, pattern recognition,databases, statistics, AI, knowledge acquisitionfor expert systems, data visualization, andhigh-performance computing. The unifyinggoal is extracting high-level knowledge fromlow-level data in the context of large data sets.The data-mining component of KDD cur-rently relies heavily on known techniquesfrom machine learning, pattern recognition,and statistics to find patterns from data in thedata-mining step of the KDD process. A natu-ral question is, How is KDD different from pat-tern recognition or machine learning (and re-lated fields)? The answer is that these fieldsprovide some of the data-mining methodsthat are used in the data-mining step of theKDD process. KDD focuses on the overall pro-cess of knowledge discovery from data, includ-ing how the data are stored and accessed, howalgorithms can be scaled to massive data setsThe basicproblemaddressed bythe KDDprocess isone ofmappinglow-leveldata intoother formsthat might bemorecompact,moreabstract,or moreuseful.ArticlesFALL 1996 39A driving force behind KDD is the database field (the second D in KDD). Indeed, the problem of effective data manipulation when data cannot fit in the main memory is of fun-damental importance to KDD. Database tech-niques for gaining efficient data access,grouping and ordering operations when ac-cessing data, and optimizing queries consti-tute the basics for scaling algorithms to larger data sets. Most data-mining algorithms from statistics, pattern recognition, and machine learning assume data are in the main memo-ry and pay no attention to how the algorithm breaks down if only limited views of the data are possible.A related field evolving from databases is data warehousing,which refers to the popular business trend of collecting and cleaning transactional data to make them available for online analysis and decision support. Data warehousing helps set the stage for KDD in two important ways: (1) data cleaning and (2)data access.Data cleaning: As organizations are forced to think about a unified logical view of the wide variety of data and databases they pos-sess, they have to address the issues of map-ping data to a single naming convention,uniformly representing and handling missing data, and handling noise and errors when possible.Data access: Uniform and well-defined methods must be created for accessing the da-ta and providing access paths to data that were historically difficult to get to (for exam-ple, stored offline).Once organizations and individuals have solved the problem of how to store and ac-cess their data, the natural next step is the question, What else do we do with all the da-ta? This is where opportunities for KDD natu-rally arise.A popular approach for analysis of data warehouses is called online analytical processing (OLAP), named for a set of principles pro-posed by Codd (1993). OLAP tools focus on providing multidimensional data analysis,which is superior to SQL in computing sum-maries and breakdowns along many dimen-sions. OLAP tools are targeted toward simpli-fying and supporting interactive data analysis,but the goal of KDD tools is to automate as much of the process as possible. Thus, KDD is a step beyond what is currently supported by most standard database systems.Basic DefinitionsKDD is the nontrivial process of identifying valid, novel, potentially useful, and ultimate-and still run efficiently, how results can be in-terpreted and visualized, and how the overall man-machine interaction can usefully be modeled and supported. The KDD process can be viewed as a multidisciplinary activity that encompasses techniques beyond the scope of any one particular discipline such as machine learning. In this context, there are clear opportunities for other fields of AI (be-sides machine learning) to contribute to KDD. KDD places a special emphasis on find-ing understandable patterns that can be inter-preted as useful or interesting knowledge.Thus, for example, neural networks, although a powerful modeling tool, are relatively difficult to understand compared to decision trees. KDD also emphasizes scaling and ro-bustness properties of modeling algorithms for large noisy data sets.Related AI research fields include machine discovery, which targets the discovery of em-pirical laws from observation and experimen-tation (Shrager and Langley 1990) (see Kloes-gen and Zytkow [1996] for a glossary of terms common to KDD and machine discovery),and causal modeling for the inference of causal models from data (Spirtes, Glymour,and Scheines 1993). Statistics in particular has much in common with KDD (see Elder and Pregibon [1996] and Glymour et al.[1996] for a more detailed discussion of this synergy). Knowledge discovery from data is fundamentally a statistical endeavor. Statistics provides a language and framework for quan-tifying the uncertainty that results when one tries to infer general patterns from a particu-lar sample of an overall population. As men-tioned earlier, the term data mining has had negative connotations in statistics since the 1960s when computer-based data analysis techniques were first introduced. The concern arose because if one searches long enough in any data set (even randomly generated data),one can find patterns that appear to be statis-tically significant but, in fact, are not. Clearly,this issue is of fundamental importance to KDD. Substantial progress has been made in recent years in understanding such issues in statistics. Much of this work is of direct rele-vance to KDD. Thus, data mining is a legiti-mate activity as long as one understands how to do it correctly; data mining carried out poorly (without regard to the statistical as-pects of the problem) is to be avoided. KDD can also be viewed as encompassing a broader view of modeling than statistics. KDD aims to provide tools to automate (to the degree pos-sible) the entire process of data analysis and the statistician’s “art” of hypothesis selection.Data mining is a step in the KDD process that consists of ap-plying data analysis and discovery al-gorithms that produce a par-ticular enu-meration ofpatterns (or models)over the data.Articles40AI MAGAZINEly understandable patterns in data (Fayyad, Piatetsky-Shapiro, and Smyth 1996).Here, data are a set of facts (for example, cases in a database), and pattern is an expres-sion in some language describing a subset of the data or a model applicable to the subset. Hence, in our usage here, extracting a pattern also designates fitting a model to data; find-ing structure from data; or, in general, mak-ing any high-level description of a set of data. The term process implies that KDD comprises many steps, which involve data preparation, search for patterns, knowledge evaluation, and refinement, all repeated in multiple itera-tions. By nontrivial, we mean that some search or inference is involved; that is, it is not a straightforward computation of predefined quantities like computing the av-erage value of a set of numbers.The discovered patterns should be valid on new data with some degree of certainty. We also want patterns to be novel (at least to the system and preferably to the user) and poten-tially useful, that is, lead to some benefit to the user or task. Finally, the patterns should be understandable, if not immediately then after some postprocessing.The previous discussion implies that we can define quantitative measures for evaluating extracted patterns. In many cases, it is possi-ble to define measures of certainty (for exam-ple, estimated prediction accuracy on new data) or utility (for example, gain, perhaps indollars saved because of better predictions orspeedup in response time of a system). No-tions such as novelty and understandabilityare much more subjective. In certain contexts,understandability can be estimated by sim-plicity (for example, the number of bits to de-scribe a pattern). An important notion, calledinterestingness(for example, see Silberschatzand Tuzhilin [1995] and Piatetsky-Shapiro andMatheus [1994]), is usually taken as an overallmeasure of pattern value, combining validity,novelty, usefulness, and simplicity. Interest-ingness functions can be defined explicitly orcan be manifested implicitly through an or-dering placed by the KDD system on the dis-covered patterns or models.Given these notions, we can consider apattern to be knowledge if it exceeds some in-terestingness threshold, which is by nomeans an attempt to define knowledge in thephilosophical or even the popular view. As amatter of fact, knowledge in this definition ispurely user oriented and domain specific andis determined by whatever functions andthresholds the user chooses.Data mining is a step in the KDD processthat consists of applying data analysis anddiscovery algorithms that, under acceptablecomputational efficiency limitations, pro-duce a particular enumeration of patterns (ormodels) over the data. Note that the space ofArticlesFALL 1996 41Figure 1. An Overview of the Steps That Compose the KDD Process.methods, the effective number of variables under consideration can be reduced, or in-variant representations for the data can be found.Fifth is matching the goals of the KDD pro-cess (step 1) to a particular data-mining method. For example, summarization, clas-sification, regression, clustering, and so on,are described later as well as in Fayyad, Piatet-sky-Shapiro, and Smyth (1996).Sixth is exploratory analysis and model and hypothesis selection: choosing the data-mining algorithm(s) and selecting method(s)to be used for searching for data patterns.This process includes deciding which models and parameters might be appropriate (for ex-ample, models of categorical data are differ-ent than models of vectors over the reals) and matching a particular data-mining method with the overall criteria of the KDD process (for example, the end user might be more in-terested in understanding the model than its predictive capabilities).Seventh is data mining: searching for pat-terns of interest in a particular representa-tional form or a set of such representations,including classification rules or trees, regres-sion, and clustering. The user can significant-ly aid the data-mining method by correctly performing the preceding steps.Eighth is interpreting mined patterns, pos-sibly returning to any of steps 1 through 7 for further iteration. This step can also involve visualization of the extracted patterns and models or visualization of the data given the extracted models.Ninth is acting on the discovered knowl-edge: using the knowledge directly, incorpo-rating the knowledge into another system for further action, or simply documenting it and reporting it to interested parties. This process also includes checking for and resolving po-tential conflicts with previously believed (or extracted) knowledge.The KDD process can involve significant iteration and can contain loops between any two steps. The basic flow of steps (al-though not the potential multitude of itera-tions and loops) is illustrated in figure 1.Most previous work on KDD has focused on step 7, the data mining. However, the other steps are as important (and probably more so) for the successful application of KDD in practice. Having defined the basic notions and introduced the KDD process, we now focus on the data-mining component,which has, by far, received the most atten-tion in the literature.patterns is often infinite, and the enumera-tion of patterns involves some form of search in this space. Practical computational constraints place severe limits on the sub-space that can be explored by a data-mining algorithm.The KDD process involves using the database along with any required selection,preprocessing, subsampling, and transforma-tions of it; applying data-mining methods (algorithms) to enumerate patterns from it;and evaluating the products of data mining to identify the subset of the enumerated pat-terns deemed knowledge. The data-mining component of the KDD process is concerned with the algorithmic means by which pat-terns are extracted and enumerated from da-ta. The overall KDD process (figure 1) in-cludes the evaluation and possible interpretation of the mined patterns to de-termine which patterns can be considered new knowledge. The KDD process also in-cludes all the additional steps described in the next section.The notion of an overall user-driven pro-cess is not unique to KDD: analogous propos-als have been put forward both in statistics (Hand 1994) and in machine learning (Brod-ley and Smyth 1996).The KDD ProcessThe KDD process is interactive and iterative,involving numerous steps with many deci-sions made by the user. Brachman and Anand (1996) give a practical view of the KDD pro-cess, emphasizing the interactive nature of the process. Here, we broadly outline some of its basic steps:First is developing an understanding of the application domain and the relevant prior knowledge and identifying the goal of the KDD process from the customer’s viewpoint.Second is creating a target data set: select-ing a data set, or focusing on a subset of vari-ables or data samples, on which discovery is to be performed.Third is data cleaning and preprocessing.Basic operations include removing noise if appropriate, collecting the necessary informa-tion to model or account for noise, deciding on strategies for handling missing data fields,and accounting for time-sequence informa-tion and known changes.Fourth is data reduction and projection:finding useful features to represent the data depending on the goal of the task. With di-mensionality reduction or transformationArticles42AI MAGAZINEThe Data-Mining Stepof the KDD ProcessThe data-mining component of the KDD pro-cess often involves repeated iterative applica-tion of particular data-mining methods. This section presents an overview of the primary goals of data mining, a description of the methods used to address these goals, and a brief description of the data-mining algo-rithms that incorporate these methods.The knowledge discovery goals are defined by the intended use of the system. We can distinguish two types of goals: (1) verification and (2) discovery. With verification,the sys-tem is limited to verifying the user’s hypothe-sis. With discovery,the system autonomously finds new patterns. We further subdivide the discovery goal into prediction,where the sys-tem finds patterns for predicting the future behavior of some entities, and description, where the system finds patterns for presenta-tion to a user in a human-understandableform. In this article, we are primarily con-cerned with discovery-oriented data mining.Data mining involves fitting models to, or determining patterns from, observed data. The fitted models play the role of inferred knowledge: Whether the models reflect useful or interesting knowledge is part of the over-all, interactive KDD process where subjective human judgment is typically required. Two primary mathematical formalisms are used in model fitting: (1) statistical and (2) logical. The statistical approach allows for nondeter-ministic effects in the model, whereas a logi-cal model is purely deterministic. We focus primarily on the statistical approach to data mining, which tends to be the most widely used basis for practical data-mining applica-tions given the typical presence of uncertain-ty in real-world data-generating processes.Most data-mining methods are based on tried and tested techniques from machine learning, pattern recognition, and statistics: classification, clustering, regression, and so on. The array of different algorithms under each of these headings can often be bewilder-ing to both the novice and the experienced data analyst. It should be emphasized that of the many data-mining methods advertised in the literature, there are really only a few fun-damental techniques. The actual underlying model representation being used by a particu-lar method typically comes from a composi-tion of a small number of well-known op-tions: polynomials, splines, kernel and basis functions, threshold-Boolean functions, and so on. Thus, algorithms tend to differ primar-ily in the goodness-of-fit criterion used toevaluate model fit or in the search methodused to find a good fit.In our brief overview of data-mining meth-ods, we try in particular to convey the notionthat most (if not all) methods can be viewedas extensions or hybrids of a few basic tech-niques and principles. We first discuss the pri-mary methods of data mining and then showthat the data- mining methods can be viewedas consisting of three primary algorithmiccomponents: (1) model representation, (2)model evaluation, and (3) search. In the dis-cussion of KDD and data-mining methods,we use a simple example to make some of thenotions more concrete. Figure 2 shows a sim-ple two-dimensional artificial data set consist-ing of 23 cases. Each point on the graph rep-resents a person who has been given a loanby a particular bank at some time in the past.The horizontal axis represents the income ofthe person; the vertical axis represents the to-tal personal debt of the person (mortgage, carpayments, and so on). The data have beenclassified into two classes: (1) the x’s repre-sent persons who have defaulted on theirloans and (2) the o’s represent persons whoseloans are in good status with the bank. Thus,this simple artificial data set could represent ahistorical data set that can contain usefulknowledge from the point of view of thebank making the loans. Note that in actualKDD applications, there are typically manymore dimensions (as many as several hun-dreds) and many more data points (manythousands or even millions).ArticlesFALL 1996 43Figure 2. A Simple Data Set with Two Classes Used for Illustrative Purposes.。
核磁共振光谱NMR光谱
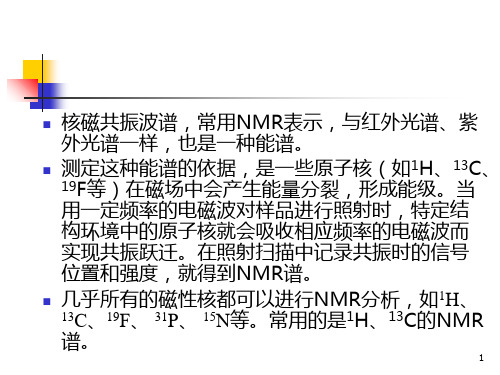
弛豫可分为纵向弛豫和横向弛豫。
32
纵向弛豫:
处于高能级的核将其能量及时转移给周围分子骨架(晶格)
中的其它核,从而使自己返回到低能态的现象。又称自旋
-晶格弛豫。
其半衰期用T1表示
横向弛豫: 当两个相邻的核处于不同能级,但进动频率相同时,高 能级核与低能级核通过自旋状态的交换而实现能量转移 所发生的弛豫现象。又称自旋-自旋弛豫。
N NH i N NL
j
E
h
e kT e kT
通 过 计 算 , 在 常 温 下 , 1H 处 于 B0 为 2.3488T的磁场中,处于低能级的1H 核数目仅比高能级的核数目多出百万 分之十六!
会造成什么后果?
27
随实验进行,低能级核越来越少,最后高、低能级上的 核数目相等--------饱和-----从低到高与从高到低能级的 跃迁的数目相同---体系净吸收为0-----共振信号消失!
问世,NMR开始广泛应用
4
第二阶段 70年代:Fourier Transform的应用
13C-NMR技术(碳骨架) (GC,TLC,HPLC技术的发展) 第三阶段 80年代:Two-dimensional (2D) NMR诞生 (COSY,碳骨架连接顺序,非键原 子间距离,生物大分子结构,……)
5
这个过程称之弛豫过程(Relaxation),即 高能态的核以非辐射的形式放出能量回到 低能态重建Boltzmann分布。
30
两种弛豫过程:
N
h
Relaxation
N+
31
谱线宽度
据Heisenberg测不准原理,激发能量E与体系处于激发态的平均时 间(寿命)成反比,与谱线变宽成正比,即:
综述:热障涂层技术
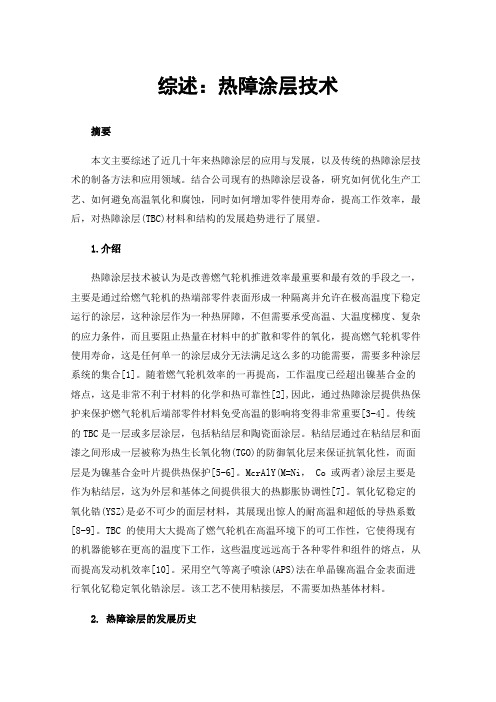
综述:热障涂层技术摘要本文主要综述了近几十年来热障涂层的应用与发展,以及传统的热障涂层技术的制备方法和应用领域。
结合公司现有的热障涂层设备,研究如何优化生产工艺、如何避免高温氧化和腐蚀,同时如何增加零件使用寿命,提高工作效率,最后,对热障涂层(TBC)材料和结构的发展趋势进行了展望。
1.介绍热障涂层技术被认为是改善燃气轮机推进效率最重要和最有效的手段之一,主要是通过给燃气轮机的热端部零件表面形成一种隔离并允许在极高温度下稳定运行的涂层,这种涂层作为一种热屏障,不但需要承受高温、大温度梯度、复杂的应力条件,而且要阻止热量在材料中的扩散和零件的氧化,提高燃气轮机零件使用寿命,这是任何单一的涂层成分无法满足这么多的功能需要,需要多种涂层系统的集合[1]。
随着燃气轮机效率的一再提高,工作温度已经超出镍基合金的熔点,这是非常不利于材料的化学和热可靠性[2],因此,通过热障涂层提供热保护来保护燃气轮机后端部零件材料免受高温的影响将变得非常重要[3-4]。
传统的TBC是一层或多层涂层,包括粘结层和陶瓷面涂层。
粘结层通过在粘结层和面漆之间形成一层被称为热生长氧化物(TGO)的防御氧化层来保证抗氧化性,而面层是为镍基合金叶片提供热保护[5-6]。
McrAlY(M=Ni, Co 或两者)涂层主要是作为粘结层,这为外层和基体之间提供很大的热膨胀协调性[7]。
氧化钇稳定的氧化锆(YSZ)是必不可少的面层材料,其展现出惊人的耐高温和超低的导热系数[8-9]。
TBC 的使用大大提高了燃气轮机在高温环境下的可工作性,它使得现有的机器能够在更高的温度下工作,这些温度远远高于各种零件和组件的熔点,从而提高发动机效率[10]。
采用空气等离子喷涂(APS)法在单晶镍高温合金表面进行氧化钇稳定氧化锆涂层。
该工艺不使用粘接层, 不需要加热基体材料。
2.热障涂层的发展历史在热障涂层的开发之前,我们必须了解热障涂层的发展历史。
其中物理气相沉积法自1980年(Aicro Temescal)发展以来,一直致力于燃气轮机热端部零部件的防护,火焰筒和燃烧室部件都是最初应用的部位,80年代中期EB-PVD技术向航空涡轮发动机的转子叶片和导向叶片上制备热障涂层方向发展(Pratt&Whitney, GE), 并且在同一时期前苏联成功地采用EB-PVD技术在转子叶片上制备出热障涂层,并将该涂层应用在军用飞机上[11]。
Simulation of the release
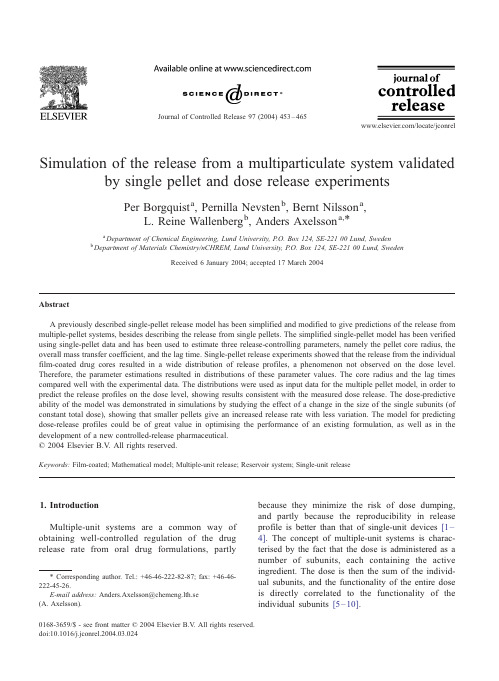
1. Introduction
Multiple-unit systems are a common way of obtaining well-controlled regulation of the drug release rate from oral drug formulations, partly
0168-3659/$ - see front matter D 2004 Elsevier B.V. All rights reserved. doi:10.1016/j.jconrel.2004.03.024
454
P. Borgquist et al. / Journal of Controlled Release 97 (2004) 453–465
Per Borgquista, Pernilla Nevstenb, Bernt Nilssona, L. Reine Wallenbergb, Anders Axelssona,*
a Department of Chemical Engineering, Lund University, P.O. Box 124, SE-221 00 Lund, Sweden b Department of Materials Chemistry/nCHREM, Lund University, P.O. Box 124, SE-221 00 Lund, Sw. Tel.: +46-46-222-82-87; fax: +46-46222-45-26.
Advances in
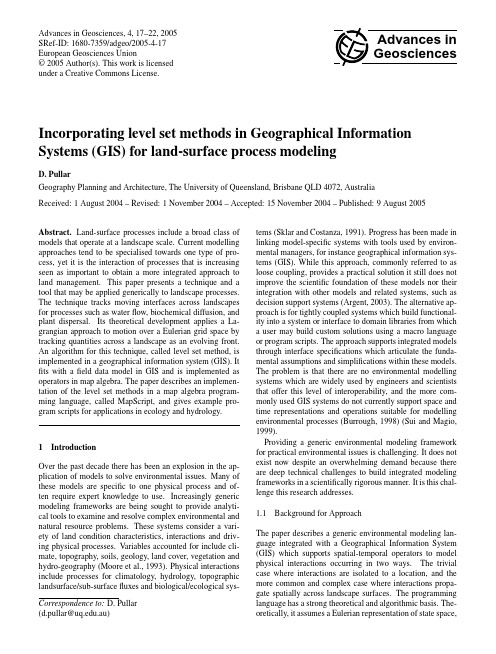
Advances in Geosciences,4,17–22,2005 SRef-ID:1680-7359/adgeo/2005-4-17 European Geosciences Union©2005Author(s).This work is licensed under a Creative CommonsLicense.Advances in GeosciencesIncorporating level set methods in Geographical Information Systems(GIS)for land-surface process modelingD.PullarGeography Planning and Architecture,The University of Queensland,Brisbane QLD4072,Australia Received:1August2004–Revised:1November2004–Accepted:15November2004–Published:9August2005nd-surface processes include a broad class of models that operate at a landscape scale.Current modelling approaches tend to be specialised towards one type of pro-cess,yet it is the interaction of processes that is increasing seen as important to obtain a more integrated approach to land management.This paper presents a technique and a tool that may be applied generically to landscape processes. The technique tracks moving interfaces across landscapes for processes such as waterflow,biochemical diffusion,and plant dispersal.Its theoretical development applies a La-grangian approach to motion over a Eulerian grid space by tracking quantities across a landscape as an evolving front. An algorithm for this technique,called level set method,is implemented in a geographical information system(GIS).It fits with afield data model in GIS and is implemented as operators in map algebra.The paper describes an implemen-tation of the level set methods in a map algebra program-ming language,called MapScript,and gives example pro-gram scripts for applications in ecology and hydrology.1IntroductionOver the past decade there has been an explosion in the ap-plication of models to solve environmental issues.Many of these models are specific to one physical process and of-ten require expert knowledge to use.Increasingly generic modeling frameworks are being sought to provide analyti-cal tools to examine and resolve complex environmental and natural resource problems.These systems consider a vari-ety of land condition characteristics,interactions and driv-ing physical processes.Variables accounted for include cli-mate,topography,soils,geology,land cover,vegetation and hydro-geography(Moore et al.,1993).Physical interactions include processes for climatology,hydrology,topographic landsurface/sub-surfacefluxes and biological/ecological sys-Correspondence to:D.Pullar(d.pullar@.au)tems(Sklar and Costanza,1991).Progress has been made in linking model-specific systems with tools used by environ-mental managers,for instance geographical information sys-tems(GIS).While this approach,commonly referred to as loose coupling,provides a practical solution it still does not improve the scientific foundation of these models nor their integration with other models and related systems,such as decision support systems(Argent,2003).The alternative ap-proach is for tightly coupled systems which build functional-ity into a system or interface to domain libraries from which a user may build custom solutions using a macro language or program scripts.The approach supports integrated models through interface specifications which articulate the funda-mental assumptions and simplifications within these models. The problem is that there are no environmental modelling systems which are widely used by engineers and scientists that offer this level of interoperability,and the more com-monly used GIS systems do not currently support space and time representations and operations suitable for modelling environmental processes(Burrough,1998)(Sui and Magio, 1999).Providing a generic environmental modeling framework for practical environmental issues is challenging.It does not exist now despite an overwhelming demand because there are deep technical challenges to build integrated modeling frameworks in a scientifically rigorous manner.It is this chal-lenge this research addresses.1.1Background for ApproachThe paper describes a generic environmental modeling lan-guage integrated with a Geographical Information System (GIS)which supports spatial-temporal operators to model physical interactions occurring in two ways.The trivial case where interactions are isolated to a location,and the more common and complex case where interactions propa-gate spatially across landscape surfaces.The programming language has a strong theoretical and algorithmic basis.The-oretically,it assumes a Eulerian representation of state space,Fig.1.Shows a)a propagating interface parameterised by differ-ential equations,b)interface fronts have variable intensity and may expand or contract based onfield gradients and driving process. but propagates quantities across landscapes using Lagrangian equations of motion.In physics,a Lagrangian view focuses on how a quantity(water volume or particle)moves through space,whereas an Eulerian view focuses on a localfixed area of space and accounts for quantities moving through it.The benefit of this approach is that an Eulerian perspective is em-inently suited to representing the variation of environmen-tal phenomena across space,but it is difficult to conceptu-alise solutions for the equations of motion and has compu-tational drawbacks(Press et al.,1992).On the other hand, the Lagrangian view is often not favoured because it requires a global solution that makes it difficult to account for local variations,but has the advantage of solving equations of mo-tion in an intuitive and numerically direct way.The research will address this dilemma by adopting a novel approach from the image processing discipline that uses a Lagrangian ap-proach over an Eulerian grid.The approach,called level set methods,provides an efficient algorithm for modeling a natural advancing front in a host of settings(Sethian,1999). The reason the method works well over other approaches is that the advancing front is described by equations of motion (Lagrangian view),but computationally the front propagates over a vectorfield(Eulerian view).Hence,we have a very generic way to describe the motion of quantities,but can ex-plicitly solve their advancing properties locally as propagat-ing zones.The research work will adapt this technique for modeling the motion of environmental variables across time and space.Specifically,it will add new data models and op-erators to a geographical information system(GIS)for envi-ronmental modeling.This is considered to be a significant research imperative in spatial information science and tech-nology(Goodchild,2001).The main focus of this paper is to evaluate if the level set method(Sethian,1999)can:–provide a theoretically and empirically supportable methodology for modeling a range of integral landscape processes,–provide an algorithmic solution that is not sensitive to process timing,is computationally stable and efficient as compared to conventional explicit solutions to diffu-sive processes models,–be developed as part of a generic modelling language in GIS to express integrated models for natural resource and environmental problems?The outline for the paper is as follow.The next section will describe the theory for spatial-temporal processing us-ing level sets.Section3describes how this is implemented in a map algebra programming language.Two application examples are given–an ecological and a hydrological ex-ample–to demonstrate the use of operators for computing reactive-diffusive interactions in landscapes.Section4sum-marises the contribution of this research.2Theory2.1IntroductionLevel set methods(Sethian,1999)have been applied in a large collection of applications including,physics,chemistry,fluid dynamics,combustion,material science,fabrication of microelectronics,and computer vision.Level set methods compute an advancing interface using an Eulerian grid and the Lagrangian equations of motion.They are similar to cost distance modeling used in GIS(Burroughs and McDonnell, 1998)in that they compute the spread of a variable across space,but the motion is based upon partial differential equa-tions related to the physical process.The advancement of the interface is computed through time along a spatial gradient, and it may expand or contract in its extent.See Fig.1.2.2TheoryThe advantage of the level set method is that it models mo-tion along a state-space gradient.Level set methods start with the equation of motion,i.e.an advancing front with velocity F is characterised by an arrival surface T(x,y).Note that F is a velocityfield in a spatial sense.If F was constant this would result in an expanding series of circular fronts,but for different values in a velocityfield the front will have a more contorted appearance as shown in Fig.1b.The motion of thisinterface is always normal to the interface boundary,and its progress is regulated by several factors:F=f(L,G,I)(1)where L=local properties that determine the shape of advanc-ing front,G=global properties related to governing forces for its motion,I=independent properties that regulate and influ-ence the motion.If the advancing front is modeled strictly in terms of the movement of entity particles,then a straightfor-ward velocity equation describes its motion:|∇T|F=1given T0=0(2) where the arrival function T(x,y)is a travel cost surface,and T0is the initial position of the interface.Instead we use level sets to describe the interface as a complex function.The level set functionφis an evolving front consistent with the under-lying viscosity solution defined by partial differential equa-tions.This is expressed by the equation:φt+F|∇φ|=0givenφ(x,y,t=0)(3)whereφt is a complex interface function over time period 0..n,i.e.φ(x,y,t)=t0..tn,∇φis the spatial and temporal derivatives for viscosity equations.The Eulerian view over a spatial domain imposes a discretisation of space,i.e.the raster grid,which records changes in value z.Hence,the level set function becomesφ(x,y,z,t)to describe an evolv-ing surface over time.Further details are given in Sethian (1999)along with efficient algorithms.The next section de-scribes the integration of the level set methods with GIS.3Map algebra modelling3.1Map algebraSpatial models are written in a map algebra programming language.Map algebra is a function-oriented language that operates on four implicit spatial data types:point,neighbour-hood,zonal and whole landscape surfaces.Surfaces are typ-ically represented as a discrete raster where a point is a cell, a neighbourhood is a kernel centred on a cell,and zones are groups of mon examples of raster data include ter-rain models,categorical land cover maps,and scalar temper-ature surfaces.Map algebra is used to program many types of landscape models ranging from land suitability models to mineral exploration in the geosciences(Burrough and Mc-Donnell,1998;Bonham-Carter,1994).The syntax for map algebra follows a mathematical style with statements expressed as equations.These equations use operators to manipulate spatial data types for point and neighbourhoods.Expressions that manipulate a raster sur-face may use a global operation or alternatively iterate over the cells in a raster.For instance the GRID map algebra (Gao et al.,1993)defines an iteration construct,called do-cell,to apply equations on a cell-by-cell basis.This is triv-ially performed on columns and rows in a clockwork manner. However,for environmental phenomena there aresituations Fig.2.Spatial processing orders for raster.where the order of computations has a special significance. For instance,processes that involve spreading or transport acting along environmental gradients within the landscape. Therefore special control needs to be exercised on the order of execution.Burrough(1998)describes two extra control mechanisms for diffusion and directed topology.Figure2 shows the three principle types of processing orders,and they are:–row scan order governed by the clockwork lattice struc-ture,–spread order governed by the spreading or scattering ofa material from a more concentrated region,–flow order governed by advection which is the transport of a material due to velocity.Our implementation of map algebra,called MapScript (Pullar,2001),includes a special iteration construct that sup-ports these processing orders.MapScript is a lightweight lan-guage for processing raster-based GIS data using map alge-bra.The language parser and engine are built as a software component to interoperate with the IDRISI GIS(Eastman, 1997).MapScript is built in C++with a class hierarchy based upon a value type.Variants for value types include numeri-cal,boolean,template,cells,or a grid.MapScript supports combinations of these data types within equations with basic arithmetic and relational comparison operators.Algebra op-erations on templates typically result in an aggregate value assigned to a cell(Pullar,2001);this is similar to the con-volution integral in image algebras(Ritter et al.,1990).The language supports iteration to execute a block of statements in three ways:a)docell construct to process raster in a row scan order,b)dospread construct to process raster in a spreadwhile(time<100)dospreadpop=pop+(diffuse(kernel*pop))pop=pop+(r*pop*dt*(1-(pop/K)) enddoendwhere the diffusive constant is stored in thekernel:Fig.3.Map algebra script and convolution kernel for population dispersion.The variable pop is a raster,r,K and D are constants, dt is the model time step,and the kernel is a3×3template.It is assumed a time step is defined and the script is run in a simulation. Thefirst line contained in the nested cell processing construct(i.e. dospread)is the diffusive term and the second line is the population growth term.order,c)doflow to process raster byflow order.Examples are given in subsequent sections.Process models will also involve a timing loop which may be handled as a general while(<condition>)..end construct in MapScript where the condition expression includes a system time variable.This time variable is used in a specific fashion along with a system time step by certain operators,namely diffuse()andfluxflow() described in the next section,to model diffusion and advec-tion as a time evolving front.The evolving front represents quantities such as vegetation growth or surface runoff.3.2Ecological exampleThis section presents an ecological example based upon plant dispersal in a landscape.The population of a species follows a controlled growth rate and at the same time spreads across landscapes.The theory of the rate of spread of an organism is given in Tilman and Kareiva(1997).The area occupied by a species grows log-linear with time.This may be modelled by coupling a spatial diffusion term with an exponential pop-ulation growth term;the combination produces the familiar reaction-diffusion model.A simple growth population model is used where the reac-tion term considers one population controlled by births and mortalities is:dN dt =r·N1−NK(4)where N is the size of the population,r is the rate of change of population given in terms of the difference between birth and mortality rates,and K is the carrying capacity.Further dis-cussion of population models can be found in Jrgensen and Bendoricchio(2001).The diffusive term spreads a quantity through space at a specified rate:dudt=Dd2udx2(5) where u is the quantity which in our case is population size, and D is the diffusive coefficient.The model is operated as a coupled computation.Over a discretized space,or raster,the diffusive term is estimated using a numerical scheme(Press et al.,1992).The distance over which diffusion takes place in time step dt is minimally constrained by the raster resolution.For a stable computa-tional process the following condition must be satisfied:2Ddtdx2≤1(6) This basically states that to account for the diffusive pro-cess,the term2D·dx is less than the velocity of the advancing front.This would not be difficult to compute if D is constant, but is problematic if D is variable with respect to landscape conditions.This problem may be overcome by progressing along a diffusive front over the discrete raster based upon distance rather than being constrained by the cell resolution.The pro-cessing and diffusive operator is implemented in a map al-gebra programming language.The code fragment in Fig.3 shows a map algebra script for a single time step for the cou-pled reactive-diffusion model for population growth.The operator of interest in the script shown in Fig.3is the diffuse operator.It is assumed that the script is run with a given time step.The operator uses a system time step which is computed to balance the effect of process errors with effi-cient computation.With knowledge of the time step the it-erative construct applies an appropriate distance propagation such that the condition in Eq.(3)is not violated.The level set algorithm(Sethian,1999)is used to do this in a stable and accurate way.As a diffusive front propagates through the raster,a cost distance kernel assigns the proper time to each raster cell.The time assigned to the cell corresponds to the minimal cost it takes to reach that cell.Hence cell pro-cessing is controlled by propagating the kernel outward at a speed adaptive to the local context rather than meeting an arbitrary global constraint.3.3Hydrological exampleThis section presents a hydrological example based upon sur-face dispersal of excess rainfall across the terrain.The move-ment of water is described by the continuity equation:∂h∂t=e t−∇·q t(7) where h is the water depth(m),e t is the rainfall excess(m/s), q is the discharge(m/hr)at time t.Discharge is assumed to have steady uniformflow conditions,and is determined by Manning’s equation:q t=v t h t=1nh5/3ts1/2(8)putation of current cell(x+ x,t,t+ ).where q t is theflow velocity(m/s),h t is water depth,and s is the surface slope(m/m).An explicit method of calcula-tion is used to compute velocity and depth over raster cells, and equations are solved at each time step.A conservative form of afinite difference method solves for q t in Eq.(5). To simplify discussions we describe quasi-one-dimensional equations for theflow problem.The actual numerical com-putations are normally performed on an Eulerian grid(Julien et al.,1995).Finite-element approximations are made to solve the above partial differential equations for the one-dimensional case offlow along a strip of unit width.This leads to a cou-pled model with one term to maintain the continuity offlow and another term to compute theflow.In addition,all calcu-lations must progress from an uphill cell to the down slope cell.This is implemented in map algebra by a iteration con-struct,called doflow,which processes a raster byflow order. Flow distance is measured in cell size x per unit length. One strip is processed during a time interval t(Fig.4).The conservative solution for the continuity term using afirst or-der approximation for Eq.(5)is derived as:h x+ x,t+ t=h x+ x,t−q x+ x,t−q x,txt(9)where the inflow q x,t and outflow q x+x,t are calculated in the second term using Equation6as:q x,t=v x,t·h t(10) The calculations approximate discharge from previous time interval.Discharge is dynamically determined within the continuity equation by water depth.The rate of change in state variables for Equation6needs to satisfy a stability condition where v· t/ x≤1to maintain numerical stabil-ity.The physical interpretation of this is that afinite volume of water wouldflow across and out of a cell within the time step t.Typically the cell resolution isfixed for the raster, and adjusting the time step requires restarting the simulation while(time<120)doflow(dem)fvel=1/n*pow(depth,m)*sqrt(grade)depth=depth+(depth*fluxflow(fvel)) enddoendFig.5.Map algebra script for excess rainfallflow computed over a 120minute event.The variables depth and grade are rasters,fvel is theflow velocity,n and m are constants in Manning’s equation.It is assumed a time step is defined and the script is run in a simulation. Thefirst line in the nested cell processing(i.e.doflow)computes theflow velocity and the second line computes the change in depth from the previous value plus any net change(inflow–outflow)due to velocityflux across the cell.cycle.Flow velocities change dramatically over the course of a storm event,and it is problematic to set an appropriate time step which is efficient and yields a stable result.The hydrological model has been implemented in a map algebra programming language Pullar(2003).To overcome the problem mentioned above we have added high level oper-ators to compute theflow as an advancing front over a land-scape.The time step advances this front adaptively across the landscape based upon theflow velocity.The level set algorithm(Sethian,1999)is used to do this in a stable and accurate way.The map algebra script is given in Fig.5.The important operator is thefluxflow operator.It computes the advancing front for waterflow across a DEM by hydrologi-cal principles,and computes the local drainageflux rate for each cell.Theflux rate is used to compute the net change in a cell in terms offlow depth over an adaptive time step.4ConclusionsThe paper has described an approach to extend the function-ality of tightly coupled environmental models in GIS(Ar-gent,2004).A long standing criticism of GIS has been its in-ability to handle dynamic spatial models.Other researchers have also addressed this issue(Burrough,1998).The con-tribution of this paper is to describe how level set methods are:i)an appropriate scientific basis,and ii)able to perform stable time-space computations for modelling landscape pro-cesses.The level set method provides the following benefits:–it more directly models motion of spatial phenomena and may handle both expanding and contracting inter-faces,–is based upon differential equations related to the spatial dynamics of physical processes.Despite the potential for using level set methods in GIS and land-surface process modeling,there are no commercial or research systems that use this mercial sys-tems such as GRID(Gao et al.,1993),and research systems such as PCRaster(Wesseling et al.,1996)offerflexible andpowerful map algebra programming languages.But opera-tions that involve reaction-diffusive processing are specific to one context,such as groundwaterflow.We believe the level set method offers a more generic approach that allows a user to programflow and diffusive landscape processes for a variety of application contexts.We have shown that it pro-vides an appropriate theoretical underpinning and may be ef-ficiently implemented in a GIS.We have demonstrated its application for two landscape processes–albeit relatively simple examples–but these may be extended to deal with more complex and dynamic circumstances.The validation for improved environmental modeling tools ultimately rests in their uptake and usage by scientists and engineers.The tool may be accessed from the web site .au/projects/mapscript/(version with enhancements available April2005)for use with IDRSIS GIS(Eastman,1997)and in the future with ArcGIS. It is hoped that a larger community of users will make use of the methodology and implementation for a variety of environmental modeling applications.Edited by:P.Krause,S.Kralisch,and W.Fl¨u gelReviewed by:anonymous refereesReferencesArgent,R.:An Overview of Model Integration for Environmental Applications,Environmental Modelling and Software,19,219–234,2004.Bonham-Carter,G.F.:Geographic Information Systems for Geo-scientists,Elsevier Science Inc.,New York,1994. Burrough,P.A.:Dynamic Modelling and Geocomputation,in: Geocomputation:A Primer,edited by:Longley,P.A.,et al., Wiley,England,165-191,1998.Burrough,P.A.and McDonnell,R.:Principles of Geographic In-formation Systems,Oxford University Press,New York,1998. Gao,P.,Zhan,C.,and Menon,S.:An Overview of Cell-Based Mod-eling with GIS,in:Environmental Modeling with GIS,edited by: Goodchild,M.F.,et al.,Oxford University Press,325–331,1993.Goodchild,M.:A Geographer Looks at Spatial Information Theory, in:COSIT–Spatial Information Theory,edited by:Goos,G., Hertmanis,J.,and van Leeuwen,J.,LNCS2205,1–13,2001.Jørgensen,S.and Bendoricchio,G.:Fundamentals of Ecological Modelling,Elsevier,New York,2001.Julien,P.Y.,Saghafian,B.,and Ogden,F.:Raster-Based Hydro-logic Modelling of Spatially-Varied Surface Runoff,Water Re-sources Bulletin,31(3),523–536,1995.Moore,I.D.,Turner,A.,Wilson,J.,Jenson,S.,and Band,L.:GIS and Land-Surface-Subsurface Process Modeling,in:Environ-mental Modeling with GIS,edited by:Goodchild,M.F.,et al., Oxford University Press,New York,1993.Press,W.,Flannery,B.,Teukolsky,S.,and Vetterling,W.:Numeri-cal Recipes in C:The Art of Scientific Computing,2nd Ed.Cam-bridge University Press,Cambridge,1992.Pullar,D.:MapScript:A Map Algebra Programming Language Incorporating Neighborhood Analysis,GeoInformatica,5(2), 145–163,2001.Pullar,D.:Simulation Modelling Applied To Runoff Modelling Us-ing MapScript,Transactions in GIS,7(2),267–283,2003. Ritter,G.,Wilson,J.,and Davidson,J.:Image Algebra:An Overview,Computer Vision,Graphics,and Image Processing, 4,297–331,1990.Sethian,J.A.:Level Set Methods and Fast Marching Methods, Cambridge University Press,Cambridge,1999.Sklar,F.H.and Costanza,R.:The Development of Dynamic Spa-tial Models for Landscape Ecology:A Review and Progress,in: Quantitative Methods in Ecology,Springer-Verlag,New York, 239–288,1991.Sui,D.and R.Maggio:Integrating GIS with Hydrological Mod-eling:Practices,Problems,and Prospects,Computers,Environ-ment and Urban Systems,23(1),33–51,1999.Tilman,D.and P.Kareiva:Spatial Ecology:The Role of Space in Population Dynamics and Interspecific Interactions.Princeton University Press,Princeton,New Jersey,USA,1997. Wesseling C.G.,Karssenberg, D.,Burrough,P. A.,and van Deursen,W.P.:Integrating Dynamic Environmental Models in GIS:The Development of a Dynamic Modelling Language, Transactions in GIS,1(1),40–48,1996.。
计量经济学中英文词汇对照

Controlled experiments Conventional depth Convolution Corrected factor Corrected mean Correction coefficient Correctness Correlation coefficient Correlation index Correspondence Counting Counts Covaห้องสมุดไป่ตู้iance Covariant Cox Regression Criteria for fitting Criteria of least squares Critical ratio Critical region Critical value
Asymmetric distribution Asymptotic bias Asymptotic efficiency Asymptotic variance Attributable risk Attribute data Attribution Autocorrelation Autocorrelation of residuals Average Average confidence interval length Average growth rate BBB Bar chart Bar graph Base period Bayes' theorem Bell-shaped curve Bernoulli distribution Best-trim estimator Bias Binary logistic regression Binomial distribution Bisquare Bivariate Correlate Bivariate normal distribution Bivariate normal population Biweight interval Biweight M-estimator Block BMDP(Biomedical computer programs) Boxplots Breakdown bound CCC Canonical correlation Caption Case-control study Categorical variable Catenary Cauchy distribution Cause-and-effect relationship Cell Censoring
基于混合特征和分层最近邻法的人脸表情识别
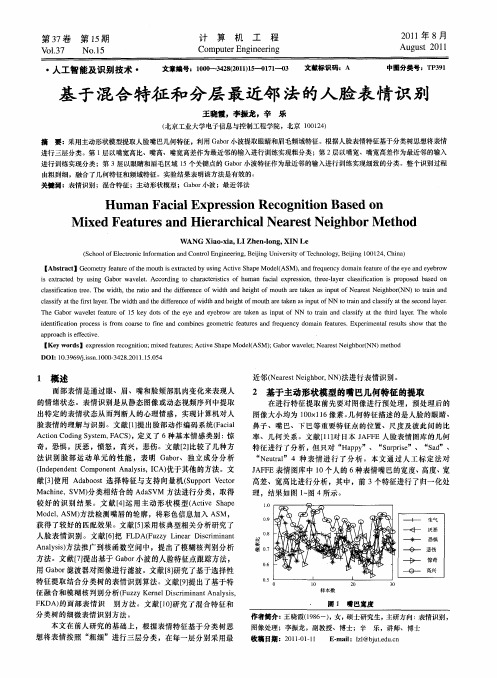
H u a c a p e so c g ii n Ba e n m n Fa i l Ex r s i n Re o n to s d 0
M i e a ur sa d H i r r h c l a e tNe g b rM e h d x d Fe t e n e a c i a Ne r s i h o t o
第 3 卷 第 1 7 5期
、 .7 b1 3
・
计
算
机
工
程
21 0 1年 8月
Au u t 2 1 g s 0 1
No 1 .5
Co u e n i e rn mp t r g n e i g E
人工 智 能及 识别 技术 ・
文章编号:10_ 4801 5_7—0 文献标识码: 0oI 2( 11 0 1_ 3 2 )— 1 3 A
[ b ta t A src ]Geme faue fh uhie t ce yuigAcieS aeMo e( M)adfeu nyd ma a r fh y n y bo o 时 etr emo t xr tdb s t hp d l ot s a n v AS ,n q e c o i f t eo eeea d e rw r neu t e
W ANG a - i , h n- n , N Xi o x a LIZ e l g XI Le o
(c o l f l t ncIfr t na d nrl n ier g Be igUnvri f e h oo yBe ig10 2 , hn ) S h o Ee r i nomai n Co t E gn e n , in o co o o i j ies yo T c n lg , in 0 14 C ia t j
Multimodality Image Registration by Maximization of Mutual Information
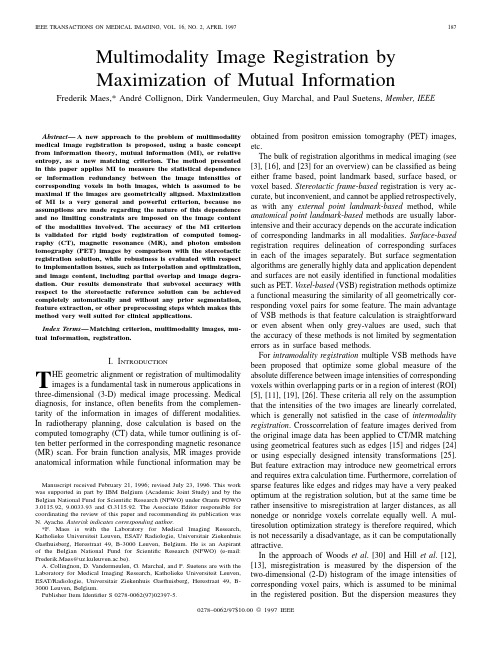
Multimodality Image Registration byMaximization of Mutual Information Frederik Maes,*Andr´e Collignon,Dirk Vandermeulen,Guy Marchal,and Paul Suetens,Member,IEEEAbstract—A new approach to the problem of multimodality medical image registration is proposed,using a basic concept from information theory,mutual information(MI),or relative entropy,as a new matching criterion.The method presented in this paper applies MI to measure the statistical dependence or information redundancy between the image intensities of corresponding voxels in both images,which is assumed to be maximal if the images are geometrically aligned.Maximization of MI is a very general and powerful criterion,because no assumptions are made regarding the nature of this dependence and no limiting constraints are imposed on the image content of the modalities involved.The accuracy of the MI criterion is validated for rigid body registration of computed tomog-raphy(CT),magnetic resonance(MR),and photon emission tomography(PET)images by comparison with the stereotactic registration solution,while robustness is evaluated with respect to implementation issues,such as interpolation and optimization, and image content,including partial overlap and image degra-dation.Our results demonstrate that subvoxel accuracy with respect to the stereotactic reference solution can be achieved completely automatically and without any prior segmentation, feature extraction,or other preprocessing steps which makes this method very well suited for clinical applications.Index Terms—Matching criterion,multimodality images,mu-tual information,registration.I.I NTRODUCTIONT HE geometric alignment or registration of multimodality images is a fundamental task in numerous applications in three-dimensional(3-D)medical image processing.Medical diagnosis,for instance,often benefits from the complemen-tarity of the information in images of different modalities. In radiotherapy planning,dose calculation is based on the computed tomography(CT)data,while tumor outlining is of-ten better performed in the corresponding magnetic resonance (MR)scan.For brain function analysis,MR images provide anatomical information while functional information may beManuscript received February21,1996;revised July23,1996.This work was supported in part by IBM Belgium(Academic Joint Study)and by the Belgian National Fund for Scientific Research(NFWO)under Grants FGWO 3.0115.92,9.0033.93and G.3115.92.The Associate Editor responsible for coordinating the review of this paper and recommending its publication was N.Ayache.Asterisk indicates corresponding author.*F.Maes is with the Laboratory for Medical Imaging Research, Katholieke Universiteit Leuven,ESAT/Radiologie,Universitair Ziekenhuis Gasthuisberg,Herestraat49,B-3000Leuven,Belgium.He is an Aspirant of the Belgian National Fund for Scientific Research(NFWO)(e-mail: Frederik.Maes@uz.kuleuven.ac.be).A.Collingnon,D.Vandermeulen,G.Marchal,and P.Suetens are with the Laboratory for Medical Imaging Research,Katholieke Universiteit Leuven, ESAT/Radiologie,Universitair Ziekenhuis Gasthuisberg,Herestraat49,B-3000Leuven,Belgium.Publisher Item Identifier S0278-0062(97)02397-5.obtained from positron emission tomography(PET)images, etc.The bulk of registration algorithms in medical imaging(see [3],[16],and[23]for an overview)can be classified as being either frame based,point landmark based,surface based,or voxel based.Stereotactic frame-based registration is very ac-curate,but inconvenient,and cannot be applied retrospectively, as with any external point landmark-based method,while anatomical point landmark-based methods are usually labor-intensive and their accuracy depends on the accurate indication of corresponding landmarks in all modalities.Surface-based registration requires delineation of corresponding surfaces in each of the images separately.But surface segmentation algorithms are generally highly data and application dependent and surfaces are not easily identified in functional modalities such as PET.Voxel-based(VSB)registration methods optimize a functional measuring the similarity of all geometrically cor-responding voxel pairs for some feature.The main advantage of VSB methods is that feature calculation is straightforward or even absent when only grey-values are used,such that the accuracy of these methods is not limited by segmentation errors as in surface based methods.For intramodality registration multiple VSB methods have been proposed that optimize some global measure of the absolute difference between image intensities of corresponding voxels within overlapping parts or in a region of interest(ROI) [5],[11],[19],[26].These criteria all rely on the assumption that the intensities of the two images are linearly correlated, which is generally not satisfied in the case of intermodality registration.Crosscorrelation of feature images derived from the original image data has been applied to CT/MR matching using geometrical features such as edges[15]and ridges[24] or using especially designed intensity transformations[25]. But feature extraction may introduce new geometrical errors and requires extra calculation time.Furthermore,correlation of sparse features like edges and ridges may have a very peaked optimum at the registration solution,but at the same time be rather insensitive to misregistration at larger distances,as all nonedge or nonridge voxels correlate equally well.A mul-tiresolution optimization strategy is therefore required,which is not necessarily a disadvantage,as it can be computationally attractive.In the approach of Woods et al.[30]and Hill et al.[12], [13],misregistration is measured by the dispersion of the two-dimensional(2-D)histogram of the image intensities of corresponding voxel pairs,which is assumed to be minimal in the registered position.But the dispersion measures they0278–0062/97$10.00©1997IEEEpropose are largely heuristic.Hill’s criterion requires seg-mentation of the images or delineation of specific histogram regions to make the method work [20],while Woods’criterion is based on additional assumptions concerning the relationship between the grey-values in the different modalities,which reduces its applicability to some very specific multimodality combinations (PET/MR).In this paper,we propose to use the much more general notion of mutual information (MI)or relative entropy [8],[22]to describe the dispersive behavior of the 2-D histogram.MI is a basic concept from information theory,measuring the statistical dependence between two random variables or the amount of information that one variable contains about the other.The MI registration criterion presented here states that the MI of the image intensity values of corresponding voxel pairs is maximal if the images are geometrically aligned.Because no assumptions are made regarding the nature of the relation between the image intensities in both modalities,this criterion is very general and powerful and can be applied automatically without prior segmentation on a large variety of applications.This paper expands on the ideas first presented by Collignon et al .[7].Related work in this area includes the work by Viola and Wells et al .[27],[28]and by Studholme et al .[21].The theoretical concept of MI is presented in Section II,while the implementation of the registration algorithm is described in Section III.In Sections IV,V,and VI we evaluate the accuracy and the robustness of the MI matching criterion for rigid body CT/MR and PET/MR registration.Section VII summarizes our current findings,while Section VIII gives some directions for further work.In the Appendexes,we discuss the relationship of the MI registration criterion to other multimodality VSB criteria.II.T HEORYTwo randomvariables,,with marginal probabilitydistributions,and:.MI,and(1)MI is related to entropy by theequationsandgivengiven(5)(7)Theentropy,whilewhenknowingby the knowledge of another randomvariablecontainsaboutandandand.The MI registration criterion states that the images are geometrically aligned by thetransformation forwhichMAES et al.:MULTIMODALITY IMAGE REGISTRATION BY MAXIMIZATION OF MUTUAL INFORMATION189(a)(b)Fig.1.Joint histogram of the overlapping volume of the CT and MR brain images of dataset A in Tables II and III:(a)Initial position:I (CT;MR )=0:46,(b)registered position:I (CT;MR )=0:89.Misregistration was about 20mm and 10 (see the parameters in Table III).If both marginaldistributionsand,the MI criterion reduces to minimizing the jointentropyor ,which is the case if one of the images is always completely contained in the other,the MI criterion reduces to minimizing the conditionalentropyisvariedandand.The MI criterion takes this into accountexplicitly,as becomes clear in (2),which can be interpreted as follows [27]:“maximizing MI will tend to find as much as possible of the complexity that is in the separate datasets (maximizing the first two terms)so that at the same time they explain each other well (minimizing the last term).”For.Thisrequiresis varied,which will be the case if the image intensity values are spatially correlated.This is illustrated by the graphs in Fig.2,showing the behaviorofaxis along the row direction,theaxis along the plane direction.One of the images is selected to be the floatingimage,are taken and transformed intothe referenceimage,or a sub-or superset thereof.Subsampling of the floating image might be used to increase speed performance,while supersampling aims at increasing accuracy.For each value of the registrationparameterfalls inside the volumeofis a six-component vector consisting of three rotationanglestoimage(8)with3diagonal matrixes representing thevoxel sizes ofimages,respectively (inmillimeters),3rotation matrix,with thematrixes-,-axis,respectively,and190IEEE TRANSACTIONS ON MEDICAL IMAGING,VOL.16,NO.2,APRIL1997Fig.3.Graphical illustration of NN,TRI,and PV interpolation in 2-D.NN and TRI interpolation find the reference image intensity value at position T s and update the corresponding joint histogram entry,while PV interpolation distributes the contribution of this sample over multiple histogram entries defined by its NN intensities,using the same weights as for TRI interpolation.B.CriterionLetatposition.The joint image intensityhistogramis computed by binning the image intensitypairs forallbeing the total number of bins in the joint histogram.Typically,weusewill not coincide with a grid pointofis generally insufficient to guaranteesubvoxel accuracy,as it is insensitive to translations up to one voxel.Other interpolation methods,such as trilinear (TRI)interpolation,may introduce new intensity values which are originally not present in the reference image,leading tounpredictable changes in the marginaldistributionof the reference image for small variationsof,the contribution of the imageintensityofon the gridofis varied.Estimations for the marginal and joint image intensitydistributionsis then evaluatedby(12)and the optimal registrationparameter is foundfrom,using Brent’s one-dimensional optimization algorithm for the line minimizations [18].The direction matrix is initialized with unit vectors in each of the parameter directions.An appropriate choice for the order in which the parameters are optimized needs to be specified,as this may influence optimization robustness.For instance,when matching images of the brain,the horizontal translation and the rotation around the vertical axis are more constrained by the shape of the head than the pitching rotation around the left-to-right horizontal axis.There-fore,first aligning the images in the horizontal plane by first optimizing the in-planeparameters may facilitate the optimization of the out-of-planeparametersMAES et al.:MULTIMODALITY IMAGE REGISTRATION BY MAXIMIZATION OF MUTUAL INFORMATION 191TABLE IID ATASETS U SEDIN THEE XPERIMENTS D ISCUSSED IN S ECTIONS VANDVIIV.E XPERIMENTSThe performance of the MI registration criterion was eval-uated for rigid-body registration of MR,CT,and PET images of the brain of the same patient.The rigid-body assumption is well satisfied inside the skull in 3-D scans of the head if patient related changes (due to for instance interscanning operations)can be neglected,provided that scanner calibration problems and problems of geometric distortions have been minimized by careful calibration and scan parameter selection,respectively.Registration accuracy is evaluated in Section V by comparison with external marker-based registration results and other retrospective registration methods,while the robust-ness of the method is evaluated in Section VI with respect to implementation issues,such as sampling,interpolation and op-timization,and image content,including image degradations,such as noise,intensity inhomogeneities and distortion,and partial image overlap.Four different datasets are used in the experiments described below (Table II).Dataset A 1contains high-resolution MR and CT images,while dataset B was obtained by smoothing and subsampling the images of dataset A to simulate lower resolution data.Dataset C 2contains stereotactically acquired MR,CT,and PET images,which have been edited to remove stereotactic markers.Dataset D contains an MR image only and is used to illustrate the effect of various image degradations on the registration criterion.All images consist of axial slices and in all casestheaxis is directedhorizontally front to back,andthedirection.In all experiments,the joint histogram size is256axis (0.7direction due to an offset inthedirection for the solution obtainedusing PV interpolation due to a 1rotation parameter.For MR to PET as well as for PET to MR registration,PV interpolation yields the smallest differences with the stereotactic reference solution,especially inthedirection due to offsets inthe192IEEE TRANSACTIONS ON MEDICAL IMAGING,VOL.16,NO.2,APRIL 1997TABLE IIIR EFERENCE AND MI R EGISTRATION P ARAMETERS FOR D ATASETS A,B,AND C AND THE M EAN AND M AXIMAL A BSOLUTE D IFFERENCE E V ALUATED AT E IGHT P OINTS N EAR THE B RAIN SURFACEvolume as the floating image and using different interpolation methods.For each combination,various optimization strate-gies were tried by changing the order in which the parameters were optimized,each starting from the same initial position with all parameters set to zero.The results are summarized in Fig.5.These scatter plots compare each of the solutions found (represented by their registrationparameterson the horizontal axis (using mm and degreesfor the translation and rotation parameters,respectively)and by the difference in the value of the MI criterion(MI)on the vertical axis.Although the differences are small for each of the interpolation methods used,MR to CT registration seems to be somewhat more robust than CT to MR registration.More importantly,the solutions obtained using PV interpolation are much more clustered than those obtained using NN or TRI interpolation,indicating that the use of PV interpolation results in a much smoother behavior of the registration criterion.This is also apparent from traces in registration space computed around the optimal solution for NN,TRI,and PV interpolation (Fig.6).These traces look very similar when a large parameter range is considered,but in the neighborhood of the registration solution,traces obtained with NN and TRI interpolation are noisy and show manylocal maxima,while traces obtained with PV interpolation are almost quadratic around the optimum.Remark that the MI values obtained using TRI interpolation are larger than those obtained using NN or PV interpolation,which can be interpreted according to (2):The TRI averaging and noise reduction of the reference image intensities resulted in a larger reduction of the complexity of the joint histogram than the corresponding reduction in the complexity of the reference image histogram itself.B.SubsamplingThe computational complexity of the MI criterion is pro-portional to the number of samples that is taken from the floating image to compute the joint histogram.Subsampling of the floating image can be applied to increase speed perfor-mance,as long as this does not deteriorate the optimization behavior.This was investigated for dataset A by registration of the subsampled MR image with the original CT image using PV interpolation.Subsampling was performed by takingsamples on a regular grid at sample intervalsofand direction,respectively,using NNinterpolation.No averaging or smoothing of the MR image before subsampling was applied.Weused,and .The same optimization strategy was used in each case.RegistrationsolutionsandMAES et al.:MULTIMODALITY IMAGE REGISTRATION BY MAXIMIZATION OF MUTUAL INFORMATION193(a)(b)Fig.5.Evaluation of the MI registration robustness for dataset A.Horizontal axis:norm of the difference vector j 0 3j for different optimization strategies,using NN,TRI,and PV interpolation. 3corresponds to the registration solution with the best value for the registration criterion for each of the interpolation schemes applied.Vertical axis:difference in the registration criterion between each solution and the optimal one.(a)Using the CT image as the floating image.(b)Using the MR image as the floatingimage.(a)(b)(c)(d)Fig.6.MI traces around the optimal registration position for dataset A:Rotation around the x axis in the range from 0180to +180 (a)and from 00.5to +0.5 (bottom row),using NN (b),TRI (c),and PV (d)interpolation.intheand 0.2mm off from the solutionfound without subsampling.C.Partial OverlapClinically acquired images typically only partially overlap,as CT scanning is often confined to a specific region to minimize the radiation dose while MR protocols frequently image larger volumes.The influence of partial overlap on the registration robustness was evaluated for dataset A for CT to MR registration using PV interpolation.The images were initially aligned as in the experiment in Section V and the same optimization strategy was applied,but only part of the CT data was considered when computing the MI criterion.More specifically,three 50-slice slabs were selected at the bottom (the skull basis),the middle,and the top part of the dataset.The results are summarized in Table IV and compared with the solution found using the full dataset by the mean and194IEEE TRANSACTIONS ON MEDICAL IMAGING,VOL.16,NO.2,APRIL 1997TABLE IVI NFLUENCEOFP ARTIAL O VERLAPONTHE R EGISTRATION R OBUSTNESSFORCTTOMR R EGISTRATIONOFD ATASETAFig.7.Effect of subsampling the MR floating image of dataset A on the registration solution.Horizontal axis:subsampling factor f ,indicating that only one out of f voxels was considered when evaluating the MI criterion.Vertical axis:norm of the difference vector j 0 3j . 3corresponds to the registration solution obtained when no subsampling is applied.maximal absolute difference evaluated over the full image at the same eight points as in Section V.The largest parameter differences occur for rotation aroundthedirection,resulting in maximal coordinate differencesup to 1.5CT voxel inthe direction,but on average all differences are subvoxel with respect to the CT voxel sizes.D.Image DegradationVarious MR image degradation effects,such as noise,in-tensity inhomogeneity,and geometric distortion,alter the intensity distribution of the image which may affect the MI registration criterion.This was evaluated for the MR image of dataset D by comparing MI registration traces obtained for the original image and itself with similar traces obtained for the original image and its degraded version (Fig.8).Such traces computed for translation inthewas alteredinto(15)(a)(b)(c)(d)Fig.8.(a)Slice 15of the original MR image of dataset D,(b)zero mean noise added with variance of 500grey-value units,(c)quadratic inhomogeneity (k =0:004),and (d)geometric distortion (k =0:00075).with being the image coordinates of the point around which the inhomogeneity is centeredand.All traces for all param-eters reach their maximum at the same position and the MI criterion is not affected by the presence of the inhomogeneity.3)Geometric Distortion:Geometricdistortions(16)(17)(18)withthe image coordinates of the center of each image planeandtranslation parameter proportionalto the averagedistortionMAES et al.:MULTIMODALITY IMAGE REGISTRATION BY MAXIMIZATION OF MUTUAL INFORMATION195(a)(b)(c)(d)Fig.9.MI traces using PV interpolation for translation in the x direction of the original MR image of dataset D over its degraded version in the range from 010to +10mm:(a)original,(b)noise,(c)intensity inhomogeneity,and (d)geometric distortion.VII.D ISCUSSIONThe MI registration criterion presented in this paper assumes that the statistical dependence between corresponding voxel intensities is maximal if both images are geometrically aligned.Because no assumptions are made regarding the nature of this dependence,the MI criterion is highly data independent and allows for robust and completely automatic registration of multimodality images in various applications with min-imal tuning and without any prior segmentation or other preprocessing steps.The results of Section V demonstrate that subvoxel registration differences with respect to the stereo-tactic registration solution can be obtained for CT/MR and PET/MR matching without using any prior knowledge about the grey-value content of both images and the correspondence between them.Additional experiments on nine other datasets similar to dataset C within the Retrospective Registration Evaluation Project by Fitzpatrick et al .[10]have verified these results [29],[14].Moreover,Section VI-C demonstrated the robustness of the method with respect to partial over-lap,while it was shown in Section VI-D that large image degradations,such as noise and intensity inhomogeneities,have no significant influence on the MI registration crite-rion.Estimations of the image intensity distributions were ob-tained by simple normalization of the joint histogram.In all experiments discussed in this paper,the joint histogram was computed from the entire overlapping part of both images,using the original image data and a fixed number of bins of256andand .For low-resolutionimages,the optimization often did not converge to the global optimum if a different parameter order was specified,due to the occurrence of local optima especially forthe196IEEE TRANSACTIONS ON MEDICAL IMAGING,VOL.16,NO.2,APRIL1997theand40mm,but we have not extensivelyinvestigated the robustness of the method with respect to theinitial positioning of the images,for instance by using multiplerandomised starting estimates.The choice of thefloating imagemay also influence the behavior of the registration criterion.In the experiment of Section VI-A,MR to CT matching wasfound to be more robust than CT to MR matching.However,it is not clear whether this was caused by sampling andinterpolation issues or by the fact that the MR image is morecomplex than the CT image and that the spatial correlation ofimage intensity values is higher in the CT image than in theMR image.We have not tuned the design of the search strategy towardspecific applications.For instance,the number of criterionevaluations required may be decreased by taking the limitedimage resolution into account when determining convergence.Moreover,the results of Section VI-B demonstrate that forhigh-resolution images subsampling of thefloating imagecan be applied without deteriorating optimization robustness.Important speed-ups can,thus,be realized by using a mul-tiresolution optimization strategy,starting with a coarselysampled image for efficiency and increasing the resolution asthe optimization proceeds for accuracy[20].Furthermore,thesmooth behavior of the MI criterion,especially when usingPV interpolation,may be exploited by using gradient-basedoptimization methods,as explicit formulas for the derivativesof the MI function with respect to the registration parameterscan be obtained[27].All the experiments discussed in this paper were for rigid-body registration of CT,MR,and PET images of the brainof the same patient.However,it is clear that the MI criterioncan equally well be applied to other applications,using moregeneral geometric transformations.We have used the samemethod successfully for patient-to-patient matching of MRbrain images for correlation of functional MR data and forthe registration of CT images of a hardware phantom to itsgeometrical description to assess the accuracy of spiral CTimaging[14].MI measures statistical dependence by comparing the com-plexity of the joint distribution with that of the marginals.Bothmarginal distributions are taken into account explicitly,whichis an important difference with the measures proposed by Hillet al.[13](third-order moment of the joint histogram)andCollignon et al.[6](entropy of the joint histogram),whichfocus on the joint histogram only.In Appendexes A and B wediscuss the relationship of these criteria and of the measureof Woods et al.[30](variance of intensity ratios)to the MIcriterion.MI is only one of a family of measures of statisticaldependence or information redundancy(see Appendix C).We have experimentedwith,the entropy correlation coefficient[1].In some cases these measures performed better thanthe original MI criterion,but we could not establish a clearpreference for either of these.Furthermore,the use of MIfor multimodality image registration is not restricted to theoriginal image intensities only:other derived features such asedges or ridges can be used as well.Selection of appropriatefeatures is an area for further research.VIII.C ONCLUSIONThe MI registration criterion presented in this paper allowsfor subvoxel accurate,highly robust,and completely automaticregistration of multimodality medical images.Because themethod is largely data independent and requires no userinteraction or preprocessing,the method is well suited to beused in clinical practice.Further research is needed to better understand the influenceof implementation issues,such as sampling and interpolation,on the registration criterion.Furthermore,the performance ofthe registration method on clinical data can be improved bytuning the optimization method to specific applications,whilealternative search strategies,including multiresolution andgradient-based methods,have to be investigated.Finally,otherregistration criteria can be derived from the one presented here,using alternative information measures applied on differentfeatures.A PPENDIX AWe show the relationship between the multimodality reg-istration criterion devised by Hill et al.[12]and the jointentropy th-order moment of thescatter-plot[22]with the following properties.1)andand with。
涡流均匀性对柴油机燃烧影响的数值研究
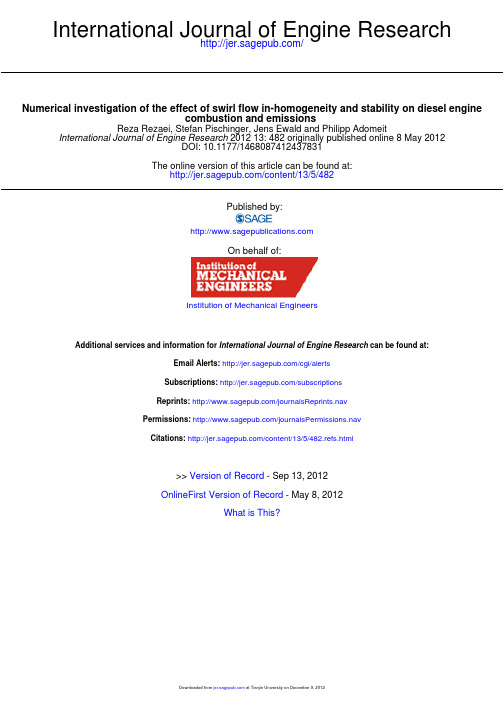
/International Journal of Engine Research/content/13/5/482The online version of this article can be found at:DOI: 10.1177/14680874124378312012 13: 482 originally published online 8 May 2012International Journal of Engine Research Reza Rezaei, Stefan Pischinger, Jens Ewald and Philipp Adomeitcombustion and emissionsNumerical investigation of the effect of swirl flow in-homogeneity and stability on diesel enginePublished by: On behalf of:Institution of Mechanical Engineers can be found at:International Journal of Engine Research Additional services and information for/cgi/alerts Email Alerts:/subscriptions Subscriptions: /journalsReprints.nav Reprints:/journalsPermissions.nav Permissions:/content/13/5/482.refs.html Citations:What is This?- May 8, 2012OnlineFirst Version of Record- Sep 13, 2012Version of Record >>OriginalArticleInternational J of Engine Research13(5)482–496ÓRWTH Aachen University2012Reprints and permissions:/journalsPermissions.navDOI:10.1177/1468087412437831Numerical investigation of the effect ofswirl flow in-homogeneity and stabilityon diesel engine combustion andemissionsReza Rezaei1,Stefan Pischinger1,Jens Ewald2and Philipp Adomeit2AbstractThe present study is aimed at numerically investigating the effect of in-cylinder charge motion on mixture preparation, combustion and emission formation in a high-speed direct-injection diesel engine.Previous investigations have shown that different valve-lift strategies nominally lead to similar in-cylinder filling and global swirl levels.However,significant differences in engine-out emissions,especially soot emission,give rise to the assumption that the flow structure and local differences of the swirl motion distribution have a noticeable effect on emission behaviour.In this work,different swirl generation strategies applying different intake valve actuation schemes are numerically investigated by applying transient in-cylinder computational fluid dynamic simulations using both the Reynolds-averaged Navier–Stokes model and the multi-cycle large-eddy simulation approach.T wo operating points within the operating range of current diesel passenger cars during federal test procedure75and new European driving cycles are simulated.The injection and combustion simulations of different valve strategies show that an in-homogeneity in the in-cylinder flow structure leads to a signifi-cant increase in soot emissions,and agree with the observed trends of corresponding experimental investigations. KeywordsDiesel engine,simulation,in-cylinder flow,combustion,emission formationDate received:7October2010;accepted:11October2011IntroductionIn order to achieve new emission standards and reduce fuel consumption in future diesel engines,the combus-tion system requires intense development.In order to simultaneously improve the soot–nitrogen oxide(NO x) trade-off and decrease fuel consumption in comparison to traditional combustion systems,numerous advanced technologies are taken into consideration.These include high-pressure injection equipment using fast-opening piezo-actuated injectors on the one hand and,on the other hand,careful design of the piston bowl in order to reach an optimized distribution of the air–fuel mix-ture between bowl and squish volume.In addition to these,an optimization of the in-cylinder swirl charge motion is of vital importance.The effects of the in-cylinder charge motion distribution on combustion and emission behaviour of diesel engines are investigated in this study.The effects of in-cylinder flow and swirl in-homogeneity have been studied by several investiga-tors.In1995,Stephenson and Rutland1simulated intake flow and combustion in a heavy-duty direct-injection(DI)diesel engine resulting from different intake flow configurations and compared these with the significance of spray–wall interaction effects,using the computational fluid dynamic(CFD)code KIVA-3. Two separate computational grids were applied in KIVA:one for intake flow simulation and one for com-bustion simulation.At the time directly after intake valve closing(IVC),the data were mapped from the first grid to the combustion grid.Different valve-lift configurations with one and two active valves were simulated.Variations in the in-cylinder flow in terms of turbulent length scales and intensity,as well as their significance to combustion and emissions parameters, 1Institute for Combustion Engines,RWTH Aachen University,Germany 2FEV Motorentechnik GmbH,GermanyCorresponding author:R Rezaei,Institute for Combustion Engines,RWTH Aachen University, Schinkelstr.8,52062Aachen,Germany.Email:reza.rezaei@rwth.aachen.dewere compared with the significance of spray–wall interaction effects.It was concluded that at idling oper-ation,the differences in intake flow were considerably less important than at3/4load.Furthermore,it was found that valve deactivation led to higher turbulent kinetic energy and turbulent length scale.Bianchi et al.2investigated the influence of different initial flow conditions on combustion and emissions in a small-bore high-speed direct-injection(HSDI)diesel engine.The analysis was carried out by applying STAR-CD software for intake stroke simulation and KIVA-II for the compression stroke and combustion simulation.It was concluded that a detailed definition of the initial conditions is required to properly predict the mean and turbulent flow fields at the time of injec-tion near top dead centre(TDC),especially for small-bore HSDI diesel engines.The injection and combustion simulation using a full cylinder mesh was compared with simulation results considering a sector mesh simulation by Antila et al.3 In the case of an HSDI diesel engine,the difference between a sector mesh simulation and a full-cylinder mesh simulation was found to be considerable.The predicted injection velocity was found to have a note-worthy effect on the simulated heat release.3In2006,Adomeit et al.4showed that an eccentricity in the in-cylinder swirl flow pattern,observed by apply-ing the particle image velocimetry(PIV)measurement technique and intake stroke CFD analysis,can strongly affect the soot oxidation processes.Non-symmetric soot distribution was observed in laser-induced incan-descence(LII)measurements at the end of combustion due to an eccentric swirl flow before start of injection.In2008,Ge et al.5modelled the effect of the in-cylinder flow field on HSDI diesel engine performance and emissions.Two combustion models,KIVA-CHEMKIN and GAMUT(KIVA-CHEMKIN-G), coupled with a two-step and a multi-step phenomeno-logical soot model were applied.Numerical results were compared with experimental optical diagnostics obtained using laser-induced fluorescence(LIF),LII and PIV.It was concluded that the influence of the off-centred swirl flow on volume-averaged values,includ-ing in-cylinder pressure,temperature and heat release rate was negligible.The off-centred swirl flow was found to have higher turbulent kinetic energy and also higher turbulent viscosity.They have observed that an eccentric flow field detected in PIV measurements led to higher amounts of engine soot emissions.It should be noted that in Ge et al.5the intake stroke was not simulated and off-centred swirl flows with an assumed radial velocity distribution were initialized.Experimental investigations on gas exchange optimi-zation and its impact on emission reduction were pre-sented in previous work.6It was shown that increasing the swirl ratio up to a certain optimum level can improve the engine-out emissions and,simultaneously, the fuel consumption.Furthermore,it was observed that the optimum value of the swirl ratio depends on the operating conditions and engine speed.Therefore, in order to provide the corresponding flexibility,an HSDI diesel engine concept was developed that fea-tures a variable intake valve-lift system.In this previous work,the concept of numerically assessing the in-homogeneity of the in-cylinder swirl charge motion was introduced.Very-large-eddy simulation(VLES),a hybrid approach between large-eddy simulation(LES) and Reynolds-averaged Navier–Stokes(RANS)simu-lation of the in-cylinder flow fields for different valve-lift strategies of the same HSDI diesel engine were car-ried out and correlated to experimentally observed combustion performance.Multi-cycle simulations of the same operating point to cover cyclic instability and CFD simulations of combustion were not carried out at that stage of the research.In the present study,the work is extended to simu-late multi-cycle combustion.Both RANS modelling and the LES multi-cycle approach are employed for intake and compression flow simulations.Different swirl flow patterns are assessed and the numerically predicted emission behaviour,with regards to flow in-homogeneity,is compared to engine measurement results.MethodologyTest engineThe engine simulated in the present work is a state-of-the-art,small-size class,common-rail4V HSDI diesel engine with a dual intake port concept with seat swirl chamfers.6The piezo-actuated injector is located verti-cally at the centre of the fire deck and has a nozzle tip with eight evenly distributed holes.The engine specifi-cations are summarized in Table1.Detailed descrip-tions of the experimental setup can be found in Adolph et al.6The gas exchange process is optimized by using an intake port concept consisting of a filling and a tangen-tial port,both with seat swirl chamfers.6One important goal of this port concept is to provide a high volumetric efficiency by an optimized flow coefficient.Figure1 shows the flow measurements of the port design per-formed on a steady-state flow test bench.T able1.T est engine specification data.Bore(mm)75Stroke(mm)88.3Squish height(mm)0.7 Compression ratio15.1Fuel injection system Bosch2000barpiezo-actuated No.of nozzle hole8Nozzle hole diameter(m m)113Spray angle(°)153Intake valve opens(°CA ATDC)2355Engine control Bosch EDC16Rezaei et al.483At low valve lifts,an increase of the swirl ratio due to the effect of the swirl chamfers is visible.The use of the seat swirl chamfers on both intake valves ensures a higher swirl ratio and charge motion at low valve lifts. Using this port concept in combination with a variable intake-valve-lift system enables us to adapt the swirl ratio at each operating point.Four different maximum valve lifts from3.2mm to 8.0mm are investigated.The intake valve opening (IVO)and IVC times are kept constant while the maxi-mum valve lift is varied.The experimental results of two important operating points22801/min,9.4bar indicated mean effective pres-sure(IMEP)and15001/min,4.3bar IMEP6are intro-duced briefly in this section.These two operating points are selected for the numerical analysis because they are most frequently used in the new European driving cycle (NEDC)and federal test procedure(FTP)75cycles, and are therefore significant for the total engine-out emissions of these cycles.Start of injection is kept con-stant and exhaust gas recirculation(EGR)rate is var-ied.More discussion and comparison with CFD results will be given in the following sections.Figure2illustrates that the reduction of the intake valve lift from8.0mm to4.8mm significantly reduces smoke emission without any significant impact on fuel consumption for the15001/min operating point.A fur-ther reduction of the valve lift to3.2mm leads to an increase of gas exchange losses,and results in an increased fuel consumption without any improvement of the soot emissions.Another strategy to maintain an increased swirl ratio is the filling port deactivation in combination with a dual8.0–8.0mm valve lift(8.0mm port deactivation(PD)).In this case,both the soot emis-sions are deteriorated with a negative impact on fuel consumption compared to the4.8–4.8mm valve lift.The experimental investigations on the single-cylinder test engine for the operating point22801/min, 9.4bar IMEP are summarized in Figure3.It can be observed in the figure that reducing the maximum valve lift from8.0mm to4.8mm slightly improves the soot emissions.The8.0mm PD valve strategy produces a high amount of soot emissions similar to the8.0mm Figure2.Experimental observations of the effect of increasing the swirl ratio on emissions and fuel consumption,n=15001/min,IMEP=6.8bar.6Reprinted with permission from SAE paper2009-01-0653Ó2009SAE International.ISFC: indicated specific fuel consumption;FSN:fuel smokenumber. Figure3.Experimental observation of the effect of increasing the swirl ratio on emission and fuel consumption,n=22801/min, IMEP=9.4bar.6Reprinted with permission from SAE paper2009-01-0653Ó2009SAE International.FSN:fuel smoke number.Figure1.Stationary measured flow coefficient and swirl ratio.484International J of Engine Research13(5)dual valve lift.The reasons will be discussed in the next sections.Simulation procedure and numerical modelsIn this study,the KIVA-3V release2code,7with further developments by the Engine Research Center of Wisconsin University,8is used for injection and combus-tion simulation.Due to the availability of the source code,this is used for implementation of the one-equation LES approach.Additionally,the code is extended by further soot and NO x emission models.However,the capability of the code to fully resolve complex geometries is limited.Therefore,in this work,the software STAR-CD/ES-ICE is combined with the KIVA code in order to simulate in-cylinder charge motion generation,includ-ing the complete engine geometry.In-cylinder intake and compression simulation are carried out with the STAR-CD software.The simulated in-cylinder flow field is then mapped onto a mesh of the KIVA code shortly before start of injection.A schematic of the simulation chain is depicted in Figure4.For in-cylinder flow and combustion simulation, both the RANS model and the LES approach are applied.The renormalization group(RNG)k–e model8 is used in the KIVA code in the case of RANS simula-tion.Time averaging of the Navier–Stokes equations, commonly referred to as‘Reynolds averaging’greatly reduces the computational time,as it filters out the tran-sient spectrum of turbulence,which is modelled instead. Therefore,it is recognized that time-averaging models do not have the ability to capture cycle-to-cycle varia-tions in the flow field inside the combustion chamber accurately.More details about the RANS approach,its applications and limitations are given,for example,by Launder and Spalding9and Bardina et al.10The LES approach is a numerical technique to close the equations of turbulent flows by using spatial filter-ing.In the LES approach,larger turbulent structures (eddies)are directly calculated in a space-and time-accurate manner,while smaller eddies that are smaller than a filter length are modelled using sub-grid scale (SGS)models.By applying the LES approach,unsteady flow and engine cyclic variations can be resolved,while a RANS simulation averages out many cyclic phenomena.More details can be found,for example,in Sone et al.11In this study,the k-equation SGS model12is imple-mented into the KIVA-3V code.For brevity,the govern-ing equations and model formulation are not given here and can be found in Sone et al.,13with more details.The multi-cycle intake flow simulations in STAR-CD are carried out using the LES Smagorinsky model14 from IVO to30°crank angle(CA)after top dead centre (ATDC).Numerical simulation of injection,combus-tion and emission formation are subsequently carried out by the KIVA-LES code using the simulated in-cylinder flow field from STAR-CD.The applied spray atomization model accounts for combined Kelvin–Helmholtz and Rayleigh–Taylor dro-plet instabilities.8A phenomenological nozzle flow model15considering the nozzle passage inlet configura-tion is used.The commonly so-called‘Shell’five-species autoignition kinetic model16in combination with the characteristic-time combustion model8is used for igni-tion and combustion modelling.For modelling the soot emissions,the simple model of Hiroyasu and Kadota17and a well-validated multi-step soot model developed at the Institute for Combustion Engines at RWTH Aachen University18 are used.The phenomenological soot model is based on the eight-step soot formation of Kazakov and Foster19and a three-step oxidation model.20The gas sampling measurement as well as the LII and Raman laser diagnostic investigations were applied for local and time-dependent validation of the simu-lated gas temperature,as well as concentration of spe-cies like CO,O2and also the predicted soot emission inside the combustion chamber.18Furthermore,for the operating point1500l/min dis-cussed in the following,the applied combustion and soot models are evaluated by experimental results of ignition,flame propagation and local soot formation obtained from the LII and flame light emission measurement techniques,considering different valve strategies.21Figure4.The applied simulation strategy.Rezaei et al.485Boundary conditionsThe intake,compression and combustion simulations are carried out for following two operating points: engine speed 22801/min,9.4bar IMEP;engine speed 15001/min,6.8bar IMEP.Different valve strategies are simulated using both the RANS model and the LES approach for both operating points.For the case of 22801/min,the following two valve actuation strategies are simulated: dual opening with 4.8–4.8mm maximum valve lift (4.8–4.8mm);dual opening with 8.0–8.0mm maximum valve lift,but with port deactivation by closing the filling port (8.0mm PD).For each of the above valve actuation strategies 10cycles are simulated using the LES approach.The boundary conditions,such as wall temperatures,are kept the same for each valve strategy during the multi-cycle simulations.Figure 5shows the measured intake pressure curves and its standard deviation of 10cycles with 4.8–4.8mm maximum valve lift,which are directly applied as boundary conditions to the CFD simulations.There are very small perturbations in the measured intake pressure boundary conditions.These perturbationsfinally cause stochastic variations in the in-cylinder flow structures on the microscopic and intermediate length and time scales.The result are cycle-to-cycle variations,for example,in terms of the flow in-homogeneity.In the LES approach,such small perturbations and fluc-tuations in flow turbulence are not filtered out as in the RANS model.Furthermore,previous work 6showed that flow structures predicted by LES approaches exhi-bit much stronger differences between different valve actuations strategies than RANS.This makes the LES an appropriate model for simulation and analysis of transient phenomena like engine cycle-to-cycle variability.The second operating point investigated in this work is 15001/min with 6.8bar IMEP.Three valve strategies 8.0–8.0mm,8.0mm PD and 4.8–4.8mm are selected for the numerical investigations applying the RANS and the LES approach.For brevity,only the cycle-averaged intake pressure curves of the three valve stra-tegies are depicted in Figure 6.Computational meshIn this study,a complete mesh consisting of intake and exhaust ports,seat swirl chamfers,piston and a cylinder head for different valve strategies is generated by the ES-ICE software and applied in STAR-CD for intake and compression flow simulation.As depicted in Figure 7(a),the grid used for intake and compression simula-tion extends exactly to the intake pressure sensor loca-tion in order to use the measured intake pressure as boundary conditions of the simulation.A good level of mesh resolution is essential for accu-rate turbulence modelling employing the LES approach.The quality of the computational grid used is evaluated with two different methods,21which are not given here for brevity.For the a priori analysis,the size of the large energy containing eddies obtained by the RANS model is compared with the grid size in the intake ports and the combustion chamber.21It is shown by Rezaei 21that the mesh resolution during the intake phase at some local positions,like near valveregions,Figure 5.Measured and standard deviation of intake pressure boundary condition,4.8–4.8mm,n=22801/min,IMEP=9.4bar.ABDC:after bottom dead centre.Figure 6.Averaged intake pressure boundary condition of all simulated valve strategies,multi-cycle,n=15001/min,IMEP=6.8bar.486International J of Engine Research 13(5)must be improved.As a further evaluation,it is shownthat the amount of the turbulent kinetic energy directly resolved by the LES approach is about 85–90%of the total turbulent kinetic energy in the intake and com-pression phase.21Figure 7(b)illustrates the side and top view of the mesh used in KIVA at the mapping point 230°CA ATDC.A summary of the number of computational cells used in each mesh type is given in Table 2.ResultsTraditionally,charge motion in diesel engines is described by the swirl ratio,which is a global measure obtained by integration of the swirl momentum in the entire combustion chamber.Recent experimental and numerical investigations have shown that in-homogeneity and local differences in the in-cylinder flow structure have noticeable impacts on combustion and emissions.In the following sections,the effects of different flow structures generated by different swirl generation strategies on combustion and hence emis-sion behaviour are described.Evaluation of in-cylinder flow and swirl qualityThe intake pressure is adjusted at the test bench in order to reach the same in-cylinder filling for both cases.For evaluation and assessment of the in-cylinder flow field in HSDI diesel engines,Adolph et al.6introduced the homogeneity of the swirl flow as an additional criterion for quantitative assessment of swirl flow pattern and its homogeneity.Here,we will employ the definition of flow in-homogeneity as proposed in Adolph et al.6The in-homogeneity,I in-hom ,is defined as the root mean square of the tangential in-cylinder flow velocity at each cross-section normalized by the absolute value of the flow-velocity component in the same tangential direc-tion,averaged in the complete in-cylinder volume,I in Àhom =P N sectionsection =1RMS V u ,section A sectionP N sectionsection =1V u ,section A sectionð1Þwhere A section is the area of the considered cross-section,V u ,sec tion and RMS V u ,sec tion are the mean and root mean square of the tangential velocity at the considered cross-section,respectively.In other words,it is a measure of deviation of the in-cylinder swirl from a rigid-body rotation such that for a more in-homogeneous flow field,higher values of the in-homogeneity index are calculated.As already shown in Adolph et al.6(see also Figure 8),applyingFigure 8.In-homogeneity index calculated for the complete combustion chamber,n=22801/min,IMEP=9.4bar:(a)RANS results and (b)LES results.IntakeExhaustSwirl chamferTangentialportIntake pressureFilling port(Closed in case of port deactivation)Figure putation meshes applied in this study.(a)Full geometry mesh used for intake and compression simulation by STAR-CD.(b)Full cylinder mesh applied in the KIVA code for injection and combustion simulation.T able 2.Mesh types and number of computational cells.Mesh type Number of cells STAR-CD 935,000KIVA450,000Rezaei et al.487the RANS and LES models,the variant4.8–4.8mm and the8.0mm PD valve strategies nominally lead to similar global swirl levels,but because of different flow structures,the calculated in-homogeneity indexes are different.The in-homogeneity index for the4.8–4.8mm valve strategy is lower than for8.0mm PD.For brev-ity,only the operating point22801/min is considered.As depicted in Figure8,due to the capability of the LES approach in capturing the unsteady flow and tran-sient phenomena that are not averaged out,as in the RANS model,the cycle-to-cycle variations in the in-cylinder flow homogeneity can be observed.The role of cyclic variation of in-cylinder flow field on combustion and emission behaviour is not discussed here for brev-ity,but is given in Rezaei21with more details,including the experimental investigations.The velocity magnitude at the cross-sectional view at the middle of the bowl,for two operating points 22801/min and15001/min simulated by the RANS model as well as ensemble average results of the LES multi-cycle approach,are plotted in Figure9.As is qualitatively illustrated in Figure9,the velocity distri-bution in a circumferential section shortly before start of injection for the variant8.0mm PD is more in-homogeneous.The effect of in-cylinder flow on combustionand emissionsIn-cylinder swirl flow motion can enhance mixing pro-cesses,may lead to a more homogeneous mixture and,consequently,can improve emission formation. Increasing the swirl ratio(until an optimal value is achieved);for example,by means of combining the seat swirl chamfer and a reduction of the valve lift,leads to a better air–fuel mixing and mixture preparation,which decreases pollutant emissions.Another effect that is studied here in more detail is the in-homogeneity of the swirl flow pattern,which affects the distribution of mixture during the combustion process and hence the emission formation.Numerical investigations of in-cylinder flow show that the variant8.0mm PD has a higher flow in-homogeneity than4.8–4.8mm.A noticeable increase in the soot emissions was observed when comparing both valve strategies at the test bench.6The results of numer-ical investigations of combustion and emission forma-tion are presented in the following sections.RANS modelling.Numerical simulations of injection, combustion and emission formation are carried out using a modified version of KIVA-3V release2.7The two operating points given above are investigated.22801/min,9.4bar IMEP.In order to study only the effect of the in-cylinder flow and to isolate other effects, all other operating conditions,in-cylinder mass,air–fuel ratio,injection system,and so on,are kept the same for both valve strategies,4.8–4.8mm and8.0mm PD in the numerical and experimental investigations. The intake pressure was adjusted at the test bench to get the same in-cylinder air mass for both cases.The measured intake pressures are directly used in the CFD simulations.For the8.0mm PD case,both intake valves have8.0mm maximum lift and the filling port is closed.The operating conditions are summarized in Table3.According to the measured EGR ratio,com-position of the intake air is calculated.Figure10compares the simulated pressure curves and the heat release rate of the operating point22801/ min,9.4bar IMEP employing both the4.8–4.8mm and 8.0mm PD valve strategies with the measurements car-ried out on the single-cylinder test engine.Good agree-ment is found between measured and simulated KIVA results.Figure9.Cross-sectional view of the velocity magnitude at the middle of the bowl:(a)RANS results and(b)ensemble average LES results,n=2280and15001/min.T able3.Engine operating conditions,22801/min,9.4bar IMEP. Engine speed(1/min)2280 Lambda 2.22 EGR rate31% IMEP(bar)9.4 Injected mass(mg)19.6 Rail pressure(bar)970 Start of pilot injection(°CA ATDC)222.5 Start of main injection(°CA ATDC)20.2488International J of Engine Research13(5)The calculated in-cylinder emissions for both valve strategies are plotted in Figure 11.The transient curves of the soot concentration show first of all that the sim-ple one-step soot model of Hiroyasu and Kadota 17fails to detect the observed trend of soot increase between the cases at the end of the exhaust stroke.In contrast,the phenomenological multi-step 18soot mechanism exhibits the difference between the two valve-lift strate-gies from approximately 16°CA ATDC.Furthermore,with this model,it is seen that before that crank angle position the soot concentration for the 4.8–4.8mm valve strategy is predicted to be higher,but thereafter,the oxidation rate of soot is stronger for this strategy.Another possibility to analyse the differences in mix-ture formation is the comparison in terms of airutilization.Air utilization is defined here as the volume fraction of mixture with a relative air–fuel ratio l between 0.0and 2.0.For the 4.8–4.8mm case,higher values of air utilization are calculated.On the other hand,there are only marginal differences in the CO and NO x emissions between both valve strategies.In order to compare the simulated trend in the soot emissions with the observed trend on the test bench,both experimental and numerical investigations are now normalized to the corresponding 4.8–4.8mm valve strategy.Figure 12illustrates the trend of increased soot emissions by applying 8.0mm PD instead of 4.8–4.8mm valve lift,for both CFD predictions and mea-surements of the operating point 22801/min and 9.4bar IMEP.Both analyses show a significant increase in the same direction for soot emissions between the two dif-ferent valve-lift strategies.In order to analyse the mixture formation in a more detailed way,the air utilization is given in Figure 13,considering four different ranges in air–fuel ratio.The difference between the two additional curves shows the volume fraction of the mixture with a lambdavalueFigure 10.Heat release analysis of the CFD simulation using the RANS model in comparison with the engine measurement data at n=22801/min,IMEP=9.4bar.Figure 11.Calculated air utilization and emissions using the RANS model,n=22801/min,IMEP=9.4bar.Rezaei et al.489。
- 1、下载文档前请自行甄别文档内容的完整性,平台不提供额外的编辑、内容补充、找答案等附加服务。
- 2、"仅部分预览"的文档,不可在线预览部分如存在完整性等问题,可反馈申请退款(可完整预览的文档不适用该条件!)。
- 3、如文档侵犯您的权益,请联系客服反馈,我们会尽快为您处理(人工客服工作时间:9:00-18:30)。
S¯a dhan¯a V ol.32,Part5,October2007,pp.597–617.©Printed in IndiaMulti-dimensional modelling of spray,in-cylinder air motion and fuel–air mixing in a direct-injection engine N ABANI1,S BAKSHI2and R V RA VIKRISHNA3Department of Mechanical Engineering,Indian Institute of Science,Bangalore560012∗e-mail:ravikris@mecheng.iisc.ernet.inMs received29September2006;revised10May2007Abstract.In this work,three-dimensional fuel–air mixing inside a conventionalspark ignition engine cylinder is simulated under direct injection conditions.Themotivation is to explore retrofitting of conventional engines for direct injection totake advantage of low emissions and high thermal efficiency of the direct injectionconcept.Fuel–air mixing is studied at different loads by developing and apply-ing a model based on the Lagrangian-drop and Eulerian-fluid(LDEF)procedurefor modelling the two-phaseflow.The Taylor Analogy Breakup(TAB)model formodelling the hollow cone spray and appropriate models for droplet impingement,drag and evaporation are used.Moving boundary algorithm and two-way inter-action between both phases are implemented.Fuel injection timing and quantityis varied with load.Results show that near-stoichiometric fuel–air ratio region isobserved at different locations depending on the load.The model developed servesto predict the fuel–air mixing spatially and temporally,and hence is a useful toolin design and optimization of direct injection engines with regards to injector andspark plug locations.Simulations over a range of speed and load indicate the needfor a novel ignition strategy involving dual spark plugs and also provide guidelinesin deciding spark plug locations.Keywords.SI engine;direct injection;in-cylinder fuel–air mixing;CFD;two-phaseflow.1.IntroductionDirect Injection(DI)of petrol/gasoline has been an emerging and successful technology in lowering emissions and improving the thermal efficiency of engines.Jost(2001)mentions about fuel-stratified injection(FSI)used in a recent engine design of V olkswagen,wherein 1Current address:University of Wisconsin-Madison,USA2Current address:Department of Mechanical Engineering,Indian Institute of Technology, Chennai3Corresponding author597598N Abani,S Bakshi and R V Ravikrishnafuel savings of up to15%and reduction in emissions have been achieved.There is great interest in the potential for retrofitting existing engines for direct fuel injection to take advan-tage of the higher efficiency and lower emissions.It has been widely known that the engine performance and emissions are dependent on the initial fuel vapour distribution in the cylin-der.Hence,the location of the injector,air motion and fuel delivery characteristics become important factors for study.The efficiency of a DI engine depends on the mixture preparation and its distribution inside the chamber.This phenomenon is based on the interaction of the in-cylinder air motion,either tumble or swirl,and the spray characteristics from the high pressure swirl injector which are generally employed for these engines.The location of injector,spark plug,piston geometry, cylinder geometry and injection timing are important criteria in the design of DI engines. These engines have potential to achieve greater fuel economy compared with a diesel engine at partial loads and give better performance than port fuel injection(PFI)spark ignition engines at high loads.The thermodynamic potential for reducing specific fuel consumption (sfc),coupled with the advantages of quicker starting,enhanced transient response,and more precise control of the mixture air–fuel ratio,have resulted in a renewed worldwide interest in DI engines as an automotive power plant.The simplest DI engine system is the one that operates in the early-injection,homogeneous, stoichiometric mode.This engine must utilize throttling for load control,thus the full fuel economy associated with the elimination of throttling will not be realized.Engines operating in this mode however,do experience the thermodynamic advantages of intake charge cooling and rapid starting and fuel cut-off on deceleration.Next level of complexity involves using a leaner homogeneous mixture with reduced throttling for some degree of load control.Making use of the full potential of the DI concept requires a design that generates a stable,stratified mixture using an overall lean air–fuel ratio for operating at part load,and which can undergo a smooth transition to heavy or full load operation by injecting increased volumes of fuel at earlier times in the cycle.In this paper,the objectives are to predict the spatial and temporal distribution of fuel–air mixing inside the cylinder under conditions of direct injection of petrol/gasoline.A three-dimensional model for simulating the two-phaseflow with two-way coupling is developed and applied.Two-way coupling implies that the effect of the surrounding air motion on the droplets,and the effect of the droplets on the air motion are accounted for.Simulations are performed for different load-speed conditions.Based on results of the simulation,a novel ignition methodology involving use of dual spark plugs is suggested.The following sections present details of the engine configuration,the methodology adopted,operating conditions, and results of the simulations.2.Engine configurationThe engine chosen is a conventionalflat-piston design into which gasoline is injected directly through an injector that gives a hollow cone fuel spray.The timing and amount of fuel injection depends on the load at the engine.At low loads,the fuel is injected late,i.e.,in the latter part of the compression stroke so as to create a stratified mixture to save fuel and ensure that the engine continues to run with the benefit that the mixture is near-stoichiometric near the spark plug location as suggested by Iwamoto et al(1997).At high loads,the fuel is injected early in the suction stroke to allow the fuel droplets to mix with the in-cylinder air and create a homogeneous mixture at the end of compression.DI engines usually employ a hollow cone fuel injector.This gives a wider spread of the spray and droplet dispersion.Multi-dimensional modelling of spray in a direct-injection engine599Figure1.Schematic of the computational domain.Since the objective is to explore the retrofitting of conventional engines,aflat piston geom-etry as shown infigure1was chosen.The bore of the cylinder is80mm and the stroke is 88mm.The intake port was a spherical hole of diameter20mm at theflat top cylinder head towards the sidewall and away from the cylinder center.In the present case,the location of fuel injector isfixed and is located near the inlet port with a slight offset distance.The fuel injector is oriented at an angle of45◦with respect to the cylinder axis and is in a plane pass-ing through both the injector and the axis of the cylinder.The layout of the cylinder and the computational domain are also shown infigure1.The solid wall of the cylinder is simulated by specifying a very high value of viscosity,since it is included in the computation domain. The full computation domain was100mm×100mm in x-y plane and20mm at18◦of crank angle in z-direction.The in-cylinder domain in the x-y plane has a dimension of80mm.Total grid size was30×40×40with30cells in z-direction and40each in x-direction and y-direction.The compression ratio of the engine was10·5:1.The computation was performed over324◦of crank angle,starting from18◦ATDC to18◦BTDC.Other details regarding the operating conditions are listed in table1.3.Model description and numerical methodologyThe main objective here is to study the two-phaseflow and the distribution of the fuel-vapour air mixture.A comprehensive three-dimensional model is developed using the Lagrangian-drop and Eulerian-fluid(LDEF)approach.The spray droplets are modelled in a statistical approach where discrete droplets are tracked using a Lagrangian approach.The gas phase is modelled in the Eulerian approach,which is the conventional continuum approach using600N Abani,S Bakshi and R V RavikrishnaTable1.Engine details.Engine and computational detailsCompression ratio10·5Intake pressure(MPa)0·11Intake gas temperature(K)320Temperature inside cylinder at start(K)550Piston surface temperature(K)380Cylinder head temperature(K)380Cylinder liner temperature(K)380Fuel Iso-octaneStart of computation18◦ATDCEnd of computation18◦BTDCStart of suction18◦ATDCEnd of suction9◦ABDCInlet velocity of air during suction(m/s)120Distance between inlet port center and cylinder liner wall(mm)19Distance between fuel injector and the cylinder top head(mm)2Diameter of inlet port30mmFigure2.Mesh generated for the three-dimensional engine simulation.Multi-dimensional modelling of spray in a direct-injection engine601Figure3.At t=0·6ms:(a)Laser sheet image showing spray structure;(b)Predicted spray structure along the same plane as that of the experimental image;(c)Predicted overall spray structure showing locations of all droplet parcels;(d)SMD distribution in the same plane as that of the experimental image.The grayscale bar on the right indicates the SMD in m.Finite V olume Method(FVM).The interaction of the two phases is achieved by coupling the respective mass,momentum and energy conservation equations.The effect of the gas phase on liquid phase is achieved by considering relative terms and gradients for mass,momentum and energy.The transfer of thermal energy is towards the droplet from the surrounding gas phase.With this gain in thermal energy,the droplet temperature increases.At the droplet temperature,the fuel-vapour concentration at the droplet surface is considered saturated. The difference in the concentration at the droplet surface and in the surrounding gas phase causes a concentration gradient.This results in mass transfer from the droplet surface to the surrounding gas phase.Similarly,the transfer of momentum to either of the phase is a two-way phenomenon and the momentum lost by the droplets is gained by the surrounding gas phase and similarly the momentum of the gas phase causes change in momentum of the droplets.The governing equations offlow,the spray model equations and the moving boundary algorithm are not detailed here for want of space;however,a short description is given below.Details of the gas-phase model incorporating the moving boundary algorithm can be found in an earlier work(Bakshi&Ravikrishna2003).602N Abani,S Bakshi and R V RavikrishnaFigure4.At t=1·2ms:(a)Laser sheet image showing spray structure;(b)Predicted spray structure along the same plane as that of the experimental image;(c)Predicted overall spray structure showing locations of all droplet parcels;(d)SMD distribution in the same plane as that of the experimental image.The grayscale bar on the right indicates the SMD in m.3.1Spray modelThe spray model includes tracking of droplets in a Lagrangian manner,which constitute the hollow cone fuel spray.The approach adopted here is a discrete blob approach where set of blobs emerges from the injector as proposed by Han et al(1997).These blobs are placed along the circumference of the injector and are given an initial velocity andflow direction depending on the sheet velocity and swirl angles.The swirlingflow is provided by providing direction cosines based on the tangential and radial angle of the spray.These blobs are subjected to break-up based on the assumption that the blobs oscillate and distort about their equator and break into smaller sizes once the break-up criteria is satisfied. The break-up model implemented was adopted from the works of O’Rourke&Amsden (1987),which is based on Taylor’s Analogy Break-up(TAB)mechanism(1963).Collision and Coalescence is neglected,as its probability in an evaporating environment for a hollow cone fuel spray is minimal since the droplets soon spread over the large area.The blobs are not subjected to drag,as the chunk of liquid mass,which is represented by the blobs,will haveMulti-dimensional modelling of spray in a direct-injection engine603Figure5.Spray tip penetration variation with time.negligible drag and evaporation.However,once the blobs break-up into smaller droplets,the droplets are subjected to drag and evaporation.An impingement model accounting for droplet impingement model on aflat wall has been considered that depends on the wall temperature.The spray impingement is modelled for a high temperature dry wall as found in the works of Senda et al(1994).This considers heat transfer from the wall to the droplet,the breakup behaviour of impinging droplets.To account for the dispersion process of newly formed droplets,impingement model of Naber and Reitz (1988)has been used.3.2Gas phase modelThe mesh generated in the domain of the gas phase simulation is shown infigure2.The gas phase here is the in-cylinder air motion is modelled using an Eulerian approach and is solved for fuel vapour–air mixture.To model the in-cylinder air motion SIMPLE algorithm(Patankar 1980)in conjunction with modification suggested by Doormal and Raithby(1984)is used to solve the coupled conservation equation for three-dimensional compressibleflow.The piston motion is modelled using a moving boundary approach(Ferziger&Peric2003),and a pseudo flow velocity(Heywood1988)approach is used to model theflow through the intake valve. Inclusion of moving boundary approach modified the standard Navier–Stokes equation by the inclusion of the convectiveflux taking into account using the relative velocity of thefluid with respect to the piston motion.The gas phase gains mass as the droplet evaporates.The lost fuel-vapour is added to the corresponding gas phase control volume as a source term in the604N Abani,S Bakshi and R V Ravikrishnacontinuity equation and the species equation.Similarly,the momentum lost by the droplet is fed to the corresponding control volume as the rate of change of the droplet momentum in that control volume.Since combustion is not modelled here,energy coupling is not implemented, as its effect will be very minimal.3.3Model validationThis section describes the validation of the two-phase model with experiments from literature (Xu&Markle1998).For the hollow cone spray,an outwardly opening injector with no sac volume is selected.The massflow rate through this type of injectors is fairly constant throughout the injection pulse.These injectors give veryfine drop sizes and hence,shorter penetration.A square waveform is assumed for the injection,i.e.,a constant massflow rate.A mass of14mg of gasoline was injected for1·3ms at a rail pressure of10MPa.This section describes the validation of our model predictions for the spray by comparing with experimental laser sheet images of the spray from the work of Xu&Markle(1998).The scanned laser sheet images are compared with model predictions along a single plane.These comparisons are shown infigures3and4.Eachfigure has four parts,a,b,c and d.Part(a)is a laser sheet image of the spray structure in a plane passing through the injector,from the experiments of Xu and Markle(1998).Part(b)is the spray structure from the simulation performed in a plane passing through the axis of the spray and the injector.Part(c)represents the overall spray structure showing locations of all parcels and part(d)shows the predicted variation of SMD of the spray in a plane passing through the axis of the spray.This plane is the same as the one corresponding to the experimental data in Part(a).Figure6.Case1:Distribution of equivalence ratio in a plane near cylinder head at18◦BTDC.Multi-dimensional modelling of spray in a direct-injection engine605Figure7.Case1:Distribution of equivalence ratio in a plane near the piston surface at18◦BTDC. Figure3is at0·60ms after the start of injection(SOI).The smaller droplets are observed to move towards center of the spray due to the gas velocity induced by the spray.The larger droplets continue to travel in the original direction.This is clear from the SMD information in part(d)of the samefigure.Experimental results in part(a)show similar trends.Since the laser light intensity is proportional to the droplet size,the very low signal in the central core region indicates very small or no droplets.This is in agreement with predictions as observed from the SMD information in part(d)of the samefigure.This is evident from the spray structureFigure8.Case1:Distribution of equivalence ratio in the central plane at18◦BTDC.606N Abani,S Bakshi and R V RavikrishnaFigure9.Case1:Overall view of the spray indicating all droplet positions inside the cylinder at 137·8◦ATDC.in part(b).Thus,experimental results seem to confirm predictions regarding spray structure. The spray shown in part(c)is observed to be a cone-shaped structure.At1·2ms after SOI,the structure and shape remains same as shown infigures4(b)and(c) with the smaller droplets dispersing more at the corners of the spray giving a mist like appearance and a more evident toroidal shape in the central region.Again,the SMD plot in part(d)shows that the predicted SMD is low in the central region with the experi-mental image in part(a)also showing a corresponding low intensity of laser light in this region.Figure5shows the comparison of predicted spray tip penetration with the experi-ment.The experimental spray tip penetration is based on a certain minimum light intensity at the spray tip which normally accounts for95%of total fuel mass at a given instant.The corresponding numerically spray tip penetration at any instant of time is determined based on the penetration of the95%of the total fuel mass injected till that time.The initial part compares well.Overall,the comparison is favourable,although there is a slight discrep-ancy towards the later stage.This is because the penetration is greatly dependent on theFigure10.Case1:Overall view of the spray indicating all droplet positions inside the cylinder at 73·0◦ABDC.induced gas motion and the actual droplet size which may slightly differ from the predicted size.3.4Simulation detailsAfter validation,the aforementioned model was applied to simulate different operating con-ditions of the engine configuration mentioned earlier.For high and medium loads,the fuel is injected earlier so as to have a near-homogeneous mixture by allowing droplets to mix with air completely and for low loads,fuel is injected late during compression stroke so as to stratify the mixture with less fuel and the near-stoichiometric region becomes the point of ignition. The injection pressure is kept constant and mass of fuel injected is varied depending upon the load.However,the fuel injection rate is maintained constant in all cases.This implies thatthe structure of the hollow cone spray sheet is similar for all cases.The cases are chosen atFigure11.Case2:Distribution of equivalence ratio in a plane near cylinder head at18◦BTDC. different operating conditions based on load and speed in order to cover the engine-operating envelope.In the following sections,the various cases studied are presented with results show-ing the fuel-vapour concentration distribution inside the cylinder at different crank angles. The study here is based on different load and speed with engine geometry remaining the same,i.e.flat-piston andflat cylinder head geometry in all cases.The loads speed and the mass of fuel injected are chosen so as to cover the high,mid and low operating points.Table2 lists the different operating conditions for which the simulations were performed.Table2.Different operating conditions of the engine.Fuel injected OverallCases Speed(rpm)(mg)equivalance ratio Start of injectionCase-14000441·090◦ATDCCase-21000441·090◦ATDCCase-33000330·7590◦ATDCCase-43000220·590◦BTDCCase-5800180·460◦ABDCFigure12.Case2:Overall view of the spray indicating all droplet positions inside the cylinder at 134◦ATDC.4.Results and discussionAlthough the computations give a complete picture of the interaction of the spray with the in-cylinder air-motion as a function of crank angle,results presented here are in terms of the location of near-stoichiometric region in all cases,which can be the possible location of the spark-plug for combustion initiation.In other words,results presented here can serve as a tool in deciding the location of the spark plug.A usual strategy for high load in GDI engines is to inject the fuel early during the intake stroke with overall stoichiometric orslightly rich fuel–air mixture.This allows the droplets to penetrate the entire cylinder andFigure13.Case4:Distribution of equivalence ratio in a plane near cylinder head at18◦BTDC. fuel vapour to mix better with the air.The desired fuel vapour distribution for high load at the end of compression is to have a homogeneous stoichiometric or slightly rich mixture. This is because for the high load,a homogeneous near-stoichiometric mixture will lead to combustion with less emission and provide the required high mean effective pressure (MEP)for the load requirement.For low loads,the overall mixture is lean as the required MEP is not high.With injecting late,i.e.during the compression stroke,the fuel vapour is stratified,as the fuel vapour does not have sufficient time to mix with the in-cylinder air.In the stratified mode,soot formed due to presence of rich mixture zones in the stratified mixture, will get burned due to the excess air surrounding the vapour–air mixture.Cases-1and2 represent the high load case with maximum fuel injected to obtain overall equivalence ratio as stoichiometric.Case-1is at high speed of4000rpm and Case-2is operated at a low speed of 1000rpm.In Case-1,we study the fuel–air mixing at high load and high speed,i.e.,overall equivalence ratio of1·0and speed of4000rpm.In this analysis,44mg of fuel was injected at a crank angle of90◦ATDC.The duration of injection was2·6ms and the injection pressure was assumed to be60bars.The droplets are observed to impinge on to the piston surface.This is because the inlet air temperature is low and hence,the droplet vaporization is not substantial.Figure14.Case4:Overall view of the spray indicating all droplet positions inside the cylinder at 25·2◦ABDC.Figure6shows distribution of mixture equivalence ratio near the cylinder head at18◦BTDC. This distribution is in a plane1mm below the cylinder head surface and will be the plane of investigation for other cases as well in this work.The charge distribution is stratified and the mixture is observed to be stoichiometric to slightly fuel-rich in a narrow stretching from one end of the cylinder to the other.Figure7shows the distribution in a plane near the piston surface.The distribution shows rich mixture near the piston surface.This is because,most of the droplets impinge on the piston surface and the resulting break-up and evaporation of droplets causes this region to be fuel-rich.Figure8shows the distribution of equivalence ratioin a plane passing through the cylinder axis,injector and the axis of inlet port.This planeFigure15.Case5:Distribution of equivalence ratio in a plane near cylinder head at18◦BTDC. will be referred to as the central plane in all subsequent discussions.The equivalence ratio distribution in the central plane shows that the fuel-vapour is stratified and continues to mix with the in-cylinder air.The overall view of spray structure at a crank angle of137·8◦ATDC is shown infigure9.It is observed that most of the droplets continue to impinge on the piston surface.Some droplets are observed to be trapped in a vortex in the upper-left corner of the cylinder.Fromfigure10,it is observed that at a later crank angle,the tumble motion of the air drives the droplets,which is intense at this speed.The droplets continue to travel along the cylinder boundary and evaporate simultaneously.The smaller droplets evaporate faster. Next,we consider the case where the engine experiences high load at low speeds,equivalent to a situation when the vehicle climbs a steep incline.The engine speed has been selected as1000rpm and the overall equivalence ratio( )is assumed to be stoichiometric.The fuel is injected at a crank angle of90◦ATDC.Again,the pressure of injection is maintained at60bars as in the previous case and the fuel is injected for2·6ms.Figure11shows the predicted fuel vapour distribution near the cylinder head at18◦BTDC,expressed in terms of equivalence ratio.The mixture is in a stratified form near the cylinder head.The maximum equivalence ratio is observed to be3near the right extreme of the cylinder head periphery. The near-stoichiometric mixture region is again formed in a narrow band stretching across the cylinder as shown by the =1·0contour.The structure of this region is similar to that in Case-1,except that in Case-1,it was moving away from the injector whereas in Case-2,it is moving towards the injector.Thus,the near-stoichiometric region seems to be in the same location in Case-1and Case-2.Figure16.Case5:Overall view of the spray indicating all droplet positions inside the cylinder at 111·6◦ABDC.Since most of the droplets impinge on to the piston wall as observed infigure12at 134◦ATDC,the droplets and vapour continue to travel along the cylinder surface to reach back to the cylinder head.Hence,the mixing is not sufficient enough to obtain a homo-geneous near-stoichiometric condition.The deflection of spray from its original direction and the effect of the tumble motion on the spray can be easily visualized infigure12. The smaller droplets have already evaporated and the mixing of vapour and air continues, though the maximum concentration remains near the walls signifying poor mixing in this region.Next,Case-3is simulated which involves medium load and medium speed and an overall equivalence ratio of0·75.The engine speed considered here is3000rpm.The fuel is injectedat a crank angle of90◦ATDC.Other injection details are same as in the previous case.Themaximum equivalence ratio is around0·85near the cylinder head as shown infigure13, which also shows the -contours in a plane near the cylinder head.There is no stoichiometric mixture near the cylinder head.In this case,the overall equivalence ratio was around0·75, and hence the mixture equivalence ratio is low near the cylinder head.This is also due to early injection of fuel that allows the fuel droplets to mix more and cause a near-homogeneous mixture.This suggests that by reducing the effective timing of mixing of fuel droplets with in-cylinder air,we can create more stratified charge and can obtain at least a certain portion with near-stoichiometric mixture.Hence,in this case,more delay in injection could have resulted in portion of region near cylinder head to be near-stoichiometric.Figure14shows that at around25·2◦ABDC,the tumble motion drives the spray structure after impingement and the droplets travel along the cylinder boundary.A corner vortex is again observed as in earlier cases with some droplets getting trapped in it.Since the speed is more than the previous case,the droplets do not have sufficient time to travel all the way back to the cylinder head and many droplets impinge on the piston surface.The conditions discussed so far represented high and medium loads at different engine speeds.In all the conditions so far,the fuel was injected early into the suction stroke of the engine.However,the engine at low loads and at idling responds differently and hence the fuel-injection strategy needs to be different.In case of low loads,the strategy is to inject the fuel late during compression stroke and get a stratified mixture with a near-stoichiometric ratio near the spark plug.In this particular case(Case-4),engine experiences low load at medium speed and is operated very lean at =0·5.The engine speed selected is3000rpm.The fuel is injected at68·4◦ABDC,i.e.,during the compression stroke.In this case,the maximum equivalence ratio obtained near the cylinder head is around 2·0,which is near the fuel injector itself.This is observed fromfigure15,which shows the -contours near the cylinder head at a crank angle of18◦BTDC.The near-stoichiometricmixture is observed to be in a circular region surrounding the inlet port.The maximum equivalence ratio inside the whole cylinder domain is around3·5.This signifies that the mixture is extremely stratified.Unlike in previous cases,the near-stoichiometric mixture is located not in the region opposite to the port area of the cylinder head,but in a diametrically-opposite region.This is because the tumble intensity is low compared to previous cases and this affects the droplet motion and vapour mixing.Thus,with the low intensity tumble motion at this stage,the droplets do not penetrate the other side of the cylinder head.This also leads to less distortion of spray structure due to low tumble motion as against the previous conditions and hence,more impingement on the piston surface is observed.Figure16represents the overall spray structure at111·6◦ABDC.The spray is observed to cover a larger volume than in the previous cases of early injection.This is because the spray does not get as deflected as in previous cases owing to the weak tumble motion.It is clear from thefigures that the droplets evaporate in a region near the injector location as opposed to the previous cases of early injection where the droplets travel in a loop and evaporate in the region opposite to the injector location.Hence,for the condition of low load and medium speed,the near-stoichiometric mixture is found near the injector itself.In Case-5,we study fuel–air mixing at the idling condition.The engine speed is assumed to be800rpm and the overall equivalence ratio is0·4.The fuel is injected very late at around 60◦BTDC,i.e.,during the later stages of the compression stroke.During idling,the strategy is similar to the previous case,where the fuel is injected late and a stratified distribution with a near-stoichiometric ratio is ensured near the vicinity of spark plug.This ensures running of the engine at idling speed,with a saving in fuel and the overall benefit of low emission as the overall mixture is lean.Figure17shows the concentration contours at18◦BTDC in a plane。