25 years of time series forecasting
forcasting
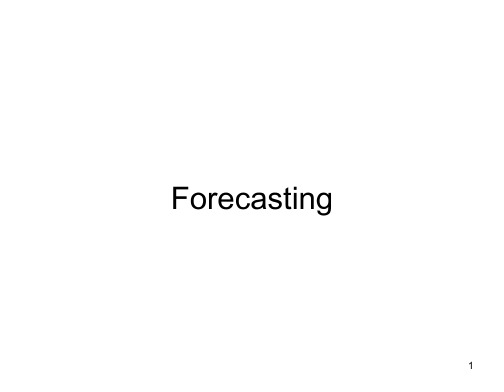
Mo., Qtr., Yr.
© 1984-1994 T/Maker Co.
19
Seasonal Component
• Regular pattern of up & down fluctuations • Due to weather, customs etc. • Occurs within 1 year
17
Time Series Components
Trend Cyclical
Seasonal
Random
18
Trend Component
• Persistent, overall upward or downward pattern • Due to population, technology etc. • Several years duration
– Existing products – Current technology
• Involves intuition, experience
on Internet
• Involves mathematical techniques – e.g., forecasting sales
– e.g., forecasting sales of color televisions
7
Realities of Forecasting
• Forecasts are seldom perfect • Most forecasting methods assume that there is some underlying stability in the system • Both product family and aggregated product forecasts are more accurate than individual product forecasts
预测软件英语作文模板

预测软件英语作文模板英文回答:Forecasting Software。
Forecasting software is a type of software that is used to predict future outcomes based on historical data. It is used in a variety of fields, including business, finance, and meteorology. Forecasting software can be used topredict a wide range of outcomes, including sales, stock prices, and weather conditions.There are a number of different types of forecasting software available. Some of the most common types include:Time series forecasting: This type of forecasting software uses historical data to predict future values of a time series.Regression forecasting: This type of forecastingsoftware uses a regression model to predict therelationship between a dependent variable and one or more independent variables.Machine learning forecasting: This type of forecasting software uses machine learning algorithms to predict future outcomes.Forecasting software can be a valuable tool for businesses and organizations. It can help them to make better decisions about the future by providing them with accurate predictions of future outcomes.中文回答:预测软件。
计量经济学Test-bank-questions-Chapter-5
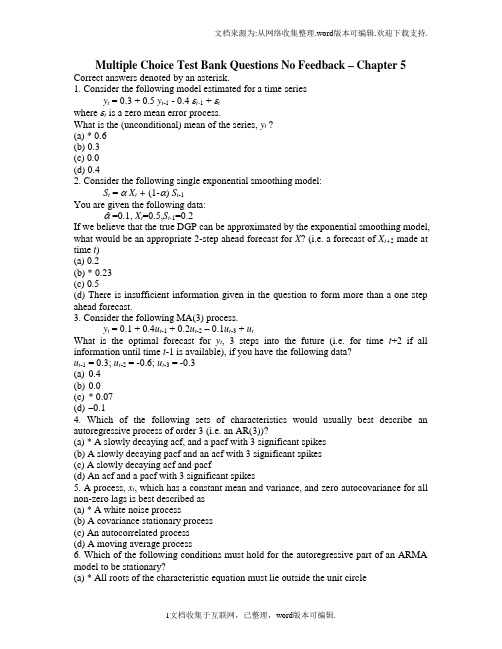
Multiple Choice Test Bank Questions No Feedback – Chapter 5 Correct answers denoted by an asterisk.1. Consider the following model estimated for a time seriesy t = 0.3 + 0.5 y t-1 - 0.4εt-1 +εtwhere εt is a zero mean error process.What is the (unconditional) mean of the series, y t ?(a) * 0.6(b) 0.3(c) 0.0(d) 0.42. Consider the following single exponential smoothing model:S t = α X t + (1-α) S t-1You are given the following data:αˆ=0.1, X t=0.5,S t-1=0.2If we believe that the true DGP can be approximated by the exponential smoothing model, what would be an appropriate 2-step ahead forecast for X? (i.e. a forecast of X t+2 made at time t)(a) 0.2(b) * 0.23(c) 0.5(d) There is insufficient information given in the question to form more than a one step ahead forecast.3. Consider the following MA(3) process.y t = 0.1 + 0.4u t-1 + 0.2u t-2– 0.1u t-3 + u tWhat is the optimal forecast for y t, 3 steps into the future (i.e. for time t+2 if all information until time t-1 is available), if you have the following data?u t-1 = 0.3; u t-2 = -0.6; u t-3 = -0.3(a)0.4(b)0.0(c)* 0.07(d)–0.14. Which of the following sets of characteristics would usually best describe an autoregressive process of order 3 (i.e. an AR(3))?(a) * A slowly decaying acf, and a pacf with 3 significant spikes(b) A slowly decaying pacf and an acf with 3 significant spikes(c) A slowly decaying acf and pacf(d) An acf and a pacf with 3 significant spikes5. A process, x t, which has a constant mean and variance, and zero autocovariance for all non-zero lags is best described as(a) * A white noise process(b) A covariance stationary process(c) An autocorrelated process(d) A moving average process6. Which of the following conditions must hold for the autoregressive part of an ARMA model to be stationary?(a) * All roots of the characteristic equation must lie outside the unit circle(b) All roots of the characteristic equation must lie inside the unit circle(c) All roots must be smaller than unity(d) At least one of the roots must be bigger than one in absolute value.7. Which of the following statements are true concerning time-series forecasting?(i) All time-series forecasting methods are essentially extrapolative.(ii) Forecasting models are prone to perform poorly following a structural break in a series.(iii) Forecasting accuracy often declines with prediction horizon.(iv) The mean squared errors of forecasts are usually very highly correlated with the profitability of employing those forecasts in a trading strategy.(a) (i), (ii), (iii), and (iv)(b) * (i), (ii) and (iii) only(c) (ii), (iii) only(d) (ii) and (iv) only8. If a series, y t, follows a random walk (with no drift), what is the optimal 1-step ahead forecast for y?(a)* The current value of y.(b)Zero.(c)The historical unweighted average of y.(d)An exponentially weighted average of previous values of y.9. Consider a series that follows an MA(1) with zero mean and a moving average coefficient of 0.4. What is the value of the autocorrelation function at lag 1?(a)0.4(b)1(c)*0.34(d)It is not possible to determine the value of the autocovariances without knowing thedisturbance variance.10. Which of the following statements are true?(i)An MA(q) can be expressed as an AR(infinity) if it is invertible(ii)An AR(p) can be written as an MA(infinity) if it is stationary(iii)The (unconditional) mean of an ARMA process will depend only on the intercept and on the AR coefficients and not on the MA coefficients(iv) A random walk series will have zero pacf except at lag 1(a)(ii) and (iv) only(b)(i) and (iii) only(c)(i), (ii), and (iii) only(d)* (i), (ii), (iii), and (iv).11. Consider the following picture and suggest the model from the following list that best characterises the process:(a)An AR(1)(b)An AR(2)(c)* An ARMA(1,1)(d)An MA(3)The acf is clearly declining very slowly in this case, which is consistent with their being an autoregressive part to the appropriate model. The pacf is clearly significant for lags one and two, but the question is does it them become insignificant for lags 2 and 4,indicating an AR(2) process, or does it remain significant, which would be more consistent with a mixed ARMA process? Well, given the huge size of the sample that gave rise to this acf and pacf, even a pacf value of 0.001 would still be statistically significant. Thus an ARMA process is the most likely candidate, although note that it would not be possible to tell from the acf and pacf which model from the ARMA family was more appropriate. The DGP for the data that generated this plot was y_t = 0.9 y_(t-1) – 0.3 u_(t-1) + u_t.12. Which of the following models can be estimated using ordinary least squares?(i)An AR(1)(ii) An ARMA(2,0)(iii) An MA(1)(iv) An ARMA(1,1)(a)(i) only(b)* (i) and (ii) only(c)(i), (ii), and (iii) only(d)(i), (ii), (iii), and (iv).13. If a series, y, is described as “mea n-reverting”, which model from the following list is likely to produce the best long-term forecasts for that series y?(a)A random walk(b)* The long term mean of the series(c)A model from the ARMA family(d)A random walk with drift14. Consider the following AR(2) model. What is the optimal 2-step ahead forecast for y if all information available is up to and including time t, if the values of y at time t, t-1 and t-2 are –0.3, 0.4 and –0.1 respectively, and the value of u at time t-1 is 0.3?y t = -0.1 + 0.75y t-1 - 0.125y t-2 + u t(a)-0.1(b)0.27(c)* -0.34(d)0.3015. What is the optimal three-step ahead forecast from the AR(2) model given in question 14?(a)-0.1(b)0.27(c)-0.34(d)* -0.3116. Suppose you had to guess at the most likely value of a one hundred step-ahead forecast for the AR(2) model given in question 14 – what would your forecast be?(a)-0.1(b)0.7(c)* –0.27(d)0.75。
高考英语《语法填空+阅读理解+应用文写作》真题含答案
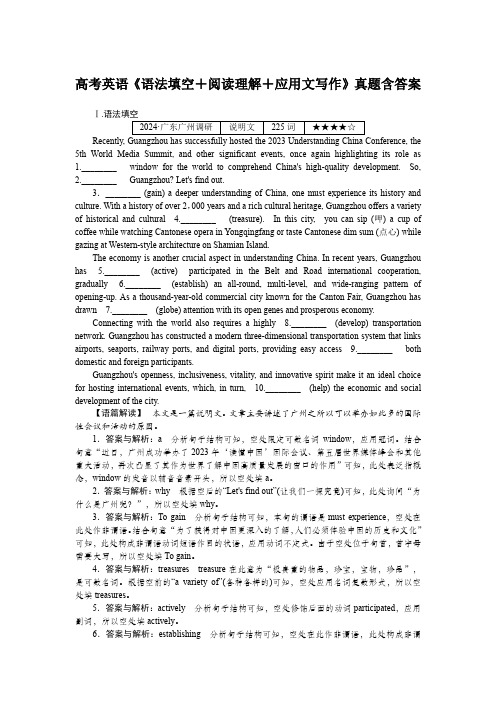
高考英语《语法填空+阅读理解+应用文写作》真题含答案Ⅰ.语法填空5th World Media Summit, and other significant events, once again highlighting its role as1.________ window for the world to comprehend China's highquality development. So,2.________ Guangzhou? Let's find out.3.________ (gain) a deeper understanding of China, one must experience its history and culture. With a history of over 2,000 years and a rich cultural heritage, Guangzhou offers a variety of historical and cultural 4.________ (treasure). In this city, you can sip (呷) a cup of coffee while watching Cantonese opera in Yongqingfang or taste Cantonese dim sum (点心) while gazing at Westernstyle architecture on Shamian Island.The economy is another crucial aspect in understanding China. In recent years, Guangzhou has 5.________ (active) participated in the Belt and Road international cooperation, gradually 6.________ (establish) an allround, multilevel, and wideranging pattern of openingup. As a thousandyearold commercial city known for the Canton Fair, Guangzhou has drawn 7.________ (globe) attention with its open genes and prosperous economy.Connecting with the world also requires a highly 8.________ (develop) transportation network. Guangzhou has constructed a modern threedimensional transportation system that links airports, seaports, railway ports, and digital ports, providing easy access 9.________ both domestic and foreign participants.Guangzhou's openness, inclusiveness, vitality, and innovative spirit make it an ideal choice for hosting international events, which, in turn, 10.________ (help) the economic and social development of the city.【语篇解读】本文是一篇说明文。
Forecasting

ForecastingWhy forecast?Features Common to all Forecasts∙Conditions in the past will continue in the future∙Rarely perfect∙Forecasts for groups tend to be more accurate than forecasts for individuals ∙Forecast accuracy declines as time horizon increasesElements of a Good Forecast∙Timely∙Accurate∙Reliable (should work consistently)∙Forecast expressed in meaningful units∙Communicated in writing∙Simple to understand and useSteps in Forecasting Process∙Determine purpose of the forecast∙Establish a time horizon∙Select forecasting technique∙Gather and analyze the appropriate data∙Prepare the forecast∙Monitor the forecastTypes of Forecasts∙Qualitativeo Judgment and opiniono Sales forceo Consumer surveyso Delphi technique∙Quantitativeo Regression and Correlation (associative)o Time seriesForecasts Based on Time Series Data∙What is Time Series?∙Components (behavior) of Time Series datao Trendo Cycleo Seasonalo Irregularo Random variationsNaïve MethodsNaïve Forecast – uses a single previous value of a time series as the basis of a forecast.Techniques for Averaging∙What is the purpose of averaging?∙Common Averaging Techniqueso Moving Averageso Exponential smoothingMoving AverageExponential SmoothingTechniques for TrendLinear Trend Equationline the of slope at of value pe riod time for fore cast from pe riods time of numbe r spe cifie d =====b ty a ty t t where t t 0:Curvilinear Trend Equationline the of slope at of value pe riod time for fore cast from pe riods time of numbe r spe cifie d =====b ty a ty t t where t t 0:Techniques for Seasonality∙ What is seasonality?∙ What are seasonal relatives or indexes?∙ How seasonal indexes are used:o Deseasonalizing datao Seasonalizing data∙ How indexes are computed (see Example 7 on page 109)Accuracy and Control of ForecastsMeasures of Accuracyo Mean Absolute Deviation (MAD)o Mean Squared Error (MSE)o Mean Absolute Percentage Error (MAPE) Forecast Control Measureo Tracking SignalMean Absolute Deviation (MAD)Mean Squared Error (or Deviation) (MSE)Mean Square Percentage Error (MAPE)Tracking SignalProblems:2 – Plot, Linear, MA, exponential Smoothing5 – Applying a linear trend to forecast15 – Computing seasonal relatives17 – Using indexes to deseasonalize values26 – Using MAD, MSE to measure forecast accuracyProblem 2 (110)National Mixer Inc., sells can openers. Monthly sales for a seven-month period were as follows:(a) Plot the monthly data on a sheet of graph paper.(b) Forecast September sales volume using each of the following:(1) A linear trend equation(2) A five-month moving average(3) Exponential smoothing with a smoothing constant equal to 0.20, assuming March forecast of19(000)(4) The Naïve Approach(5) A weighted average using 0.60 for August, 0.30 for July, and 0.10 for June(c) Which method seems least appropriate? Why?(d) What does use of the term sales rather than demand presume?EXCEL SOLUTION(a) Plot of the monthly dataHow to superimpose a trend line on the graph∙Click on the graph created above (note that when you do this an item called CHART will appear on the Excel menu bar)∙Click on Chart > Add Trend Line∙Click on the most appropriate Trend Regression Type∙Click OK(b) Forecast September sales volume using:(1) Linear Trend Equation∙Create a column for time period (t) codes (see column B)∙Click Tools > Data Analysis > Regression∙Fill in the appropriate information in the boxes in the Regression box that appearsCoded time periodSales dataCoded time period(2) Five-month moving average(3) Exponential Smoothing with a smoothing constant of 0.20, assuming March forecast of 19(000)∙Enter the smoothing factor in D1∙Enter “19” in D5 as forecast for March∙Create the exponential smoothing formula in D6, then copy it onto D7 to D11(4) The Naïve Approach(5) A weighted average using 0.60 for August, 0.30 for July, and 0.10 for JuneProblem 5 (110)A cosmetics manufactur er’s marketing department has developed a linear trend equation that can be used to predict annual sales of its popular Hand & Foot Cream.y t =80 + 15 twhere: y t = Annual sales (000 bottles) t0 = 1990(a) Are the annual sales increasing or decreasing? By how much?(b) Predict annual sales for the year 2006 using the equationProblem 15 (113)Obtain estimates of daily relatives for the number of customers at a restaurant for the evening meal, given the following data. (Hint: Use a seven-day moving average)Excel Solution∙Type a 7-day average formula in E6 ( =average(C3:c9) )∙In F6, type the formula =C6/E6∙Copy the formulas in E6 and F6 onto cells E7 to E27∙Compute the average ratio for Day 1 (see formula in E12)∙Copy and paste the formula in E12 onto E13 to E18 to complete the indexes for Days 2 to 7Problem 17 (113) – Using indexes to deseasonalize valuesNew car sales for a dealer in Cook County, Illinois, for the past year are shown in the following table, along with monthly (seasonal) relatives, which are supplied to the dealer by the regional distributor.(a) Plot the data. Does there seem to be a trend?(b) Deseasonalize car sales(c) Plot the deseasonalized data on the same graph as the original data. Comment on the two graphs.Excel Solution(a) Plot of original data (seasonalized car sales)(b) Deseasonalized Car Sales(c) Graph of seasonalized car sales versus deseasonalized car salesProblem 26 (115) – Using MAD, MSE, and MAPE to measure forecast accuracyTwo different forecasting techniques (F1 and F2) were used to forecast demand for cases of bottled water. Actual demand and the two sets of forecasts are as follows:(a) Compute MAD for each set of forecasts. Given your results, which forecast appears to be the mostaccurate? Explain.(b) Compute MSE for each set of forecasts. Given your results, which forecast appears to be the mostaccurate? Explain.(c) In practice, either MAD or MSE would be employed to compute forecast errors. What factors might leadyou to choose one rather than the other?(d) Compute MAPE for each data set. Which forecast appears to be more accurate?Excel Solution。
Sale Forecasting
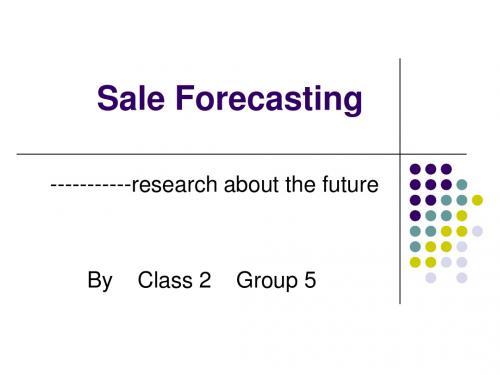
Forecasting options
Executive opinion ------A convenient and inexpensive way to forecast. ------not a scientific technique. Sale force composite ------the sale representatives is the person most familiar with the local market area,especiallly competitive activity (the best position to predict local behavior) ------render subjective predictions and forecasting from a perspective that is too limited.
Forecasting options
Survey of customers ----this method is best for established product. ----For a new product concept ,customers’expections may not adequately indicate their actual behavior. Analysis of market factors ----is used when there is an association between sales and another varible ,called a factor. (For example , new housing starts may be a factor that predicts lumber sales.)
Forecasting
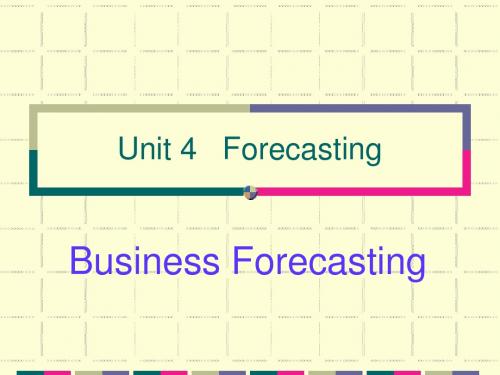
Business Forecaห้องสมุดไป่ตู้ting
The second possibility, management services, suffers from some of the problems of the data processing unit in being remote from the decision taking. Yet when the forecasts are for strategic decisions at board level this solution can be successful.
Business Forecasting
Forecasting is the process of using past events to make systematic predictions about future outcomes or trends. An observation popular with many forecasters is that “the only thing certain about a forecast is that it will be wrong.” Beneath the irony, this observation touches on an important point:
Business Forecasting
Introduction: Structure
The use of forecasting:
Managers must try to predict future conditions so that their goals and plans are realistic.
Time-Series Data AnalysisPPT教学课件
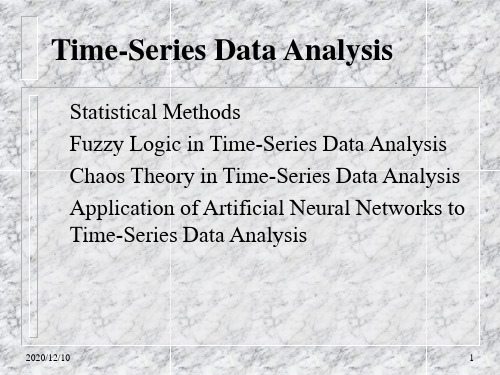
Exponential smoothing---a tool for noise filtering and forecasting
simple exponential smoothing--- the best
model for one-period-ahead forecasting
among 25 time series methods(Markridakis
et la )
2020/12/10
6
Statistical Methods- Exponential smoothing)
formula of the exponential smoothing:
Xt = b + t St = Xt + (1 - )St-1 Where
t: error b: constant
Time-Series Data Analysis
Statistical Methods Fuzzy Logic in Time-Series Data Analysis Chaos Theory in Time-Series Data Analysis Application of Artificial Neural Networks to Time-Series Data Analysis
Trend component --- a long-term change that does not repeat at the time range being considered Seasonal component(seasonality)--- regular fluctuations in systematic intervals. Noise (error)---irregular component
Forecasting

“Prediction is very difficult, especially if it's about the future.”
Nils Bohr
Objectives
• Give the fundamental rules of forecasting
• Calculate a forecast using a moving average, weighted moving average, and exponential smoothing • Calculate the accuracy of a forecast
Actual demand (past sales) Predicted demand
What’s Forecasting All About?
From the March 10, 2006 WSJ:
Ahead of the Oscars, an economics professor, at the request of Weekend Journal, processed data about this year's films nominated for best picture through his statistical model and predicted with 97.4% certainty that "Brokeback Mountain" would win. Oops. Last year, the professor tuned his model until it correctly predicted 18 of the previous 20 best-picture awards; then it predicted that "The Aviator" would win; "Million Dollar Baby" won instead.
Models for Time Series and ForecastingPPT
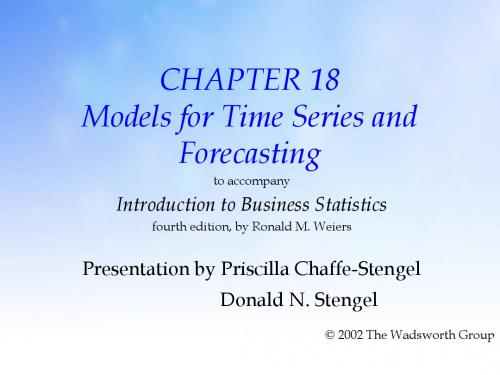
to accompany
Introduction to Business Statistics
fourth edition, by Ronald M. Weiers
Presentation by Priscilla Chaffe-Stengel
© 2002 The Wadsworth Group
? = b0 + b1x y • Linear: ? = b + b x + b x2 • Quadratic: y 0 1 2
Trend Equations
? = the trend line estimate of y y x = time period b0, b1, and b2 are coefficients that are selected to minimize the deviations between the ? and the actual data values trend estimates y y for the past time periods. Regression methods are used to determine the best values for the coefficients.
• Trend equation • Moving average • Exponential smoothing
ቤተ መጻሕፍቲ ባይዱ
• Seasonal index • Ratio to moving average method • Deseasonalizing • MAD criterion • MSE criterion • Constructing an index using the CPI • Shifting the base of an index
Forecasting--预测分析模型
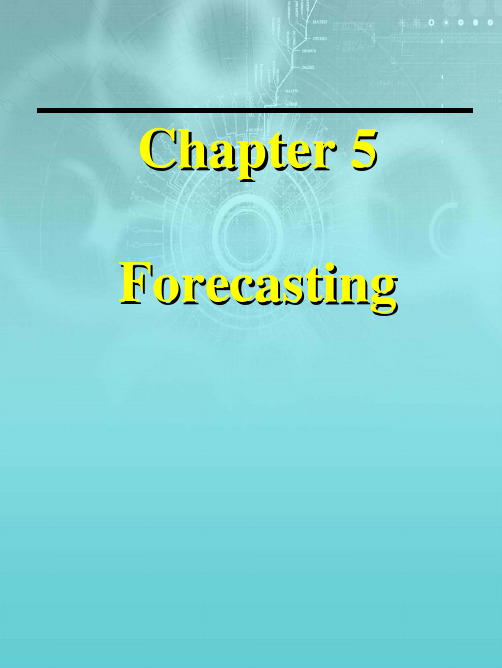
Chapter 5 ForecastingLearning ObjectivesStudents will be able to:1.Understand and know when to usevarious families of forecasting models.pare moving averages, exponentialsmoothing, and trend time-seriesmodels.3.Seasonally adjust data.4.Understand Delphi and otherqualitative decision-makingapproaches.pute a variety of error measures.Chapter Outline5.1Introduction5.2Types of Forecasts5.3Scatter Diagrams and TimeSeries5.4Measures of Forecast Accuracy 5.5Time-Series Forecasting Models 5.6Monitoring and ControllingForecasts5.7Using the Computer to ForecastIntroductionEight steps to forecasting:1.Determine the use of the forecast.2.Select the items or quantities to beforecasted.3.Determine the time horizon of theforecast.4.Select the forecasting model ormodels.5.Gather the data needed to make theforecast.6.Validate the forecasting model.7.Make the forecast.8.Implement the results.These steps provide a systematic way of initiating, designing, and implementing a forecasting system.Types of ForecastsMoving Average Exponential SmoothingTrend Projections Time-Series Methods:include historical data over a time intervalForecasting TechniquesNo single method is superiorDelphi Methods Jury of Executive Opinion Sales Force Composite Consumer Market SurveyQualitative Models :attempt to include subjective factorsCausal Methods:include a variety of factorsRegression Analysis Multiple RegressionDecompositionQualitative MethodsDelphi Methodinteractive group process consisting ofobtaining information from a group ofrespondents through questionnaires andsurveysJury of Executive Opinionobtains opinions of a small group of high-level managers in combination withstatistical modelsSales Force Compositeallows each sales person to estimate thesales for his/her region and then compiles the data at a district or national levelConsumer Market Surveysolicits input from customers or potential customers regarding their futurepurchasing plansScatter Diagrams050100150200250300350400450024681012Time (Years)A n n u a l S a l e sR a d i o sTelevisionsCom p a c t D i s c s Scatter diagrams are helpful whenforecasting time-series data because they depict the relationship between variables.Measures of ForecastAccuracy Forecast errors allow one to see how well the forecast model works and compare that model with other forecast models.Forecast error= actual value –forecast valueMeasures of Forecast Accuracy (continued)Measures of forecast accuracy include: Mean Absolute Deviation (MAD)Mean Squared Error (MSE)Mean Absolute Percent Error (MAPE)= ∑|forecast errors|n= ∑(errors)n=∑actualn100%error2Hospital Days –Forecast Error ExampleMs. Smith forecastedtotal hospitalinpatient days last year. Now that the actual data are known, she is reevaluatingher forecasting model. Compute the MAD, MSE, and MAPE for her forecast.Month Forecast Actual JAN250243 FEB320315 MAR275286 APR260256 MAY250241 JUN275298 JUL300292 AUG325333 SEP320326 OCT350378 NOV365382 DEC380396Hospital Days –Forecast Error ExampleForecastActual|error|error^2|error/actual|JAN 2502437490.03FEB 3203155250.02MAR 275286111210.04APR 2602564160.02MAY 2502419810.04JUN 275298235290.08JUL 3002928640.03AUG 3253338640.02SEP 3203266360.02OCT 350378287840.07NOV 365382172890.04DEC 380396162560.04AVERAGE11.83192.833.68MAD =MSE =MAPE = .0368*100=Decomposition of a Time-SeriesTime series can be decomposed into:Trend (T):gradual up or downmovement over timeSeasonality (S):pattern offluctuations above or below trendline that occurs every yearCycles(C):patterns in data thatoccur every several yearsRandom variations (R):“blips”inthe data caused by chance andunusual situationsComponents of Decomposition-150-5050150250350450550650012345Time (Years)D e m a n d TrendActual DataCyclicRandomDecomposition of Time-Series: Two ModelsMultiplicative model assumes demand is the product of the four components.demand = T * S * C * RAdditive model assumes demand is the summation of the four components.demand = T + S + C + RMoving Averages Moving average methods consist of computing an average of the most recent n data values for the time series and using this average for the forecast of the next period.Simple moving average =∑demand in previous n periodsnWallace Garden Supply’s Three-Month Moving AverageMonth ActualShedSalesThree-Month Moving AverageJanuary10 February12 March13April16 May19 June23 July26(10+12+13)/3 = 11 2/3 (12+13+16)/3 = 13 2/3 (13+16+19)/3 = 16 (16+19+23)/3 = 19 1/3Weighted MovingAveragesWeighted moving averages use weights to put more emphasis on recent periods.Weighted moving average =∑(weight for period n) (demand in period n)∑weightsCalculating Weighted Moving AveragesWeightsApplied PeriodLast month3Two months ago21Three months ago3*Sales last month +2*Sales two months ago +1*Sales threemonths ago6Sum of weightsWallace Garden ’s Weighted Three -Month Moving AverageMonthActual Shed SalesThree-Month Weighted Moving Average101213161923January February March April May June July26[3*13+2*12+1*10]/6 = 12 1/6[3*16+2*13+1*12]/6 =14 1/3[3*19+2*16+1*13]/6 = 17[3*23+2*19+1*16]/6 = 20 1/2Exponential SmoothingExponential smoothing is a type of moving average technique that involves little record keeping of past data.New forecast= previous forecast + α(previous actual –previous forecast)Mathematically this is expressed as:F t = F t-1 + α(Y t-1 -F t-1)F t-1= previous forecast α= smoothing constant F t = new forecastY t-1= previous period actualQtr ActualRounded Forecast using α=0.10 TonnageUnloaded11801752168176= 175.00+0.10(180-175) 3159175 =175.50+0.10(168-175.50) 4175173 =174.75+0.10(159-174.75) 5190173 =173.18+0.10(175-173.18) 6205175 =173.36+0.10(190-173.36) 7180178 =175.02+0.10(205-175.02) 8182178 =178.02+0.10(180-178.02) 9?179= 178.22+0.10(182-178.22)Qtr ActualRounded Forecast using α=0.50 TonnageUnloaded11801752168178 =175.00+0.50(180-175) 3159173 =177.50+0.50(168-177.50) 4175166 =172.75+0.50(159-172.75) 5190170 =165.88+0.50(175-165.88) 6205180 =170.44+0.50(190-170.44) 7180193 =180.22+0.50(205-180.22) 8182186 =192.61+0.50(180-192.61) 9?184 =186.30+0.50(182-186.30)Selecting a SmoothingConstantActualForecast witha = 0.10Absolute DeviationsForecast witha = 0.50Absolute Deviations180175517551681768178101591751617314175173216691901731717020205175301802518017821931318217841864MAD10.012To select the best smoothing constant, evaluate the accuracy of each forecasting model.The lowest MAD results from α= 0.10PM Computer: Moving Average ExamplePM Computer assembles customized personal computers from generic parts. The owners purchase generic computer parts in volume at a discount from a variety of sources whenever they see a good deal.It is important that they develop a good forecast of demand for their computers so they can purchase component parts efficiently.PM Computers: DataPeriod month actual demand1Jan372Feb403Mar414Apr375May456June507July438Aug479Sept56Compute a 2-month moving averageCompute a 3-month weighted average using weights of 4,2,1 for the past three months ofdataCompute an exponential smoothing forecast using α= 0.7Using MAD, what forecast is most accurate?PM Computers: Moving Average Solution2 monthMA Abs. Dev 3 month WMA Abs. Dev Exp.Sm.Abs. Dev37.0037.00 3.0038.50 2.5039.10 1.9040.50 3.5040.14 3.1440.43 3.4339.00 6.0038.57 6.4338.03 6.9741.009.0042.147.8642.917.0947.50 4.5046.71 3.7147.87 4.8746.500.5045.29 1.7144.46 2.5445.0011.0046.299.7146.249.7651.5051.5753.07MAD5.29 5.43 4.95 Exponential smoothing resulted in the lowest MAD.Simple exponential smoothing fails to respond to trends, so a more complex model is necessary with trend adjustment. Simple exponential smoothing -first-order smoothingTrend adjusted smoothing -second-order smoothingLow βgives less weight to morerecent trends, while high βgiveshigher weight to more recent trends.Forecast including trend (FIT t+1) = new forecast (F t ) + trend correction(T t )whereT t = (1 -β)T t-1 + β(F t –F t-1)T i = smoothed trend for period 1T i-1= smoothed trend for the preceding period β= trend smoothing constantF t = simple exponential smoothed forecast for period t F t-1= forecast for period t-1Trend ProjectionTrend projections are used to forecast time-series data that exhibit a linear trend.Least squares may be used to determine a trend projection for future forecasts. Least squares determines the trend line forecast by minimizing the mean squared error between the trend line forecasts and the actual observed values. The independent variable is the time period and the dependent variable is the actual observed value in the time series.Trend Projection(continued)The formula for the trend projection is:Y= b+ b X0 1where: Y = predicted valueb1 = slope of the trend lineb0 = interceptX = time period (1,2,3…n)Midwestern Manufacturing Trend Projection Example Midwestern Manufacturing Company’s demand for electrical generators over the period of 1996 –2000 is given below.Year Time Sales1996174199727919983801999490200051052001614220027122Midwestern Manufacturing CompanyTrend SolutionSUMMARY OUTPUTRegression StatisticsMultiple R0.89R Square0.80Adjusted R Square0.76Standard Error12.43Observations7.00ANOVAdf SS MS F Sign. F Regression 1.003108.043108.0420.110.01 Residual 5.00772.82154.56Total 6.003880.86Coefficients StandardError t Stat P-valueLower95%Intercept56.7110.51 5.400.0029.70 Time10.54 2.35 4.480.01 4.50 Sales = 56.71 + 10.54 (time)MidwesternManufacturing ’s Trend60708090100110120130140150160199319941995199619971998199920002001Forecast pointsTrend LineActual demand lineXy 54.1070.56+=Seasonal Variations Seasonal indices can be used to make adjustments in the forecast for seasonality.A seasonal index indicates how a particular season compares with an average season.The seasonal index can be found by dividing the average value for a particular season by the average of all the data.Eichler Supplies: Seasonal Index ExampleMonthSales DemandAverage Two-Year DemandAverageMonthly DemandSeasonal IndexYear 1Year28010090940.957758580940.851809085940.9049011010094 1.064Jan Feb Mar Apr May11513112394 1.309………………Total Average Demand 1,128Seasonal Index:= Average 2 -year demand/Average monthly demandSeasonal Variationswith TrendSteps of Multiplicative Time-Series Model pute the CMA for each pute seasonal ratio (observation/CMA).3.Average seasonal ratios to get seasonal indices.4.If seasonal indices do not add to thenumber of seasons, multiply each index by (number of seasons)/(sum of the indices).Centered Moving Average (CMA)is an approach that prevents a variation due to trend from being incorrectly interpreted as a variation due to the season.Turner Industries Seasonal Variations with TrendTurner Industries’sales figures are shown below with the CMA andseasonal ratio.Year Quarter Sales CMA Seasonal Ratio1110821253150132 1.1364141134.125 1.051 21116136.3750.851234138.8750.9653159141.125 1.1274152143 1.063 31123145.1250.8482142147.1870.9631684165CMA (qtr 3 / yr 1 ) = .5(108) + 125 + 150 + 141+ .5(116)4Seasonal Ratio = Sales Qtr 3 = 150CMA 132Decomposition Method with Trend and Seasonal Components Decomposition is the process of isolating linear trend and seasonal factors to develop more accurate forecasts.There are five steps to decomposition:pute the seasonal index for eachseason.2.Deseasonalize the data by dividingeach number by its seasonal index.pute a trend line with thedeseasonalized data.e the trend line to forecast.5.Multiply the forecasts by the seasonalindex.Turner Industries has noticed a trend in quarterly sales figures. There is also aseasonal component. Below is the seasonal index and deseasonalized sales data.Yr Qtr Sales Seasonal Index DesasonalizedSales 111080.85127.05921250.96130.2083150 1.13132.7434141 1.06133.019211160.85136.47121340.96139.5833159 1.13140.7084152 1.06143.396311230.85144.70621420.96147.9173168 1.13148.67341651.06155.660*•This value is derived by averaging the season rations for each quarter.Refer to slide 5-37.Seasonal Index for Qtr 1 =0.851+0.848 = 0.8521080.85=Using the deseasonalized data, the following trend line was computed: SUMMARY OUTPUTRegression StatisticsMultiple R0.98981389R Square0.97973154Adjusted R Squar0.9777047Standard Error 1.26913895Observations12ANOVAdf SS MS F ignificance F Regression1778.5826246778.5826246483.37748.49E-10 Residual1016.10713664 1.610713664Total11794.6897613Coefficients Standard Error t Stat P-value Lower 95% Intercept124.780.781101025159.8320597 2.26E-18123.1046 Time 2.340.1061307321.985846048.49E-10 2.0969Sales = 124.78 + 2.34XTurner Industries: Decomposition Method Using the trend line, the following forecast was computed:Sales = 124.78 + 2.34XFor period 13 (quarter 1/ year 4):Sales = 124.78 + 2.34 (13)= 155.2 (before seasonality adjustment) After seasonality adjustment:Sales = 155.2 (0.85) = 131.92Seasonal index for quarter 1Multiple Regression with Trend and Seasonal Components Multiple regression can be used to develop an additive decomposition model.One independent variable is time. Seasons are represented by dummy independent variables.Y = a + b X + b X + b X + b X Where X = time period X = 1 if quarter 2= 0 otherwiseX = 1 if quarter 3= 0 otherwiseX = 1 if quarter 4= 0 otherwise1 12 23 34 41234Monitoring and Controlling Forecasts n |errors forecast |Σ MAD MAD i) period in demand forecast i period in demand Σ(actual MADRSFE gnal TrackingSi =−==whereTracking signals measure how well predictions fit actual data.Monitoring and Controlling Forecasts。
利用Excel进行统计分析-Chapter16-Time Series Forecasting and Index Numbers
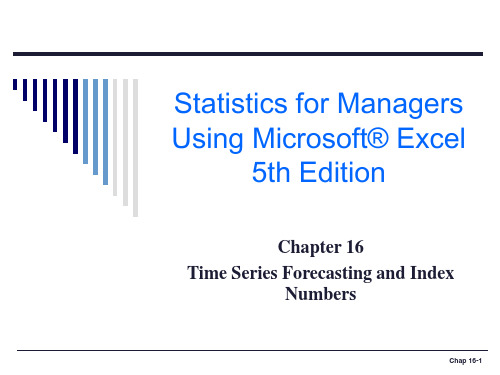
Second average:
Y2 + Y3 + Y4 + Y5 + Y6 MA(5) = 5
Chap 16-15
Example: Annual Data
Year 1 2 3 4 5 6 7 8 9 10 11 etc… Sales 23 40 25 27 32 48 33 37 37 50 40 etc…
1 Cycle Sales
Year
Chap 16-9
Irregular Component
Unpredictable, random, “residual” fluctuations Due to random variations of
Nature Accidents or unusual events
Time-Series Components
Time Series
Trend Component Seasonal Component Cyclical Component Irregular Component
Chap 16-5
Trend Component
Long-run increase or decrease over time (overall upward or downward movement) Data taken over a long period of time
The weight is:
Close to 0 for smoothing out unwanted cyclical and irregular components Close to 1 for forecasting
Chap 16-20
Exponential Smoothing Model
现代物流-英文版测试题-第七章需求管理、订单管理
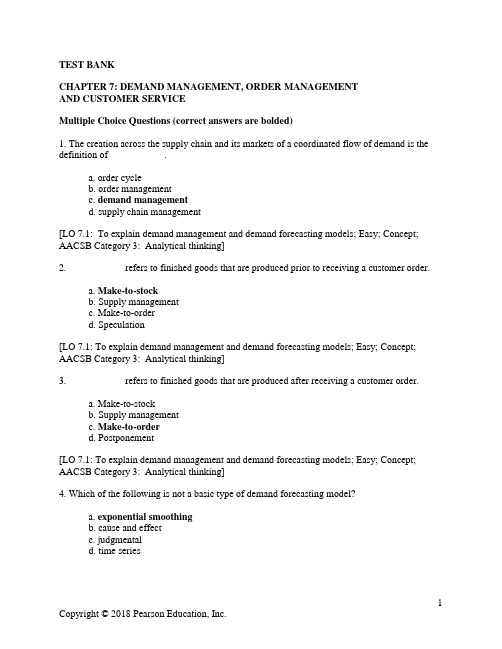
TEST BANKCHAPTER 7: DEMAND MANAGEMENT, ORDER MANAGEMENTAND CUSTOMER SERVICEMultiple Choice Questions (correct answers are bolded)1. The creation across the supply chain and its markets of a coordinated flow of demand is the definition of ___________.a. order cycleb. order managementc. demand managementd. supply chain management[LO 7.1: To explain demand management and demand forecasting models; Easy; Concept; AACSB Category 3: Analytical thinking]2. ___________ refers to finished goods that are produced prior to receiving a customer order.a. Make-to-stockb. Supply managementc. Make-to-orderd. Speculation[LO 7.1: To explain demand management and demand forecasting models; Easy; Concept; AACSB Category 3: Analytical thinking]3. ___________ refers to finished goods that are produced after receiving a customer order.a. Make-to-stockb. Supply managementc. Make-to-orderd. Postponement[LO 7.1: To explain demand management and demand forecasting models; Easy; Concept; AACSB Category 3: Analytical thinking]4. Which of the following is not a basic type of demand forecasting model?a. exponential smoothingb. cause and effectc. judgmentald. time series[LO 7.1: To explain demand management and demand forecasting models; Moderate; Synthesis; AACSB Category 3: Analytical thinking]5. Surveys and analog techniques are examples of ___________ forecasting.a. cause and effectb. time seriesc. exponential smoothingd. judgmental[LO 7.1: To explain demand management and demand forecasting models; Moderate; Application; AACSB Category 3: Analytical thinking]6. An underlying assumption of ___________ forecasting is that future demand is dependent on past demand.a. trial and errorb. time seriesc. judgmentald. cause and effect[LO 7.1: To explain demand management and demand forecasting models; Moderate; Concept; AACSB Category 3: Analytical thinking]7. Which forecasting technique assumes that one or more factors are related to demand and that this relationship can be used to estimate future demand?a. exponential smoothingb. judgmentalc. cause and effectd. time series[LO 7.1: To explain demand management and demand forecasting models; Moderate; Concept; AACSB Category 3: Analytical thinking]8. Which forecasting technique tends to be appropriate when there is little or no historical data?a. exponential smoothingb. judgmentalc. time seriesd. cause and effect[LO 7.1: To explain demand management and demand forecasting models; Moderate; Application; AACSB Category 3: Analytical thinking]9. ___________ suggests that supply chain partners will be working from a collectively agreed-to single demand forecast number as opposed to each member working off its own demand forecast projection.a. Supply chain orientationb. Collaborative planning, forecasting, and replenishment (CPFR) conceptc. Order managementd. Supply chain analytics[LO 7.1: To explain demand management and demand forecasting models; Easy; Concept; AACSB Category 3: Analytical thinking]10. ___________ refers to the management of various activities associated with the order cycle.a. Logisticsb. Order processingc. Demand managementd. Order management[LO 7.2: To examine the order cycle and its four components; Easy; Concept; AACSB Category3: Analytical thinking]11. The order cycle is ___________.a. the time that it takes for a check to clearb. the time that it takes from when a customer places an order until the selling firm receives the orderc. also called the replenishment cycled. also called the vendor cycle[LO 7.2: To examine the order cycle and its four components; Moderate; Concept; AACSB Category3: Analytical thinking]12. The order cycle is composed of each of the following except:a. order retrieval.b. order delivery.c. order picking and assembly.d. order transmittal.[LO 7.2: To examine the order cycle and its four components; Difficult; Synthesis; AACSB Category 3: Analytical thinking]13. Which of the following statements is false?a. Some organizations have expanded the order management concept to include the length of time it takes for an organization to receive payment for an order.b. The order cycle should be analyzed in terms of total cycle time and cycle time variability.c. Order management has been profoundly impacted by advances in information systems.d. Order management is synonymous with order cycle.[LO 7.2: To examine the order cycle and its four components; Difficult; Synthesis; AACSB Category 3: Analytical thinking]14. Order transmittal is ___________.a. the series of events that occurs from the time a customer places an order and the time the customer receives the orderb. the series of events that occurs between the time a customer places an order and the time the seller receives the orderc. the series of events that occurs between the time a customer perceives the need for a product and the time the seller receives the orderd. the series of events that occurs between the time a customer places an order and the time the order cycle begins[LO 7.2: To examine the order cycle and its four components; Moderate; Concept; AACSB Category 3: Analytical thinking]15. In general, there are ___________ possible ways to transmit orders.a. threeb. fourc. fived. six[LO 7.2: To examine the order cycle and its four components; Moderate; Application; AACSB Category 3: Analytical thinking]16. ___________ and electronic ordering are order transmittal techniques that have emerged over the last 30 years.a. In-personb. Mailc. Faxd. Telephone[LO 7.2: To examine the order cycle and its four components; Easy; Application; AACSB Category 3: Analytical thinking]17. What is the second phase of the order cycle?a.order transmittalb.order processingc.order picking and assemblyd.order delivery[LO 7.2: To examine the order cycle and its four components; Moderate; Synthesis; AACSB Category 3: Analytical thinking]18. ___________ refers to the time from when the seller receives an order until an appropriate location is authorized to fill the order.a. Order processingb. Order cyclec. Order managementd. Order transmittal[LO 7.2: To examine the order cycle and its four components; Moderate; Concept; AACSB Category 3: Analytical thinking]19. Classifying orders according to pre-established guidelines so that a company can prioritize how orders are to be filled refers to ___________.a. order fill rateb. order managementc. order processingd. order triage[LO 7.2: To examine the order cycle and its four components; Moderate; Concept; AACSB Category 3: Analytical thinking]20. Order picking and assembly is ___________.a. the final stage of the order cycleb. the most important component of the order cyclec. the order cycle component that follows order processingd. the order cycle component that follows order transmittal[LO 7.2: To examine the order cycle and its four components; Moderate; Synthesis; AACSB Category 3: Analytical thinking]21. The text suggests that ___________ often represents the best opportunity to improve the effectiveness and efficiency of an order cycle.a. order transmittalb. order picking and assemblyc. order deliveryd. order processing[LO 7.2: To examine the order cycle and its four components; Difficult; Synthesis; AACSB Category 3: Analytical thinking]22. Which of the following is not a characteristic of contemporary voice-based order picking systems?a. easily disrupted by other noisesb. better voice qualityc. more powerfuld. less costly[LO 7.2: Order Management; Difficult; Synthesis; AACSB Category 3: Analytical thinking]23. Which of the following is not a benefit of voice-based order picking?a. fewer picking errorsb. improved productivityc. minimal training time to learn the technologyd. fewer employee accidents[LO 7.2: To examine the order cycle and its four components; Difficult; Synthesis; AACSB Category 3: Analytical thinking]24. The final phase of the order cycle is called order ___________.a. picking and assemblyb. deliveryc. receivingd. replenishment[LO 7.2: To examine the order cycle and its four components; Moderate; Synthesis; AACSB Category 3: Analytical thinking]25. The time span within which an order must arrive refers to ___________.a. transit time reliabilityb. order deliveryc. delivery windowd. transit time[LO 7.2: To examine the order cycle and its four components; Moderate; Concept; AACSB Category 3: Analytical thinking]26. A commonly used rule of thumb is that it costs approximately ___________ times as much to get a new customer as it does to keep an existing customer.a. threeb. fourc. fived. six[LO 7.3: To understand the four dimensions of customer service as they pertain to logistics; Moderate; Application; AACSB Category 3: Analytical thinking]27. An unhappy customer will tell ___________ other people about her/his unhappiness.a. sevenb. ninec. twelved. fifteen[LO 7.3: To understand the four dimensions of customer service as they pertain to logistics; Moderate; Application; AACSB Category 3: Analytical thinking]28. The ability of logistics management to satisfy users in terms of time, dependability, communication, and convenience is the definition of ___________.a. customer serviceb. the order cyclec. a perfect orderd. customer satisfaction[LO 7.3: To understand the four dimensions of customer service as they pertain to logistics; Moderate; Concept; AACSB Category 3: Analytical thinking]29. The order cycle is an excellent example of the ___________ dimension of customer service.a. timeb. conveniencec. dependabilityd. communication[LO 7.3: To understand the four dimensions of customer service as they pertain to logistics; Moderate; Application; AACSB Category 3: Analytical thinking]30. The percentage of orders that can be completely and immediately filled from existing stock is the ___________ rate.a. optimal inventoryb. order cyclec. perfect orderd. order fill[LO 7.3: To understand the four dimensions of customer service as they pertain to logistics; Easy; Concept; AACSB Category 3: Analytical thinking]31. What component of customer service focuses on the ease of doing business with a seller?a. convenienceb. dependabilityc. timed. communication[LO 7.3: To understand the four dimensions of customer service as they pertain to logistics; Easy; Concept; AACSB Category 3: Analytical thinking]32. What are multichannel marketing systems?a. channels that have multiple intermediaries between the producer and the consumerb. separate marketing channels that serve an individual customerc. the same thing as horizontal marketing systemsd. channels that combine horizontal and vertical marketing systems[LO 7.3: To understand the four dimensions of customer service as they pertain to logistics; Moderate; Concept; AACSB Category 3: Analytical thinking]33. Objectives should be SMART—that is, ___________, measurable, achievable, realistic, and timely.a. specificb. strategicc. staticd. striving[LO 7.4: To familiarize you with select managerial issues associated with customer service; Moderate; Application; AACSB Category 3: Analytical thinking]34. Which of the following statements is false?a. Goals tend to be broad, generalized statements regarding the overall results that the firm is trying to achieve.a. Objectives are more specific than goals.c. A central element to the establishment of customer service goals and objectives is determining the customer’s viewpoint.d. Objectives should be specific, measurable, achievable, and responsive.[LO 7.4: To familiarize you with select managerial issues associated with customer service; Difficult; Synthesis; AACSB Category 3: Analytical thinking]35. ___________ refers to a process that continuously identifies, understands, and adapts outstanding processes inside and outside an organization.a. Environmental scanningb. Quality managementc. Benchmarkingd. Continuous improvement[LO 7.4: To familiarize you with select managerial issues associated with customer service; Moderate; Concept; AACSB Category 3: Analytical thinking]36. ___________ is the process of taking corrective action when measurements indicate that the goals and objectives of customer service are not being achieved.a. Benchmarkingb. Leadershipc. Controld. Managing[LO 7.4: To familiarize you with select managerial issues associated with customer service; Moderate; Concept; AACSB Category 3: Analytical thinking]37. Which statement about measuring customer service is true?a. Firms should choose those aspects of customer service that are easiest to measure.b. Order cycle time is the most commonly used customer service measure.c. Firms should use as many customer service measures as they can.d. It is possible for organizations to use only one customer service metric.[LO 7.4: To familiarize you with select managerial issues associated with customer service; Difficult; Synthesis; AACSB Category 3: Analytical thinking]38. ___________ refers to the allocation of revenues and costs to customer segments or individual customers to calculate the profitability of the segments or customers.a. Customer profitability analysisb. Net present valuec. Customer lifetime valued. Activity-based costing (ABC)[LO 7.4: To familiarize you with select managerial issues associated with customer service; Easy; Concept; AACSB Category 3: Analytical thinking]39. Which of the following statements is false?a. The service recovery paradox is where a customer holds the responsible company in higher regard after the service recovery than if a service failure had not occurred in the first place.b. A set formula that companies should follow for service recovery exists.c. One service recovery guideline involves fair treatment for customers.d. Service recovery refers to a process for returning a customer to a state of satisfaction after a service or product has failed to live up to expectations.[LO 7.4: To familiarize you with select managerial issues associated with customer service; Difficult; Synthesis; AACSB Category 3: Analytical thinking]True-False Questions1.Demand management is important because efficient and effective supply chains have learnedto match both supply and demand. (True)[LO: Beginning of the chapter material; Moderate; Application; AACSB Category 3: Analytical thinking]2.In make-to-order situations, finished goods are produced after receiving a customer order.(True)[LO 7.1: To explain demand management and demand forecasting models; Easy; Concept; AACSB Category 3: Analytical thinking]3.Simple moving averages and weighted moving averages are examples of judgmentalforecasting. (False)[LO 7.1: To explain demand management and demand forecasting models; Moderate; Application; AACSB Category 3: Analytical thinking]4.Judgmental forecasting is appropriate when there is little or no historical data. (True)[LO 7.1: To explain demand management and demand forecasting models; Moderate; Application; AACSB Category 3: Analytical thinking]5.Forecasting accuracy refers to the relationship between the actual and forecasted demand.(True)[LO 7.1: To explain demand management and demand forecasting models; Easy; Concept; AACSB Category 3: Analytical thinking]6.Demand chain management is where supply chain partners share planning and forecastingdata to better match up supply and demand. (False)[LO 7.1: To explain demand management and demand forecasting models; Moderate; Concept; AACSB Category 3: Analytical thinking]7.In general terms, order management refers to management of the various activities associatedwith the order cycle. (True)[LO 7.2: To examine the order cycle and its four components; Easy; Concept; AACSB Category 3: Analytical thinking]8.The order cycle is usually the time from when a customer places an order to when the firmreceives the order. (False)[LO 7.2: To examine the order cycle and its four components; Moderate; Concept; AACSB Category 3: Analytical thinking]9.There are four possible ways to transmit orders. (False)[LO 7.2: To examine the order cycle and its four components; Moderate; Application; AACSB Category 3: Analytical thinking]10.Order information is checked for completeness and accuracy in the order processingcomponent of the order cycle. (True)[LO 7.2: To examine the order cycle and its four components; Moderate; Synthesis; AACSB Category 3: Analytical thinking]11.The order triage function refers to correcting mistakes that may occur with order picking.(False)[LO 7.2: To examine the order cycle and its four components; Moderate; Concept; AACSB Category 3: Analytical thinking]12.A commonsense approach is to fill an order from the facility location that is closest to thecustomer, with the idea that this should generate lower transportation costs as well as ashorter order cycle time. (True)[LO 7.2: To examine the order cycle and its four components; Easy; Application; AACSB Category 3: Analytical thinking]13.Order processing often represents the best opportunity to improve the effectiveness andefficiency of the order cycle. (False)[LO 7.2: To examine the order cycle and its four components; Moderate; Application; AACSB Category 3: Analytical thinking]14.Travel time accounts for a majority of an order picker’s total pick time. (True)[LO 7.2: To examine the order cycle and its four components; Moderate; Synthesis; AACSB Category 3: Analytical thinking]15.Pick-to-light technology is an order picking technique that has grown in popularity in recentyears. (True)[LO 7.2: To examine the order cycle and its four components; Moderate; Application; AACSB Category 3: Analytical thinking]16.Order retrieval is the final phase of the order cycle. (False)[LO 7.2: To examine the order cycle and its four components; Moderate; Synthesis; AACSB Category 3: Analytical thinking]17.A key change in the order delivery component of the order cycle is that more and moreshippers are emphasizing both the elapsed transit time and transit time variability. (True) [LO 7.2: To examine the order cycle and its four components; Moderate; Application; AACSB Category 3: Analytical thinking]18.It costs about five times as much to get a new customer as it does to keep an existingcustomer. (True)[LO 7.3: To understand the four dimensions of customer service as they pertain to logistics; Easy; Application; AACSB Category 3: Analytical thinking]19.Consumers are demanding about the same levels of service today as in years past. (False) [LO 7.3: To understand the four dimensions of customer service as they pertain to logistics; Moderate; Synthesis; AACSB Category 3: Analytical thinking]20.The increased use of vendor quality-control programs necessitates higher levels of customerservice. (True)[LO 7.3: To understand the four dimensions of customer service as they pertain to logistics; Moderate; Synthesis; AACSB Category 3: Analytical thinking]21.Customer service can be defined as the ability of logistics management to satisfy users interms of quality, dependability, communication, and convenience. (False)[LO 7.3: To understand the four dimensions of customer service as they pertain to logistics; Moderate; Concept; AACSB Category 3: Analytical thinking]22.Dependability consists of consistent order cycles, safe delivery, and complete delivery.(True)[LO 7.3: To understand the four dimensions of customer service as they pertain to logistics; Moderate; Synthesis; AACSB Category 3: Analytical thinking]panies today will not accept slower order cycles in exchange for higher order cycleconsistency. (False)[LO 7.3: To understand the four dimensions of customer service as they pertain to logistics; Moderate; Synthesis; AACSB Category 3: Analytical thinking]24.Order fill rate is the percentage of orders that can be completely and immediately filled fromexisting stock. (True)[LO 7.3: To understand the four dimensions of customer service as they pertain to logistics; Easy; Concept; AACSB Category 3: Analytical thinking]25.Text messaging and the Internet have lessened the need for telephone interaction and face-to-face contact between seller and customer. (False)[LO 7.3: To understand the four dimensions of customer service as they pertain to logistics; Moderate; Synthesis; AACSB Category 3: Analytical thinking]26.The convenience component of customer service focuses on the ease of doing business with aseller. (True)[LO 7.3: To understand the four dimensions of customer service as they pertain to logistics; Easy; Concept; AACSB Category 3: Analytical thinking]27.Today’s customer likes to have multiple purchasing options at her/his disposal, andorganizations have responded by developing hybrid marketing channels—that is, separate marketing channels to serve an individual customer. (False)[LO 7.3: To understand the four dimensions of customer service as they pertain to logistics; Moderate; Concept; AACSB Category 3: Analytical thinking]28.Goals are the means by which objectives are achieved. (False)[LO 7.4: To familiarize you with select managerial issues associated with customer service; Moderate; Concept; AACSB Category 3: Analytical thinking]29.Objectives should be specific, measurable, achievable, realistic, and timely. (True)[LO 7.4: To familiarize you with select managerial issues associated with customer service; Moderate; Synthesis; AACSB Category 3: Analytical thinking]30.Continuous improvement refers to a process that continuously identifies, understands, andadapts outstanding processes found inside and outside an organization. (False)[LO 7.4: To familiarize you with select managerial issues associated with customer service; Moderate; Concept; AACSB Category 3: Analytical thinking]31.Benchmarking should only involve numerical comparisons of relevant metrics. (False) [LO 7.4: To familiarize you with select managerial issues associated with customer service; Moderate; Synthesis; AACSB Category 3: Analytical thinking]32.The nature of the product can affect the level of customer service that should be offered.(True)[LO 7.4: To familiarize you with select managerial issues associated with customer service; Moderate; Synthesis; AACSB Category 3: Analytical thinking]33.A product just being introduced needs a different level of service support than one that is in amature or declining market stage. (True)[LO 7.4: To familiarize you with select managerial issues associated with customer service; Moderate; Synthesis; AACSB Category 3: Analytical thinking]34.Leadership is the process of taking corrective action when measurements indicate that thegoals and objectives of customer service are not being achieved. (False)[LO 7.4: To familiarize you with select managerial issues associated with customer service; Moderate; Concept; AACSB Category 3: Analytical thinking]35.The customer service metrics that are chosen should be relevant and important from thecustomer’s perspective. (True)[LO 7.4: To familiarize you with select managerial issues associated with customer service; Moderate; Synthesis; AACSB Category 3: Analytical thinking]36.It is possible for organizations to use only one customer service metric to measure customerservice. (True)[LO 7.4: To familiarize you with select managerial issues associated with customer service; Easy; Application; AACSB Category 3: Analytical thinking37.Customer profitability analysis explicitly recognizes that all customers are not the same andthat some customers are more valuable than others to an organization. (True)[LO 7.4: To familiarize you with select managerial issues associated with customer service; Moderate; Concept; AACSB Category 3: Analytical thinking]38.Customer profitability analysis is grounded in traditional accounting cost allocation methods.(False)[LO 7.4: To familiarize you with select managerial issues associated with customer service; Moderate; Application; AACSB Category 3: Analytical thinking]39.Poor customer experiences cost U.S. business in excess of $75 billion per year. (False) [LO 7.4: To familiarize you with select managerial issues associated with customer service; Moderate; Synthesis; AACSB Category 3: Analytical thinking]40.In the service recovery paradox, a customer holds the responsible company in higher regardafter the service than if a service failure had not occurred in the first place. (True)[LO 7.4: To familiarize you with select managerial issues associated with customer service; Moderate; Application; AACSB Category 3: Analytical thinking]。
利用Excel进行统计分析-Chapter16-Time-Series-Forecasting-and-Index-Numbers

Time Series Plot
A time-series plot is a twodimensional plot of time-series data
the vertical axis 16.00 measures the variable 14.00
of interest
12.00 10.00
Data taken over a long period of time
Sales
Time
Chap 16-6
Chap 16-7
Seasonal Component
Sales
Short-term regular wave-like patterns Observed within 1 year Often monthly or quarterly
Statistics for Managers Using Microsoft® Excel
5th Edition
Chapter 16 Time Series Forecasting and Index
Numbers
Chap 16-1
Learning Objectives
In this chapter, you learn: About seven different time-series forecasting models:
8.00
the horizontal axis
6.00
corresponds to the
4.00 2.00
time periods
0.00
U.S. Inflation Rate
Year
Chap 16-4
Time-Series Components
时间序列预测流程

时间序列预测流程Time series forecasting is the process of predicting future data points based on previously observed values at regular intervals. It plays a crucial role in various industries, including finance, retail, healthcare, and manufacturing. There are several methods that can be used for time series forecasting, such as autoregressive integrated moving average (ARIMA), exponential smoothing, and machine learning algorithms. Each method has its own strengths and weaknesses, depending on the nature of the time series data and the specific use case.时间序列预测是根据先前观察到的定期间隔数值,预测未来数据点的过程。
它在金融、零售、医疗保健和制造等行业中发挥着关键作用。
可以使用多种方法进行时间序列预测,例如自回归综合移动平均(ARIMA)、指数平滑和机器学习算法。
每种方法都有自己的优点和缺点,取决于时间序列数据的性质和具体用例。
One of the first steps in the time series forecasting process is data collection and preprocessing. This involves gathering historical data, identifying any missing or irregular values, and transforming the datainto a suitable format for analysis. Data preprocessing may also include removing outliers, handling seasonality, and normalizing the data to make it more consistent and easier to work with. Accurate and comprehensive data preprocessing is essential for improving the accuracy of time series forecasting models.时间序列预测过程中的第一步是数据收集和预处理。
Stock_Price_Forecasting_Based_on_the_MDT-CNN-CBAM-
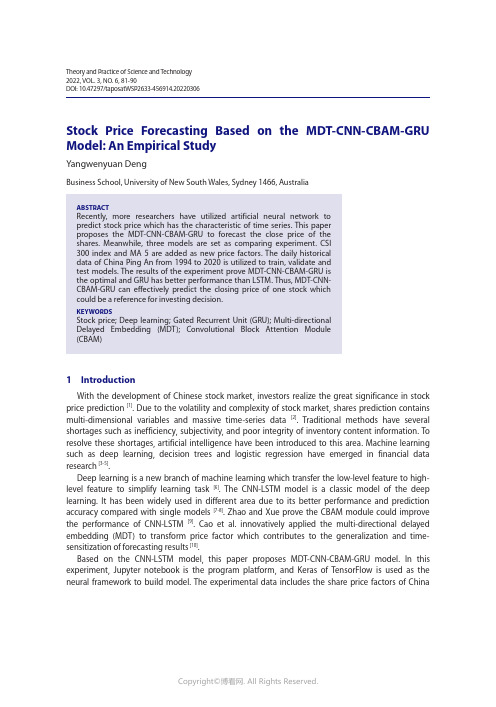
Theory and Practice of Science and Technology2022, VOL. 3, NO. 6, 81-90DOI: 10.47297/taposatWSP2633-456914.20220306Stock Price Forecasting Based on the MDT-CNN-CBAM-GRU Model: An Empirical StudyYangwenyuan DengBusiness School, University of New South Wales, Sydney 1466, AustraliaABSTRACTRecently, more researchers have utilized artificial neural network topredict stock price which has the characteristic of time series. This paperproposes the MDT-CNN-CBAM-GRU to forecast the close price of theshares. Meanwhile, three models are set as comparing experiment. CSI300 index and MA 5 are added as new price factors. The daily historicaldata of China Ping An from 1994 to 2020 is utilized to train, validate andtest models. The results of the experiment prove MDT-CNN-CBAM-GRU isthe optimal and GRU has better performance than LSTM. Thus, MDT-CNN-CBAM-GRU can effectively predict the closing price of one stock whichcould be a reference for investing decision.KEYWORDSStock price; Deep learning; Gated Recurrent Unit (GRU); Multi-directionalDelayed Embedding (MDT); Convolutional Block Attention Module(CBAM)1 IntroductionWith the development of Chinese stock market, investors realize the great significance in stock price prediction [1]. Due to the volatility and complexity of stock market, shares prediction contains multi-dimensional variables and massive time-series data [2]. Traditional methods have several shortages such as inefficiency, subjectivity, and poor integrity of inventory content information. To resolve these shortages, artificial intelligence have been introduced to this area. Machine learning such as deep learning, decision trees and logistic regression have emerged in financial data research [3-5].Deep learning is a new branch of machine learning which transfer the low-level feature to high-level feature to simplify learning task [6]. The CNN-LSTM model is a classic model of the deep learning. It has been widely used in different area due to its better performance and prediction accuracy compared with single models [7-8]. Zhao and Xue prove the CBAM module could improve the performance of CNN-LSTM [9]. Cao et al. innovatively applied the multi-directional delayed embedding (MDT) to transform price factor which contributes to the generalization and time-sensitization of forecasting results [10].Based on the CNN-LSTM model, this paper proposes MDT-CNN-CBAM-GRU model. In this experiment, Jupyter notebook is the program platform, and Keras of TensorFlow is used as the neural framework to build model. The experimental data includes the share price factors of ChinaYangwenyuan Deng 82Ping An 1. This experiment will verify the effectiveness of CBAM module and MDT module. Meanwhile, the performance of GRU is compared with LSTM include the time efficiency and prediction errors. Three evaluation indexes are used to present the prediction results.2 Related WorkRecently, machine learning has become a hot spot in financial areas [11]. Artificial neural network (ANN) has been proved as a feasible tool to forecast complex nonlinear statistics while the time efficiency of neural networks is low [12]. In addition, gradient vanishing and local optimal solution affect the further development of ANN model. Based on ANN, recurrent neural network (RNN) was proposed which would memorize short part information of previous stage [13]. In 2014, gated recurrent unit (GRU) is proposed by Cho et al. as a variant of LSTM [14-15]. LSTM and GRU could address the gradient vanishing issue of RNN.Lecun et al. propose the Convolutional Neural Network in 1988 which is a feedforward neural network to solve time series issues [16-17]. CNN-LSTM is widely used in time financial area and further research have been taken to improve it.The first method to improve model is building more complex models. Wang et al. state the CNN-BiSLSTM model has better forecasting accuracy than CNN-LSTM [18]. Kim T and Kim HY prove that CNN-LSTM model combined with stock price features is more effective [19]. Dai et al. proposed a Dual-path attention mechanism with VT-LSTM which improve the model accuracy [20].Price factors selection and pre-processing is another direction to improve models. Zhang et al. add industry factor as model inputs which contributes to better prediction results [21]. The research of Kang et al. proves the self-attention input contributes smaller prediction error [22]. Yu et al. verified that the amount of training samples affects the effectiveness and accuracy of deep learning models [23].3 MDTThe traditional data processing method for the deep learning is the sliding window method [24]. It divides a time series into multiple consecutive subsequences of length along the time step. The two-dimensional time series matrix will be divided it into multiple fixed-size sub-matrices as the inputs of deep learning.The sliding windows fails to consider the correlations of multidimensional time series. To solve this issue, this paper introduces the multi-directional delayed embedding (MDT) tensor processing technology. Shi et al. combine the MDT method and ARIMA model to prove MDT will improve the accuracy of model [25].MDT method will transform daily stock factor vector x=(x1,x2,…,x n),T∈R n into a Hankel matrix M(x) shown in Figure 1:τ1 China Ping An Insurance (Group) Co., Ltd. (hereinafter referred to as "Ping An",) was born in Shekou, Shenzhen in 1988. It is the first joint-stock insurance enterprise in China, and has developed into an integrated, close and diversified comprehensive financial service group integrating financial insurance, banking, investment and other financial businesses.Theory and Practice of Science and Technology The MDT operation can be represented by following formula:M τ(x )=fold (n ,τ)(Cx )Function fold (n ,τ):R τ×(n -τ+1)→R τ×(n -τ+1)is a folding operator that converts vectors into a matrix. Set the Hankel matrix M τ(x )=(v 1,v 2,…v n -τ+1), where v i represents the number i column vector of the Hankel matrix:vi =(xi ,xi +1,…xτ)T 4 CNN-CBAM-GRU(1) CNNCNN is widely used in time series data prediction because of its good performance and time saving. CNN includes pooling layers which transform the data to reduce the feature dimension:l t =tanh (x t *k t +b t )Where l t represent the output of after convolution neural network, x t represents the input vector, k t represents the weight of the convolution kernel, b t is the convolution kernel bias, and tanh is the activation function.(2) CBAMSanghyun et al. introduce the Convolutional Block Attention Module in 2018 which is a simple and effective module which has been widely used in CNN model [26]. The overview of CBAM is presented in Figure 2:The technological process can be concluded as:F 1=Mc (F )⊗F ,F 2=Ms (F 1)⊗F 1,F represents the input which is intermediate feature map F ∈R C ×H ×W . Mc ∈R C ×1×1is a 1D channel attention map and Ms ∈R 1×H ×W is a 2D spatial attention map. ⊗ is the element-wise multiplication which broadcasts the attention values.Channel attention module compress the spatial feature dimension of the input by utilizing the Figure 1 The transformed Hankel matrixFigure 2 The schematic diagram of CBAM 83Yangwenyuan Deng Avg Pooling and Max Pooling at the same time:Mc (F )=σ(MLP (AvgPool (F ))+MLP (MaxPool (F )))=σ(W 1(W 0(F c avg )))+W 1(W 0(F ))Where W 0∈R cr ×c ,W 1∈r cr ×c . the Spatial Attention Module address the issue of where the efficient information area is by aggregating two pooling operations to generate two 2D maps:Mc (F )=σ(f 7×7([AvgPool (F )]))MLP (MaxPool (F ))=σ(f 7×7[F s avg ,F s max ])(3) GRUGRU merge input gate and forget gat into an update gate to improve the efficiency of training while maintain the model accuracy [27]. GRU has two gate structure which respectively are update gate and reset gate. The overview of GRU is presented in Figure 3:1) r t represent the reset gate which controls the amount of the information needed to be forgotten in previous hidden layer h t -1.2) The update gate Z t control the extent to which the information of previous status is brought into current status h ~t .3) W is the weight matrix, b is the bias vector, [h t -1,x t ] represents the connection of the two vectors. σ and tanh are the sigmoid or hyperbolic tangent functions.The process of GRU could be summarized as follow:Z t =σ(W z ⋅h t -1+W z ⋅x t ),rt =σ(W r ⋅ht -1+W r ⋅x t ),h ~t =tanh (W h ~⋅(r t ⊙h t -1)+W h ~⋅x t ),h t =(1-Z t )⊙h t -1+Z t ⊙h t Where ⋅ represents matrix multiplication, and ⊙ represents matrix corresponding elementmultiplication.Figure 3 Gated Recurrent Unit 84Theory and Practice of Science and Technology (4) CNN-CBAM-GRU training and prediction process1) Standardized inputs: Before the MDT process, data of each column have been processed with Z-score normalization:z i =x i -μσ2) Where μ is the mean, σ is the standard deviation. Then, the normalized data will be transferred to Hankel matrices by MDT.3) Network Initialization: initialize the weights and biases of CNN-CBAM-GRU layers.4) CNN layers: through CNN layers, the key features of Hankel matrices are drawn as the input for later layers.5) CBAM module: The CBAM module will further process the features.6) GRU layers: the processed data are used by GRU to predict the close price.7) Output layer: full connection layers utilize the outputs of GRU to calculate the weight of model.8) Prediction result test and circulation: Judge whether the validation loss reduce after training. Return to step 3 until finish all epochs.9) Saving the best model: If validation loss of this epoch is smaller than the previous stored one, save current model as the best model in the experiment folder.10) Load the best model: load the model structure and weight.11) Prediction and denormalization: utilize the weight of best model to predict the test set close price. The prediction result will be denormalized and compared with true value.12) Experiment result: visualize the result and present the evaluation index results.5 Experiments(1) Experimental EnvironmentA notebook computer, equipped with NVIDIA GeForce GTX 1060 6G and Intel 8750H, implements all experiments. Python 3.9 is the programming language. Anaconda with Jupyter notebook is used as the program platform and Keras built in TensorFlow package construct the neuralnetworkFigure 4 The process of model 85Yangwenyuan Deng structure.(2) Experimental DataChina Ping An price factors is used as experimental data and the close price is the forecasting target. The experimental data contain 6000-day price data from 1994 to 2020 downloaded from the Baostock. Total data is divided into 3 parts: 80% for train set and 10% for both validation set and test set. This paper innovatively takes the CSI 300 index and moving average 5 as price factors. There is total 11 parameters to forecast the close price which is presented in Table 1:(3) Model ImplementationEvery model will independently run for 15 times to find the optimal weights. This paper chooses three evaluation indexes, respectively root mean square error (RMSE), mean absolute error (MAE), and R-square (R2) to evaluate the performance of different models. The formulas of them are calculated as follows:MAE =1n i =1n ||||||y ^i-y i ,RMSE =R 2=1-(∑i =1n (y ^i -y i )2)/n (∑i =1n ()y ^i -y i 2)/n ,Where y ^i represent the prediction value of models and y i is the true value. The closer value of MAE and RMSE to 0 indicates the better performance of model. The close value of R 2 to 1 represent the higher accuracy of model.(4) Implementation of MDT-CNN-CBAM-GRUThe pre-setting parameters of MDT-CNN-CBAM-GRU model are listed in Table 2.6 ResultsThe visual results are presented in Figure 5 to Figure 8. Where the orange line with * represent the prediction value of close price and the blue line represent the true value of close price.The evaluation index results of models are presented in Table 3:The average time for each step training is shown in Table 4:Table 1 Stock price factorsDate94-07Amount 1.165176e+07Volume 1385000Turn 0.51547Index 3.84893Open 0.41541PeTTM 12.58321PbMRQ 2.855036PctChg 3.026634Ma50.410159High 0.422353Low 0.42185886Theory and Practice of Science and TechnologyTable 2 Model parametersParametersConvolution layer filters Convolution layer kernel_size Convolution layer activation function MaxPooling2D pool_sizePooling layer paddingPooling layer activation function Dropout layersCBAM_attention reduce axisGRU layerskernel_regularizerNumber of hidden units in GRU layer 1 Number of hidden units in GRU layer 2 GRU layer activation function Dense layers kernel_initializer Dropout layers 2Learning rateTime_stepLoss functionBatch_sizeOptimizerEpochsValue643Relu2SameRelu0.232L2(0.01)12864Relu Random normal0.250.0011Mean square error64Adam200Figure 5 The prediction of CNN-LSTM8788Yangwenyuan Deng ArrayThe prediction of MDT-CNN-GRUFigure 6 The prediction of MDT-CNN-LSTMFigure 7 Theory and Practice of Science and Technology 7 ConclusionThe MDT-CNN-CBAM-GRU proposed has the optimal forecasting accuracy and satisfied time efficiency, which could provide reference for investors investing in share market.Compared with LSTM, GRU has better prediction accuracy and faster speed. However, here are some details to be improved in further research:(1) If time is enough, 30-time independent training for each model will be a better choice.(2) In further research, more experiment of GRU need to be conducted as the GRU has better performance compared with LSTM.(3) The generalization of models needs to be tested in future research by predicting different financial product such as funds, options and other stocks.About the AuthorYangwenyuan Deng, Master of Commerce in Finance of University of New South Wales, and his research field is Finance & Machine Learning.References[1] Meng, S., Fang, H. & Yu, D. (2020). Fractal characteristics, multiple bubbles, and jump anomalies in the Chinese stock market. Complexity, 2020: 7176598.[2] ABU-MOSTAFA, YS. & ATIYA, AF. (1996). Introduction to financial forecasting. Applied intelligence, 6: 205-213.[3] Huang, QP ., Zhou, X., Wei, Y & Gan, JY. (2015). Application of SVM and neural network model in the stock prediction research. Microcomputer and Application, 34: 88-90.[4] Chen, S., Goo, YJ. & Shen, ZD. (2014). A Hybrid Approach of Stepwise Regression, Logistic Regression, Support Vector Machine, and Decision Tree for Forecasting Fraudulent Financial Statements. The Scientific World Journal, 2014: 968712.[5] Fang, X., Cao, HY. & Li, XD. (2019). Stock trend prediction based on improved random forest algorithm. Journal of Hangzhou Dianzi University, 39: 25-30.[6] Zhang, QY., Yang, DM. & Hang, JT. (2021). Research on Stock Price Prediction Combined with Deep Learning and Decomposition Algorithm. Computer Engineering and Applications, 57: 56-64.[7] Luo, X. & Zhang, JL. (2020). Stock price forecasting based on multi time scale compound depth neural network. Wuhan Finance, 2020: 32-40.[8] Lu, W., Li, J., Li, Y., Sun, A. & Wang, J. (2020). A CNN-LSTM-Based Model to Forecast Stock Prices. Complexity, 2020: 6622927.[9] Zhao, HR. & Xue, L. (2021). Research on Stock Forecasting Based on LSTM-CNN-CBAM Model. Computer EngineeringTable 3 Evaluation index values of different modelsModelCNN-GRUMDT-CNN-LSTMMDT-CNN-GRUMDT-CNN-CBAM-GRU RMSE 0.32200.15980.09100.0890MAE 0.22680.13200.07940.0639R 20.94180.98660.99590.9959Table 4 Average training timeModelCNN-GRUMDT-CNN-GRUMDT-CNN-LSTMMDT-CNN-CBAM-GRU Time 2s 11ms / step 1s 9ms/ step 2s 10ms/ step 2s 10ms/ step 89Yangwenyuan Deng 90and Applications, 57: 203-207.[10] Cao, CF., Luo, ZN., Xie, JX. & Li, L. (2022). Stock Price Prediction Based on MDT-CNN-LSTM Model. ComputerEngineering and Applications, 58: 280-286.[11] Li, J., Pan, S., Huang, L. & Zhu, X. (2019). A machine learning based method for customer behavior prediction.Tehnicki Vjesnik Technical Gazette, 26: 1670-1676.[12] L¨angkvist, M., Karlsson, L. & Loutfi, A. (2014). A review of unsupervised feature learning and deep learning for time-series modeling. Pattern Recognition Letters, 42: 11-24.[13] Sherstinsky, A. (2020). Fundamentals of Recurrent Neural Network (RNN) and Long Short-Term Memory (LSTM)network. Physica D: Nonlinear Phenomena, 404: 132306.[14] Hochreiter, S. & Schmidhuber, J. (1997). Long short-term memory. Neural Computation, 9: 1735–1780.[15] Cho, K., Van Merrienboer, B., Gulcehre, C., Bahdanau, D., Bougares, F., Schwenk, H. & Bengio, Y. (2014). Learningphrase representations using RNN encoder-decoder for statistical machine translation. arXiv, 1406: 1078.[16] Lecun, Y., Bottou, L., Bengio, Y. & Haffner, P. (1998). Gradient based learning applied to document recognition.Proceedings of the IEEE, 86: 2278-2324.[17] Hu, Y. (2018). Stock market timing model based on convolutional neural network – a case study of Shanghaicomposite index. Finance& Economy, 4: 71-74.[18] Wang, HY., Wang, JX., Cao, LH., Sun, Q. & Wang, JY. (2021). A Stock Closing Price Prediction Model Based on CNN-BiSLSTM. Complexity, 2021: 5360828.[19] Kim, T. & Kim, HY. (2019). Forecasting stock prices with a feature fusion LSTM-CNN model using differentrepresentations of the same data. PLOS ONE, 14: 0212320.[20] Dai, YR., An, JX. & Tao, QH. (2022). Financial Time-Series Prediction by Fusing Dual-Pathway Attention with VT-LSTM.Computer Engineering and Applications. 6:10.[21] Zhang, YF., Wang, J. Wu, ZH. & L, YF. (2022). Stock movement prediction with dynamic and hierarchical macroinformation of market. Journal of Computer Applications, 6:7.[22] Kang, RX., Niu, BN., Li, X. & Miao, YX. (2021). Predicting Stock Prices Using LSTM with the Self-attention Mechanismand Multi-source Data. Journal of Chinese Computer Systems,12: 9.[23] Yu, SS., Chu, SW., Chan, YK. & Wang, CM. (2019). Share Price Trend Prediction Using CRNN with LSTM Structure.Smart Science, 7: 189-197.[24] Li, XF., Liang, X. & Zhou, XP. (2016). An Empirical Study on Manifold Learning of Sliding Window of Stock Price TimeSeries. Chinese Journal of Management Science, 24: 495-503.[25] SHI, Q., YIN, J. & CAI, J. (2020). Block Hankel tensor ARIMA for multiple short time series forecasting. Proceedings ofthe AAAI Conference on Artificial Intelligence, 34: 5758-5766.[26] Woo, S., Park, J., Lee, JY. & Kweon, S. (2018). CBAM:convolutional block attention module. Proceedings of theEuropean Conference on Computer Vision (ECCV), 2018: 3-19.[27] Dang, JW. & Cong, XQ. (2021). Research on hybrid stock index forecasting model based on CNN and GRU.Computer Engineering and Applications, 57: 167-174.。
pytorch-forecasting timeseriesdataset格式 -回复

pytorch-forecasting timeseriesdataset格式-回复pytorchforecasting timeseriesdataset格式PyTorchForecasting是一个用于时间序列预测的开源库,它建立在PyTorch深度学习框架之上,并提供了多种模型和方法来处理时间序列数据。
其中,TimeseriesDataset是该库中的一个关键类,用于处理和准备时间序列数据。
本文将详细讨论TimeseriesDataset的格式和用法,以帮助读者更好地理解和使用该类。
首先,让我们从TimeseriesDataset的基本概念开始。
TimeseriesDataset 是PyTorchForecasting中的一个数据集类,用于将时间序列数据转换为可供深度学习模型使用的格式。
它的主要目的是为了简化数据的准备和处理过程,以便在PyTorch中进行训练和预测。
TimeseriesDataset的格式要求如下:1. 时间索引:每个时间序列数据必须包含一个时间索引列,通常是日期或时间戳。
这个时间索引是数据在时间上的顺序,并且应当是递增的。
在构建TimeseriesDataset时,需要传入一个时间索引列的名称。
2. 静态特征:除了时间索引以外,时间序列数据还可以包含静态特征,这些特征在整个时间序列中保持不变。
例如,如果你正在预测销售量,静态特征可以是店铺的面积、位置等。
在构建TimeseriesDataset时,需要传入一个静态特征列表。
3. 动态特征:动态特征是随时间变化的特征,每个时间步都有不同的值。
例如,如果你正在预测销售量,动态特征可以是过去几天的销售数据。
在构建TimeseriesDataset时,需要传入一个动态特征列表。
除了以上三个主要要求,TimeseriesDataset还提供了其他可选参数和功能,以适应不同的时间序列预测任务。
比如,你可以指定训练集和测试集的时间范围、定义滑动窗口的大小、指定目标列等。
pytorch-forecasting timeseriesdataset格式 -回复
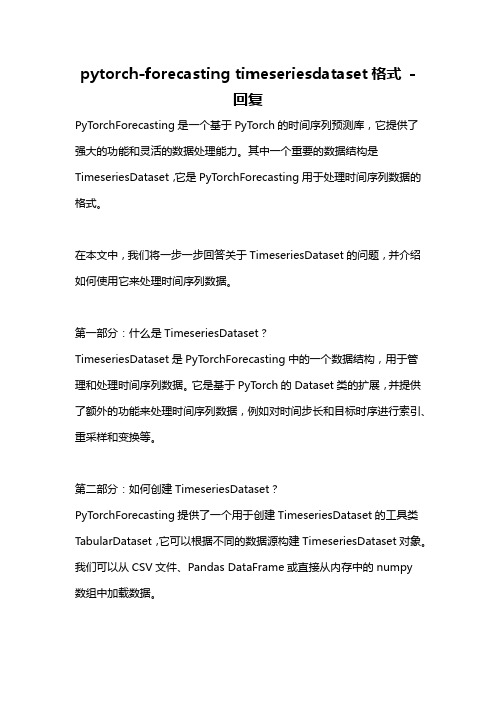
pytorch-forecasting timeseriesdataset格式-回复PyTorchForecasting是一个基于PyTorch的时间序列预测库,它提供了强大的功能和灵活的数据处理能力。
其中一个重要的数据结构是TimeseriesDataset,它是PyTorchForecasting用于处理时间序列数据的格式。
在本文中,我们将一步一步回答关于TimeseriesDataset的问题,并介绍如何使用它来处理时间序列数据。
第一部分:什么是TimeseriesDataset?TimeseriesDataset是PyTorchForecasting中的一个数据结构,用于管理和处理时间序列数据。
它是基于PyTorch的Dataset类的扩展,并提供了额外的功能来处理时间序列数据,例如对时间步长和目标时序进行索引、重采样和变换等。
第二部分:如何创建TimeseriesDataset?PyTorchForecasting提供了一个用于创建TimeseriesDataset的工具类TabularDataset,它可以根据不同的数据源构建TimeseriesDataset对象。
我们可以从CSV文件、Pandas DataFrame或直接从内存中的numpy数组中加载数据。
1. 从CSV文件加载数据使用TabularDataset.from_csv方法可以从CSV文件中加载数据。
需要提供CSV文件的路径、时间索引列的名称以及其中包含的输入变量和目标变量的名称。
可以选择性地指定其他参数,例如转换函数和缺失值处理方法。
2. 从Pandas DataFrame加载数据使用TabularDataset.from_data_frame方法可以基于Pandas DataFrame加载数据。
需要提供一个包含输入变量和目标变量的DataFrame,以及时间索引列的名称。
同样,可以选择性地指定其他参数以进行数据转换和处理。
- 1、下载文档前请自行甄别文档内容的完整性,平台不提供额外的编辑、内容补充、找答案等附加服务。
- 2、"仅部分预览"的文档,不可在线预览部分如存在完整性等问题,可反馈申请退款(可完整预览的文档不适用该条件!)。
- 3、如文档侵犯您的权益,请联系客服反馈,我们会尽快为您处理(人工客服工作时间:9:00-18:30)。
25years of time series forecastingJan G.De Gooijer a,1,Rob J.Hyndman b ,*aDepartment of Quantitative Economics,University of Amsterdam,Roetersstraat 11,1018WB Amsterdam,The NetherlandsbDepartment of Econometrics and Business Statistics,Monash University,VIC 3800,AustraliaAbstractWe review the past 25years of research into time series forecasting.In this silver jubilee issue,we naturally highlight results published in journals managed by the International Institute of Forecasters (Journal of Forecasting 1982–1985and International Journal of Forecasting 1985–2005).During this period,over one third of all papers published in these journals concerned time series forecasting.We also review highly influential works on time series forecasting that have been published elsewhere during this period.Enormous progress has been made in many areas,but we find that there are a large number of topics in need of further development.We conclude with comments on possible future research directions in this field.D 2006International Institute of Forecasters.Published by Elsevier B.V .All rights reserved.Keywords:Accuracy measures;ARCH;ARIMA;Combining;Count data;Densities;Exponential smoothing;Kalman filter;Long memory;Multivariate;Neural nets;Nonlinearity;Prediction intervals;Regime-switching;Robustness;Seasonality;State space;Structural models;Transfer function;Univariate;V AR1.IntroductionThe International Institute of Forecasters (IIF)was established 25years ago and its silver jubilee provides an opportunity to review progress on time series forecasting.We highlight research published in journals sponsored by the Institute,although we also cover key publications in other journals.In 1982,the IIF set up the Journal of Forecasting (JoF ),publishedwith John Wiley and Sons.After a break with Wiley in 1985,2the IIF decided to start the International Journal of Forecasting (IJF ),published with Elsevier since 1985.This paper provides a selective guide to the literature on time series forecasting,covering the period 1982–2005and summarizing over 940papers including about 340papers published under the b IIF-flag Q .The proportion of papers that concern time series forecasting has been fairly stable over time.We also review key papers and books published else-where that have been highly influential to various developments in the field.The works referenced0169-2070/$-see front matter D 2006International Institute of Forecasters.Published by Elsevier B.V .All rights reserved.doi:10.1016/j.ijforecast.2006.01.001*Corresponding author.Tel.:+61399052358;fax:+61399055474.E-mail addresses: j.g.degooijer@uva.nl (J.G.De Gooijer),Rob.Hyndman@.au (R.J.Hyndman).1Tel.:+31205254244;fax:+31205254349.2The IIF was involved with JoF issue 44:1(1985).International Journal of Forecasting 22(2006)443–473/locate/ijforecastcomprise380journal papers and20books andmonographs.It was felt to be convenient to first classify thepapers according to the models(e.g.,exponentialsmoothing,ARIMA)introduced in the time seriesliterature,rather than putting papers under a headingassociated with a particular method.For instance,Bayesian methods in general can be applied to allmodels.Papers not concerning a particular modelwere then classified according to the various problems(e.g.,accuracy measures,combining)they address.Inonly a few cases was a subjective decision needed onour part to classify a paper under a particular sectionheading.To facilitate a quick overview in a particularfield,the papers are listed in alphabetical order undereach of the section headings.Determining what to include and what not toinclude in the list of references has been a problem.There may be papers that we have missed and papersthat are also referenced by other authors in this SilverAnniversary issue.As such the review is somewhatb selective Q,although this does not imply that a particular paper is unimportant if it is not reviewed.The review is not intended to be critical,but rathera(brief)historical and personal tour of the maindevelopments.Still,a cautious reader may detectcertain areas where the fruits of25years of intensiveresearch interest has been limited.Conversely,clearexplanations for many previously anomalous timeseries forecasting results have been provided by theend of2005.Section13discusses some currentresearch directions that hold promise for the future,but of course the list is far from exhaustive.2.Exponential smoothing2.1.PreambleTwenty-five years ago,exponential smoothingmethods were often considered a collection of adhoc techniques for extrapolating various types ofunivariate time series.Although exponential smooth-ing methods were widely used in business andindustry,they had received little attention fromstatisticians and did not have a well-developedstatistical foundation.These methods originated inthe1950s and1960s with the work of Brown(1959,1963),Holt(1957,reprinted2004),and Winters (1960).Pegels(1969)provided a simple but useful classification of the trend and the seasonal patterns depending on whether they are additive(linear)or multiplicative(nonlinear).Muth(1960)was the first to suggest a statistical foundation for simple exponential smoothing(SES) by demonstrating that it provided the optimal fore-casts for a random walk plus noise.Further steps towards putting exponential smoothing within a statistical framework were provided by Box and Jenkins(1970),Roberts(1982),and Abraham and Ledolter(1983,1986),who showed that some linear exponential smoothing forecasts arise as special cases of ARIMA models.However,these results did not extend to any nonlinear exponential smoothing methods.Exponential smoothing methods received a boost from two papers published in1985,which laid the foundation for much of the subsequent work in this area.First,Gardner(1985)provided a thorough review and synthesis of work in exponential smooth-ing to that date and extended Pegels’classification to include damped trend.This paper brought together a lot of existing work which stimulated the use of these methods and prompted a substantial amount of additional ter in the same year,Snyder (1985)showed that SES could be considered as arising from an innovation state space model(i.e.,a model with a single source of error).Although this insight went largely unnoticed at the time,in recent years it has provided the basis for a large amount of work on state space models underlying exponential smoothing methods.Most of the work since1980has involved studying the empirical properties of the methods(e.g.,Barto-lomei&Sweet,1989;Makridakis&Hibon,1991), proposals for new methods of estimation or initiali-zation(Ledolter&Abraham,1984),evaluation of the forecasts(McClain,1988;Sweet&Wilson,1988),or has concerned statistical models that can be consid-ered to underly the methods(e.g.,McKenzie,1984). The damped multiplicative methods of Taylor(2003) provide the only genuinely new exponential smooth-ing methods over this period.There have,of course, been numerous studies applying exponential smooth-ing methods in various contexts including computer components(Gardner,1993),air passengers(Grubb&J.G.De Gooijer,R.J.Hyndman/International Journal of Forecasting22(2006)443–473 444Masa,2001),and production planning(Miller& Liberatore,1993).The Hyndman,Koehler,Snyder,and Grose(2002) taxonomy(extended by Taylor,2003)provides a helpful categorization for describing the various methods.Each method consists of one of five types of trend(none,additive,damped additive,multiplica-tive,and damped multiplicative)and one of three types of seasonality(none,additive,and multiplica-tive).Thus,there are15different methods,the best known of which are SES(no trend,no seasonality), Holt’s linear method(additive trend,no seasonality), Holt–Winters’additive method(additive trend,addi-tive seasonality),and Holt–Winters’multiplicative method(additive trend,multiplicative seasonality).2.2.VariationsNumerous variations on the original methods have been proposed.For example,Carreno and Madina-veitia(1990)and Williams and Miller(1999)pro-posed modifications to deal with discontinuities,and Rosas and Guerrero(1994)looked at exponential smoothing forecasts subject to one or more con-straints.There are also variations in how and when seasonal components should be wton (1998)argued for renormalization of the seasonal indices at each time period,as it removes bias in estimates of level and seasonal components.Slightly different normalization schemes were given by Roberts(1982)and McKenzie(1986).Archibald and Koehler(2003)developed new renormalization equations that are simpler to use and give the same point forecasts as the original methods.One useful variation,part way between SES and Holt’s method,is SES with drift.This is equivalent to Holt’s method with the trend parameter set to zero. Hyndman and Billah(2003)showed that this method was also equivalent to Assimakopoulos and Nikolo-poulos(2000)b Theta method Q when the drift param-eter is set to half the slope of a linear trend fitted to the data.The Theta method performed extremely well in the M3-competition,although why this particular choice of model and parameters is good has not yet been determined.There has been remarkably little work in developing multivariate versions of the exponential smoothing methods for forecasting.One notable exception is Pfeffermann and Allon(1989)who looked at Israeli tourism data.Multivariate SES is used for process control charts(e.g.,Pan,2005),where it is called b multivariate exponentially weighted moving averages Q, but here the focus is not on forecasting.2.3.State space modelsOrd,Koehler,and Snyder(1997)built on the work of Snyder(1985)by proposing a class of innovation state space models which can be considered as underlying some of the exponential smoothing meth-ods.Hyndman et al.(2002)and Taylor(2003) extended this to include all of the15exponential smoothing methods.In fact,Hyndman et al.(2002) proposed two state space models for each method, corresponding to the additive error and the multipli-cative error cases.These models are not unique and other related state space models for exponential smoothing methods are presented in Koehler,Snyder, and Ord(2001)and Chatfield,Koehler,Ord,and Snyder(2001).It has long been known that some ARIMA models give equivalent forecasts to the linear exponential smoothing methods.The significance of the recent work on innovation state space models is that the nonlinear exponential smoothing methods can also be derived from statistical models.2.4.Method selectionGardner and McKenzie(1988)provided some simple rules based on the variances of differenced time series for choosing an appropriate exponential smoothing method.Tashman and Kruk(1996)com-pared these rules with others proposed by Collopy and Armstrong(1992)and an approach based on the BIC. Hyndman et al.(2002)also proposed an information criterion approach,but using the underlying state space models.2.5.RobustnessThe remarkably good forecasting performance of exponential smoothing methods has been addressed by several authors.Satchell and Timmermann(1995) and Chatfield et al.(2001)showed that SES is optimal for a wide range of data generating processes.In a small simulation study,Hyndman(2001)showed thatJ.G.De Gooijer,R.J.Hyndman/International Journal of Forecasting22(2006)443–473445simple exponential smoothing performed better than first order ARIMA models because it is not so subject to model selection problems,particularly when data are non-normal.2.6.Prediction intervalsOne of the criticisms of exponential smoothing methods25years ago was that there was no way to produce prediction intervals for the forecasts.The first analytical approach to this problem was to assume that the series were generated by deterministic functions of time plus white noise(Brown,1963;Gardner,1985; McKenzie,1986;Sweet,1985).If this was so,a regression model should be used rather than expo-nential smoothing methods;thus,Newbold and Bos (1989)strongly criticized all approaches based on this assumption.Other authors sought to obtain prediction intervals via the equivalence between exponential smoothing methods and statistical models.Johnston and Harrison (1986)found forecast variances for the simple and Holt exponential smoothing methods for state space models with multiple sources of errors.Yar and Chatfield(1990)obtained prediction intervals for the additive Holt–Winters’method by deriving the underlying equivalent ARIMA model.Approximate prediction intervals for the multiplicative Holt–Win-ters’method were discussed by Chatfield and Yar (1991),making the assumption that the one-step-ahead forecast errors are independent.Koehler et al. (2001)also derived an approximate formula for the forecast variance for the multiplicative Holt–Winters’method,differing from Chatfield and Yar(1991)only in how the standard deviation of the one-step-ahead forecast error is estimated.Ord et al.(1997)and Hyndman et al.(2002)used the underlying innovation state space model to simulate future sample paths,and thereby obtained prediction intervals for all the exponential smoothing methods.Hyndman,Koehler,Ord,and Snyder (2005)used state space models to derive analytical prediction intervals for15of the30methods, including all the commonly used methods.They provide the most comprehensive algebraic approach to date for handling the prediction distribution problem for the majority of exponential smoothing methods.2.7.Parameter space and model propertiesIt is common practice to restrict the smoothing parameters to the range0to1.However,now that underlying statistical models are available,the natural (invertible)parameter space for the models can be used instead.Archibald(1990)showed that it is possible for smoothing parameters within the usual intervals to produce non-invertible models.Conse-quently,when forecasting,the impact of change in the past values of the series is non-negligible.Intuitively, such parameters produce poor forecasts and the forecast performance wton(1998)also discussed this problem.3.ARIMA models3.1.PreambleEarly attempts to study time series,particularly in the19th century,were generally characterized by the idea of a deterministic world.It was the major contribution of Yule(1927)which launched the notion of stochasticity in time series by postulating that every time series can be regarded as the realization of a stochastic process.Based on this simple idea,a number of time series methods have been developed since then.Workers such as Slutsky,Walker,Yaglom, and Yule first formulated the concept of autoregres-sive(AR)and moving average(MA)models.Wold’s decomposition theorem led to the formulation and solution of the linear forecasting problem of Kolmo-gorov(1941).Since then,a considerable body of literature has appeared in the area of time series, dealing with parameter estimation,identification, model checking,and forecasting;see,e.g.,Newbold (1983)for an early survey.The publication Time Series Analysis:Forecasting and Control by Box and Jenkins(1970)3integrated the existing knowledge.Moreover,these authors developed a coherent,versatile three-stage iterative3The book by Box,Jenkins,and Reinsel(1994)with Gregory Reinsel as a new co-author is an updated version of the b classic Q Box and Jenkins(1970)text.It includes new material on intervention analysis,outlier detection,testing for unit roots,and process control.J.G.De Gooijer,R.J.Hyndman/International Journal of Forecasting22(2006)443–473 446cycle for time series identification,estimation,and verification(rightly known as the Box–Jenkins approach).The book has had an enormous impact on the theory and practice of modern time series analysis and forecasting.With the advent of the computer,it popularized the use of autoregressive integrated moving average(ARIMA)models and their extensions in many areas of science.Indeed,forecast-ing discrete time series processes through univariate ARIMA models,transfer function(dynamic regres-sion)models,and multivariate(vector)ARIMA models has generated quite a few IJF papers.Often these studies were of an empirical nature,using one or more benchmark methods/models as a comparison. Without pretending to be complete,Table1gives a list of these studies.Naturally,some of these studies are more successful than others.In all cases,the forecasting experiences reported are valuable.They have also been the key to new developments,which may be summarized as follows.3.2.UnivariateThe success of the Box–Jenkins methodology is founded on the fact that the various models can, between them,mimic the behaviour of diverse types of series—and do so adequately without usually requiring very many parameters to be estimated in the final choice of the model.However,in the mid-sixties,the selection of a model was very much a matter of the researcher’s judgment;there was no algorithm to specify a model uniquely.Since then,Table1A list of examples of real applicationsDataset Forecast horizon Benchmark ReferenceUnivariate ARIMAElectricity load(min)1–30min Wiener filter Di Caprio,Genesio,Pozzi,and Vicino(1983)Quarterly automobile insurancepaid claim costs8quarters Log-linear regression Cummins and Griepentrog(1985)Daily federal funds rate1day Random walk Hein and Spudeck(1988)Quarterly macroeconomic data1–8quarters Wharton model Dhrymes and Peristiani(1988) Monthly department store sales1month Simple exponential smoothing Geurts and Kelly(1986,1990),Pack(1990)Monthly demand for telephone services3years Univariate state space Grambsch and Stahel(1990)Yearly population totals20–30years Demographic models Pflaumer(1992)Monthly tourism demand1–24months Univariate state space,multivariate state spacedu Preez and Witt(2003)Dynamic regression/transfer functionMonthly telecommunications traffic1month Univariate ARIMA Layton,Defris,and Zehnwirth(1986) Weekly sales data2years n.a.Leone(1987)Daily call volumes1week Holt–Winters Bianchi,Jarrett,and Hanumara(1998) Monthly employment levels1–12months Univariate ARIMA Weller(1989)Monthly and quarterly consumptionof natural gas1month/1quarter Univariate ARIMA Liu and Lin(1991)Monthly electricity consumption1–3years Univariate ARIMA Harris and Liu(1993)VARIMAYearly municipal budget data Yearly(in-sample)Univariate ARIMA Downs and Rocke(1983)Monthly accounting data1month Regression,univariate,ARIMA,transfer functionHillmer,Larcker,and Schroeder(1983)Quarterly macroeconomic data1–10quarters Judgmental methods,univariateARIMAO¨ller(1985)Monthly truck sales1–13months Univariate ARIMA,Holt–Winters Heuts and Bronckers(1988)Monthly hospital patient movements2years Univariate ARIMA,Holt–Winters Lin(1989)Quarterly unemployment rate1–8quarters Transfer function Edlund and Karlsson(1993)J.G.De Gooijer,R.J.Hyndman/International Journal of Forecasting22(2006)443–473447many techniques and methods have been suggested to add mathematical rigour to the search process of an ARMA model,including Akaike’s information crite-rion(AIC),Akaike’s final prediction error(FPE),and the Bayes information criterion(BIC).Often these criteria come down to minimizing(in-sample)one-step-ahead forecast errors,with a penalty term for overfitting.FPE has also been generalized for multi-step-ahead forecasting(see, e.g.,Bhansali,1996, 1999),but this generalization has not been utilized by applied workers.This also seems to be the case with criteria based on cross-validation and split-sample validation(see,e.g.,West,1996)principles, making use of genuine out-of-sample forecast errors; see Pen˜a and Sa´nchez(2005)for a related approach worth considering.There are a number of methods(cf.Box et al., 1994)for estimating the parameters of an ARMA model.Although these methods are equivalent asymptotically,in the sense that estimates tend to the same normal distribution,there are large differ-ences in finite sample properties.In a comparative study of software packages,Newbold,Agiakloglou, and Miller(1994)showed that this difference can be quite substantial and,as a consequence,may influ-ence forecasts.They recommended the use of full maximum likelihood.The effect of parameter esti-mation errors on the probability limits of the forecasts was also noticed by Zellner(1971).He used a Bayesian analysis and derived the predictive distri-bution of future observations by treating the param-eters in the ARMA model as random variables.More recently,Kim(2003)considered parameter estimation and forecasting of AR models in small samples.He found that(bootstrap)bias-corrected parameter esti-mators produce more accurate forecasts than the least squares ndsman and Damodaran(1989) presented evidence that the James-Stein ARIMA parameter estimator improves forecast accuracy relative to other methods,under an MSE loss criterion.If a time series is known to follow a univariate ARIMA model,forecasts using disaggregated obser-vations are,in terms of MSE,at least as good as forecasts using aggregated observations.However,in practical applications,there are other factors to be considered,such as missing values in disaggregated series.Both Ledolter(1989)and Hotta(1993)analyzed the effect of an additive outlier on theforecast intervals when the ARIMA model parametersare estimated.When the model is stationary,Hotta andCardoso Neto(1993)showed that the loss ofefficiency using aggregated data is not large,even ifthe model is not known.Thus,prediction could bedone by either disaggregated or aggregated models.The problem of incorporating external(prior)information in the univariate ARIMA forecasts hasbeen considered by Cholette(1982),Guerrero(1991),and de Alba(1993).As an alternative to the univariate ARIMAmethodology,Parzen(1982)proposed the ARARMAmethodology.The key idea is that a time series istransformed from a long-memory AR filter to a short-memory filter,thus avoiding the b harsher Q differenc-ing operator.In addition,a different approach to thed conventional T Box–Jenkins identification step is used.In the M-competition(Makridakis et al.,1982),the ARARMA models achieved the lowestMAPE for longer forecast horizons.Hence,it issurprising to find that,apart from the paper by Meadeand Smith(1985),the ARARMA methodology hasnot really taken off in applied work.Its ultimate valuemay perhaps be better judged by assessing the studyby Meade(2000)who compared the forecastingperformance of an automated and non-automatedARARMA method.Automatic univariate ARIMA modelling has beenshown to produce one-step-ahead forecasts as accu-rate as those produced by competent modellers(Hill&Fildes,1984;Libert,1984;Poulos,Kvanli,&Pavur,1987;Texter&Ord,1989).Several softwarevendors have implemented automated time seriesforecasting methods(including multivariate methods);see,e.g.,Geriner and Ord(1991),Tashman and Leach(1991),and Tashman(2000).Often these methods actas black boxes.The technology of expert systems(Me´lard&Pasteels,2000)can be used to avoid thisproblem.Some guidelines on the choice of anautomatic forecasting method are provided by Chat-field(1988).Rather than adopting a single AR model for allforecast horizons,Kang(2003)empirically investi-gated the case of using a multi-step-ahead forecastingAR model selected separately for each horizon.Theforecasting performance of the multi-step-ahead pro-cedure appears to depend on,among other things,J.G.De Gooijer,R.J.Hyndman/International Journal of Forecasting22(2006)443–473 448optimal order selection criteria,forecast periods, forecast horizons,and the time series to be forecast.3.3.Transfer functionThe identification of transfer function models can be difficult when there is more than one input variable.Edlund(1984)presented a two-step method for identification of the impulse response function when a number of different input variables are correlated.Koreisha(1983)established various rela-tionships between transfer functions,causal implica-tions,and econometric model specification.Gupta (1987)identified the major pitfalls in causality testing. Using principal component analysis,a parsimonious representation of a transfer function model was suggested by del Moral and Valderrama(1997). Krishnamurthi,Narayan,and Raj(1989)showed how more accurate estimates of the impact of interventions in transfer function models can be obtained by using a control variable.3.4.MultivariateThe vector ARIMA(VARIMA)model is a multivariate generalization of the univariate ARIMA model.The population characteristics of V ARMA processes appear to have been first derived by Quenouille(1957),although software to implement them only became available in the1980s and1990s. Since V ARIMA models can accommodate assump-tions on exogeneity and on contemporaneous relation-ships,they offered new challenges to forecasters and policymakers.Riise and Tjøstheim(1984)addressed the effect of parameter estimation on VARMA forecasts.Cholette and Lamy(1986)showed how smoothing filters can be built into V ARMA models. The smoothing prevents irregular fluctuations in explanatory time series from migrating to the forecasts of the dependent series.To determine the maximum forecast horizon of V ARMA processes,De Gooijer and Klein(1991)established the theoretical properties of cumulated multi-step-ahead forecasts and cumulat-ed multi-step-ahead forecast errors.Lu¨tkepohl(1986) studied the effects of temporal aggregation and systematic sampling on forecasting,assuming that the disaggregated(stationary)variable follows a V ARMA process with unknown ter,Bidar-kota(1998)considered the same problem but with the observed variables integrated rather than stationary.Vector autoregressions(VARs)constitute a special case of the more general class of V ARMA models.In essence,a VAR model is a fairly unrestricted (flexible)approximation to the reduced form of a wide variety of dynamic econometric models.V AR models can be specified in a number of ways.Funke (1990)presented five different V AR specifications and compared their forecasting performance using monthly industrial production series.Dhrymes and Thomakos(1998)discussed issues regarding the identification of structural V ARs.Hafer and Sheehan (1989)showed the effect on V AR forecasts of changes in the model structure.Explicit expressions for V AR forecasts in levels are provided by Arin˜o and Franses (2000);see also Wieringa and Horva´th(2005). Hansson,Jansson,and Lo¨f(2005)used a dynamic factor model as a starting point to obtain forecasts from parsimoniously parametrized V ARs.In general,VAR models tend to suffer from d overfitting T with too many free insignificant param-eters.As a result,these models can provide poor out-of-sample forecasts,even though within-sample fit-ting is good;see,e.g.,Liu,Gerlow,and Irwin(1994) and Simkins(1995).Instead of restricting some of the parameters in the usual way,Litterman(1986)and others imposed a prior distribution on the parameters, expressing the belief that many economic variables behave like a random walk.BV AR models have been chiefly used for macroeconomic forecasting(Artis& Zhang,1990;Ashley,1988;Holden&Broomhead, 1990;Kunst&Neusser,1986),for forecasting market shares(Ribeiro Ramos,2003),for labor market forecasting(LeSage&Magura,1991),for business forecasting(Spencer,1993),or for local economic forecasting(LeSage,1989).Kling and Bessler(1985) compared out-of-sample forecasts of several then-known multivariate time series methods,including Litterman’s BV AR model.The Engle and Granger(1987)concept of cointe-gration has raised various interesting questions re-garding the forecasting ability of error correction models(ECMs)over unrestricted V ARs and BV ARs. Shoesmith(1992),Shoesmith(1995),Tegene and Kuchler(1994),and Wang and Bessler(2004) provided empirical evidence to suggest that ECMs outperform V ARs in levels,particularly over longerJ.G.De Gooijer,R.J.Hyndman/International Journal of Forecasting22(2006)443–473449。